- Privacy Policy
Buy Me a Coffee

Home » Background of The Study – Examples and Writing Guide

Background of The Study – Examples and Writing Guide
Table of Contents

Background of The Study
Definition:
Background of the study refers to the context, circumstances, and history that led to the research problem or topic being studied. It provides the reader with a comprehensive understanding of the subject matter and the significance of the study.
The background of the study usually includes a discussion of the relevant literature, the gap in knowledge or understanding, and the research questions or hypotheses to be addressed. It also highlights the importance of the research topic and its potential contributions to the field. A well-written background of the study sets the stage for the research and helps the reader to appreciate the need for the study and its potential significance.
How to Write Background of The Study
Here are some steps to help you write the background of the study:
Identify the Research Problem
Start by identifying the research problem you are trying to address. This problem should be significant and relevant to your field of study.
Provide Context
Once you have identified the research problem, provide some context. This could include the historical, social, or political context of the problem.
Review Literature
Conduct a thorough review of the existing literature on the topic. This will help you understand what has been studied and what gaps exist in the current research.
Identify Research Gap
Based on your literature review, identify the gap in knowledge or understanding that your research aims to address. This gap will be the focus of your research question or hypothesis.
State Objectives
Clearly state the objectives of your research . These should be specific, measurable, achievable, relevant, and time-bound (SMART).
Discuss Significance
Explain the significance of your research. This could include its potential impact on theory , practice, policy, or society.
Finally, summarize the key points of the background of the study. This will help the reader understand the research problem, its context, and its significance.
How to Write Background of The Study in Proposal
The background of the study is an essential part of any proposal as it sets the stage for the research project and provides the context and justification for why the research is needed. Here are the steps to write a compelling background of the study in your proposal:
- Identify the problem: Clearly state the research problem or gap in the current knowledge that you intend to address through your research.
- Provide context: Provide a brief overview of the research area and highlight its significance in the field.
- Review literature: Summarize the relevant literature related to the research problem and provide a critical evaluation of the current state of knowledge.
- Identify gaps : Identify the gaps or limitations in the existing literature and explain how your research will contribute to filling these gaps.
- Justify the study : Explain why your research is important and what practical or theoretical contributions it can make to the field.
- Highlight objectives: Clearly state the objectives of the study and how they relate to the research problem.
- Discuss methodology: Provide an overview of the methodology you will use to collect and analyze data, and explain why it is appropriate for the research problem.
- Conclude : Summarize the key points of the background of the study and explain how they support your research proposal.
How to Write Background of The Study In Thesis
The background of the study is a critical component of a thesis as it provides context for the research problem, rationale for conducting the study, and the significance of the research. Here are some steps to help you write a strong background of the study:
- Identify the research problem : Start by identifying the research problem that your thesis is addressing. What is the issue that you are trying to solve or explore? Be specific and concise in your problem statement.
- Review the literature: Conduct a thorough review of the relevant literature on the topic. This should include scholarly articles, books, and other sources that are directly related to your research question.
- I dentify gaps in the literature: After reviewing the literature, identify any gaps in the existing research. What questions remain unanswered? What areas have not been explored? This will help you to establish the need for your research.
- Establish the significance of the research: Clearly state the significance of your research. Why is it important to address this research problem? What are the potential implications of your research? How will it contribute to the field?
- Provide an overview of the research design: Provide an overview of the research design and methodology that you will be using in your study. This should include a brief explanation of the research approach, data collection methods, and data analysis techniques.
- State the research objectives and research questions: Clearly state the research objectives and research questions that your study aims to answer. These should be specific, measurable, achievable, relevant, and time-bound.
- Summarize the chapter: Summarize the chapter by highlighting the key points and linking them back to the research problem, significance of the study, and research questions.
How to Write Background of The Study in Research Paper
Here are the steps to write the background of the study in a research paper:
- Identify the research problem: Start by identifying the research problem that your study aims to address. This can be a particular issue, a gap in the literature, or a need for further investigation.
- Conduct a literature review: Conduct a thorough literature review to gather information on the topic, identify existing studies, and understand the current state of research. This will help you identify the gap in the literature that your study aims to fill.
- Explain the significance of the study: Explain why your study is important and why it is necessary. This can include the potential impact on the field, the importance to society, or the need to address a particular issue.
- Provide context: Provide context for the research problem by discussing the broader social, economic, or political context that the study is situated in. This can help the reader understand the relevance of the study and its potential implications.
- State the research questions and objectives: State the research questions and objectives that your study aims to address. This will help the reader understand the scope of the study and its purpose.
- Summarize the methodology : Briefly summarize the methodology you used to conduct the study, including the data collection and analysis methods. This can help the reader understand how the study was conducted and its reliability.
Examples of Background of The Study
Here are some examples of the background of the study:
Problem : The prevalence of obesity among children in the United States has reached alarming levels, with nearly one in five children classified as obese.
Significance : Obesity in childhood is associated with numerous negative health outcomes, including increased risk of type 2 diabetes, cardiovascular disease, and certain cancers.
Gap in knowledge : Despite efforts to address the obesity epidemic, rates continue to rise. There is a need for effective interventions that target the unique needs of children and their families.
Problem : The use of antibiotics in agriculture has contributed to the development of antibiotic-resistant bacteria, which poses a significant threat to human health.
Significance : Antibiotic-resistant infections are responsible for thousands of deaths each year and are a major public health concern.
Gap in knowledge: While there is a growing body of research on the use of antibiotics in agriculture, there is still much to be learned about the mechanisms of resistance and the most effective strategies for reducing antibiotic use.
Edxample 3:
Problem : Many low-income communities lack access to healthy food options, leading to high rates of food insecurity and diet-related diseases.
Significance : Poor nutrition is a major contributor to chronic diseases such as obesity, type 2 diabetes, and cardiovascular disease.
Gap in knowledge : While there have been efforts to address food insecurity, there is a need for more research on the barriers to accessing healthy food in low-income communities and effective strategies for increasing access.
Examples of Background of The Study In Research
Here are some real-life examples of how the background of the study can be written in different fields of study:
Example 1 : “There has been a significant increase in the incidence of diabetes in recent years. This has led to an increased demand for effective diabetes management strategies. The purpose of this study is to evaluate the effectiveness of a new diabetes management program in improving patient outcomes.”
Example 2 : “The use of social media has become increasingly prevalent in modern society. Despite its popularity, little is known about the effects of social media use on mental health. This study aims to investigate the relationship between social media use and mental health in young adults.”
Example 3: “Despite significant advancements in cancer treatment, the survival rate for patients with pancreatic cancer remains low. The purpose of this study is to identify potential biomarkers that can be used to improve early detection and treatment of pancreatic cancer.”
Examples of Background of The Study in Proposal
Here are some real-time examples of the background of the study in a proposal:
Example 1 : The prevalence of mental health issues among university students has been increasing over the past decade. This study aims to investigate the causes and impacts of mental health issues on academic performance and wellbeing.
Example 2 : Climate change is a global issue that has significant implications for agriculture in developing countries. This study aims to examine the adaptive capacity of smallholder farmers to climate change and identify effective strategies to enhance their resilience.
Example 3 : The use of social media in political campaigns has become increasingly common in recent years. This study aims to analyze the effectiveness of social media campaigns in mobilizing young voters and influencing their voting behavior.
Example 4 : Employee turnover is a major challenge for organizations, especially in the service sector. This study aims to identify the key factors that influence employee turnover in the hospitality industry and explore effective strategies for reducing turnover rates.
Examples of Background of The Study in Thesis
Here are some real-time examples of the background of the study in the thesis:
Example 1 : “Women’s participation in the workforce has increased significantly over the past few decades. However, women continue to be underrepresented in leadership positions, particularly in male-dominated industries such as technology. This study aims to examine the factors that contribute to the underrepresentation of women in leadership roles in the technology industry, with a focus on organizational culture and gender bias.”
Example 2 : “Mental health is a critical component of overall health and well-being. Despite increased awareness of the importance of mental health, there are still significant gaps in access to mental health services, particularly in low-income and rural communities. This study aims to evaluate the effectiveness of a community-based mental health intervention in improving mental health outcomes in underserved populations.”
Example 3: “The use of technology in education has become increasingly widespread, with many schools adopting online learning platforms and digital resources. However, there is limited research on the impact of technology on student learning outcomes and engagement. This study aims to explore the relationship between technology use and academic achievement among middle school students, as well as the factors that mediate this relationship.”
Examples of Background of The Study in Research Paper
Here are some examples of how the background of the study can be written in various fields:
Example 1: The prevalence of obesity has been on the rise globally, with the World Health Organization reporting that approximately 650 million adults were obese in 2016. Obesity is a major risk factor for several chronic diseases such as diabetes, cardiovascular diseases, and cancer. In recent years, several interventions have been proposed to address this issue, including lifestyle changes, pharmacotherapy, and bariatric surgery. However, there is a lack of consensus on the most effective intervention for obesity management. This study aims to investigate the efficacy of different interventions for obesity management and identify the most effective one.
Example 2: Antibiotic resistance has become a major public health threat worldwide. Infections caused by antibiotic-resistant bacteria are associated with longer hospital stays, higher healthcare costs, and increased mortality. The inappropriate use of antibiotics is one of the main factors contributing to the development of antibiotic resistance. Despite numerous efforts to promote the rational use of antibiotics, studies have shown that many healthcare providers continue to prescribe antibiotics inappropriately. This study aims to explore the factors influencing healthcare providers’ prescribing behavior and identify strategies to improve antibiotic prescribing practices.
Example 3: Social media has become an integral part of modern communication, with millions of people worldwide using platforms such as Facebook, Twitter, and Instagram. Social media has several advantages, including facilitating communication, connecting people, and disseminating information. However, social media use has also been associated with several negative outcomes, including cyberbullying, addiction, and mental health problems. This study aims to investigate the impact of social media use on mental health and identify the factors that mediate this relationship.
Purpose of Background of The Study
The primary purpose of the background of the study is to help the reader understand the rationale for the research by presenting the historical, theoretical, and empirical background of the problem.
More specifically, the background of the study aims to:
- Provide a clear understanding of the research problem and its context.
- Identify the gap in knowledge that the study intends to fill.
- Establish the significance of the research problem and its potential contribution to the field.
- Highlight the key concepts, theories, and research findings related to the problem.
- Provide a rationale for the research questions or hypotheses and the research design.
- Identify the limitations and scope of the study.
When to Write Background of The Study
The background of the study should be written early on in the research process, ideally before the research design is finalized and data collection begins. This allows the researcher to clearly articulate the rationale for the study and establish a strong foundation for the research.
The background of the study typically comes after the introduction but before the literature review section. It should provide an overview of the research problem and its context, and also introduce the key concepts, theories, and research findings related to the problem.
Writing the background of the study early on in the research process also helps to identify potential gaps in knowledge and areas for further investigation, which can guide the development of the research questions or hypotheses and the research design. By establishing the significance of the research problem and its potential contribution to the field, the background of the study can also help to justify the research and secure funding or support from stakeholders.
Advantage of Background of The Study
The background of the study has several advantages, including:
- Provides context: The background of the study provides context for the research problem by highlighting the historical, theoretical, and empirical background of the problem. This allows the reader to understand the research problem in its broader context and appreciate its significance.
- Identifies gaps in knowledge: By reviewing the existing literature related to the research problem, the background of the study can identify gaps in knowledge that the study intends to fill. This helps to establish the novelty and originality of the research and its potential contribution to the field.
- Justifies the research : The background of the study helps to justify the research by demonstrating its significance and potential impact. This can be useful in securing funding or support for the research.
- Guides the research design: The background of the study can guide the development of the research questions or hypotheses and the research design by identifying key concepts, theories, and research findings related to the problem. This ensures that the research is grounded in existing knowledge and is designed to address the research problem effectively.
- Establishes credibility: By demonstrating the researcher’s knowledge of the field and the research problem, the background of the study can establish the researcher’s credibility and expertise, which can enhance the trustworthiness and validity of the research.
Disadvantages of Background of The Study
Some Disadvantages of Background of The Study are as follows:
- Time-consuming : Writing a comprehensive background of the study can be time-consuming, especially if the research problem is complex and multifaceted. This can delay the research process and impact the timeline for completing the study.
- Repetitive: The background of the study can sometimes be repetitive, as it often involves summarizing existing research and theories related to the research problem. This can be tedious for the reader and may make the section less engaging.
- Limitations of existing research: The background of the study can reveal the limitations of existing research related to the problem. This can create challenges for the researcher in developing research questions or hypotheses that address the gaps in knowledge identified in the background of the study.
- Bias : The researcher’s biases and perspectives can influence the content and tone of the background of the study. This can impact the reader’s perception of the research problem and may influence the validity of the research.
- Accessibility: Accessing and reviewing the literature related to the research problem can be challenging, especially if the researcher does not have access to a comprehensive database or if the literature is not available in the researcher’s language. This can limit the depth and scope of the background of the study.
About the author
Muhammad Hassan
Researcher, Academic Writer, Web developer
You may also like

Data Collection – Methods Types and Examples

Delimitations in Research – Types, Examples and...

Research Process – Steps, Examples and Tips

Research Design – Types, Methods and Examples

Institutional Review Board – Application Sample...

Evaluating Research – Process, Examples and...
- Resources Home 🏠
- Try SciSpace Copilot
- Search research papers
- Add Copilot Extension
- Try AI Detector
- Try Paraphraser
- Try Citation Generator
- April Papers
- June Papers
- July Papers

How to Write an Effective Background of the Study: A Comprehensive Guide

Table of Contents
The background of the study in a research paper offers a clear context, highlighting why the research is essential and the problem it aims to address.
As a researcher, this foundational section is essential for you to chart the course of your study, Moreover, it allows readers to understand the importance and path of your research.
Whether in academic communities or to the general public, a well-articulated background aids in communicating the essence of the research effectively.
While it may seem straightforward, crafting an effective background requires a blend of clarity, precision, and relevance. Therefore, this article aims to be your guide, offering insights into:
- Understanding the concept of the background of the study.
- Learning how to craft a compelling background effectively.
- Identifying and sidestepping common pitfalls in writing the background.
- Exploring practical examples that bring the theory to life.
- Enhancing both your writing and reading of academic papers.
Keeping these compelling insights in mind, let's delve deeper into the details of the empirical background of the study, exploring its definition, distinctions, and the art of writing it effectively.
What is the background of the study?
The background of the study is placed at the beginning of a research paper. It provides the context, circumstances, and history that led to the research problem or topic being explored.
It offers readers a snapshot of the existing knowledge on the topic and the reasons that spurred your current research.
When crafting the background of your study, consider the following questions.
- What's the context of your research?
- Which previous research will you refer to?
- Are there any knowledge gaps in the existing relevant literature?
- How will you justify the need for your current research?
- Have you concisely presented the research question or problem?
In a typical research paper structure, after presenting the background, the introduction section follows. The introduction delves deeper into the specific objectives of the research and often outlines the structure or main points that the paper will cover.
Together, they create a cohesive starting point, ensuring readers are well-equipped to understand the subsequent sections of the research paper.
While the background of the study and the introduction section of the research manuscript may seem similar and sometimes even overlap, each serves a unique purpose in the research narrative.
Difference between background and introduction
A well-written background of the study and introduction are preliminary sections of a research paper and serve distinct purposes.
Here’s a detailed tabular comparison between the two of them.
What is the relevance of the background of the study?
It is necessary for you to provide your readers with the background of your research. Without this, readers may grapple with questions such as: Why was this specific research topic chosen? What led to this decision? Why is this study relevant? Is it worth their time?
Such uncertainties can deter them from fully engaging with your study, leading to the rejection of your research paper. Additionally, this can diminish its impact in the academic community, and reduce its potential for real-world application or policy influence .
To address these concerns and offer clarity, the background section plays a pivotal role in research papers.
The background of the study in research is important as it:
- Provides context: It offers readers a clear picture of the existing knowledge, helping them understand where the current research fits in.
- Highlights relevance: By detailing the reasons for the research, it underscores the study's significance and its potential impact.
- Guides the narrative: The background shapes the narrative flow of the paper, ensuring a logical progression from what's known to what the research aims to uncover.
- Enhances engagement: A well-crafted background piques the reader's interest, encouraging them to delve deeper into the research paper.
- Aids in comprehension: By setting the scenario, it aids readers in better grasping the research objectives, methodologies, and findings.
How to write the background of the study in a research paper?
The journey of presenting a compelling argument begins with the background study. This section holds the power to either captivate or lose the reader's interest.
An effectively written background not only provides context but also sets the tone for the entire research paper. It's the bridge that connects a broad topic to a specific research question, guiding readers through the logic behind the study.
But how does one craft a background of the study that resonates, informs, and engages?
Here, we’ll discuss how to write an impactful background study, ensuring your research stands out and captures the attention it deserves.
Identify the research problem
The first step is to start pinpointing the specific issue or gap you're addressing. This should be a significant and relevant problem in your field.
A well-defined problem is specific, relevant, and significant to your field. It should resonate with both experts and readers.
Here’s more on how to write an effective research problem .
Provide context
Here, you need to provide a broader perspective, illustrating how your research aligns with or contributes to the overarching context or the wider field of study. A comprehensive context is grounded in facts, offers multiple perspectives, and is relatable.
In addition to stating facts, you should weave a story that connects key concepts from the past, present, and potential future research. For instance, consider the following approach.
- Offer a brief history of the topic, highlighting major milestones or turning points that have shaped the current landscape.
- Discuss contemporary developments or current trends that provide relevant information to your research problem. This could include technological advancements, policy changes, or shifts in societal attitudes.
- Highlight the views of different stakeholders. For a topic like sustainable agriculture, this could mean discussing the perspectives of farmers, environmentalists, policymakers, and consumers.
- If relevant, compare and contrast global trends with local conditions and circumstances. This can offer readers a more holistic understanding of the topic.
Literature review
For this step, you’ll deep dive into the existing literature on the same topic. It's where you explore what scholars, researchers, and experts have already discovered or discussed about your topic.
Conducting a thorough literature review isn't just a recap of past works. To elevate its efficacy, it's essential to analyze the methods, outcomes, and intricacies of prior research work, demonstrating a thorough engagement with the existing body of knowledge.
- Instead of merely listing past research study, delve into their methodologies, findings, and limitations. Highlight groundbreaking studies and those that had contrasting results.
- Try to identify patterns. Look for recurring themes or trends in the literature. Are there common conclusions or contentious points?
- The next step would be to connect the dots. Show how different pieces of research relate to each other. This can help in understanding the evolution of thought on the topic.
By showcasing what's already known, you can better highlight the background of the study in research.
Highlight the research gap
This step involves identifying the unexplored areas or unanswered questions in the existing literature. Your research seeks to address these gaps, providing new insights or answers.
A clear research gap shows you've thoroughly engaged with existing literature and found an area that needs further exploration.
How can you efficiently highlight the research gap?
- Find the overlooked areas. Point out topics or angles that haven't been adequately addressed.
- Highlight questions that have emerged due to recent developments or changing circumstances.
- Identify areas where insights from other fields might be beneficial but haven't been explored yet.
State your objectives
Here, it’s all about laying out your game plan — What do you hope to achieve with your research? You need to mention a clear objective that’s specific, actionable, and directly tied to the research gap.
How to state your objectives?
- List the primary questions guiding your research.
- If applicable, state any hypotheses or predictions you aim to test.
- Specify what you hope to achieve, whether it's new insights, solutions, or methodologies.
Discuss the significance
This step describes your 'why'. Why is your research important? What broader implications does it have?
The significance of “why” should be both theoretical (adding to the existing literature) and practical (having real-world implications).
How do we effectively discuss the significance?
- Discuss how your research adds to the existing body of knowledge.
- Highlight how your findings could be applied in real-world scenarios, from policy changes to on-ground practices.
- Point out how your research could pave the way for further studies or open up new areas of exploration.
Summarize your points
A concise summary acts as a bridge, smoothly transitioning readers from the background to the main body of the paper. This step is a brief recap, ensuring that readers have grasped the foundational concepts.
How to summarize your study?
- Revisit the key points discussed, from the research problem to its significance.
- Prepare the reader for the subsequent sections, ensuring they understand the research's direction.
Include examples for better understanding
Research and come up with real-world or hypothetical examples to clarify complex concepts or to illustrate the practical applications of your research. Relevant examples make abstract ideas tangible, aiding comprehension.
How to include an effective example of the background of the study?
- Use past events or scenarios to explain concepts.
- Craft potential scenarios to demonstrate the implications of your findings.
- Use comparisons to simplify complex ideas, making them more relatable.
Crafting a compelling background of the study in research is about striking the right balance between providing essential context, showcasing your comprehensive understanding of the existing literature, and highlighting the unique value of your research .
While writing the background of the study, keep your readers at the forefront of your mind. Every piece of information, every example, and every objective should be geared toward helping them understand and appreciate your research.
How to avoid mistakes in the background of the study in research?
To write a well-crafted background of the study, you should be aware of the following potential research pitfalls .
- Stay away from ambiguity. Always assume that your reader might not be familiar with intricate details about your topic.
- Avoid discussing unrelated themes. Stick to what's directly relevant to your research problem.
- Ensure your background is well-organized. Information should flow logically, making it easy for readers to follow.
- While it's vital to provide context, avoid overwhelming the reader with excessive details that might not be directly relevant to your research problem.
- Ensure you've covered the most significant and relevant studies i` n your field. Overlooking key pieces of literature can make your background seem incomplete.
- Aim for a balanced presentation of facts, and avoid showing overt bias or presenting only one side of an argument.
- While academic paper often involves specialized terms, ensure they're adequately explained or use simpler alternatives when possible.
- Every claim or piece of information taken from existing literature should be appropriately cited. Failing to do so can lead to issues of plagiarism.
- Avoid making the background too lengthy. While thoroughness is appreciated, it should not come at the expense of losing the reader's interest. Maybe prefer to keep it to one-two paragraphs long.
- Especially in rapidly evolving fields, it's crucial to ensure that your literature review section is up-to-date and includes the latest research.
Example of an effective background of the study
Let's consider a topic: "The Impact of Online Learning on Student Performance." The ideal background of the study section for this topic would be as follows.
In the last decade, the rise of the internet has revolutionized many sectors, including education. Online learning platforms, once a supplementary educational tool, have now become a primary mode of instruction for many institutions worldwide. With the recent global events, such as the COVID-19 pandemic, there has been a rapid shift from traditional classroom learning to online modes, making it imperative to understand its effects on student performance.
Previous studies have explored various facets of online learning, from its accessibility to its flexibility. However, there is a growing need to assess its direct impact on student outcomes. While some educators advocate for its benefits, citing the convenience and vast resources available, others express concerns about potential drawbacks, such as reduced student engagement and the challenges of self-discipline.
This research aims to delve deeper into this debate, evaluating the true impact of online learning on student performance.
Why is this example considered as an effective background section of a research paper?
This background section example effectively sets the context by highlighting the rise of online learning and its increased relevance due to recent global events. It references prior research on the topic, indicating a foundation built on existing knowledge.
By presenting both the potential advantages and concerns of online learning, it establishes a balanced view, leading to the clear purpose of the study: to evaluate the true impact of online learning on student performance.
As we've explored, writing an effective background of the study in research requires clarity, precision, and a keen understanding of both the broader landscape and the specific details of your topic.
From identifying the research problem, providing context, reviewing existing literature to highlighting research gaps and stating objectives, each step is pivotal in shaping the narrative of your research. And while there are best practices to follow, it's equally crucial to be aware of the pitfalls to avoid.
Remember, writing or refining the background of your study is essential to engage your readers, familiarize them with the research context, and set the ground for the insights your research project will unveil.
Drawing from all the important details, insights and guidance shared, you're now in a strong position to craft a background of the study that not only informs but also engages and resonates with your readers.
Now that you've a clear understanding of what the background of the study aims to achieve, the natural progression is to delve into the next crucial component — write an effective introduction section of a research paper. Read here .
Frequently Asked Questions
The background of the study should include a clear context for the research, references to relevant previous studies, identification of knowledge gaps, justification for the current research, a concise overview of the research problem or question, and an indication of the study's significance or potential impact.
The background of the study is written to provide readers with a clear understanding of the context, significance, and rationale behind the research. It offers a snapshot of existing knowledge on the topic, highlights the relevance of the study, and sets the stage for the research questions and objectives. It ensures that readers can grasp the importance of the research and its place within the broader field of study.
The background of the study is a section in a research paper that provides context, circumstances, and history leading to the research problem or topic being explored. It presents existing knowledge on the topic and outlines the reasons that spurred the current research, helping readers understand the research's foundation and its significance in the broader academic landscape.
The number of paragraphs in the background of the study can vary based on the complexity of the topic and the depth of the context required. Typically, it might range from 3 to 5 paragraphs, but in more detailed or complex research papers, it could be longer. The key is to ensure that all relevant information is presented clearly and concisely, without unnecessary repetition.

You might also like

AI for Meta Analysis — A Comprehensive Guide
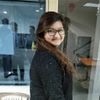
How To Write An Argumentative Essay

Beyond Google Scholar: Why SciSpace is the best alternative
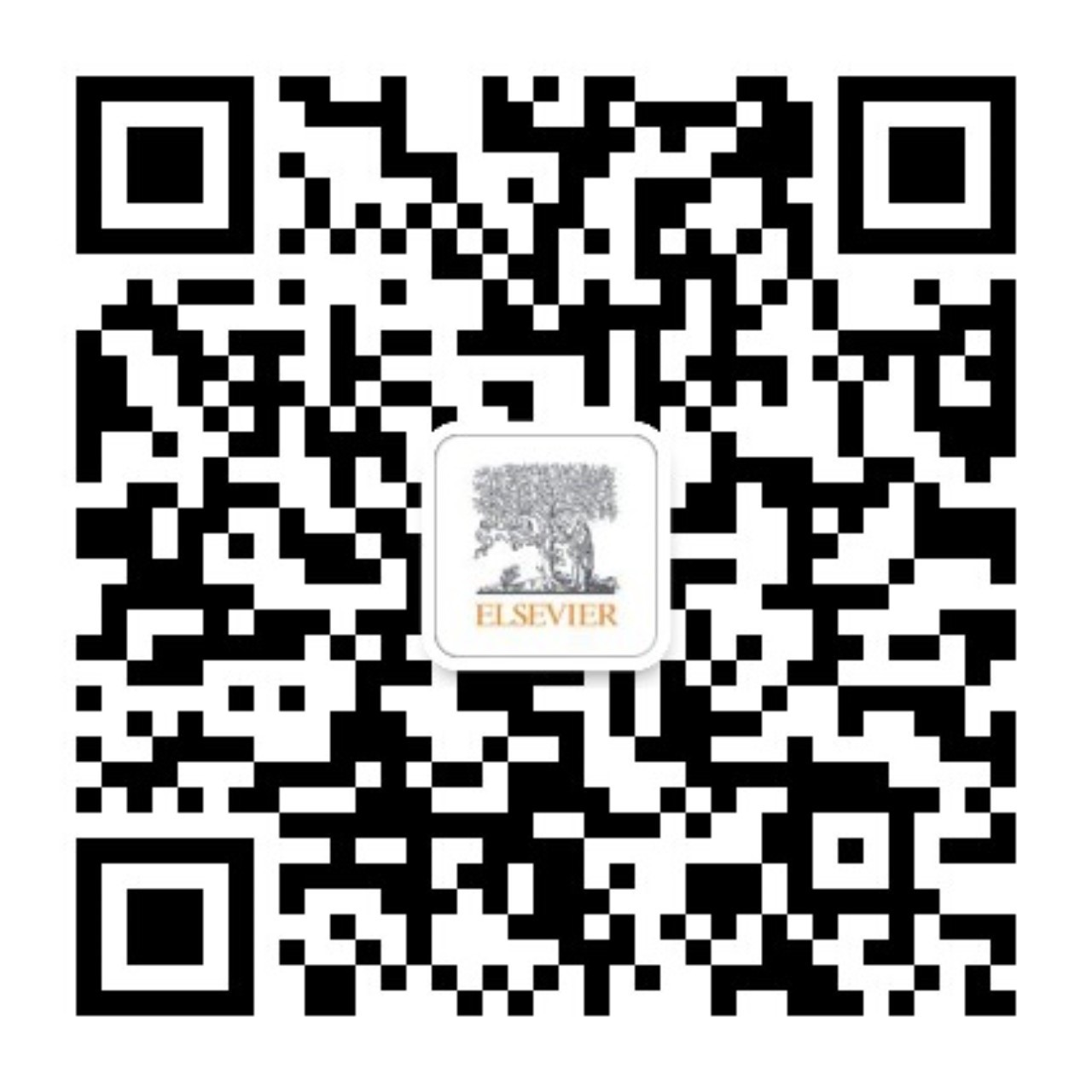
- Manuscript Preparation
What is the Background of a Study and How Should it be Written?
- 3 minute read
- 857.4K views
Table of Contents
The background of a study is one of the most important components of a research paper. The quality of the background determines whether the reader will be interested in the rest of the study. Thus, to ensure that the audience is invested in reading the entire research paper, it is important to write an appealing and effective background. So, what constitutes the background of a study, and how must it be written?
What is the background of a study?
The background of a study is the first section of the paper and establishes the context underlying the research. It contains the rationale, the key problem statement, and a brief overview of research questions that are addressed in the rest of the paper. The background forms the crux of the study because it introduces an unaware audience to the research and its importance in a clear and logical manner. At times, the background may even explore whether the study builds on or refutes findings from previous studies. Any relevant information that the readers need to know before delving into the paper should be made available to them in the background.
How is a background different from the introduction?
The introduction of your research paper is presented before the background. Let’s find out what factors differentiate the background from the introduction.
- The introduction only contains preliminary data about the research topic and does not state the purpose of the study. On the contrary, the background clarifies the importance of the study in detail.
- The introduction provides an overview of the research topic from a broader perspective, while the background provides a detailed understanding of the topic.
- The introduction should end with the mention of the research questions, aims, and objectives of the study. In contrast, the background follows no such format and only provides essential context to the study.
How should one write the background of a research paper?
The length and detail presented in the background varies for different research papers, depending on the complexity and novelty of the research topic. At times, a simple background suffices, even if the study is complex. Before writing and adding details in the background, take a note of these additional points:
- Start with a strong beginning: Begin the background by defining the research topic and then identify the target audience.
- Cover key components: Explain all theories, concepts, terms, and ideas that may feel unfamiliar to the target audience thoroughly.
- Take note of important prerequisites: Go through the relevant literature in detail. Take notes while reading and cite the sources.
- Maintain a balance: Make sure that the background is focused on important details, but also appeals to a broader audience.
- Include historical data: Current issues largely originate from historical events or findings. If the research borrows information from a historical context, add relevant data in the background.
- Explain novelty: If the research study or methodology is unique or novel, provide an explanation that helps to understand the research better.
- Increase engagement: To make the background engaging, build a story around the central theme of the research
Avoid these mistakes while writing the background:
- Ambiguity: Don’t be ambiguous. While writing, assume that the reader does not understand any intricate detail about your research.
- Unrelated themes: Steer clear from topics that are not related to the key aspects of your research topic.
- Poor organization: Do not place information without a structure. Make sure that the background reads in a chronological manner and organize the sub-sections so that it flows well.
Writing the background for a research paper should not be a daunting task. But directions to go about it can always help. At Elsevier Author Services we provide essential insights on how to write a high quality, appealing, and logically structured paper for publication, beginning with a robust background. For further queries, contact our experts now!
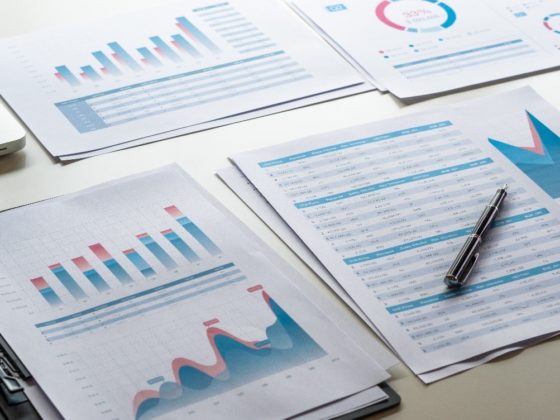
How to Use Tables and Figures effectively in Research Papers
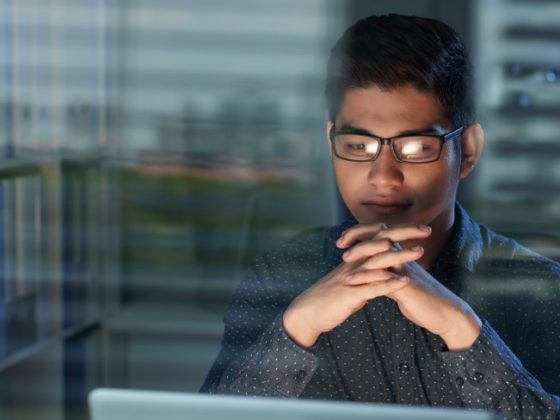
- Research Process
The Top 5 Qualities of Every Good Researcher
You may also like.
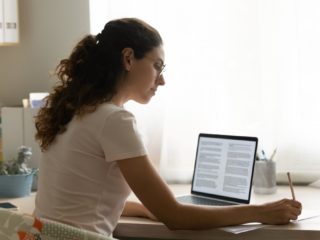
Make Hook, Line, and Sinker: The Art of Crafting Engaging Introductions
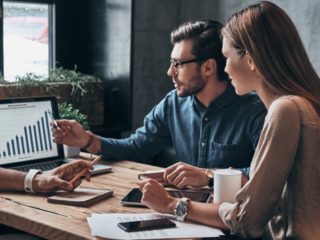
Can Describing Study Limitations Improve the Quality of Your Paper?
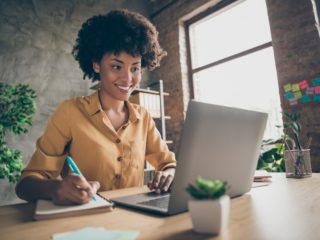
A Guide to Crafting Shorter, Impactful Sentences in Academic Writing
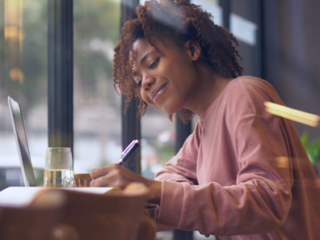
6 Steps to Write an Excellent Discussion in Your Manuscript
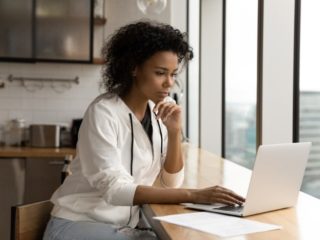
How to Write Clear and Crisp Civil Engineering Papers? Here are 5 Key Tips to Consider
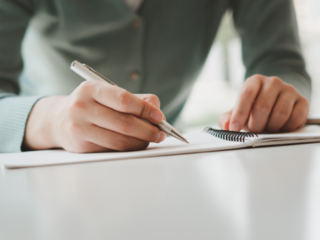
The Clear Path to An Impactful Paper: ②
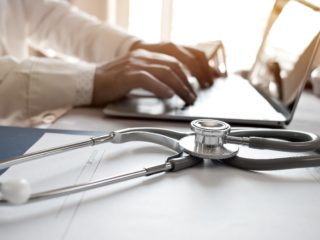
The Essentials of Writing to Communicate Research in Medicine
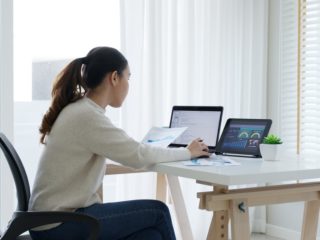
Changing Lines: Sentence Patterns in Academic Writing
Input your search keywords and press Enter.
- Affiliate Program

