Click through the PLOS taxonomy to find articles in your field.
For more information about PLOS Subject Areas, click here .
Loading metrics
Open Access
Peer-reviewed
Research Article

Coordinating virus research: The Virus Infectious Disease Ontology
Roles Conceptualization, Formal analysis, Investigation, Methodology, Supervision, Writing – original draft, Writing – review & editing
* E-mail: [email protected]
Affiliations Department of Philosophy, University at Buffalo, Buffalo, NY, United States of America, National Center for Ontological Research, Buffalo, NY, United States of America

Roles Conceptualization, Investigation, Validation, Writing – review & editing
Affiliations National Center for Ontological Research, Buffalo, NY, United States of America, Air Force Research Laboratory, Wright Patterson Air Force Base, Riverside, OH, United States of America
Roles Conceptualization, Investigation, Methodology, Project administration, Validation, Writing – review & editing
Affiliation Department of Cognitive Science, Northwestern University, Evanston, IL, United States of America
Roles Conceptualization, Formal analysis, Investigation, Writing – review & editing
Affiliation Department of Clinical Sciences, University of Texas Southwestern Medical Center, Dallas, TX, United States of America
Roles Conceptualization, Formal analysis, Writing – review & editing
Affiliation Department of Philosophy, Loyola University, Chicago, IL, United States of America
Affiliation Computational Medicine and Bioinformatics, University of Michigan Medical School, He Group, Ann Arbor, MI, United States of America
Roles Conceptualization, Investigation, Methodology, Writing – review & editing
Affiliations National Center for Ontological Research, Buffalo, NY, United States of America, Department of Philosophy, Northwestern University, Evanston, IL, United States of America
Roles Conceptualization, Investigation, Methodology, Validation, Writing – review & editing
Roles Conceptualization, Methodology, Validation, Writing – review & editing
Affiliations Department of Informatics, J. Craig Venter Institute, La Jolla, CA, United States of America, Department of Pathology, University of California, San Diego, CA, United States of America, Division of Vaccine Discovery, La Jolla Institute for Immunology, La Jolla, CA, United States of America
Roles Conceptualization, Formal analysis, Investigation, Methodology, Supervision, Writing – review & editing
- John Beverley,
- Shane Babcock,
- Gustavo Carvalho,
- Lindsay G. Cowell,
- Sebastian Duesing,
- Yongqun He,
- Regina Hurley,
- Eric Merrell,
- Richard H. Scheuermann,
- Barry Smith
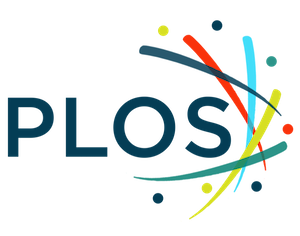
- Published: January 18, 2024
- https://doi.org/10.1371/journal.pone.0285093
- Peer Review
- Reader Comments
The COVID-19 pandemic prompted immense work on the investigation of the SARS-CoV-2 virus. Rapid, accurate, and consistent interpretation of generated data is thereby of fundamental concern. Ontologies–structured, controlled, vocabularies–are designed to support consistency of interpretation, and thereby to prevent the development of data silos. This paper describes how ontologies are serving this purpose in the COVID-19 research domain, by following principles of the Open Biological and Biomedical Ontology (OBO) Foundry and by reusing existing ontologies such as the Infectious Disease Ontology (IDO) Core, which provides terminological content common to investigations of all infectious diseases. We report here on the development of an IDO extension, the Virus Infectious Disease Ontology (VIDO), a reference ontology covering viral infectious diseases. We motivate term and definition choices, showcase reuse of terms from existing OBO ontologies, illustrate how ontological decisions were motivated by relevant life science research, and connect VIDO to the Coronavirus Infectious Disease Ontology (CIDO). We next use terms from these ontologies to annotate selections from life science research on SARS-CoV-2, highlighting how ontologies employing a common upper-level vocabulary may be seamlessly interwoven. Finally, we outline future work, including bacteria and fungus infectious disease reference ontologies currently under development, then cite uses of VIDO and CIDO in host-pathogen data analytics, electronic health record annotation, and ontology conflict-resolution projects.
Citation: Beverley J, Babcock S, Carvalho G, Cowell LG, Duesing S, He Y, et al. (2024) Coordinating virus research: The Virus Infectious Disease Ontology. PLoS ONE 19(1): e0285093. https://doi.org/10.1371/journal.pone.0285093
Editor: Barry L. Bentley, Cardiff Metropolitan University, UNITED KINGDOM
Received: November 28, 2022; Accepted: April 12, 2023; Published: January 18, 2024
Copyright: © 2024 Beverley et al. This is an open access article distributed under the terms of the Creative Commons Attribution License , which permits unrestricted use, distribution, and reproduction in any medium, provided the original author and source are credited.
Data Availability: The Virus Infectious Disease Ontology artifact can be found in the following Github repository: https://github.com/infectious-disease-ontology-extensions/ido-virus . The Coronavirus Infectious Disease Ontology artifact can be found in the following Github repository: https://github.com/CIDO-ontology/cido .
Funding: Sources of funding for this article for John Beverley and Shane Babcock stem from the NIH / NLM T5 Biomedical Informatics and Data Science Research Training Programs. Barry Smith’s source of funding stemmed from the NIH under NCATS 1UL1TR001412 (Buffalo Clinical and Translational Research Center). No other co-authors were funded to pursue work on this project. Moreover, the funders had no role in the study design, data collection and analysis, decision to publish, or preparation of the manuscript.
Competing interests: The authors have declared that no competing interests exist.
Introduction
The value of cross-discipline meta-data analysis has been evident in the COVID-19 pandemic. Early in the pandemic, for example, prostate oncologists [ 1 , 2 ] attempted to leverage existing research on enzymes crucial in host cell penetration by SARS-CoV-2 to explain differences in disease severity across sex [ 3 , 4 ]; immunologists combined insights from research on SARS-CoV-1 and MERS-CoV with chemical compound profiles to identify treatment options for SARS-CoV-2 [ 5 – 7 ]; pediatric researchers, observing that children have fewer nasal epithelia susceptible to SARS-CoV-2 infection than adults, suggested this difference may explain symptom disparities between the two groups [ 8 , 9 ]. The sheer volume of data collected by life-science researchers, the speed at which it is generated, range of its sources, quality, accuracy, and urgency of need for assessment of usefulness, has resulted in complex, multidimensional datasets, often annotated using discipline- or institution-specific terminologies and coding systems that lead to data silos [ 10 – 12 ].
Data silos emerge in life science research when data concerning an area of research is stored in a manner that makes it accessible to one group, but inaccessible to others. The use of proprietary information systems, differing storage methods, and distinct coding standards across life science that is characteristic of such silos undermines interoperability, meta-data analysis, pattern identification, and discovery across disciplines [ 13 , 14 ]. Ontologies–interoperable, logically well-defined, controlled vocabularies representing common entities and relations across disciplines using consensus terminologies–constitute a well-known solution to these problems through mitigation of the formation of data silos. The need for rapid analysis of evolving datasets representing coronavirus research motivated the development of the Virus Infectious Disease Ontology (VIDO; https://bioportal.bioontology.org/ontologies/VIDO ), comprised of textual definitions for terms and relations and logical axioms supporting automated consistency checking, querying over datasets, and interoperability with other ontologies. VIDO is an extension of the widely-used Infectious Disease Ontology Core (IDO Core; https://bioportal.bioontology.org/ontologies/IDO ) [ 15 , 16 ], which comprises terminological content common to all investigations of infectious disease. VIDO extends IDO with terms specific to the domain of infectious diseases caused by viruses and provides a foundation for ontologies representing specific viral infectious diseases, such as COVID-19.
VIDO is available under the Creative Commons Attribution 4.0 license ( https://creativecommons.org/licenses/by/4.0/ ) and its current and past versions can be found at the National Center for Biomedical Ontology (NCBO) Bioportal [ 17 ], the Ontobee repository ( http://www.ontobee.org/ ), and the Ontology Lookup Service ( https://www.ebi.ac.uk/ols/index ). VIDO was developed in collaboration with relevant domain experts, including immunologists and virologists, and by drawing on the expertise of the IDO developers to ensure alignment with principles outlined by the Open Biological and Biomedical Ontology (OBO) Foundry [ 18 ], thereby supporting interoperability with existing Foundry ontologies [ 19 ]. VIDO development is a transparent process, with all discussions available on GitHub ( https://github.com/infectious-disease-ontology-extensions ). All aspects of development, including addition of new terms, are driven by the needs of researchers investigating viruses and nearby domains. The ontology is thus not viewed as exhaustive of the domain of virus research but remains sensitive to evolving knowledge.
OWL, Protégé, Mace4, and Prover9
VIDO is formally represented in the OWL2 Web Ontology Language ( https://www.w3.org/TR/owl2-overview/ ). OWL2 is an expansion of the Resource Description Framework (RDF; https://www.w3.org/TR/rdf-primer/ ) and of RDF Schema, which represent data as sets of subject- predicate-object directed graphs, and which can be queried using the SPARQL Protocol and RDF Query Language ( https://www.w3.org/TR/sparql11-query/ ). OWL2 supplements these languages by allowing for description of classes, members of classes, relations among individuals, and annotation properties. Formally, the OWL2 vocabulary can be mapped to a decidable fragment of first-order logic, meaning there is an algorithm which can determine the truth-value for any statement expressed in the language in a finite number of steps [ 20 ]. Restricting expressions to a decidable language allows automated consistency and satisfiability checking [ 21 ]. VIDO was developed using the Protégé-OWL editor ( https://protege.stanford.edu/ ) and tested against OWL reasoners such as HermiT [ 22 ] and Pellet [ 23 ]. Additionally, logical axioms underwriting these ontologies were translated into the Common Logic Interchange Format, and subsequently evaluated using the Mace4 model checker and Prover9 proof generator within the Macleod toolkit ( https://github.com/thahmann/macleod ).
Alignment with OBO Foundry ontologies
Ontologies are widely used in bioinformatics, supporting data standardization, integration, sharing, reproducibility, and automated reasoning. The Gene Ontology (GO; https://bioportal.bioontology.org/ontologies/GO ), for example, maintains species-neutral annotations of gene products and functions, and since its inception in 1998 it has inspired an explosion of biomedical ontologies covering all domains of the life sciences [ 19 , 24 , 25 ]. These early developments led to worries, however, that data silos–the very problem ontologies were designed to address–might reemerge [ 10 ] as researchers developed ontologies using concepts local to their discipline. By 2007, the Open Biomedical and Biological Ontologies (OBO) Foundry [ 18 ] was created to provide guidance for ontology developers and promote alignment and interoperability. OBO Foundry design principles require that ontologies: use a well-specified syntax that is unambiguous, with a common space of identifiers; that they be openly available in the public domain, have a specified scope, be developed in a modular fashion in a collaboration with ontologists covering nearby domains, and import a common set of relations from the Relations Ontology (RO; https://obofoundry.org/ontology/ro.html ). The OBO library ( http://obofoundry.org/ ) presently consists of over 250 ontologies, including some externally developed ontologies such as the NCI Thesaurus ( https://ncithesaurus.nci.nih.gov/ncitbrowser/ ) and the NCBI Taxonomy ( https://www.ncbi.nlm.nih.gov/taxonomy ). It also contains some constructed ab initio to satisfy OBO principles. At its core is Basic Formal Ontology (BFO; https://bioportal.bioontology.org/ontologies/BFO ), a top-level ontology covering general classes such as material entity , quality , process , function and role [ 10 , 26 – 29 ] which provides the architecture “on which OBO Foundry ontologies are built.” BFO is, moreover, an ISO/IEC approved standard 21838–2 ( https://www.iso.org/standard/74572.html ).
Where BFO is domain-neutral, other OBO Foundry ontologies represent types of entities in more specific domains, using terms such as disease , cell division , surgical procedure , and so forth. Ideally, domain ontologies are constructed using a methodology for formulating definitions through a process of downward population from BFO. The resulting alignment with BFO, and the conformance to OBO Foundry principles, foster integration across ontologies. VIDO was designed with alignment and conformance in mind. Development of each ontology follows metadata conventions adopted by many OBO Foundry ontologies [ 30 ]. These conventions require that every term introduced into the ontology has a unique IRI, textual definitions, definition source, designation of term editor(s), and preferred term label. In the interest of coordinating development with existing OBO ontologies, VIDO developers imported terms where possible from existing OBO library ontologies and constructed logical definitions using imported terms. Development was guided by best practices for definition construction [ 10 , 31 ]. New primitive terms were introduced when needed after consultation with domain experts, review of relevant literature, and careful examination of the OBO library to avoid redundancy.
Hub and spokes approach
VIDO follows the “hub and spokes” methodology [ 32 , 33 ] for ontology development. That is, VIDO is a spoke ontology, extending from the Infectious Disease Ontology Core (IDO Core; https://bioportal.bioontology.org/ontologies/IDO ) as its hub. IDO Core is an OBO ontology consisting of terms, relations, natural language definitions and associated logical axioms representing phenomena common across research in infectious diseases [ 15 ]. IDO Core has long provided a base from which more specific infectious disease ontologies extend, and it has been recently updated to keep pace with scientific and top-level architecture changes [ 16 ]. Extensions of IDO Core covering specific infectious diseases are created, first, by importing needed terms from IDO Core and other OBO Foundry ontologies, and second, by constructing the domain-specific terms where needed to adequately characterize entities in the relevant domain. Fig 1 illustrates example extensions, such as the Brucellosis Infectious Disease Ontology (IDOBRU; https://bioportal.bioontology.org/ontologies/IDOBRU ) the Influenza Infectious Disease Ontology (IDOFLU; https://bioportal.bioontology.org/ontologies/FLU ), and more recently the Coronavirus Infectious Disease Ontology (CIDO; https://bioportal.bioontology.org/ontologies/CIDO ). Each aims to be semantically interoperable with OBO library ontologies [ 11 , 34 – 36 ]. VIDO was designed to occupy the ontological space between such virus-specific ontologies and IDO Core. As a result, more specific virus-related ontologies such as CIDO [ 37 ] and IDOFLU are being curated to extend directly from VIDO, rather than directly from IDO Core.
- PPT PowerPoint slide
- PNG larger image
- TIFF original image
https://doi.org/10.1371/journal.pone.0285093.g001
The Virus Infectious Disease Ontology
VIDO takes IDO Core as its starting point, but also imports terms relevant to the domain of viruses from several other OBO Foundry ontologies, such as GO, the Ontology for General Medical Science (OGMS; https://bioportal.bioontology.org/ontologies/OGMS ) and the Ontology for Biomedical Investigation (OBI; https://bioportal.bioontology.org/ontologies/OBI ) [ 14 ]. The color- coded Fig 1 illustrates several importing relationships which provide the basis for VIDO definitions which we examine in what follows.
Acellular structure.
Like IDO Core, VIDO imports from OGMS. Examples of such imported terms are:
disorder = def Material entity that is a clinically abnormal part of an extended organism.
A part of a material entity is “clinically abnormal” if it is not expected in the life plan for entities of the relevant type and is causally linked to elevated risk–that is, risk exceeding some threshold–of illness, death, or disfunction [ 38 ]. Extended organism is imported from OGMS and organism from OBI, where they are defined as follows:
extended organism = def An object aggregate consisting of an organism and all material entities located within the organism, overlapping the organism, or occupying sites formed in part by the organism.
organism = def A material entity that is an individual living system, such as animal, plant, bacteria, or virus, that is capable of replicating or reproducing, growth and maintenance in the right environment. An organism may be unicellular or made up, like humans, of many billions of cells divided into specialized tissues and organs.
Here we run into the first of several ontological puzzles that emerged while developing VIDO. On the one hand, this definition aligns with common usage of the term “organism” among researchers for whom its instances are cellular entities [ 39 , 40 ]. On the other hand, the textual definition includes viruses among its instances, which are in every case acellular. Debates over organism ( https://github.com/OBOFoundry/COB/issues/6 ) among ontology developers have resulted in deprecation of the OBI term in favor of a nearby term from the Common Anatomy Reference Ontology ( https://bioportal.bioontology.org/ontologies/CARO ) with the label: organism or virus or viroid . At first glance, this appears to avoid the preceding worries, but further inspection reveals that this class is annotated as being an “exact synonym” of organism, and so suffers from the same issues raised above. Even if we put aside this latter issue, however, there are still two further concerns. First, introducing disjunctive classes is ad hoc [ 10 ]. Second, this disjunctive class leads naturally to debates over whether viruses are alive, since it classifies viruses alongside paradigmatic living entities. Decades of discussion have not resolved this question [ 41 – 46 ], and it is not obvious that we need an answer for the purposes of ontological modelling. It is unclear where consensus will land; in the interest of future-proofing our ontologies we should provide virus content in a way that neutral in regard to this issue to the maximal extent that this is possible. Rather than introduce an ad hoc disjunctive class, IDO Core, VIDO, and CIDO developers collaborated to add the following classes to IDO Core [ 47 ]:
self-replicating organic structure = def Object consisting of an organic structure that is able to initiate replication of its structure in a host.
acellular self-replicating organic structure = def Self-replicating organic structure comprised of acellular organic parts.
Which is imported to VIDO as the parent class of the term virus . The term virus is imported from the NCBITaxon [ 48 ] ( https://bioportal.bioontology.org/ontologies/NCBITAXON ), alongside terms relevant to virology, such as prion and satellite .
The NCBITaxon provides an extensive list of life science terms, but it has its limitations. As discussed in [ 16 ], NCBITaxon categorizes virus terms using the International Committee on Taxonomy of Viruses (ICTV). While an impressive taxonomy, the ICTV exhibits gaps in virus classification [ 49 , 50 ]. Additionally, NCBITaxon combined with standard ontology engineering tools such as Ontofox ( http://ontofox.hegroup.org/ ) [ 51 ] often leads to ontology developers importing superfluous portions of ICTV structured hierarchies, resulting in overwhelming taxonomies that are challenging for users to navigate. Fig 2 illustrates such a taxonomy found in IDOBRU, but importing an entire ICTV hierarchy is not uncommon (see for example the Schistosomiasis Ontology (IDOSCHISTO) [ 16 ]). Lastly, the NCBITaxon does not provide textual definitions for most terms within its scope. As stated, we seek to respect OBO Foundry metadata conventions [ 30 ] and ontology engineering best practices [ 10 , 31 ]; consequently, virus and other terminological content in VIDO must have textual definitions supplied.
https://doi.org/10.1371/journal.pone.0285093.g002
Standard definitions of “virus” provide a starting point for a textual definition, but caution is once again needed. Viruses are often described as obligate pathogens [ 52 , 53 ], since virus replication requires host machinery for production and assembly of viral components. However, defining a class virus solely in terms of what viruses typically do runs the risk of overlooking what viruses are, materially speaking. Compare: Homo sapiens are obligate aerobes, but this is no definition of the class. Insofar as we are defining the virus class, it is better to attend to genetic and structural components common to all viruses, and best to define the material entity in a way that captures obligate pathogenicity. VIDO accordingly defines:
virus = def Acellular self-replicating organic structure with RNA or DNA genetic material which uses host metabolic resources for RNA or DNA replication.
VIDO developers have contacted NCBITaxon developers proposing that the definitions we provide below be added to respective NCBITaxon terms. Other requests have been submitted, for example, to update the label of the NCBITaxon term from “Viruses” to “virus” in order to avoid ambiguous reference between classes and their instances [ 10 ].
Rather than import in accordance with the ICTV taxonomy, subclasses of virus are imported from NCBITaxon in alignment with the Baltimore Classification [ 54 ], which groups viruses into seven exhaustive classes based on genetic structure. For example, one subclass of virus is:
positive-sense single-stranded RNA virus = def Virus with genetic material encoded in single-stranded RNA that can be translated directly into proteins.
Which is a class representing one of the seven Baltimore Classification categories. These Baltimore Classification classes provide parent classes from which terms for more specific viruses can extend. Fig 3 illustrates how the Baltimore Classification appears in the Protégé visualization of the class positive-sense single-stranded RNA virus alongside viral replication pathways underwritten by genetic differences in viruses.
https://doi.org/10.1371/journal.pone.0285093.g003
By incorporating the Baltimore Classification, we provide developers of virus-specific domain ontologies an ontological representation of viruses that is simpler and easier to navigate than the ICTV, which currently underwrites the NCBITaxon.
VIDO subclasses of virus include those common in virology research, such as bacteriophage– viruses that infect bacteria– virophage– viruses that infect viruses– oncovirus– viruses that cause cancer–and mycovirus– viruses that infect fungi. As well as:
virion = def Virus that is in its assembled state consisting of genomic material (DNA or RNA) surrounded by coating molecules.
Some researchers use “virion” and “virus” synonymously [ 55 ]. Some define “virion” so that instances only exist outside host cells [ 56 ], or they distinguish virions outside host cells from those inside host cells, calling the former “mature virions.” Some claim “virion” is best understood as analogous to a sperm cell [ 57 , 58 ]. Ontologically speaking, one might model the relationship between a virus and its virion in a variety of ways: virion is to a virus as human infant is to human, or as human student is to human, or as human gamete is to human. Treating virions as akin to gametes is uncommon among researchers. Between the remaining options, we adopt the first, treating virion as a type of virus , since adopting the alternative would suggest a virion is simply a virus that is in a specific context, a result that overlooks the importance of genomic assembly to identifying virions.
Incidentally, some viruses do not replicate faithfully, perhaps resulting in genetically distinct mutants or–in extreme cases–an inactive aggregate of virion components. Virus mutations may undermine host immune system recognition of viral threats, as evidenced by the difficulty in developing vaccines for certain influenza strains. If there are too many mutations, however, then a virus may lose its ability to replicate, an observation used in development of treatments for polio and hepatitis C which exacerbate respective virus mutations [ 59 , 60 ]. VIDO thus provides the term:
disordered virus = def Acellular self-replicating organic structure having some clinically abnormal arrangement of viral components (e.g. viral capsid, viral DNA/RNA).
Viruses falling in this class may be associated with diseases much different from those associated with viruses that do not exhibit disorder. Terms for viral components are imported to VIDO: from GO, viral nucleocapsid , viral capsid , capsomere , viral envelope ; from the Chemical Entities of Biological Interest (ChEBI) ontology ( https://bioportal.bioontology.org/ontologies/CHEBI ) [ 61 , 62 ], nucleic acid and ribonucleic acid ; from the Protein Ontology ( https://bioportal.bioontology.org/ontologies/PR ), protein and viral protein .
Infectious structure.
The term “pathogen” is indexed to species or to stages in the developmental cycle of a species. A given virus may engage in mutual symbiosis with one species, while exhibiting pathogenic behavior towards others [ 63 , 64 ]. Mature plants are often susceptible to different pathogens than developing plants [ 65 – 68 ]. We capture virus pathogenicity in VIDO in steps. From IDO Core [ 16 ], we import dispositions borne by pathogens and infectious agents, as follows:
pathogenic disposition = def Disposition borne by a material entity to establish localization in, or produce toxins that can be transmitted to, an organism or acellular structure, either of which may form disorder in the entity or immunocompetent members of the entity’s species.
infectious disposition = def Pathogenic disposition borne by a pathogen to be transmitted to a host and then become part of an infection in that host or in immunocompetent members of the same species as the host.
The class infectious agent in IDO Core is a subclass of organism , and so cannot include instances of virus . To address this issue, the term infectious structure was developed to parallel the IDO Core term infectious agent and to provide a logically defined subclass of acellular self-replicating organic structure . The term infectious disposition bridges infectious acellular structures and infectious organisms since instances of each bear an infectious disposition. Moreover, the logical definitions of infectious structure and infectious agent are such that, though the former is a defined subclass of acellular self-replicating organic structure and the latter a subclass of organism , they are both inferred subclasses of pathogen .
As discussed in [ 16 ], establishment of localization used in pathogenic disposition is characterized using the IDO Core term establishment of localization in host representing tethering or adhesion to a host, while “formation of disorder” abbreviates appearance of disorder , which is a process that results in formation of a disorder . The definition of pathogenic disposition is meant to reflect a temporal ordering between establishment of localization and appearance of disorder. This is reflected explicitly in the logical axioms associated with the class. Similarly, in the definition of infectious disposition there is an intended temporal ordering between transmission to a host–represented by pathogen transmission process imported from the Pathogen Transmission Ontology ( https://bioportal.bioontology.org/ontologies/PTRANS )–and becoming part of an infection–represented by the IDO Core process of establishing an infection . A pathogen bearing an infectious disposition that generates disorder in a host will have been transmitted to the host prior to establishing localization in the host and will have established an infection prior to the appearance of disorder.
The complexity of the definitions of pathogenic disposition and infectious disposition reflect the variety of pathogen examples documented in contemporary literature. Consider S . aureus , an opportunistic pathogen [ 56 ] in humans. We count S . aureus as a pathogen, even when it does not realize disorder in a host, since it is nevertheless disposed to localize in a human host and generate disorder if given the opportunity. This is a BFO disposition of S . aureus as it is an “internally-grounded” property of the entity [ 32 ]. That is, it is part of the material basis of S . aureus to generate disorder in human hosts if given the chance. This is analogous to the way salt has a disposition to dissolve, based on its lattice structure, independently of whether it ever realizes this disposition. Opportunistic pathogens are not pathogens because of an opportunity; they are pathogens because they are disposed to localize and cause disorder in a host.
Consider now, C . botulinum , a pathogen which produces a toxin and which may produce a spore ingested by humans. This bacterium is a pathogen for adult humans since the toxins often result in disorder when ingested. Furthermore, C . botulinum may cause infection in human infants if, say honey colonized by C . botulinum is ingested. The sugar content of honey inhibits C . botulinum growth, but in the low-oxygen, low-acid intestines of human infants, spores can localize, grow, and produce toxins resulting in disorder. Thus, C . botulinum counts as a human infant pathogen. Nevertheless, because C . botulinum is not itself disposed to invade or be transmitted to human infants, we do not say the bacterium is infectious [ 69 ]. Being part of an infection is not itself sufficient for something to be counted as infectious. Pathogens bearing an infectious disposition must be disposed to both transmit and become part of an infection. Many opportunistic pathogens, for example, are not infectious.