- UNITED STATES
- 台灣 (TAIWAN)
- TÜRKIYE (TURKEY)
- Academic Editing Services
- - Research Paper
- - Journal Manuscript
- - Dissertation
- - College & University Assignments
- Admissions Editing Services
- - Application Essay
- - Personal Statement
- - Recommendation Letter
- - Cover Letter
- - CV/Resume
- Business Editing Services
- - Business Documents
- - Report & Brochure
- - Website & Blog
- Writer Editing Services
- - Script & Screenplay
- Our Editors
- Client Reviews
- Editing & Proofreading Prices
- Wordvice Points
- Partner Discount
- Plagiarism Checker
- APA Citation Generator
- MLA Citation Generator
- Chicago Citation Generator
- Vancouver Citation Generator
- - APA Style
- - MLA Style
- - Chicago Style
- - Vancouver Style
- Writing & Editing Guide
- Academic Resources
- Admissions Resources
What is the Background of the Study and How to Write It
What is the Background of the Study in Research?
The background of the study is the first section of a research paper and gives context surrounding the research topic. The background explains to the reader where your research journey started, why you got interested in the topic, and how you developed the research question that you will later specify. That means that you first establish the context of the research you did with a general overview of the field or topic and then present the key issues that drove your decision to study the specific problem you chose.
Once the reader understands where you are coming from and why there was indeed a need for the research you are going to present in the following—because there was a gap in the current research, or because there is an obvious problem with a currently used process or technology—you can proceed with the formulation of your research question and summarize how you are going to address it in the rest of your manuscript.
Why is the Background of the Study Important?
No matter how surprising and important the findings of your study are, if you do not provide the reader with the necessary background information and context, they will not be able to understand your reasons for studying the specific problem you chose and why you think your study is relevant. And more importantly, an editor who does not share your enthusiasm for your work (because you did not fill them in on all the important details) will very probably not even consider your manuscript worthy of their and the reviewers’ time and will immediately send it back to you.
To avoid such desk rejections , you need to make sure you pique the reader’s interest and help them understand the contribution of your work to the specific field you study, the more general research community, or the public. Introducing the study background is crucial to setting the scene for your readers.
Table of Contents:
- What is “Background Information” in a Research Paper?
- What Should the Background of a Research Paper Include?
- Where Does the Background Section Go in Your Paper?
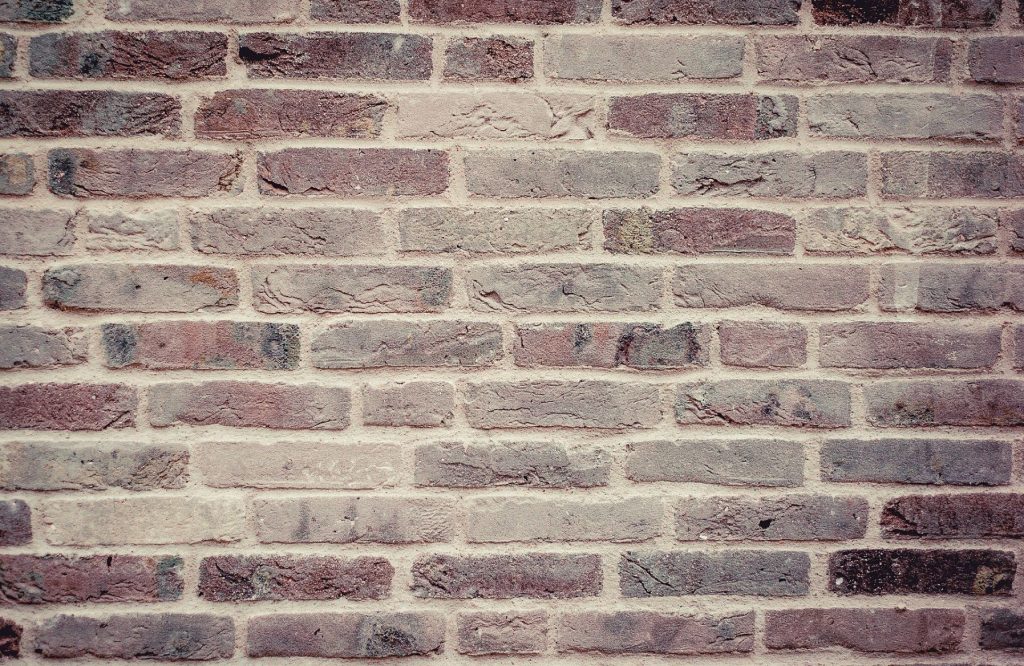
Background of the Study Structure
Before writing your study background, it is essential to understand what to include. The following elements should all be included in the background and are presented in greater detail in the next section:
- A general overview of the topic and why it is important (overlaps with establishing the “importance of the topic” in the Introduction)
- The current state of the research on the topic or on related topics in the field
- Controversies about current knowledge or specific past studies that undergird your research methodology
- Any claims or assumptions that have been made by researchers, institutions, or politicians that might need to be clarified
- Methods and techniques used in the study or from which your study deviated in some way
Presenting the Study Background
As you begin introducing your background, you first need to provide a general overview and include the main issues concerning the topic. Depending on whether you do “basic” (with the aim of providing further knowledge) or “applied” research (to establish new techniques, processes, or products), this is either a literature review that summarizes all relevant earlier studies in the field or a description of the process (e.g., vote counting) or practice (e.g., diagnosis of a specific disease) that you think is problematic or lacking and needs a solution.
Example s of a general overview
If you study the function of a Drosophila gene, for example, you can explain to the reader why and for whom the study of fly genetics is relevant, what is already known and established, and where you see gaps in the existing literature. If you investigated how the way universities have transitioned into online teaching since the beginning of the Covid-19 pandemic has affected students’ learning progress, then you need to present a summary of what changes have happened around the world, what the effects of those changes have been so far, and where you see problems that need to be addressed. Note that you need to provide sources for every statement and every claim you make here, to establish a solid foundation of knowledge for your own study.
Describing the current state of knowledge
When the reader understands the main issue(s), you need to fill them in more specifically on the current state of the field (in basic research) or the process/practice/product use you describe (in practical/applied research). Cite all relevant studies that have already reported on the Drosophila gene you are interested in, have failed to reveal certain functions of it, or have suggested that it might be involved in more processes than we know so far. Or list the reports from the education ministries of the countries you are interested in and highlight the data that shows the need for research into the effects of the Corona-19 pandemic on teaching and learning.
Discussing controversies, claims, and assumptions
Are there controversies regarding your topic of interest that need to be mentioned and/or addressed? For example, if your research topic involves an issue that is politically hot, you can acknowledge this here. Have any earlier claims or assumptions been made, by other researchers, institutions, or politicians, that you think need to be clarified?
Mentioning methodologies and approaches
While putting together these details, you also need to mention methodologies : What methods/techniques have been used so far to study what you studied and why are you going to either use the same or a different approach? Are any of the methods included in the literature review flawed in such a way that your study takes specific measures to correct or update? While you shouldn’t spend too much time here justifying your methods (this can be summarized briefly in the rationale of the study at the end of the Introduction and later in the Discussion section), you can engage with the crucial methods applied in previous studies here first.
When you have established the background of the study of your research paper in such a logical way, then the reader should have had no problem following you from the more general information you introduced first to the specific details you added later. You can now easily lead over to the relevance of your research, explain how your work fits into the bigger picture, and specify the aims and objectives of your study. This latter part is usually considered the “ statement of the problem ” of your study. Without a solid research paper background, this statement will come out of nowhere for the reader and very probably raise more questions than you were planning to answer.
Where does the study background section go in a paper?
Unless you write a research proposal or some kind of report that has a specific “Background” chapter, the background of your study is the first part of your introduction section . This is where you put your work in context and provide all the relevant information the reader needs to follow your rationale. Make sure your background has a logical structure and naturally leads into the statement of the problem at the very end of the introduction so that you bring everything together for the reader to judge the relevance of your work and the validity of your approach before they dig deeper into the details of your study in the methods section .
Consider Receiving Professional Editing Services
Now that you know how to write a background section for a research paper, you might be interested in our AI text editor at Wordvice AI. And be sure to receive professional editing services , including academic editing and proofreading , before submitting your manuscript to journals. On the Wordvice academic resources website, you can also find many more articles and other resources that can help you with writing the other parts of your research paper , with making a research paper outline before you put everything together, or with writing an effective cover letter once you are ready to submit.
Writing Quantitative Research Studies
- Reference work entry
- First Online: 13 January 2019
- Cite this reference work entry
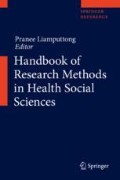
- Ankur Singh 2 ,
- Adyya Gupta 3 &
- Karen G. Peres 4
1249 Accesses
1 Citations
Summarizing quantitative data and its effective presentation and discussion can be challenging for students and researchers. This chapter provides a framework for adequately reporting findings from quantitative analysis in a research study for those contemplating to write a research paper. The rationale underpinning the reporting methods to maintain the credibility and integrity of quantitative studies is outlined. Commonly used terminologies in empirical studies are defined and discussed with suitable examples. Key elements that build consistency between different sections (background, methods, results, and the discussion) of a research study using quantitative methods in a journal article are explicated. Specifically, recommended standard guidelines for randomized controlled trials and observational studies for reporting and discussion of findings from quantitative studies are elaborated. Key aspects of methodology that include describing the study population, sampling strategy, data collection methods, measurements/variables, and statistical analysis which informs the quality of a study from the reviewer’s perspective are described. Effective use of references in the methods section to strengthen the rationale behind specific statistical techniques and choice of measures has been highlighted with examples. Identifying ways in which data can be most succinctly and effectively summarized in tables and graphs according to their suitability and purpose of information is also detailed in this chapter. Strategies to present and discuss the quantitative findings in a structured discussion section are also provided. Overall, the chapter provides the readers with a comprehensive set of tools to identify key strategies to be considered when reporting quantitative research.
This is a preview of subscription content, log in via an institution to check access.
Access this chapter
- Available as PDF
- Read on any device
- Instant download
- Own it forever
- Available as EPUB and PDF
- Durable hardcover edition
- Dispatched in 3 to 5 business days
- Free shipping worldwide - see info
Tax calculation will be finalised at checkout
Purchases are for personal use only
Institutional subscriptions
Bhaumik S, Arora M, Singh A, Sargent JD. Impact of entertainment media smoking on adolescent smoking behaviours. Cochrane Database Syst Rev. 2015;6:1–12. https://doi.org/10.1002/14651858.CD011720 .
Article Google Scholar
Dickersin K, Manheimer E, Wieland S, Robinson KA, Lefebvre C, McDonald S. Development of the Cochrane Collaboration’s CENTRAL register of controlled clinical trials. Eval Health Prof. 2002;25(1):38–64.
Google Scholar
Docherty M, Smith R. The case for structuring the discussion of scientific papers: much the same as that for structuring abstracts. Br Med J. 1999;318(7193):1224–5.
Greenland S, Pearl J, Robins JM. Causal diagrams for epidemiologic research. Epidemiology. 1999;10(1):37–48.
Horton R. The rhetoric of research. Br Med J. 1995;310(6985):985–7.
Kool B, Ziersch A, Robinson P, Wolfenden L, Lowe JB. The ‘Seven deadly sins’ of rejected papers. Aust N Z J Public Health. 2016;40(1):3–4.
Mannocci A, Saulle R, Colamesta V, D’Aguanno S, Giraldi G, Maffongelli E, et al. What is the impact of reporting guidelines on public health journals in Europe? The case of STROBE, CONSORT and PRISMA. J Public Health. 2015;37(4):737–40.
Rothwell PM. External validity of randomised controlled trials: “to whom do the results of this trial apply?”. Lancet. 2005;365(9453):82–93.
Schulz KF, Altman DG, Moher D. CONSORT 2010 statement: updated guidelines for reporting parallel group randomised trials. PLoS Med. 2010;7(3):e1000251.
Szklo M. Quality of scientific articles. Rev Saude Publica. 2006;40 Spec no:30–5.
Vandenbroucke JP, von Elm E, Altman DG, Gotzsche PC, Mulrow CD, Pocock SJ, et al. Strengthening the reporting of observational studies in epidemiology (STROBE): explanation and elaboration. PLoS Med. 2007;4(10):e297.
Weiss NS, Koepsell TD, Psaty BM. Generalizability of the results of randomized trials. Arch Intern Med. 2008;168(2):133–5.
Singh A, Gupta A, Peres MA, Watt RG, Tsakos G, Mathur MR. Association between tooth loss and hypertension among a primarily rural middle aged and older Indian adult population. J Public Health Dent. 2016;76:198–205.
Download references
Author information
Authors and affiliations.
Centre for Health Equity, Melbourne School of Population and Global Health, The University of Melbourne, Melbourne, VIC, Australia
Ankur Singh
School of Public Health, The University of Adelaide, Adelaide, SA, Australia
Adyya Gupta
Australian Research Centre for Population Oral Health (ARCPOH), Adelaide Dental School, The University of Adelaide, Adelaide, SA, Australia
Karen G. Peres
You can also search for this author in PubMed Google Scholar
Corresponding author
Correspondence to Ankur Singh .
Editor information
Editors and affiliations.
School of Science and Health, Western Sydney University, Penrith, NSW, Australia
Pranee Liamputtong
Rights and permissions
Reprints and permissions
Copyright information
© 2019 Springer Nature Singapore Pte Ltd.
About this entry
Cite this entry.
Singh, A., Gupta, A., Peres, K.G. (2019). Writing Quantitative Research Studies. In: Liamputtong, P. (eds) Handbook of Research Methods in Health Social Sciences. Springer, Singapore. https://doi.org/10.1007/978-981-10-5251-4_117
Download citation
DOI : https://doi.org/10.1007/978-981-10-5251-4_117
Published : 13 January 2019
Publisher Name : Springer, Singapore
Print ISBN : 978-981-10-5250-7
Online ISBN : 978-981-10-5251-4
eBook Packages : Social Sciences Reference Module Humanities and Social Sciences Reference Module Business, Economics and Social Sciences
Share this entry
Anyone you share the following link with will be able to read this content:
Sorry, a shareable link is not currently available for this article.
Provided by the Springer Nature SharedIt content-sharing initiative
- Publish with us
Policies and ethics
- Find a journal
- Track your research

What Is Background in a Research Paper?
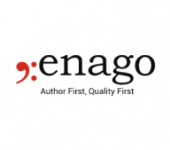
So you have carefully written your research paper and probably ran it through your colleagues ten to fifteen times. While there are many elements to a good research article, one of the most important elements for your readers is the background of your study.
What is Background of the Study in Research
The background of your study will provide context to the information discussed throughout the research paper . Background information may include both important and relevant studies. This is particularly important if a study either supports or refutes your thesis.
Why is Background of the Study Necessary in Research?
The background of the study discusses your problem statement, rationale, and research questions. It links introduction to your research topic and ensures a logical flow of ideas. Thus, it helps readers understand your reasons for conducting the study.
Providing Background Information
The reader should be able to understand your topic and its importance. The length and detail of your background also depend on the degree to which you need to demonstrate your understanding of the topic. Paying close attention to the following questions will help you in writing background information:
- Are there any theories, concepts, terms, and ideas that may be unfamiliar to the target audience and will require you to provide any additional explanation?
- Any historical data that need to be shared in order to provide context on why the current issue emerged?
- Are there any concepts that may have been borrowed from other disciplines that may be unfamiliar to the reader and need an explanation?
Related: Ready with the background and searching for more information on journal ranking? Check this infographic on the SCImago Journal Rank today!
Is the research study unique for which additional explanation is needed? For instance, you may have used a completely new method
How to Write a Background of the Study
The structure of a background study in a research paper generally follows a logical sequence to provide context, justification, and an understanding of the research problem. It includes an introduction, general background, literature review , rationale , objectives, scope and limitations , significance of the study and the research hypothesis . Following the structure can provide a comprehensive and well-organized background for your research.
Here are the steps to effectively write a background of the study.
1. Identify Your Audience:
Determine the level of expertise of your target audience. Tailor the depth and complexity of your background information accordingly.
2. Understand the Research Problem:
Define the research problem or question your study aims to address. Identify the significance of the problem within the broader context of the field.
3. Review Existing Literature:
Conduct a thorough literature review to understand what is already known in the area. Summarize key findings, theories, and concepts relevant to your research.
4. Include Historical Data:
Integrate historical data if relevant to the research, as current issues often trace back to historical events.
5. Identify Controversies and Gaps:
Note any controversies or debates within the existing literature. Identify gaps , limitations, or unanswered questions that your research can address.
6. Select Key Components:
Choose the most critical elements to include in the background based on their relevance to your research problem. Prioritize information that helps build a strong foundation for your study.
7. Craft a Logical Flow:
Organize the background information in a logical sequence. Start with general context, move to specific theories and concepts, and then focus on the specific problem.
8. Highlight the Novelty of Your Research:
Clearly explain the unique aspects or contributions of your study. Emphasize why your research is different from or builds upon existing work.
Here are some extra tips to increase the quality of your research background:
Example of a Research Background
Here is an example of a research background to help you understand better.
The above hypothetical example provides a research background, addresses the gap and highlights the potential outcome of the study; thereby aiding a better understanding of the proposed research.
What Makes the Introduction Different from the Background?
Your introduction is different from your background in a number of ways.
- The introduction contains preliminary data about your topic that the reader will most likely read , whereas the background clarifies the importance of the paper.
- The background of your study discusses in depth about the topic, whereas the introduction only gives an overview.
- The introduction should end with your research questions, aims, and objectives, whereas your background should not (except in some cases where your background is integrated into your introduction). For instance, the C.A.R.S. ( Creating a Research Space ) model, created by John Swales is based on his analysis of journal articles. This model attempts to explain and describe the organizational pattern of writing the introduction in social sciences.
Points to Note
Your background should begin with defining a topic and audience. It is important that you identify which topic you need to review and what your audience already knows about the topic. You should proceed by searching and researching the relevant literature. In this case, it is advisable to keep track of the search terms you used and the articles that you downloaded. It is helpful to use one of the research paper management systems such as Papers, Mendeley, Evernote, or Sente. Next, it is helpful to take notes while reading. Be careful when copying quotes verbatim and make sure to put them in quotation marks and cite the sources. In addition, you should keep your background focused but balanced enough so that it is relevant to a broader audience. Aside from these, your background should be critical, consistent, and logically structured.
Writing the background of your study should not be an overly daunting task. Many guides that can help you organize your thoughts as you write the background. The background of the study is the key to introduce your audience to your research topic and should be done with strong knowledge and thoughtful writing.
The background of a research paper typically ranges from one to two paragraphs, summarizing the relevant literature and context of the study. It should be concise, providing enough information to contextualize the research problem and justify the need for the study. Journal instructions about any word count limits should be kept in mind while deciding on the length of the final content.
The background of a research paper provides the context and relevant literature to understand the research problem, while the introduction also introduces the specific research topic, states the research objectives, and outlines the scope of the study. The background focuses on the broader context, whereas the introduction focuses on the specific research project and its objectives.
When writing the background for a study, start by providing a brief overview of the research topic and its significance in the field. Then, highlight the gaps in existing knowledge or unresolved issues that the study aims to address. Finally, summarize the key findings from relevant literature to establish the context and rationale for conducting the research, emphasizing the need and importance of the study within the broader academic landscape.
The background in a research paper is crucial as it sets the stage for the study by providing essential context and rationale. It helps readers understand the significance of the research problem and its relevance in the broader field. By presenting relevant literature and highlighting gaps, the background justifies the need for the study, building a strong foundation for the research and enhancing its credibility.

The presentation very informative

It is really educative. I love the workshop. It really motivated me into writing my first paper for publication.

an interesting clue here, thanks.
thanks for the answers.
Good and interesting explanation. Thanks
Thank you for good presentation.
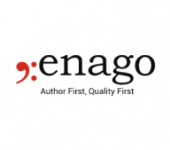
Hi Adam, we are glad to know that you found our article beneficial
The background of the study is the key to introduce your audience to YOUR research topic.
Awesome. Exactly what i was looking forwards to 😉
Hi Maryam, we are glad to know that you found our resource useful.
my understanding of ‘Background of study’ has been elevated.
Hi Peter, we are glad to know that our article has helped you get a better understanding of the background in a research paper.
thanks to give advanced information
Hi Shimelis, we are glad to know that you found the information in our article beneficial.
When i was studying it is very much hard for me to conduct a research study and know the background because my teacher in practical research is having a research so i make it now so that i will done my research
Very informative……….Thank you.
The confusion i had before, regarding an introduction and background to a research work is now a thing of the past. Thank you so much.
Thanks for your help…
Thanks for your kind information about the background of a research paper.
Thanks for the answer
Very informative. I liked even more when the difference between background and introduction was given. I am looking forward to learning more from this site. I am in Botswana
Hello, I am Benoît from Central African Republic. Right now I am writing down my research paper in order to get my master degree in British Literature. Thank you very much for posting all this information about the background of the study. I really appreciate. Thanks!
The write up is quite good, detailed and informative. Thanks a lot. The article has certainly enhanced my understanding of the topic.
Rate this article Cancel Reply
Your email address will not be published.
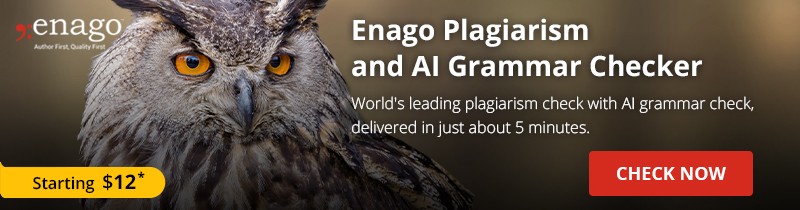
Enago Academy's Most Popular Articles
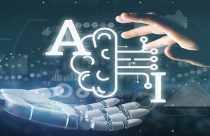
- AI in Academia
- Infographic
- Manuscripts & Grants
- Reporting Research
- Trending Now
Can AI Tools Prepare a Research Manuscript From Scratch? — A comprehensive guide
As technology continues to advance, the question of whether artificial intelligence (AI) tools can prepare…
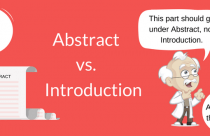
Abstract Vs. Introduction — Do you know the difference?
Ross wants to publish his research. Feeling positive about his research outcomes, he begins to…
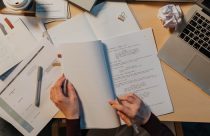
- Old Webinars
- Webinar Mobile App
Demystifying Research Methodology With Field Experts
Choosing research methodology Research design and methodology Evidence-based research approach How RAxter can assist researchers
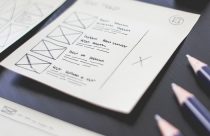
- Manuscript Preparation
- Publishing Research
How to Choose Best Research Methodology for Your Study
Successful research conduction requires proper planning and execution. While there are multiple reasons and aspects…
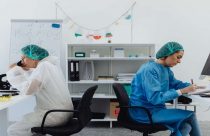
Top 5 Key Differences Between Methods and Methodology
While burning the midnight oil during literature review, most researchers do not realize that the…
How to Draft the Acknowledgment Section of a Manuscript
Discussion Vs. Conclusion: Know the Difference Before Drafting Manuscripts

Sign-up to read more
Subscribe for free to get unrestricted access to all our resources on research writing and academic publishing including:
- 2000+ blog articles
- 50+ Webinars
- 10+ Expert podcasts
- 50+ Infographics
- 10+ Checklists
- Research Guides
We hate spam too. We promise to protect your privacy and never spam you.
I am looking for Editing/ Proofreading services for my manuscript Tentative date of next journal submission:
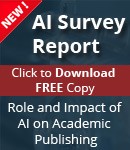
What should universities' stance be on AI tools in research and academic writing?
Have a language expert improve your writing
Run a free plagiarism check in 10 minutes, generate accurate citations for free.
- Knowledge Base
Methodology
- What Is Quantitative Research? | Definition, Uses & Methods
What Is Quantitative Research? | Definition, Uses & Methods
Published on June 12, 2020 by Pritha Bhandari . Revised on June 22, 2023.
Quantitative research is the process of collecting and analyzing numerical data. It can be used to find patterns and averages, make predictions, test causal relationships, and generalize results to wider populations.
Quantitative research is the opposite of qualitative research , which involves collecting and analyzing non-numerical data (e.g., text, video, or audio).
Quantitative research is widely used in the natural and social sciences: biology, chemistry, psychology, economics, sociology, marketing, etc.
- What is the demographic makeup of Singapore in 2020?
- How has the average temperature changed globally over the last century?
- Does environmental pollution affect the prevalence of honey bees?
- Does working from home increase productivity for people with long commutes?
Table of contents
Quantitative research methods, quantitative data analysis, advantages of quantitative research, disadvantages of quantitative research, other interesting articles, frequently asked questions about quantitative research.
You can use quantitative research methods for descriptive, correlational or experimental research.
- In descriptive research , you simply seek an overall summary of your study variables.
- In correlational research , you investigate relationships between your study variables.
- In experimental research , you systematically examine whether there is a cause-and-effect relationship between variables.
Correlational and experimental research can both be used to formally test hypotheses , or predictions, using statistics. The results may be generalized to broader populations based on the sampling method used.
To collect quantitative data, you will often need to use operational definitions that translate abstract concepts (e.g., mood) into observable and quantifiable measures (e.g., self-ratings of feelings and energy levels).
Note that quantitative research is at risk for certain research biases , including information bias , omitted variable bias , sampling bias , or selection bias . Be sure that you’re aware of potential biases as you collect and analyze your data to prevent them from impacting your work too much.

Here's why students love Scribbr's proofreading services
Discover proofreading & editing
Once data is collected, you may need to process it before it can be analyzed. For example, survey and test data may need to be transformed from words to numbers. Then, you can use statistical analysis to answer your research questions .
Descriptive statistics will give you a summary of your data and include measures of averages and variability. You can also use graphs, scatter plots and frequency tables to visualize your data and check for any trends or outliers.
Using inferential statistics , you can make predictions or generalizations based on your data. You can test your hypothesis or use your sample data to estimate the population parameter .
First, you use descriptive statistics to get a summary of the data. You find the mean (average) and the mode (most frequent rating) of procrastination of the two groups, and plot the data to see if there are any outliers.
You can also assess the reliability and validity of your data collection methods to indicate how consistently and accurately your methods actually measured what you wanted them to.
Quantitative research is often used to standardize data collection and generalize findings . Strengths of this approach include:
- Replication
Repeating the study is possible because of standardized data collection protocols and tangible definitions of abstract concepts.
- Direct comparisons of results
The study can be reproduced in other cultural settings, times or with different groups of participants. Results can be compared statistically.
- Large samples
Data from large samples can be processed and analyzed using reliable and consistent procedures through quantitative data analysis.
- Hypothesis testing
Using formalized and established hypothesis testing procedures means that you have to carefully consider and report your research variables, predictions, data collection and testing methods before coming to a conclusion.
Despite the benefits of quantitative research, it is sometimes inadequate in explaining complex research topics. Its limitations include:
- Superficiality
Using precise and restrictive operational definitions may inadequately represent complex concepts. For example, the concept of mood may be represented with just a number in quantitative research, but explained with elaboration in qualitative research.
- Narrow focus
Predetermined variables and measurement procedures can mean that you ignore other relevant observations.
- Structural bias
Despite standardized procedures, structural biases can still affect quantitative research. Missing data , imprecise measurements or inappropriate sampling methods are biases that can lead to the wrong conclusions.
- Lack of context
Quantitative research often uses unnatural settings like laboratories or fails to consider historical and cultural contexts that may affect data collection and results.
If you want to know more about statistics , methodology , or research bias , make sure to check out some of our other articles with explanations and examples.
- Chi square goodness of fit test
- Degrees of freedom
- Null hypothesis
- Discourse analysis
- Control groups
- Mixed methods research
- Non-probability sampling
- Inclusion and exclusion criteria
Research bias
- Rosenthal effect
- Implicit bias
- Cognitive bias
- Selection bias
- Negativity bias
- Status quo bias
Quantitative research deals with numbers and statistics, while qualitative research deals with words and meanings.
Quantitative methods allow you to systematically measure variables and test hypotheses . Qualitative methods allow you to explore concepts and experiences in more detail.
In mixed methods research , you use both qualitative and quantitative data collection and analysis methods to answer your research question .
Data collection is the systematic process by which observations or measurements are gathered in research. It is used in many different contexts by academics, governments, businesses, and other organizations.
Operationalization means turning abstract conceptual ideas into measurable observations.
For example, the concept of social anxiety isn’t directly observable, but it can be operationally defined in terms of self-rating scores, behavioral avoidance of crowded places, or physical anxiety symptoms in social situations.
Before collecting data , it’s important to consider how you will operationalize the variables that you want to measure.
Reliability and validity are both about how well a method measures something:
- Reliability refers to the consistency of a measure (whether the results can be reproduced under the same conditions).
- Validity refers to the accuracy of a measure (whether the results really do represent what they are supposed to measure).
If you are doing experimental research, you also have to consider the internal and external validity of your experiment.
Hypothesis testing is a formal procedure for investigating our ideas about the world using statistics. It is used by scientists to test specific predictions, called hypotheses , by calculating how likely it is that a pattern or relationship between variables could have arisen by chance.
Cite this Scribbr article
If you want to cite this source, you can copy and paste the citation or click the “Cite this Scribbr article” button to automatically add the citation to our free Citation Generator.
Bhandari, P. (2023, June 22). What Is Quantitative Research? | Definition, Uses & Methods. Scribbr. Retrieved April 9, 2024, from https://www.scribbr.com/methodology/quantitative-research/
Is this article helpful?
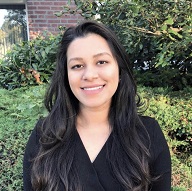
Pritha Bhandari
Other students also liked, descriptive statistics | definitions, types, examples, inferential statistics | an easy introduction & examples, "i thought ai proofreading was useless but..".
I've been using Scribbr for years now and I know it's a service that won't disappoint. It does a good job spotting mistakes”
- USC Libraries
- Research Guides
Organizing Your Social Sciences Research Paper
- Quantitative Methods
- Purpose of Guide
- Design Flaws to Avoid
- Independent and Dependent Variables
- Glossary of Research Terms
- Reading Research Effectively
- Narrowing a Topic Idea
- Broadening a Topic Idea
- Extending the Timeliness of a Topic Idea
- Academic Writing Style
- Applying Critical Thinking
- Choosing a Title
- Making an Outline
- Paragraph Development
- Research Process Video Series
- Executive Summary
- The C.A.R.S. Model
- Background Information
- The Research Problem/Question
- Theoretical Framework
- Citation Tracking
- Content Alert Services
- Evaluating Sources
- Primary Sources
- Secondary Sources
- Tiertiary Sources
- Scholarly vs. Popular Publications
- Qualitative Methods
- Insiderness
- Using Non-Textual Elements
- Limitations of the Study
- Common Grammar Mistakes
- Writing Concisely
- Avoiding Plagiarism
- Footnotes or Endnotes?
- Further Readings
- Generative AI and Writing
- USC Libraries Tutorials and Other Guides
- Bibliography
Quantitative methods emphasize objective measurements and the statistical, mathematical, or numerical analysis of data collected through polls, questionnaires, and surveys, or by manipulating pre-existing statistical data using computational techniques . Quantitative research focuses on gathering numerical data and generalizing it across groups of people or to explain a particular phenomenon.
Babbie, Earl R. The Practice of Social Research . 12th ed. Belmont, CA: Wadsworth Cengage, 2010; Muijs, Daniel. Doing Quantitative Research in Education with SPSS . 2nd edition. London: SAGE Publications, 2010.
Need Help Locating Statistics?
Resources for locating data and statistics can be found here:
Statistics & Data Research Guide
Characteristics of Quantitative Research
Your goal in conducting quantitative research study is to determine the relationship between one thing [an independent variable] and another [a dependent or outcome variable] within a population. Quantitative research designs are either descriptive [subjects usually measured once] or experimental [subjects measured before and after a treatment]. A descriptive study establishes only associations between variables; an experimental study establishes causality.
Quantitative research deals in numbers, logic, and an objective stance. Quantitative research focuses on numeric and unchanging data and detailed, convergent reasoning rather than divergent reasoning [i.e., the generation of a variety of ideas about a research problem in a spontaneous, free-flowing manner].
Its main characteristics are :
- The data is usually gathered using structured research instruments.
- The results are based on larger sample sizes that are representative of the population.
- The research study can usually be replicated or repeated, given its high reliability.
- Researcher has a clearly defined research question to which objective answers are sought.
- All aspects of the study are carefully designed before data is collected.
- Data are in the form of numbers and statistics, often arranged in tables, charts, figures, or other non-textual forms.
- Project can be used to generalize concepts more widely, predict future results, or investigate causal relationships.
- Researcher uses tools, such as questionnaires or computer software, to collect numerical data.
The overarching aim of a quantitative research study is to classify features, count them, and construct statistical models in an attempt to explain what is observed.
Things to keep in mind when reporting the results of a study using quantitative methods :
- Explain the data collected and their statistical treatment as well as all relevant results in relation to the research problem you are investigating. Interpretation of results is not appropriate in this section.
- Report unanticipated events that occurred during your data collection. Explain how the actual analysis differs from the planned analysis. Explain your handling of missing data and why any missing data does not undermine the validity of your analysis.
- Explain the techniques you used to "clean" your data set.
- Choose a minimally sufficient statistical procedure ; provide a rationale for its use and a reference for it. Specify any computer programs used.
- Describe the assumptions for each procedure and the steps you took to ensure that they were not violated.
- When using inferential statistics , provide the descriptive statistics, confidence intervals, and sample sizes for each variable as well as the value of the test statistic, its direction, the degrees of freedom, and the significance level [report the actual p value].
- Avoid inferring causality , particularly in nonrandomized designs or without further experimentation.
- Use tables to provide exact values ; use figures to convey global effects. Keep figures small in size; include graphic representations of confidence intervals whenever possible.
- Always tell the reader what to look for in tables and figures .
NOTE: When using pre-existing statistical data gathered and made available by anyone other than yourself [e.g., government agency], you still must report on the methods that were used to gather the data and describe any missing data that exists and, if there is any, provide a clear explanation why the missing data does not undermine the validity of your final analysis.
Babbie, Earl R. The Practice of Social Research . 12th ed. Belmont, CA: Wadsworth Cengage, 2010; Brians, Craig Leonard et al. Empirical Political Analysis: Quantitative and Qualitative Research Methods . 8th ed. Boston, MA: Longman, 2011; McNabb, David E. Research Methods in Public Administration and Nonprofit Management: Quantitative and Qualitative Approaches . 2nd ed. Armonk, NY: M.E. Sharpe, 2008; Quantitative Research Methods. Writing@CSU. Colorado State University; Singh, Kultar. Quantitative Social Research Methods . Los Angeles, CA: Sage, 2007.
Basic Research Design for Quantitative Studies
Before designing a quantitative research study, you must decide whether it will be descriptive or experimental because this will dictate how you gather, analyze, and interpret the results. A descriptive study is governed by the following rules: subjects are generally measured once; the intention is to only establish associations between variables; and, the study may include a sample population of hundreds or thousands of subjects to ensure that a valid estimate of a generalized relationship between variables has been obtained. An experimental design includes subjects measured before and after a particular treatment, the sample population may be very small and purposefully chosen, and it is intended to establish causality between variables. Introduction The introduction to a quantitative study is usually written in the present tense and from the third person point of view. It covers the following information:
- Identifies the research problem -- as with any academic study, you must state clearly and concisely the research problem being investigated.
- Reviews the literature -- review scholarship on the topic, synthesizing key themes and, if necessary, noting studies that have used similar methods of inquiry and analysis. Note where key gaps exist and how your study helps to fill these gaps or clarifies existing knowledge.
- Describes the theoretical framework -- provide an outline of the theory or hypothesis underpinning your study. If necessary, define unfamiliar or complex terms, concepts, or ideas and provide the appropriate background information to place the research problem in proper context [e.g., historical, cultural, economic, etc.].
Methodology The methods section of a quantitative study should describe how each objective of your study will be achieved. Be sure to provide enough detail to enable the reader can make an informed assessment of the methods being used to obtain results associated with the research problem. The methods section should be presented in the past tense.
- Study population and sampling -- where did the data come from; how robust is it; note where gaps exist or what was excluded. Note the procedures used for their selection;
- Data collection – describe the tools and methods used to collect information and identify the variables being measured; describe the methods used to obtain the data; and, note if the data was pre-existing [i.e., government data] or you gathered it yourself. If you gathered it yourself, describe what type of instrument you used and why. Note that no data set is perfect--describe any limitations in methods of gathering data.
- Data analysis -- describe the procedures for processing and analyzing the data. If appropriate, describe the specific instruments of analysis used to study each research objective, including mathematical techniques and the type of computer software used to manipulate the data.
Results The finding of your study should be written objectively and in a succinct and precise format. In quantitative studies, it is common to use graphs, tables, charts, and other non-textual elements to help the reader understand the data. Make sure that non-textual elements do not stand in isolation from the text but are being used to supplement the overall description of the results and to help clarify key points being made. Further information about how to effectively present data using charts and graphs can be found here .
- Statistical analysis -- how did you analyze the data? What were the key findings from the data? The findings should be present in a logical, sequential order. Describe but do not interpret these trends or negative results; save that for the discussion section. The results should be presented in the past tense.
Discussion Discussions should be analytic, logical, and comprehensive. The discussion should meld together your findings in relation to those identified in the literature review, and placed within the context of the theoretical framework underpinning the study. The discussion should be presented in the present tense.
- Interpretation of results -- reiterate the research problem being investigated and compare and contrast the findings with the research questions underlying the study. Did they affirm predicted outcomes or did the data refute it?
- Description of trends, comparison of groups, or relationships among variables -- describe any trends that emerged from your analysis and explain all unanticipated and statistical insignificant findings.
- Discussion of implications – what is the meaning of your results? Highlight key findings based on the overall results and note findings that you believe are important. How have the results helped fill gaps in understanding the research problem?
- Limitations -- describe any limitations or unavoidable bias in your study and, if necessary, note why these limitations did not inhibit effective interpretation of the results.
Conclusion End your study by to summarizing the topic and provide a final comment and assessment of the study.
- Summary of findings – synthesize the answers to your research questions. Do not report any statistical data here; just provide a narrative summary of the key findings and describe what was learned that you did not know before conducting the study.
- Recommendations – if appropriate to the aim of the assignment, tie key findings with policy recommendations or actions to be taken in practice.
- Future research – note the need for future research linked to your study’s limitations or to any remaining gaps in the literature that were not addressed in your study.
Black, Thomas R. Doing Quantitative Research in the Social Sciences: An Integrated Approach to Research Design, Measurement and Statistics . London: Sage, 1999; Gay,L. R. and Peter Airasain. Educational Research: Competencies for Analysis and Applications . 7th edition. Upper Saddle River, NJ: Merril Prentice Hall, 2003; Hector, Anestine. An Overview of Quantitative Research in Composition and TESOL . Department of English, Indiana University of Pennsylvania; Hopkins, Will G. “Quantitative Research Design.” Sportscience 4, 1 (2000); "A Strategy for Writing Up Research Results. The Structure, Format, Content, and Style of a Journal-Style Scientific Paper." Department of Biology. Bates College; Nenty, H. Johnson. "Writing a Quantitative Research Thesis." International Journal of Educational Science 1 (2009): 19-32; Ouyang, Ronghua (John). Basic Inquiry of Quantitative Research . Kennesaw State University.
Strengths of Using Quantitative Methods
Quantitative researchers try to recognize and isolate specific variables contained within the study framework, seek correlation, relationships and causality, and attempt to control the environment in which the data is collected to avoid the risk of variables, other than the one being studied, accounting for the relationships identified.
Among the specific strengths of using quantitative methods to study social science research problems:
- Allows for a broader study, involving a greater number of subjects, and enhancing the generalization of the results;
- Allows for greater objectivity and accuracy of results. Generally, quantitative methods are designed to provide summaries of data that support generalizations about the phenomenon under study. In order to accomplish this, quantitative research usually involves few variables and many cases, and employs prescribed procedures to ensure validity and reliability;
- Applying well established standards means that the research can be replicated, and then analyzed and compared with similar studies;
- You can summarize vast sources of information and make comparisons across categories and over time; and,
- Personal bias can be avoided by keeping a 'distance' from participating subjects and using accepted computational techniques .
Babbie, Earl R. The Practice of Social Research . 12th ed. Belmont, CA: Wadsworth Cengage, 2010; Brians, Craig Leonard et al. Empirical Political Analysis: Quantitative and Qualitative Research Methods . 8th ed. Boston, MA: Longman, 2011; McNabb, David E. Research Methods in Public Administration and Nonprofit Management: Quantitative and Qualitative Approaches . 2nd ed. Armonk, NY: M.E. Sharpe, 2008; Singh, Kultar. Quantitative Social Research Methods . Los Angeles, CA: Sage, 2007.
Limitations of Using Quantitative Methods
Quantitative methods presume to have an objective approach to studying research problems, where data is controlled and measured, to address the accumulation of facts, and to determine the causes of behavior. As a consequence, the results of quantitative research may be statistically significant but are often humanly insignificant.
Some specific limitations associated with using quantitative methods to study research problems in the social sciences include:
- Quantitative data is more efficient and able to test hypotheses, but may miss contextual detail;
- Uses a static and rigid approach and so employs an inflexible process of discovery;
- The development of standard questions by researchers can lead to "structural bias" and false representation, where the data actually reflects the view of the researcher instead of the participating subject;
- Results provide less detail on behavior, attitudes, and motivation;
- Researcher may collect a much narrower and sometimes superficial dataset;
- Results are limited as they provide numerical descriptions rather than detailed narrative and generally provide less elaborate accounts of human perception;
- The research is often carried out in an unnatural, artificial environment so that a level of control can be applied to the exercise. This level of control might not normally be in place in the real world thus yielding "laboratory results" as opposed to "real world results"; and,
- Preset answers will not necessarily reflect how people really feel about a subject and, in some cases, might just be the closest match to the preconceived hypothesis.
Research Tip
Finding Examples of How to Apply Different Types of Research Methods
SAGE publications is a major publisher of studies about how to design and conduct research in the social and behavioral sciences. Their SAGE Research Methods Online and Cases database includes contents from books, articles, encyclopedias, handbooks, and videos covering social science research design and methods including the complete Little Green Book Series of Quantitative Applications in the Social Sciences and the Little Blue Book Series of Qualitative Research techniques. The database also includes case studies outlining the research methods used in real research projects. This is an excellent source for finding definitions of key terms and descriptions of research design and practice, techniques of data gathering, analysis, and reporting, and information about theories of research [e.g., grounded theory]. The database covers both qualitative and quantitative research methods as well as mixed methods approaches to conducting research.
SAGE Research Methods Online and Cases
- << Previous: Qualitative Methods
- Next: Insiderness >>
- Last Updated: Apr 11, 2024 1:27 PM
- URL: https://libguides.usc.edu/writingguide
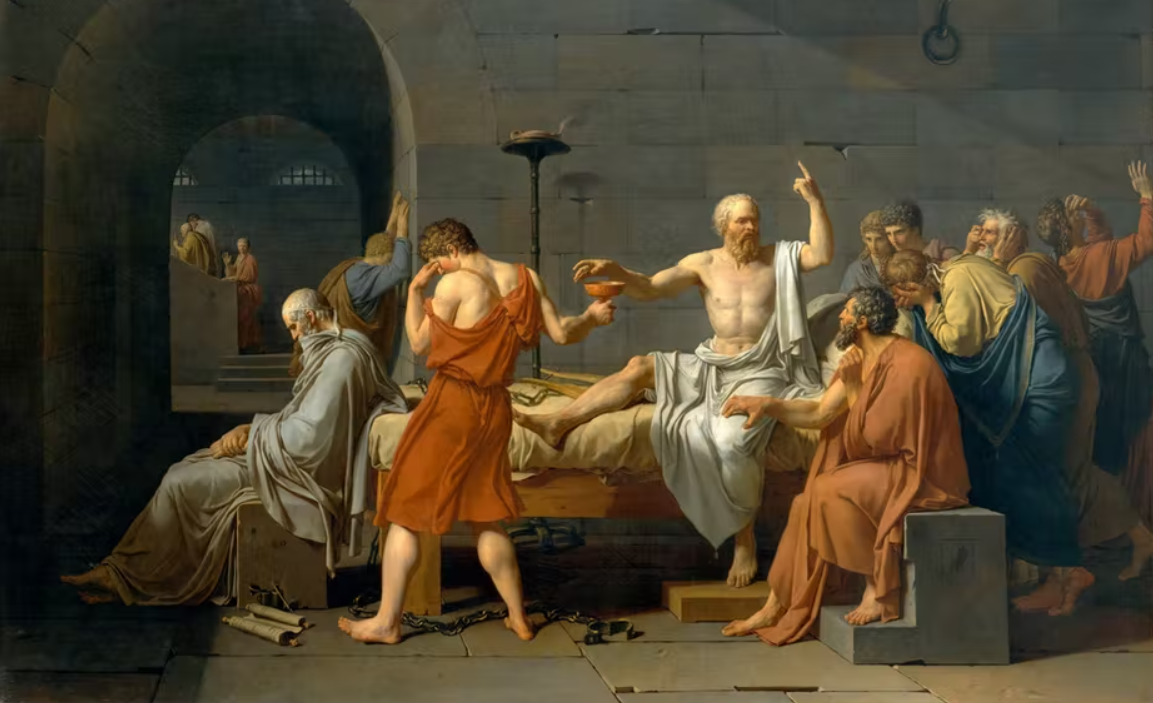
PHILO-notes
Free Online Learning Materials
How to Write the Background of the Study in Research (Part 1)
Background of the Study in Research: Definition and the Core Elements it Contains
Before we embark on a detailed discussion on how to write the background of the study of your proposed research or thesis, it is important to first discuss its meaning and the core elements that it should contain. This is obviously because understanding the nature of the background of the study in research and knowing exactly what to include in it allow us to have both greater control and clear direction of the writing process.
So, what really is the background of the study and what are the core elements that it should contain?
The background of the study, which usually forms the first section of the introduction to a research paper or thesis, provides the overview of the study. In other words, it is that section of the research paper or thesis that establishes the context of the study. Its main function is to explain why the proposed research is important and essential to understanding the main aspects of the study.
The background of the study, therefore, is the section of the research paper or thesis that identifies the problem or gap of the study that needs to addressed and justifies the need for conducting the study. It also articulates the main goal of the study and the thesis statement, that is, the main claim or argument of the paper.
Given this brief understanding of the background of the study, we can anticipate what readers or thesis committee members expect from it. As we can see, the background of the study should contain the following major points:
1) brief discussion on what is known about the topic under investigation; 2) An articulation of the research gap or problem that needs to be addressed; 3) What the researcher would like to do or aim to achieve in the study ( research goal); 4) The thesis statement, that is, the main argument or contention of the paper (which also serves as the reason why the researcher would want to pursue the study); 5) The major significance or contribution of the study to a particular discipline; and 6) Depending on the nature of the study, an articulation of the hypothesis of the study.
Thus, when writing the background of the study, you should plan and structure it based on the major points just mentioned. With this, you will have a clear picture of the flow of the tasks that need to be completed in writing this section of your research or thesis proposal.
Now, how do you go about writing the background of the study in your proposed research or thesis?
The next lessons will address this question.
How to Write the Opening Paragraphs of the Background of the Study?
To begin with, let us assume that you already have conducted a preliminary research on your chosen topic, that is, you already have read a lot of literature and gathered relevant information for writing the background of your study. Let us also assume that you already have identified the gap of your proposed research and have already developed the research questions and thesis statement. If you have not yet identified the gap in your proposed research, you might as well go back to our lesson on how to identify a research gap.
So, we will just put together everything that you have researched into a background of the study (assuming, again, that you already have the necessary information). But in this lesson, let’s just focus on writing the opening paragraphs.
It is important to note at this point that there are different styles of writing the background of the study. Hence, what I will be sharing with you here is not just “the” only way of writing the background of the study. As a matter of fact, there is no “one-size-fits-all” style of writing this part of the research or thesis. At the end of the day, you are free to develop your own. However, whatever style it would be, it always starts with a plan which structures the writing process into stages or steps. The steps that I will share with below are just some of the most effective ways of writing the background of the study in research.
So, let’s begin.
It is always a good idea to begin the background of your study by giving an overview of your research topic. This may include providing a definition of the key concepts of your research or highlighting the main developments of the research topic.
Let us suppose that the topic of your study is the “lived experiences of students with mathematical anxiety”.
Here, you may start the background of your study with a discussion on the meaning, nature, and dynamics of the term “mathematical anxiety”. The reason for this is too obvious: “mathematical anxiety” is a highly technical term that is specific to mathematics. Hence, this term is not readily understandable to non-specialists in this field.
So, you may write the opening paragraph of your background of the study with this:
“Mathematical anxiety refers to the individual’s unpleasant emotional mood responses when confronted with a mathematical situation.”
Since you do not invent the definition of the term “mathematical anxiety”, then you need to provide a citation to the source of the material from which you are quoting. For example, you may now say:
“Mathematical anxiety refers to the individual’s unpleasant emotional mood responses when confronted with a mathematical situation (Eliot, 2020).”
And then you may proceed with the discussion on the nature and dynamics of the term “mathematical anxiety”. You may say:
“Lou (2019) specifically identifies some of the manifestations of this type of anxiety, which include, but not limited to, depression, helplessness, nervousness and fearfulness in doing mathematical and numerical tasks.”
After explaining to your readers the meaning, nature, and dynamics (as well as some historical development if you wish to) of the term “mathematical anxiety”, you may now proceed to showing the problem or gap of the study. As you may already know, the research gap is the problem that needs to be addressed in the study. This is important because no research activity is possible without the research gap.
Let us suppose that your research problem or gap is: “Mathematical anxiety can negatively affect not just the academic achievement of the students but also their future career plans and total well-being. Also, there are no known studies that deal with the mathematical anxiety of junior high school students in New Zealand.” With this, you may say:
“If left unchecked, as Shapiro (2019) claims, this problem will expand and create a total avoidance pattern on the part of the students, which can be expressed most visibly in the form of cutting classes and habitual absenteeism. As we can see, this will negatively affect the performance of students in mathematics. In fact, the study conducted by Luttenberger and Wimmer (2018) revealed that the outcomes of mathematical anxiety do not only negatively affect the students’ performance in math-related situations but also their future career as professionals. Without a doubt, therefore, mathematical anxiety is a recurring problem for many individuals which will negatively affect the academic success and future career of the student.”
Now that you already have both explained the meaning, nature, and dynamics of the term “mathematical anxiety” and articulated the gap of your proposed research, you may now state the main goal of your study. You may say:
“Hence, it is precisely in this context that the researcher aims to determine the lived experiences of those students with mathematical anxiety. In particular, this proposed thesis aims to determine the lived experiences of the junior high school students in New Zealand and identify the factors that caused them to become disinterested in mathematics.”
Please note that you should not end the first paragraph of your background of the study with the articulation of the research goal. You also need to articulate the “thesis statement”, which usually comes after the research goal. As is well known, the thesis statement is the statement of your argument or contention in the study. It is more of a personal argument or claim of the researcher, which specifically highlights the possible contribution of the study. For example, you may say:
“The researcher argues that there is a need to determine the lived experiences of these students with mathematical anxiety because knowing and understanding the difficulties and challenges that they have encountered will put the researcher in the best position to offer some alternatives to the problem. Indeed, it is only when we have performed some kind of a ‘diagnosis’ that we can offer practicable solutions to the problem. And in the case of the junior high school students in New Zealand who are having mathematical anxiety, determining their lived experiences as well as identifying the factors that caused them to become disinterested in mathematics are the very first steps in addressing the problem.”
If we combine the bits and pieces that we have written above, we can now come up with the opening paragraphs of your background of the study, which reads:
As we can see, we can find in the first paragraph 5 essential elements that must be articulated in the background of the study, namely:
1) A brief discussion on what is known about the topic under investigation; 2) An articulation of the research gap or problem that needs to be addressed; 3) What the researcher would like to do or aim to achieve in the study (research goal); 4) The thesis statement , that is, the main argument or claim of the paper; and 5) The major significance or contribution of the study to a particular discipline. So, that’s how you write the opening paragraphs of your background of the study. The next lesson will talk about writing the body of the background of the study.
How to Write the Body of the Background of the Study?
If we liken the background of the study to a sitting cat, then the opening paragraphs that we have completed in the previous lesson would just represent the head of the cat.
This means we still have to write the body (body of the cat) and the conclusion (tail). But how do we write the body of the background of the study? What should be its content?
Truly, this is one of the most difficult challenges that fledgling scholars faced. Because they are inexperienced researchers and didn’t know what to do next, they just wrote whatever they wished to write. Fortunately, this is relatively easy if they know the technique.
One of the best ways to write the body of the background of the study is to attack it from the vantage point of the research gap. If you recall, when we articulated the research gap in the opening paragraphs, we made a bold claim there, that is, there are junior high school students in New Zealand who are experiencing mathematical anxiety. Now, you have to remember that a “statement” remains an assumption until you can provide concrete proofs to it. This is what we call the “epistemological” aspect of research. As we may already know, epistemology is a specific branch of philosophy that deals with the validity of knowledge. And to validate knowledge is to provide concrete proofs to our statements. Hence, the reason why we need to provide proofs to our claim that there are indeed junior high school students in New Zealand who are experiencing mathematical anxiety is the obvious fact that if there are none, then we cannot proceed with our study. We have no one to interview with in the first. In short, we don’t have respondents.
The body of the background of the study, therefore, should be a presentation and articulation of the proofs to our claim that indeed there are junior high school students in New Zealand who are experiencing mathematical anxiety. Please note, however, that this idea is true only if you follow the style of writing the background of the study that I introduced in this course.
So, how do we do this?
One of the best ways to do this is to look for literature on mathematical anxiety among junior high school students in New Zealand and cite them here. However, if there are not enough literature on this topic in New Zealand, then we need to conduct initial interviews with these students or make actual classroom observations and record instances of mathematical anxiety among these students. But it is always a good idea if we combine literature review with interviews and actual observations.
Assuming you already have the data, then you may now proceed with the writing of the body of your background of the study. For example, you may say:
“According to records and based on the researcher’s firsthand experience with students in some junior high schools in New Zealand, indeed, there are students who lost interest in mathematics. For one, while checking the daily attendance and monitoring of the students, it was observed that some of them are not always attending classes in mathematics but are regularly attending the rest of the required subjects.”
After this sentence, you may insert some literature that will support this position. For example, you may say:
“As a matter of fact, this phenomenon is also observed in the work of Estonanto. In his study titled ‘Impact of Math Anxiety on Academic Performance in Pre-Calculus of Senior High School’, Estonanto (2019) found out that, inter alia, students with mathematical anxiety have the tendency to intentionally prioritize other subjects and commit habitual tardiness and absences.”
Then you may proceed saying:
“With this initial knowledge in mind, the researcher conducted initial interviews with some of these students. The researcher learned that one student did not regularly attend his math subject because he believed that he is not good in math and no matter how he listens to the topic he will not learn.”
Then you may say:
“Another student also mentioned that she was influenced by her friends’ perception that mathematics is hard; hence, she avoids the subject. Indeed, these are concrete proofs that there are some junior high school students in New Zealand who have mathematical anxiety. As already hinted, “disinterest” or the loss of interest in mathematics is one of the manifestations of a mathematical anxiety.”
If we combine what we have just written above, then we can have the first two paragraphs of the body of our background of the study. It reads:
“According to records and based on the researcher’s firsthand experience with students in some junior high schools in New Zealand, indeed there are students who lost interest in mathematics. For one, while checking the daily attendance and monitoring of the students, it was observed that some of them are not always attending classes in mathematics but are regularly attending the rest of the required subjects. As a matter of fact, this phenomenon is also observed in the work of Estonanto. In his study titled ‘Impact of Math Anxiety on Academic Performance in Pre-Calculus of Senior High School’, Estonanto (2019) found out that, inter alia, students with mathematical anxiety have the tendency to intentionally prioritize other subjects and commit habitual tardiness and absences.
With this initial knowledge in mind, the researcher conducted initial interviews with some of these students. The researcher learned that one student did not regularly attend his math subject because he believed that he is not good in math and no matter how he listens to the topic he will not learn. Another student also mentioned that she was influenced by her friends’ perception that mathematics is hard; hence, she avoids the subject. Indeed, these are concrete proofs that there are some junior high school students in New Zealand who have mathematical anxiety. As already hinted, “disinterest” or the loss of interest in mathematics is one of the manifestations of a mathematical anxiety.”
And then you need validate this observation by conducting another round of interview and observation in other schools. So, you may continue writing the body of the background of the study with this:
“To validate the information gathered from the initial interviews and observations, the researcher conducted another round of interview and observation with other junior high school students in New Zealand.”
“On the one hand, the researcher found out that during mathematics time some students felt uneasy; in fact, they showed a feeling of being tensed or anxious while working with numbers and mathematical problems. Some were even afraid to seat in front, while some students at the back were secretly playing with their mobile phones. These students also show remarkable apprehension during board works like trembling hands, nervous laughter, and the like.”
Then provide some literature that will support your position. You may say:
“As Finlayson (2017) corroborates, emotional symptoms of mathematical anxiety involve feeling of helplessness, lack of confidence, and being nervous for being put on the spot. It must be noted that these occasionally extreme emotional reactions are not triggered by provocative procedures. As a matter of fact, there are no personally sensitive questions or intentional manipulations of stress. The teacher simply asked a very simple question, like identifying the parts of a circle. Certainly, this observation also conforms with the study of Ashcraft (2016) when he mentions that students with mathematical anxiety show a negative attitude towards math and hold self-perceptions about their mathematical abilities.”
And then you proceed:
“On the other hand, when the class had their other subjects, the students show a feeling of excitement. They even hurried to seat in front and attentively participating in the class discussion without hesitation and without the feeling of being tensed or anxious. For sure, this is another concrete proof that there are junior high school students in New Zealand who have mathematical anxiety.”
To further prove the point that there indeed junior high school students in New Zealand who have mathematical anxiety, you may solicit observations from other math teachers. For instance, you may say:
“The researcher further verified if the problem is also happening in other sections and whether other mathematics teachers experienced the same observation that the researcher had. This validation or verification is important in establishing credibility of the claim (Buchbinder, 2016) and ensuring reliability and validity of the assertion (Morse et al., 2002). In this regard, the researcher attempted to open up the issue of math anxiety during the Departmentalized Learning Action Cell (LAC), a group discussion of educators per quarter, with the objective of ‘Teaching Strategies to Develop Critical Thinking of the Students’. During the session, one teacher corroborates the researcher’s observation that there are indeed junior high school students in New Zealand who have mathematical anxiety. The teacher pointed out that truly there were students who showed no extra effort in mathematics class in addition to the fact that some students really avoided the subject. In addition, another math teacher expressed her frustrations about these students who have mathematical anxiety. She quipped: “How can a teacher develop the critical thinking skills or ability of the students if in the first place these students show avoidance and disinterest in the subject?’.”
Again, if we combine what we have just written above, then we can now have the remaining parts of the body of the background of the study. It reads:
So, that’s how we write the body of the background of the study in research . Of course, you may add any relevant points which you think might amplify your content. What is important at this point is that you now have a clear idea of how to write the body of the background of the study.
How to Write the Concluding Part of the Background of the Study?
Since we have already completed the body of our background of the study in the previous lesson, we may now write the concluding paragraph (the tail of the cat). This is important because one of the rules of thumb in writing is that we always put a close to what we have started.
It is important to note that the conclusion of the background of the study is just a rehashing of the research gap and main goal of the study stated in the introductory paragraph, but framed differently. The purpose of this is just to emphasize, after presenting the justifications, what the study aims to attain and why it wants to do it. The conclusion, therefore, will look just like this:
“Given the above discussion, it is evident that there are indeed junior high school students in New Zealand who are experiencing mathematical anxiety. And as we can see, mathematical anxiety can negatively affect not just the academic achievement of the students but also their future career plans and total well-being. Again, it is for this reason that the researcher attempts to determine the lived experiences of those junior high school students in New Zealand who are experiencing a mathematical anxiety.”
If we combine all that we have written from the very beginning, the entire background of the study would now read:
If we analyze the background of the study that we have just completed, we can observe that in addition to the important elements that it should contain, it has also addressed other important elements that readers or thesis committee members expect from it.
On the one hand, it provides the researcher with a clear direction in the conduct of the study. As we can see, the background of the study that we have just completed enables us to move in the right direction with a strong focus as it has set clear goals and the reasons why we want to do it. Indeed, we now exactly know what to do next and how to write the rest of the research paper or thesis.
On the other hand, most researchers start their research with scattered ideas and usually get stuck with how to proceed further. But with a well-written background of the study, just as the one above, we have decluttered and organized our thoughts. We have also become aware of what have and have not been done in our area of study, as well as what we can significantly contribute in the already existing body of knowledge in this area of study.
Please note, however, as I already mentioned previously, that the model that I have just presented is only one of the many models available in textbooks and other sources. You are, of course, free to choose your own style of writing the background of the study. You may also consult your thesis supervisor for some guidance on how to attack the writing of your background of the study.
Lastly, and as you may already know, universities around the world have their own thesis formats. Hence, you should follow your university’s rules on the format and style in writing your research or thesis. What is important is that with the lessons that you learned in this course, you can now easily write the introductory part of your thesis, such as the background of the study.
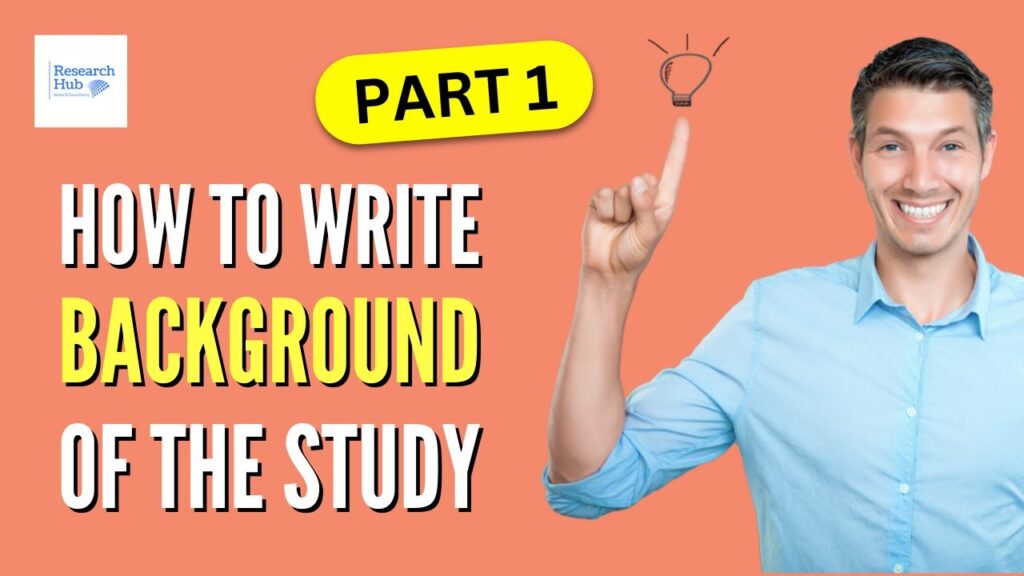
Quantitative research in education : Background information
- Background information
- SAGE researchmethods SAGE Research Methods is a tool created to help researchers, faculty and students with their research projects. Users can explore methods concepts to help them design research projects, understand particular methods or identify a new method, conduct their research, and write up their findings. Since SAGE Research Methods focuses on methodology rather than disciplines, it can be used across the social sciences, health sciences, and other areas of research.
- The American freshman, national norms for ... From the Higher Education Research Institute, University of California, Los Angeles
- Education at a glance : OECD indicators
- Global education digest From UNESCO
- Next: Recent e-books >>
- Recent e-books
- Recent print books
- Connect to Stanford e-resources

- Last Updated: Jan 23, 2024 12:46 PM
- URL: https://guides.library.stanford.edu/quantitative_research_in_ed
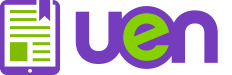
Key Concepts in Quantitative Research
In this module, we are going to explore the nuances of quantitative research, including the main types of quantitative research, more exploration into variables (including confounding and extraneous variables), and causation.
Content includes:
- Flaws, “Proof”, and Rigor
- The Steps of Quantitative Methodology
- Major Classes of Quantitative Research
- Experimental versus Non-Experimental Research
- Types of Experimental Research
- Types of Non-Experimental Research
- Research Variables
- Confounding/Extraneous Variables
- Causation versus correlation/association
Objectives:
- Discuss the flaws, proof, and rigor in research.
- Describe the differences between independent variables and dependent variables.
- Describe the steps in quantitative research methodology.
- Describe experimental, quasi-experimental, and non-experimental research studies
- Describe confounding and extraneous variables.
- Differentiate cause-and-effect (causality) versus association/correlation
Flaws, Proof, and Rigor in Research
One of the biggest hurdles that students and seasoned researchers alike struggle to grasp, is that research cannot “ prove ” nor “ disprove ”. Research can only support a hypothesis with reasonable, statistically significant evidence.
Indeed. You’ve heard it incorrectly your entire life. You will hear professors, scientists, radio ads, podcasts, and even researchers comment something to the effect of, “It has been proven that…” or “Research proves that…” or “Finally! There is proof that…”
We have been duped. Consider the “ prove ” word a very bad word in this course. The forbidden “P” word. Do not say it, write it, allude to it, or repeat it. And, for the love of avocados and all things fluffy, do not include the “P” word on your EBP poster. You will be deducted some major points.
We can only conclude with reasonable certainty through statistical analyses that there is a high probability that something did not happen by chance but instead happened due to the intervention that the researcher tested. Got that? We will come back to that concept but for now know that it is called “statistical significance”.
All research has flaws. We might not know what those flaws are, but we will be learning about confounding and extraneous variables later on in this module to help explain how flaws can happen.
Remember this: Sometimes, the researcher might not even know that there was a flaw that occurred. No research project is perfect. There is no 100% awesome. This is a major reason why it is so important to be able to duplicate a research project and obtain similar results. The more we can duplicate research with the same exact methodology and protocols, the more certainty we have in the results and we can start accounting for flaws that may have sneaked in.
Finally, not all research is equal. Some research is done very sloppily, and other research has a very high standard of rigor. How do we know which is which when reading an article? Well, within this module, we will start learning about some things to look for in a published research article to help determine rigor. We do not want lazy research to determine our actions as nurses, right? We want the strongest, most reliable, most valid, most rigorous research evidence possible so that we can take those results and embed them into patient care. Who wants shoddy evidence determining the actions we take with your grandmother’s heart surgery?
Independent Variables and Dependent Variables
As we were already introduced to, there are measures called “variables” in research. This will be a bit of a review but it is important to bring up again, as it is a hallmark of quantitative research. In quantitative studies, the concepts being measured are called variables (AKA: something that varies). Variables are something that can change – either by manipulation or from something causing a change. In the article snapshots that we have looked at, researchers are trying to find causes for phenomena. Does a nursing intervention cause an improvement in patient outcomes? Does the cholesterol medication cause a decrease in cholesterol level? Does smoking cause cancer?
The presumed cause is called the independent variable. The presumed effect is called the dependent variable. The dependent variable is “dependent” on something causing it to change. The dependent variable is the outcome that a researcher is trying to understand, explain, or predict.
Think back to our PICO questions. You can think of the intervention (I) as the independent variable and the outcome (O) as the dependent variable.
The independent variable is manipulated by the researcher or can be variants of influence. Whereas the dependent variable is never manipulated.
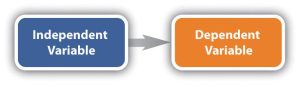
Variables do not always measure cause-and-effect. They can also measure a direction of influence.
Here is an example of that: If we compared levels of depression among men and women diagnosed with pancreatic cancer and found men to be more depressed, we cannot conclude that depression was caused by gender. However, we can note that the direction of influence clearly runs from gender to depression. It makes no sense to suggest the depression influenced their gender.
In the above example, what is the independent variable (IV) and what is the dependent variable (DV)? If you guessed gender as the IV and depression as the DV, you are correct! Important to note in this case that the researcher did not manipulate the IV, but the IV is manipulated on its own (male or female).
Researchers do not always have just one IV. In some cases, more than one IV may be measured. Take, for instance, a study that wants to measure the factors that influence one’s study habits. Independent variables of gender, sleep habits, and hours of work may be considered. Likewise, multiple DVs can be measured. For example, perhaps we want to measure weight and abdominal girth on a plant-based diet (IV).
Now, some studies do not have an intervention. We will come back to that when we talk about non-experimental research.
The point of variables is so that researchers have a very specific measurement that they seek to study.
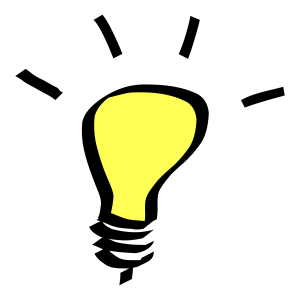
Let’s look at a couple of examples:
Now you try! Identify the IVs and DVs:
IV and DV Case Studies (Leibold, 2020)
Case Three: Independent variable: Healthy Lifestyle education with a focus on physical activity; Dependent variable: Physical activity rate before and after education intervention, Heart rate before and after education intervention, Blood pressures before and after education intervention.
Case Four: Independent variable: Playing classical music; Dependent variable: Grade point averages post classical music, compared to pre-classical music.
Case Five: Independent variable: No independent variable as there is no intervention. Dependent variable: The themes that emerge from the qualitative data.
The Steps in Quantitative Research Methodology
Now, as we learned in the last module, quantitative research is completely objective. There is no subjectivity to it. Why is this? Well, as we have learned, the purpose of quantitative research is to make an inference about the results in order to generalize these results to the population.
In quantitative studies, there is a very systematic approach that moves from the beginning point of the study (writing a research question) to the end point (obtaining an answer). This is a very linear and purposeful flow across the study, and all quantitative research should follow the same sequence.
- Identifying a problem and formulating a research question . Quantitative research begins with a theory . As in, “something is wrong and we want to fix it or improve it”. Think back to when we discussed research problems and formulating a research question. Here we are! That is the first step in formulating a quantitative research plan.
- Formulate a hypothesis . This step is key. Researchers need to know exactly what they are testing so that testing the hypothesis can be achieved through specific statistical analyses.
- A thorough literature review . At this step, researchers strive to understand what is already known about a topic and what evidence already exists.
- Identifying a framework . When an appropriate framework is identified, the findings of a study may have broader significance and utility (Polit & Beck, 2021).
- Choosing a study design . The research design will determine exactly how the researcher will obtain the answers to the research question(s). The entire design needs to be structured and controlled, with the overarching goal of minimizing bias and errors. The design determines what data will be collected and how, how often data will be collected, what types of comparisons will be made. You can think of the study design as the architectural backbone of the entire study.
- Sampling . The researcher needs to determine a subset of the population that is to be studied. We will come back to the sampling concept in the next module. However, the goal of sampling is to choose a subset of the population that adequate reflects the population of interest.
- I nstruments to be used to collect data (with reliability and validity as a priority). Researchers must find a way to measure the research variables (intervention and outcome) accurately. The task of measuring is complex and challenging, as data needs to be collected reliably (measuring consistently each time) and valid. Reliability and validity are both about how well a method measures something. The next module will cover this in detail.
- Obtaining approval for ethical/legal human rights procedures . As we will learn in an upcoming module, there needs to be methods in place to safeguard human rights.
- Data collection . The fun part! Finally, after everything has been organized and planned, the researcher(s) begin to collect data. The pre-established plan (methodology) determines when data collection begins, how to accomplish it, how data collection staff will be trained, and how data will be recorded.
- Data analysis . Here comes the statistical analyses. The next module will dive into this.
- Discussion . After all the analyses have been complete, the researcher then needs to interpret the results and examine the implications. Researchers attempt to explain the findings in light of the theoretical framework, prior evidence, theory, clinical experience, and any limitations in the study now that it has been completed. Often, the researcher discusses not just the statistical significance, but also the clinical significance, as it is common to have one without the other.
- Summary/references . Part of the final steps of any research project is to disseminate (AKA: share) the findings. This may be in a published article, conference, poster session, etc. The point of this step is to communicate to others the information found through the study. All references are collected so that the researchers can give credit to others.
- Budget and funding . As a last mention in the overall steps, budget and funding for research is a consideration. Research can be expensive. Often, researchers can obtain a grant or other funding to help offset the costs.
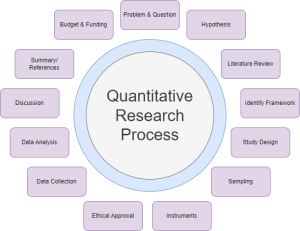
Edit: Steps in Quantitative Research video. Step 12 should say “Dissemination” (sharing the results).
Experimental, Quasi-Experimental, and Non-Experimental Studies
To start this section, please watch this wonderful video by Jenny Barrow, MSN, RN, CNE, that explains experimental versus nonexperimental research.
(Jenny Barrow, 2019)
Now that you have that overview, continue reading this module.
Experimental Research : In experimental research, the researcher is seeking to draw a conclusion between an independent variable and a dependent variable. This design attempts to establish cause-effect relationships among the variables. You could think of experimental research as experimenting with “something” to see if it caused “something else”.
A true experiment is called a Randomized Controlled Trial (or RCT). An RCT is at the top of the echelon as far as quantitative experimental research. It’s the gold standard of scientific research. An RCT, a true experimental design, must have 3 features:
- An intervention : The experiment does something to the participants by the option of manipulating the independent variable.
- Control : Some participants in the study receive either the standard care, or no intervention at all. This is also called the counterfactual – meaning, it shows what would happen if no intervention was introduced.
- Randomization : Randomization happens when the researcher makes sure that it is completely random who receives the intervention and who receives the control. The purpose is to make the groups equal regarding all other factors except receipt of the intervention.
Note: There is a lot of confusion with students (and even some researchers!) when they refer to “ random assignment ” versus “ random sampling ”. Random assignment is a signature of a true experiment. This means that if participants are not truly randomly assigned to intervention groups, then it is not a true experiment. We will talk more about random sampling in the next module.
One very common method for RCT’s is called a pretest-posttest design . This is when the researcher measures the outcome before and after the intervention. For example, if the researcher had an IV (intervention/treatment) of a pain medication, the DV (pain) would be measured before the intervention is given and after it is given. The control group may just receive a placebo. This design permits the researcher to see if the change in pain was caused by the pain medication because only some people received it (Polit & Beck, 2021).
Another experimental design is called a crossover design . This type of design involves exposing participants to more than one treatment. For example, subject 1 first receives treatment A, then treatment B, then treatment C. Subject 2 might first receive treatment B, then treatment A, and then treatment C. In this type of study, the three conditions for an experiment are met: Intervention, randomization, and control – with the subjects serving as their own control group.
Control group conditions can be done in 4 ways:
- No intervention is used; control group gets no treatment at all
- “Usual care” or standard of care or normal procedures used
- An alternative intervention is uses (e.g. auditory versus visual stimulation)
- A placebo or pseudo-intervention, presumed to have no therapeutic value, is used
Quasi-Experimental Research : Quasi-experiments involve an experiment just like true experimental research. However, they lack randomization and some even lack a control group. Therefore, there is implementation and testing of an intervention, but there is an absence of randomization.
For example, perhaps we wanted to measure the effect of yoga for nursing students. The IV (intervention of yoga) is being offered to all nursing students and therefore randomization is not possible. For comparison, we could measure quality of life data on nursing students at a different university. Data is collected from both groups at baseline and then again after the yoga classes. Note, that in quasi-experiments, the phrase “comparison group” is sometimes used instead of “control group” against which outcome measures are collected.
Sometimes there is no comparison group either. This would be called a one-group pretest-posttest design .
Non-Experimental Research : Sometimes, cause-problem research questions cannot be answered with an experimental or quasi-experimental design because the IV cannot be manipulated. For example, if we want to measure what impact prerequisite grades have on student success in nursing programs, we obviously cannot manipulate the prerequisite grades. In another example, if we wanted to investigate how low birth weight impacts developmental progression in children, we cannot manipulate the birth weight. Often, you will see the word “observational” in lieu of non-experimental researcher. This does not mean the researcher is just standing and watching people, but instead it refers to the method of observing data that has already been established without manipulation.
There are various types of non-experimental research:
Correlational research : A correlational research design investigates relationships between two variables (or more) without the researcher controlling or manipulating any of them. In the example of prerequisites and nursing program success, that is a correlational design. Consider hypothetically, a researcher is studying a correlation between cancer and marriage. In this study, there are two variables: disease and marriage. Let us say marriage has a negative association with cancer. This means that married people are less likely to develop cancer.
Cohort design (also called a prospective design) : In a cohort study, the participants do not have the outcome of interest to begin with. They are selected based on the exposure status of the individual. They are then followed over time to evaluate for the occurrence of the outcome of interest. Cohorts may be divided into exposure categories once baseline measurements of a defined population are made. For example, the Framingham Cardiovascular Disease Study (CVD) used baseline measurements to divide the population into categories of CVD risk factors. Another example: An example of a cohort study is comparing the test scores of one group of people who underwent extensive tutoring and a special curriculum and those who did not receive any extra help. The group could be studied for years to assess whether their scores improve over time and at what rate.
Retrospective design : In retrospective studies, the outcome of interest has already occurred (or not occurred – e.g., in controls) in each individual by the time s/he is enrolled, and the data are collected either from records or by asking participants to recall exposures. There is no follow-up of participants. For example, a researcher might examine the medical histories of 1000 elderly women to identify the causes of health problems.
Case-control design : A study that compares two groups of people: those with the disease or condition under study (cases) and a very similar group of people who do not have the condition. For example, investigators conducted a case-control study to determine if there is an association between colon cancer and a high fat diet. Cases were all confirmed colon cancer cases in North Carolina in 2010. Controls were a sample of North Carolina residents without colon cancer.
Descriptive research : Descriptive research design is a type of research design that aims to obtain information to systematically describe a phenomenon, situation, or population. More specifically, it helps answer the what, when, where, and how questions regarding the research problem, rather than the why. For example, the researcher might wish to discover the percentage of motorists who tailgate – the prevalence of a certain behavior.
There are two other designs to mention, which are both on a time continuum basis.
Cross-sectional design : All data are collected at a single point in time. Retrospective studies are usually cross-sectional. The IV usually concerns events or behaviors occurring in the past. One cross-sectional study example in medicine is a data collection of smoking habits and lung cancer incidence in a given population. A cross-sectional study like this cannot solely determine that smoking habits cause lung cancer, but it can suggest a relationship that merits further investigation. Cross-sectional studies serve many purposes, and the cross-sectional design is the most relevant design when assessing the prevalence of disease, attitudes and knowledge among patients and health personnel, in validation studies comparing, for example, different measurement instruments, and in reliability studies.
Longitudinal design : Data are collected two or more times over an extended period. Longitudinal designs are better at showing patterns of change and at clarifying whether a cause occurred before an effect (outcome). A challenge in longitudinal studies is attrition or the loss of participants over time. In a longitudinal study subjects are followed over time with continuous or repeated monitoring of risk factors or health outcomes, or both. Such investigations vary enormously in their size and complexity. At one extreme a large population may be studied over decades. An example of a longitudinal design is a multiyear comparative study of the same children in an urban and a suburban school to record their cognitive development in depth.
Confounding and Extraneous Variables
Confounding variables are a type of extraneous variable that occur which interfere with or influence the relationship between the independent and dependent variables. In research that investigates a potential cause-and-effect relationship, a confounding variable is an unmeasured third variable that influences both the supposed cause and the supposed effect.
It’s important to consider potential confounding variables and account for them in research designs to ensure results are valid. You can imagine that if something sneaks in to influence the measured variables, it can really muck up the study!
Here is an example:
You collect data on sunburns and ice cream consumption. You find that higher ice cream consumption is associated with a higher probability of sunburn. Does that mean ice cream consumption causes sunburn?
Here, the confounding variable is temperature: hot temperatures cause people to both eat more ice cream and spend more time outdoors under the sun, resulting in more sunburns.
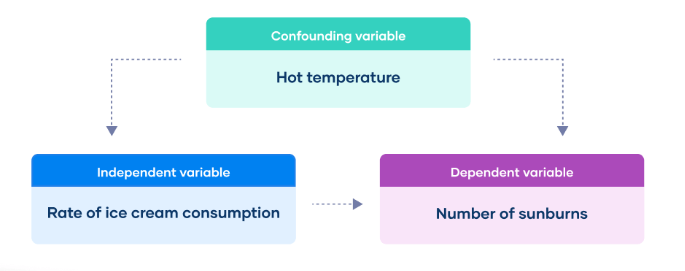
To ensure the internal validity of research, the researcher must account for confounding variables. If he/she fails to do so, the results may not reflect the actual relationship between the variables that they are interested in.
For instance, they may find a cause-and-effect relationship that does not actually exist, because the effect they measure is caused by the confounding variable (and not by the independent variable).
Here is another example:
The researcher finds that babies born to mothers who smoked during their pregnancies weigh significantly less than those born to non-smoking mothers. However, if the researcher does not account for the fact that smokers are more likely to engage in other unhealthy behaviors, such as drinking or eating less healthy foods, then he/she might overestimate the relationship between smoking and low birth weight.
Extraneous variables are any variables that the researcher is not investigating that can potentially affect the outcomes of the research study. If left uncontrolled, extraneous variables can lead to inaccurate conclusions about the relationship between IVs and DVs.
Extraneous variables can threaten the internal validity of a study by providing alternative explanations for the results. In an experiment, the researcher manipulates an independent variable to study its effects on a dependent variable.
In a study on mental performance, the researcher tests whether wearing a white lab coat, the independent variable (IV), improves scientific reasoning, the dependent variable (DV).
Students from a university are recruited to participate in the study. The researcher manipulates the independent variable by splitting participants into two groups:
- Participants in the experimental group are asked to wear a lab coat during the study.
- Participants in the control group are asked to wear a casual coat during the study.
All participants are given a scientific knowledge quiz, and their scores are compared between groups.
When extraneous variables are uncontrolled, it’s hard to determine the exact effects of the independent variable on the dependent variable, because the effects of extraneous variables may mask them.
Uncontrolled extraneous variables can also make it seem as though there is a true effect of the independent variable in an experiment when there’s actually none.
In the above experiment example, these extraneous variables can affect the science knowledge scores:
- Participant’s major (e.g., STEM or humanities)
- Participant’s interest in science
- Demographic variables such as gender or educational background
- Time of day of testing
- Experiment environment or setting
If these variables systematically differ between the groups, you can’t be sure whether your results come from your independent variable manipulation or from the extraneous variables.
In summary, an extraneous variable is anything that could influence the dependent variable. A confounding variable influences the dependent variable, and also correlates with or causally affects the independent variable.
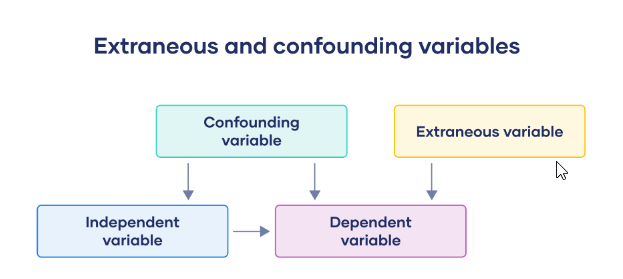
Cause-and-Effect (Causality) Versus Association/Correlation
A very important concept to understand is cause-and-effect, also known as causality, versus correlation. Let’s look at these two concepts in very simplified statements. Causation means that one thing caused another thing to happen. Correlation means there is some association between the two thing we are measuring.
It would be nice if it were as simple as that. These two concepts can indeed by confused by many. Let’s dive deeper.
Two or more variables are considered to be related or associated, in a statistical context, if their values change so that as the value of one variable increases or decreases so does the value of the other variable (or the opposite direction).
For example, for the two variables of “hours worked” and “income earned”, there is a relationship between the two if the increase in hours is associated with an increase in income earned.
However, correlation is a statistical measure that describes the size and direction of a relationship between two or more variables. A correlation does not automatically mean that the change in one variable caused the change in value in the other variable.
Theoretically, the difference between the two types of relationships is easy to identify — an action or occurrence can cause another (e.g. smoking causes an increase in the risk of developing lung cancer), or it can correlate with another (e.g. smoking is correlated with alcoholism, but it does not cause alcoholism). In practice, however, it remains difficult to clearly establish cause and effect, compared with establishing correlation.
Simplified in this image, we can say that hot and sunny weather causes an increase in ice cream consumption. Similarly, we can demise that hot and sunny weather increases the incidence of sunburns. However, we cannot say that ice cream caused a sunburn (or that a sunburn increases consumption of ice cream). It is purely coincidental. In this example, it is pretty easy to anecdotally surmise correlation versus causation. However, in research, we have statistical tests that help researchers differentiate via specialized analyses.
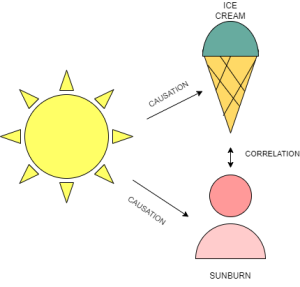
Here is a great Khan Academy video of about 5 minutes that shows a worked example of correlation versus causation with regard to sledding accidents and frostbite cases:
https://www.khanacademy.org/test-prep/praxis-math/praxis-math-lessons/gtp–praxis-math–lessons–statistics-and-probability/v/gtp–praxis-math–video–correlation-and-causation
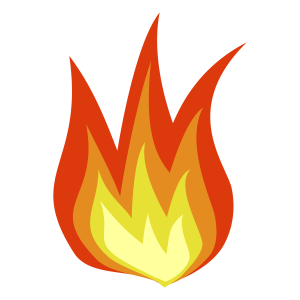
References & Attribution
“ Light bulb doodle ” by rawpixel licensed CC0 .
“ Magnifying glass ” by rawpixel licensed CC0
“ Orange flame ” by rawpixel licensed CC0 .
Jenny Barrow. (2019). Experimental versus nonexperimental research. https://www.youtube.com/watch?v=FJo8xyXHAlE
Leibold, N. (2020). Research variables. Measures and Concepts Commonly Encountered in EBP. Creative Commons License: BY NC
Polit, D. & Beck, C. (2021). Lippincott CoursePoint Enhanced for Polit’s Essentials of Nursing Research (10th ed.). Wolters Kluwer Health.
Evidence-Based Practice & Research Methodologies Copyright © by Tracy Fawns is licensed under a Creative Commons Attribution-NonCommercial-ShareAlike 4.0 International License , except where otherwise noted.
Share This Book