Consider lastly, the respective definitions of infectious disposition and pathogenic disposition address instances where mutations in hosts may block realization of disorder or infection. In such cases, an infectious pathogen may nevertheless be transmissible and cause disorder or infection in others. For example, HIV-1 is a pathogen that may localize in a host with CCR-5 mutations [ 70 ] that block the virus from attaching to host cells, and so block pathogenesis to AIDS. Similarly, P . falciparum may be transmitted to a host with a sickle-cell trait that blocks manifestation of the disease malaria [ 71 , 72 ]. However, P . falciparum and HIV-1 count as pathogens even if they do not result in the formation of disorders for hosts with a sickle-cell trait or CCR-5 mutation, respectively. IDO Core reflects this characterization. Each pathogen may be transmitted to immunocompetent members of the same species as the host, and so count as bearing instances of infectious disposition and pathogenic disposition . Note, the fact that P . falciparum and HIV-1 do not result in the formation of disorders in hosts with sickle-cell traits or CCR-5 mutations should not suggest that there are no clinical abnormalities associated with these traits or mutations. Individuals with, say, CCR-5 mutations do exhibit clinical abnormalities, and so do exhibit disorders. But these disorders are due to the CCR-5 mutation rather than the HIV-1 infection.
Whenever an infectious disposition is realized, this is always in some site in a host, via some transmission to that host, and some generation of infection and disorder in that host. Infectious structures– such as viruses–bear this disposition. For example, each SARS-CoV-2 virus is disposed to be transmitted to hosts, localize, cause infection, and result in disorder.
Pathogen host.
Until recently, microbiologists, immunologists, virologists, and others studying pathogenesis have engaged in either host-centered or pathogen-centered pathogenesis research [ 73 – 77 ]. Each approach has led to impressive research results. But emphasizing one aspect of host-pathogen interactions at the expense of the other may leave valuable questions unanswered [ 78 ]. Emphasis, for example, solely on pathogenic factors of SARS-CoV-2 will provide only a partial explanation of various pathogenesis pathways observed in clinical settings. IDO Core and VIDO prioritize neither host nor pathogen in representation of pathogens and associated diseases, adopting the Damage Response Framework (DRF) for guidance in development of relevant terms [ 79 – 82 ]. According to the DRF, pathogenesis results from interactions between both host and pathogen interacting primarily through host damage, which is a function of the intensity and degree of host response and pathogen factors. Host and pathogen interactions thus influence manifestations of signs, symptoms, and disease. IDO Core now defines hosts and pathogens in terms of roles and allows that acellular structures may also be pathogen hosts, such as when a virus hosts a virophage:
host role = def Role borne by either an organism whose extended organism contains a distinct material entity, or an acellular structure containing a distinct material entity, realized in use of that structure or organism as a site of reproduction or replication.
pathogen host role = def Host role borne by an organism or acellular structure having a pathogen as part.
Following BFO, roles are “externally grounded” realizable entities that may be gained or lost based on circumstance without necessarily involving material change to their bearer, such as the role a student acquired once enrolled in a university.
The Symptom Ontology ( https://bioportal.bioontology.org/ontologies/SYMP ) provides a extensive terminological content for representing symptoms owing to viral infection, such as fever , taste alteration , and so on [ 83 ]. Given the importance of asymptomatic carriers in viral infection spread, moreover, attention is also given in IDO Core to:
symptomatic carrier role = def Pathogen host role borne by an organism whose extended organism contains a pathogen bearing an infectious disposition towards the host, and the host has manifested symptoms of the infectious disease caused by the pathogen.
asymptomatic carrier role = def Pathogen host role borne by an organism whose extended organism contains a pathogen bearing an infectious disposition towards the host, and the host has no symptoms of the infectious disease caused by the pathogen.
subclinical infection = def Infection that is part of an asymptomatic carrier.
The definition of the term subclinical infection reflects standard use of the terms “subclinical” and “asymptomatic” while allowing for asymptomatic clinically abnormal infections. VIDO extends subclinical infection to subclinical virus infection , namely, those subclinical infections caused by a virus. These remarks bring us full circle to the term disorder introduced above, since clinical abnormality is associated with disorder. When that disorder stems from infection it counts as an:
infectious disorder = def Disorder that is part of an extended organism which has an infectious pathogen part, that exists as a result of a process of formation of disorder initiated by the infectious pathogen.
And when the adverted pathogen is a virus, it falls in the VIDO class:
virus disorder = def Infectious disorder that exists as a result of a process of formation of disorder initiated by a virus.
Viral disease.
Medical researchers draw a distinction between symptoms and signs, a distinction which OBO Foundry ontologies respect (from OGMS [ 38 ]):
symptom = def Process experienced by the patient which can only be experienced by the patient, that is hypothesized to be clinically relevant.
qualitative sign = def Abnormal observable quality of a part of a patient that is hypothesized to be clinically relevant.
processual sign = def Abnormal processual entity occurring in a patient that is hypothesized to be clinically relevant.
An asymptomatic carrier infected with SARS-CoV-2 likely exhibits signs indicating that the infection is clinically abnormal, such as ground-glass opacities. Such asymptomatic carriers exhibit an instance of the VIDO class virus disorder which is the material basis of a viral disease :
infectious disease = def Disease whose physical basis is an infectious disorder.
viral disease = def Infectious disease inhering in a virus disorder that is a disorder due to the presence of the virus.
Worth noting is that these definitions are consistent with the CDC’s case criteria definitions adopted between April 5, 2020 and February 28, 2023, which indicate that the presence of the SARS-CoV-2 genome or relevant antigens in an individual is sufficient to count as a case of COVID-19, asymptomatic or not [ 84 – 86 ]. A viral disease may be realized in a disease course:
infectious disease course = def Disease course that is the realization of an infectious disease.
viral disease course = def Infectious disease course whose physical basis is a virus disorder that is clinically abnormal in virtue of the presence of the relevant virus.
Here infectious disease and infectious disease course are imported from IDO Core, and are subclasses, respectively, of disease and disease course , which are imported from OGMS.
Viral epidemiology.
Changes in viral disease and infection incidence are among the targets of epidemiological investigation. VIDO imports from IDO Core:
infectious disease incidence = def Quality that inheres in an organism population and is the number of realizations of an infectious disease for which the infectious disease course begins during a specified period.
infectious disease incidence rate = def Quality that inheres in an organism population and is the infectious disease incidence proportion per unit time.
infectious disease incidence proportion = def Quality that inheres in an organism population and is the proportion of members of the population not experiencing an infectious disease course at the beginning of a specified period and in whom the infectious disease begins during the specified period.
organism population = def Aggregate of organisms of the same species.
Additionally, VIDO imports from IDO Core other important epidemiological terms, such as infection prevalence , infectivity , and infectious disease mortality rate . Each are specifically dependent entities inhering in some material entity, though not always in some organism population. For example, infectivity is a quality inhering in instances of pathogen . Additionally, VIDO imports from IDO Core:
infection incidence = def Quality that inheres in an organism population and is the number of organisms in the population that become infected with a pathogen during a specified period.
on which infectious disease incidences depend, as infectious disease realizations require infection.
A quality process profile is a type of process which tracks changes of specific qualities in material entities over time [ 26 ]. For example, a patient’s temperature will likely fluctuate over time, as will many other qualities of the patient. The specific fluctuations of temperature in the patient over time is a process profile , which reflects common abstractions used in clinical diagnosis and testing and manifesting in charts prepared from time-series data. Changes in qualities of clinical interest may follow several patterns, each of which can be defined as a subclass of process profile . A patient’s temperature may exhibit a linear increase followed by a linear decrease. Similarly, there are process profile instances of cyclical patterns, for instance the seasonal patterns of influenza [ 87 ]. Such patterns can be tracked in VIDO by classes such as:
viral disease incidence profile = def Infectious disease incidence profile comprised of a series of determinate viral disease incidence qualities caused by a specific virus in a population over time.
viral disease incidence proportion profile = def Infectious disease incidence proportion profile comprised of a series of viral disease incidence proportion qualities caused by a specific virus per unit time.
viral disease incidence rate profile = def Infectious disease incidence rate profile comprised of a series of viral disease rate qualities caused by a specific virus per unit time.
Extending VIDO to CIDO
VIDO serves as a bridge between IDO Core and the IDO extension ontologies representing specific viral diseases. Of particular importance during the pandemic has been the Coronavirus Infectious Disease Ontology (CIDO; https://bioportal.bioontology.org/ontologies/CIDO ), developed by the He Group at the University of Michigan. CIDO provides terminological content that facilitates representations of coronavirus genome, protein structures, epidemiological surveillance, vaccine development, and treatment options. The ontology has been used to annotate data pertaining to 136 known anti-coronavirus drugs [ 6 ], as well as in the identification of approximately 110 candidate drugs [ 7 ] for potential drug repurposing projects with respect to COVID-19 [ 88 ]. More recently, CIDO has been employed as a general framework for understanding host-pathogen interactions [ 78 ]. IDO Core, VIDO, and CIDO development teams work together closely in the interest of ensuring ontology alignment.
CIDO will extend from VIDO by adopting, among other terms:
coronavirus disease = def Viral disease inhering in a coronavirus disorder.
coronavirus disease course = def Viral disease course that is the realization of some coronavirus disease and has as a participant a coronavirus.
This extension example illustrates a downward population recipe useful for aligning CIDO terms to VIDO, by starting with a given virus term from the latter, and restricting subclasses based on features of coronaviruses and associated diseases. Moreover, common coronavirus features can be reused from OBO ontologies to complement the CIDO characterization of the virus, such as the viral envelop glycoprotein spikes [ 89 , 90 ]. Some such terms are specializations of terms from the Protein Ontology ( https://bioportal.bioontology.org/ontologies/PRO ), e.g. spike glycoprotein (SARS- CoV-2) . CIDO covers existing and novel coronaviruses in general, and so provides resources for detailed comparison of coronavirus biological profiles. Fig 4 illustrates various links between VIDO and CIDO, and the IDO Core and GO ontologies.
https://doi.org/10.1371/journal.pone.0285093.g004
SARS-CoV-2 pathogenesis.
Characterizing pathogenesis to COVID-19 is aided by terms such as:
COVID-19 = def Coronavirus disease inhering in a SARS-CoV-2 disorder.
COVID-19 disease course = def Coronavirus disease course that is the realization of some COVID-19 disease and has participant SARS-CoV-2.
Ontologically precise representation of COVID-19 pathogenesis is crucial for understanding the range of symptoms and signs which appear across demographics [ 91 – 94 ]. Ontological representation of COVID-19 pathogenesis is aided by reusing OBO Foundry ontology terms, resulting in the following definition:
SARS-CoV-2 pathogenesis = def Coronavirus pathogenesis process realization of an infectious
disposition inhering in SARS-CoV-2 or a SARS-CoV-2 population, having at least the proper process parts:
- pathogen transmission,
- establishment of localization in host,
- process of establishing an infection, and
- appearance of a virus disorder.
Instances of SARS-CoV-2 pathogenesis are asserted as part of some COVID-19 disease course . The term coronavirus pathogenesis will ultimately be imported to CIDO, as a subclass of the VIDO term viral pathogenesis , itself a subclass of:
pathogenesis = def Process that generates the ability of a pathogen to induce disorder in an organism.
which is imported from GO. As defined, pathogenesis is a success term [ 25 ], in that it encompasses formation of disorder in an entity. Of course, this is not meant to imply that SARS-CoV-2 infections necessarily lead to successful pathogenesis. SARS-CoV-2 infections, for example, may not lead to host disorder, in which case there would be no pathogenesis. Just as important as it is to represent SARS-CoV-2 pathogenesis to COVID-19, adequate representation of the target domain requires representation of pathogenesis to acute respiratory distress syndrome (ARDS), one of the leading causes of death in those infected by SARS-CoV-2 [ 95 , 96 ]:
acute respiratory distress syndrome = def Progressive and life-threatening pulmonary distress in the absence of an underlying pulmonary condition, usually following major trauma or surgery.
which may be imported from the Experimental Factor Ontology ( https://bioportal.bioontology.org/ontologies/EFO ). Similar remarks apply to other diseases associated with SARS-CoV-2 pathogenesis.
SARS-CoV-2 pathogenesis involves transmission of SARS-CoV-2 virions. From PTRANS ( https://bioportal.bioontology.org/ontologies/PTRANS ) is imported:
pathogen transmission process = def Process during which a pathogen is transmitted directly or indirectly to a new host.
From which SARS-CoV-2 specific terms can be constructed. Additionally, IDO Core provides important role terms relevant to pathogen transmission, such as:
pathogen transporter role = def Role borne by a material entity in or on which a pathogen is located, from which the pathogen may be transmitted to a new host.
An important subclass fomite role– roughly, a pathogen transporter role borne by a non-living entity–may feature in SARS-CoV-2 transmission via instances of fomite role bearing:
respiratory droplet = def Respiratory secretion composed of a bounded portion of liquid which maintains its shape due to surface tension.
respiratory droplet SARS-CoV-2 fomite = def Respiratory droplet fomite with SARS-CoV-2 part.
Knowledge of transmission steps supports strategies designed to break the transmission chain.
Worth noting is that the OBO library ontology APOLLO-SV ( https://bioportal.bioontology.org/ontologies/APOLLO-SV ) also contains terms, such as contact tracing and quarantine control strategy , which may be leveraged to represent virus-specific transmission control strategies.
SARS-CoV-2 replication.
SARS-CoV-2 pathogenesis involves replication in a host. The term virus replication is defined in VIDO as a subclass of the IDO Core term replication , specifically:
virus replication = def Replication process in which a virus containing some portion of genetic material inherited from a parent virus is replicated.
And instances of viral disease course and virus pathogenesis have virus replication as parts. SARS-CoV-2 replication occurs within an:
incubation process = def Process beginning with the establishment of an infection in a host and ending with the onset of symptoms by the host, during which pathogens are multiplying in the host.
Which occupies an incubation interval and may precede a communicability interval . The corresponding process during which SARS-CoV-2 hosts bear a contagiousness disposition has proper part some latency process which itself has an eclipse process as part::
communicability interval = def One-dimensional temporal region during which a pathogen host bears a contagiousness disposition.
latency process = def Process beginning with the establishing of an infection in a host and ending when the host becomes contagious, during which pathogens are multiplying in the host.
eclipse process = def Process beginning with the establishment of a virus in a host and ending with the first appearance of a virion following viral release, during which an infecting virus is uncoating to begin genome replication.
The last are specific to viruses, and so specific to VIDO. Viral dormancy is a virus-specific term from VIDO occurring over a:
viral dormancy interval = def One-dimensional temporal region on which a virus is no longer replicating but remains within a host cell and which may be reactivated to begin replication again.
Viral dormancy is characteristic of familiar viruses such as varicella zoster and herpes simplex .
VIDO includes as a temporal subdivision of a virus developmental process:
virus generative stage = def Infectious structure generative stage that is a temporal subdivision of a virus developmental process.
Subclasses of which include the stages through which viruses may proceed during replication:
virus attachment stage = def Virus generative stage during which a virion protein binds to molecules on the host surface or host cell surface projection.
virus penetration stage = def Virus generative stage during which a virion or viral nucleic acid breaches the barriers of a host.
SARS-CoV-2 attachment stage = def Virus attachment stage during which SARS-CoV-2 bonds with a host cell.
SARS-CoV-2 penetration stage = def Virus penetration stage during which SARS-CoV-2 penetrates a host cell.
SARS-CoV-2 susceptibility.
Only cells with certain features are susceptible to SARS-CoV-2 infection [ 16 ]. For example, successful infection in humans typically involves SARS-CoV-2 attachment to alveolar epithelial cells through angiotensin-converting enzyme 2 (ACE2) receptors [ 97 – 99 ]. Cells lacking ACE2 receptors seem protected from attachment by SARS-CoV-2. Those with receptors can be represented in CIDO using:
SARS-CoV-2 adhesion susceptible cell = def Virus adhesion susceptible cell with a functional receptor part bearing an adhesion disposition realized in a SARS-CoV-2 attachment stage.
adhesion disposition = def Disposition borne by a macromolecule that is the disposition to participate in an adhesion process.
Where adhesion disposition is imported from IDO Core and virus adhesion susceptible cell defined in VIDO. The ACE2 functional receptor is defined in the Protein Ontology ( https://bioportal.bioontology.org/ontologies/PRO ):
angiotensin-converting enzyme 2 = def A protein that is a translation product of the human ACE2 gene or a 1:1 ortholog thereof.
Attachment is frequently followed by cell penetration, where cell cleavage is aided by transmembrane protease serine 2 (TMPRSS2) prior to SARS-CoV-2 cell membrane fusion [ 100 , 101 ]. These observations motivate introducing terminological content for defining SARS-CoV-2 penetration susceptible cell such as:
SARS-CoV-2 penetration disposition = def Virus penetration disposition borne by a functional receptor complex that is the disposition to participate in a SARS-CoV-2 penetration process.
Ontological representation of the SARS-CoV-2 replication cycle provides targets for disruption or regulation of that cycle, which is important to rational drug design [ 102 – 106 ]:
negative regulation of SARS-CoV-2 attachment = def Negative regulation of coronavirus replication process that stops, prevents, or reduces the frequency of some SARS-CoV- 2 attachment stage.
negative regulation of SARS-CoV-2 penetration = def Negative regulation of coronavirus replication that stops, prevents, or reduces the frequency of some SARS-CoV-2 penetration stage.
Following our strategy of linking VIDO and CIDO, parent classes of negative regulation of coronavirus classes have a proper home in CIDO, while their parent classes–negative regulation of viruses more generally–have a proper home in VIDO.
Annotations.
Coverage in VIDO and CIDO can be illustrated by annotation of coronavirus research articles.
Consider the following overview of SARS-CoV-2 pathogenesis (compare bold with Fig 5 ): Following replication , cell lysis of SARS-CoV-2 coronavirus virions causes host cells to release molecules which function to warn nearby cells . When recognized by epithelial cells , endothelial , and alveolar macrophages , proteins such as IL-6 , IP-10 , and MCPI , are released which attract T cells , macrophages , and monocytes to the site of infection , promoting inflammation . In disordered immune systems , immune cells accumulate in the lungs , then propagate to and damage other organs . In normal immune systems , inflammation attracts T cells which neutralize the virus at the site of infection . Antibodies circulate , preventing SARS-CoV-2 infection , and alveolar macrophages recognize SARS-CoV-2 and eliminate virions via phagocytosis [ 92 , 107 , 108 ].
https://doi.org/10.1371/journal.pone.0285093.g005
In a more ontologically oriented language, we speak of the relevant part of a host’s immune response as being disposed to manifest a response that eliminates SARS-CoV-2 infection, while SARS-CoV-2 has a disposition to block manifestation of this immune system response. Consider next a color-coded selection from the Lancet [ 109 ] concerning SARS-CoV-2:
“The viral load s in throat swab s and sputum sample s peaked at around 5–6 days after symptom onset , ranging from around 10^4 to 10^7 copies per mL during this time .”
SARS-CoV-2 infected hosts contain the highest concentration of SARS-CoV-2 virions–the viral load– during the incubation interval [ 110 ]. Viral load is a common measurement of the proportion of virions to fluid, and for SARS-CoV-2 is frequently measured from host sputum. VIDO provides the resources for annotating virus quantification:
viral load = def Quality inhering in a portion of fluid that is the proportion of virions to volume of that portion of fluid
Our color-coding of the above passage from the Lancet models term reuse across existing ontologies. For example, developers can use VIDO and CIDO terms alongside terms from the Common Core Ontology ( https://github/com/CommonCoreOntology/CommonCoreOntologies ) such as is measured by , measurement information content entity , has integer value , uses measurement unit , and milliliter measurement unit . Other virus quantification metric terms, such as multiplicity of viral infection ‐ the ratio of virions to susceptible cells in a target area–can be found in VIDO as well.
Motivated to standardize virus ontology extensions of IDO Core, we have developed VIDO and provided a recipe for connecting VIDO to more virus-specific extensions of IDO Core, illustrated by connecting VIDO to CIDO. Summarizing our results, we have introduced acellular structure as a parent class to virus , motivated using the Baltimore classification to model viruses rather than the International Committee on Taxonomy of Viruses classification, revised IDO Core’s pathogen and host classes to accommodate acellular structures, and extended IDO Core’s infectious disease , infectious disease course , and infectious disease epidemiology classes to cover viruses. We then introduced bridge classes in VIDO to better align IDO Core and CIDO, illustrating throughout how CIDO terminological content can be extended and enriched to represent SARS-CoV-2 pathogenesis, associated transmission processes, virus transporters, replication stages and associated temporal extents, as well as pathogenesis regulation. Our attention was then turned to annotations of texts concerning SARS-CoV-2 and COVID-19, by which we highlighted how ontologies using common vocabularies may be seamlessly interwoven to provide broad annotation coverage for the domain.
VIDO and CIDO are not the only ontologies developed to support curation of COVID-19 data [ 111 – 114 ]. However, most alternatives are stand-alone initiatives, and so subject to the silo problems typically found in ontologies developed outside the scope of the OBO Foundry and with no attention to its principles. That said, VIDO and CIDO developers have participated in harmonization efforts aimed at semantic integration across COVID-19 ontologies [ 115 ]. Notably, harmonization efforts have resulted in the deprecation of the COVID-19 Infectious Disease Ontology (IDO-COVID-19)–introduced in a preprint version of the current paper [ 116 ]–with parties agreeing its scope was subsumed by CIDO. Additionally, VIDO and CIDO have been used to highlight ontology conflict resolution strategies [ 117 ]. It is not uncommon for ontology researchers working independently in nearby domains to construct overlapping ontology content.
The harmonization efforts of the VIDO and CIDO development teams signal to the wider ontology community our willingness to reuse terms where possible and obsolete terms or cede terms to other ontologies when needed.
VIDO and CIDO enable extensive representation of virus-related research. The very scope of VIDO provides challenges, however, as does the specificity of CIDO. For these reasons, attempts have been made to foster community-driven development of both. The development team for each ontology spanned disciplines in the life sciences, and to ensure the computational viability of the formal representation of each ontology, included specialists in logic. Often, terms were developed then presented to domain specialists for vetting, after which they were refined through discussion.
As in the case of all scientific ontologies, refinement will continue as research advances, and further collaborators are welcome. Interested parties may contact the corresponding author to be invited to on-going VIDO development meetings and may contact co-author He for invitation to development meetings concerning CIDO. Additionally, collaborators are encouraged to raise issues on respective GitHub issue trackers for VIDO ( https://github.com/infectious-disease-ontology-extensions/VIDO ) and CIDO ( https://github.com/CIDO-ontology/cido ).
The existence of IDO Core extensions covering infectious disease-causing entities other than viruses suggests a need for the creation of reference ontology extensions of IDO covering bacteria, fungi, and parasites. To that end, development of the Bacteria Infectious Disease Ontology is underway ( https://github.com/infectious-disease-ontology-extensions/Bacteria-Infectious-Disease- O ntology) as is the development of the Fungal Infectious Disease Ontology ( https://github.com/SydCo99/MIDO ). The methodology illustrated in the development of VIDO provides a recipe for such reference ontology creation. Additionally, the methodology illustrated in the development of CIDO provides a recipe for the creation of novel virus-specific ontologies, namely, by extending them from existing virus ontologies. Adoption of these methodologies by developers during ontology construction will significantly reduce the labor involved in ontology creation. Related, linking research on infectious diseases to developments on non-infectious diseases is no less important than our focus here. In this respect, VIDO and CIDO benefit from alignment with IDO Core, which itself aligns with the Ontology of General Medical Science (OGMS), whose scope extends beyond infectious disease. What this means in practice is that, for example, kidney disease [ 118 ] and cancer [ 119 ] researchers accurately using the OGMS vocabulary to represent data, invariably use ontology terms and methodologies common to IDO Core, VIDO, and CIDO, thereby lowering barriers to data integration and interoperability.
VIDO and CIDO are being used to annotate host-coronavirus protein-protein interactions, in the interest of developing more effective treatment strategies for those infected by SARS-CoV-2 or variants [ 37 ]. While various treatments have been authorized for emergency use [ 120 ], there is significant room for improvement. Rather than focus on a single drug to treat infected patients, VIDO and CIDO developers have pursued investigating drug cocktail strategies to improve treatment outcomes. Foundational to these investigations has been proper characterization of viral proteins playing different roles in host-coronavirus interactions which impact pathogenesis [ 78 ].
From another direction, VIDO and CIDO have been used in automated electronic health-record annotation [ 121 ], in particular those involving COVID-19 data, which highlights the importance of providing researchers with terminological content relevant to nearby domains. Recent developments at the intersection of ontology engineering and machine learning research have, moreover, motivated the need for formally well-defined ontologies in machine learning pipelines using minimal data [ 122 ]. By exploiting formal axioms in, for example, the Gene Ontology, impressive zero-shot predictions for protein functions can be generated [ 123 ]. We believe the formal axiomatization of VIDO makes it a particularly promising ontology for inclusion in zero-shot research and intend to explore how VIDO may supplement machine learning efforts in future work.
Ontologies provide important tools for overcoming contemporary big data challenges. It is incumbent on working ontologists representing life science research to seek harmonization with nearby ontologies, else we run the risk of reinstating the same big data challenges ontologies have previously been so successful at addressing. VIDO represents a substantial effort to characterize viruses in general, in a collaborative, computationally tractable manner. CIDO too represents a significant effort to characterize coronaviruses in a specific, no less collaborative, no less computationally tractable manner. Connecting VIDO to CIDO improves semantic interoperability among IDO Core-conformant infectious disease ontologies and, moreover, improves interoperability with other BFO-conformant ontologies, ranging from the OBO Foundry to numerous other ontology projects employing BFO as a top-level architecture. Consequently, our work provides researchers resources for gathering and coordinating life science data while avoiding issues that so frequently undermine automating integration and analyses of the data flood in which we so often find ourselves [ 124 – 126 ].
Acknowledgments
Many thanks to Asiyah Yu Lin for assistance in VIDO development and harmonization; to Darren Natale and Sydney Cohen for helpful critical feedback on earlier drafts; to Amanda Hicks and Neil Otte for comments on the VIDO rdf files; to participants at the 2022 International Conference on Biomedical Ontologies, with particular thanks to Alexander Diehl and Chris Stoeckert for their helpful feedback before, during, and after the conference. Figures were designed by or in consultation with Rain Yuan.