An official website of the United States government
The .gov means it’s official. Federal government websites often end in .gov or .mil. Before sharing sensitive information, make sure you’re on a federal government site.
The site is secure. The https:// ensures that you are connecting to the official website and that any information you provide is encrypted and transmitted securely.
- Publications
- Account settings
Preview improvements coming to the PMC website in October 2024. Learn More or Try it out now .
- Advanced Search
- Journal List
- Front Psychol
Quantitative and Qualitative Approaches to Generalization and Replication–A Representationalist View
In this paper, we provide a re-interpretation of qualitative and quantitative modeling from a representationalist perspective. In this view, both approaches attempt to construct abstract representations of empirical relational structures. Whereas quantitative research uses variable-based models that abstract from individual cases, qualitative research favors case-based models that abstract from individual characteristics. Variable-based models are usually stated in the form of quantified sentences (scientific laws). This syntactic structure implies that sentences about individual cases are derived using deductive reasoning. In contrast, case-based models are usually stated using context-dependent existential sentences (qualitative statements). This syntactic structure implies that sentences about other cases are justifiable by inductive reasoning. We apply this representationalist perspective to the problems of generalization and replication. Using the analytical framework of modal logic, we argue that the modes of reasoning are often not only applied to the context that has been studied empirically, but also on a between-contexts level. Consequently, quantitative researchers mostly adhere to a top-down strategy of generalization, whereas qualitative researchers usually follow a bottom-up strategy of generalization. Depending on which strategy is employed, the role of replication attempts is very different. In deductive reasoning, replication attempts serve as empirical tests of the underlying theory. Therefore, failed replications imply a faulty theory. From an inductive perspective, however, replication attempts serve to explore the scope of the theory. Consequently, failed replications do not question the theory per se , but help to shape its boundary conditions. We conclude that quantitative research may benefit from a bottom-up generalization strategy as it is employed in most qualitative research programs. Inductive reasoning forces us to think about the boundary conditions of our theories and provides a framework for generalization beyond statistical testing. In this perspective, failed replications are just as informative as successful replications, because they help to explore the scope of our theories.
Introduction
Qualitative and quantitative research strategies have long been treated as opposing paradigms. In recent years, there have been attempts to integrate both strategies. These “mixed methods” approaches treat qualitative and quantitative methodologies as complementary, rather than opposing, strategies (Creswell, 2015 ). However, whilst acknowledging that both strategies have their benefits, this “integration” remains purely pragmatic. Hence, mixed methods methodology does not provide a conceptual unification of the two approaches.
Lacking a common methodological background, qualitative and quantitative research methodologies have developed rather distinct standards with regard to the aims and scope of empirical science (Freeman et al., 2007 ). These different standards affect the way researchers handle contradictory empirical findings. For example, many empirical findings in psychology have failed to replicate in recent years (Klein et al., 2014 ; Open Science, Collaboration, 2015 ). This “replication crisis” has been discussed on statistical, theoretical and social grounds and continues to have a wide impact on quantitative research practices like, for example, open science initiatives, pre-registered studies and a re-evaluation of statistical significance testing (Everett and Earp, 2015 ; Maxwell et al., 2015 ; Shrout and Rodgers, 2018 ; Trafimow, 2018 ; Wiggins and Chrisopherson, 2019 ).
However, qualitative research seems to be hardly affected by this discussion. In this paper, we argue that the latter is a direct consequence of how the concept of generalizability is conceived in the two approaches. Whereas most of quantitative psychology is committed to a top-down strategy of generalization based on the idea of random sampling from an abstract population, qualitative studies usually rely on a bottom-up strategy of generalization that is grounded in the successive exploration of the field by means of theoretically sampled cases.
Here, we show that a common methodological framework for qualitative and quantitative research methodologies is possible. We accomplish this by introducing a formal description of quantitative and qualitative models from a representationalist perspective: both approaches can be reconstructed as special kinds of representations for empirical relational structures. We then use this framework to analyze the generalization strategies used in the two approaches. These turn out to be logically independent of the type of model. This has wide implications for psychological research. First, a top-down generalization strategy is compatible with a qualitative modeling approach. This implies that mainstream psychology may benefit from qualitative methods when a numerical representation turns out to be difficult or impossible, without the need to commit to a “qualitative” philosophy of science. Second, quantitative research may exploit the bottom-up generalization strategy that is inherent to many qualitative approaches. This offers a new perspective on unsuccessful replications by treating them not as scientific failures, but as a valuable source of information about the scope of a theory.
The Quantitative Strategy–Numbers and Functions
Quantitative science is about finding valid mathematical representations for empirical phenomena. In most cases, these mathematical representations have the form of functional relations between a set of variables. One major challenge of quantitative modeling consists in constructing valid measures for these variables. Formally, to measure a variable means to construct a numerical representation of the underlying empirical relational structure (Krantz et al., 1971 ). For example, take the behaviors of a group of students in a classroom: “to listen,” “to take notes,” and “to ask critical questions.” One may now ask whether is possible to assign numbers to the students, such that the relations between the assigned numbers are of the same kind as the relations between the values of an underlying variable, like e.g., “engagement.” The observed behaviors in the classroom constitute an empirical relational structure, in the sense that for every student-behavior tuple, one can observe whether it is true or not. These observations can be represented in a person × behavior matrix 1 (compare Figure 1 ). Given this relational structure satisfies certain conditions (i.e., the axioms of a measurement model), one can assign numbers to the students and the behaviors, such that the relations between the numbers resemble the corresponding numerical relations. For example, if there is a unique ordering in the empirical observations with regard to which person shows which behavior, the assigned numbers have to constitute a corresponding unique ordering, as well. Such an ordering coincides with the person × behavior matrix forming a triangle shaped relation and is formally represented by a Guttman scale (Guttman, 1944 ). There are various measurement models available for different empirical structures (Suppes et al., 1971 ). In the case of probabilistic relations, Item-Response models may be considered as a special kind of measurement model (Borsboom, 2005 ).

Constructing a numerical representation from an empirical relational structure; Due to the unique ordering of persons with regard to behaviors (indicated by the triangular shape of the relation), it is possible to construct a Guttman scale by assigning a number to each of the individuals, representing the number of relevant behaviors shown by the individual. The resulting variable (“engagement”) can then be described by means of statistical analyses, like, e.g., plotting the frequency distribution.
Although essential, measurement is only the first step of quantitative modeling. Consider a slightly richer empirical structure, where we observe three additional behaviors: “to doodle,” “to chat,” and “to play.” Like above, one may ask, whether there is a unique ordering of the students with regard to these behaviors that can be represented by an underlying variable (i.e., whether the matrix forms a Guttman scale). If this is the case, we may assign corresponding numbers to the students and call this variable “distraction.” In our example, such a representation is possible. We can thus assign two numbers to each student, one representing his or her “engagement” and one representing his or her “distraction” (compare Figure 2 ). These measurements can now be used to construct a quantitative model by relating the two variables by a mathematical function. In the simplest case, this may be a linear function. This functional relation constitutes a quantitative model of the empirical relational structure under study (like, e.g., linear regression). Given the model equation and the rules for assigning the numbers (i.e., the instrumentations of the two variables), the set of admissible empirical structures is limited from all possible structures to a rather small subset. This constitutes the empirical content of the model 2 (Popper, 1935 ).

Constructing a numerical model from an empirical relational structure; Since there are two distinct classes of behaviors that each form a Guttman scale, it is possible to assign two numbers to each individual, correspondingly. The resulting variables (“engagement” and “distraction”) can then be related by a mathematical function, which is indicated by the scatterplot and red line on the right hand side.
The Qualitative Strategy–Categories and Typologies
The predominant type of analysis in qualitative research consists in category formation. By constructing descriptive systems for empirical phenomena, it is possible to analyze the underlying empirical structure at a higher level of abstraction. The resulting categories (or types) constitute a conceptual frame for the interpretation of the observations. Qualitative researchers differ considerably in the way they collect and analyze data (Miles et al., 2014 ). However, despite the diverse research strategies followed by different qualitative methodologies, from a formal perspective, most approaches build on some kind of categorization of cases that share some common features. The process of category formation is essential in many qualitative methodologies, like, for example, qualitative content analysis, thematic analysis, grounded theory (see Flick, 2014 for an overview). Sometimes these features are directly observable (like in our classroom example), sometimes they are themselves the result of an interpretative process (e.g., Scheunpflug et al., 2016 ).
In contrast to quantitative methodologies, there have been little attempts to formalize qualitative research strategies (compare, however, Rihoux and Ragin, 2009 ). However, there are several statistical approaches to non-numerical data that deal with constructing abstract categories and establishing relations between these categories (Agresti, 2013 ). Some of these methods are very similar to qualitative category formation on a conceptual level. For example, cluster analysis groups cases into homogenous categories (clusters) based on their similarity on a distance metric.
Although category formation can be formalized in a mathematically rigorous way (Ganter and Wille, 1999 ), qualitative research hardly acknowledges these approaches. 3 However, in order to find a common ground with quantitative science, it is certainly helpful to provide a formal interpretation of category systems.
Let us reconsider the above example of students in a classroom. The quantitative strategy was to assign numbers to the students with regard to variables and to relate these variables via a mathematical function. We can analyze the same empirical structure by grouping the behaviors to form abstract categories. If the aim is to construct an empirically valid category system, this grouping is subject to constraints, analogous to those used to specify a measurement model. The first and most important constraint is that the behaviors must form equivalence classes, i.e., within categories, behaviors need to be equivalent, and across categories, they need to be distinct (formally, the relational structure must obey the axioms of an equivalence relation). When objects are grouped into equivalence classes, it is essential to specify the criterion for empirical equivalence. In qualitative methodology, this is sometimes referred to as the tertium comparationis (Flick, 2014 ). One possible criterion is to group behaviors such that they constitute a set of specific common attributes of a group of people. In our example, we might group the behaviors “to listen,” “to take notes,” and “to doodle,” because these behaviors are common to the cases B, C, and D, and they are also specific for these cases, because no other person shows this particular combination of behaviors. The set of common behaviors then forms an abstract concept (e.g., “moderate distraction”), while the set of persons that show this configuration form a type (e.g., “the silent dreamer”). Formally, this means to identify the maximal rectangles in the underlying empirical relational structure (see Figure 3 ). This procedure is very similar to the way we constructed a Guttman scale, the only difference being that we now use different aspects of the empirical relational structure. 4 In fact, the set of maximal rectangles can be determined by an automated algorithm (Ganter, 2010 ), just like the dimensionality of an empirical structure can be explored by psychometric scaling methods. Consequently, we can identify the empirical content of a category system or a typology as the set of empirical structures that conforms to it. 5 Whereas the quantitative strategy was to search for scalable sub-matrices and then relate the constructed variables by a mathematical function, the qualitative strategy is to construct an empirical typology by grouping cases based on their specific similarities. These types can then be related to one another by a conceptual model that describes their semantic and empirical overlap (see Figure 3 , right hand side).

Constructing a conceptual model from an empirical relational structure; Individual behaviors are grouped to form abstract types based on them being shared among a specific subset of the cases. Each type constitutes a set of specific commonalities of a class of individuals (this is indicated by the rectangles on the left hand side). The resulting types (“active learner,” “silent dreamer,” “distracted listener,” and “troublemaker”) can then be related to one another to explicate their semantic and empirical overlap, as indicated by the Venn-diagram on the right hand side.
Variable-Based Models and Case-Based Models
In the previous section, we have argued that qualitative category formation and quantitative measurement can both be characterized as methods to construct abstract representations of empirical relational structures. Instead of focusing on different philosophical approaches to empirical science, we tried to stress the formal similarities between both approaches. However, it is worth also exploring the dissimilarities from a formal perspective.
Following the above analysis, the quantitative approach can be characterized by the use of variable-based models, whereas the qualitative approach is characterized by case-based models (Ragin, 1987 ). Formally, we can identify the rows of an empirical person × behavior matrix with a person-space, and the columns with a corresponding behavior-space. A variable-based model abstracts from the single individuals in a person-space to describe the structure of behaviors on a population level. A case-based model, on the contrary, abstracts from the single behaviors in a behavior-space to describe individual case configurations on the level of abstract categories (see Table 1 ).
Variable-based models and case-based models.
From a representational perspective, there is no a priori reason to favor one type of model over the other. Both approaches provide different analytical tools to construct an abstract representation of an empirical relational structure. However, since the two modeling approaches make use of different information (person-space vs. behavior-space), this comes with some important implications for the researcher employing one of the two strategies. These are concerned with the role of deductive and inductive reasoning.
In variable-based models, empirical structures are represented by functional relations between variables. These are usually stated as scientific laws (Carnap, 1928 ). Formally, these laws correspond to logical expressions of the form
In plain text, this means that y is a function of x for all objects i in the relational structure under consideration. For example, in the above example, one may formulate the following law: for all students in the classroom it holds that “distraction” is a monotone decreasing function of “engagement.” Such a law can be used to derive predictions for single individuals by means of logical deduction: if the above law applies to all students in the classroom, it is possible to calculate the expected distraction from a student's engagement. An empirical observation can now be evaluated against this prediction. If the prediction turns out to be false, the law can be refuted based on the principle of falsification (Popper, 1935 ). If a scientific law repeatedly withstands such empirical tests, it may be considered to be valid with regard to the relational structure under consideration.
In case-based models, there are no laws about a population, because the model does not abstract from the cases but from the observed behaviors. A case-based model describes the underlying structure in terms of existential sentences. Formally, this corresponds to a logical expression of the form
In plain text, this means that there is at least one case i for which the condition XYZ holds. For example, the above category system implies that there is at least one active learner. This is a statement about a singular observation. It is impossible to deduce a statement about another person from an existential sentence like this. Therefore, the strategy of falsification cannot be applied to test the model's validity in a specific context. If one wishes to generalize to other cases, this is accomplished by inductive reasoning, instead. If we observed one person that fulfills the criteria of calling him or her an active learner, we can hypothesize that there may be other persons that are identical to the observed case in this respect. However, we do not arrive at this conclusion by logical deduction, but by induction.
Despite this important distinction, it would be wrong to conclude that variable-based models are intrinsically deductive and case-based models are intrinsically inductive. 6 Both types of reasoning apply to both types of models, but on different levels. Based on a person-space, in a variable-based model one can use deduction to derive statements about individual persons from abstract population laws. There is an analogous way of reasoning for case-based models: because they are based on a behavior space, it is possible to deduce statements about singular behaviors. For example, if we know that Peter is an active learner, we can deduce that he takes notes in the classroom. This kind of deductive reasoning can also be applied on a higher level of abstraction to deduce thematic categories from theoretical assumptions (Braun and Clarke, 2006 ). Similarly, there is an analog for inductive generalization from the perspective of variable-based modeling: since the laws are only quantified over the person-space, generalizations to other behaviors rely on inductive reasoning. For example, it is plausible to assume that highly engaged students tend to do their homework properly–however, in our example this behavior has never been observed. Hence, in variable-based models we usually generalize to other behaviors by means of induction. This kind of inductive reasoning is very common when empirical results are generalized from the laboratory to other behavioral domains.
Although inductive and deductive reasoning are used in qualitative and quantitative research, it is important to stress the different roles of induction and deduction when models are applied to cases. A variable-based approach implies to draw conclusions about cases by means of logical deduction; a case-based approach implies to draw conclusions about cases by means of inductive reasoning. In the following, we build on this distinction to differentiate between qualitative (bottom-up) and quantitative (top-down) strategies of generalization.
Generalization and the Problem of Replication
We will now extend the formal analysis of quantitative and qualitative approaches to the question of generalization and replicability of empirical findings. For this sake, we have to introduce some concepts of formal logic. Formal logic is concerned with the validity of arguments. It provides conditions to evaluate whether certain sentences (conclusions) can be derived from other sentences (premises). In this context, a theory is nothing but a set of sentences (also called axioms). Formal logic provides tools to derive new sentences that must be true, given the axioms are true (Smith, 2020 ). These derived sentences are called theorems or, in the context of empirical science, predictions or hypotheses . On the syntactic level, the rules of logic only state how to evaluate the truth of a sentence relative to its premises. Whether or not sentences are actually true, is formally specified by logical semantics.
On the semantic level, formal logic is intrinsically linked to set-theory. For example, a logical statement like “all dogs are mammals,” is true if and only if the set of dogs is a subset of the set of mammals. Similarly, the sentence “all chatting students doodle” is true if and only if the set of chatting students is a subset of the set of doodling students (compare Figure 3 ). Whereas, the first sentence is analytically true due to the way we define the words “dog” and “mammal,” the latter can be either true or false, depending on the relational structure we actually observe. We can thus interpret an empirical relational structure as the truth criterion of a scientific theory. From a logical point of view, this corresponds to the semantics of a theory. As shown above, variable-based and case-based models both give a formal representation of the same kinds of empirical structures. Accordingly, both types of models can be stated as formal theories. In the variable-based approach, this corresponds to a set of scientific laws that are quantified over the members of an abstract population (these are the axioms of the theory). In the case-based approach, this corresponds to a set of abstract existential statements about a specific class of individuals.
In contrast to mathematical axiom systems, empirical theories are usually not considered to be necessarily true. This means that even if we find no evidence against a theory, it is still possible that it is actually wrong. We may know that a theory is valid in some contexts, yet it may fail when applied to a new set of behaviors (e.g., if we use a different instrumentation to measure a variable) or a new population (e.g., if we draw a new sample).
From a logical perspective, the possibility that a theory may turn out to be false stems from the problem of contingency . A statement is contingent, if it is both, possibly true and possibly false. Formally, we introduce two modal operators: □ to designate logical necessity, and ◇ to designate logical possibility. Semantically, these operators are very similar to the existential quantifier, ∃, and the universal quantifier, ∀. Whereas ∃ and ∀ refer to the individual objects within one relational structure, the modal operators □ and ◇ range over so-called possible worlds : a statement is possibly true, if and only if it is true in at least one accessible possible world, and a statement is necessarily true if and only if it is true in every accessible possible world (Hughes and Cresswell, 1996 ). Logically, possible worlds are mathematical abstractions, each consisting of a relational structure. Taken together, the relational structures of all accessible possible worlds constitute the formal semantics of necessity, possibility and contingency. 7
In the context of an empirical theory, each possible world may be identified with an empirical relational structure like the above classroom example. Given the set of intended applications of a theory (the scope of the theory, one may say), we can now construct possible world semantics for an empirical theory: each intended application of the theory corresponds to a possible world. For example, a quantified sentence like “all chatting students doodle” may be true in one classroom and false in another one. In terms of possible worlds, this would correspond to a statement of contingency: “it is possible that all chatting students doodle in one classroom, and it is possible that they don't in another classroom.” Note that in the above expression, “all students” refers to the students in only one possible world, whereas “it is possible” refers to the fact that there is at least one possible world for each of the specified cases.
To apply these possible world semantics to quantitative research, let us reconsider how generalization to other cases works in variable-based models. Due to the syntactic structure of quantitative laws, we can deduce predictions for singular observations from an expression of the form ∀ i : y i = f ( x i ). Formally, the logical quantifier ∀ ranges only over the objects of the corresponding empirical relational structure (in our example this would refer to the students in the observed classroom). But what if we want to generalize beyond the empirical structure we actually observed? The standard procedure is to assume an infinitely large, abstract population from which a random sample is drawn. Given the truth of the theory, we can deduce predictions about what we may observe in the sample. Since usually we deal with probabilistic models, we can evaluate our theory by means of the conditional probability of the observations, given the theory holds. This concept of conditional probability is the foundation of statistical significance tests (Hogg et al., 2013 ), as well as Bayesian estimation (Watanabe, 2018 ). In terms of possible world semantics, the random sampling model implies that all possible worlds (i.e., all intended applications) can be conceived as empirical sub-structures from a greater population structure. For example, the empirical relational structure constituted by the observed behaviors in a classroom would be conceived as a sub-matrix of the population person × behavior matrix. It follows that, if a scientific law is true in the population, it will be true in all possible worlds, i.e., it will be necessarily true. Formally, this corresponds to an expression of the form
The statistical generalization model thus constitutes a top-down strategy for dealing with individual contexts that is analogous to the way variable-based models are applied to individual cases (compare Table 1 ). Consequently, if we apply a variable-based model to a new context and find out that it does not fit the data (i.e., there is a statistically significant deviation from the model predictions), we have reason to doubt the validity of the theory. This is what makes the problem of low replicability so important: we observe that the predictions are wrong in a new study; and because we apply a top-down strategy of generalization to contexts beyond the ones we observed, we see our whole theory at stake.
Qualitative research, on the contrary, follows a different strategy of generalization. Since case-based models are formulated by a set of context-specific existential sentences, there is no need for universal truth or necessity. In contrast to statistical generalization to other cases by means of random sampling from an abstract population, the usual strategy in case-based modeling is to employ a bottom-up strategy of generalization that is analogous to the way case-based models are applied to individual cases. Formally, this may be expressed by stating that the observed qualia exist in at least one possible world, i.e., the theory is possibly true:
This statement is analogous to the way we apply case-based models to individual cases (compare Table 1 ). Consequently, the set of intended applications of the theory does not follow from a sampling model, but from theoretical assumptions about which cases may be similar to the observed cases with respect to certain relevant characteristics. For example, if we observe that certain behaviors occur together in one classroom, following a bottom-up strategy of generalization, we will hypothesize why this might be the case. If we do not replicate this finding in another context, this does not question the model itself, since it was a context-specific theory all along. Instead, we will revise our hypothetical assumptions about why the new context is apparently less similar to the first one than we originally thought. Therefore, if an empirical finding does not replicate, we are more concerned about our understanding of the cases than about the validity of our theory.
Whereas statistical generalization provides us with a formal (and thus somehow more objective) apparatus to evaluate the universal validity of our theories, the bottom-up strategy forces us to think about the class of intended applications on theoretical grounds. This means that we have to ask: what are the boundary conditions of our theory? In the above classroom example, following a bottom-up strategy, we would build on our preliminary understanding of the cases in one context (e.g., a public school) to search for similar and contrasting cases in other contexts (e.g., a private school). We would then re-evaluate our theoretical description of the data and explore what makes cases similar or dissimilar with regard to our theory. This enables us to expand the class of intended applications alongside with the theory.
Of course, none of these strategies is superior per se . Nevertheless, they rely on different assumptions and may thus be more or less adequate in different contexts. The statistical strategy relies on the assumption of a universal population and invariant measurements. This means, we assume that (a) all samples are drawn from the same population and (b) all variables refer to the same behavioral classes. If these assumptions are true, statistical generalization is valid and therefore provides a valuable tool for the testing of empirical theories. The bottom-up strategy of generalization relies on the idea that contexts may be classified as being more or less similar based on characteristics that are not part of the model being evaluated. If such a similarity relation across contexts is feasible, the bottom-up strategy is valid, as well. Depending on the strategy of generalization, replication of empirical research serves two very different purposes. Following the (top-down) principle of generalization by deduction from scientific laws, replications are empirical tests of the theory itself, and failed replications question the theory on a fundamental level. Following the (bottom-up) principle of generalization by induction to similar contexts, replications are a means to explore the boundary conditions of a theory. Consequently, failed replications question the scope of the theory and help to shape the set of intended applications.
We have argued that quantitative and qualitative research are best understood by means of the structure of the employed models. Quantitative science mainly relies on variable-based models and usually employs a top-down strategy of generalization from an abstract population to individual cases. Qualitative science prefers case-based models and usually employs a bottom-up strategy of generalization. We further showed that failed replications have very different implications depending on the underlying strategy of generalization. Whereas in the top-down strategy, replications are used to test the universal validity of a model, in the bottom-up strategy, replications are used to explore the scope of a model. We will now address the implications of this analysis for psychological research with regard to the problem of replicability.
Modern day psychology almost exclusively follows a top-down strategy of generalization. Given the quantitative background of most psychological theories, this is hardly surprising. Following the general structure of variable-based models, the individual case is not the focus of the analysis. Instead, scientific laws are stated on the level of an abstract population. Therefore, when applying the theory to a new context, a statistical sampling model seems to be the natural consequence. However, this is not the only possible strategy. From a logical point of view, there is no reason to assume that a quantitative law like ∀ i : y i = f ( x i ) implies that the law is necessarily true, i.e.,: □(∀ i : y i = f ( x i )). Instead, one might just as well define the scope of the theory following an inductive strategy. 8 Formally, this would correspond to the assumption that the observed law is possibly true, i.e.,: ◇(∀ i : y i = f ( x i )). For example, we may discover a functional relation between “engagement” and “distraction” without referring to an abstract universal population of students. Instead, we may hypothesize under which conditions this functional relation may be valid and use these assumptions to inductively generalize to other cases.
If we take this seriously, this would require us to specify the intended applications of the theory: in which contexts do we expect the theory to hold? Or, equivalently, what are the boundary conditions of the theory? These boundary conditions may be specified either intensionally, i.e., by giving external criteria for contexts being similar enough to the ones already studied to expect a successful application of the theory. Or they may be specified extensionally, by enumerating the contexts where the theory has already been shown to be valid. These boundary conditions need not be restricted to the population we refer to, but include all kinds of contextual factors. Therefore, adopting a bottom-up strategy, we are forced to think about these factors and make them an integral part of our theories.
In fact, there is good reason to believe that bottom-up generalization may be more adequate in many psychological studies. Apart from the pitfalls associated with statistical generalization that have been extensively discussed in recent years (e.g., p-hacking, underpowered studies, publication bias), it is worth reflecting on whether the underlying assumptions are met in a particular context. For example, many samples used in experimental psychology are not randomly drawn from a large population, but are convenience samples. If we use statistical models with non-random samples, we have to assume that the observations vary as if drawn from a random sample. This may indeed be the case for randomized experiments, because all variation between the experimental conditions apart from the independent variable will be random due to the randomization procedure. In this case, a classical significance test may be regarded as an approximation to a randomization test (Edgington and Onghena, 2007 ). However, if we interpret a significance test as an approximate randomization test, we test not for generalization but for internal validity. Hence, even if we use statistical significance tests when assumptions about random sampling are violated, we still have to use a different strategy of generalization. This issue has been discussed in the context of small-N studies, where variable-based models are applied to very small samples, sometimes consisting of only one individual (Dugard et al., 2012 ). The bottom-up strategy of generalization that is employed by qualitative researchers, provides such an alternative.
Another important issue in this context is the question of measurement invariance. If we construct a variable-based model in one context, the variables refer to those behaviors that constitute the underlying empirical relational structure. For example, we may construct an abstract measure of “distraction” using the observed behaviors in a certain context. We will then use the term “distraction” as a theoretical term referring to the variable we have just constructed to represent the underlying empirical relational structure. Let us now imagine we apply this theory to a new context. Even if the individuals in our new context are part of the same population, we may still get into trouble if the observed behaviors differ from those used in the original study. How do we know whether these behaviors constitute the same variable? We have to ensure that in any new context, our measures are valid for the variables in our theory. Without a proper measurement model, this will be hard to achieve (Buntins et al., 2017 ). Again, we are faced with the necessity to think of the boundary conditions of our theories. In which contexts (i.e., for which sets of individuals and behaviors) do we expect our theory to work?
If we follow the rationale of inductive generalization, we can explore the boundary conditions of a theory with every new empirical study. We thus widen the scope of our theory by comparing successful applications in different contexts and unsuccessful applications in similar contexts. This may ultimately lead to a more general theory, maybe even one of universal scope. However, unless we have such a general theory, we might be better off, if we treat unsuccessful replications not as a sign of failure, but as a chance to learn.
Author Contributions
MB conceived the original idea and wrote the first draft of the paper. MS helped to further elaborate and scrutinize the arguments. All authors contributed to the final version of the manuscript.
Conflict of Interest
The authors declare that the research was conducted in the absence of any commercial or financial relationships that could be construed as a potential conflict of interest.
Acknowledgments
We would like to thank Annette Scheunpflug for helpful comments on an earlier version of the manuscript.
1 A person × behavior matrix constitutes a very simple relational structure that is common in psychological research. This is why it is chosen here as a minimal example. However, more complex structures are possible, e.g., by relating individuals to behaviors over time, with individuals nested within groups etc. For a systematic overview, compare Coombs ( 1964 ).
2 This notion of empirical content applies only to deterministic models. The empirical content of a probabilistic model consists in the probability distribution over all possible empirical structures.
3 For example, neither the SAGE Handbook of qualitative data analysis edited by Flick ( 2014 ) nor the Oxford Handbook of Qualitative Research edited by Leavy ( 2014 ) mention formal approaches to category formation.
4 Note also that the described structure is empirically richer than a nominal scale. Therefore, a reduction of qualitative category formation to be a special (and somehow trivial) kind of measurement is not adequate.
5 It is possible to extend this notion of empirical content to the probabilistic case (this would correspond to applying a latent class analysis). But, since qualitative research usually does not rely on formal algorithms (neither deterministic nor probabilistic), there is currently little practical use of such a concept.
6 We do not elaborate on abductive reasoning here, since, given an empirical relational structure, the concept can be applied to both types of models in the same way (Schurz, 2008 ). One could argue that the underlying relational structure is not given a priori but has to be constructed by the researcher and will itself be influenced by theoretical expectations. Therefore, abductive reasoning may be necessary to establish an empirical relational structure in the first place.
7 We shall not elaborate on the metaphysical meaning of possible worlds here, since we are only concerned with empirical theories [but see Tooley ( 1999 ), for an overview].
8 Of course, this also means that it would be equally reasonable to employ a top-down strategy of generalization using a case-based model by postulating that □(∃ i : XYZ i ). The implications for case-based models are certainly worth exploring, but lie beyond the scope of this article.
- Agresti A. (2013). Categorical Data Analysis, 3rd Edn. Wiley Series In Probability And Statistics . Hoboken, NJ: Wiley. [ Google Scholar ]
- Borsboom D. (2005). Measuring the Mind: Conceptual Issues in Contemporary Psychometrics . Cambridge: Cambridge University Press; 10.1017/CBO9780511490026 [ CrossRef ] [ Google Scholar ]
- Braun V., Clarke V. (2006). Using thematic analysis in psychology . Qual. Res. Psychol . 3 , 77–101. 10.1191/1478088706qp063oa [ CrossRef ] [ Google Scholar ]
- Buntins M., Buntins K., Eggert F. (2017). Clarifying the concept of validity: from measurement to everyday language . Theory Psychol. 27 , 703–710. 10.1177/0959354317702256 [ CrossRef ] [ Google Scholar ]
- Carnap R. (1928). The Logical Structure of the World . Berkeley, CA: University of California Press. [ Google Scholar ]
- Coombs C. H. (1964). A Theory of Data . New York, NY: Wiley. [ Google Scholar ]
- Creswell J. W. (2015). A Concise Introduction to Mixed Methods Research . Los Angeles, CA: Sage. [ Google Scholar ]
- Dugard P., File P., Todman J. B. (2012). Single-Case and Small-N Experimental Designs: A Practical Guide to Randomization Tests 2nd Edn . New York, NY: Routledge; 10.4324/9780203180938 [ CrossRef ] [ Google Scholar ]
- Edgington E., Onghena P. (2007). Randomization Tests, 4th Edn. Statistics. Hoboken, NJ: CRC Press; 10.1201/9781420011814 [ CrossRef ] [ Google Scholar ]
- Everett J. A. C., Earp B. D. (2015). A tragedy of the (academic) commons: interpreting the replication crisis in psychology as a social dilemma for early-career researchers . Front. Psychol . 6 :1152. 10.3389/fpsyg.2015.01152 [ PMC free article ] [ PubMed ] [ CrossRef ] [ Google Scholar ]
- Flick U. (Ed.). (2014). The Sage Handbook of Qualitative Data Analysis . London: Sage; 10.4135/9781446282243 [ CrossRef ] [ Google Scholar ]
- Freeman M., Demarrais K., Preissle J., Roulston K., St. Pierre E. A. (2007). Standards of evidence in qualitative research: an incitement to discourse . Educ. Res. 36 , 25–32. 10.3102/0013189X06298009 [ CrossRef ] [ Google Scholar ]
- Ganter B. (2010). Two basic algorithms in concept analysis , in Lecture Notes In Computer Science. Formal Concept Analysis, Vol. 5986 , eds Hutchison D., Kanade T., Kittler J., Kleinberg J. M., Mattern F., Mitchell J. C., et al. (Berlin, Heidelberg: Springer Berlin Heidelberg; ), 312–340. 10.1007/978-3-642-11928-6_22 [ CrossRef ] [ Google Scholar ]
- Ganter B., Wille R. (1999). Formal Concept Analysis . Berlin, Heidelberg: Springer Berlin Heidelberg; 10.1007/978-3-642-59830-2 [ CrossRef ] [ Google Scholar ]
- Guttman L. (1944). A basis for scaling qualitative data . Am. Sociol. Rev . 9 :139 10.2307/2086306 [ CrossRef ] [ Google Scholar ]
- Hogg R. V., Mckean J. W., Craig A. T. (2013). Introduction to Mathematical Statistics, 7th Edn . Boston, MA: Pearson. [ Google Scholar ]
- Hughes G. E., Cresswell M. J. (1996). A New Introduction To Modal Logic . London; New York, NY: Routledge; 10.4324/9780203290644 [ CrossRef ] [ Google Scholar ]
- Klein R. A., Ratliff K. A., Vianello M., Adams R. B., Bahník Š., Bernstein M. J., et al. (2014). Investigating variation in replicability . Soc. Psychol. 45 , 142–152. 10.1027/1864-9335/a000178 [ CrossRef ] [ Google Scholar ]
- Krantz D. H., Luce D., Suppes P., Tversky A. (1971). Foundations of Measurement Volume I: Additive And Polynomial Representations . New York, NY; London: Academic Press; 10.1016/B978-0-12-425401-5.50011-8 [ CrossRef ] [ Google Scholar ]
- Leavy P. (2014). The Oxford Handbook of Qualitative Research . New York, NY: Oxford University Press; 10.1093/oxfordhb/9780199811755.001.0001 [ CrossRef ] [ Google Scholar ]
- Maxwell S. E., Lau M. Y., Howard G. S. (2015). Is psychology suffering from a replication crisis? what does “failure to replicate” really mean? Am. Psychol. 70 , 487–498. 10.1037/a0039400 [ PubMed ] [ CrossRef ] [ Google Scholar ]
- Miles M. B., Huberman A. M., Saldaña J. (2014). Qualitative Data Analysis: A Methods Sourcebook, 3rd Edn . Los Angeles, CA; London; New Delhi; Singapore; Washington, DC: Sage. [ Google Scholar ]
- Open Science, Collaboration (2015). Estimating the reproducibility of psychological science . Science 349 :Aac4716. 10.1126/science.aac4716 [ PubMed ] [ CrossRef ] [ Google Scholar ]
- Popper K. (1935). Logik Der Forschung . Wien: Springer; 10.1007/978-3-7091-4177-9 [ CrossRef ] [ Google Scholar ]
- Ragin C. (1987). The Comparative Method : Moving Beyond Qualitative and Quantitative Strategies . Berkeley, CA: University Of California Press. [ Google Scholar ]
- Rihoux B., Ragin C. (2009). Configurational Comparative Methods: Qualitative Comparative Analysis (Qca) And Related Techniques . Thousand Oaks, CA: Sage Publications, Inc; 10.4135/9781452226569 [ CrossRef ] [ Google Scholar ]
- Scheunpflug A., Krogull S., Franz J. (2016). Understanding learning in world society: qualitative reconstructive research in global learning and learning for sustainability . Int. Journal Dev. Educ. Glob. Learn. 7 , 6–23. 10.18546/IJDEGL.07.3.02 [ CrossRef ] [ Google Scholar ]
- Schurz G. (2008). Patterns of abduction . Synthese 164 , 201–234. 10.1007/s11229-007-9223-4 [ CrossRef ] [ Google Scholar ]
- Shrout P. E., Rodgers J. L. (2018). Psychology, science, and knowledge construction: broadening perspectives from the replication crisis . Annu. Rev. Psychol . 69 , 487–510. 10.1146/annurev-psych-122216-011845 [ PubMed ] [ CrossRef ] [ Google Scholar ]
- Smith P. (2020). An Introduction To Formal Logic . Cambridge: Cambridge University Press. 10.1017/9781108328999 [ CrossRef ] [ Google Scholar ]
- Suppes P., Krantz D. H., Luce D., Tversky A. (1971). Foundations of Measurement Volume II: Geometrical, Threshold, and Probabilistic Representations . New York, NY; London: Academic Press. [ Google Scholar ]
- Tooley M. (Ed.). (1999). Necessity and Possibility. The Metaphysics of Modality . New York, NY; London: Garland Publishing. [ Google Scholar ]
- Trafimow D. (2018). An a priori solution to the replication crisis . Philos. Psychol . 31 , 1188–1214. 10.1080/09515089.2018.1490707 [ CrossRef ] [ Google Scholar ]
- Watanabe S. (2018). Mathematical Foundations of Bayesian Statistics. CRC Monographs On Statistics And Applied Probability . Boca Raton, FL: Chapman And Hall. [ Google Scholar ]
- Wiggins B. J., Chrisopherson C. D. (2019). The replication crisis in psychology: an overview for theoretical and philosophical psychology . J. Theor. Philos. Psychol. 39 , 202–217. 10.1037/teo0000137 [ CrossRef ] [ Google Scholar ]
- Open access
- Published: 26 April 2022
Definition and conceptualization of the patient-centered care pathway, a proposed integrative framework for consensus: a Concept analysis and systematic review
- Jean-Baptiste Gartner 1 , 2 , 3 , 4 , 5 ,
- Kassim Said Abasse 1 , 2 , 3 , 5 ,
- Frédéric Bergeron 6 ,
- Paolo Landa 3 , 7 ,
- Célia Lemaire 8 &
- André Côté 1 , 2 , 3 , 4 , 5
BMC Health Services Research volume 22 , Article number: 558 ( 2022 ) Cite this article
7049 Accesses
18 Citations
1 Altmetric
Metrics details
Confusion exists over the definition of the care pathway concept and existing conceptual frameworks contain various inadequacies which have led to implementation difficulties. In the current global context of rapidly changing health care systems, there is great need for a standardized definition and integrative framework that can guide implementation. This study aims to propose an accurate and up-to-date definition of care pathway and an integrative conceptual framework.
An innovative hybrid method combining systematic review, concept analysis and bibliometric analysis was undertaken to summarize qualitative, quantitative, and mixed-method studies. Databases searched were PubMed, Embase and ABI/Inform. Methodological quality of included studies was then assessed.
Forty-four studies met the inclusion criteria. Using concept analysis, we developed a fine-grained understanding, an integrative conceptual framework, and an up-to-date definition of patient-centered care pathway by proposing 28 subcategories grouped into seven attributes. This conceptual framework considers both operational and social realities and supports the improvement and sustainable transformation of clinical, administrative, and organizational practices for the benefit of patients and caregivers, while considering professional experience, organizational constraints, and social dynamics. The proposed attributes of a fluid and effective pathway are (i) the centricity of patients and caregivers, (ii) the positioning of professional actors involved in the care pathway, (iii) the operation management through the care delivery process, (iv) the particularities of coordination structures, (v) the structural context of the system and organizations, (vi) the role of the information system and data management and (vii) the advent of the learning system. Antecedents are presented as key success factors of pathway implementation. By using the consequences and empirical referents, such as outcomes and evidence of care pathway interventions, we went beyond the single theoretical aim, proposing the application of the conceptual framework to healthcare management.
Conclusions
This study has developed an up-to-date definition of patient-centered care pathway and an integrative conceptual framework. Our framework encompasses 28 subcategories grouped into seven attributes that should be considered in complex care pathway intervention. The formulation of these attributes, antecedents as success factors and consequences as potential outcomes, allows the operationalization of this model for any pathway in any context.
Peer Review reports
While having a performant healthcare system is a crucial issue for every country, the health sector operates in silos that need to be challenged. Indeed, many authors have pointed to fragmented care processes as a cause of breakdowns in the continuity of healthcare services [ 1 ], unnecessary waiting times [ 2 , 3 ], flaws in the flow of information between the different episodes [ 4 ] and the realization of exams that may be superfluous [ 5 ]. This fragmentation results in a sub-optimal use of material and financial resources and unsatisfactory team management [ 4 ]. Based on this observation, several repeated calls to improve the quality and performance of healthcare services have been made since 2001 by national and international institutions such as the Institute of Medicine of America (IOM) in 2001 [ 6 ] and 2013 [ 7 ], the National Academies of Sciences, Engineering, Medicine in 2018 [ 8 ] and the World Health Organization (WHO) in 2016 [ 9 ] and 2020 [ 10 ]. These calls have progressively shifted from an injunction to improve quality based on criteria to provide safe, effective, efficient, timely, equitable and patient-centered care [ 6 ], to the development of models for the organization of health care and services that meet the current challenges of effectiveness and efficiency in healthcare systems. The WHO urges member countries to base their quality improvement policies on the entire continuum of care, taking into account at least the criteria of effectiveness, safety, equity, efficiency, integrated care and timeliness [ 11 ]. These calls also emphasize the need to improve care pathways by focusing on outcomes that matter to the patient from a clinical, quality of life and health system experience perspective [ 12 , 13 , 14 , 15 ], rather than on the needs of the production units. This change of perspective leads to the study of the redesign of performance evaluation models by focusing on the needs and expectations of the patient [ 16 , 17 ]. The problem is that there is confusion about the definition and characterization of a care and health service pathway. Indeed, Bergin et al. [ 2 ] identified 37 different definitions of the term care pathway based on a review of the literature. Definitions and characteristics vary across countries and include multiple phases ranging from prevention or screening to cure or palliative care. This confusion has led to wide variability in the outcomes of these interventions, resulting in underutilization of care pathway improvement programs [ 2 ]. Furthermore, such confusion leads to great variability in the analysis and modeling of care pathways. For example, in their scoping review, Khan et al. [ 18 ] showed the great variability that exists among studies of oncology care pathways in both the phases of care represented, and their characteristics. The lack of a common definition and clearly defined criteria leads to a lack of standardization, resulting in an inability to conduct reliable comparative studies of care pathway programs internationally [ 19 ].
The Oxford Concise Medical Dictionary 10th ed. [ 20 ] and the Oxford Dictionary of Nursing 8th ed. [ 21 ] define, in a concise way, care pathway as “a multidisciplinary plan for delivering health and social care to patients with a specific condition or set of symptoms. Such plans are often used for the management of common conditions and are intended to improve patient care by reducing unnecessary deviation from best practice”. The concept of a care pathway is one originally used in the field of Health Operations Management, whose definition was proposed by Vissers and Beech [ 22 ]. However, these definitions seem to be too imprecise and address neither the aim nor the social reality of implementing such pathways. The European Pathway Association (EPA) adopts the more precise definition from the 2007 thesis of Vanhaecht [ 23 ]. However this has not yet led to an international consensus, as confusion over the concepts remains high. Moreover, this definition does not clearly define the antecedents or factors favoring the success of such interventions, the means by which to implement them or the best practices through which to support them; nor does it sufficiently take into account the importance of the patient-centered care and patient-centered services approach. Similarly, the proposed implementation models largely neglected the social reality and the social dynamic of organizations [ 24 ], resulting in major implementation difficulties, as care pathways still being considered as complex interventions [ 25 , 26 ].
However, care pathway programs have recently demonstrated encouraging results in terms of reduced variation in care, improved accessibility, quality, sustainability, and cost effectiveness of care [ 2 ]. The definition we aim to develop through this research is significant and timely, in that it has the potential to guide the ongoing development, implementation, monitoring and evaluation of care pathway programs within the rapidly changing service and system contexts that we are experiencing. For example, the following initial barriers to the systemic and holistic implementation of care pathways have recently been removed. Firstly, limited access to valid and reliable data from multiple organizations [ 27 ] has been offset by a massive investment in Electronic Medical Records [ 28 ]. Secondly, the main difficulties in highlighting the complexity of the referral trajectory [ 29 ], frequently resulting from the clinicians’ perspective, have been overcome by proposing new approaches such as data mining or qualitative methods, focusing on the real care trajectory and the qualitative part of the patients’ experience [ 16 , 17 , 30 ]. Therefore, the evolution of knowledge and information technology and the investment of health systems in data-sharing infrastructure, as well as a definition of the levers of patient engagement and the advent of patient-centered-care and patient-centered services, make it possible to define a powerful model for improving them by placing the patient’s needs and expectations at the center of the care pathway. It is therefore the right time to define a recognized definition and an integrative conceptual framework that meets the demand for sharing knowledge internationally regarding the development, implementation, and evaluation of care pathways.
The concept of patient-centered care is defined as “care provision that is consistent with the values, needs, and desires of patients and is achieved when clinicians involve patients in healthcare discussions and decisions” [ 31 ]. This approach is known to provide benefits by improving health outcomes, patient satisfaction, but also to reducing health costs [ 32 ].
A preliminary search for existing reviews was conducted in Cochrane Database, JBI Database of Systematic Reviews and Implementation Reports and PROSPERO. Care pathways have been the subject of few reviews, but these were limited to a single pathology such as cancer in general [ 33 ], blunt thoracic injury [ 34 ], cardiovascular disease [ 35 ], adolescent idiopathic scoliosis [ 36 ] or for particular pathway phases [ 37 ]. In the end, focusing on a single condition is not entirely consistent with a patient-centered approach to care insofar as patients often have comorbidities. The only review that did not focus on one specific pathology was made in 2006 [ 38 ] and was interested in the concept of clinical pathway. Authors reviewed literature published within 3 years using only one bibliographic database. Therefore, the aim of this article is to propose an accurate and up-to-date definition of care pathway and to develop an integrative conceptual framework for the patient-centered care pathway concept in a holistic operational approach of the concept.
Combining systematic review, concept analysis and bibliometric analysis
To achieve a fine-grained understanding of the concept, we have chosen a hybrid method combining the systematic review, the concept analysis and the bibliometric analysis methodologies. We followed the latest PRISMA (Preferred Reporting Items for Systematic reviews and Meta-Analyses) statement for conducting and reporting a systematic review [ 39 ]. However, the systematic review methodology presents some limitations on the qualitative analysis of literature, hence derives our interest to use Concept analysis. Concept analysis [ 40 ] aims specifically to clarify a specific concept including a semantic field linked to a specific theoretical framework. This approach is based on eight steps allowing to: (1) select the concept, (2) determine the aims or purposes of the analysis, (3) identify all uses of the concept, (4) determine the defining attributes, (5) identify a model case, (6) identify additional cases, (7) identify antecedents and consequences and (8) define empirical referents. However, this method does not provide a systematic and rigorous procedure for identifying and selecting relevant literature. Therefore, we decided to combine the strengths of both methods to overcome the limitations of each. In order to make our analysis more robust and to base our inferences, specifically in the comparative analysis of the related concepts, we performed a bibliometric analysis allowing us to link the attributes of each of the concepts to make a comparison.
Information sources and search strategy
We developed a search strategy, in collaboration with a Health Sciences Librarian who specializes in systematic literature review in healthcare, to identify relevant peer-reviewed studies. An initial limited search of MEDLINE and CINAHL was conducted, followed by analysis of the text words containing title and abstract and index terms used to describe the article. This informed the development of a search strategy that was tailored toward each information source. The search strategy was applied to the following databases: PubMed, Embase and ABI/Inform. The complete search strategy is provided in Additional file 1 .
Eligibility criteria
This review considers studies that focus on quantitative and/or qualitative data, with no limitation in terms of methodology. Our search focused on peer-reviewed scientific articles. Therefore, books, doctoral or master’s theses were excluded due to time and resource limitations. In order to guide the selection, we chose the Population, Context, Concept (PCC) mnemonic criteria [ 41 ]. The population considers all types of patients managed by healthcare delivery systems. The context studied is composed of healthcare providers in any geographic area, including all providers of primary, secondary, tertiary, and quaternary care. For the concept, this review focuses on theoretical and empirical studies that contribute to the definition and conceptualization of the different related concepts of care processes at the organizational or system level, such as care pathway, clinical pathway, patient journey and care processes. Quantitative, qualitative and mixed method studies involving a single episode of care limited in time (a one-time treatment) or space (a single hospital service/department) were excluded to the extent that care pathway involves multiple points of interaction over time [ 13 , 42 ] and multiple organizational structures or intra-organizational entities along the care continuum [ 43 ]. In addition, studies with no theoretical or conceptual input were excluded. Finally, there was no language or geographic restrictions applied to the search, and the study period was limited from 1995 to 2020.
These studies were imported into the Covidence® software (version 2020). The team developed screening questions and forms for levels 1 (abstract) and 2 (full text) screening based on the inclusion and exclusion criteria. Two reviewers independently screened the titles and abstracts. In case of disagreement, two senior reviewers decided after analysis and discussion. Review author pairs then screened the full-text articles against inclusion and exclusion criteria. In case of disagreement, the same process as for the title and abstract selection was implemented. Reasons for excluding studies were recorded.
Assessment of methodological quality
Because of the heterogeneity of the methods used in the selected articles, we decided to use a separate appraisal tool for each study type. The following appraisal tools were selected for their clarity, relevance, and because their items covered the most common assessment criteria comparing to other tools:
For qualitative studies: the JBI Qualitative Assessment Research Instrument (QARI) [ 41 ]
For surveys: the Center for Evidence Based Management (CEBMa) Appraisal Questions for a Survey [ 44 ]
For descriptive cross-sectional studies: the Institute for Public Health Sciences 11 questions to help you make sense of descriptive/cross-sectional studies [ 45 ]
For mixed-method: the scoring system for appraising mixed methods research [ 46 ]
No articles were excluded from this systematic review due to the weaknesses of their methodological quality, so as not to exclude valuable information [ 47 ].
Data extraction and analysis
Descriptive numerical summary analysis followed the systematic review guidelines, and the following items were systematically extracted: Reference, Title, First Author country, Case country, Year of publication, Type of publication, Target patient population, Phases of the pathway included, People involved in the modeling process, Study parameters and level of analysis.
Qualitative data were extracted using MaxQDA® software (version 2020) by two independent analysts. The data extraction followed the concept analysis guideline [ 40 ] and the following items were systematically extracted: Variant concept studied, Concept uses, Concept definition, Concept attributes, Antecedents, Consequences and empirical referents. In order to develop a detailed analysis and arrive at a robust theoretical framework, we relied on general inductive analysis [ 48 ], consisting of coding, categorization, linking, integration and modeling. Each step has been validated by at least two senior authors.
A bibliometric analysis was performed with the complete texts of the 44 selected studies using Vosviewer® software (version 2020).
The systematic review was reported following the latest PRISMA statement for conducting and reporting a systematic review [ 39 ] and mobilized the PRISMA 2020 checklist (see Additional file 2 ).
The interrogation of the three databases resulted in 15,281 articles. Figure 1 details the selection process following the PRISMA 2020 statement [ 39 ]. After deleting the duplicates, 15,072 records were reviewed but only 44 publications ultimately met the inclusion and exclusion criteria.
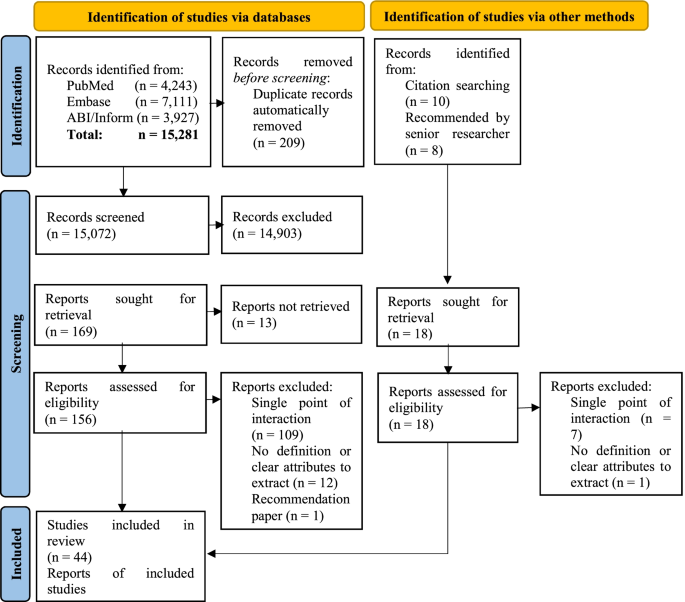
PRISMA 2020 flow diagram of the systematic review process
Description and methodological quality appraisal of studies
A summary table containing a brief description of selected studies and their evaluation results for methodological quality is presented in Table 1 . Quality appraisal of selected studies is presented in Additional file 3 .
Published articles, describing care pathways as multiple points, in time and space, of patient interaction appeared in the early 2000s. However, most of this work has been published since 2010, with a progressive and growing interest, whatever the theoretical position, to reach 22 articles in the last 3 years (see Fig. 2 ).
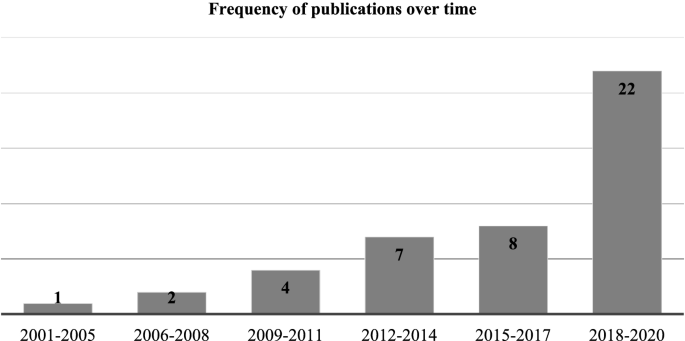
Frequency of selected publications over time
The countries of the first authors interested in this concept are predominantly anglophone such as the United Kingdom (k = 9), Australia (k = 5), the United States (k = 4), and Canada (k = 3). Researchers from other countries are less represented.
Three types of publications were found; 34 were original research studies, eight were literature reviews and two were perspective studies. In the original research studies, 23 used a qualitative approach to study either the implementation of a care pathway program or patient experience of a care pathway, four used a descriptive cross-sectional approach, four used a mix-method approach and three used a survey.
Since the definition of the concept is still unclear and terminology is important, the studies meeting the selection criteria reported several terminologies. The most frequently used terms in the selected studies were the patient journey (k = 14) and the care pathway (k = 13) with their some country-specific modifications namely integrated care pathway mainly in the United Kingdom [ 73 , 74 ], optimal care pathway in Australia [ 2 ] and standardized care pathway in Sweden [ 15 ]. The other terms used were clinical pathway (k = 8), patient-centered care (k = 4), care process (k = 3), disease pathway management (k = 1) and value-based integrated care (k = 1).
Studies focused mainly on the care of chronic conditions (k = 24), followed by acute diseases (k = 11). Of those with a chronic care focus, cancer was by far the most studied disease (k = 10), followed by stroke, hearing impairment and mental disease. Acute care studies covered, articular pathologies of the hip and knee, and pregnancy.
Concerning the level of the study, most addressed the systemic (k = 31) rather than the organizational (k = 13) level. Most authors, in their approach to the concept, largely focused on the treatment phase (k = 39), but some included, more or less, pretreatment and subsequent phases. Only seven articles took a global approach starting from the prevention phase and screening to survivorship or palliative care phase.
Concept analysis results
The conceptual analysis followed an automatic data extraction method in the proposed main categories and then, after several iterations, resulted in a coding of subcategories grouped into main themes. The detailed results of the coding are presented in Additional file 4 .
Concept uses
Uses of the concepts of care pathway have evolved in the literature over time with a strong tendency to focus on the care pathway at the systemic level. Main objectives have been improving quality and safety (k = 26), improving efficiency in the delivery of care (k = 24), optimizing the delivery process through an operation management point of view (k = 22) and integrating best practices through guidelines and evidence-based medicine (k = 17). These objectives were widely shared and present throughout the period. However, interest emerged in 2009 and quickly grew, in improving the patient experience through the analysis of the patient journey (k = 17). To a lesser extent, the goals of developing patient-centered care (k = 13), improving patient outcomes (k = 13), improving coordination of service delivery (k = 13), and standardizing care delivery (k = 12) were also present. Beyond standardization, reduced variation in care practices (k = 9) was not well addressed, nor was continuous performance assessment (k = 8). The aim of meeting the patient’s needs (k = 6) has been addressed more frequently in recent years, since its first appearance in 2011 [ 71 ], and is considered of crucial importance by some authors. Other concept uses were proposed, such as to improve interprofessional collaboration (k = 5), support changes (k = 5), support clinical decision making (k = 4), improve communication (k = 3), consider needs of healthcare workers, improve referral system, define shared purposes and meaningful objectives (k = 2), monitor staff compliance, support the knowledge management, improve patient and family member access to information, adopt a system approach and understanding power dynamics and relational factors (k = 1). As described previously, these concept uses came mainly from the chronic disease care context, although acute care was also represented.
Defining attributes
Definitional attributes are features commonly encountered in definitions of the concept or frequently used to describe it [ 40 ]. Twenty-eight attributes were inductively extracted and categorized into seven main themes, ordered by level of empirical importance: (1) The centricity of patients and caregivers; (2) the positioning of professional actors involved in the care pathway; (3) the operation management through the care delivery process; (4) the particularities of coordination structures; (5) the structural context of the system and organizations; (6) the special role of the information system and data management; and (7) the advent of the learning system (k = 3).
Attribute theme 1: The centricity of patients and caregivers
Firstly, there has been a growing interest in the patient experience (k = 15), mainly through the concept of the patient journey [ 5 , 13 , 14 , 15 , 24 , 30 , 42 , 51 , 52 , 58 ], which has progressively emerged as the third pillar of quality in healthcare with clinical effectiveness and patient quality and safety [ 30 ]. It is formed by all the interactions at the meeting point, or point of contact, between health services and patient [ 14 , 30 , 42 , 51 ]. However, taking the patient experience into account is complex insofar as it requires a detailed understanding of what influences it. Therefore, some authors have defined the dimensions that can influence the patient experience as the temporal dimension, meaning that accessibility and short waiting times are valued [ 13 , 15 , 30 , 42 , 51 ], the spatial dimension [ 30 ], and the geographical position of the services [ 42 ], the emotional dimension [ 13 , 30 , 42 ] and the social and cognitive dimensions [ 13 , 42 ]. All these dimensions can be the source of both positive outcomes [ 13 , 30 ] and negative outcomes [ 15 ] or for socio-political authors, a feeling of considerable disempowerment [ 53 ]. Although authors are increasingly interested in it, the patient experience is still sometimes overlooked [ 14 ].
Patient information and education (k = 15) were addressed in numerous studies. Patient information contributes to the quality of the patient experience [ 3 , 15 , 36 , 42 , 53 , 64 , 71 , 75 ]. Beyond the simple satisfaction, the provision of information, at an appropriate health literacy level, increases patient awareness [ 36 , 51 ] and thus increases patient education. This results in a better detection of the symptoms at an early stage by the patient [ 3 , 36 ], the development of the “expert patient” [ 51 , 57 , 58 , 71 ], which aids adherence to treatment, supports shared decision-making [ 57 ] and improves self-management [ 51 , 58 ]. However, many empirical studies showed there to be a lack of patient information throughout patient journeys [ 5 , 14 , 15 , 42 , 51 , 53 , 64 ].
Patient engagement (k = 15) was an important attribute of this theme in the more recent literature. The management by the patient of his or her care treatment plan has become increasingly important [ 24 , 50 , 51 , 53 , 67 ]. This translates into shared decision-making on care and treatment [ 3 , 14 , 24 , 35 , 51 , 53 , 55 , 54 , 55 , 58 , 64 , 65 ]. According to Devi et al. [ 51 ], this process can only be viable if supported by good information about treatment possibilities and possible outcomes. However, socio-political authors see this as a major issue of patient empowerment, which is “seen as a solution to many of the most pressing problems facing modern healthcare” [ 53 ].
Proposed only since 2014, and strongly present in the last 3 years, relationship as the basic need (k = 9) is also a subject of interest. Part of the patient experience, the relational quality reflects how patients perceive their interactions [ 13 , 42 ]. Some empirical studies have shown that a poor relationship can negatively affect other processes and tasks [ 3 , 5 ]. Therefore, quality of the relationship seems a fundamental prerequisite [ 14 , 64 ]. For this reason, some authors have placed the notion of trust as essential to the quality of interactions and to the patient’s follow-up through the care pathway [ 3 , 12 , 58 ].
Patient and Public Involvement (k = 9) is part of these new topics. Its importance in the design and improvement of the care pathway is supported by some international organizations [ 9 ]. The objective is to improve the quality of care provided by assessing patients’ perceptions [ 12 , 13 ]. In this way, the design of care delivery can be based on the real needs and expectations of patients [ 12 , 13 , 51 , 56 , 62 ]. However, some models have been criticized as tokenistic rather than being viable solution for balancing power between patients and health care providers [ 53 ].
Although the stated goal of care pathways incorporates an approach aimed at standardizing care practices, several authors have raised the need for individualized care (k = 8). Joosten et al. [ 74 ] saw a potential conflict between standardization and the demand for a personalized approach to healthcare. However, several authors have subsequently agreed that there is still room for individualization of care beyond the standardization [ 55 ], in particular through the definition of personalized treatment goals [ 51 ], or even maintaining flexibility in the interaction to better adapt to the patient’s specific needs [ 64 , 65 ].
Developed only since 2016, the importance of psychosocial support (k = 8) has increased rapidly. Although the need has been clearly identified and documented [ 5 , 15 , 42 , 58 ] and many international guidelines have integrated it, it seems that its translation within the care pathway is still complex [ 62 ] and no obvious answer was provided.
The inclusion of family and caregiver (k = 8) is also a new topic of the last 5 years which highlights the potential of family or caregivers involvement in decision-making [ 50 , 51 , 57 , 65 ]; notably by supporting both the integration of information and personal decision-making [ 14 , 15 ].
Attribute theme 2: The positioning of professional actors involved in the care pathway
Firstly, most authors consider the care pathway as a tool to develop patient-centered care (k = 18). The patient-centered care approach has a disease-specific orientation [ 25 ] and considers the patient as a real partner [ 51 , 25 ]. In doing so, this approach recognizes an individual’s specific health needs and preferences as the driving force in all healthcare decisions [ 13 , 51 , 65 , 67 ]. Thus, professional actors emphasize their accessibility and their attitudes and behaviors towards patients [ 13 ]. In addition, this approach considers the importance of integrating family and caregivers and is recognized as a necessary attribute of healthcare quality [ 65 ]. Finally, its implementation seems to improve patient satisfaction by moving toward an individualized therapy approach and personalized treatment goals [ 51 ].
Not surprisingly, multidisciplinary team-working (k = 17), and attribute which is consistent with previous definitions, is supported by several authors. The enrollment of all professional categories involved directly or indirectly in the care pathway at all steps is valued [ 2 , 50 , 75 ]. The multidisciplinary teamwork allows tackling the complexity of patient care across the pathway and developing a shared understanding supported by knowledge sharing among professionals [ 53 , 72 ]. In addition, it allows outlining the optimal sequence and timing of interventions [ 38 , 59 ] and to focus only on patient needs and engagement rather than on problems of a particular profession [ 56 ]. From an operational view, multidisciplinary care teams make it possible to share formal screening between disciplines [ 62 ]. Recently, multidisciplinary engagement was identified as a mandatory prerequisite for successful care pathway programs [ 24 , 50 ].
Staff skills (k = 10) could be considered equally important for care pathways. However, they were not addressed in this literature before 2014. Authors gave little attention to technical skills, except to point out possible deficiencies, particularly in diagnosis [ 3 , 13 ], but also in training [ 3 ]. Rather, authors focused almost exclusively on interpersonal skills [ 3 , 12 , 13 , 15 , 51 , 64 ], which were considered critical, both in the relations between professionals [ 12 , 15 , 51 , 56 , 64 ] as well as those with patients and their caregivers [ 15 , 51 , 64 ]. Interpersonal skills could be seen as facilitators or barriers to the patient experience [ 64 ]. Some authors have recently suggested that peer cooperation was critical [ 5 , 50 , 56 ] and that creating a culture of mutual respect among both medical and administrative colleagues can ultimately improve the fluidity of care [ 3 , 5 ].
Few authors have highlighted that the implementation of a care pathway leads professionals to examine their roles and responsibilities (k = 6). The need to define each step in the care process requires professionals to describe precisely the tasks and roles of professional actors [ 25 ]. In doing so, it creates a rare opportunity to step back from daily tasks and reassess competences, roles and responsibilities [ 12 , 51 , 73 ].
Finally, very recently, authors have been interested in the experience of staff (k = 2) in care pathway programs. These authors have demonstrated the link between staff experiences and their individual performance [ 24 , 53 ]. They therefore support the idea that staff well-being is directly related to engagement and performance and, thus, a negative staff experience can influence patient, clinician, and organizational outcomes.
Attribute theme 3: The operation management through the care delivery process
This analysis has shown, unsurprisingly, that the process approach to care delivery (k = 23) was the core of the care pathway approach across the literature to date. From an engineering perspective, as define by the International Organization for Standardization, a process is “a set of interrelated or interacting activities that transforms inputs into outputs” (ISO 9000:2000 clause 3.4.1). Through this approach, the care process can be defined as an arrangement of tasks or actions sequenced in time resulting in a time matrix [ 24 , 30 , 38 , 52 , 60 , 68 , 25 , 73 ]. What distinguishes the different process approaches to care delivery are the tasks and actions included with them. Some authors tend to focus on operational planning by treating tasks, actions and their timing through business processes [ 43 , 49 , 54 , 60 , 69 ], while other authors consider both the context of action through the physical and organizational environment [ 24 , 30 ] and social dynamic through the experience of actors [ 24 , 52 , 53 ]. Through this approach to care processes, some authors focus on patients and caregivers [ 52 ] and other authors focus on human actors, both patients and caregivers and the professional actors involved in the care pathway [ 24 ]. In 2018, Ponsignon et al. [ 13 ] proposed to differentiate the direct, indirect and independent interactions (those disconnected from the delivery system), in care processes. Direct interactions constitute the points of contact between patients and the system, and so are responsible, along with indirect interactions, for the patient version of the pathway that some authors call the patient journey [ 5 , 13 , 30 , 51 , 53 ]. More recently, the complexity of the care process has led some authors to consider that the care pathway should involve pathway rules which control the process [ 70 ]. Thus, decision-making becomes a central element in the smooth running of the care pathway [ 60 ]. In addition, many authors consider that healthcare decisions and care pathways are intertwined so that it becomes imperative to co-design both care pathways and the decision-making activities [ 60 ].
The issue of process management for the delivery of care naturally raises the question of process modeling methods (k = 18). In the empirical articles, the use of the Business Process Modeling Notation (BPMN) developed by the Object Management Group seems to be progressively imposed, sometimes improved by decision modeling [ 4 , 43 , 54 , 60 , 68 , 69 ]. The use of process mapping or flowcharts with sometimes less formal rules seems to be favored for global approaches to processes, especially for the patient journey, although some authors such as Combi et al. [ 60 ], have demonstrated that BPMN modeling was quite compatible with the systemic approach.
For healthcare service designers, the methods for building care pathways are important considerations. Several methods exist, but all involve the discovery of a different path, thus change is inevitable and change management a necessity. The initial method came mainly from the expertise of professionals through interviews, focus groups or Delphi methods [ 49 , 59 ]. The advantage of collaboration with staff and experts is that more information can be gathered about certain decisions and possible variances from the pathway [ 49 ]. However, this method did not consider the real trajectory or the ideal pathway but rather the one integrating the constraints of the professionals. Since these early efforts, data driven approaches has developed considerably [ 43 , 49 ]. Their advantage is that they inform pathway development from data derived factually and objectively from actual occurrences of the pathway [ 49 ]. Moreover, data on the perspectives of patients through experience mapping, interviews, focus groups or observations [ 5 , 13 , 30 ], and patient shadowing [ 53 ] can be integrated to better reflect the real trajectory and to define the ideal pathway according to the needs and expectations of patients and caregivers. However, this approach does not allow for the integration of context and organizational constraints. Finally, few authors adopt an approach that consists of comparing the experience of professionals and patients, making it possible to define the lived experience, the patient’s journey, and its confrontation with operational realities and constraints through the experience of professionals [ 1 , 3 , 4 , 15 , 65 , 71 ].
Regarding the process of care delivery, the management of operations aims to integrate the organization of the delivery process with its ongoing improvement (k = 11) by focusing as much on analyzing the variations as on eliminating the wastes [ 74 ]. Process improvement tools serve as much to redesign the processes as define a workflow management system to monitor the care pathway [ 4 ]. The information generated [ 60 , 61 , 63 ] can be used for process re-engineering, objective reassessment or supporting non-clinical decision-making [ 60 ], such as the identification of bottlenecks [ 61 , 67 ] or highlighting interfacing problems between organizations [ 61 ]. The output generated by the analysis of the process-related data allows defining standardized expedited diagnostic processes [ 4 , 60 ]. Finally, the data obtained allows the use of simulation and optimization models. On this subject, Aspland et al.’s literature review [ 49 ] provides an exhaustive review of available methods.
Attribute theme 4: The particularities of coordination structures
In line with most of the definitions, the integration of the clinical practice guidelines, based on evidenced-based medicine, into the care pathway (k = 24) has been accepted since the beginning of such programs. The clinical decisions directly affect the flow of the care delivery process and thus the process performance and the quality of outcomes [ 60 ]. Therefore, the adherence to clinical practice guidelines must support decision-making [ 70 , 73 ] and aid diagnosis and treatment in order to improve patient outcomes [ 50 , 51 , 58 ]. In 2010, Vanhaecht et al. [ 25 ] expressed concern about a lack of evidence-based key interventions within care pathways. The care pathway can be an effective method to integrate and guarantee the appropriate use of evidence-based interventions and clinical practice guidelines [ 55 ] and may help to overcome two limitations of clinical practice guideline use, which are emerging as key issues [ 60 , 66 ]. Firstly, that they should not be followed blindly as they represent only explicit medical knowledge [ 67 ], but rather require integration of the contextual knowledge of healthcare professionals for appropriate use [ 72 ]. Secondly, it has been shown that physicians can be unaware of updates and changes to clinical guidelines [ 3 ], and so, integrating them into care pathway maps may improve guideline use and adherence. Finally, collectively integrating and discussing clinical practice guidelines appears to improve interprofessional collaboration and clarify roles [ 36 ], but also could benefit the involvement of patients in the co-design of the care pathway [ 35 ].
Some authors consider information continuity (k = 13) as a key factor. Not only because sharing information must support decision-making [ 60 , 75 ] and facilitate communication [ 2 , 12 , 38 ], but more broadly because the disruption of the information flow can lead to coordination problems and easily avoidable costs linked to the repetition of examinations [ 5 , 56 , 59 ]. Therefore, the continuity of information must be supported to ensure sustainable health improvements [ 51 , 70 ]. Some authors insist on the importance of defining an information medium throughout the pathway which is as accessible to care professionals as it is to patients and caregivers [ 65 ].
Recently, some authors have dealt with the subject of leadership of the care pathway (k = 9). The importance of defining a leader for each step of the care pathway was noted [ 25 ]. The lack of coordination without a responsible actor has been shown, especially when the care pathway includes actors in several contexts such as primary care [ 3 ]. Thus, new roles have been defined, such as case managers, joint program or nurse coordinators [ 4 , 15 , 42 , 65 ], roles that enhance coordination among providers through the improvement of the continuity and quality of the information as well as communication [ 15 ].
More recently, the integration of services (k = 9) has been addressed. Because the care pathway approach can involve multiple partnerships between organizations and primary care, it is essential to integrate all stakeholders. The integration needs to be both organizational, at the macro and meso-level through shared purpose and priorities [ 4 , 57 , 25 ] and shared governance mechanisms [ 4 , 12 , 14 , 59 ], and functional at the micro level through communication mechanisms and tools [ 4 , 12 , 14 ]. The unifying element is discussed between the shared interest for the patient [ 56 , 57 ] or the outcomes [ 12 ] to align strategic goals. For Louis et al. [ 56 ], achieving shared purpose is part of the structural context.
Finally, the care pathway is seen as a means of health knowledge management (k = 7) that optimizes quality, efficiency, and organization [ 68 , 70 , 72 ]. But this topic, although strongly addressed between 2011 and 2012, did not seem to be unanimously agreed upon because it was not very well addressed afterwards. However, particular attention can be paid to the elicitation and integration of the contextual knowledge of the various actors involved throughout the care pathway into daily healthcare routine [ 3 , 70 , 72 ].
Attribute theme 5: The structural context of the system and organizations
Firstly, the local physical context (k = 10), topical in the recent literature, includes both the number of units and their positions [ 12 , 67 ], but also the variety of services offered [ 13 ], and can be either an asset in terms of choice and accessibility or a constraint becoming a source of delay [ 14 ]. These barriers are important as the pathway crosses several formal healthcare organizations or informal care settings [ 24 ]. Therefore, the challenge of service integration has become essential [ 51 ].
Secondly, the availability of resources (k = 10) (human, material and financial) has a direct impact on the care pathway and the ability to meet the needs of the population [ 2 , 62 , 25 ]. A lack of adequate resources is an obvious obstacle to care pathways [ 50 ]. A lack of material and human resources, such as the availability of time at each service point [ 52 , 53 ], or the lack of an electronic medical record [ 5 ], meant the unnecessary repetition of history taking, examinations and full investigations. From a financial point of view, the financial and personal resources that people have, are also key to determinants of the care pathways followed by patients [ 51 ].
Thirdly, the social context (k = 7) is less addressed in the current literature but has shown rapid growth in recent years. Social structure includes material and social resources including roles, rules, norms, and values [ 3 , 24 , 53 , 68 ]. Some authors consider the social context as regularities of perception, behavior, belief and value that are expressed as customs, habits, patterns of behavior and other cultural artifacts [ 68 ]. Other authors consider that social structures shape people’s actions and that through people’s interactions they can then reproduce or change these social structures [ 53 ]. While others consider, for their part, that social and physical contexts can be at the origin of boundaries that mitigate against collaboration, adding to the complexity of shared clinical practices in this field [ 3 , 24 ].
Attribute theme 6: The special role of the information system and data management
Data management (k = 14) plays an increasingly important role in the analysis and improvement of care pathways. The implementation of a care flow management system aligned to clinical workflows [ 67 , 69 ], allows real-world data to be used [ 51 ], and visualized through performance dashboards to generate timely corrective action [ 4 ]. It also enables the analysis and monitoring of the variance in time and space within care pathways [ 43 ]. It is considered responsible for the rise of accountability [ 12 , 75 ].
The Electronic Health Record system is a support tool (k = 13) in several aspects. Numerous authors consider that it supports the patient-centered approach [ 51 , 67 ]. In particular, it has the capacity to support communication between health professionals, and between them and the patient [ 5 , 12 , 65 , 67 , 73 , 75 ], but also to support healthcare knowledge learning [ 67 , 73 ], and integrate clinical decision support into IT applications and clinical workflows [ 70 ]. This support throughout the care pathway can improve the quality of care and health outcomes by reducing medication errors and unnecessary investigations [ 5 ]. As stated by Fung-Kee-Fung et al. [ 4 ], the information system provides the fundamental connectivity across silos and professional groups to support the creation of care pathways and sustainable change at the system level.
The issue of digitalization (k = 5) has been treated very recently. It raises the issue of system integration throughout the care pathway. Despite the technological advances and the support of international organizations such as the guidelines on evidence-based digital health interventions for health system strengthening released by the WHO [ 76 ], there are still inefficiencies associated with trying to integrate EHRs across organizations [ 56 ]. These are frequently due to the use of different technological solutions by different stakeholders [ 30 ]. The challenge is therefore to propose a model for integrating information systems throughout the care pathway that are accessible to all stakeholders including patients themselves [ 4 , 50 , 51 , 65 ].
Attribute theme 7: The advent of the learning system
Although it was not frequently addressed, some authors have developed, very recently, the importance of setting up a learning system (k = 3) to support the care pathway. Resulting from the work of Quinn [ 77 ] and Senge [ 78 ], it consists of the development of a system to learn from itself and its past experience and improve the effectiveness, efficiency, safety, and patient and family/caregiver experiences [ 65 ] through a feedback loop [ 24 ]. Data on outcomes can be used as feedback to identify improvement opportunities at various stages of the process or at specific interfaces between stakeholders. The learning system promotes “individual competence, systems thinking, cohesive vision, team learning, and integrating different perspectives” [ 4 ].
Related concepts
The related concepts are confusingly close or even integrated with the main concept studied [ 40 ]. Given the complexity of the use of concepts, we have relied, in addition to definitions found on an analysis of a bibliometric network by integrating all 44 articles, excluding abstracts and bibliographies, into the Vosviewer® software (version 2020). The results help us to refine our understanding of the concepts which define the links between the different keywords. The care pathway bibliometric links are provided as a comparator (see Fig. 3 ).
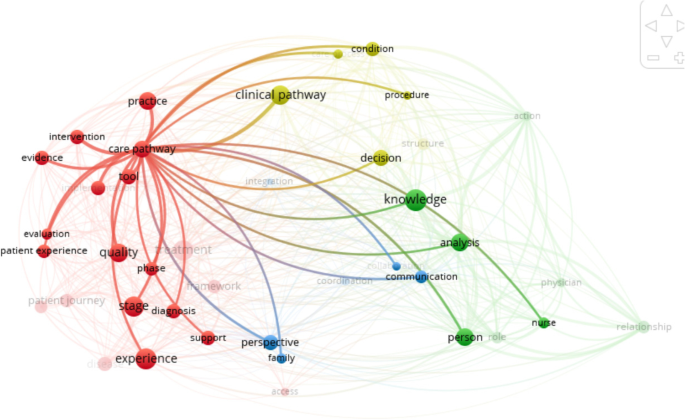
Care pathway bibliometric links
Clinical pathway (Fig. 4 ) was initially defined by De Bleser et al. [ 38 ]. It is a multidisciplinary intervention that aims to integrate the guidelines into daily routine and manage medical activities in order to improve the quality of service and optimize the use of resources [ 70 ]. It integrates a process of care approach [ 72 ] and aims at standardize care on a procedure or an episode of care [ 38 , 49 , 68 ], integrating decision-making supported by knowledge. What differentiates it from the care pathway is that it is restrained in time and is anchored in an organization [ 25 ], or even a service, and does not deal with the patient experience in any way. Clinical pathways are thus integrated in care pathways at the local level and focus on a single phase of care.
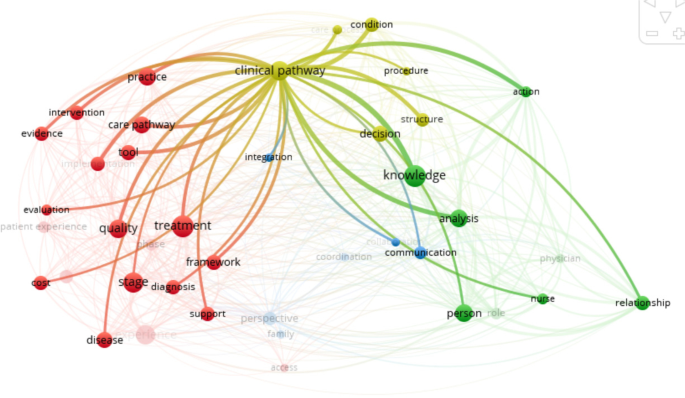
Clinical pathway bibliometric links
Patient journey (Fig. 5 ) consisted of sequential steps in the clinical process of the patient through their experience. It can be defined as “the spatiotemporal distribution of patients’ interactions with multiple care settings over time” [ 24 ]. By analyzing and mapping the patient experience from their perspective [ 5 , 14 , 57 , 58 , 71 ], the objective is to improve the quality of the service provided [ 14 , 52 ]. In this approach, the patient journey is an integral part, and an essential component, of the care pathway. Although it also integrates the process approach, it is not linked to decision-making or knowledge management and does not consider structural constraints or the perception of the providers.
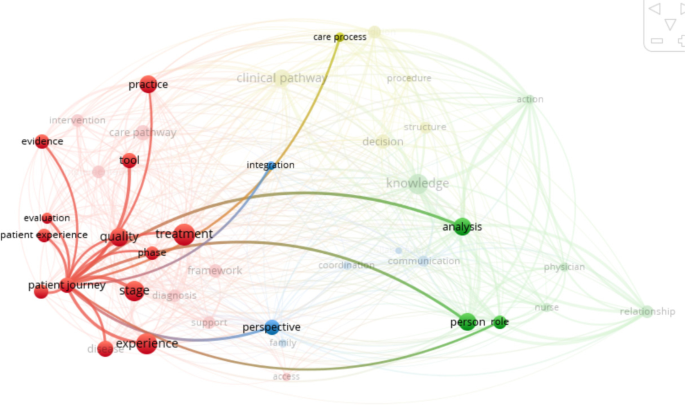
Patient journey bibliometric links
Finally, the care process (Fig. 6 ) is involved across the care continuum to standardize and streamline end-to-end care using management tools [ 4 ]. It is directly linked to the care pathway, the clinical pathway and the patient journey. However, although it supports coordination through decision-making and knowledge management, it does not consider the patient experience, the social relationships and the social dynamics. So, the care process is an integral part of the care pathway but does not consider all the characteristics of the latter.
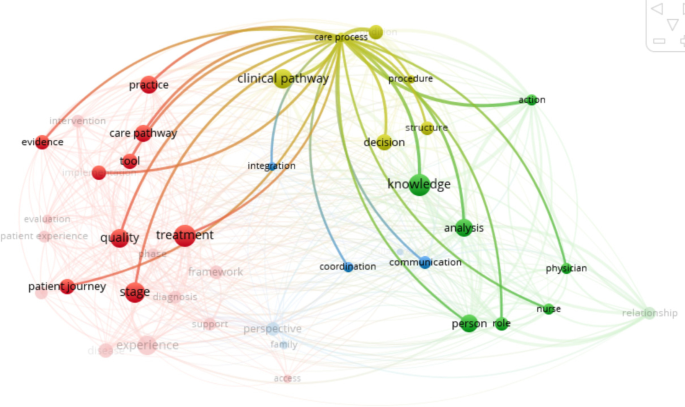
Care process bibliometric links
Antecedents of the concept
Antecedents are events occurring or in place before the concept can emerge [ 40 ]. Our analysis has highlighted several prerequisites for care pathway implementation (see Additional file 4 ).
Firstly, several authors have stressed the importance of the availability of managerial skills (k = 10). They recommend the creation of a change management team [ 49 , 55 ] consisting of a multidisciplinary team integrating not only knowledge about care pathways [ 60 , 70 ], but also knowledge about operations research, information systems and industrial engineering [ 49 , 55 ]. In addition, some authors advocate the presence of key change leaders in the group included clinicians, administrators, IT leaders, process experts, data analysts, nurses, and patient and family members [ 4 , 24 ]. The project leaders must be available on a long-term basis [ 50 , 75 ], have the ability to understand system interdependencies [ 24 ] and have the ability to create a safe learning environment in which openness is encouraged and everyone’s opinion is valued [ 3 , 50 ]. This could be achieved by using consensus-driven approaches that could address institutional process barriers, resistance to change, and conflicting targets and priorities [ 4 ].
Secondly, care pathway projects should have a priori the adequate resources (k = 4), but their availability must be verified [ 62 , 75 ]. The presence of an EHR is necessary to have access to reliable data at the pre-analysis phase and during the implementation phase to identify the relationships between the context, the mechanisms and the results obtained [ 2 , 73 ].
Finally, other key success factors emerged from the literature (k = 10). Some authors noted that rules of co-involvement and a bottom-up strategy was needed [ 55 ]. Other authors emphasized that the selection of areas where there were clearly established deficiencies was essential given the cost of such projects, but also that the identification of any subgroups for whom its use may not be appropriate, was also required [ 73 ]. They highlighted the importance of following guidelines to achieve professional adherence [ 2 , 50 , 62 , 72 , 73 ], while maintaining flexibility in the approach to implementing a care pathway improvement program [ 62 ]. They also pointed to the importance of communicating on the progress of the project [ 50 ] and of monitoring the applicability of daily work tasks [ 73 ]. Finally, they consider it essential to embed the pathway into policy and strategy [ 2 , 50 , 72 , 75 ]. While others, for their part, highlighted the importance of defining an iterative feedback loop for individuals and aggregated operational and clinical data [ 4 , 24 ].
Consequences (outcomes) and identification of empirical referents
Consequences are events that are the results of the mobilization of the concept [ 40 ] and empirical referents, for their part, consist of observable phenomena by which defining attributes are recognized [ 40 ] (see Additional file 4 ). In a larger sense, this could be the Key Performance Indicators (KPIs) by which one can recognize the defining attributes and their outcomes.
Although the terms of quality and safety, efficiency and process improvement were the first themes in terms of aims, the most frequently occurring theme in the findings pertained to effects on the patient experience (k = 16). These were measured in different ways, including the impact of waiting times (k = 10), patient satisfaction (k = 7) and the patient quality of life (QALYs) (k = 4). There were also attempts to analyze the patient experience more broadly (k = 5), and to integrate patient needs into the redesign of the care pathway [ 5 , 13 , 56 ].
Efficiency of care (k = 15) was strongly supported by some authors as a desired outcome in care pathways. This outcome was first seen, as an objective, through the costs and cost effectiveness of programs [ 49 , 55 , 61 , 70 ], however, more recently it has been considered a consequence of process improvements, rather than a program objective. It has been clearly defined as the reduction of costs through the reduction of the use of healthcare services [ 57 ]. Moreover, reduction in time spent in care, such as the length of stay or cycle time [ 2 , 55 ], is commonly the consequence of process improvements.
Quality of care (k = 11) was addressed but much less frequently than expected. In the global approach, time to diagnostic is a good empirical referent to analyze the capacity of the first steps of the care pathway [ 4 , 69 ]. Other referents such as reduction of unnecessary investigations and medication errors are also addressed but the number and types of complaints were addressed only by socio-political authors [ 53 ].
Health outcomes (k = 11) were also proposed but only since 2009 [ 73 ]. Clinical outcomes and mortality rates are empirical referents that are unanimously accepted. Recovery time and readmission rates were less frequently considered. Single disease index evaluation was proposed by very few authors [ 49 , 70 ].
Process metrics and patient flow (k = 11) was addressed but only the execution time was unanimously accepted as an empirical referent. Apart from the process variance which is shared, only few authors have developed other KPIs such as the percentage of pathway completion [ 70 ], and evaluation for the reasons of pathway failure [ 70 ].
The variance of practices (k = 9) was not frequently addressed as an empirical referent; however, this is one of the objectives of the care pathway addressed in the literature. The introduction of guidelines [ 2 ] aims to decrease the variation within or between practices (k = 3).
Continuity of care (k = 6) was poorly addressed, even though we might assume that this is one of the primary objectives of the care pathway. This may be due to the difficulty of providing tangible results given the duration of such interventions.
Some authors noted an improvement in documentation and data collection (k = 5), measured by rate of documentation [ 54 ], the ability to better understand resource adequacy (k = 3) and a better comprehension of the links between decision outcomes and process performance (k = 2).
Not defined as an outcome, the Human Resources metrics are proposed by some authors and notably diagnostic quality and referral appropriateness, professional competences and staffing levels. Only Carayon et al. [ 24 ] proposed to integrate the quality of working life as an indicator, based on the principle that well-being at work has a direct impact on individual performance and on the results of the care pathway.
Moreover, not present in the empirical references, the measure of the team relationship and coordination (k = 4) has been proposed by some authors, however, the type of indicator has not been clearly explained.
An integrative definition and conceptual framework of patient-centered care pathways
Given the results of our systematic review and concept analysis and our main objective of defining an integrative framework, we suggest the following definition:
“A patient-centered care pathway is a long-term and complex managerial intervention adopting a systemic approach, for a well-defined group of patients who journey across the entire continuum of care, from prevention and screening to recovery or palliative care. This intervention:
prioritizes the centricity of patients and caregivers by analyzing the patient experience through their needs and expectations, taking into account the need for information, education, engagement and involvement and integrates the patient relationships as a fundamental need.
supports the roles of professional actors involved in the care pathway by developing adherence to the patient-centered care approach; working on interdisciplinarity through the development of skills, both technical and above all relational; the clarification of roles and responsibilities; and by taking into account the experience of professionals both in understanding the organizational constraints and their well-being at work.
integrates a process of care approach through the modeling and improvement of the care pathway by continuously integrating the latest knowledge and information to support clinical decision-making and by defining feedback loops to continuously improve clinical and non-clinical process supported by operation management contained within process improvement methodology approaches;
embeds coordination structures through: the implementation of best practices and the translation of guidelines into daily practice; the support of informational continuity through the integration of services at the systemic level; the implementation of knowledge management along the care continuum; and the identification of leaders at each step of the care pathway;
adapts to the contexts of both the physical and social structures by integrating the human, material, economic and financial resource constraints, as well as the social dynamics of power and trust relationships;
is supported by information systems and data management, enabled by digitalization, which ensure the flow of information within the right context at the right time and place, and allows the continuous integration of the latest knowledge into the care flow and the management of accessible data in real time to monitor and evaluate variances in practices and outcomes;
promotes the development of a learning health system to support the care pathway.
The aim and shared goal of a care pathway is to meet the needs and expectations of patients through continuous improvement of patient experience, patient outcomes, quality and safety while taking into account operational and social realities of the system.”
We know that this definition is important but feel that there is a great need for clarification of this concept and how these interventions can be successful given the costs involved. Furthermore, we consider that the proper sequencing of the care pathway should be defined according to the following eight phases: (1) Prevention and screening; (2) Signs and symptoms; (3) Early detection; (4) Diagnostic; (5) Referral systems; (6) Treatment; (7) Follow-ups; (8) Reeducation or Palliative care. In this way, the development of recognized KPIs enabling international comparisons of care pathways should finally make it possible to share knowledge and improve care pathways.
According to this definition and based on the literature review, we propose the following integrative conceptual framework illustrated in Fig. 7 .
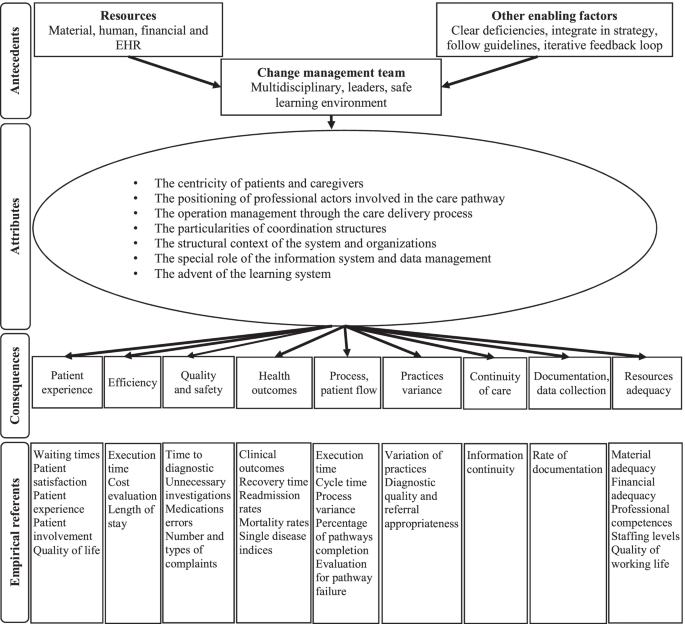
Integrative conceptual framework of care pathway
Using systematic review, concept analysis and bibliometric analysis, it was possible to develop a detailed understanding of the care pathway concept enabling us to propose an integrative conceptual framework and definition to try to meet the need for an international consensus and thus enabling international comparisons and improvement of care pathways.
The results of our work have highlighted the evolution and advances of the various uses of care pathways. Initially focused more on an organizational approach, there is growing support in the literature for a holistic approach that addresses the entire care across the continuum at the system level [ 4 , 24 , 42 , 60 ]. Thus, patient centeredness has become the primary focus as more and more authors focus on the patient experience as the unit of quality analysis. In doing so, they have given greater importance to social relationships and especially to the relationship as a basic need and highlighted the need to design the service line structures mirroring patients’ needs [ 56 ]. They therefore approach the patient, not only as the individual who follows the pathway, but as a social being who has needs and expectations to fulfill, making meeting the needs and expectations of the patient and caregivers the core of the care pathway [ 24 , 50 , 51 , 57 ]. However, the evaluation of the quality of healthcare services by the patient still raises several methodological questions to finally go beyond the simple consideration of satisfaction. Finally, patient and public involvement and patient engagement are also important issues to the point that some authors see a real power struggle between patients and clinicians [ 53 ] that can lead to tokenistic involvement.
The professional actors involved in the care pathway are naturally essential players, both because of their professional competencies and their ability to orient themselves towards the needs of the patient. However, they are also often part of a neglected factor. Some authors have shown one of the key criteria for the potential failure of care pathways is a failure to take into account the prevailing social dynamics and the importance of the buy-in of all stakeholders [ 65 ]. Moreover, some authors insist on the importance of the actors involved in the pathway to both integrate the social dynamics and confront the patient’s needs with operational realities and organizational constraints [ 24 ].
The operation management of process approach to care delivery also raises many challenges. Thus, some authors have developed tools for modeling and improving care processes by applying them in a systemic approach to incorporate clinical decision support into the modeling method [ 60 ]. This issue of continuous integration of updated guidelines into care pathways is indeed a major challenge given the rapid evolution of knowledge and the limited capacity of professionals to continuously integrate new knowledge. In addition, data simulation and data analysis methods coupled with process improvement methods are undeniable contributions to improve the issue of fluidity of processes and therefore the overall performance [ 49 ]. However, one of the pitfalls of staying focused on the process would be a failure to consider the social dimension, particularly the prevailing social dynamics.
Coordination structures are one of the points of improvement in the systemic approach. Ensuring the continuity of information along the care pathway, as well as having a formal leader for each portion of the pathway, would solve many of the problems of path breaks or unnecessary repetition of exams that cause unnecessary costs [ 5 , 56 , 59 ]. This begins with the implementation of a single information system and the integration of IT infrastructures across the entire care pathway at the system level and accessible to care professionals as well as patients and caregivers [ 4 , 50 , 51 , 65 ].
The structural context of the system and organizations cannot be neglected because it directly impacts the results of the implementation of the care pathway. Firstly, because some physical constraints such as distances between several organizational entities [ 12 , 14 ] can only be solved by major transformations in the infrastructures or in the initial process. Secondly, because failing to consider the dominant social dynamics could immediately call into question the entire care pathway intervention [ 3 , 24 ] by implementing only cosmetic changes and not transforming clinical, administrative and organizational practices in a sustainable manner.
The information system plays a special role in care pathway, not only because it is the support of the informational continuity, but also because it enables real-time data analysis to support decision-making within the care pathway in the form of feedback loops [ 4 , 24 , 51 ].
Finally, it seems clear that care pathway programs at the systemic level are one potential intervention which could benefit from the implementation of a learning system [ 4 ]. Care pathway outcome data can be used as feedback to identify improvement opportunities at various stages of the process or at specific interfaces between stakeholders. This approach makes it possible to support the continuous improvement of the care process.
Given the richness of the contributions of the last 20 years, we advocate an integrated approach resulting in a fine-grained and comprehensive understanding of care pathway. Our proposal is compatible with the definition of Vanhaecht et al. [ 25 ] currently used by the EPA, but in our opinion, enriches it. It allows users to specify the operational realities to which stakeholders should pay attention. Moreover, it insists on adaptation to the social realities and the changes that inevitably accompany it and directly impact the success or failure. However, we were surprised that the approach to managing organizational change and transformation of practices were little addressed. Only Van Citters et al. [ 65 ] had noted that change management approaches were critical for successful care transformation and that they had been largely neglected in care pathways. We share this point of view and believe that care pathway intervention leaders must develop communicative action skills to support practices transformation. Not mentioned in the selected literature, we propose to enrich our conceptual framework of communicative action proposed by Habermas [ 79 ]. From our point of view, this dimension could explain the failures of such interventions or at least the difficulty in developing sustainable transformations in practices.
In general, the concept analysis approach has raised several questions about the depth of concept analysis and its place in knowledge advancement [ 80 ]. However, we believe that the combination of systematic review rigor and concept analysis richness, was necessary to meet the aims of this study and produced an integrated conceptual framework which is ready for use. However, this research has some limitations. Although interest is growing, few studies offer comprehensive empirical results on the deployment of a care pathway and its outcomes in a global systemic approach over the entire continuum of care. Moreover, there are a few examples of in-depth analysis of car pathways over a long period of time. Together, this means that the literature still offers little insight into potential outcomes of care pathways. Lastly, our analysis was limited to peer-reviewed articles; including other contributions such as theses and dissertations as well as grey literature could have brought out other categories or themes.
This study has resulted in a fine-grained understanding of care pathways and in a clear definition relying on a powerful conceptual framework. It responds to a strong need for conceptual precision, as previous reviews have not addressed the care pathway on a systemic scale and in a holistic manner. In addition, our framework offers a holistic view of the pathway without being specific to a particular condition or context. Our framework encompasses 28 subcategories grouped into seven care pathway attributes that should be considered in complex care pathway intervention. It considers both operational and social realities and supporting the improvement and sustainable transformation of clinical, administrative, and organizational practices for the benefit of patients and caregivers, while taking into account professional experience, organizational constraints, and social dynamics. The formulation of these attributes, antecedents as success factors and consequences as potential outcomes, linked to their KPIs, allows the operationalization of this model for any pathway in any context. We believe that these results are of particular interest to policymakers, decision makers, managers and researchers alike, and that they could lead to an international consensus that would finally allow comparison of care pathway improvement programs. However, we consider that the development of a framework for analyzing the performance of such an intervention has yet to be developed in a more in-depth manner, such as by focusing on certain particularities of each phase so that managers and decision makers can rely on validated dashboards and KPIs. More empirical work needs to be done on the comprehensive approach, as defined in our proposed definition, to provide reliable results on the ability of these interventions to result in an overall improvement. In addition, the question of the understanding of social evaluation of the quality of care by the patient remains an open question, as the patient experience does not yet have conclusive KPIs as it is too often limited to patient satisfaction or QALYs.
Availability of data and materials
This systematic review is based on an analysis of 44 published papers which are all referenced within this manuscript. Data supporting our findings are included in the form of additional files.
Abbreviations
European Pathway Association
Institute of Medicine of America
Key Performance Indicator
Preferred Reporting Items for Systematic reviews and Meta-Analyses
Quality Adjusted Life Year
World Health Organization
Kelly J, Dwyer J, Mackean T, O’Donnell K, Willis E. Coproducing Aboriginal patient journey mapping tools for improved quality and coordination of care. Aust J Prim Health. 2018;23(6):536–42. https://doi.org/10.1071/PY16069 .
Article Google Scholar
Bergin RJ, Whitfield K, White V, et al. Optimal care pathways: a national policy to improve quality of cancer care and address inequalities in cancer outcomes. J Cancer Policy. 2020;25:100245. https://doi.org/10.1016/j.jcpo.2020.100245 .
Hutchinson K, Herkes G, Shih P, et al. Identification and referral of patients with refractory epilepsy from the primary to the tertiary care interface in New South Wales, Australia. Epilepsy Behav. 2020;111:107232. https://doi.org/10.1016/j.yebeh.2020.107232 .
Article PubMed Google Scholar
Fung-Kee-Fung M, Maziak D, Pantarotto J, et al. Regional process redesign of lung cancer care: a learning health system pilot project. Curr Oncol. 2018;25(1):59–66. https://doi.org/10.3747/co.25.3719 .
Article CAS PubMed PubMed Central Google Scholar
Alkandari M, Ryan K, Hollywood A. The experiences of people living with peripheral neuropathy in Kuwait-a process map of the patient journey. Pharmacy (Basel, Switzerland). 2019;7(3):127. https://doi.org/10.3390/pharmacy7030127 .
Institute of Medicine of America. Committee on quality of health Care in a. crossing the quality chasm: a new health system for the 21st century. Washington, D.C.: National Academy Press; 2001. https://doi.org/10.1136/bmj.323.7322.1192 .
Book Google Scholar
Institute of Medicine of America. Committee on improving the quality of cancer care: addressing the challenges of an aging population, board on healthcare services, Institute of Medicine, delivering high-quality Cancer care: charting a new course for a system in crisis. Washington, D.C.: National Academy Press; 2013. https://doi.org/10.17226/18359 .
National Academies of sciences, engineering, medicine. Committee on improving the quality of health care globally. Crossing the global quality chasm: improving healthcare worldwide. Washington (DC): National Academies Press (US); 2018. https://doi.org/10.17226/25152 .
World Health Organization. Framework on integrated, people-centered health services: report by the secretariat. Geneva, Switzerland: World Health Organization; 2016. https://apps.who.int/gb/ebwha/pdf_files/WHA69/A69_39-en.pdf . Accessed June 16, 2020
Google Scholar
World Health Organization. Report on Cancer: setting priorities, investing wisely and providing Care for all. Geneva, Switzerland: World Health Organization; 2020. https://apps.who.int/iris/handle/10665/330745 . Accessed June 20, 2020
World Health Organization. Guide to developing a national quality policy and strategy: a practical approach to formulating a policy and strategy for quality of care improvement. Geneva, Switzerland: World Health Organization; 2020. https://www.who.int/publications/i/item/9789241565561 . Accessed June 21, 2020
Valentijn PP, Biermann C, Bruijnzeels MA. Value-based integrated (renal) care: setting a development agenda for research and implementation strategies. BMC Health Serv Res. 2016;16(1):1–11. https://doi.org/10.1186/s12913-016-1586-0 .
Ponsignon F, Smart A, Phillips L. A customer journey perspective on service delivery system design: insights from healthcare. Int J Qual Relia Manag. 2018;35(10):2328–47. https://doi.org/10.1108/IJQRM-03-2018-0073 .
Gualandi R, Masella C, Viglione D, Tartaglini D. Exploring the hospital patient journey: what does the patient experience? PLoS One. 2019;14(12):1–15. https://doi.org/10.1371/journal.pone.0224899 .
Article CAS Google Scholar
Schildmeijer K, Frykholm O, Kneck Å, Ekstedt M. Not a straight line-Patients' experiences of prostate Cancer and their journey through the healthcare system. Cancer Nurs. 2019;42(1):E36–43. https://doi.org/10.1097/NCC.0000000000000559 .
Burns K. ISQUA18-2613Patients measuring their experiences with their healthcare system: targeting improvement in access, quality, safety and patient and family Centred care outcomes. Int J Qual Health Care. 2018;30((suppl_2):22–3. https://doi.org/10.1093/intqhc/mzy167.29 .
Nuti S, De Rosis S, Bonciani M, Murante AM. Rethinking healthcare performance evaluation systems towards the people-Centredness approach: their pathways, their experience, their evaluation. Healthc Pap. 2018;17(2):56–64. https://doi.org/10.12927/hcpap.2017.25408 .
Khan AI, Arthurs E, Gradin S, MacKinnon M, Sussman J, Kukreti V. Integrated care planning for cancer patients: a scoping review. Int J Integr Care. 2017;17(6):5. https://doi.org/10.5334/ijic.2543 .
Article PubMed PubMed Central Google Scholar
Ran T, Cheng C-Y, Misselwitz B, Brenner H, Ubels J, Schlander M. Cost-effectiveness of colorectal cancer screening strategies—a systematic review. Clin Gastroenterol Hepatol. 2019;17(10):1969–1981. e1915. https://doi.org/10.1016/j.cgh.2019.01.014 .
Oxford Dictionaries. Concise medical dictionary: Main edition (10th edition). Oxford University Press. 2020. https://www.oxfordreference.com/view/10.1093/acref/9780198836612.001.0001/acref-9780198836612 . Accessed June 18, 2020.
Oxford Dictionaries. A dictionary of nursing (8th edition). Oxford University Press 2021. https://www.oxfordreference.com/view/10.1093/acref/9780198864646.001.0001/acref-9780198864646 . Accessed June 18, 2020.
Visser J, Beech R. Health operations management: patient flow logistics in health care. Psychol Press. 2005. https://doi.org/10.4324/9780203356791 .
Vanhaecht K. The impact of clinical pathways on the organisation of care processes. [PhD Thesis, Katholieke Universiteit Leuven]. 2007; https://lirias.kuleuven.be/1718750?limo=0 . Accessed January 18, 2020.
Carayon P, Wooldridge A, Hoonakker P, Hundt AS, Kelly MM. SEIPS 3.0: human-centered design of the patient journey for patient safety. Appl Ergon. 2020;84:103033. https://doi.org/10.1016/j.apergo.2019.103033 .
Vanhaecht K, Panella M, van Zelm R, Sermeus W. An overview on the history and concept of care pathways as complex interventions. Int J Care Coord. 2010;14(3):117–23. https://doi.org/10.1016/j.apergo.2019.103033 .
Seys D, Panella M, VanZelm R, et al. Care pathways are complex interventions in complex systems: new European pathway association framework. Int J Care Coord. 2019;22(1):5–9. https://doi.org/10.1177/2053434519839195 .
Solomon R, Damba C, Bryant S. Measuring quality at a system level: an impossible task? The Toronto central LHIN experience. Healthc Q. 2013;16(4):36–42.
Rayner J, Khan T, Chan C, Wu C. Illustrating the patient journey through the care continuum: leveraging structured primary care electronic medical record (EMR) data in Ontario, Canada using chronic obstructive pulmonary disease as a case study. Int J Med Inform. 2020;140:104159. https://doi.org/10.1016/j.ijmedinf.2020.104159 .
Galvin M, Madden C, Maguire S, et al. Patient journey to a specialist amyotrophic lateral sclerosis multidisciplinary clinic: an exploratory study. BMC Health Serv Res. 2015;15(1):1–8. https://doi.org/10.1186/s12913-015-1229-x .
McCarthy S, O'Raghallaigh P, Woodworth S, Lim YL, Kenny LC, Adam F. An integrated patient journey mapping tool for embedding quality in healthcare service reform. J Decis Syst. 2016;25:354–68. https://doi.org/10.1080/12460125.2016.1187394 .
Mead N, Bower P. Patient-centredness: a conceptual framework and review of the empirical literature. Soc Sci Med. 2000;51(7):1087–110. https://doi.org/10.1016/S0277-9536(00)00098-8 .
Article CAS PubMed Google Scholar
Stewart M, Brown JB, Weston W, McWhinney IR, McWilliam CL, Freeman T. Patient-centered medicine: transforming the clinical method. Abingdon: Radcliffe Medical Press; 2003.
Van Hoeve JC, Vernooij RW, Fiander M, Nieboer P, Siesling S, Rotter T. Effects of oncological care pathways in primary and secondary care on patient, professional and health systems outcomes: a systematic review and meta-analysis. Syst Rev. 2020;9(1):1–15. https://doi.org/10.1186/s13643-018-0693-x .
Baker E, Woolley A, Xyrichis A, Norton C, Hopkins P, Lee G. How does the implementation of a patient pathway-based intervention in the acute care of blunt thoracic injury impact on patient outcomes? A systematic review of the literature. Injury. 2020;51(8):1733–43. https://doi.org/10.1016/j.injury.2020.06.002 .
Seguin ML, Rangnekar A, Renedo A, Palafox B, McKee M, Balabanova D. Systematic review of frameworks used to conceptualise health pathways of individuals diagnosed with cardiovascular diseases. BMJ Glob Health. 2020;5(9):e002464. https://doi.org/10.1136/bmjgh-2020-002464 .
Beausejour M, Goulet L, Debbie Ehrmann F, et al. Pathways of healthcare utilisation in patients with suspected adolescent idiopathic scoliosis: a cross-sectional study. BMC Health Serv Res. 2015;15:500. https://doi.org/10.1186/s12913-015-1152-1 .
Chan RJ, Webster J, Bowers A. End-of-life care pathways for improving outcomes in caring for the dying. Cochrane Database Syst Rev. 2016;2(2):CD008006. https://doi.org/10.1002/14651858.CD008006.pub4 .
De Bleser L, Depreitere R, Waele KD, Vanhaecht K, Vlayen J, Sermeus W. Defining pathways. J Nurs Manag. 2006;14(7):553–63. https://doi.org/10.1111/j.1365-2934.2006.00702.x .
Page MJ, McKenzie JE, Bossuyt PM, Boutron I, Hoffmann TC, Mulrow CD, et al. The PRISMA 2020 Statement: an updated guideline for reporting systematic reviews. BMJ. 2021:372. https://doi.org/10.1136/bmj.n71 .
Walker L, Avant K, Concept analysis. Strategies for theory construction in nursing (pp. 157–179). Upper Saddle River: Pearson; 2011.
Aromataris E, Munn Z (Editors). Joanna Briggs Institute Reviewer’s Manual (pp. 1-487). The Joanna Briggs Institute; 2017. Available from: https://reviewersmanual.joannabriggs.org/ .
Cherif E, Martin-Verdier E, Rochette C. Investigating the healthcare pathway through patients’ experience and profiles: implications for breast cancer healthcare providers. BMC Health Serv Res. 2020;20:1–11. https://doi.org/10.1186/s12913-020-05569-9 .
Kempa-Liehr AW, Lin CY-C, Britten R, et al. Healthcare pathway discovery and probabilistic machine learning. Int J Med Inform. 2020;137:104087. https://doi.org/10.1016/j.ijmedinf.2020.104087 .
Center for Evidence-Based Management. Critical appraisal of a survey [Available from: https://www.cebma.org/wp-content/uploads/Critical-Appraisal-Questions-for-a-Survey.pdf ]. Accessed September 27, 2020.
Institute for Public Health Sciences. 11 questions to help you make sense of descriptive/cross-sectional studies. Yeshiva University New York; 2002. [Available from: https://reache.files.wordpress.com/2010/03/cross-sectional-appraisal-tool.pdf ]. Accessed October 03, 2020.
Pluye P, Gagnon M-P, Griffiths F, Johnson-Lafleur J. A scoring system for appraising mixed methods research, and concomitantly appraising qualitative, quantitative and mixed methods primary studies in mixed studies reviews. Int J Nurs Stud. 2009;46(4):529–46. https://doi.org/10.1016/j.ijnurstu.2009.01.009 .
Hannes K, Booth A, Harris J, et al. Celebrating methodological challenges and changes: reflecting on the emergence and importance of the role of qualitative evidence in Cochrane reviews. Syst Rev. 2013;2:84. https://doi.org/10.1186/2046-4053-2-84 .
Thomas DR. A general inductive approach for analyzing qualitative evaluation data. Am J Eval. 2006;27(2):237–46. https://doi.org/10.1177/1098214005283748 .
Aspland E, Gartner D, Harper P. Clinical pathway modelling: a literature review. Health Syst. 2020;10(1):1–23. https://doi.org/10.1080/20476965.2019.1652547 .
Busari JO, Yaldiz H, Gans RO, Duits AJ. Clinical leadership as an agent for change: a health system improvement intervention in Curaçao. J Multidiscip Healthc. 2020;13:787–98. https://doi.org/10.2147/JMDH.S262415 .
Devi R, Kanitkar K, Narendhar R, Sehmi K, Subramaniam K. A narrative review of the patient journey through the Lens of non-communicable diseases in low- and middle-income countries. Adv Ther. 2020;37(12):4808–30. https://doi.org/10.1007/s12325-020-01519-3 .
Elkhuizen SG, Vissers JM, Mahdavi M, Van De Klundert JJ. Modeling patient journeys for demand segments in chronic care, with an illustration to type 2 diabetes. Front. Public Health. 2020;8:428. https://doi.org/10.3389/fpubh.2020.00428 eCollection 2020.
Ocloo J, Goodrich J, Tanaka H, Birchall-Searle J, Dawson D, Farr M. The importance of power, context and agency in improving patient experience through a patient and family centred care approach. Health Res Policy Syst. 2020;18(1):10. https://doi.org/10.1186/s12961-019-0487-1 .
Ayachi FL, Rahmouni HB, Ammar MB, Mahjoubi H. A reverse-engineering methodology for medical enhancement processes. Proc Comput Sci. 2019;164:714–23. https://doi.org/10.1016/j.procs.2019.12.240 .
De Belvis AG, Lohmeyer FM, Barbara A, et al. Ischemic stroke: clinical pathway impact. Int J Health Care Qual Assur. 2019;32(3):588–98. https://doi.org/10.1108/IJHCQA-05-2018-0111 .
Louis CJ, Clark JR, Gray B, Brannon D, Parker V. Service line structure and decision-maker attention in three health systems: implications for patient-centered care. Health Care Manag Rev. 2019;44(1):41–56. https://doi.org/10.1097/HMR.0000000000000172 .
Meyer MA. Mapping the patient journey across the continuum: lessons learned from one Patient's experience. J Patient Exp. 2019;6(2):103–7. https://doi.org/10.1177/2374373518783763 .
Mohr P, Galderisi S, Boyer P, et al. Value of schizophrenia treatment I: The patient journey. Eur Psychiatry. 2018;53:107–15. https://doi.org/10.1016/j.eurpsy.2018.06.007 .
Aziz AFA, Nordin NAM, Ali MF, Abd Aziz NA, Sulong S, Aljunid SM. The integrated care pathway for post stroke patients (iCaPPS): a shared care approach between stakeholders in areas with limited access to specialist stroke care services. BMC Health Serv Res. 2017;17(1):35. https://doi.org/10.1186/s12913-016-1963-8 .
Combi C, Oliboni B, Zardini A, Zerbato F. Ieee Seamless Design of Decision-Intensive Care Pathways. 2016:35–45. https://doi.org/10.1109/ICHI.2016.9 .
Gillespie J, McClean S, Garg L, Barton M, Scotney B, Fullerton K. A multi-phase DES modelling framework for patient-centred care. J Oper Res Soc. 2016;67(10):1239–49. https://doi.org/10.1057/jors.2015.114 .
Shaw JM, Price MA, Clayton JM, et al. Developing a clinical pathway for the identification and management of anxiety and depression in adult cancer patients: an online Delphi consensus process. Support Care Cancer. 2016;24(1):33–41. https://doi.org/10.1007/s00520-015-2742-5 .
Walker C, O’Sullivan M, Ziedins I, Furian N. Faster Cancer treatment: using timestamp data to improve patient journeys. Healthc. 2016;4:252–8. https://doi.org/10.1016/j.hjdsi.2016.04.012 .
Grenness C, Hickson L, Laplante-Lévesque A, Davidson B. Patient-centred audiological rehabilitation: perspectives of older adults who own hearing aids. Int J Audiol. 2014;53(sup1):S68–75. https://doi.org/10.3109/14992027.2013.866280 .
Van Citters AD, Fahlman C, Goldmann DA, et al. Developing a pathway for high-value, patient-centered Total joint Arthroplasty. Clin Orthop Relat Res. 2014;472(5):1619–35. https://doi.org/10.1007/s11999-013-3398-4 .
Evans WK, Ung YC, Assouad N, Chyjek A, Sawka C. Improving the quality of lung cancer care in Ontario: the lung cancer disease pathway initiative. J Thorac Oncol. 2013;8(7):876–82. https://doi.org/10.1097/JTO.0b013e31828cb548 .
Huang B, Zhu P, Wu C. Customer-centered careflow modeling based on guidelines. J Med Syst. 2012;36(5):3307–19. https://doi.org/10.1007/s10916-012-9823-5 .
Tehrani J, Liu K, Michel V. Ontology modeling for generation of clinical pathways. J Ind Eng Manag. 2012;5(2):442–56. https://doi.org/10.3926/jiem.586 .
Vandborg MP, Edwards K, Kragstrup J, Vedsted P, Hansen DG, Mogensen O. A new method for analyzing diagnostic delay in gynecological cancer. Int J Gynecol Cancer. 2012;22(5):712–7. https://doi.org/10.1097/IGC.0b013e31824c6d0e .
Yang H, Li W, Liu K, Zhang J. Knowledge-based clinical pathway for medical quality improvement. Inf Syst Front. 2012;14(1):105–17. https://doi.org/10.1007/s10796-011-9307-z .
Manchaiah VK, Stephens D, Meredith R. The patient journey of adults with hearing impairment: the patients’ views. Clin Otolaryngol. 2011;36(3):227–34. https://doi.org/10.1111/j.1749-4486.2011.02320.x .
Yamazaki T, Ikeda M, Umemoto K. Enhancement of healthcare quality using clinical-pathways activities. Vine. 2011;41(1):63–71. https://doi.org/10.1108/03055721111115557 .
Allen D, Gillen E, Rixson L. Systematic review of the effectiveness of integrated care pathways: what works, for whom, in which circumstances? Int J Evid Based Healthc. 2009;7(2):61–74. https://doi.org/10.1111/j.1744-1609.2009.00127.x .
Joosten TC, Bongers IM, Meijboom IBR. Care programmes and integrated care pathways. Int J Health Care Qual Assur. 2008;21(5):472–86. https://doi.org/10.1108/09526860810890440 .
Bond S, Balogh R, McKeever M. Care pathways: integrated clinical record or management information tool? J Integr Care Pathways. 2001;5(2):54–63. https://doi.org/10.1177/147322970100500204 .
World Health Organization. WHO guideline: recommendations on digital interventions for health system strengthening. Geneva: World Health Organization; 2019.
Quinn JB. Intelligent Enterprise: a knowledge and service based paradigm for Industr: Simon and Schuster; 1992.
Senge PM. The fifth discipline: The art and practice of the learning organization (pp. 1-464). Currency; 2006[1990].
Habermas J. The theory of communicative action: Reason and the rationalization of society. Vol 1: Beacon press; 1984.
Lam Wai Shun P, Swaine B, Bottari C. Combining scoping review and concept analysis methodologies to clarify the meaning of rehabilitation potential after acquired brain injury. Disabil Rehabil. 2020:1–9. https://doi.org/10.1080/09638288.2020.1779825 .
Download references
Acknowledgements
Not applicable.
No funding.
Author information
Authors and affiliations.
Département de management, Faculté des sciences de l’administration, Université Laval, 2325 rue de la Terrasse, Québec, QC, G1V 0A6, Canada
Jean-Baptiste Gartner, Kassim Said Abasse & André Côté
Centre de recherche en gestion des services de santé, Université Laval, Québec, QC, Canada
Centre de recherche du CHU de Québec, Université Laval, Québec, QC, Canada
Jean-Baptiste Gartner, Kassim Said Abasse, Paolo Landa & André Côté
Centre de recherche du CISSS de Chaudière-Appalaches, Québec, QC, Canada
Jean-Baptiste Gartner & André Côté
VITAM, Centre de recherche en santé durable, Université Laval, Québec, QC, Canada
Bibliothèque-Direction des services-conseils, Université Laval, Québec, QC, Canada
Frédéric Bergeron
Département d’opérations et systèmes de décision, Université Laval, Québec, QC, Canada
Paolo Landa
Université de Strasbourg, EM Strasbourg-Business School, HuManiS, Strasbourg, France
Célia Lemaire
You can also search for this author in PubMed Google Scholar
Contributions
JBG study selection, appraisal and analysis, review design, manuscript writing, KSA study selection, study appraisal, review design, content discussion and manuscript review, FB review design, content discussion, critical input and manuscript review, PL study analysis, content discussion, critical input and manuscript review, CL study analysis, content discussion, critical input and manuscript review, and AC study analysis, review design, content discussion and manuscript review. All authors read and approved the final manuscript.
Authors’ information
JBG: MSc, PhD candidate in the Department of Management, Laval University; KSA: PhD, Post-doctoral researcher in the Department of Management, Laval University; Frédéric Bergeron: Health Sciences Librarian, Laval University; PL: PhD, Assistant Professor in the Department of Operations and Decision Systems, Laval University; CL: PhD, Associate Professor at the EM Strasbourg Business School, Strasbourg University and AC: PhD, Full Professor & Director of the Centre for Health Services Management Research, Laval University.
Corresponding author
Correspondence to Jean-Baptiste Gartner .
Ethics declarations
Ethics approval and consent to participate, consent for publication, competing interests.
The authors declare that they have no competing interests.
Additional information
Publisher’s note.
Springer Nature remains neutral with regard to jurisdictional claims in published maps and institutional affiliations.
Supplementary Information
Additional file 1..
Search strategy.
Additional file 2.
PRISMA 2020 checklist.
Additional file 3.
Quality appraisal of studies.
Additional file 4.
Concept analysis coding.
Rights and permissions
Open Access This article is licensed under a Creative Commons Attribution 4.0 International License, which permits use, sharing, adaptation, distribution and reproduction in any medium or format, as long as you give appropriate credit to the original author(s) and the source, provide a link to the Creative Commons licence, and indicate if changes were made. The images or other third party material in this article are included in the article's Creative Commons licence, unless indicated otherwise in a credit line to the material. If material is not included in the article's Creative Commons licence and your intended use is not permitted by statutory regulation or exceeds the permitted use, you will need to obtain permission directly from the copyright holder. To view a copy of this licence, visit http://creativecommons.org/licenses/by/4.0/ . The Creative Commons Public Domain Dedication waiver ( http://creativecommons.org/publicdomain/zero/1.0/ ) applies to the data made available in this article, unless otherwise stated in a credit line to the data.
Reprints and permissions
About this article
Cite this article.
Gartner, JB., Abasse, K.S., Bergeron, F. et al. Definition and conceptualization of the patient-centered care pathway, a proposed integrative framework for consensus: a Concept analysis and systematic review. BMC Health Serv Res 22 , 558 (2022). https://doi.org/10.1186/s12913-022-07960-0
Download citation
Received : 06 February 2022
Accepted : 13 April 2022
Published : 26 April 2022
DOI : https://doi.org/10.1186/s12913-022-07960-0
Share this article
Anyone you share the following link with will be able to read this content:
Sorry, a shareable link is not currently available for this article.
Provided by the Springer Nature SharedIt content-sharing initiative
- Care pathway
- Patient journey
- Care process
- Patient-centered
- Healthcare management
- Sustainable transformation
- Learning health system
- Concept analysis
- Systematic review
BMC Health Services Research
ISSN: 1472-6963
- General enquiries: [email protected]
This paper is in the following e-collection/theme issue:
Published on 11.4.2024 in Vol 26 (2024)
Evaluating the Digital Health Experience for Patients in Primary Care: Mixed Methods Study
Authors of this article:

Original Paper
- Melinda Ada Choy 1, 2 , BMed, MMed, DCH, MD ;
- Kathleen O'Brien 1 , BSc, GDipStats, MBBS, DCH ;
- Katelyn Barnes 1, 2 , BAPSC, MND, PhD ;
- Elizabeth Ann Sturgiss 3 , BMed, MPH, MForensMed, PhD ;
- Elizabeth Rieger 1 , BA, MClinPsych, PhD ;
- Kirsty Douglas 1, 2 , MBBS, DipRACOG, Grad Cert HE, MD
1 School of Medicine and Psychology, College of Health and Medicine, The Australian National University, Canberra, Australia
2 Academic Unit of General Practice, Office of Professional Leadership and Education, ACT Health Directorate, Canberra, Australia
3 School of Primary and Allied Health Care, Monash University, Melbourne, Australia
Corresponding Author:
Melinda Ada Choy, BMed, MMed, DCH, MD
School of Medicine and Psychology
College of Health and Medicine
The Australian National University
Phone: 61 51244947
Email: [email protected]
Background: The digital health divide for socioeconomic disadvantage describes a pattern in which patients considered socioeconomically disadvantaged, who are already marginalized through reduced access to face-to-face health care, are additionally hindered through less access to patient-initiated digital health. A comprehensive understanding of how patients with socioeconomic disadvantage access and experience digital health is essential for improving the digital health divide. Primary care patients, especially those with chronic disease, have experience of the stages of initial help seeking and self-management of their health, which renders them a key demographic for research on patient-initiated digital health access.
Objective: This study aims to provide comprehensive primary mixed methods data on the patient experience of barriers to digital health access, with a focus on the digital health divide.
Methods: We applied an exploratory mixed methods design to ensure that our survey was primarily shaped by the experiences of our interviewees. First, we qualitatively explored the experience of digital health for 19 patients with socioeconomic disadvantage and chronic disease and second, we quantitatively measured some of these findings by designing and administering a survey to 487 Australian general practice patients from 24 general practices.
Results: In our qualitative first phase, the key barriers found to accessing digital health included (1) strong patient preference for human-based health services; (2) low trust in digital health services; (3) high financial costs of necessary tools, maintenance, and repairs; (4) poor publicly available internet access options; (5) reduced capacity to engage due to increased life pressures; and (6) low self-efficacy and confidence in using digital health. In our quantitative second phase, 31% (151/487) of the survey participants were found to have never used a form of digital health, while 10.7% (52/487) were low- to medium-frequency users and 48.5% (236/487) were high-frequency users. High-frequency users were more likely to be interested in digital health and had higher self-efficacy. Low-frequency users were more likely to report difficulty affording the financial costs needed for digital access.
Conclusions: While general digital interest, financial cost, and digital health literacy and empowerment are clear factors in digital health access in a broad primary care population, the digital health divide is also facilitated in part by a stepped series of complex and cumulative barriers. Genuinely improving digital health access for 1 cohort or even 1 person requires a series of multiple different interventions tailored to specific sequential barriers. Within primary care, patient-centered care that continues to recognize the complex individual needs of, and barriers facing, each patient should be part of addressing the digital health divide.
Introduction
The promise of ehealth.
The rapid growth of digital health, sped up by the COVID-19 pandemic and associated lockdowns, brings the promise of improved health care efficiency, empowerment of consumers, and health care equity [ 1 ]. Digital health is the use of information and communication technology to improve health [ 2 ]. eHealth, which is a type of digital health, refers to the use of internet-based technology for health care and can be used by systems, providers, and patients [ 2 ]. At the time of this study (before COVID-19), examples of eHealth used by patients in Australia included searching for web-based health information, booking appointments on the web, participating in online peer-support health forums, using mobile phone health apps (mobile health), emailing health care providers, and patient portals for electronic health records.
Digital health is expected to improve chronic disease management and has already shown great potential in improving chronic disease health outcomes [ 3 , 4 ]. Just under half of the Australian population (47.3%) has at least 1 chronic disease [ 5 ]. Rates of chronic disease and complications from chronic disease are overrepresented among those with socioeconomic disadvantage [ 6 ]. Therefore, patients with chronic disease and socioeconomic disadvantage have a greater need for the potential benefits of digital health, such as an improvement in their health outcomes. However, there is a risk that those who could benefit most from digital health services are the least likely to receive them, exemplifying the inverse care law in the digital age by Hart [ 7 ].
Our Current Understanding of the Digital Health Divide
While the rapid growth of digital health brings the promise of health care equity, it may also intensify existing inequities [ 8 ]. The digital health divide for socioeconomic disadvantage describes a pattern in which patients considered socioeconomically disadvantaged who are already marginalized through poor access to traditional health care are additionally hindered through poor access to digital health [ 9 ]. In Australia, only 67.4% of households in the lowest household income quintile have home internet access, compared to 86% of the general population and 96.9% of households in the highest household income quintile [ 10 ]. Survey-based studies have also shown that even with internet access, effective eHealth use is lower in populations considered disadvantaged, which speaks to broader barriers to digital health access [ 11 ].
The ongoing COVID-19 global pandemic has sped up digital health transitions with the rapid uptake of telephone and video consultations, e-prescription, and the ongoing rollout of e-mental health in Australia. These have supported the continuation of health care delivery while limiting physical contact and the pandemic spread; however, the early evidence shows that the digital health divide remains problematic. A rapid review identified challenges with reduced digital access and digital literacy among the older adults and racial and ethnic minority groups, which are both groups at greater health risk from COVID-19 infections [ 12 ]. An Australian population study showed that the rapid uptake of telehealth during peak pandemic was not uniform, with the older adults, very young, and those with limited English language proficiency having a lower uptake of general practitioner (GP) telehealth services [ 13 ].
To ensure that digital health improves health care outcome gaps, it is essential to better understand the nature and nuance of the digital health divide for socioeconomic disadvantage. The nature of the digital health divide for socioeconomic disadvantage has been explored primarily through quantitative survey data, some qualitative papers, a few mixed methods papers, and systematic reviews [ 11 , 14 - 16 ]. Identified barriers include a lack of physical hardware and adequate internet bandwidth, a reduced inclination to seek out digital health, and a low ability and confidence to use digital health effectively [ 16 ]. The few mixed methods studies that exist on the digital health divide generally triangulate quantitative and qualitative data on a specific disease type or population subgroup to draw a combined conclusion [ 17 , 18 ]. These studies have found digital health access to be associated with education, ethnicity, and gender as well as trust, complementary face-to-face services, and the desire for alternative sources of information [ 17 , 19 ].
What This Work Adds
This project sought to extend previous research by using an exploratory mixed methods design to ensure that the first step and driver of our survey of a larger population was primarily shaped by the experiences of our interviewees within primary care. This differs from the triangulation method, which places the qualitative and quantitative data as equal first contributors to the findings and does not allow one type of data to determine the direction of the other [ 18 ]. We qualitatively explored the experience of digital health for patients with socioeconomic disadvantage and chronic disease and then quantitatively measured some of the qualitative findings via a survey of the Australian general practice patient population. Our key objective was to provide comprehensive primary mixed methods data, describing the experience and extent of barriers to accessing digital health and its benefits, with a focus on the digital health divide. We completed this research in a primary care context to investigate a diverse community-based population with conceivable reasons to seek digital help in managing their health. Findings from this mixed methods study were intended to provide health care providers and policy makers with a more detailed understanding of how specific barriers affect different aspects or steps of accessing digital health. Ultimately, understanding digital health access can influence the future design and implementation of digital health services by more effectively avoiding certain barriers or building in enablers to achieve improved digital health access not only for everyone but also especially for those in need.
Study Design
We conducted a sequential exploratory mixed methods study to explore a complex phenomenon in depth and then measure its prevalence. We qualitatively explored the experience of digital health for patients with chronic disease and socioeconomic disadvantage in the first phase. Data from the first phase informed a quantitative survey of the phenomenon across a wider population in the second phase [ 18 ]. Both stages of research were conducted before the COVID-19 pandemic in Australia.
Recruitment
Qualitative phase participants.
The eligibility criteria for the qualitative phase were as follows: English-speaking adults aged ≥18 years with at least 1 self-reported chronic disease and 1 marker of socioeconomic disadvantage (indicated by ownership of a Health Care Card or receiving a disability pension, unemployment, or a user of public housing). A chronic disease was defined to potential participants as a diagnosed long-term health condition that had lasted at least 6 months (or is expected to last for at least 6 months; examples are listed in Multimedia Appendix 1 ). The markers of socioeconomic disadvantage we used to identify potential participants were based on criteria typically used by local general practices to determine which patients can have lower or no out-of-pocket expenses. Apart from unemployment, the 3 other criteria to identify socioeconomic disadvantage are means-tested government-allocated public social services [ 20 ]. Qualitative phase participants were recruited from May to July 2019 through 3 general practices and 1 service organization that serve populations considered socioeconomically disadvantaged across urban, regional, and rural regions in the Australian Capital Territory and South Eastern New South Wales. A total of 2 recruitment methods were used in consultation with and as per the choice of the participating organizations. Potential participants were either provided with an opportunity to engage with researchers (KB and MAC) in the general practice waiting room or identified by the practice or organization as suitable for an interview. Interested participants were given a detailed verbal and written description of the project in a private space before providing written consent to be interviewed. All interview participants received an Aus $50 (US $32.68) grocery shopping voucher in acknowledgment of their time.
Quantitative Phase Participants
Eligibility for the quantitative phase was English-speaking adults aged ≥18 years. The eligibility criteria for the quantitative phase were deliberately broader than those for the qualitative phase to achieve a larger sample size within the limitations of recruitment and with the intention that the factors of socioeconomic disadvantage and having a chronic disease could be compared to the digital health access of a more general population. The quantitative phase participants were recruited from November 2019 to February 2020. Study information and paper-based surveys were distributed and collected through 24 general practices across the Australian Capital Territory and South Eastern New South Wales regions, with an option for web-based completion.
Ethical Considerations
Qualitative and quantitative phase research protocols, including the participant information sheet, were approved by the Australian Capital Territory Health Human Research Ethics Committee (2019/ETH/00013) and the Australian National University Human Research Ethics Committee (2019/ETH00003). Qualitative phase participants were given a verbal and written explanation of the study, including how and when they could opt out, before they provided written consent. All interview participants received an Aus $50 (US $32.68) grocery shopping voucher in acknowledgment of their time. Quantitative participants were given a written explanation and their informed consent was implied by return of a completed survey. Participants in both phases of the study were told that all their data was deidentified. Consent was implied through the return of a completed survey.
Qualitative Data Collection and Analysis
Participants were purposively sampled to represent a range in age, gender, degree of socioeconomic disadvantage, and experience of digital health. The sampling and sample size were reviewed regularly by the research team as the interviews were being completed to identify potential thematic saturation.
The interview guide was developed by the research team based on a review of the literature and the patient dimensions of the framework of access by Levesque et al [ 21 ]. The framework by Levesque et al [ 21 ] is a conceptualization of health care access comprising 5 service and patient dimensions of accessibility and ability. The patient dimensions are as follows: (1) ability to perceive, (2) ability to seek, (3) ability to reach, (4) ability to pay, and (5) ability to engage [ 21 ]. The key interview topics included (1) digital health use and access, including facilitators and barriers; (2) attitudes toward digital health; and (3) self-perception of digital health skills and potential training. The interview guide was reviewed for face and content validity by the whole research team, a patient advocate, a digital inclusion charity representative, and the general practices where recruitment occurred. The questions and guide were iteratively refined by the research team to ensure relevance and support reaching data saturation. The interview guide has been provided as Multimedia Appendix 1 . The interviews, which took 45 minutes on average, were taped and transcribed. An interview summary sheet and reflective journal were completed by the interviewer after each interview to also capture nonverbal cues and tone.
Interview transcriptions were coded and processed by inductive thematic analysis. Data collection and analysis were completed in parallel to support the identification of data saturation. Data saturation was defined as no significant new information arising from new interviews and was identified by discussion with the research team [ 22 ]. The 2 interviewers (MAC and KB) independently coded the first 5 transcripts and reflected on them with another researcher (EAS) to ensure intercoder validity and reliability. The rest of the interviews were coded independently by the 2 interviewers, who regularly met to reflect on emerging themes and thematic saturation. Data saturation was initially indicated after 15 interviews and subsequently confirmed with a total of 19 interviews. Coding disagreements and theme development were discussed with at least 1 other researcher (EAS, ER, and KD). Thematic saturation and the final themes were agreed upon by the entire research team.
Quantitative Survey Development
The final themes derived in the qualitative phase of the project guided the specific quantitative phase research questions. The final themes were a list of ordered cumulative barriers experienced by participants in accessing digital health and its benefits ( Figure 1 ). The quantitative survey was designed to test the association between barriers to access and the frequency of use of digital health as a proxy measure for digital health access.