- View Article
- PubMed/NCBI
- Google Scholar
- 10. Arp R, Smith B, Spear A. (2015) Building Ontologies with Basic Formal Ontology. Cambridge, MA: MIT Press.
- 15. Cowell LG, Smith B. (2010) Infectious Diseases Ontology. In: Sintchenko V, editor. Infectious Disease Informatics. New York, NY: Springer. p. 373–95.
- 18. The Open Biomedical Ontologies Foundry. http://obofoundry.org/ Accessed 11 Mar 2023.
- 20. Baader F, Horrocks I, Lutz C, Sattler U. (2017). An Introduction to Description Logic. Cambridge University Press. https://doi.org/10.1017/9781139025355
- 21. Homer H, Selman AL. (2011) Computability and Complexity Theory: Texts in Computer Science. 2nd Edition. Springer.
- 27. Smith B. (2012) On Classifying Material Entities in Basic Formal Ontology. Interdisciplinary Ontology: Proceedings of the Third Interdisciplinary Ontology Meeting. Tokyo: Keio University Press. 1–13.
- 32. Goldfain A, Smith B, Cowell LG. (2010) Dispositions and the infectious disease ontology. In: Galton A, Mizoguchi R, editors. Formal Ontology in Information Systems: Proceedings of the 6th International Conference (FOIS 2010). Amsterdam: IOS Press.
- 44. Claverie JM. (2008) Encyclopedia of Virology 3rd Edition.
- 47. He Y. (2022). Ontological Classification of Self-replicating Organic Structures. Preprint. 1–8. https://doi.org/10.31219/osf.io/n2zkh
- 52. Dimmock NJ, et. al. (2007). Introduction to Modern Virology. 6th edition. Blackwell Publishing.
- 56. Bauman R. (2017). Microbiology with Disease Taxonomy. Pearson Publishing.
- 69. Ananthanarayan R., Paniker J. (2005). Textbook of Microbiology. 7th Edition.
- 78. Yu H, et al. (2022) A New Framework for Host-Pathogen Interaction Research. Frontiers in Immunology. https://doi.org/10.3389/fimmu.2022.1066733
- 84. Centers for Disease Control and Prevention. (2020). Coronavirus Disease 2019 (COVID-19) 2020 Interim Case Definition, Approved April 2, 2020. https://ndc.services.cdc.gov/case-definitions/coronavirus-disease-2019-2020/ Accessed Mar 11 2023.
- 85. Council of State of Territorial Epidemiologists. (2023). Standardization Surveillance Case Definition and National Notification for 2019 Coronavirus Disease (COVID-19). https://ndc.services.cdc.gov/conditions/coronavirus-disease-2019-covid-19/ Accessed Mar 11 2023.
- 86. Centers for Disease Control and Prevention. (2023). Coronavirus Disease 2019 (COVID-19) 2023 Case Definition, Approved February 28, 2023. https://ndc.services.cdc.gov/case-definitions/coronavirus-disease-2019-covid-19/ Accessed Mar 11 2023.
- 103. Moderna Announces Phase 3 COVE Study of mRNA Vaccine against COVID-19 (mRNA-1273) Begins. Press Release (2020); https://investors.modernatx.com/news-releases/news-release-details/moderna-announces-phase-3-cove-study-mrna-vaccine-against-covid.
- 104. A Study to Evaluate Efficacy, Safety, and Immunogenicity of mRNA-1273 Vaccine in Adults Aged 18 Years and Older to Prevent COVID-19, ClinicalTrials.gov Identifier: NCT04470427, https://clinicaltrials.gov/ct2/show/NCT04470427 .
- 105. Pfizer and Biotech Choose Lead mRNA Vaccine Candidate against COVID-19 and Commence Pivotal Phase 2/3 Global Study. Press Release. (2020). https://www.pfizer.com/news/press-release/press-release-detail/pfizer-and-biontech-choose-lead-mrna-vaccine-candidate-0
- 111. WHO COVID-19 Rapid Version CRF. https://bioportal.bioontology.org/ontologies/COVIDCRFRAPID Accessed Mar 11 2023.
- 112. COVID-19 Surveillance Ontology. https://bioportal.bioontology.org/ontologies/COVID19 Accessed Mar 11 2023.
- 113. Linked COVID-19 Data Ontology. https://github.com/Research-Squirrel-Engineers/COVID-19 . Accessed Mar 11 2023.
- 114. COVID-19 Research Knowledge Graph. https://github.com/nasa-jpl-cord-19/covid19-knowledge-graph . Accessed Mar 11 2023.
- 124. Apolinario-Arzube Ó. et al. (2020) CollaborativeHealth: Smart Technologies to Surveil Outbreaks of Infectious Diseases Through Direct and Indirect Citizen Participation. In: Silhavy R. (eds) Applied Informatics and Cybernetics in Intelligent Systems. Advances in Intelligent Systems and Computing. 1226. https://doi.org/10.1007/978-3-030-51974-2_1
Ontology extension with NLP-based concept extraction for domain experts in catalytic sciences
- Regular Paper
- Open access
- Published: 15 July 2023
- Volume 65 , pages 5503–5522, ( 2023 )
Cite this article
You have full access to this open access article
- Alexander S. Behr 1 ,
- Marc Völkenrath 1 &
- Norbert Kockmann 1
2612 Accesses
Explore all metrics
Ontologies store semantic knowledge in a machine-readable way and represent domain knowledge in controlled vocabulary. In this work, a workflow is set up to derive classes from a text dataset using natural language processing (NLP) methods. Furthermore, ontologies and thesauri are browsed for those classes and corresponding existing textual definitions are extracted. A base ontology is selected to be extended with knowledge from catalysis science, while word similarity is used to introduce new classes to the ontology based on the class candidates. Relations are introduced to automatically reference them to already existing classes in the selected ontology. The workflow is conducted for a text dataset related to catalysis research on methanation of CO \(_2\) and seven semantic artifacts assisting ontology extension by domain experts. Undefined concepts and unstructured relations can be more easily introduced automatically into existing ontologies. Domain experts can then revise the resulting extended ontology by choosing the best fitting definition of a class and specifying suggested relations between concepts of catalyst research. A structured extension of ontologies supported by NLP methods is made possible to facilitate a Findable, Accessible, Interoperable, Reusable (FAIR) data management workflow.
Similar content being viewed by others
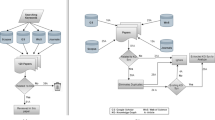
Recent trends in knowledge graphs: theory and practice
BEAR: Revolutionizing Service Domain Knowledge Graph Construction with LLM
A Semantic Search System for the Supremo Tribunal de Justiça
Avoid common mistakes on your manuscript.
1 Introduction
In the current research data management, interconnection of the data produced and its interpretation are essential for comprehensible deductions of new knowledge. Research data need to be FAIR (Findable, Accessible, Interoperable, and Reusable) by humans and machines in order to make proper use of data recorded in experiments, e.g., in electronic laboratory notebooks [ 1 , 2 ]. While a researcher can easily grasp and interpret semantics expressed in texts using their implicit knowledge [ 3 ], a machine cannot perform this without having a representation of such knowledge embedded. Here, ontologies are used to describe implicit knowledge in an explicit way as they represent explicit specifications of conceptualizations [ 4 ]. Ontologies are informatic constructs used to represent relations among classes, such as catalyst or reactor .
As classification is an important concept of ontologies, the hierarchic sorting of the classes in turn represents the backbone of the ontologies. While the connection of classes within ontologies is important for their definition, short definition sentences (definition strings) are used as class annotation. This helps humans using the ontology to define and understand the classes of the ontology properly. Not only ontologies can be used to obtain definition strings for classes. Thesauri also provides classes with respective definition strings, such as the NCIT [ 5 ]. While they do not necessarily have semantic relations between their concepts like ontologies, they often contain more concepts and respective definition strings than ontologies.
For a domain expert who wants to represent the domain knowledge in an ontology, the hurdle to include ontology classes in the correct form into an ontology might be quite challenging and time consuming. Being experts in certain scientific fields, domain experts might also omit some knowledge because it is considered as trivial. Extending an ontology for own needs often is tedious work [ 6 , 7 ]; thus, approaches are desired to simplify extension of ontologies and reduce consumed time for domain experts in order to raise acceptance of ontologies.
Since already existing ontologies do not necessarily contain all classes essential to describe the respective knowledge domain, an automated extension of ontologies is desirable. In addition, plenty of information is presented in scientific research in textual form, e.g., research papers by many domain experts. Those research papers contain a high number of domain-specific vocabulary. Using techniques from Natural Language Processing (NLP), in turn, can help to automate the setup of ontologies based on unstructured (natural) text as contained in research papers [ 8 ]. Exemplarily, by using Part of Speech (POS) tagging, nouns can be sorted out automatically from a given text and afterward be brought to their nominative singular form by lemmatizing.
While methods exist to extract ontologies from documents fully automatically, they usually provide ontologies that are not really useful for further reuse [ 9 ]. The ConTrOn (continuously trained ontology) project shows how user feedback can be integrated by a human-in-the-loop system [ 10 , 11 ]. Here, a domain-specific ontology is augmented automatically and extended on basis of textual data and external sources of knowledge such as Wikidata and WordNet [ 12 ]. While the approach represents a solution to integrate information from data sheets to ontologies, the extraction of knowledge and relations between ontology classes from text is missing. In addition, a comparison of classes and their definitions with WikiData is done, while a comparison of classes and their definitions with other ontologies also would make sense. This is due to the fact that other ontologies also might contain knowledge not represented in WikiData, as ontologies focus more on expert knowledge.
The scope of this work is to use NLP techniques to extract vocabulary relevant to a domain of knowledge represented in a set of scientific papers. This vocabulary then is annotated by definitions derived from existing semantic artifacts (such as ontologies and thesauri) to help domain experts in later steps with sorting out the classes best fitting to the domain of knowledge. In addition, NLP is used to assist domain experts by including suggested classes automatically into an existing ontology and suggesting semantic relations between the classes based on text vectorization models of the texts. As classes should be only defined once to avoid ambiguities, already existing definitions of the added classes are included in the resulting extended ontology to later aid domain experts with selection of the most fitting definition to the automatically added classes. Thus, words necessary to describe a knowledge domain are included in a holistic, automated way into an ontology by including knowledge from a variety of scientific papers on a certain topic of interest.
2 Methodological background
This section describes the text dataset and the semantic artifacts used later to apply the workflow. Furthermore, the vectorization with Word2Vec is explained as its cosine similarity and min_count parameter serve as key classificators of later results.
2.1 Text dataset
The dataset deals with scientific publications focusing on catalytic methanation reactions. Here, a total of 25 research papers and three review papers are collected on research topics of methanation of CO \(_2\) . Besides continuous text, the dataset also contains other data, such as figures, diagrams, tables, and chemical formulas. In addition, the header and footer of pages often contains text with no further domain-specific information. Thus, preprocessing of the scientific publications focuses on extraction of token of the continuous text of the text dataset and omitting data waste. The method of preprocessing is described further in Sect. 3.1 . The publications used as text dataset in this work are presented in Table A1 in Appendix A.
2.2 Semantic artifacts
For extension and annotation of ontologies, five ontologies and two thesauri are selected based on the set of ontologies deemed as important to the catalysis research domain by the NFDI4Cat project [ 1 , 13 , 14 ]. The Allotrope Foundation Ontology (AFO) [ 15 ], Chemical Entities of Biological Interest (CHEBI) [ 16 ], and Chemical Methods Ontology (CHMO) [ 17 ] are closely related to the chemical domain and contain concepts related to chemical experiments in laboratories. In contrast, the BioAssay Ontology (BAO) [ 18 ] focuses on biological screening assays and their results. While the scope of the BAO might not be intuitively fitting to the chosen text dataset, certain concepts are contained in the BAO such as chemical roles of substances (e.g., catalyst), which also play a role in the text dataset. Similar to that, the scope of the Systems Biology Ontology (SBO) [ 19 ] is system biology and computational modelling. Similar to the BAO, it is chosen as it also contains relations regarding substances and also general laboratory contexts, which also are contained in the text dataset.
In addition to these ontologies, two thesauri are used: the IUPAC Compendium of Chemical Terminology (IUPAC-Goldbook) [ 20 ] and the National Cancer Institute Thesaurus (NCIT) [ 5 ]. They cover vast amounts of chemical species and domain-specific words of the chemical domain of knowledge while also providing definition strings for the respective words. In order to be processed properly, all ontologies and the NCIT were used in the OWL file format in RDF/XML syntax and converted to OWL (RDF/XML), when only available in, e.g., TTL-serialization using Protégé [ 21 ]. IUPAC-Goldbook was used in json-file format as provided by the homepage [ 20 ]. The semantic artifacts discussed and used in this work are listed in Table 1 along with the number of classes or concepts they contain.
2.3 Vectorization with Word2Vec
After preprocessing the data, it is further used to get semantic similarity of the token extracted. For this, the algorithm Word2Vec implemented in the python module gensim is used [ 22 ]. It vectorizes words to learn relations between token and thus, represents a statistical method. Using the preprocessed text as input, Word2Vec creates a vocabulary, vectorizing each word to a vector of user defined length. While a longer vector corresponds to a higher dimension of the vector space used for the vectorization, it also results in longer computational time resulting in a trade-off between computational time and expressivity of the vectors [ 23 ]. The similarity of two concepts can be calculated with the help of the cosine similarity by calculating the cosine of the angle \(\varphi \) between two vectors \(\vec {a}\) and \(\vec {b}\) using the equation
resulting in a value close to one for token close to each other and close to minus one for token far away from each other. Because this is a statistical method, the frequency of occurrence of the token within the text corpus is important to consider. This is reflected in the Word2Vec parameter min_count setting the number of occurrences in the text corpus, a token must have at least to be considered by the model. The higher this number is set, the smaller the overall considered number of words gets; thus, the model focuses only on the most occurring words. A lower min_count is more prone to include token based on, e.g., typing errors or are those of less relevance to the overall domain of knowledge represented in the text corpus.
To obtain information from scientific papers, the text corpus first needs to be extracted and preprocessed to be viable in further steps. Part of Speech tagging (POS-tagging) is used to extract only nouns as candidates for new ontological classes. Searching for these extracted concepts (token) in already existing semantic artifacts (ontologies or thesauri) yields token annotated with definition strings and linkage to the respective semantic artifact, the definition was taken from. To extend an already existing ontology with concepts based on the found token, a Word2Vec model is trained that vectorizes the text data. This in turn allows to output tokens with small cosine similarity to the already contained classes of an ontology and introducing those as new classes in the ontology. In addition, relations to denote semantic relation of these classes are posed, to connect the already contained ontology class to the automatically created classes based on Word2Vec. This overall workflow is depicted in Fig. 1 with the start of the workflow denoted in red and the output of the workflow in green. The following sections explain the main three steps of this general workflow in more detail. First, the text extraction is explained in detail, as the text corpus first needs to be extracted and preprocessed to be useful in further steps. Then, POS-tagging and search of the token in already existing ontologies takes place to annotate the extracted token. In the final step, the extension of an ontology by new classes based on the text dataset is explained.
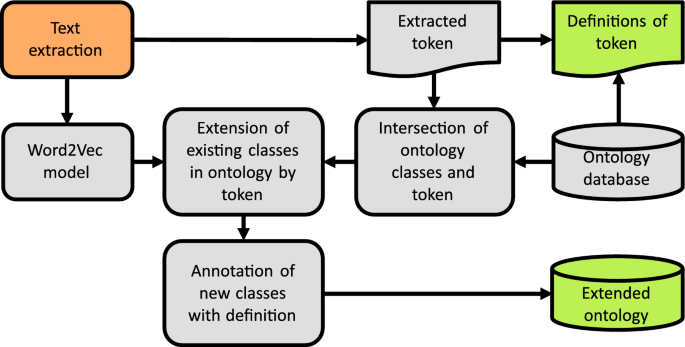
Overall workflow conducted in this work to extract token from text, supply them with definitions based on ontologies and extend ontologies with new classes. The red box denotes the start of the workflow, while the output boxes are colored green (Color figure online)
3.1 Text extraction
Data from the text dataset contains, besides textual information, also information that is either non-textual or meaningless. Non-textual information, such as figures, can be neglected to reduce the file size. Text fragments without further domain-specific information also can be deleted to get a more condensed text dataset.
Thus, all figures, tables, and diagrams that do not contain complete sentences are removed first by hand with acrobat reader [ 24 ] and using the python module pdfminer [ 25 ]. Annotations and tables containing text in bullet point form are considered individually. Furthermore, lists such as references, table of figures, and table of nomenclature are removed, as these usually represent a list of individual words and symbols that do not reflect any context or relations. However, definition directories containing technical terms explained by short sentences are not removed, since they can contain relevant information. Subsequently, textual content that occurs repeatedly is removed, such as a DOI contained in the footer of each page or the journal name in the header of each page. These have no informative value and would negatively influence the creation of the model. Captions are also removed, since their information content is marginal and repeat often without enhancement of the textual dataset (such as“Introduction” or“Conclusion”). Those cleaned files of the dataset are read in as strings using python code as a singular string such that each dataset contains a single string. The module SpaCy [ 26 ] is used to apply POS-tagging. This transforms the read-in string into a nested list, where each sentence is represented as list entry in a separate list. Using interpunction and space characters as separators, token are extracted and lemmatized using the vocabulary en_core_web_sm . This categorizes each word contained in each sentence regarding its lexical category (e.g., noun, verb, number,...).
3.2 Annotation of extracted token
As ontology classes are mostly nouns, only token with categories “noun” and “proper noun” are retained from the dataset and used in further procedures. Thus, a search of those token in ontologies is performed to determine the amount of token contained in each ontology as a class. The result helps to decide, which ontology can be taken as basis in further extension steps. Further help is provided by extraction of definitions of classes contained as string values in the ontologies, enabling for an easy determination of the best definition by domain experts in later steps.
To choose a fitting ontology to the dataset and enrich it by the concepts gathered by pre-processing, existing definitions of token contained in the ontologies should be known. Thus, python code is produced, which loads ontologies based on a local database using owlready2 [ 27 ]. Then, all class labels as well as their definition strings are read in from the ontologies and stored as key-value pairs in dictionaries. Nested dictionaries are used to store all classes and their definitions of a single ontology in a dictionary with the ontology name as key and the dictionary containing class names and their definitions as value. Token found by text extraction, as discussed in Sect. 3.1 , is read in, and the dictionary is browsed for those token in class names. Finally, the number of found token per ontology can be accessed. In addition, the token is stored in a table along with the respective definitions, each assigned to its source ontology for later review of domain experts. The workflow of the code constructed for the annotation of extracted token is depicted in Fig. 2 . The red elements denote the needed input of the workflow, i.e., the ontology database and the token obtained by text extraction, while the output boxes are colored green.
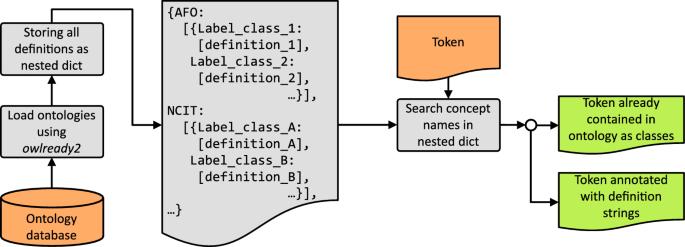
Workflow of the code constructed for the annotation of extracted token. The red elements denote the input of the workflow, while the output boxes are colored green (Color figure online)
3.3 Extension of an ontology by new classes based on text dataset
The Word2Vec model is trained on the textual data obtained by the methods discussed in Sect. 3.1 . Following [ 23 ], a vector size of 300 was set. While the Word2Vec model could be used for hierarchic clustering, the resulting clusters would not yield hierarchies in an ontological, semantic way. This is due to the nature of relations between token extracted by vectorization of concepts. As the text-clusters contain semantic similarities of words important for domains of knowledge, no classification and hierarchical information is obtained from the Word2Vec model. Thus, hierarchical clustering with, e.g., dendrograms, would not necessarily yield classifications (ontology classes and respective subclasses) of concepts. However, Word2Vec is able to give token with high cosine similarity to an initial input concept.
To use this functionality of similar token, the output of the workflow presented in Sect. 3.2 is used. The workflow not only annotates token of a text dataset with definitions contained in ontologies, but also can be used to output which token already are contained in each investigated ontology.
Picking the ontology with most common classes, these already contained classes are used as input for the Word2Vec model trained on the text dataset. The model then is used to retrieve the closest n token regarding cosine similarity of the input word. This is accompanied by a threshold value, restricting the amount of output token also with regards to the minimal cosine similarity allowed. This would allow for, e.g., setting a necessary minimal cosine similarity of 0.999, which would in turn only yield token very close to the input, while a minimal similarity of 0.8 would also include broader token, farther away in the vector space. As those token are most similar to the already contained ontology class, the ontology class and the token retrieved in this way by Word2Vec are assumed to have some kind of a semantic relationship.
If a token output by Word2Vec in this way is not already contained in the ontology, a new class has to be created, reflecting the token. To have an overarching class of newly included classes, not yet defined properly by semantic means, a class called w2vConcept is created as a subclass of owl:Thing class. Token output by the Word2Vec model and not yet contained in the ontology are then created as class. In addition, they are set to be subclasses of the also automatically created class w2vConcept , which in turn is set as subclass of the ontology root class owl:Thing . This is done to help in the later revision of the automatically created classes as they are more easy to find using an ontology editor, e.g., Protégé, when listed as subclass of the same class. Furthermore, this ensures that the integration of new classes does not disturb the semantic integrity of the ontology. The unique classes are also connected via an automatically created relationship to the classes deemed as similar by the Word2Vec model. This object property is called conceptually related to and is intended to ease the later definition of the exact relation between the two classes. To annotate the classes with missing definition strings, the workflow presented in Sect. 3.2 is used to search for definition strings of the newly created classes in other semantic artifacts. The code cannot decide by itself which definition might be more fitting when multiple definition strings are found. Thus, each definition string obtained is listed in a separate rdfs:comment of the class along with a note on the source of the definition.
After storing the resulting extended ontology, domain experts thus can go through newly added classes and easily accept or neglect the classes and modify the conceptually related to relation to a relation more fitting. This workflow of code to extend an ontology automatically is depicted in Fig. 3 . The ontology used as input is denoted red, while the extended ontology, which poses the output of the workflow, is colored green.
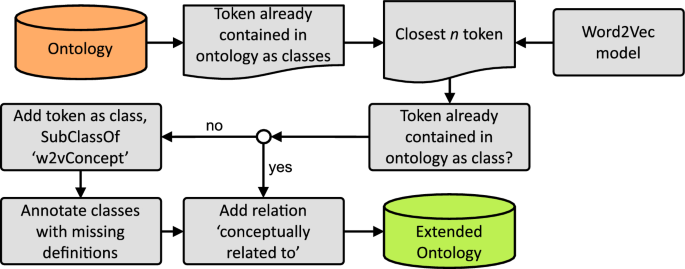
Workflow of code to extend an ontology by new classes based on text dataset. The ontology used as input is denoted red, while the extended ontology, which poses the output of the workflow, is colored green (Color figure online)
4 Results and discussion
The textual data of 28 scientific texts are preprocessed and extracted according to Sect. 3.1 . This yields a dataset of overall 858,014 symbols which result in 4,170 noun token identified for further use in the workflows proposed in Sect. 3 . Applying different min_count parameters in the range min_count \(=[1...25]\) yields different amounts of token as shown in Fig. 4 . While higher min_count parameters yield lower amounts of token, the token contained is deemed the more important ones, as they occur more often in the dataset.
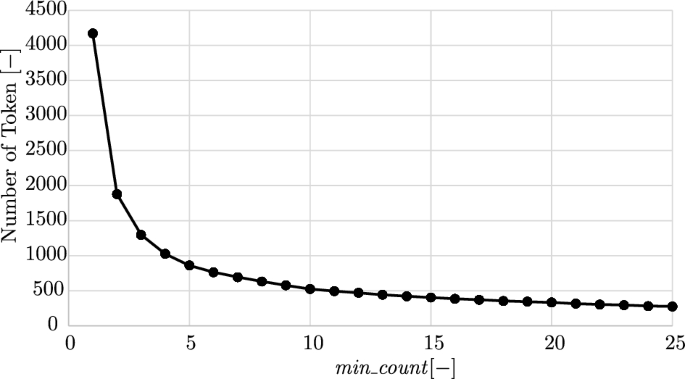
Number of token obtained from the text dataset of 28 scientific papers for different min_count parameters
The resulting sets of token are then used as concept names to search for fitting classes in the seven semantic artifacts proposed in Sect. 2.2 . This yields the number of token already contained in the respective ontology as classes as well as textual definitions of the classes in an automated way. In addition to this, the count of classes already contained can be used to suggest the ontology most fitting with regards to the respective text dataset.
Table 2 lists the resulting numbers of found classes in semantic artifacts of the performed annotation for six different min_count in the range [1...100]. Each token only needs to be annotated with a textual definition at least once; thus, the overall sum of annotated token is calculated for each set of token. Thus, if a token has annotations from multiple semantic artifacts, it is counted each respective row, while it only gets counted once in the row of sum of annotated token. Dividing the sum of annotated token by the overall amount of token then yields the rate of annotated token. A high rate of annotated token is desired in order to reduce later workload in revising the ontology, as coming up with definitions for classes is more difficult than agreeing on an already existing one. However, a high sum of annotated token also is desired as integrating more classes into an ontology results in a higher expressivity of the latter.
While sets obtained by setting a low min_count contain more token than those with higher min_count , the rate of annotated token rises with higher min_count parameters. This also might indicate a higher relevance of the token contained in the sets with high min_count parameters. In addition, the rate of annotated token for a min_count \(=1\) is quite low with \( 28.25~\%\) compared to the other rates. This might be due to the inclusion of typing mistakes and non-domain relevant token at lower min_count , as one occurrence would suffice for the token to be contained in the text dataset. On the other hand, lower min_count parameters take into account more concepts not yet defined in the ontologies. These concepts in turn allow for generation of more new candidates of classes in the respective ontologies. The ontologies themselves have lower amounts of token contained compared to the thesauri. However, the AFO is expected to be the ontology best fitting to the dataset as it has the highest number of annotated token while not having the highest amount of classes compared to the other ontologies. This indicates an intersection of topics represented in the text dataset and the AFO.