In the survey, the participants were asked about their demographic details, health and chronic diseases, knowledge, use and experience of digital health tools, internet access, perception of digital resource affordability, trust in digital health and traditional health services, perceived capability, health care empowerment, eHealth literacy, and relationship with their GP.
Existing scales and questions from the literature and standardized Australian-based surveys were used whenever possible. We used selected questions and scales from the Australian Bureau of Statistics standards, the eHealth Literacy Scale (eHEALS), the eHealth Literacy Questionnaire, and the Southgate Institute for Health Society and Equity [ 17 , 23 - 26 ]. We adapted other scales from the ICEpop Capability Measure for Adults, the Health Care Empowerment Inventory (HCEI), the Patient-Doctor Relationship Questionnaire, and the Chao continuity questionnaire [ 23 , 27 - 29 ]. Where an existing scale to measure a barrier or theme did not exist, the research team designed the questions based on the literature. Our questions around the frequency of digital health use were informed by multiple existing Australian-based surveys on general technology use [ 30 , 31 ]. Most of the questions used a Likert scale. Every choice regarding the design, adaptation, or copy of questions for the survey was influenced by the qualitative findings and decided on by full agreement among the 2 researchers who completed and coded the interviews. A complete copy of the survey is provided in Multimedia Appendix 2 .
Pilot-testing of the survey was completed with 5 patients, 2 experts on digital inclusion, and 3 local GPs for both the paper surveys and web-based surveys via Qualtrics Core XM (Qualtrics LLC). The resulting feedback on face and content validity, functionality of the survey logic, and feasibility of questionnaire completion was incorporated into the final version of the survey.
The survey was offered on paper with a participant information sheet, which gave the patients the option to complete the web-based survey. The survey was handed out to every patient on paper to avoid sampling bias through the exclusion of participants who could not complete the web-based survey [ 32 ].
Quantitative Data Treatment and Analysis
Data were exported from Qualtrics Core XM to an SPSS (version 26; IBM Corp) data set. Data cleaning and screening were undertaken (KB and KO).
Descriptive statistics (number and percentage) were used to summarize participant characteristics, preference measures, and frequency of eHealth use. Significance testing was conducted using chi-square tests, with a threshold of P <.05; effect sizes were measured by the φ coefficient for 2×2 comparisons and Cramer V statistic for all others. Where the cells sizes were too small, the categories were collapsed for the purposes of significance testing. The interpretation of effect sizes was as per the study by Cohen [ 33 ]. The analysis was conducted in SPSS and SAS (version 9.4; SAS Institute).
Participant Characteristics
Participants’ self-reported characteristics included gender, indigenous status, income category, highest level of education, marital status, and language spoken at home.
Age was derived from participant-reported year of birth and year of survey completion as of 2019 and stratified into age groups. The state or territory of residence was derived from the participant-reported postcode. The remoteness area was derived using the postcode reported by the participants and mapped to a modified concordance from the Australian Bureau of Statistics. Occupation-free text responses were coded using the Australian Bureau of Statistics Census statistics level 1 and 2 descriptors. The country of birth was mapped to Australia, other Organisation for Economic Cooperation and Development countries, and non–Organisation for Economic Cooperation and Development countries.
Frequency of eHealth Use
A summary measure of the frequency of eHealth use was derived from the questions on the use of different types of eHealth.
Specifically, respondents were asked if they had ever used any form of web-based health (“eHealth“) and, if so, to rate how often (never, at least once, every now and then, and most days) against 6 types of “eHealth” (searching for health information online, booking appointments online, emailing health care providers, using health-related mobile phone apps, accessing My Health Record, and accessing online health forums). The frequency of eHealth use was then classified as follows:
- High user: answered “most days” to at least 1 question on eHealth use OR answered “every now and then” to at least 2 questions on eHealth use
- Never user: answered “no” to having ever used any form of eHealth OR “never” to all 6 questions on eHealth use
- Low or medium user: all other respondents.
The frequency of eHealth use was reported as unweighted descriptive statistics (counts and percentages) against demographic characteristics and for the elements of each of the themes identified in phase 1.
Overview of Key Themes
Data were reported against the 6 themes from the phase 1 results of preference, trust, cost, structural access, capacity to engage, and self-efficacy. Where the components of trust, cost, capacity to engage, and self-efficacy had missing data (for less than half of the components only), mean imputation was used to minimize data loss. For each theme, the analysis excluded those for whom the frequency of eHealth use was unknown.
Preference measures (survey section D1 parts 1 to 3) asked participants to report against measures with a 4-point Likert scale (strongly disagree, disagree, agree, and strongly agree). Chi-square tests were conducted after the categories were condensed into 2 by combining strongly disagree and as well as combining strongly agree and agree.
Summary measures for trust were created in 4 domains: trust from the eHealth Literacy Questionnaire (survey section D1 parts 4 to 8), trust from Southgate—GPs, specialists, or allied health (survey section D2 parts 1 to 5), trust from Southgate—digital health (survey section D2 parts 6, 7, 9, and 10), and trust from Southgate—books or pamphlets (survey section D2 part 8). The data were grouped as low, moderate, and high trust based on the assigned scores from the component data. Chi-square tests were conducted comparing low-to-moderate trust against high trust for GP, specialists, or allied health and comparing low trust against moderate-to-high trust for book or pamphlet.
Summary measures for cost were created from survey item C10. To measure cost, participants were asked about whether they considered certain items or services to be affordable. These included cost items mentioned in the qualitative phase interviews relating to mobile phones (1 that connects to the internet, 1 with enough memory space to download apps, downloads or apps requiring payment, repairs, and maintenance costs), having an iPad or tablet with internet connectivity, a home computer or laptop (owning, repairs, and maintenance), home fixed internet access, and an adequate monthly data allowance. These 9 items were scored as “yes definitely”=1 or 0 otherwise. Chi-square tests were conducted with never and low or medium eHealth users combined.
Structural Access
Structural access included asking where the internet is used by participants (survey section C8) and factors relating to internet access (survey section C8 parts 1-3) reporting against a 4-point Likert scale (strongly disagree, disagree, agree, and strongly agree). Chi-square tests were conducted with strongly disagree, disagree, agree, or strongly agree, and never, low, or medium eHealth use combined.
Capacity to Engage
Summary measures for capacity to engage were created from survey section E1. To measure the capacity to engage, participants were asked about feeling “settled and secure,” “being independent,” and “achievement and progress” as an adaptation of the ICEpop Capability Measure for Adults [ 27 ], reporting against a 4-point Likert-like scale. Responses were scored from 1 (“I am unable to feel settled and secure in any areas of my life”) to 4 (“I am able to feel settled and secure in all areas of my life”).
The summary capacity measure was derived by the summation of responses across the 3 questions, which were classified into 4 groups, A to D, based on these scores. Where fewer than half of the responses were missing, mean imputation was used; otherwise, the record was excluded. Groups A and B were combined for significance testing.
Self-Efficacy
Summary measures for self-efficacy were adapted from the eHEALS (E3) and the HCEI (E2) [ 23 , 24 ].
Survey section E3—eHEALS—comprised 8 questions, with participants reporting against a 5-point Likert scale for each (strongly disagree, disagree, neither, agree, and strongly agree). These responses were assigned 1 to 5 points, respectively. The summary eHEALS measure was derived by the summation of responses across the 8 questions, which were classified into 5 groups, A to E, based on these scores. Where fewer than half of the responses were missing, mean imputation was used; otherwise, the record was excluded. Groups A to C and D to E were combined for significance testing.
Survey section E2—HCEI—comprised 5 questions, with participants reporting against a 5-point Likert scale for each (strongly disagree, disagree, neither, agree, and strongly agree). Strongly disagree and disagree and neither were combined, and similarly agree and strongly agree were combined for significance testing.
Qualitative Results
The demographic characteristics of the patients that we interviewed are presented in Table 1 .
The key barriers found to accessing digital health included (1) strong patient preference for human-based health services; (2) low trust in digital health services; (3) high financial costs of necessary tools, maintenance, and repairs; (4) poor publicly available internet access options; (5) reduced capacity to engage due to increased life pressures; and (6) low self-efficacy and confidence in using digital health.
Rather than being an equal list of factors, our interviewees described these barriers as a stepped series of cumulative hurdles, which is illustrated in Figure 1 . Initial issues of preference and trust were foundational to a person even when considering the option of digital health, while digital health confidence and literacy were barriers to full engagement with and optimal use of digital health. Alternatively, interviewees who did use digital health had been enabled by the same factors that were barriers to others.
a GP: general practitioner.
b Multiple answers per respondent.
Strong Patient Preference for Human-Based Health Services
Some patients expressed a strong preference for human-based health services rather than digital health services. In answer to a question about how digital health services could be improved, a patient said the following:
Well, having an option where you can actually bypass actually having to go through the app and actually talk directly to someone. [Participant #10]
For some patients, this preference for human-based health services appeared to be related to a lack of exposure to eHealth. These patients were not at all interested in or had never thought about digital health options. A participant responded the following to the interviewer’s questions:
Interviewer: So when...something feels not right, how do you find out what’s going on?
Respondent: I talk to Doctor XX.
Interviewer: Do you ever Google your symptoms or look online for information?
Respondent: No, I have never even thought of doing that actually. [Participant #11]
For other patients, their preference for human-based health care stemmed from negative experiences with technology. These patients reported actively disliking computers and technology in general and were generally frustrated with what they saw as the pitfalls of technology. A patient stated the following:
If computers and internet weren’t so frigging slow because everything is on like the slowest speed network ever and there’s ads blocking everything. Ads, (expletive) ads. [Participant #9]
A patient felt that he was pushed out of the workforce due his inability to keep up with technology-based changes and thus made a decision to never own a computer:
But, you know, in those days when I was a lot younger those sorts of things weren’t about and they’re just going ahead in leaps and bounds and that’s one of the reasons why I retired early. I retired at 63 because it was just moving too fast and it’s all computers and all those sorts of things and I just couldn’t keep up. [Participant #17]
Low Trust in Digital Health Services
Several patients described low trust levels for digital and internet-based technology in general. Their low trust was generally based on stories they had heard of other people’s negative experiences. A patient said the following:
I don’t trust the internet to be quite honest. You hear all these stories about people getting ripped off and I’ve worked too hard to get what I’ve got rather than let some clown get it on the internet for me. [Participant #11]
Some of this distrust was specific to eHealth. For example, some patients were highly suspicious of the government’s motives with regard to digital health and were concerned about the privacy of their health information, which made them hesitant about the concept of a universal electronic health record. In response to the interviewer’s question, a participant said the following:
Interviewer: Are there any other ways you think that eHealth might help you?
Respondent: I’m sorry but it just keeps coming back to me, Big Brother. [Participant #7]
Another participant said the following:
I just would run a mile from it because I just wouldn’t trust it. It wouldn’t be used to, as I said, for insurance or job information. [Participant #16]
High Financial Costs of the Necessary Tools, Maintenance, and Repairs
A wide variety of patients described affordability issues across several different aspects of the costs involved in digital health. They expressed difficulty in paying for the following items: a mobile phone that could connect to the internet, a mobile phone with enough memory space to download apps, mobile phone apps requiring extra payment without advertisements, mobile phone repair costs such as a broken screen, a computer or laptop, home internet access, and adequate monthly data allowance and speeds to functionally use the internet. Current popular payment systems, such as plans, were not feasible for some patients. A participant stated the following:
I don’t have a computer...I’m not in the income bracket to own a computer really. Like I could, if I got one on a plan kind of thing or if I saved up for x-amount of time. But then like if I was going on the plan I’d be paying interest for having it on like lay-buy kind of thing, paying it off, and if it ever got lost or stolen I would still have to repay that off, which is always a hassle. And yeah. Yeah, I’m like financially not in the state where I’m able to...own a computer right now as I’m kind of paying off a number of debts. [Participant #9]
Poor Publicly Available Internet Access Options
Some patients described struggling without home internet access. While they noted some cost-free public internet access points, such as libraries, hotel bars, and restaurants, they often found these to be inconvenient, lacking in privacy, and constituting low-quality options for digital health. A patient stated the following:
...it’s incredibly slow at the library. And I know why...a friend I went to school with used to belong to the council and the way they set it up, they just got the raw end of the stick and it is really, really slow. It’s bizarre but you can go to the X Hotel and it’s heaps quicker. [Participant #15]
In response to the interviewer's question, a participant said the following:
Interviewer: And do you feel comfortable doing private stuff on computers at the library...?
Respondent: Not really, no, but I don’t have any other choice, so, yeah. [Participant #9]
Reduced Capacity to Engage Due to Increased Life Pressures
When discussing why they were not using digital health or why they had stopped using digital health, patients often described significant competing priorities and life pressures that affected their capacity to engage. An unemployed patient mentioned that his time and energy on the internet were focused primarily on finding work and that he barely had time to focus on his health in general, let alone engage in digital health.
Other patients reported that they often felt that their ability to learn about and spend time on digital health was taken up by caring for sick family members, paying basic bills, or learning English. Some patients said that the time they would have spent learning digital skills when they were growing up had been lost to adverse life circumstances such as being in jail:
So we didn’t have computers in the house when I was growing up. And I didn’t know I’ve never...I’ve been in and out of jail for 28 odd years so it sort of takes away from learning from this cause it’s a whole different… it’s a whole different way of using a telephone from a prison. [Participant #11]
Low Self-Efficacy and Confidence in Starting the Digital Health Process
Some patients had a pervasive self-perception of being slow learners and being unable to use technology. Their stories of being unconfident learners seemed to stem from the fact that they had been told throughout their lives that they were intellectually behind. A patient said the following:
The computer people...wouldn’t take my calls because I’ve always been dumb with that sort of stuff. Like I only found out this later on in life, but I’m actually severely numerically dyslexic. Like I have to triple-check everything with numbers. [Participant #7]
Another patient stated the following:
I like went to two English classes like a normal English class with all the kids and then another English class with about seven kids in there because I just couldn’t I don’t know maybe because I spoke another language at home and they sort of like know I was a bit backward. [Participant #6]
These patients and others had multiple missing pieces of information that they felt made it harder to engage in digital health compared to “easier” human-based services. A patient said the following:
Yeah I’ve heard of booking online but I just I don’t know I find it easier just to ring up. And I’ll answer an email from a health care provider but I wouldn’t know where to start to look for their email address. [Participant #11]
In contrast, the patients who did connect with digital health described themselves as independent question askers and proactive people. Even when they did not know how to use a specific digital health tool, they were confident in attempting to and asking for help when they needed it. A patient said the following:
I’m a “I will find my way through this, no matter how long it takes me” kind of person. So maybe it’s more my personality...If I have to ask for help from somewhere, wherever it is, I will definitely do that. [Participant #3]
Quantitative Results
A total of 487 valid survey responses were received from participants across 24 general practices. The participant characteristics are presented in detail in Table S1 in Multimedia Appendix 3 .
The mean age of the participants was approximately 50 years (females 48.9, SD 19.4 years; males 52.8, SD 20.0 years), and 68.2% (332/487) of the participants identified as female. Overall, 34.3% (151/439) of respondents reported never using eHealth, and 53.8% (236/439) reported high eHealth use.
There were statistically significant ( P <.05) differences in the frequency of eHealth use in terms of age group, gender, state, remoteness, highest level of education, employment status, occupation group, marital status, and language spoken at home, with effect sizes being small to medium. Specifically, high eHealth characteristics were associated with younger age, being female, living in an urban area, and being employed.
Table 2 presents the frequency of eHealth use against 3 internet preference questions.
Preference for using the internet and technology in general and for health needs in particular were significantly related to the frequency of eHealth use ( P <.05 for each), with the effect sizes being small to medium.
a Excludes those for whom frequency of eHealth use is unknown.
b Chi-square tests conducted with strongly disagree and disagree combined, and agree and strongly agree combined.
Table 3 presents the frequency of eHealth use against 4 measures of trust.
The degree of trust was not statistically significantly different for the frequency of eHealth use for any of the domains.
b eHLQ: eHealth Literacy Questionnaire.
c Derived from survey question D1, parts 4 to 8. Mean imputation used where ≤2 responses were missing. If >2 responses were missing, the records were excluded.
d Derived from survey question D2, parts 1 to 5. Mean imputation used where ≤2 responses were missing. If >2 responses were missing, the records were excluded.
e Chi-square test conducted comparing low-to-moderate trust against high trust.
f Derived from survey question D2, parts 6, 7, 9, and 10. Mean imputation used where ≤2 responses were missing. If >2 responses were missing, the records were excluded.
g Derived from survey question D2 part 8.
h Chi-square test conducted comparing low trust against moderate-to-high trust.
Affordability of items and services was reported as No cost difficulty or Cost difficulty. eHealth frequency of use responses were available for 273 participants; among those with no cost difficulty , 1% (2/204) were never users, 14.2% (29/204) were low or medium users, and 84.8% (173/204) were high users of eHealth; among those with cost difficulty , 1% (1/69) were never users, 26% (18/69) were low or medium users, and 73% (50/69) were high users. There was a statistically significant difference in the presence of cost as a barrier between never and low or medium eHealth users compared to high users ( χ 2 1 =5.25; P =.02), although the effect size was small.
Table 4 presents the frequency of eHealth use for elements of structural access.
Quality of internet access and feeling limited in access to the internet were significantly associated with frequency of eHealth use ( P <.05), although the effect sizes were small.
b N/A: not applicable (cell sizes insufficient for chi-square test).
c Chi-square tests conducted with strongly disagree and disagree combined, agree and strongly agree combined, and never and low or medium categories combined.
Table 5 presents the frequency of eHealth use against respondents’ capacity to engage.
Capacity to engage was not significantly different for the frequency of eHealth use ( P =.54).
b Derived from survey item E1. Where 1 response was missing, the mean imputation was used. If >1 response was missing, the record was excluded.
c Chi-square tests conducted with groups A and B combined.
Table 6 presents the frequency of eHealth use for elements of self-efficacy.
Statistically significant results were observed for the relationship between self-efficacy by eHEALS (moderate effect size) and frequency of eHealth use as well as for some of the questions from the HCEI (reliance on health professionals or others to access and explain information; small effect size; P <.05).
b eHEALS: eHealth Literacy Scale.
c eHEALS derived from item E3 (8 parts). Where ≤ 4 responses were missing, mean imputation was used. If >4 responses were missing, the records were excluded. Groups A to C as well as groups D to E were combined for the chi-square test.
d Strongly disagree, disagree, neither, and agree or strongly agree combined for significance testing.
Principal Findings
This paper reports on the findings of a sequential exploratory mixed methods study on the barriers to digital health access for a group of patients in Australian family medicine, with a particular focus on chronic disease and socioeconomic disadvantage.
In the qualitative first phase, the patients with socioeconomic disadvantage and chronic disease described 6 cumulative barriers, as demonstrated in Figure 1 . Many nonusers of digital health preferred human-based services and were not interested in technology, while others were highly suspicious of the technology in general. Some digitally interested patients could not afford quality hardware and internet connectivity, a barrier that was doubled by low quality and privacy when accessing publicly available internet connections. Furthermore, although some digitally interested patients had internet access, their urgent life circumstances left scarce opportunity to access digital health and develop digital health skills and confidence.
In our quantitative second phase, 31% (151/487) of the survey participants from Australian general practices were found to have never used a form of digital health. Survey participants were more likely to use digital health tools frequently when they also had a general digital interest and a digital health interest. Those who did not frequently access digital health were more likely to report difficulty affording the financial costs needed for digital access. The survey participants who frequently accessed digital health were more likely to have high eHealth literacy and high levels of patient empowerment.
Comparison With Prior Work
In terms of general digital health access, the finding that 31% (151/487) of the survey participants had never used one of the described forms of eHealth is in keeping with an Australian-based general digital participation study that found that approximately 9% of the participants were nonusers and 17% rarely engaged with the internet at all [ 34 ]. With regard to the digital health divide, another Australian-based digital health divide study found that increased age, living in a lower socioeconomic area, being Aboriginal or Torres Strait Islander, being male, and having no tertiary education were factors negatively associated with access to digital health services [ 17 ]. Their findings correspond to our findings that higher-frequency users of eHealth were associated with younger age, being female, living in an urban area, and being employed. Both studies reinforce the evidence of the digital health divide based on gender, age, and socioeconomic disadvantage in Australia.
With regard to digital health barriers, our findings provide expanded details on the range of digital health items and services that present a cost barrier to consumers. Affordability is a known factor in digital access and digital health access, and it is measured often by general self-report or relative expenditure on internet access to income [ 30 ]. Our study revealed the comprehensive list of relevant costs for patients. Our study also demonstrated factors of cost affordability beyond the dollar value of an item, as interviewees described the struggle of using slow public internet access without privacy features and the risks involved in buying a computer in installments. When we reflected on the complexity and detail of the cost barrier in our survey, participants demonstrated a clear association between cost and the frequency of digital health use. This suggests that a way to improve digital health access for some people is to improve the quality, security, and accessibility of public internet access options as well as to provide free or subsidized hardware, internet connection, and maintenance options for those in need, work that is being done by at least 1 digital inclusion charity in the United Kingdom [ 35 ].
Many studies recognize the factors of eHealth literacy and digital confidence for beneficial digital health access [ 36 ]. Our interviews demonstrated that some patients with socioeconomic disadvantage have low digital confidence, but that this is often underlined by a socially reinforced lifelong low self-confidence in their intellectual ability. In contrast, active users, regardless of other demographic factors, described themselves as innately proactive question askers. This was reinforced by our finding of a relationship between health care empowerment and the frequency of eHealth use. This suggests that while digital health education and eHealth literacy programs can improve access for some patients, broader and deeper long-term solutions addressing socioeconomic drivers of digital exclusion are needed to improve digital health access for some patients with socioeconomic disadvantage [ 8 ]. The deep permeation of socially enforced low self-confidence and lifelong poverty experienced by some interviewees demonstrate that the provision of free hardware and a class on digital health skills can be, for some, a superficial offering when the key underlying factor is persistent general socioeconomic inequality.
The digital health divide literature tends to identify the digital health divide, the factors and barriers that contribute to it, and the potential for it to widen if not specifically addressed [ 16 ]. Our findings have also identified the divide and the barriers, but what this study adds through our qualitative phase in particular is a description of the complex interaction of those barriers and the stepped nature of some of those barriers as part of the individual’s experience in trying to access digital health.
Strengths and Limitations
A key strength of this study is the use of a sequential exploratory mixed methods design. The initial qualitative phase guided a phenomenological exploration of digital health access experiences for patients with chronic disease and socioeconomic disadvantage. Our results in both study phases stem from the patients’ real-life experiences of digital health access. While some of our results echo the findings of other survey-based studies on general digital and digital health participation, our method revealed a greater depth and detail of some of these barriers, as demonstrated in how our findings compare to prior work.
As mentioned previously, the emphasis of this study on the qualitative first phase is a strength that helped describe the interactions between different barriers. The interviewees described their experiences as cumulative unequal stepped barriers rather than as producing a nonordered list of equal barriers. These findings expand on the known complexity of the issue of digital exclusion and add weight to the understanding that improving digital health access needs diverse, complex solutions [ 17 ]. There is no panacea for every individual’s digital health access, and thus, patient-centered digital health services, often guided by health professionals within the continuity of primary care, are also required to address the digital health divide [ 37 ].
While the sequential exploratory design is a strength of the study, it also created some limitations for the second quantitative phase. Our commitment to using the qualitative interview findings to inform the survey questions meant that we were unable to use previously validated scales for every question and that our results were less likely to lead to a normal distribution. This likely affected our ability to demonstrate significant associations for some barriers. We expect that further modeling is required to control for baseline characteristics and determine barrier patterns for different types of users.
One strength of this study is that the survey was administered to a broad population of Australian family medicine patients with diverse patterns of health via both paper-based and digital options. Many other digital health studies use solely digital surveys, which can affect the sample. However, we cannot draw conclusions from our survey about patients with chronic disease due to the limitations of the sample size for these subgroups.
Another sample-based limitation of this study was that our qualitative population did not include anyone aged from 18 to 24 years, despite multiple efforts to recruit. Future research will hopefully address this demographic more specifically.
While not strictly a limitation, we recognize that because this research was before COVID-19, it did not include questions about telehealth, which has become much more mainstream in recent years. The patients may also have changed their frequency of eHealth use because of COVID-19 and an increased reliance on digital services in general. Future work in this area or future versions of this survey should include telehealth and acknowledge the impact of COVID-19. However, the larger concept of the digital health divide exists before and after COVID-19, and in fact, our widespread increased reliance on digital services makes the digital divide an even more pressing issue [ 12 ].
Conclusions
The experience of digital health access across Australian primary care is highly variable and more difficult to access for those with socioeconomic disadvantage. While general digital interest, financial cost, and digital health literacy and empowerment are clear factors in digital health access in a broad primary care population, the digital health divide is also facilitated in part by a stepped series of complex and cumulative barriers.
Genuinely improving digital health access for 1 cohort or even 1 person requires a series of multiple different interventions tailored to specific sequential barriers. Given the rapid expansion of digital health during the global COVID-19 pandemic, attention to these issues is necessary if we are to avoid entrenching inequities in access to health care. Within primary care, patient-centered care that continues to recognize the complex individual needs of, and barriers facing, each patient should be a part of addressing the digital health divide.
Acknowledgments
The authors are thankful to the patients who shared their experiences with them via interview and survey completion. The authors are also very grateful to the general practices in the Australian Capital Territory and New South Wales who kindly gave their time and effort to help organize interviews, administer, and post surveys in the midst of the stress of day-to-day practice life and the bushfires of 2018-2019. The authors thank and acknowledge the creators of the eHealth Literacy Scale, the eHealth Literacy Questionnaire, the ICEpop Capability Measure for Adults, the Health Care Empowerment Inventory, the Patient-Doctor Relationship Questionnaire, the Chao continuity questionnaire, and the Southgate Institute for Health Society and Equity for their generosity in sharing their work with the authors [ 17 , 19 - 25 ]. This study would not have been possible without the support of the administrative team of the Academic Unit of General Practice. This project was funded by the Royal Australian College of General Practitioners (RACGP) through the RACGP Foundation IPN Medical Centres Grant, and the authors gratefully acknowledge their support.
Data Availability
The data sets generated during this study are not publicly available due to the nature of our original ethics approval but are available from the corresponding author on reasonable request.
Authors' Contributions
MAC acquired the funding, conceptualized the project, and organized interview recruitment. MAC and KB conducted interviews and analyzed the qualitative data. EAS, ER, and KD contributed to project planning, supervision and qualitative data analysis. MAC, KB and KO wrote the survey and planned quantitative data analysis. MAC and KB recruited practices for survey administration. KO and KB conducted the quantitative data analysis. MAC and KO, with KB drafted the paper. EAS, ER, and KD helped with reviewing and editing the paper.
Conflicts of Interest
None declared.
Phase 1 interview guide.
Phase 2 survey: eHealth and digital divide.
Phase 2 participant characteristics by frequency of eHealth use.
- Eysenbach G. What is e-health? J Med Internet Res. 2001;3(2):E20. [ FREE Full text ] [ CrossRef ] [ Medline ]
- Iyawa GE, Herselman M, Botha A. Digital health innovation ecosystems: from systematic literature review to conceptual framework. Procedia Comput Sci. 2016;100:244-252. [ FREE Full text ] [ CrossRef ]
- Berrouiguet S, Baca-García E, Brandt S, Walter M, Courtet P. Fundamentals for future mobile-health (mHealth): a systematic review of mobile phone and web-based text messaging in mental health. J Med Internet Res. Jun 10, 2016;18(6):e135. [ FREE Full text ] [ CrossRef ] [ Medline ]
- Shen H, van der Kleij RM, van der Boog PJ, Chang X, Chavannes NH. Electronic health self-management interventions for patients with chronic kidney disease: systematic review of quantitative and qualitative evidence. J Med Internet Res. Nov 05, 2019;21(11):e12384. [ FREE Full text ] [ CrossRef ] [ Medline ]
- Australia's health 2018. Australian Institute of Health and Welfare. 2018. URL: https://www.aihw.gov.au/reports/australias-health/australias-health-2018/contents/table-of-contents [accessed 2024-04-04]
- Australian Institute of Health and Welfare. Chronic Diseases and Associated Risk Factors in Australia, 2006. Canberra, Australia. Australian Institute of Health and Welfare; 2006.
- Hart JT. The inverse care law. The Lancet. Feb 27, 1971;297(7696):405-412. [ CrossRef ]
- Davies AR, Honeyman M, Gann B. Addressing the digital inverse care law in the time of COVID-19: potential for digital technology to exacerbate or mitigate health inequalities. J Med Internet Res. Apr 07, 2021;23(4):e21726. [ FREE Full text ] [ CrossRef ] [ Medline ]
- Choi NG, Dinitto DM. The digital divide among low-income homebound older adults: internet use patterns, eHealth literacy, and attitudes toward computer/internet use. J Med Internet Res. May 02, 2013;15(5):e93. [ FREE Full text ] [ CrossRef ] [ Medline ]
- Household use of information technology. Australian Bureau of Statistics. 2018. URL: https://tinyurl.com/4efm6u92 [accessed 2024-03-24]
- Kontos E, Blake KD, Chou WY, Prestin A. Predictors of eHealth usage: insights on the digital divide from the health information national trends survey 2012. J Med Internet Res. Jul 16, 2014;16(7):e172. [ FREE Full text ] [ CrossRef ] [ Medline ]
- Litchfield I, Shukla D, Greenfield S. Impact of COVID-19 on the digital divide: a rapid review. BMJ Open. Oct 12, 2021;11(10):e053440. [ FREE Full text ] [ CrossRef ] [ Medline ]
- Butler DC, Joshy G, Douglas KA, Sayeed MS, Welsh J, Douglas A, et al. Changes in general practice use and costs with COVID-19 and telehealth initiatives: analysis of Australian whole-population linked data. Br J Gen Pract. Apr 27, 2023;73(730):e364-e373. [ FREE Full text ] [ CrossRef ] [ Medline ]
- Arsenijevic J, Tummers L, Bosma N. Adherence to electronic health tools among vulnerable groups: systematic literature review and meta-analysis. J Med Internet Res. Feb 06, 2020;22(2):e11613. [ FREE Full text ] [ CrossRef ] [ Medline ]
- Kontos EZ, Bennett GG, Viswanath K. Barriers and facilitators to home computer and internet use among urban novice computer users of low socioeconomic position. J Med Internet Res. Oct 22, 2007;9(4):e31. [ FREE Full text ] [ CrossRef ] [ Medline ]
- Latulippe K, Hamel C, Giroux D. Social health inequalities and eHealth: a literature review with qualitative synthesis of theoretical and empirical studies. J Med Internet Res. Apr 27, 2017;19(4):e136. [ FREE Full text ] [ CrossRef ] [ Medline ]
- Foley K, Freeman T, Ward P, Lawler A, Osborne R, Fisher M. Exploring access to, use of and benefits from population-oriented digital health services in Australia. Health Promot Int. Aug 30, 2021;36(4):1105-1115. [ CrossRef ] [ Medline ]
- Cresswell JW, Plano Clark VL. Designing and Conducting Mixed Methods Research. Thousand Oaks, CA. SAGE Publications; 2007.
- Tappen RM, Cooley ME, Luckmann R, Panday S. Digital health information disparities in older adults: a mixed methods study. J Racial Ethn Health Disparities. Feb 2022;9(1):82-92. [ FREE Full text ] [ CrossRef ] [ Medline ]
- Who can get a card. Services Australia. URL: https://www.servicesaustralia.gov.au/who-can-get-health-care-card?context=21981 [accessed 2023-11-03]
- Levesque JF, Harris MF, Russell G. Patient-centred access to health care: conceptualising access at the interface of health systems and populations. Int J Equity Health. Mar 11, 2013;12:18. [ FREE Full text ] [ CrossRef ] [ Medline ]
- Bryant A, Charmaz K. The SAGE Handbook of Grounded Theory, Paperback Edition. Thousand Oaks, CA. SAGE Publications; 2010.
- Johnson MO, Rose CD, Dilworth SE, Neilands TB. Advances in the conceptualization and measurement of health care empowerment: development and validation of the health care empowerment inventory. PLoS One. 2012;7(9):e45692. [ FREE Full text ] [ CrossRef ] [ Medline ]
- Norman CD, Skinner HA. eHEALS: the eHealth literacy scale. J Med Internet Res. Nov 14, 2006;8(4):e27. [ FREE Full text ] [ CrossRef ] [ Medline ]
- Kayser L, Karnoe A, Furstrand D, Batterham R, Christensen KB, Elsworth G, et al. A multidimensional tool based on the eHealth literacy framework: development and initial validity testing of the eHealth Literacy Questionnaire (eHLQ). J Med Internet Res. Feb 12, 2018;20(2):e36. [ FREE Full text ] [ CrossRef ] [ Medline ]
- Standards. Australian Bureau of Statistics. URL: https://www.abs.gov.au/statistics/standards [accessed 2024-04-04]
- Al-Janabi H, Flynn TN, Coast J. Development of a self-report measure of capability wellbeing for adults: the ICECAP-A. Qual Life Res. Feb 2012;21(1):167-176. [ FREE Full text ] [ CrossRef ] [ Medline ]
- Van der Feltz-Cornelis CM, Van Oppen P, Van Marwijk HW, De Beurs E, Van Dyck R. A patient-doctor relationship questionnaire (PDRQ-9) in primary care: development and psychometric evaluation. Gen Hosp Psychiatry. 2004;26(2):115-120. [ CrossRef ] [ Medline ]
- Chao J. Continuity of care: incorporating patient perceptions. Fam Med. 1988;20(5):333-337. [ Medline ]
- Wilson CK, Thomas J, Barraket J. Measuring digital inequality in Australia: the Australian digital inclusion index. JTDE. Jun 30, 2019;7(2):102-120. [ CrossRef ]
- Digital participation: a view of Australia's online behaviours. Australia Post. Jul 2017. URL: https://auspost.com.au/content/dam/auspost_corp/media/documents/white-paper-digital-inclusion.pdf [accessed 2024-04-04]
- Poli A, Kelfve S, Motel-Klingebiel A. A research tool for measuring non-participation of older people in research on digital health. BMC Public Health. Nov 08, 2019;19(1):1487. [ FREE Full text ] [ CrossRef ] [ Medline ]
- Cohen J. Statistical Power Analysis for the Behavioral Sciences Second Edition. London, UK. Routledge; 1988.
- Borg K, Smith L. Digital inclusion and online behaviour: five typologies of Australian internet users. Behav Inf Technol. Feb 15, 2018;37(4):367-380. [ CrossRef ]
- Mathers A, Richardson J, Vincent S, Joseph C, Stone E. Good Things Foundation COVID-19 response report. Good Things Foundation. 2020. URL: https://tinyurl.com/2peu3kak [accessed 2024-04-04]
- Norman CD, Skinner HA. eHealth literacy: essential skills for consumer health in a networked world. J Med Internet Res. Jun 16, 2006;8(2):e9. [ FREE Full text ] [ CrossRef ] [ Medline ]
- Neves AL, Burgers J. Digital technologies in primary care: implications for patient care and future research. Eur J Gen Pract. Dec 11, 2022;28(1):203-208. [ FREE Full text ] [ CrossRef ] [ Medline ]
Abbreviations
Edited by T Leung; submitted 03.07.23; peer-reviewed by T Freeman, H Shen; comments to author 16.08.23; revised version received 30.11.23; accepted 31.01.24; published 11.04.24.
©Melinda Ada Choy, Kathleen O'Brien, Katelyn Barnes, Elizabeth Ann Sturgiss, Elizabeth Rieger, Kirsty Douglas. Originally published in the Journal of Medical Internet Research (https://www.jmir.org), 11.04.2024.
This is an open-access article distributed under the terms of the Creative Commons Attribution License (https://creativecommons.org/licenses/by/4.0/), which permits unrestricted use, distribution, and reproduction in any medium, provided the original work, first published in the Journal of Medical Internet Research, is properly cited. The complete bibliographic information, a link to the original publication on https://www.jmir.org/, as well as this copyright and license information must be included.