Plotting the rate of annotated token against the min_count parameters, as in Fig. 5 , the largest jump in the rate occurs between min_count \(=1\) and min_count \(=2\) .
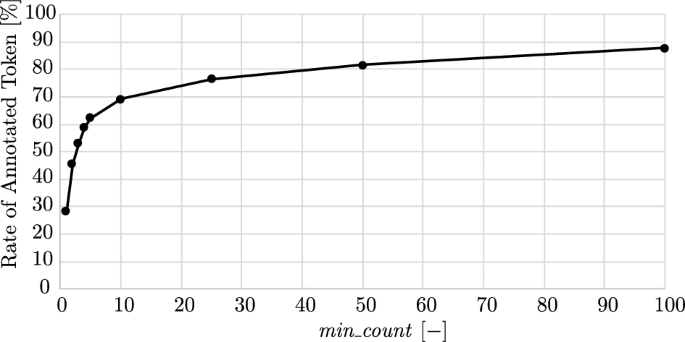
Rate of annotated token for different min_count
Taking into account the number of token found in each ontology, the AFO contains the most token for each min_count . Thus, the AFO is deemed as most fitting ontology of the five ontologies for the description of the knowledge domain contained in the text dataset and accordingly chosen as ontology to be extended by the method elucidated in Sect. 3.3 .
Word2Vec models are trained on token sets based on min_count parameters in the range min_count \(=[1...25]\) . Then, class labels from the AFO that are also contained in the token set are used as input to determine the most similar words. As the similarity of the words is determined by the cosine similarity, thresholds can be set to confine the amount of output words with regards to their similarity to the input word. A maximum amount of five output words per input word is set, and the threshold varied in the range of [0.8, ..., 0.999]. As some words are contained in multiple output sets for different input words, the amount of unique token generated by Word2Vec is calculated by only counting each word generated as a class candidate of the ontology once. With the AFO as ontology to be extended, Fig. 6 shows the amount of unique token found for different min_count parameters and different cosine similarity thresholds.
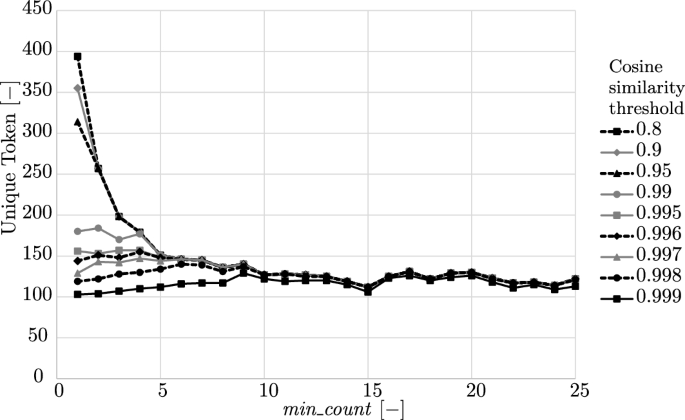
Amount of unique token output by Word2Vec for classes of AFO with different min_count and cosine similarity thresholds varied between [0.8, ..., 0.999]
While the cosine similarity threshold has an impact on the amount of unique token generated for low min_count , the effect seems to be mitigated for thresholds in the range [0.8, ..., 0.995] and min_count \(>5\) . Using different min_count and a cosine similarity threshold of 0.999, the AFO is extended automatically by new classes suggested by the Word2Vec model. The new classes are furthermore annotated by respective textual definitions obtained from the classes and concepts of the other semantic artifacts presented in Sect. 2.2 . Object properties conceptually related to are asserted, pointing to the respective ontology classes already contained in the AFO before extension.
Table 3 lists the resulting number of new classes inserted into the AFO obtained by setting the cosine similarity threshold to 0.999 and applying different min_count parameters in the range [1, ..., 25]. In addition, the amount of annotated new classes is listed along with the number of textual definitions according to the source of the textual definition related to the corresponding semantic artifact. Here, a min_count of 10 seems to be the most promising one, as the number of new classes (91) and number of annotated new classes (68) are highest. Thus, the AFO is extended by 91 classes which are created automatically based on the text dataset. From these new classes, 68 are annotated based on the other semantic artifacts achieving an annotation rate of \(68/91 = 74.73\%\) . Of these 68 annotated new classes, 6 are annotated based on BAO class-definitions, 7 based on CHEBI, 3 based on CHMO, and 9 based on SBO classes. Furthermore, 28 classes are annotated based on IUPAC-Goldbook concepts and 58 based on the NCIT. The sum of these annotations is greater than 68, indicating multiple annotations for some new classes in the extended AFO.
The automatically added classes are concepts taken from the text dataset; thus, they may be used to describe the context represented in the 28 scientific texts. Furthermore, the semantic artifacts chosen in this publication all deal somehow with the domain of chemistry or at least are situated in the domain of natural sciences that deal with chemical substances. Thus, the annotation of the classes is assumed to be in the correct domain as the source of the annotation already is situated close to the needed domain of knowledge. As the annotations often only vary in small details, the decision on (re-)use of specific ontology classes should be done by domain experts.
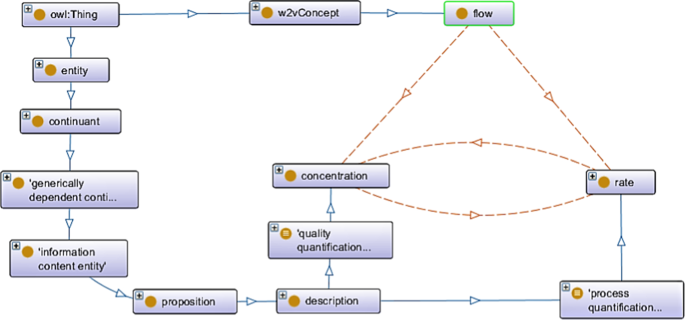
Visualization of class hierarchy of new class flow in Protégé. Class flow and relations conceptually related to to existing classes created automatically by the workflow with min_count = 10 and cosine similarity threshold = 0.999. Solid blue arrows indicate relation has subclass , dashed orange arrows denote relation conceptually related to
To provide an example of the resulting extension, Protégé is used for visualization of the resulting ontology. Figure 7 shows the class hierarchy of the already contained AFO classes concentration and rate using blue arrows for the hierarchical relation has subclass .
The new class flow is inserted based on the workflow as subclass of w2vConcept and gets assigned the relation of conceptually related to (denoted by dashed orange arrows) connecting it to the classes concentration and rate .
Furthermore, the new class flow gets annotated by the textual definition of the concept flow found in the NCIT. The resulting annotations of the class flow are depicted in Fig. 8 . The first entry contains the label of the class, while the next two entries point to the word-input that led to the generation of the class. The bottommost entry contains a textual definition found in the NCIT. The remark ‘Found in [NCIT]’ gives the link to the underlying class of the ontology, allowing for later reuse of the respective entity. As the new classes are generated automatically, an arbitrary amount of such rdfs:comment can be assigned to a class, but only one rdfs:label is assigned.
Thus, an existing ontology can be extended automatically by concepts based on scientific texts. After extension of the ontology, an evaluation by domain experts should be conducted, as not every resulting definition and relation might be correct.
This in turn can be used for an automated, ontology aligned annotation of research data: When a researcher uploads their research data and corresponding textual documentation to a database, the workflow presented in this work can then be used to automatically choose the best fitting ontology and extend it. The extended ontology could then in turn be used to annotate the previous uploaded research data, linking data entries with relations as posed in the textual documentation.
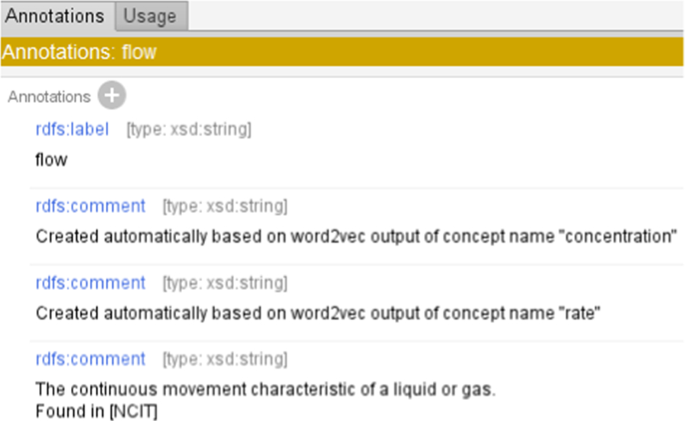
Annotations of new class flow visualized in Protégé for later review by domain experts
5 Summary and outlook
5.1 conclusion.
Ontologies are used to describe knowledge in an explicit and machine-readable way, while still being human-readable. Thus, they are used to model knowledge and semantic relations between data and concepts of scientific knowledge domains.
In this contribution, a method is set up to automatically make use of natural language processing (NLP) techniques to extract concepts contained in a text dataset in order to extend existing ontologies by these concepts relevant to a domain of knowledge. A search for textual concept definitions from different sources such as different ontologies and thesauri allows for automated annotation of these concepts found. This also helps in picking the right ontology to be extended in the second part of the workflow, where the extension of an ontology is performed by new classes based on the text dataset. Different word vectorization models using Word2Vec are trained based on different allowed numbers of repetitions of the token within the preprocessed text dataset ( min_count ) and used to suggest new classes and relations between them. Finally, the classes are annotated with textual definition based on other ontologies and thesauri, where possible.
This workflow allows for automated extension of ontologies by classes contained as concepts in a text dataset. A text dataset of 28 papers on the topic of catalytic methanation of CO \(_2\) reactions, five ontologies and two thesauri are used as a proof-of-concept. While use of a low min_count parameter results in higher numbers of new classes suggested, it also allows for integration of concepts not that important to the domain of knowledge, as the lower rates of annotated token suggest. Using a min_count parameter of 10, the Allotrope Foundation Ontology (AFO) is extended automatically by 91 new classes obtained by the text dataset. Of these classes, 68 classes are provided automatically with at least one textual definition based on the other semantic artifacts (i.e., the other ontologies and thesauri) provided.
This workflow can easily be adapted for other ontologies and text datasets to extend existing ontologies. Additionally, the database of semantic artifacts can be set for a larger number of ontologies and thesauri. While this can be adjusted quickly, the use of other definition databases such as WikiData can be implemented with some code adjustments.
5.2 Limitations and future work
The workflow only uses single-word tokens, thus only is able to search for and add single-word classes to the ontology. Detecting multi-word concepts with the presented workflow is not yet possible, but desirable as often ontology classes consist of more than one word. In the future, manipulation of the applied POS-tagging is planned to mitigate the limitation of only single-word classes being considered by the presented workflow. Here, e.g., neighboring noun token could be combined to one class, such as “flow rate”, or pairs of neighboring adjective and noun pairs, like “catalytic reaction.” Furthermore, the use of more sophisticated methods, such as named entity recognition (NER) [ 28 ], can be used. However, this method requires the pre-definition of categories. While this is already quite available for general categories, the definition of catalysis-related categories for NER is yet to be implemented to the best knowledge of the authors.
The second major limitation of the presented workflow is the missing refinement of the “semantically related to” relation used to link existing and newly created classes. The relationships could not be further refined because the semantic relation of the concepts is not appropriately given by word2vec. For example, no distinction is made between a hierarchical relationship or an object property. This is also due to the fact that only nouns are included as classes into the ontology; thus, verbs and adjectives are not considered, which would be the more fitting candidates for ontology properties and relations. In future work, relationship extraction and entity linking, i.e., the Radbound Entity Linker [ 29 ] could be used to develop more sophisticated relationship extraction. After extracting the relations, additional linking to already existing ontology relationships is also in the scope.
To evaluate the usefulness of the workflow, an evaluation by domain experts should be conducted, to classify the number of valuable classes and relations generated automatically by the workflow. Extending an ontology by textual input as shown in this work also will help domain experts in the future to automatically annotate research data when uploading a set of research data together with a corresponding paper to a research database.
6 Supplementary information
The code developed in this work is available in a GitHub repository here: https://github.com/TUDoAD/NLP-Based-Ontology-Extender .
The pre-processed pdf-files and the ontology files are available in a zenodo repository here: https://zenodo.org/record/7956870 .
Wulf C, Beller M, Boenisch T, Deutschmann O, Hanf S, Kockmann N, Kraehnert R, Oezaslan M, Palkovits S, Schimmler S, Schunk SA, Wagemann K, Linke D (2021) A unified research data infrastructure for catalysis research-challenges and concepts. ChemCatChem 13(14):3223–3236. https://doi.org/10.1002/cctc.202001974
Article Google Scholar
Wilkinson MD, Dumontier M, Aalbersberg IJ, Appleton G, Axton M, Baak A, Blomberg N, Boiten J-W, da Silva Santos LB, Bourne PE, Bouwman J, Brookes AJ, Clark T, Crosas M, Dillo I, Dumon O, Edmunds S, Evelo CT, Finkers R, Gonzalez-Beltran A, Gray AJG, Groth P, Goble C, Grethe JS, Heringa J, ’t Hoen PAC, Hooft R, Kuhn T, Kok R, Kok J, Lusher SJ, Martone ME, Mons A, Packer AL, Persson B, Rocca-Serra P, Roos M, van Schaik R, Sansone S-A, Schultes E, Sengstag T, Slater T, Strawn G, Swertz MA, Thompson M, van der Lei J, van Mulligen E, Velterop J, Waagmeester A, Wittenburg P, Wolstencroft K, Zhao J, Mons B (2016) The fair guiding principles for scientific data management and stewardship. Sci Data 3(1):160018. https://doi.org/10.1038/sdata.2016.18
Strömert P, Hunold J, Castro A, Neumann S, Koepler O (2022) Ontologies4chem: the landscape of ontologies in chemistry. Pure Appl Chem 94(6):605–622. https://doi.org/10.1515/pac-2021-2007
Gruber TR (1993) A translation approach to portable ontology specifications. Knowl Acquis 5(2):199–220. https://doi.org/10.1006/knac.1993.1008
National Cancer Institue: National Cancer Institue Thesaurus. https://ncit.nci.nih.gov (2022)
Grühn J, Behr AS, Eroglu TH, Trögel V, Rosenthal K, Kockmann N (2022) From coiled flow inverter to stirred tank reactor—bioprocess development and ontology design. Chem Ing Tec 94(6):852–863. https://doi.org/10.1002/cite.202100177
Menke MJ, Behr AS, Rosenthal K, Linke D, Kockmann N, Bornscheuer UT, Dörr M (2022) Development of an ontology for biocatalysis. Chemie Ingenieur Technik 94(11):1827–1835. https://doi.org/10.1002/cite.202200066
Asim MN, Wasim M, Khan MUG, Mahmood W, Abbasi HM (2018) A survey of ontology learning techniques and applications. Database. https://doi.org/10.1093/database/bay101
Dal A, Maria J (2012) Simple method for ontology automatic extraction from documents. Int J Adv Comput Sci Appl. https://doi.org/10.14569/ijacsa.2012.031206
Opasjumruskit K, Peters D, Schindler S (2020) DSAT: Ontology-based information extraction on technical data sheets. ISWC 2020, 2–6, Nov. 2020. https://ceur-ws.org/Vol-2721/paper563.pdf
Opasjumruskit K, Böning S, Schindler S, Peters D (2022) OntoHuman: ontology-based information extraction tools with human-in-the-loop interaction. In: International conference on cooperative design, visualization and engineering. Springer, Berlin, pp 68–74
Opasjumruskit K (2020) NLP for ontology development-a use case in spacecraft design domain. https://elib.dlr.de/136233/
Horsch M, Petrenko T, Kushnarenko V, Schembera B, Wentzel B, Behr A, Kockmann N, Schimmler S, Bönisch T (2022) Interoperability and architecture requirements analysis and metadata standardization for a research data infrastructure in catalysis. In: Pozanenko A, Stupnikov S, Thalheim B, Mendez E, Kiselyova N (eds) Data analytics and management in data intensive domains. Springer, Cham, pp 166–177. https://doi.org/10.1007/978-3-031-12285-9_10
Chapter Google Scholar
NFDI4Cat: Ontology collection of NFDI4Cat. https://nfdi4cat.org/en/services/ontology-collection (2022)
Allotrope Foundation: Allotrope Foundation Ontology. https://www.allotrope.org/ontologies (2022)
Hastings J, Owen G, Dekker A, Ennis M, Kale N, Muthukrishnan V, Turner S, Swainston N, Mendes P, Steinbeck C (2015) ChEBI in 2016: improved services and an expanding collection of metabolites. Nucleic Acids Res 44(D1):1214–9
Batchelor C (2022) Chemical methods ontology. http://purl.obolibrary.org/obo/chmo.owl
Abeyruwan S, Vempati UD, Küçük-McGinty H, Visser U, Koleti A, Mir A, Sakurai K, Chung C, Bittker JA, Clemons PA, Brudz S, Siripala A, Morales AJ, Romacker M, Twomey D, Bureeva S, Lemmon V, Schürer SC (2014) Evolving BioAssay ontology (BAO): modularization, integration and applications. J Biomed Semant. https://doi.org/10.1186/2041-1480-5-s1-s5
Nguen T, Karr J, Sheriff R (2022) Systems biology ontology. http://biomodels.net/SBO/
Gold V (ed.) (2019) The IUPAC compendium of chemical terminology. International Union of Pure and Applied Chemistry (IUPAC). https://doi.org/10.1351/goldbook
Musen MA (2015) The protégé project: a look back and a look forward. AI Matters 1(4):4–12. https://doi.org/10.1145/2757001.2757003
Řehůřek R, Sojka P (2010) Software framework for topic modelling with large corpora. pp 45–50. https://doi.org/10.13140/2.1.2393.1847
Pennington J, Socher R, Manning C.D (2014) Glove: global vectors for word representation. In: Empirical methods in natural language processing (EMNLP), pp 1532–1543. http://www.aclweb.org/anthology/D14-1162
Adobe Inc (2022) Adobe Acrobat Pro PDF-reader, version 22.003.20258. https://www.adobe.com/acrobat.html
Shinyama Y (2007) PDFMiner—Python PDF Parser. https://github.com/euske/pdfminer
Honnibal M, Montani I, Van Landeghem S, Boyd A (2020) spaCy: industrial-strength natural language processing in Python. https://doi.org/10.5281/zenodo.1212303
Lamy J-B (2017) Owlready: ontology-oriented programming in python with automatic classification and high level constructs for biomedical ontologies. Artif Intell Med 80:11–28. https://doi.org/10.1016/j.artmed.2017.07.002
Nadeau D, Sekine S (2007) Named entities: recognition, classification and use. Lingvist Investig 30(1):3–26. https://doi.org/10.1075/li.30.1.03nad
van Hulst JM, Hasibi F, Dercksen K, Balog K, de Vries AP (2020) Rel: an entity linker standing on the shoulders of giants. In: Proceedings of the 43rd international ACM SIGIR conference on research and development in information retrieval. SIGIR’20. ACM
Download references
Acknowledgements
The authors thank the Deutsche Forschungsgemeinschaft (DFG) within the Nationale Forschungsdateninfrastruktur (NFDI) initiative (Grant No.: NFDI/2-1-2021) for funding part of this work as well as the fruitful discussions with researchers in NFDI4Cat. A.S.B. thanks the networking program “Sustainable Chemical Synthesis 2.0” (SusChemSys 2.0) for the support and fruitful discussions across disciplines.
Open Access funding enabled and organized by Projekt DEAL. The Deutsche Forschungsgemeinschaft (Grant No.: NFDI/2-1-2021) funded part of this work.
Author information
Authors and affiliations.
Laboratory of Equipment Design, Faculty of Biochemical and Chemical Engineering, TU-Dortmund University, Emil-Figge-Straße 68, 44139, Dortmund, North-Rhine-Westphalia, Germany
Alexander S. Behr, Marc Völkenrath & Norbert Kockmann
You can also search for this author in PubMed Google Scholar
Corresponding author
Correspondence to Alexander S. Behr .
Ethics declarations
Conflict of interest.
The authors declare no competing interests as defined by Springer, or other interests that might be perceived to influence the results and/or discussion reported in this paper.
Additional information
Publisher's note.
Springer Nature remains neutral with regard to jurisdictional claims in published maps and institutional affiliations.
Appendix A: References of text dataset
See Table 4 .
Rights and permissions
Open Access This article is licensed under a Creative Commons Attribution 4.0 International License, which permits use, sharing, adaptation, distribution and reproduction in any medium or format, as long as you give appropriate credit to the original author(s) and the source, provide a link to the Creative Commons licence, and indicate if changes were made. The images or other third party material in this article are included in the article’s Creative Commons licence, unless indicated otherwise in a credit line to the material. If material is not included in the article’s Creative Commons licence and your intended use is not permitted by statutory regulation or exceeds the permitted use, you will need to obtain permission directly from the copyright holder. To view a copy of this licence, visit http://creativecommons.org/licenses/by/4.0/ .
Reprints and permissions
About this article
Behr, A.S., Völkenrath, M. & Kockmann, N. Ontology extension with NLP-based concept extraction for domain experts in catalytic sciences. Knowl Inf Syst 65 , 5503–5522 (2023). https://doi.org/10.1007/s10115-023-01919-1
Download citation
Received : 09 January 2023
Revised : 23 May 2023
Accepted : 21 June 2023
Published : 15 July 2023
Issue Date : December 2023
DOI : https://doi.org/10.1007/s10115-023-01919-1
Share this article
Anyone you share the following link with will be able to read this content:
Sorry, a shareable link is not currently available for this article.
Provided by the Springer Nature SharedIt content-sharing initiative
- Natural language processing
- Automated ontology annotation
- Information extraction
- CO \(_2\) methanation
- Catalytic conversion
- Find a journal
- Publish with us
- Track your research
- Open access
- Published: 13 March 2023
Content and quality of physical activity ontologies: a systematic review
- Maya Braun ORCID: orcid.org/0000-0002-1240-4550 1 ,
- Stéphanie Carlier 2 ,
- Femke De Backere 2 ,
- Annick De Paepe 1 ,
- Marie Van De Velde 1 ,
- Delfien Van Dyck 3 ,
- Marta M. Marques 4 ,
- Filip De Turck 2 &
- Geert Crombez 1
International Journal of Behavioral Nutrition and Physical Activity volume 20 , Article number: 28 ( 2023 ) Cite this article
2402 Accesses
6 Altmetric
Metrics details
Introduction
Ontologies are a formal way to represent knowledge in a particular field and have the potential to transform the field of health promotion and digital interventions. However, few researchers in physical activity (PA) are familiar with ontologies, and the field can be difficult to navigate.
This systematic review aims to (1) identify ontologies in the field of PA, (2) assess their content and (3) assess their quality.
Databases were searched for ontologies on PA. Ontologies were included if they described PA or sedentary behavior, and were available in English language . We coded whether ontologies covered the user profile, activity, or context domain. For the assessment of quality, we used 12 criteria informed by the Open Biological and Biomedical Ontology (OBO) Foundry principles of good ontology practice.
Twenty-eight ontologies met the inclusion criteria. All ontologies covered PA, and 19 included information on the user profile. Context was covered by 17 ontologies (physical context, n = 12; temporal context, n = 14; social context: n = 5). Ontologies met an average of 4.3 out of 12 quality criteria. No ontology met all quality criteria.
This review did not identify a single comprehensive ontology of PA that allowed reuse. Nonetheless, several ontologies may serve as a good starting point for the promotion of PA. We provide several recommendations about the identification, evaluation, and adaptation of ontologies for their further development and use.
The idea of ontologies can be traced back to early philosophers studying how to describe and categorize ‘what is’ in the world. Ontologies refer to the various ways to structure and classify our knowledge about the world, including typologies or taxonomies such as the periodic table of Mendeleev, the Linnaean classification system of plants, the compendium of physical activities [ 1 ], or the taxonomy of behaviour change technique [ 2 ]. Within computer and information sciences, the term ‘ontologies’ has become reserved for formal classification systems that are computer-readable, provide clear definitions of concepts (classes) and their properties, and allow formal modelling of both simple and complex relationships between concepts [ 3 , 4 ] .
Advantages of such ontologies are that they are unambiguous, computer-readable, and can easily be reused and updated [ 5 ]. They can thus be easily adapted to different contexts, such as different cultural contexts, different target groups, or different target behaviours [ 5 ]. Ontologies have revolutionized collaboration and research in the biological sciences. A prominent example is the Gene Ontology [ 4 ], which has been used to automatically annotate publications and to aggregate data. It allowed the field to progress faster, and reach new insights based on cumulative knowledge, which would not have been possible by manually reviewing literature. Ontologies may have similar advantages in the behavioural sciences. For that reason, a consensus report of the National Academy of Sciences has called for a strong and collaborative investment in ontologies [ 6 ]. As yet, there are not many ontologies in the behavioural sciences [ 7 , 8 ]. A notable exception is the “Human Behaviour Change Project” (HBCP) Footnote 1 [ 9 ], which aims to develop an ontology of behaviour change interventions (see Fig. 1 ).
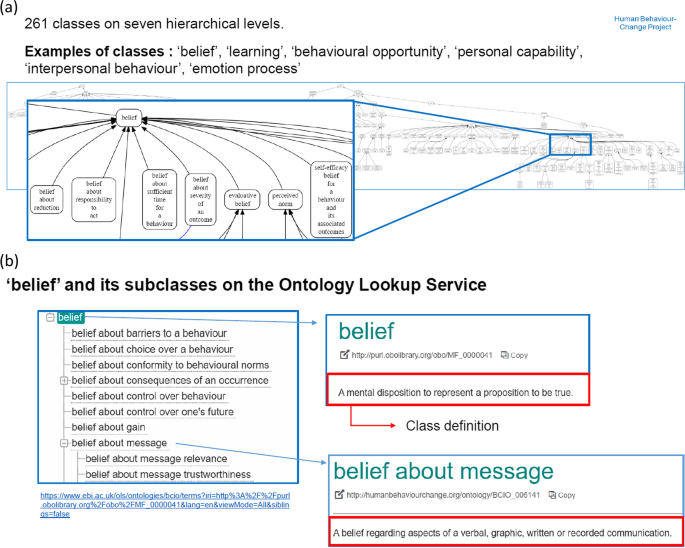
depicts part of the Behaviour Change Intervention Ontology, a showing the relationships between different classes and b showing the definitions of the two specific classes ‘belief’ and ‘belief about message’
Ontologies have the potential to accelerate health promotion research by (1) providing a controlled, unambiguous vocabulary, (2) automatizing annotation and aggregation of knowledge and (3) formalizing theories and findings.
First, ontologies can provide a controlled vocabulary for health promotion research. Without a controlled vocabulary, researchers may unintentionally assume that use of the same term reflects sameness or that use of different terms reflects differentness. Such jingle-jangle fallacies are abound in the health sciences. For example, ‘Stress’ can refer to physical pressure or tension, or a state of mental or emotional strain or tension. Although different terms, ‘Problem Solving’ and ‘Coping Planning’ refer both to identifying barriers and generating and selecting strategies to overcome them. Ontologies may counter such fallacies, and hence improve communication amongst researchers and practitioners, data integration and analyses, and the dissemination of knowledge. Importantly, a controlled vocabulary does not mean that a term cannot have multiple meanings. Instead, it allows us to specify which of the meanings we are referring to at any given moment. The National Academy of Science explicitly supports ‘ontological pluralism’, acknowledging that multiple, even competing, ways of understanding and representing reality exist [ 6 ]. In doing so, ontologies foster transparency about the used concepts, definitions and the decisions made by the developers. Therefore, documenting the background, scope and aim of ontologies, including cultural context, year of creation and updates, is an important task.
Second, ontologies can support the retrieval and the automatic aggregation of knowledge. An example of this is the HBCP, described above, which aims to automatically annotate publications concerning behavior change interventions using natural language processing [ 9 ]. The cumulative knowledge of the field being more easily and more clearly available, ontologies can then help inform, design and evaluate interventions [ 6 ].
Third, it is possible to formalize theories and findings by linking them to ontological classes and using ontological relationships to connect these. In a related effort, seventy-six behaviour change theories have been formally visualized and entered into a searchable database [ 10 ], allowing for systematic searches and easy comparison between theories.
Ontologies may also have specific advantages for physical activity (PA) research and interventions. An ontology on PA might have information on the activity itself, the person carrying out the activity, and the context of the activity. It can also link to other ontologies, for example of behaviour change interventions [ 11 ] or anatomy [ 12 ] . They can then be used to support the automatic detection and recognition of activities and their context, and improve the interoperability of sensor data (e.g. [ 13 ].) . This can be valuable for improving further innovations in the field (e.g. event-based ecological momentary assessment, and Just-in-Time-Adaptive-Interventions). Furthermore, ontologies may help in designing context-aware and personalized interventions. Context-aware and personalized interventions to promote PA are at their early stages, but have already been found to be more effective for both behavior [ 14 , 15 ] and health outcomes [ 16 ] than their non-personalized counterparts. Ideally, the interaction between or combination of various factors should be considered, including sociodemographic and cultural factors, factors relating to physical and mental well-being and contextual factors. To achieve this, we need information on “who is active, what do they do, and under which circumstances?”. Unsurprisingly, the required knowledge is vast and complex. Ontologies offer a way to organize and structure such a complex network of information. and ontological reasoning may serve as a base for personalized recommendations.
While ontologies are a promising avenue to accelerate research in PA promotion, the field can be difficult to navigate. Repositories such as BioPortal [ 17 ] and the Open Biological and Biomedial Ontology (OBO) Foundry [ 18 ] have been established to easily find ontologies. OBO Foundry hosts ontologies that adhere to predefined criteria of best practice [ 18 , 19 ]. High-quality ontologies are needed as they are meant to be adapted and maintained. If an ontology is of poor quality, researchers attempting to reuse the ontology will face difficulties. Outside of the OBO Foundry, it is difficult to identify ontologies that are both relevant and of high quality.
Current review
In the current paper, we reviewed ontologies that are relevant to PA. The review has the following objectives: 1) to identify ontologies in the literature, 2) to assess their content and 3) to assess their quality, especially with regard to reusability.
The PRISMA reporting criteria for systematic reviews [ 20 ] were used, and the protocol is available via open science framework. Footnote 2
Identification of ontologies
The following electronic databases were searched in June 2021: CINAHL (via EBSCOhost), ProQuest Psychology, Web of Science, Scopus, PubMed, EMBASE, IEEE (Institute of Electrical and Electronics Engineers) Xplore, and ACM (Association for Computing Machinery).
The search strategy included strings related to ‘physical activity’ and ‘ontologies’. We excluded publications that contained the strings “gene” “dna” or “rna”. The final search strategy for Web of Science can be found in the appendix.
Results of the search were exported to EndNote and duplicates were removed. They were then imported into rayyan [ 21 ]. Screening was first based on the title and abstract only, and then on full texts. A second researcher screened 25% of the records based on the title and abstract only . The used ontologies were identified and indexed.
Two further methods were used:
1. Reference Searching: References from relevant records were screened for missing ontologies.
2. Key ontology repositories (i.e. OBO Foundry and BioPortal) were searched for terms related to ‘physical activity’.
Inclusion criteria
Publications were included if they met the following criteria:
- Original work that describes an ontology, using definitions of concepts and presenting relationships between concepts.
- The ontology describes PA or sedentary behavior, or has been used in a behaviour change intervention targeting PA or sedentary behavior.
- The ontology is available in English. The choice was made because of the ease of integrating and reusing these ontologies.
Coding and quality assessment
Coding of ontologies occurred based on publicly available information. We coded formal characteristics , such as the ontology provider, country of origin, year of publication, version accessed and corresponding e-mail. Also, the number of classes and properties was coded . Concerning the content of the ontology, we differentiated between the physical activity domain, the profile domain, and three the context domain. The latter domain contained three subtypes (temporal, social and physical context). A description of these domains can be found in Table 1 .
The coding of the quality of the ontologies was largely based upon the OBO Foundry principles of good ontology practice [ 22 ]. We included 12 of the 14 criteria. Principles were slightly adapted to be applicable to all ontologies. The principles “Relations” (Relations should be used from the Relations Ontology) and “Commitment to Collaboration” (Foundry ontologies are expected to collaborate with other Foundry ontologies) were considered not relevant for this review. After an initial quality assessment, the authors of the ontologies were contacted and provided with the opportunity to share additional materials or documentation.
Open: The ontology should be openly available on the internet. Being available upon request was not sufficient.
Common Formal Language: The ontology should be available in an owl file using the RDF-XML syntax.
Unique URI: This criterion was met if each class and property had a unique uniform resource identifier (URI), which is a unique characters sequence that distinguishes one resource from another.
Versioning: Versions should be labelled clearly, including their date of publication and the changes made.
Textual Definitions: An ontology should have definitions for the majority of its classes, in particular for top level terms.
Naming Conventions: An ontology should have clear naming conventions. This criterion was met if names were unique and intelligible to the coding team MB and SC.
Documentation: Significant documentation should be available, e.g. in a published paper describing the ontology, websites, or in manuals for developers and users.
Locus of Authority: We coded whether contact details (at least a name and email address) of a person were provided. A corresponding author of a publication was sufficient, but the email address needed to be valid (i.e. not return an error notification).
Reuse: We coded whether ontology developers reused ontological or non-ontological resources during development. This criterion was met if there was clear documentation that content was imported from other ontological or non-ontological resources.
Documented Plurality of Users: We coded whether the usage of the ontology by multiple independent people or organizations was documented in a freely available online document. This information had to be provided by the ontology developers, not those using the ontology.
Maintenance: Ontology providers should have a plan for maintaining the ontology. and provide this information in the documentation. We also coded whether maintenance did take place, e.g. regularly update.
Responsiveness: We coded whether ontology developers offered channels for community participation and were responsive to requests. This criterion was met if developers had set up a way to track community requests and suggestions (e.g., issue tracker).
After the initial coding of the quality assessment, the authors of the ontologies were contacted with the coding results of their ontology, and provided the opportunity to share additional materials or documentation. After reviewing these responses, the results were finalized.
Figure 2 displays the number of publications identified, screened and excluded at each stage of the review process, as well as the number of ontologies identified through each method and in total. Footnote 3
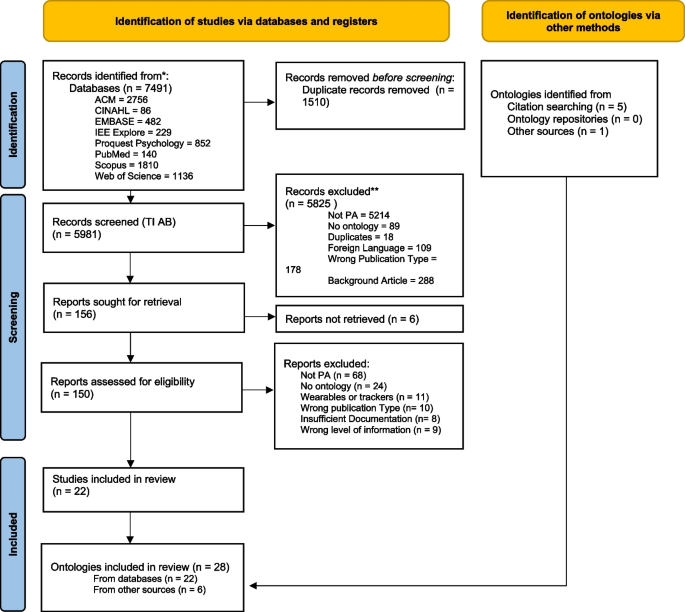
PRISMA Chart
Ontologies related to physical activity
We identified and assessed the quality of 28 ontologies. A brief summary of each ontology can be found in Table 2 . A brief description of each ontology can be found in Additional File 2 .
Content domains covered in the ontologies
Physical activity domain.
All ontologies distinguished between types of activities; 18 between type of physical activities (e.g. cycling, running, swimming), 10 between types of exercises (e.g. bicep curls, squats) and 7 between all types of activities, whether physical or not (e.g. eating, sleeping). Also well integrated was the intensity of the activity ( n = 14). Less covered features were the effects or function of an exercise (e.g. increased heart rate, stretch muscle, flexibility improvement, n = 5), the type of exercise (e.g. stretching, strengthening, n = 6), equipment needed ( n = 5), associated parts of the musculoskeletal system ( n = 6), function of activities (e.g. occupational activity, transport, n = 5), associations with specific workouts ( n = 3), phases or sessions ( n = 1), kinds of movements performed in the exercise (e.g. flexing, n = 3), contraindications ( n = 2), required user experience ( n = 1), and linked animations/visualisations ( n = 1).
Profile domain
Nineteen ontologies contained profile information. Basic sociodemographic information ( n = 18) was most often included, such as age ( n = 15), sex or gender ( n = 14), administrative identifiers, such as patient or client IDs or national register numbers ( n = 9), occupation ( n = 7), education ( n = 3), cultural or religious background ( n = 3), ethnicity (n = 2), address ( n = 2), household members ( n = 1), marital status ( n = 1), socioeconomic status ( n = 1).
Ontologies also often contained some form of clinical or health information ( n = 21). More than half of the identified ontologies included current diagnoses ( n = 15). Others included health-related risk factors for specific diseases ( n = 7). Many included health characteristics ( n = 16), including body mass ( n = 13), blood pressure or blood glucose levels ( n = 10), height ( n = 9), body fat ( n = 3), and fitness level ( n = 3) of the user. Some ontologies considered current treatments ( n = 4) and recommendations by health care providers ( n = 3).
Psychosocial features were not often presented in the ontologies ( n = 5). Some of those were general, such as emotional and psychological state ( n = 2) or feelings of insecurity ( n = 1). Others included specific information related to physical activities, such as determinants for PA (e.g. motivation, intention, self-efficacy, n = 2), fear of falling ( n = 1) or fear of fatigue ( n = 1). Six ontologies included preferences for specific activities or intensities, five included goals concerning PA or health outcomes (e.g. weight loss), and six included current lifestyle and habits.
Context domains
The physical context was integrated in 12 out of 28 ontologies. This was usually done by specifying the location, e.g. a building or place from a list ( n = 8), specifying whether it was indoors or outdoors ( n = 8) or using GPS location ( n = 2). Weather was also often included, including weather in general ( n = 4), lighting ( n = 3), and temperature ( n = 4). The surface features (e.g. water, ice, snow) and type of soil needed were each covered in one ontology. Finally, one ontology included the usability, accessibility and safety of a given location (47).
The temporal context was covered by 14 out of 26 ontologies. Most of the ontologies covered basic temporal aspects such as duration of an activity ( n = 8), start ( n = 5) and end time ( n = 2) or the frequency or regularity of an activity ( n = 4). Four ontologies defined the day that the activity took place on. Events, seasons, or times of the day were each covered by one ontology.
Five ontologies covered the domain of social context. Family, social purpose of an activity and social interactions were included in two ontologies. Social support, social networks, communities, cohabitants, groups and social events were each covered by one ontology.
Quality assessment of ontologies
The assessed quality of the ontologies are summarized in Table 3 , including the total number of criteria met per ontology, and the total number of ontologies meeting each criterion.
There was strong variability in the extent to which quality criteria were met. Notably, only eight out of 28 ontologies were freely available online. The lack of information strongly affected the assessment of the remaining criteria. This influenced specifically the rating on the criteria for common format, URI, versioning and clear definitions. Ontologies met an average of 4.23 (SD = 2.47) and median of 3 (Q1 = 3, Q3 = 6) out of 12 criteria, with a minimum of 0 and a maximum of 9 out of 12 criteria. The criteria met by most ontologies are documentation ( n = 26), clear naming ( n = 23) and locus of authority ( n = 22). The least met criteria were responsiveness ( n = 0), maintenance ( n = 2) and providing clear definitions ( n = 3). Ontologies meeting most criteria are the Physical ACtivity Ontology” (PACO), “Semantic Mining of Activity, Social, and Health data” (SMASH) ontology [ 39 ] and the “Taxonomy for Rehabilitation of Knee conditions” (TRAK) [ 45 , 46 , 47 ] ontology, meeting nine criteria each. The lowest number of criteria was met by the Sloth ontology [ 31 ] which meets none of the quality criteria.
Ontologies have the potential to increase the efficiency of research in the field of PA. However, few PA researchers are familiar with ontologies, and the field can be difficult to navigate. In the current paper, we identified relevant ontologies in the field of PA, assessed their content, and rated their quality. We identified 28 ontologies. There was a substantial variability in scope and content of the identified ontologies, ranging from knowledge systems that formally represent knowledge about a specific disease, and can reason using the knowledge residing in that ontology [ 54 ] to ontologies specifically created to describe physical activities [ 8 , 49 ] or detect behavior in a particular context [ 13 ]. There were also differences in the content covered by the ontologies. All ontologies included the activity domain, albeit in varying detail, and most covered some user profile information. Context information was covered by fewer ontologies and in less detail. No single ontology comprehensively captured PA in the context where it occurs, including physical, temporal and social aspects. Such variability was expected, as most ontologies were created for specific use cases that did not require all information. Because ontologies can be integrated and connected, it is not necessary for each ontology to contain all information relevant for PA. However, it should be avoided that identical or very similar concepts are defined independently from each other and without referencing to each other. By importing concepts from established existing ontologies, ontology developers can improve the interoperability and clarity of the ontology. For example, when different ontologies are developed for each context domain, they can easily be integrated if they all refer to the same definition of PA.
We have found that while many ontologies meet the criteria that enable them to function in its original system, such as providing the ontology in a common format and using unique identifiers, many criteria relevant for reuse, are not met. Neither do most researchers seem to maintain their ontologies. Given that the goal of ontologies is to provide unambiguous concepts, ensure reusability and reduce redundant research [ 55 ], it is surprising that many ontologies did not meet these criteria.
Remarkably, 20 out of the 28 ontologies were not published in repositories such as OBO Foundry or BioPortal. These ontologies were also not freely available elsewhere, such as on GitHub or project-specific websites. This limited our ability to adequately assess the quality criteria. Most likely these ontologies were not designed to be shared with other users. In line with this view, some authors noted in their documentation or email communication with us that their ontologies were created as a proof of concept, or to demonstrate the interaction with a specific system. They were not designed to provide a comprehensive ontology of a particular phenomenon. We, hence, strongly recommend to consider ontologies available via an ontology repository over those described in a paper but not made available.
Description of the three highest scoring ontologies
Notwithstanding that none of the ontologies met all our criteria, there were some good scoring ontologies that may serve as a good starting point for further development in the field of PA. The three best scoring ontologies are the PACO [ 25 ], SMASH ontology [ 39 ], and the TRAK ontology [ 45 ].
The “Physical ACtivity Ontology” (PACO) [ 25 ] was created to structure and standardize descriptions of PA. It extracted concepts from existing PA questionnaires and scales using natural language processing. It contains an extensive list of physical activities, including daily living activities that require the actor to be physically active. It also contains the effect of exercise, equipment, and program, and provides information about the amount, frequency, regularity, intensity, required condition (snow, ice, water, ground) and location (inside or outside) of an activity. The authors demonstrated a use case where PACO successfully standardized and classified PA descriptions.
The “Semantic Mining of Activity, Social, and Health data” (SMASH) ontology [ 39 ] was created for human behavior prediction. The ontology includes social and physical activities. SMASH is an ontology for health social networks, containing three modules, namely biomarkers, social activities and physical activities. Specifically, it contains lists of exercises, physical activities and daily living activities, sociodemographic information about the individual, social activities such as social events, interactions and relationships, and social entities such as people or communities. SMASH improves the prediction and explanation of behavior and interventions in the context of sustained weight loss.
The “Taxonomy for Rehabilitation of Knee conditions” (TRAK) ontology [ 45 ] aims to provide a framework that can be used to improve efficiency in research by collecting coded data. TRAK was developed following the OBO Foundry design principles and was informed by experts. It contains a list of events relevant for PA, such as accidents or forceful joint movements, an extensive list of exercises and sports, lists of joint movements and muscle contractions as well as anatomical entities. TRAK also contains information such as the roles of healthcare providers, and healthcare activities. It has been developed further into the KneeTex ontology [ 56 ], but these changes were out of scope for this review. TRAK has been integrated into a web-based intervention that provides patients with health information, personalized exercise plans and remote clinical support [ 46 ].
The three ontologies vary in scope and context of development. It should be noted that even within the three highest scoring ontologies, only one formulated a plan for maintenance [ 25 ], contained definitions for the majority of its classes [ 45 ], and documented the different independent users [ 39 ] respectively. None met the criterion of responsiveness. Likely, not all criteria are equally important, and their importance may depend on the goal of the research. For example, some criteria, namely using a common format and unique URIs, are necessary in order for the ontology to function in its original context. However, other criteria are critical if ontologies are meant for reuse, such as the availability of the ontology, and classes with clear naming conventions. Criteria relevant for transparency are more related to having clear documentation of the development and evaluation of the ontology available, providing clear version control, providing clear definitions for classes and having a locus of authority that researchers can reach out to if they have questions. Lastly, having and following a clear maintenance plan and being responsive to user requests are criteria that relate to improving the ontology and keeping it up to date with current scientific standards. While meeting these criteria has the potential to vastly improve an ontology, it is also associated with significant efforts.
Implications for further research
Ontologies have strong potential for PA promotion. Some benefits are generic. Ontologies provide clear definitions of concepts, allow simple and complex relationships between concepts, facilitate the aggregation of knowledge and represent the knowledge in a computer-readable format for future (re)use and adaptation. This has been done successfully in other disciplines, such as the field of genetics [ 3 , 4 ], allowing for progress by facilitating evidence synthesis and aggregating existing knowledge. Within health behavior change, the HBCP [ 9 ] is the first to make use of ontologies. The goal of HBCP is to provide answers regarding which interventions work for whom under which circumstances. Within that project, knowledge is structured into behaviour, mechanisms, intervention, exposure and context domains [ 9 ]. Multiple ontologies have already been developed in this project [ 11 , 57 , 58 , 59 ].
Use of ontologies in the field of PA may be relevant for the following reasons: ontologies can support (1) automatic recognition of PA and its context and (2) intervention development and clinical practice. First, automatically detecting and recognizing PA can be valuable for both research and applied settings [ 60 ], and is a promising avenue [ 61 ]. By having data via devices on the amount and intensity, as well as the type of PA, researchers and clinicians do not have to rely on self-report, which can be skewed due to different factors such as memory bias. It can therefore give a less obtrusive and more complete picture of the PA of an individual. Ontologies have the potential to improve automatic activity recognition [ 13 ]. Second, ontologies can support (digital) PA promotion. Because ontologies are computer-readable, they can easily be integrated into systems that provide decision support, and have been already developed in a research context (e.g. [ 33 , 40 ]). Ontologies can help to provide recommendations for specific exercise plans (e.g. [ 41 ]) for particular patient groups, or may help to plan PA more generally.
In this review, we found no ontology or set of ontologies that covered all important aspects of PA. There is a clear need for a set of ontologies that fully captures PA in its context, including the physical, temporal and social domain. Such an ontology can integrate information that is covered by the higher quality ontologies identified in this review, and build upon those following the quality principles described above.
This review can serve as a first introduction to ontologies for PA researchers, and specifically those focusing on PA promotion. We have provided an overview of existing ontologies on PA, their content and their quality. To facilitate systematic identification of useful ontologies in the future, we recommend researchers to start using an ontology repository. We recommend starting with OBO Foundry [ 22 ] and then searching BioPortal [ 17 ], as OBO Foundry guarantees for the quality of their ontologies [ 19 ]. Since the current review has been conducted, another foundry has launched, specifically targeting behavoural and social sciences, namely the Behaviour and Social Sciences Ontology (BSSO) Foundry. Footnote 4 While it does not yet contain many ontologies, it should also be considered when searching for suitable ontologies for re-use. In a second step, researchers should determine whether the content of the ontology meets their needs by investigating its respective classes. If the ontology is deemed relevant, researchers need to evaluate whether ontologies are of sufficient quality for reuse. Ontologies published in a repository are usually available online in a common format and contain URIs, as those criteria need to be met for an ontology to function. In order to be suited for reuse, ontologies should at least contain clear names, and documentation of the development, structure and evaluation of the ontology should be available to the researchers. Meeting other quality criteria defined in this review, especially containing clear definitions and following a transparent maintenance plan, provide strong additional value. However, because only few ontologies meet these criteria, researchers might not necessarily want to exclude all ontologies that do not meet them. In that case, expanding or updating the ontologies might be necessary before implementing them. We encourage collaboration with ontology engineers or other researchers with expertise in ontology development whenever changes need to be made to an ontology, or if new ontologies need to be created. Lastly, we strongly recommend to use the OBO Foundry principles for development, adaptation and evaluation of ontologies. Most importantly, we encourage researchers to make their ontology and relevant documentation freely available online, preferably on an ontology repository.
Strengths and limitations
This is one of the first systematic reviews assessing the content, methods and quality of ontologies within behavioral sciences. Due to the novelty of the topic, there are few guidelines on how to best review ontologies. We, therefore, decided to perform a broad search, including ontologies identified from research articles, ontology repositories and citation searching. We also decided to include ontologies even if they were not publicly available. This allowed us to draw a comprehensive picture of existing ontologies in the field of PA, and to discover some pitfalls not identified earlier [ 8 ]. However, due to the nature of a review, we could only assess content, methods and quality based on the documentation that was publicly available. Because the amount of documentation strongly varied, we might have coded well-documented ontologies as disproportionately more qualitative in comparison to less well-documented ontologies. While we tried to compensate for this by contacting authors and asking for additional information, the response to our requests were unfortunately low. Lastly, this review only included English language ontologies. We may have missed ontologies in other languages. not English language that it adequately covers non-English language literature.
Conclusions
We identified 28 ontologies on PA and assessed their content, methods and quality according to twelve defined quality criteria. We found that most ontologies cover the activity and profile domains of PA, whereas the context domains are covered by less ontologies and in less detail. No ontology covers all domains of PA extensively enough to paint a comprehensive picture of physical activities. Whereas most ontologies meet technical criteria for quality, many fail to ensure transparency and reusability, with only eight ontologies being publicly available.
Recommendations for researchers in the field of PA include steps to identify and evaluate ontologies. We also encourage to collaborate with ontology engineers if ontologies need to be adapted, updated or created. Finally, we call for researchers to make their ontologies and extensive documentation freely available online whenever possible in order to facilitate reuse and adaptation of their ontology.
Availability of data and materials
The protocol and coding scheme used in this study areavailable in the osf repository, [ https://osf.io/6ej38/ ].
https://www.humanbehaviourchange.org/
https://osf.io/ztp9e
Ontologies identified via other sources were identified by one of the authors during screening.
http://www.bssofoundry.org/
Abbreviations
Human Behaviour Change Project
Open Biological and Biomedical Ontology
Physical Activity
Physical ACtivity Ontology
Semantic Mining of Activity, Social and Health Data
Taxonomy for Rehabilitation of Knee conditions
Uniform Resource Identifier
Ainsworth BE, Haskell WL, Leon AS, Jacobs DR, Montoye HJ, Sallis JF, et al. Compendium of physical activities: classification of energy costs of human physical activities. Med Sci Sports Exerc. 1993;25(1):71–80.
Article CAS PubMed Google Scholar
Michie S, Richardson M, Johnston M, Abraham C, Francis J, Hardeman W, et al. The behavior change technique taxonomy (v1) of 93 hierarchically clustered techniques: building an international consensus for the reporting of behavior change interventions. Ann Behav Med. 2013;46(1):81–95.
Article PubMed Google Scholar
Bauer S. Gene-category analysis. the gene ontology handbook. Methods Mol Biol. 2017;1446:175–88.
Ashburner M, Ball CA, Blake JA, Botstein D, Butler H, Cherry JM, et al. Gene ontology: tool for the unification of biology. Nat Genet. 2000;25(1):25–9.
Article CAS PubMed PubMed Central Google Scholar
Arp R, Smith B, Spear AD. Building ontologies with basic formal ontology. Mit Press; 2015.