Role of Blood Lipids in the Shared Genetic Etiology Between Major Depressive Disorder and Myocardial Infarction: A Large-scale Multi-trait Association Analysis
- Find this author on Google Scholar
- Find this author on PubMed
- Search for this author on this site
- ORCID record for Yanchen Zhu
- ORCID record for Zhengbo Wu
- ORCID record for Yahui Wang
- ORCID record for Zhaorui Cui
- ORCID record for Fani Liu
- ORCID record for Jiqiang Hu
- For correspondence: [email protected]
- Info/History
- Supplementary material
- Preview PDF
Background The genetic role of blood lipids (BL) in the shared genetic etiology between major depressive disorder (MDD) and myocardial infarction (MI) has not been fully characterized.
Methods We first evaluated genetic associations and causal inferences between MDD, MI and the quantitative traits of BL. To further unravel the underlying genetic mechanisms, we performed multi-trait association analysis to identify novel or pleiotropic genomic risk loci, and shared causal variants for diseases involving BL. Using multiple post-GWAS methods, we explored potential genes, pathways, tissues, cells, and therapeutic targets associated with diseases from different perspectives.
Findings We found extensive global and local genetic correlations between MDD, MI and the traits of BL. Mendelian randomization (MR) analyses showed that lipid metabolism mediated 26.5% of the mediating effect of MDD leading to MI. Multi-trait association analysis successfully identified 13 MDD- and 36 MI- novel risk loci which have never been reported before. Notably, many pleiotropic loci and shared causal variants were identified across risk loci for both diseases, such as 11q23.3 (rs117937125) and 12q13.3 (rs188571756), which also colocalized for traits of BL. Pathway enrichment analysis further highlighted shared biological pathways primarily involving synaptic function, arterial development, and lipid metabolism. Lastly, gene-mapping, gene-based, transcriptome-wide and proteome-wide association, and MR-proteomic analyses revealed candidate pathogenic genes and therapeutic targets (such as ANGPTL4 and TMEM106B).
Interpretation These findings not only provide novel insights into the role of BL in the comorbidity between MDD and MI, but also benefit the development of preventive or therapeutic drugs for diseases.
Competing Interest Statement
The authors have declared no competing interest.
Funding Statement
This work was supported by the Capital Health Research and Development of Special Fund (2020-2-4203).
Author Declarations
I confirm all relevant ethical guidelines have been followed, and any necessary IRB and/or ethics committee approvals have been obtained.
The details of the IRB/oversight body that provided approval or exemption for the research described are given below:
The study used ONLY openly available human data that were originally obtained as follows:The GWAS summary statistic for major depressive disorder from Psychiatric Genomics Consortium (PGC) was available at https://pgc.unc.edu/. GWAS for myocardial infarction was obtained from the GWAS Catalog website (GCST009541). GWAS for all quantitative traits of blood lipids from Global Lipids Genetics Consortium (GLGC) were available at https://csg.sph.umich.edu/willer/public/glgc-lipids2021. The eQTL from GTEx v8 were available at http://gusevlab.org/projects/fusion/. The plasma pQTL from the UK Biobank Pharma Proteomics Project (UKB-PPP) were obtained through the website https://www.synapse.org/, from deCODE genetics via http://www.decode.com, and from ARIC study via http://nilanjanchatterjeelab.org/pwas/. The DLPFC pQTL were obtained at https://www.synapse.org/.
I confirm that all necessary patient/participant consent has been obtained and the appropriate institutional forms have been archived, and that any patient/participant/sample identifiers included were not known to anyone (e.g., hospital staff, patients or participants themselves) outside the research group so cannot be used to identify individuals.
I understand that all clinical trials and any other prospective interventional studies must be registered with an ICMJE-approved registry, such as ClinicalTrials.gov. I confirm that any such study reported in the manuscript has been registered and the trial registration ID is provided (note: if posting a prospective study registered retrospectively, please provide a statement in the trial ID field explaining why the study was not registered in advance).
I have followed all appropriate research reporting guidelines, such as any relevant EQUATOR Network research reporting checklist(s) and other pertinent material, if applicable.
Code availability
No novel custom computer code or mathematical algorithm was employed in generating the results pivotal to the derived conclusions.
View the discussion thread.
Supplementary Material
Thank you for your interest in spreading the word about medRxiv.
NOTE: Your email address is requested solely to identify you as the sender of this article.

Citation Manager Formats
- EndNote (tagged)
- EndNote 8 (xml)
- RefWorks Tagged
- Ref Manager
- Tweet Widget
- Facebook Like
- Google Plus One
Subject Area
- Genetic and Genomic Medicine
- Addiction Medicine (316)
- Allergy and Immunology (620)
- Anesthesia (160)
- Cardiovascular Medicine (2284)
- Dentistry and Oral Medicine (280)
- Dermatology (201)
- Emergency Medicine (370)
- Endocrinology (including Diabetes Mellitus and Metabolic Disease) (805)
- Epidemiology (11593)
- Forensic Medicine (10)
- Gastroenterology (681)
- Genetic and Genomic Medicine (3600)
- Geriatric Medicine (337)
- Health Economics (618)
- Health Informatics (2311)
- Health Policy (916)
- Health Systems and Quality Improvement (865)
- Hematology (335)
- HIV/AIDS (753)
- Infectious Diseases (except HIV/AIDS) (13171)
- Intensive Care and Critical Care Medicine (758)
- Medical Education (360)
- Medical Ethics (100)
- Nephrology (391)
- Neurology (3369)
- Nursing (191)
- Nutrition (508)
- Obstetrics and Gynecology (652)
- Occupational and Environmental Health (647)
- Oncology (1764)
- Ophthalmology (526)
- Orthopedics (210)
- Otolaryngology (284)
- Pain Medicine (223)
- Palliative Medicine (66)
- Pathology (441)
- Pediatrics (1008)
- Pharmacology and Therapeutics (422)
- Primary Care Research (407)
- Psychiatry and Clinical Psychology (3077)
- Public and Global Health (6005)
- Radiology and Imaging (1227)
- Rehabilitation Medicine and Physical Therapy (715)
- Respiratory Medicine (811)
- Rheumatology (367)
- Sexual and Reproductive Health (356)
- Sports Medicine (318)
- Surgery (390)
- Toxicology (50)
- Transplantation (171)
- Urology (142)

IMAGES
VIDEO
COMMENTS
Answer: The background forms the first part of the Introduction section. It provides context for your study and helps the readers understand why your research topic is important. It gives a brief overview of the research done on the topic so far and mentions the gaps that have remained unaddressed as well as the need to address them.
Here are the steps to write the background of the study in a research paper: Identify the research problem: Start by identifying the research problem that your study aims to address. This can be a particular issue, a gap in the literature, or a need for further investigation. Conduct a literature review: Conduct a thorough literature review to ...
The background of the study is a section in a research paper that provides context, circumstances, and history leading to the research problem or topic being explored. It presents existing knowledge on the topic and outlines the reasons that spurred the current research, helping readers understand the research's foundation and its significance ...
INTRODUCTION. Scientific research is usually initiated by posing evidenced-based research questions which are then explicitly restated as hypotheses.1,2 The hypotheses provide directions to guide the study, solutions, explanations, and expected results.3,4 Both research questions and hypotheses are essentially formulated based on conventional theories and real-world processes, which allow the ...
The background of a study is the first section of the paper and establishes the context underlying the research. It contains the rationale, the key problem statement, and a brief overview of research questions that are addressed in the rest of the paper. The background forms the crux of the study because it introduces an unaware audience to the ...
In this video, I shared some practical tips in writing the Introduction - Background of the Study of a Quantitative Research Paper. Hope you learn from this ...
The background of the study is the first section of a research paper and gives context surrounding the research topic. The background explains to the reader where your research journey started, why you got interested in the topic, and how you developed the research question that you will later specify. That means that you first establish the ...
Abstract. Summarizing quantitative data and its effective presentation and discussion can be challenging for students and researchers. This chapter provides a framework for adequately reporting findings from quantitative analysis in a research study for those contemplating to write a research paper. The rationale underpinning the reporting ...
The structure of a background study in a research paper generally follows a logical sequence to provide context, justification, and an understanding of the research problem. It includes an introduction, general background, literature review, rationale, objectives, scope and limitations, significance of the study and the research hypothesis.
The background section should discuss your findings in a chronological manner to accentuate the progress in the field and the missing points that need to be addressed. The background should be written as a summary of your interpretation of previous research and what your study proposes to accomplish.
Quantitative research methods. You can use quantitative research methods for descriptive, correlational or experimental research. In descriptive research, you simply seek an overall summary of your study variables.; In correlational research, you investigate relationships between your study variables.; In experimental research, you systematically examine whether there is a cause-and-effect ...
Quantitative methods emphasize objective measurements and the statistical, mathematical, or numerical analysis of data collected through polls, questionnaires, and surveys, or by manipulating pre-existing statistical data using computational techniques.Quantitative research focuses on gathering numerical data and generalizing it across groups of people or to explain a particular phenomenon.
This video lecture discusses the steps and effective techniques in writing the "Background of the Study in Research or Thesis/Dissertation". Transcript of th...
1) A brief discussion on what is known about the topic under investigation; 2) An articulation of the research gap or problem that needs to be addressed; 3) What the researcher would like to do or aim to achieve in the study (research goal); 4) The thesis statement, that is, the main argument or claim of the paper; and.
Many research documents when reviewed wholesomely in most instances fails the background study test as the authors either presume it is a section notes on the research or just a section to ensure ...
DISCLAIMER:Some contents and examples mentioned in the video is taken from the presentation of Sir Von Chua. Music: bensound.comhttps://youtu.be/h72R0Wv2nBgh...
Educational research has a strong tradition of employing state-of-the-art statistical and psychometric (psychological measurement) techniques. Commonly referred to as quantitative methods, these techniques cover a range of statistical tests and tools. The Sage encyclopedia of educational research, measurement, and evaluation by Bruce B. Frey (Ed.)
In quantitative studies, there is a very systematic approach that moves from the beginning point of the study (writing a research question) to the end point (obtaining an answer). This is a very linear and purposeful flow across the study, and all quantitative research should follow the same sequence.
Lacking a common methodological background, qualitative and quantitative research methodologies have developed rather distinct standards with regard to the aims and scope of empirical science (Freeman et al., 2007). These different standards affect the way researchers handle contradictory empirical findings.
The study "SHS STEM Students Gender Dominance, Attitude, and Self-Efficacy towards STEM-Related Courses" is a quantitative research thatutilized a descriptive- correlational research design, in which it is used to describe and measure the degree of relationship between two or more variable or set of scores (Creswell, 2012) ... Background of ...
1.1 BACKGROUND OF STUDY. Ideology been a controversial concept is said to be a ver y crucial aspe ct of politics, not only. as a cognitive structure of looking at the society generally and ...
Background of the Study (Quantitative Research) - Free download as Word Doc (.doc / .docx), PDF File (.pdf), Text File (.txt) or read online for free. Scribd is the world's largest social reading and publishing site.
Background Confusion exists over the definition of the care pathway concept and existing conceptual frameworks contain various inadequacies which have led to implementation difficulties. In the current global context of rapidly changing health care systems, there is great need for a standardized definition and integrative framework that can guide implementation. This study aims to propose an ...
Background The impact of the Covid-19 pandemic on young people's (YP) mental health has been mixed. Systematic reviews to date have focused predominantly on quantitative studies and lacked involvement from YP with lived experience of mental health difficulties. Therefore, our primary aim was to conduct a qualitative systematic review to examine the perceived impact of the Covid-19 pandemic on ...
Background: The digital health divide for socioeconomic disadvantage describes a pattern in which patients considered socioeconomically disadvantaged, who are already marginalized through reduced access to face-to-face health care, are additionally hindered through less access to patient-initiated digital health. A comprehensive understanding of how patients with socioeconomic disadvantage ...
Background: The genetic role of blood lipids (BL) in the shared genetic etiology between major depressive disorder (MDD) and myocardial infarction (MI) has not been fully characterized. Methods: We first evaluated genetic associations and causal inferences between MDD, MI and the quantitative traits of BL. To further unravel the underlying genetic mechanisms, we performed multi-trait ...