National Academies of Sciences E and Medicine. Ontologies in the Behavioral Sciences: Accelerating Research and the Spread of Knowledge. 2022;
Louise Falzon. Scoping Review of Ontologies in the Behavioral Sciences. Paper prepared for the Committee on Accelerating Behavioral Science Through Ontology Development and Use, National Academies of Sciences, Engineering, and Medicine [Internet]. 2021; Available from: https://nap.national academies.org/resource/26464/Falzon-comissioned-paper.pdf
Norris E, Finnerty AN, Hastings J, Stokes G, Michie S. A scoping review of ontologies related to human behaviour change. Nat Hum Behav. 2019;3(2):164–72.
Michie S, Thomas J, Johnston M, Mac Aonghusa P, Shawe-Taylor J, Kelly MP, et al. The human behaviour-change project: harnessing the power of artificial intelligence and machine learning for evidence synthesis and interpretation. Implement Sci. 2017;12(1):1–12.
Article Google Scholar
West R, Godinho CA, Bohlen LC, Carey RN, Hastings J, Lefevre CE, et al. Development of a formal system for representing behaviour-change theories. Nat Hum Behav. 2019;3(5):526–36.
Michie S, West R, Finnerty AN, Norris E, Wright AJ, Marques MM, et al. Representation of behaviour change interventions and their evaluation: development of the upper level of the behaviour change intervention ontology. Wellcome Open Res. 2021;5:123.
Article PubMed PubMed Central Google Scholar
Rosse C, Mejino JL. The foundational model of anatomy ontology. In: Anatomy ontologies for bioinformatics. Springer; 2008. p. 59–117.
Riboni D, Bettini C. COSAR: hybrid reasoning for context-aware activity recognition. Pers Ubiquit Comput. 2011;15(3):271–89.
Aldenaini N, Orji R, Sampalli S. How effective is personalization in persuasive interventions for reducing sedentary behav- ior and promoting physical activity: a sys- tematic review. 2020.
Davis A, Sweigart R, Ellis R. A systematic review of tailored mHealth interventions for physical activity promotion among adults. Transl Behav Med. 2020;10(5):1221–32.
PubMed Google Scholar
Lustria MLA, Noar SM, Cortese J, Van Stee SK, Glueckauf RL, Lee J. A meta-analysis of web-delivered tailored health behavior change interventions. J Health Commun. 2013;18(9):1039–69.
Noy NF, Shah NH, Whetzel PL, Dai B, Dorf M, Griffith N, et al. BioPortal: ontologies and integrated data resources at the click of a mouse. Nucleic Acids Res. 2009;37(suppl_2):W170-3.
Smith B, Ashburner M, Rosse C, Bard J, Bug W, Ceusters W, et al. The OBO Foundry: coordinated evolution of ontologies to support biomedical data integration. Nat Biotechnol. 2007;25(11):1251–5.
OBO Foundry. Principles: Overview [Internet]. 2021. Available from: http://www.obofoundry.org/principles/fp-000-summary.html
Selçuk AA. A guide for systematic reviews: PRISMA. Turk Arch Otorhinolaryngol. 2019;57(1):57.
Rayyan – Intelligent Systematic Review [Internet]. [cited 2022 May 20]. Available from: https://www.rayyan.ai/
Jackson RC, Matentzoglu N, Overton JA, Vita R, Buttigieg PL, Carbon S, et al. OBO Foundry in 2021: operationalizing open data principles to evaluate ontologies. :8.
Mamatsashvili GG, Ponichtera K, Małkiński M, Ganzha M, Paprzycki M. Semantic-based system for exercise programming and dietary advice. In: Advances in bioinformatics, multimedia, and electronics circuits and signals. Springer; 2020. p. 105–20.
Google Scholar
El-Sappagh S, Ali F, Hendawi A, Jang JH, Kwak KS. A mobile health monitoring-and-treatment system based on integration of the SSN sensor ontology and the HL7 FHIR standard. BMC Med Inform Decis Mak. 2019;19(1):97.
Kim H, Mentzer J, Taira R. Developing a physical activity ontology to support the interoperability of physical activity data. J Med Internet Res. 2019;21(4): e12776.
Livitckaia K, Koutkias V, Kouidi E, Van Gils M, Maglaveras N, Chouvarda I. “OPTImAL”: an ontology for patient adherence modeling in physical activity domain. BMC Med Inform Decis Mak. 2019;19(1):1–15.
Alian S, Li J, Pandey V. A personalized recommendation system to support diabetes self-management for American Indians. IEEE Access. 2018;6:73041–51.
Dandan R, Desprès S, Nobécourt J. OAFE: An Ontology for the description of elderly activities. In IEEE; 2018. p. 396–403.
Dash SK, Pakray P, Porzel R, Smeddinck J, Malaka R, Gelbukh A. Designing an ontology for physical exercise actions. Springer; 2017. p. 354–62.
Hoda M, Montaghami V, Al Osman H, El Saddik A. ECOPPA: Extensible Context ontology for persuasive physical-activity applications. Springer; 2018. p. 309–18.
Behnke G, Nielsen F, Schiller M, Bercher P, Kraus M, Minker W, et al. Sloth—The interactive workout planner. In IEEE; 2017. p. 1–6.
Razzaq MA, Villalonga C, Lee S, Akhtar U, Ali M, Kim ES, et al. mlCAF: Multi-level cross-domain semantic context fusioning for behavior identification. Sensors. 2017;17(10):2433.
Villalonga C, Razzaq MA, Khan WA, Pomares H, Rojas I, Lee S, et al. Ontology-based high-level context inference for human behavior identification. Sensors. 2016;16(10):1617.
Villalonga C, den Akker H op, Hermens H, Herrera LJ, Pomares H, Rojas I, et al. Ontological modeling of motivational messages for physical activity coaching. In 2017. p. 355–64.
Zhang YF, Gou L, Zhou TS, Lin DN, Zheng J, Li Y, et al. An ontology-based approach to patient follow-up assessment for continuous and personalized chronic disease management. J Biomed Inform. 2017;72:45–59.
Berges I, Antón D, Bermúdez J, Goñi A, Illarramendi A. TrhOnt: building an ontology to assist rehabilitation processes. J Biomed Semant. 2016;7(1):1–21.
Mata F, Torres-Ruiz M, Zagal R, Guzman G, Moreno-Ibarra M, Quintero R. A cross-domain framework for designing healthcare mobile applications mining social networks to generate recommendations of training and nutrition planning. Telematics Inform. 2018;35(4):837–53.
Kotzyba M, Ponomaryov DK, Low T, Thiel M, Glimm B, Nürnberger A. Ontology-supported Exploratory Search for Physical Training Exercises. In 2015.
Phan N, Dou D, Wang H, Kil D, Piniewski B. Ontology-based deep learning for human behavior prediction with explanations in health social networks. Inf Sci. 2017;384:298–313.
Faiz I, Mukhtar H, Qamar AM, Khan S. A semantic rules & reasoning based approach for Diet and Exercise management for diabetics. In IEEE; 2014. p. 94–9.
Faiz I, Mukhtar H, Khan S. An integrated approach of diet and exercise recommendations for diabetes patients. In IEEE; 2014. p. 537–42.
Garcia-Valverde T, Muñoz A, Arcas F, Bueno-Crespo A, Caballero A. Heart health risk assessment system: a nonintrusive proposal using ontologies and expert rules. Biomed Res Int. 2014;2014:959645.
Su CJ, Chiang CY, Chih MC. Ontological knowledge engine and health screening data enabled ubiquitous personalized physical fitness (UFIT). Sensors. 2014;14(3):4560–84.
Su CJ, Tang YT, Huang SF, Li Y. Ubiquitous fitting: ontology-based dynamic exercise program generation. Springer; 2019. p. 293–302.
Button K, Van Deursen RW, Soldatova L, Spasić I. TRAK ontology: defining standard care for the rehabilitation of knee conditions. J Biomed Inform. 2013;46(4):615–25.
Button K, Nicholas K, Busse M, Collins M, Spasić I. Integrating self-management support for knee injuries into routine clinical practice: TRAK intervention design and delivery. Musculoskelet Sci Pract. 2018;33:53–60.
Dunphy E, Button K, Hamilton F, Williams J, Spasic I, Murray E. Feasibility randomised controlled trial comparing TRAK-ACL digital rehabilitation intervention plus treatment as usual versus treatment as usual for patients following anterior cruciate ligament reconstruction. BMJ Open Sport Exerc Med. 2021;7(2): e001002.
Silva P, Andrade MT, Carvalho P, Mota J. A structured and flexible language for physical activity assessment and characterization. J Sports Med. 2013;2013.
Foust JC. Ontology of Physical Exercises | NCBO BioPortal [Internet]. 2013 [cited 2021 Nov 24]. Available from: https://bioportal.bioontology.org/ontologies/OPE
Kim J, Chung KY. Ontology-based healthcare context information model to implement ubiquitous environment. Multimed Tools Appl. 2014;71(2):873–88.
Kostopoulos K, Chouvarda I, Koutkias V, Kokonozi A, Van Gils M, Maglaveras N. An ontology-based framework aiming to support personalized exercise prescription: application in cardiac rehabilitation. In IEEE; 2011. p. 1567–70.
Sachinopoulou A, Leppanen J, Kaijanranta H, Lahteenmaki J. Ontology-based approach for managing personal health and wellness information. In IEEE; 2007. p. 1802–5.
Izumi S, Kuriyama D, Itabashi G, Togashi A, Kato Y, Takahashi K. An ontology-based advice system for health and exercise. In 2006. p. 95–100.
Chen L, Lu D, Zhu M, Muzammal M, Samuel OW, Huang G, et al. OMDP: An ontology-based model for diagnosis and treatment of diabetes patients in remote healthcare systems. Int J Distrib Sens Netw. 2019;15(5):1550147719847112.
Norris E, O’Connor DB. Science as behaviour: using a behaviour change approach to increase uptake of open science. Psychol Health. 2019;34(12):1397–406.
Spasić I, Zhao B, Jones CB, Button K. KneeTex: an ontology–driven system for information extraction from MRI reports. J Biomed Semant. 2015;6(1):1–26.
Norris E, Wright AJ, Hastings J, West R, Boyt N, Michie S. Specifying who delivers behaviour change interventions: development of an intervention source ontology. Wellcome Open Res. 2021;6:77.
Norris E, Marques MM, Finnerty AN, Wright AJ, West R, Hastings J, et al. Development of an intervention setting ontology for behaviour change: specifying where interventions take place. Wellcome Open Res. 2020;5:124.
Marques MM, Carey RN, Norris E, Evans F, Finnerty AN, Hastings J, et al. Delivering behaviour change interventions: development of a mode of delivery ontology. Wellcome Open Res. 2021;5:125.
Ke SR, Thuc HLU, Lee YJ, Hwang JN, Yoo JH, Choi KH. A review on video-based human activity recognition. Computers. 2013;2(2):88–131.
Haescher M. Multi-sensory environment analysis and human activity recognition via wearable technologies.
Download references
Acknowledgements
Not applicable.
This study is part of an interdisciplinary research project, funded by the Special Research Fund (Bijzonder Onderzoeksfonds) of Ghent University.
Author information
Authors and affiliations.
Department of Experimental Clinical and Health Psychology, Ghent University, Ghent, Belgium
Maya Braun, Annick De Paepe, Marie Van De Velde & Geert Crombez
IDLab, Department of Information Technology, Ghent University – imec, Ghent, Belgium
Stéphanie Carlier, Femke De Backere & Filip De Turck
Department of Movement and Sports Sciences, Ghent University, Ghent, Belgium
Delfien Van Dyck
Nova Medical School, Comprehensive Health Research Centre (CHRC), NOVA University of Lisbon, Lisbon, Portugal
Marta M. Marques
You can also search for this author in PubMed Google Scholar
Contributions
MB, ADP and GC wrote the search protocol. MB, ADP, SC, FDB and GC created the coding schemes. MB and MVV searched, screened the articles. MB reviewed the articles with supervision from FDB, ADP and GC. MB wrote the article. All authors have read drafts of the article and provided feedback. All authors have read and approved the article.
Corresponding author
Correspondence to Maya Braun .
Ethics declarations
Ethics approval and consent to participate, consent for publication, competing interests.
The authors declare that they have no competing interests.
Additional information
Publisher’s note.
Springer Nature remains neutral with regard to jurisdictional claims in published maps and institutional affiliations.
Supplementary Information
Additional file 1., additional file 2., rights and permissions.
Open Access This article is licensed under a Creative Commons Attribution 4.0 International License, which permits use, sharing, adaptation, distribution and reproduction in any medium or format, as long as you give appropriate credit to the original author(s) and the source, provide a link to the Creative Commons licence, and indicate if changes were made. The images or other third party material in this article are included in the article's Creative Commons licence, unless indicated otherwise in a credit line to the material. If material is not included in the article's Creative Commons licence and your intended use is not permitted by statutory regulation or exceeds the permitted use, you will need to obtain permission directly from the copyright holder. To view a copy of this licence, visit http://creativecommons.org/licenses/by/4.0/ . The Creative Commons Public Domain Dedication waiver ( http://creativecommons.org/publicdomain/zero/1.0/ ) applies to the data made available in this article, unless otherwise stated in a credit line to the data.
Reprints and permissions
About this article
Cite this article.
Braun, M., Carlier, S., De Backere, F. et al. Content and quality of physical activity ontologies: a systematic review. Int J Behav Nutr Phys Act 20 , 28 (2023). https://doi.org/10.1186/s12966-023-01428-y
Download citation
Received : 08 June 2022
Accepted : 24 February 2023
Published : 13 March 2023
DOI : https://doi.org/10.1186/s12966-023-01428-y
Share this article
Anyone you share the following link with will be able to read this content:
Sorry, a shareable link is not currently available for this article.
Provided by the Springer Nature SharedIt content-sharing initiative
- Physical activity
- Classification
- Quality assessment
- Systematic review
International Journal of Behavioral Nutrition and Physical Activity
ISSN: 1479-5868
- Submission enquiries: Access here and click Contact Us
- General enquiries: [email protected]
- Search Menu
- Chemical Biology and Nucleic Acid Chemistry
- Computational Biology
- Critical Reviews and Perspectives
- Data Resources and Analyses
- Gene Regulation, Chromatin and Epigenetics
- Genome Integrity, Repair and Replication
- Methods Online
- Molecular Biology
- Nucleic Acid Enzymes
- RNA and RNA-protein complexes
- Structural Biology
- Synthetic Biology and Bioengineering
- Advance Articles
- Breakthrough Articles
- Special Collections
- Scope and Criteria for Consideration
- Author Guidelines
- Data Deposition Policy
- Database Issue Guidelines
- Web Server Issue Guidelines
- Submission Site
- About Nucleic Acids Research
- Editors & Editorial Board
- Information of Referees
- Self-Archiving Policy
- Dispatch Dates
- Advertising and Corporate Services
- Journals Career Network
- Journals on Oxford Academic
- Books on Oxford Academic
Article Contents
Introduction, conclusions, acknowledgements, appendix of authors.
- < Previous
The Gene Ontology Resource: 20 years and still GOing strong
Full list provided in Appendix.
- Article contents
- Figures & tables
- Supplementary Data
The Gene Ontology Consortium, The Gene Ontology Resource: 20 years and still GOing strong, Nucleic Acids Research , Volume 47, Issue D1, 08 January 2019, Pages D330–D338, https://doi.org/10.1093/nar/gky1055
- Permissions Icon Permissions
The Gene Ontology resource (GO; http://geneontology.org ) provides structured, computable knowledge regarding the functions of genes and gene products. Founded in 1998, GO has become widely adopted in the life sciences, and its contents are under continual improvement, both in quantity and in quality. Here, we report the major developments of the GO resource during the past two years. Each monthly release of the GO resource is now packaged and given a unique identifier (DOI), enabling GO-based analyses on a specific release to be reproduced in the future. The molecular function ontology has been refactored to better represent the overall activities of gene products, with a focus on transcription regulator activities. Quality assurance efforts have been ramped up to address potentially out-of-date or inaccurate annotations. New evidence codes for high-throughput experiments now enable users to filter out annotations obtained from these sources. GO-CAM, a new framework for representing gene function that is more expressive than standard GO annotations, has been released, and users can now explore the growing repository of these models. We also provide the ‘GO ribbon’ widget for visualizing GO annotations to a gene; the widget can be easily embedded in any web page.
The Gene Ontology resource (GO; http://geneontology.org ) is the most comprehensive and widely used knowledgebase concerning the functions of genes. In GO, all functional knowledge is structured and represented in a form amenable to computational analysis, which is essential to support modern biological research. The GO knowledgebase is structured using a formal ontology, by defining classes of gene functions (GO terms) that have specified relations to each other (Figure 1A ). GO terms are often given logical definitions, or equivalence axioms, that define the term relative to other terms in the GO or other ontologies, so that their relationships can be computationally inferred using logical reasoning (Figure 1B ). The GO structure has been meticulously constructed over the course of 20 years by a small team of ontology developers; it is constantly evolving in response to new scientific discoveries and continuously refined to represent the most current state of biological knowledge. The members of the ontology development team are expert biologists and knowledge representation specialists who read the scientific literature and engage biocurators and biological domain experts to collaboratively develop this representation of biological information.

GO structure. ( A ) Graphical representation of relationships between terms: black lines represent is_a and blue lines represent part_of (representation obtained from https://www.ebi.ac.uk/QuickGO/term/GO:0060887 ). ( B ) Equivalence axiom for the term ‘GO:0060887: limb epidermis development’, as displayed in Protégé ( 12 ).
We present here the most important updates since our last contribution to this series ( 1 ). There are currently over 45 000 terms in the ontology, linked by almost 134 000 relations. The ontology covers three distinct aspects of gene function: molecular function (the activity of a gene product at the molecular level), cellular component (the location of a gene product's activity relative to biological structures), and biological process (a larger biological program in which a gene's molecular function is utilized).
The GO knowledgebase also includes GO annotations , created by linking specific gene products (from organisms across the tree of life) to the terms in the ontology. Each annotation includes the evidence it is based upon, such as a peer-reviewed publication, using evidence codes from the Evidence and Conclusion Ontology (ECO) ( 2 ). For example, in its simplest form (what we refer to as a standard annotation ), an annotation might state that ‘human MSH2 (a gene, HGNC:7325, also represented by UniProtKB:P43246) is involved in ‘GO:0006298 DNA mismatch repair’ (a GO term), based on a ‘ECO:0000314 direct assay evidence used in manual assertion’ reported in ( 4 )’. Formally, this annotation would be represented in the knowledgebase as a ‘triple’ linking the gene to the GO term using a specific relation: UniProtKB:P43246 involved_in GO:0006298. The GO knowledgebase contains over 7 million annotations to genes/gene products from over 3,200 species ( http://amigo.geneontology.org/amigo/search/annotation ), ∼10% of which (750 000) are supported by experimental data from research papers. Nearly half of these 750 000 experimental annotations refer to genes in a relatively small number of ‘model’ organisms, listed in Table 1 . These annotations are made by a consortium of expert biocurators located worldwide, who read scientific papers, ensure the correct gene is identified, and select the most accurate and meaningful GO terms to describe the biology supported by the experimental findings. The accuracy of the GO resource is continually refined by internal checks as well as feedback from the broader GO user community to identify and fix potentially incorrect or out-of-date annotations. The wealth of experimental knowledge manually curated by biocurators is further enriched by inferences from various predictive methods, both manual and automatic, described using classes from ECO as described in ( 3 ). In most cases, these annotations are inferred from one or more experimental annotations to a homologous gene product. These may be individually reviewed by a biocurator [denoted by ‘ECO:0000250 sequence similarity evidence used in manual assertion’ (ISS) or ‘ECO:0000318 biological aspect of ancestor evidence used in manual assertion’ (IBA) evidence classes] or not reviewed [denoted by ‘ECO:0000501 evidence used in automatic assertion’ (IEA)].
Number of experimental annotations in the GO knowledgebase, 5 September 2018 release (doi:10.5281/zenodo.1410625)
Note that for the molecular function annotations, we present annotations to ‘GO:0005515 protein binding’ separately from other GO:0003674 molecular functions, as these GO:0005515 annotations are used differently than other annotations (the class itself is not very informative, but each annotation includes additional information about the specific binding partner). The number of annotations for the main species annotated by the GOC are shown, and the percentage change relative to the 2016 update is indicated in parentheses.
This structure of the GO knowledgebase, the ontology plus annotations, supports queries of the sort that are typically asked in the course of biological research, such as: ‘What are all the functions for the human ABCA1 gene?’ or ‘What are all the genes involved in the DNA mismatch repair process?’. Because each annotation is associated with evidence (ECO and reference), computer programs can answer even more specific queries, such as ‘What genes have direct experimental evidence of involvement in the DNA mismatch repair process?’, or ‘Which scientific papers provide experimental evidence about the function of the human ABCA1 gene?’. The ability of the GO knowledgebase to support computational queries is a major reason for its standing as an essential tool in biomedical research. The most obvious example is its use in GO enrichment analysis, also often called pathway analysis ( 5 ). For example, a researcher might have identified a set of 1000 genes expressed at a higher level in a cancer sample than in a matched healthy tissue sample, and would like to know if there are any functions (terms from the GO molecular function, cellular component, or biological process aspects) that are unusually common among these 1000 overexpressed genes to understand what may be driving the cancer. To reach this understanding, the functions represented in the set of 1000 genes need to be compared to the functions represented in all 20 000 human protein-coding genes. A computer can use the GO knowledgebase's structure to rapidly retrieve the all the functions that are performed by each of the 20 000 human genes, and create all possible groupings by functional class. Each grouping is tested for statistical enrichment, and the small number of enriched functional classes enables the researcher to identify candidate biological processes within the complex experimental measurement of 20,000 genes.
GO resource content
The GO knowledgebase consists of the ontology and the annotations made using the ontology. As of the 5 September 2018 release (doi:10.5281/zenodo.1410625), there were ∼45 000 terms in GO: 29 698 biological processes, 11 147 molecular functions and 4201 cellular components, linked by almost 134 000 relationships. The number of annotations (as well as the percentage change since 2016 ( 1 )) are shown in Table 1 . It is important to understand that the changes reflect two distinct processes: addition of annotations based on new evidence, and obsoleting of annotations that have been superseded by newer studies. We expect the number of obsoleted annotations to increase, due to our increasing annotation quality assurance efforts, described in more detail below.
New framework and repository, for gene function ‘models’
We have developed a more expressive computational framework for representing gene functions, which subsumes our current GO annotation framework, while maintaining compatibility. We refer to the framework as GO- C ausal A ctivity M odeling (GO-CAM, formerly referred to as ‘LEGO’ ( 1 )) and to GO-CAMs as models to distinguish them from standard annotations . A paper detailing GO-CAM is in preparation, but we summarize some properties here. In GO-CAM, each model is represented as a set of triples ( subject - relation -object, with brackets {} as a set container), e.g. { ABCA1 enables cholesterol transporter activity ; cholesterol transporter activity occurs in plasma membrane , and cholesterol transporter activity part_of cholesterol homeostasis}. Each triple is supported by one or more pieces of evidence, consisting of a class from ECO and a citable source, usually a scientific publication. GO-CAM specifies the semantics of GO annotations, and how standard GO annotations can be combined into a larger model. Each GO-CAM model is represented using the Web Ontology Language (OWL), which is converted computationally to standard GO annotations (GAF format), ensuring backward compatibility. Users can browse, view, and download the available models in different formats at: http://geneontology.org/go-cam . The number of models is currently small, and most models contain only one gene product (standard annotations extended with additional contextual information, such as cell type). The GO is currently rapidly increasing the curation of GO-CAM models, and the model repository is growing. In particular, models are now available that each represent an entire regulatory or metabolic pathway.
Changes to data access: new production pipeline
Starting in March 2018, the releases of the GO resource have been generated by a new data production pipeline using a refreshed software stack. This system allows for easily extensible error checking and improved reporting of quality assurance checks that ensure the quality and integrity of the released ontology and annotations. For users, one of the most important aspects of this pipeline is that the GO resource now produces monthly releases (named by release date) that are available at the GO site and can be referenced and obtained as stable Document Object Identifiers (DOIs) via Zenodo. This feature is critical for ensuring that GO-based analyses can be replicated in a consistent and referenceable manner through the inclusion of these DOIs and/or version of both the ontology and annotation files used ( 6 ). Our data production pipeline is currently hosted at the Lawrence Berkeley National Laboratory. We provide GO annotations in multiple formats: as standard GAF (Gene Association Format) and GPAD (Gene Product Association Data) annotation files, in Turtle (OWL serialization format) [ http://current.geneontology.org/products/ttl ], and a Blazegraph [ http://current.geneontology.org/products/blazegraph ] journal, replacing the legacy MySQL output.
Interactions with the GO user community
GO is an open project, and we encourage community contributions to the knowledgebase and software.
All users : GO can be contacted using the GO Helpdesk ( http://help.geneontology.org ) for any questions or feedback about the annotations, the ontology, software, or other GO resources. If users notice an annotation that may not be correct, they should first review the original publication or data source. If the annotation still seems inaccurate, users are encouraged to report this to the GO helpdesk, and GOC members will review the annotation and remove or modify it if justified. Authors : Authors can now see if their paper has been used for GO annotation directly in PubMed. Under the ‘LinkOut - more resources’ section of the PubMed abstract page, papers with annotations will have a link labeled ‘Gene Ontology annotations from this paper - Gene Ontology’ (see, e.g. https://www.ncbi.nlm.nih.gov/pubmed/3357510 which links to a web page on the GO site that shows the annotations based on evidence in that paper). If no GO LinkOut is present, that may indicate that the publication has not been used for GO annotation. Authors can contact the helpdesk at the GO website to suggest new annotations or changes to existing annotations. Resources or consortia : The GOC collaborates with established data resources and other groups and consortia representing a particular area of biology. Recent examples include cilium biology ( 7 , 8 ), autophagy ( 9 ) and cardiac phenotypes ( 10 ). We encourage members of other interest groups to contact us to improve the ontology and annotations in their areas of expertise. Tracking all contributions: Most aspects of the GO project management are now based in GitHub ( https://github.com/geneontology ). In addition to tracking ontology change requests ( https://github.com/geneontology/go-ontology ), we now use GitHub to collect feedback on annotations ( https://github.com/geneontology/go-annotation ). For users familiar with GitHub, we encourage them to submit any requests directly to GitHub, where they can follow all further discussion and actions. Otherwise, issues and queries can be submitted to our helpdesk ( http://help.geneontology.org ).
Increased focus on annotation quality control
The GO resource is now 20 years old. The longevity of the resource adds a challenge to maintain and update the many existing annotations, as many of the findings published during that time have become much more precise, or were reinterpreted or superseded. We have made it a high priority to identify and correct inaccurate and out-of-date legacy annotations to make sure that GO continues to consistently reflect current knowledge. We have taken a number of different approaches to tackle this challenge. First, to ensure consistency and quality, GO biocurators meet regularly for training, establishment of annotation guidelines, and coordinated review of specific areas of biology. More recently, we have made significant efforts to integrate annotation review with ontology improvements, taking advantage of suggested changes to the ontology to clarify term definitions, intended usage, and coordinate annotation practices with curators. In addition, quality assurance is performed centrally, both computationally, to ensure annotations are valid, and manually, to ensure they accurately represent the experimental findings.
We have discovered that one of the most powerful approaches to quality control and consistency is the phylogenetic approach. Originally developed as a means of propagating annotations from experimentally studied genes to evolutionarily related genes in other species, the phylogenetic perspective provides a unified view of all experimental annotations within a evolutionarily-related protein family, allowing curators to more easily find outlier annotations (see e.g. ( 11 )). In parallel, the development of GO-CAM models has been useful in identifying inconsistent annotation practices, and has provided opportunities to develop consortium-wide annotation guidelines. Another observation that emerged is that older annotations from isolated phenotypic observations, taken outside of other contextual data, often do not provide evidence of direct involvement of a gene in a biological process. If inconsistencies are noticed, they are reported to the contributing group for verification and correction as appropriate.
In a pilot quality assurance effort, we have requested the review (by GO Consortium biocurators) of ∼2500 manual annotations (<0.01% of the total corpus) that were judged questionable by one of the strategies above. Approximately 70–80% of the annotations flagged for review were modified to a more appropriate term or removed. We will continue to work on improving the quality of the annotations and reviewing legacy data when appropriate. As a result, we expect that the increase in annotations and new ontology terms may not be as rapid as in the past, at least for the main species annotated by the consortium members, as a greater proportion of our efforts will be dedicated to reviewing and revising older annotations.
Ontology revision and integration
Since our last update article, we have developed an entirely new process for ontology editing and maintenance that has dramatically increased efficiency and enabled extensive real-time quality checks. Ontology editing is now performed in an OWL-based environment using the ontology editing tool Protégé ( 12 ). The ontology is versioned and tracked using a GitHub repository ( https://github.com/geneontology/go-ontology ). One major advantage of the new ontology management process is that the work can be parallelized among multiple editors, thus increasing efficiency. In addition, real-time quality checks prevent errors that would otherwise require revisiting the same editing task more than once to correct them.
GO continues to integrate and align with external ontology resources in two main ways: import of sub-ontologies used to define GO terms, and inclusion of external cross-references. GO utilizes the structure of external ontologies to aid in reasoning and in the automatic inference of relations between GO terms ( 13 ). GO imports subsets of these external ontologies that include information about anatomical structures, cell types, chemicals and taxonomic groupings: Uberon ( 14 ), Protein Ontology ( 15 ), Plant Ontology ( 16 ), ChEBI ( 17 ), Relations Ontology ( 18 ), NCBI Taxonomy ( 19 ), Sequence Ontology ( 20 ), Ontology of Biological Attributes ( http://www.obofoundry.org/ontology/oba.html ), Fungal Anatomy Ontology ( http://www.obofoundry.org/ontology/fao.html ), Phenotypic Quality Ontology ( http://obofoundry.org/ontology/pato.html ), and Common Anatomy Reference Ontology ( http://www.obofoundry.org/ontology/caro.html ). GO also maintains cross-references between terms and multiple widely-used external resources, including Reactome ( 21 ), The Annotated Reactions Database (Rhea) ( 22 ), Enzyme Commission (EC; http://www.sbcs.qmul.ac.uk/iubmb/enzyme/ ), IntAct, Complex Portal ( 23 ) and MetaCyc ( 24 ).
Refactoring the molecular function branch of GO
Previously, there was a trend in GO molecular function ontology development to focus on adding terms that describe molecular binding activities of specific gene products. The advantage of such terms is that annotations can often be made unequivocally based on results from a single experiment. However, this approach has led to a complex ontology structure and a proliferation of annotations that individually represent only a partial functional description of a gene product. Annotations to binding terms can obscure annotations to more informative function terms, making annotations more difficult to interpret. For example, one can annotate CDK1 (UniProtKB: P06493) separately with ‘GO:0030332 cyclin binding’, ‘GO:0005524 ATP binding’, ‘GO:0005515 protein binding’, and ‘GO:0004674 protein serine/threonine kinase activity’. However, these are all aspects of a more precise molecular function that is more informative than the sum of these parts: ‘GO:0004693 cyclin-dependent protein serine/threonine kinase activity’. In the GO molecular function refactoring, we recognized that, while specific binding events are an essential mechanism by which gene products function, an individual binding activity is almost never sufficient in itself to describe molecular function in a larger biological context ( 25 ). One of the primary goals of our refactoring was to ensure that the ontology contains the terms necessary to describe these higher-level functions, and that they have a path to the root of the ontology that is not simply under the generic ‘GO:0005488 binding’ term. Accordingly, we reinstated some previously obsoleted terms and added new terms, as well as additional relations. We also addressed the structure of the ontology so that the upper-level terms would be more biologically meaningful and have more uniform specificity. We removed 8 terms from the top level and added four new terms (Figure 2 ). Most of the terms that were formerly direct children of ‘GO:0003674 molecular function’ were moved under more biologically meaningful terms: for example, ‘GO:0042056 chemoattractant activity’ and ‘GO:0045499 chemorepellent activity’ were moved under ‘GO:0048018 receptor ligand activity’, while ‘GO:0036370 d -alanyl carrier activity’ and ‘GO:0016530 metallochaperone activity’ were moved under the new term ‘GO:0140104 molecular carrier activity’ (representing an activity of ‘directly binding to a specific ion or molecule and delivering it either to an acceptor molecule or to a specific location’). We have also created a new term ‘GO:0104005 hijacked molecular function’ as a parent of terms such as ‘GO:0001618 virus receptor activity’, which is, from the standpoint of the protein being annotated, not a normal function, but nevertheless relevant for some of our users.

The molecular function branch, before and after refactoring. The term marked with an ‘x’ in the left-hand panel has been obsoleted. Terms moved (assigned to a new parent) are indicated by arrows. New terms (right panel) are marked with ‘NEW’. 1 The class label ‘electron carrier activity’ was changed to ‘electron transfer activity’.
Finally, we have made significant changes to the structure representing the molecular functions of transcription factors (Figure 3 ). This refactoring was carried out in collaboration with experts in transcription factors and gene regulation from the Gene Regulation Consortium (GRECO; http://thegreco.org ). In keeping with our design principle of having terms that describe higher-level functions, we have created a new parent term to group all functions that directly regulate transcription, ‘GO:0140110 transcription regulator activity’. The formerly top-level term ‘GO:0000988 transcription factor activity, protein binding’ has been obsoleted because this activity was partly covered by other terms in the ontology and its usage was inconsistent. Accordingly, its children have either been obsoleted or subsumed under different terms (merged or moved). The new top level term ‘GO:0140110 transcription regulator activity’ has three main children - ‘GO:0003700 DNA-binding transcription factor activity’ (formerly labeled ‘transcription factor activity, sequence-specific DNA binding), ‘GO:0140223 general transcription initiation factor activity’ and ‘GO:0003712 transcription coregulator activity’.
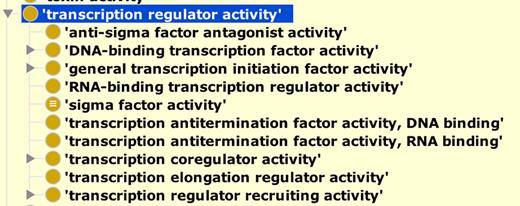
Current structure of the ‘GO:0140110 transcription regulator activity’ branch of the ontology.
The transcription factor areas of GO had previously been refactored between 2010 and 2012 ( 26 , 27 ) with the aim of more finely capturing all combinations of different types of protein and DNA binding activities (e.g. binding to different types of regulatory regions such as promoters and enhancers) and transcription regulation processes (positive and negative regulation). However, this structure, while very precise, has proved very difficult to use by biocurators, resulting in inconsistent annotations. Additionally, end-users have had difficulty with common queries, such as comprehensively identifying the set of all transcription factors in a given species. We expect that even more improvements to the ontology structure, as well as more consistent annotations to transcription regulator terms, will be available in 2019.
Defining the boundaries of biological processes: MAP kinase signaling and extracellular matrix as examples
We have used our integrated annotation review and ontology development methodology to refine two areas of the ontology, the MAP kinase signaling pathway and the representation of the extracellular matrix. Refinements to the MAPK cascade included defining the molecular functions that are parts of the process: ‘GO:0004707 MAP kinase activity’, ‘GO:0004708 MAP kinase kinase activity, ‘GO:0004709 MAP kinase kinase kinase activity’ and ‘GO:0008349 MAP kinase kinase kinase kinase activity’. All other upstream and downstream molecular functions/biological processes will be modeled in GO-CAM with causal relationships between them and the MAPK cascade. We also enumerated the types of cascades based on current literature and on discussions among expert model-organism curators, trying to keep the distinctions useful across multiple taxa. There are four direct subtypes of ‘GO:0000165 MAPK cascade’: ‘GO:0070371 ERK1 and ERK2 cascade’, ‘GO:0070375 ERK5 cascade’, ‘GO:0071507 pheromone response MAPK cascade’ and ‘GO:0051403 stress-activated MAPK cascade’. Some other MAPK processes such as ‘GO:1903616 MAPK cascade involved in axon regeneration’ will eventually be obsoleted, as these combine two or more other GO terms and can be composed in GO-CAM. For the refinement of the extracellular matrix area of the ontology, we worked with external experts to add terms that were useful grouping terms such as ‘GO:0062023 collagen-containing extracellular matrix’. We also obsoleted or merged terms that were poorly annotated and thought to represent an outdated view.
GO subsets (slims)
A GO subset (or slim) is a set of GO terms selected to provide an overview of the functions, locations or roles of a set of genes. The subset can be developed for high coverage of specific species, or to represent only certain areas of the ontology, and in most cases, contain only high-level GO terms to provide a broad biological overview. Another use of subsets is to blacklist certain terms for annotation: GO has two such subsets, one to flag terms that should not be used for manual annotation, and one for terms that should not be used at all. GO maintains two additional subsets, the Generic GO slim and the Alliance of Genome Resources ( https://www.alliancegenome.org/ ) slim. GO also hosts subsets useful to groups using GO; we currently have 11 such subsets (Table 2 ; http://www.geneontology.org/page/go-subset-guide ). Each subset provides a global overview of gene functions. Each subset now has a designated contact person to resolve any issue resulting from ontology changes (see Ontology revision and integration).
Subsets maintained in GO
Other developments
The go ribbon: a configurable tool for visualizing go annotations.
Many genes have large numbers of annotations, making it difficult to get a quick overview of a gene function, or the functions of gene sets. We have developed the GO ribbon specifically to help users visualize and explore the functions of a gene. The GO ribbon visualization metaphor borrows from a viewer originally developed by the Mouse Genome Database team ( 28 ), but in contrast, the GO ribbon was developed as a lightweight, reusable widget that can be embedded in any website, and retrieves data directly from the GO resource via API.
To generate a GO ribbon, all the functions (GO terms) associated with a gene of interest are mapped onto a specified GO subset using the ontology structure. The end result is a simple graphical representation of a gene's functions (Figure 4 ). The ribbon is interactive, allowing users to drill down to more specific functions by selecting a high-level category such as ‘GO:0030154 cell differentiation’, ‘GO:0050877 nervous system process’, or ‘GO:0003700 DNA-binding transcription factor activity’, and to filter the functions based on the evidence codes provided in the GO annotations. This overview of gene functions is particularly useful when comparing the functions of different genes in the same species, or the functions of orthologous genes across different species.

GO ribbon representation. Darker boxes indicate terms with the most annotations; white boxes represent terms that are not annotated for this protein ( Mus musculus Sox7, MGI:98369). Screenshot obtained from https://www.alliancegenome.org/gene/MGI:98369 .
The GO ribbon is a React component available on GitHub ( https://github.com/geneontology/ribbon ) and as a NPM package https://www.npmjs.com/package/@geneontology/ribbon ). The GO ribbon widget is currently used by the Alliance of Genome Resources.
GO annotations from high-throughput experiments
Data from high-throughput experiments are generally collected in a hypothesis-free manner, and consequently do not generally provide as strong evidence of gene function as small-scale molecular biology experiments that currently support most of the experimental GO annotations. In addition, high-throughput experiments can be subject to relatively high false positive rates. Users may therefore want to filter out these experimental annotations in some applications of the GO. To make this possible, starting in 2018, in collaboration with the Evidence and Conclusions Ontology ( 29 ) ( 2 ), the GO has added several new evidence codes to describe high-throughput experiments: ‘ECO:0006056 high throughput evidence used in manual assertion’ (HTP), and the subclasses: ‘ECO:0007005 high throughput direct assay evidence used in manual assertion’ (HDA), ‘ECO:0007001 high throughput mutant phenotype evidence used in manual assertion’ (HMP), ‘ECO:0007003 high throughput genetic interaction evidence used in manual assertion’ (HGI) and ‘ECO:0007007 high throughput expression pattern evidence used in manual assertion’ (HEP). To accompany the new evidence codes, we have provided annotation guidelines to help identify and curate high-throughput datasets that meet the GO Consortium annotation criteria. Consortium members have reviewed papers with more than 40 annotations using a single evidence code, and updated the evidence codes, or removed the annotations if appropriate. There are currently over 31 000 annotations that have HTP evidence codes from 140 research articles, representing <5% of experimental GO annotations. The identification of annotations derived from high-throughput experiments allows users to choose to exclude these from their analyses, if they are concerned that these annotations may lead to an increased bias in data analysis. This is likely to be particularly important, as is often the case, when GO is used to interpret types of data similar to those on which the annotations are based.
The GO resource has been under continuous development for 20 years, with no signs of slowing down. Both the ontology and annotations continue to be updated steadily, in response to new experimental findings concerning gene function, and accumulating knowledge of how genes function together in larger systems. The GO Consortium is increasing efforts to review annotations, especially those that are older and may have been superseded by newer findings. GO has always been an open, community project, and we hope that users of GO will contact us with suggestions for how we can improve the resource. GO releases are now monthly, with persistent DOI’s, and we recommend that all published GO-based analyses cite this DOI, to enable reproducibility. GO-CAM, our new framework for defining and representing gene functions with more accuracy, consistency and precision, is being used to create a growing set of curated biological models, and we encourage the analysis tool developer community to explore the new format and potential new applications of these models.
We would like to thank the domain experts Peter Yurchenco, Sylvie Ricard-Blum, Rachel Lennon, Geoff Meyer, David Sherwood and Jeff Miner for discussions leading to the refinement of the extracellular matrix area. We also want to thank all the contributors to the GO resource over the last 20 years ( http://geneontology.org/page/acknowledgments-contributors ), and all the authors of papers represented in the GO knowledgebase ( https://www.ncbi.nlm.nih.gov/pubmed/?term =loprovGeneOntol[SB]).
The GO resource is supported by grant from the National Human Genome Research Institute [U41 HG02273 to P.D.T., P.W.S., S.E.L., J.M.C., J.A.B. and supplements to grant U41 HG001315 to J.M.C., U24 HG002223 to P.W.S.]. In addition, GO Consortium members are also supported by diverse funding sources: dictyBase is supported by the National Institute of General Medical Sciences [GM064426, GM087371 to R.L.C.]; The EcoliWiki group is supported by the National Institutes of Health [GM089636]; National Science Foundation [1565146]; EMBL-EBI is funded by EMBL core funds; FlyBase is supported by the UK Medical Research Council [MR/N030117/1]; National Human Genome Research Institute [U41HG000739]; InterPro is funded by the Wellcome Trust [108433/Z/15/Z]; Biotechnology and Biological Sciences Research Council [BB/N00521X/1, BB/N019172/1, BB/L024136/1 to RDF]; The Institute for Genome Sciences GO-related work on ECO is supported by the National Science Foundation [1458400]; The Gene Regulation Consortium (GRECO) is supported by Gene Regulation Ensemble Effort for the Knowledge Commons (GREEKC) COST Action [grant CA15205]; A.L. and M.L.A. are also supported by the Research Council of Norway [project 247727]; The Institute of Cardiovascular Science, University College London (R. Lovering's group) is supported by British Heart Foundation [RG/13/5/30112]; Parkinson's UK [G-1307]; National Institute for Health Research University College London Hospitals Biomedical Research Centre; IntAct and the Complex Portal are supported by the European Molecular Biology Laboratory core funds; PomBase is supported by the Wellcome Trust [104967/Z/14/Z to S.G.O.]; MGI is supported by the National Human Genome Research Institute [HG 000330, HG 002273]; RGD is supported by and by the National Heart, Lung, and Blood Institute [HL 64541]; The UniProt Consortium is supported by the National Eye Institute, National Human Genome Research Institute, National Heart, Lung and Blood Institute, National Institute of Allergy and Infectious Diseases, National Institute of Diabetes and Digestive and Kidney Diseases, National Institute of General Medical Sciences, and National Institute of Mental Health of the National Institutes of Health under Award Number [U24HG007822], National Human Genome Research Institute under Award Numbers [U41HG007822 and U41HG002273]; National Institute of General Medical Sciences under Award Numbers [R01GM080646, P20GM103446 and U01GM120953]; Biotechnology and Biological Sciences Research Council [BB/M011674/1]; the British Heart Foundation [RG/13/5/30112]; Swiss Federal Government through the State Secretariat for Education, Research and Innovation (SERI); European Molecular Biology Laboratory core funds; The TAIR project is funded by academic institutional, corporate, and individual subscriptions. TAIR is administered by the 501(c)(3) non-profit Phoenix Bioinformatics; WormBase is supported by the US National Human Genome Research Institute [U24-HG002223]; UK Medical Research Council [MR/L001220]; UK Biotechnology and Biological Sciences Research Council [BB/K020080]; ZFIN also supported by the National Human Genome Research Institute [U41 HG002659 to M.W.]. The content is solely the responsibility of the authors and does not necessarily represent the official views of the funding agencies. Funding for open access charges: National Human Genome Research Institute [U41 HG02273].
Conflict of interest statement . None declared.
The Gene Ontology Consortium Expansion of the Gene Ontology knowledgebase and resources . Nucleic Acids Res. 2017 ; 45 : D331 – D338 .
Chibucos M.C. , Mungall C.J. , Balakrishnan R. , Christie K.R. , Huntley R.P. , White O. , Blake J.A. , Lewis S.E. , Giglio M. Standardized description of scientific evidence using the Evidence Ontology (ECO) . Database J. Biol. Databases Curation . 2014 ; 2014 : bau075 .
Google Scholar
Gaudet P. , Škunca N. , Hu J.C. , Dessimoz C. Primer on the gene ontology . Methods Mol. Biol. 2017 ; 1446 : 25 – 37 .
Fishel R. , Ewel A. , Lescoe M.K. Purified human MSH2 protein binds to DNA containing mismatched nucleotides . Cancer Res. 1994 ; 54 : 5539 – 5542 .
Khatri P. , Sirota M. , Butte A.J. Ten years of pathway analysis: current approaches and outstanding challenges . PLoS Comput. Biol. 2012 ; 8 : e1002375 .
Griffin P.C. , Khadake J. , LeMay K.S. , Lewis S.E. , Orchard S. , Pask A. , Pope B. , Roessner U. , Russell K. , Seemann T. et al. Best practice data life cycle approaches for the life sciences [version 2; referees: 2 approved] . F1000Research . 2017 ; 6 : 1618 .
Christie K.R. , Blake J.A. Sensing the cilium, digital capture of ciliary data for comparative genomics investigations . Cilia . 2018 ; 7 : 3 .
Roncaglia P. , van Dam T.J.P. , Christie K.R. , Nacheva L. , Toedt G. , Huynen M.A. , Huntley R.P. , Gibson T.J. , Lomax J. The Gene Ontology of eukaryotic cilia and flagella . Cilia . 2017 ; 6 : 10 .
Denny P. , Feuermann M. , Hill D.P. , Lovering R.C. , Plun-Favreau H. , Roncaglia P. Exploring autophagy with Gene Ontology . Autophagy . 2018 ; 14 : 419 – 436 .
Lovering R.C. , Roncaglia P. , Howe D.G. , Laulederkind S.J.F. , Khodiyar V.K. , Berardini T.Z. , Tweedie S. , Foulger R.E. , Osumi-Sutherland D. , Campbell N.H. et al. Improving interpretation of cardiac phenotypes and enhancing discovery with expanded knowledge in the Gene Ontology . Circ. Genomic Precis. Med. 2018 ; 11 : e001813 .
Feuermann M. , Gaudet P. , Mi H. , Lewis S.E. , Thomas P.D. Large-scale inference of gene function through phylogenetic annotation of Gene Ontology terms: case study of the apoptosis and autophagy cellular processes . Database J. Biol. Databases Curation . 2016 ; 2016 : baw155 .
Musen M.A. Protégé Team The Protégé Project: a look back and a look forward . AI Matters . 2015 ; 1 : 4 – 12 .
Hill D.P. , Blake J.A. , Richardson J.E. , Ringwald M. Extension and integration of the gene ontology (GO): combining GO vocabularies with external vocabularies . Genome Res. 2002 ; 12 : 1982 – 1991 .
Mungall C.J. , Torniai C. , Gkoutos G.V. , Lewis S.E. , Haendel M.A. Uberon, an integrative multi-species anatomy ontology . Genome Biol. 2012 ; 13 : R5 .
Natale D.A. , Arighi C.N. , Blake J.A. , Bona J. , Chen C. , Chen S.C. , Christie K.R. , Cowart J. , D’Eustachio P. , Diehl A.D. et al. Protein Ontology (PRO): enhancing and scaling up the representation of protein entities . Nucleic Acids Res. 2017 ; 45 : D339 – D346 .
Cooper L. , Walls R.L. , Elser J. , Gandolfo M.A. , Stevenson D.W. , Smith B. , Preece J. , Athreya B. , Mungall C. , Rensing S. et al. The plant ontology as a tool for comparative plant anatomy and genomic analyses . Plant Cell Physiol. 2013 ; 54 : e1 .
Hastings J. , Owen G. , Dekker A. , Ennis M. , Kale N. , Muthukrishnan V. , Turner S. , Swainston N. , Mendes P. , Steinbeck C. ChEBI in 2016: Improved services and an expanding collection of metabolites . Nucleic Acids Res. 2016 ; 44 : D1214 – D1219 .
Smith B. , Ceusters W. , Klagges B. , Köhler J. , Kumar A. , Lomax J. , Mungall C. , Neuhaus F. , Rector A.L. , Rosse C. Relations in biomedical ontologies . Genome Biol. 2005 ; 6 : R46 .
Federhen S. The NCBI Taxonomy database . Nucleic Acids Res. 2012 ; 40 : D136 – D143 .
Mungall C.J. , Batchelor C. , Eilbeck K. Evolution of the sequence ontology terms and relationships . J. Biomed. Inform. 2011 ; 44 : 87 – 93 .
Fabregat A. , Jupe S. , Matthews L. , Sidiropoulos K. , Gillespie M. , Garapati P. , Haw R. , Jassal B. , Korninger F. , May B. et al. The reactome pathway knowledgebase . Nucleic Acids Res. 2018 ; 46 : D649 – D655 .
Morgat A. , Lombardot T. , Axelsen K.B. , Aimo L. , Niknejad A. , Hyka-Nouspikel N. , Coudert E. , Pozzato M. , Pagni M. , Moretti S. et al. Updates in Rhea - an expert curated resource of biochemical reactions . Nucleic Acids Res. 2017 ; 45 : 4279 .
Meldal B.H.M. , Bye-A-Jee H. , Gajdoš L. , Hammerová Z. , Horáčková A. , Melicher F. , Perfetto L. , Pokorný D. , Rodriguez Lopez M. , Türková A. et al. Complex Portal 2018: extended content and enhanced visualization tools for macromolecular complexes . Nucleic Acids Res. 2019 ; doi:10.1093/nar/gky1001 .
Caspi R. , Billington R. , Fulcher C.A. , Keseler I.M. , Kothari A. , Krummenacker M. , Latendresse M. , Midford P.E. , Ong Q. , Ong W.K. et al. The MetaCyc database of metabolic pathways and enzymes . Nucleic Acids Res. 2018 ; 46 : D633 – D639 .
Thomas P.D. The gene ontology and the meaning of biological function . Methods Mol. Biol. 2017 ; 1446 : 15 – 24 .
Gene Ontology Consortium The Gene Ontology: enhancements for 2011 . Nucleic Acids Res. 2012 ; 40 : D559 – D564 .
Tripathi S. , Christie K.R. , Balakrishnan R. , Huntley R. , Hill D.P. , Thommesen L. , Blake J.A. , Kuiper M. , Lægreid A. Gene Ontology annotation of sequence-specific DNA binding transcription factors: setting the stage for a large-scale curation effort . Database J. Biol. Databases Curation . 2013 ; 2013 : bat062 .
Smith C.L. , Blake J.A. , Kadin J.A. , Richardson J.E. , Bult C.J. Mouse Genome Database Group Mouse Genome Database (MGD)-2018: knowledgebase for the laboratory mouse . Nucleic Acids Res. 2018 ; 46 : D836 – D842 .
Chibucos M.C. , Siegele D.A. , Hu J.C. , Giglio M. The Evidence and Conclusion Ontology (ECO): Supporting GO annotations . Methods Mol. Biol. 2017 ; 1446 : 245 – 259 .
Berkeley Bioinformatics Open-Source Projects (BBOP), Environmental Genomics and Systems Biology Division, Lawrence Berkeley National Laboratory (Berkeley, CA, USA): S. Carbon*, E. Douglass, N. Dunn, B. Good, N.L. Harris, S.E. Lewis, C.J. Mungall; dictyBase, Northwestern University (Chicago, IL, USA): S. Basu, R.L. Chisholm, R.J. Dodson, E. Hartline, P. Fey; Division of Bioinformatics, Department of Preventive Medicine, University of Southern California (Los Angeles, CA, USA): P.D. Thomas*, L.P Albou*, D. Ebert, M.J. Kesling, H. Mi, A. Muruganujan, X. Huang, S. Poudel, T. Mushayahama; EcoliWiki, Departments of Biology and Biochemistry and Biophysics, Texas A&M University (College Station, TX, USA): J.C. Hu, S.A. LaBonte, D.A. Siegele; FlyBase, Department of Physiology, Development and Neuroscience, University of Cambridge (Cambridge, UK): G. Antonazzo, H. Attrill, N.H. Brown, S. Fexova, P. Garapati, T.E.M. Jones, S.J. Marygold, G.H. Millburn, A.J. Rey, V. Trovisco; FlyBase, The Biological Laboratories, Harvard University (Cambridge, USA): G. dos Santos, D.B. Emmert, K. Falls, P. Zhou; FlyBase, Department of Biology, Indiana University , (Bloomington, USA): J.L. Goodman, V.B. Strelets, J. Thurmond; GO-EMBL-EBI (Hinxton, UK): M. Courtot, D. Osumi-Sutherland, H. Parkinson, P. Roncaglia; Gene Regulation Consortium (GRECO), Norwegian University of Science and Technology (Trondheim, Norway): M.L. Acencio, M. Kuiper, A. Lægreid; Gene Regulation Consortium (GRECO), Radboud University (Nijmegen, The Netherlands): C. Logie; Institute of Cardiovascular Science, University College London (London, UK): R.C. Lovering, R.P. Huntley, P. Denny, N.H. Campbell, B. Kramarz, V. Acquaah, S.H. Ahmad, H. Chen, J.H. Rawson; Institute for Genome Sciences, University of Maryland School of Medicine (Baltimore, MD, USA): M. C. Chibucos, M. Giglio, S. Nadendla, R. Tauber; IntAct/Complex Portal, EMBL-EBI (Hinxton, UK): M.J. Duesbury, N Del-Toro, B.H.M. Meldal, L. Perfetto, P. Porras, S. Orchard, A. Shrivastava, Z. Xie; InterPro, EMBL-EBI (Hinxton, UK): H.Y. Chang, R.D. Finn, A.L. Mitchell, N.D. Rawlings, L. Richardson, A. Sangrador-Vegas; Mouse Genome Informatics, The Jackson Laboratory (Bar Harbor, ME, USA): J.A. Blake, K.R. Christie, M.E. Dolan, H.J. Drabkin, D.P. Hill*, L. Ni, D. Sitnikov; PomBase, University of Cambridge (Cambridge, UK): M.A. Harris, S.G. Oliver, K. Rutherford, V. Wood; PomBase, The Francis Crick Institute (London, UK):J. Hayles; PomBase, University College London (London UK): J. Bahler, A. Lock; RGD, Medical College of Wisconsin (Milwaukee, WI, USA): E.R. Bolton, J. De Pons, M. Dwinell, G.T. Hayman, S.J.F. Laulederkind, M. Shimoyama, M. Tutaj, S.-J. Wang; Reactome, Department of Biochemistry & Molecular Pharmacology, NYU School of Medicine (New York, NY, USA): P. D’Eustachio, L. Matthews; Renaissance Computing Institute, University of North Carolina (Chapel Hill, NC, USA): J.P. Balhoff; SGD, Department of Genetics, Stanford University (Stanford, CA, USA): S.A. Aleksander, G. Binkley, B.L. Dunn, J.M. Cherry, S.R. Engel, F. Gondwe, K. Karra, K.A. MacPherson, S.R. Miyasato, R.S. Nash, P.C. Ng, T.K. Sheppard, A. Shrivatsav VP, M. Simison, M.S. Skrzypek, S. Weng, E.D. Wong; SIB Swiss Institute of Bioinformatics (Geneva, Switzerland): M. Feuermann, P. Gaudet*; TAIR, Phoenix Bioinformatics (Fremont, CA, USA): E. Bakker, T.Z. Berardini, L. Reiser, S. Subramaniam, E. Huala; UniProt: EMBL-EBI (Hinxton, UK), SIB Swiss Institute of Bioinformatics (SIB) (Geneva, Switzerland), and Protein Information Resource (PIR) (Washington, DC, USA and Newark, DE, USA): C. Arighi, A. Auchincloss, K. Axelsen, G., Argoud-Puy, A. Bateman, B. Bely, M.-C. Blatter, E. Boutet, L. Breuza, A. Bridge, R. Britto, H. Bye-A-Jee, C. Casals-Casas, E. Coudert, A. Estreicher, L. Famiglietti, P. Garmiri, G. Georghiou, A. Gos, N. Gruaz-Gumowski, E. Hatton-Ellis, U. Hinz, C. Hulo, A. Ignatchenko F. Jungo, G. Keller, K. Laiho, P. Lemercier, D. Lieberherr, Y. Lussi, A. MacDougall, M. Magrane, M. J. Martin, P. Masson, D.A. Natale, N. Hyka-Nouspikel, I. Pedruzzi, K. Pichler, S. Poux, C. Rivoire, M. Rodríguez-López, T. Sawford, E. Speretta, A. Shypitsyna, A. Stutz, S. Sundaram, M. Tognolli, N. Tyagi, K. Warner, R. Zaru, C. Wu; University at Buffalo, Department of Biomedical Informatics (Buffalo, NY, USA): AD. Diehl; WormBase California Institute of Technology (Pasadena, CA, USA), Wellcome Trust Sanger Institute (Hinxton, UK), EBI (Hinxton, UK), and Ontario Institute for Cancer Research (Toronto, Canada): J. Chan, J. Cho, S. Gao, C. Grove, M.C. Harrison, K. Howe, R. Lee, J. Mendel, H.-M. Muller, D. Raciti, K. Van Auken*, M. Berriman, L. Stein, P. W. Sternberg; ZFIN, University of Oregon (Eugene, OR, USA): D. Howe, S. Toro, M. Westerfield.
*Authors who contributed significantly to writing this manuscript.
Author notes
Email alerts, citing articles via.
- Editorial Board
Affiliations
- Online ISSN 1362-4962
- Print ISSN 0305-1048
- Copyright © 2024 Oxford University Press
- About Oxford Academic
- Publish journals with us
- University press partners
- What we publish
- New features
- Open access
- Institutional account management
- Rights and permissions
- Get help with access
- Accessibility
- Advertising
- Media enquiries
- Oxford University Press
- Oxford Languages
- University of Oxford
Oxford University Press is a department of the University of Oxford. It furthers the University's objective of excellence in research, scholarship, and education by publishing worldwide
- Copyright © 2024 Oxford University Press
- Cookie settings
- Cookie policy
- Privacy policy
- Legal notice
This Feature Is Available To Subscribers Only
Sign In or Create an Account
This PDF is available to Subscribers Only
For full access to this pdf, sign in to an existing account, or purchase an annual subscription.

An official website of the United States government
The .gov means it’s official. Federal government websites often end in .gov or .mil. Before sharing sensitive information, make sure you’re on a federal government site.
The site is secure. The https:// ensures that you are connecting to the official website and that any information you provide is encrypted and transmitted securely.
- Publications
- Account settings
Preview improvements coming to the PMC website in October 2024. Learn More or Try it out now .
- Advanced Search
- Journal List
- Cancers (Basel)

Ontologies and Knowledge Graphs in Oncology Research
Associated data.
Not applicable.
Simple Summary
Cancer is a complex phenomenon and cancer research is increasingly data-rich. Representing this knowledge in a manner that is both human and computer-friendly can help manage and analyze the high volumes of complex cancer data that are created by scientific research and health care. This review looks at the last decade of works on using ontologies—computational representations of knowledge—in cancer, describing their contributions and achievements and charting a path for future research in this area.
The complexity of cancer research stems from leaning on several biomedical disciplines for relevant sources of data, many of which are complex in their own right. A holistic view of cancer—which is critical for precision medicine approaches—hinges on integrating a variety of heterogeneous data sources under a cohesive knowledge model, a role which biomedical ontologies can fill. This study reviews the application of ontologies and knowledge graphs in cancer research. In total, our review encompasses 141 published works, which we categorized under 14 hierarchical categories according to their usage of ontologies and knowledge graphs. We also review the most commonly used ontologies and newly developed ones. Our review highlights the growing traction of ontologies in biomedical research in general, and cancer research in particular. Ontologies enable data accessibility, interoperability and integration, support data analysis, facilitate data interpretation and data mining, and more recently, with the emergence of the knowledge graph paradigm, support the application of Artificial Intelligence methods to unlock new knowledge from a holistic view of the available large volumes of heterogeneous data.
1. Introduction
Understanding complex phenomena that cannot be modeled purely mathematically is a challenging endeavor transverse to all biomedical research. Ultimately, all boils down to the complex interplay between genes and environment, which manifests in the interactions between the cells in an organism, between host and pathogen, between drug and body. From its genesis, medicine focused on understanding the phenomena which can be generalized between individuals, dating back to the first texts on anatomy by the Ancient Egyptians. Indeed, nomenclature and classification are the first steps towards understanding complex phenomena, and are inextricable from modern medicine, which relies on its precise terminology and its compendium of pathogens, diseases, symptoms, genes and mutations, and drugs and therapies, as well as of the relationships between them.
Over the last three decades, the rise of the digital age and subsequent informatization of clinical records and biomedical research drove the encoding of terminologies, classification schemes and knowledge models into digital machine-readable formats (often captured under the umbrella term ‘ontology’) to promote standardization, support information systems, and enable knowledge discovery. One of the first major efforts to this effect in the biomedical domain was the compilation of the Systematized Nomenclature of Medicine—Clinical Terms (SNOMED CT) [ 1 ] to support the standardization and interoperability of clinical information systems and electronic health records. Another major effort was the classification and trans-species standardization of gene functional characteristics under the Gene Ontology (GO) [ 2 ]. In the footsteps of these efforts, several hundred other ontologies have been developed for the biomedical domain throughout the years [ 3 ], among which we must note the National Cancer Institute Thesaurus (NCIt), a compendium of terminology spanning all aspects of cancer research and health care [ 4 ].
More recently, medicine has been witnessing a shift towards the particular, enabled by the decreasing costs of acquiring genetic information, and driven by the understanding that tailored treatments that contemplate the genetic makeup of the patient will likely be more effective and less prone to nefarious side-effects. Cancer is the family of diseases that is benefiting from these precision (or personalized) medicine approaches the most, as despite commonalities, each cancer is genetically unique, and can react very differently to different types of treatment. Moreover, understanding the fine differences between cancer cells and healthy cells can be the key for more successful and less aggressive treatments. Yet the precision medicine paradigm places additional emphasis on having a holistic understanding of the gene–environment interplay in all its manifestations, which requires the integrative analysis of large volumes of heterogeneous data that are individually already complex (e.g., clinical records, medical imaging, transcriptomic data, immunopeptidomic data) [ 5 ]. Here too, ontologies have been playing an important role in enabling data integration and facilitating data analysis.
In this article, we review the applications of ontologies in cancer research over the past decade, summarizing published works within this time frame, and categorizing them with respect to their usage of ontologies. Section 2 details core concepts underlying this review article, Section 3 outlines the methodology adopted to conduct the review, Section 4 summarizes both the ontologies reused in the works and the ones created for them, Section 5 reviews and categorizes the aforementioned published works, and Section 6 features our prospects regarding the present and future use of ontologies in cancer research.
2. Background
2.1. ontologies.
The term “ontology” was borrowed from philosophy to computer science to signify a machine-readable formalization of a conceptualization pertaining to a particular domain of knowledge [ 6 ]. That is to say, an ontology is a digital artifact that can be interpreted by both humans and computers and which encodes the terminology and the semantic relations between concepts in a given domain. The term “ontology” is often used with some latitude, also encompassing thesauri [ 7 ]. While our review of published works adopts the same encompassing perspective, it is important to make a formal distinction between ontologies proper and thesauri due to their different purposes and applications.
Ontologies proper are typically encoded in the Web Ontology Language (OWL), developed by the W3C OWL Working Group [ 8 ], which includes various serializations, namely the Open Biomedical Ontologies (OBO) format or the more popular Resource Description Framework (RDF) format in which statements take the form of triples of the form <subject> <predicate> <object>. OWL defines several types of entities which can be used in constructing ontologies, such as: classes, datatypes, object properties, data properties, annotation properties, individuals and literals, among others. All entities in an ontology are identified by an International Resource Identifier (IRI), although in OBO ontologies this is abbreviated to an alphanumeric code. Annotation properties (e.g., label ) are used to describe the entities in the ontology for human readers, and thus, encode the terminological component of ontologies; they have no semantic value. Individuals (or instances) and literals are data-level entities representing, respectively, concrete objects (e.g., my heart ) and data values (e.g., “60 beats/min”). The remaining entities are model-level, with classes representing abstract sets of individuals (e.g., heart ), datatypes representing abstract sets of literals (e.g., string ), object properties representing relations that can be used to connect individuals (e.g., part of ) and data properties representing attributes that can be used to describe individuals with literals (e.g., has heart beat ). Moreover, OWL defines intrinsic properties that can be used to connect classes ( subclass , disjoint ), to assert that individuals belong to a class ( type ), or to constrain object or data properties with respect to the classes that can have them as subjects ( domain ), the classes or datatypes they can take as objects ( range ), or their usage and logic (e.g., transitive , symmetric ). Finally, OWL enables the definition of class expressions, which are classes defined semantically, for example through application of logical operators ( union , intersection , not ) between classes, or through existential, universal or cardinality restrictions on objects or data properties (e.g., part of some chest , which can be applied to class heart ). OWL ontologies have different degrees of expressiveness depending on which of these features they use, ranging from simple class hierarchies up to semantically intricate knowledge models, which has implications on the possible applications of ontologies. Namely, OWL supports deductive reasoning, that is to say, the use of logical inference to derive non-stated facts from the collection of facts explicitly asserted in the ontology, which will be both harder and more likely to result in non-evident facts the more expressive the ontology is.
Ontologies are often published with only the model-level layer, serving as knowledge models for a given domain, without any data. In some cases, ontologies are used to annotate external data, such as text documents or database entries, without actually instantiating the ontology (e.g., the Gene Ontology is used to annotate genes and proteins, but these are not individuals of the ontology). In other cases, ontologies are developed (or adopted) to serve as the semantic backbone for describing data in a machine interpretable form. When a large number of individuals is represented in a graph that employs an ontology as its schema, we can consider it a Knowledge Graph (KG) [ 9 ]. Figure 1 depicts a simplified example of a KG, based on NCIt. Classes are represented as circles in a descending hierarchy stemming from the superclass “owl:Thing”, class instances as grey rectangles, and relationships between them are depicted as arrows, corresponding to object properties in an ontology. This KG shows the network around the concepts renal cell carcinoma, MET gene, antineoplastic agent and protein tyrosine kinase, with instances of patient (“Patient X”) and antineoplastic agent (“Sunitinib”).

Knowledge graph representing a smaller network that includes renal cell carcinoma, MET gene, antineoplastic agent and proten tyrosine kinase, with instances of a Patient X and the drug Sunitinib. All concepts are derived from the class owl:Thing. Adapted from the NCIt.
Thesauri are much simpler than ontologies, and are typically encoded in the Simple Knowledge Organization System (SKOS), which, curiously, is defined on top of OWL. In SKOS, there is no data-level layer, only a model-level layer comprised of concepts , their terminological characterization through annotations, and the loose semantic relations between them ( broader , narrower , related ). Thus, thesauri are almost exclusively terminological, and do not enable many of the more sophisticated applications of ontologies proper, namely applications that involve reasoning.
2.2. Ontologies in Cancer Research
The ability to model complex domains is the reason why ontologies are suitable for cancer research and healthcare. For an especially complex disease, such as cancer, that tends towards individual uniqueness and is comprised of various factors and variables, the ability to represent it fully in a manner that can be understood by both clinicians and researchers, and machine algorithms, is invaluable. As such, ontologies represent a unique opportunity to support the domain complexity while allowing for the construction of equally complex solutions that further aid in diagnosing and treating cancer.
At present, there are numerous publicly available biomedical ontologies that have as their principal aim the description of cancer and its characteristics. The National Cancer Institute Thesaurus (NCIt) is perhaps the most often seen. Additionally, there are other biomedical ontologies that, while not directly related to the subject of cancer, are invaluable in its research, for describing fundamental concepts of biology and medicine that form a solid base on which further information stands. Of these, the Gene Ontology (GO) is the most commonly used.
Ontologies in cancer research can be used in varied manners with differing focal objectives. First, despite the fact that cancer-focused ontologies already exist, further conceptualizations of the domain can be developed in the form of new ontologies [ 10 ]. These can be reformulations of actual ontologies, updated to include more entities, or even a new, original, ontology to establish a previously less explored section of knowledge. Furthermore, ontologies can be used to annotate data and connect it to the overall context of the domain it pertains to [ 11 ]. In this way, for instance, a single value is not simply an isolated value, it is now a single result value from an RNA Sequencing experiment that is placed in a particular section of biomedical knowledge and holds specific relationships to the remaining domain. This annotated value can then be further integrated into developing solutions and their overall context. In addition, ontologies can be directly used as vocabularies to support the organization of data according to known domain information [ 12 ]. One objective for this use is, for instance, allowing users to search data that has been annotated using ontologies in a database. Furthermore, NLP methods also need a comprehensive set of terms to use in their application, that then allows for the identification of this information in long-form text, for example [ 13 ]. Due to their axiom-based structure, ontologies can support reasoning applications, first to confirm consistency in the ontology and data themselves [ 14 ] but also to obtain further inferences from the formal definitions that are established by the ontologies [ 15 ]. Lastly, annotation of data with ontologies allows for further use in mining and analyzing this data, for example, with enrichment methods or similarity measures [ 16 , 17 ]. Additionally, there has been an increase in the use of ontology-structured data as input for ML methods, particularly in the biomedical domain with, for example, gene function predictions and clinical decision support systems [ 18 , 19 ].
3. Materials and Methods
3.1. initial search and screening.
We carried out an initial search of PubMed [ 20 ] on 10 January 2022 with the search query: (“ontology” OR “knowledge graph”) AND “cancer” . We restricted the search to open access articles between between 2012 and 2021, setting the search to both Title and Other Term , and in the case of the “cancer” query, additionally also MeSH Terms . We complemented this initial search with a search of Google Scholar [ 21 ] on 21 March 2022 with the search query: (“ontology” OR “knowledge graph”) AND (“cancer” OR “oncology”) . The search was constrained to only the title and between 2012 and 2021. The combined results of the two searches were 360 articles.
We screened the resulting lists of articles with the following exclusion criteria: duplicated articles, non-open access articles, and out of scope articles. The latter encompassed articles not related to cancer (misclassification, typos such as oncology/ontology, or mention of only cancer cell lines but not to cancer), articles which did not clearly describe the use of ontologies, and review articles. Additionally, from the Google Scholar results we also excluded theses and non-international and/or non-peer-reviewed publications (which were not an issue in the PubMed search). The screening was conducted in stages, by first examining the title and accessibility of the article, then reading the abstract, and finally reading the article in its entirety. From the initial list of 360 articles, the screening resulted in only 141. A workflow diagram of the whole process can be viewed in Figure 2 .

PRISMA flowchart with the steps taken to reach the final list of articles for categorization.
3.2. Categorization
We developed a novel categorization scheme composed of 14 hierarchical categories that describe how the reviewed works employ ontologies and knowledge graphs. These categories fall into two main branches: Terminology-focused applications and Semantic-focused applications .
The original purpose of clinical and biomedical ontologies was to serve as a source of controlled terminology to tackle the challenges of data-intensive research and clinical practice. As biomedical data production increases and the further it spreads across databases and repositories, there is a reinforced need to connect it to the overall context and to assign the same “meaning” to data that is saved in different and independent places. Ontologies represent the domain concepts in a standardized manner—using a unique identifier for each concept—and placing data into this context increases its own individual reusability by ensuring that it will be understood by anyone, but also, it allows for data from different sources to be easily matched in their relation to a specific entity.
We have organized Terminology-focused applications under four categories:
- Data Annotation: ontologies are used to describe data under a common schema, linking data objects to ontology classes that describe them.
- Data Integration: ontologies support the integration of different data sets or databases.
- Database Interface: ontologies are used to support user interfaces for databases, where labels of ontology classes and relations allow text annotation. These interfaces are notably useful in dealing with medical data, for integration and querying of different knowledge resources.
- NLP: ontologies are used as the vocabulary source for Natural Language Processing (NLP) methods, where entities, events or relations in a text are identified through the corresponding ontology labels.
Semantic-focused applications fall under two sub-categories, which are further subdividided:
- – Inference of New Knowledge: complex reasoning-based queries can reveal novel biological knowledge based on the already defined axioms.
- – Error Detection: reasoning applied to check for consistency (or contradictions) in the ontology.
- – Semantic Filtering: ontology-based annotations are used to filter and process data.
- – Semantic Similarity: ontology-based annotations are used to compare data entities.
- – Machine Learning: ontologies and KGs are explored by machine learning algorithms.
- – Gene Set Enrichment: statistical analysis of gene set ontology-annotations.
From the final list, articles were sorted into one or more of the 10 leaf categories according to how the work uses ontologies.
The schema of classification is shown in Figure 3 , outlining all the categories and their hierarchical organization used in the following sections.

Classification schema for the works included in this articles.
4. Ontologies in Oncology
4.1. ontologies used in the reviewed applications.
One of the ontologies most commonly used in cancer research is, as expected, the National Cancer Institute thesaurus (NCIt) [ 22 ], which is a comprehensive ontology devoted specifically to cancer and encompassing both the clinical and research aspects. The SNOMED-CT [ 1 ], a broad scope healthcare ontology that has played a key role in systematizing electronic health records, has been used in applications involving clinical data. UMLS [ 23 ] is also popular, and is the largest compendium of biomedical terminology, aggregating several healthcare ontologies and vocabularies (namely NCIt and SNOMED-CT) and including mappings between them to enable interoperability.
The Medical Subject Headings (MeSH) thesaurus [ 24 ], which are used to index scientific publications, have often been used for bibliographic searches and natural language processing applications. The Disease Ontology (DO) [ 25 ] is narrower in scope than the UMLS, focusing only on diseases, but also includes extensive mappings to other healthcare vocabularies (namely MeSH, NCIt and SNOMED-CT).
Other ontologies with narrower scope nevertheless describe aspects that are critical for cancer research. Among them, we include the oncology subset (ICD-O) of the International Classification of Disease (ICD) [ 26 ], which categorizes tumors; the Ontology for Biomedical Investigations (OBI) [ 27 ], which aims to describe the terms related to biological and medical investigations; the Cell Line Ontology [ 28 ] which classifies cell lines; the Time Event Ontology (TEO) [ 29 ], which models temporal expressions and is especially useful when dealing with timed occurrences as healthcare often includes; and the Gene Ontology (GO) [ 2 ], which describes gene functions. The latter is the most used ontology of the works reviewed, as it is employed in almost all Gene Set Enrichment applications.
4.2. Ontologies Created for the Reviewed Applications
Several works pertaining to ontologies in cancer research reported on the creation of new ontologies, as summarized in Table 1 . The fact that multiple ontologies have been developed in this domain reflects the fact that an ontology is a conceptualization formalized for a particular objective, which represent a given point of view of the underlying domain. As such, despite the existence of several ontologies within the domain, it is often necessary to develop new ontologies for different purposes or to model novel datasets. This is also a testament to the complexity of the cancer research domain, and the several biomedical disciplines it traverses.
New ontologies.
One common reason why new ontologies have been developed was to semantically formalize already existing standards. Within this category, Nicholson et al. [ 30 ] derived the ENCR core-data ontology from the European Network of Cancer Registries (ENCR) data-validation rules to further support the validation of cancer datasets through an unambiguous formalization and ensure coherence through automatic reasoning logic. Similarly, Zhang et al. [ 31 ] also developed the Ontology for the Documentation of vAriable selecTion and daTa sourcE Selection and inTegration (OD-ATTEST) based on a set of reporting guidelines for cancer risk factor variable and data source selection to serve as a standardization of data models. With the aim of describing cancer cells and capturing the properties of tumorigenesis, Rasmussen et al. [ 32 ] created the OncoCL. Jusoh et al. [ 33 ] built a breast cancer ontology using a hybrid approach to help integrate cancer data from different sources into a single database. Furthermore, in the breast cancer domain, Myneni et al. [ 34 ] created OntoMama to assist medical students and professionals. Malty et al. [ 35 ] created an ontology of standardized cancer treatments that maps to standard nomenclatures based on HemOnc. Dinakarpandian et al. [ 36 ] created the Temporal Ontology for Comparing the Survival Outcomes (TOCSOC), a temporal ontology of survival outcome measures of clinical trials in oncology, reusing numerous ontologies. PCLiON is a new standardized lifestyle ontology created by Chen et al. [ 37 ], reusing multiple ontologies to harmonize the different data types related to prostate cancer. Looking to generalize the pattern of definitions to correctly classify all gastrointestinal tumor configurations, Herrmann et al. [ 38 ] developed their ontology based on BioTopLite2.
Another common reason for ontology development is to create a semantic model for existing datasets. For example, Esteban-Gil et al. [ 39 ] used data from a cancer registry relational database to develop a semantic model that can then be queried to analyze patient data through ontology-driven search. The NeoMark European project [ 11 ] also developed a specialized ontology for their data content, the NeoMark Ontology, built from its existing database. Amith et al. [ 40 ] used a lightweight Open Information Extraction (OIE) tool to extract semantic information from MedlinePlus and seed a knowledge-base. To represent obesity related cancer information, organize and allow data querying, Elhefny et al. [ 41 ] reused DOID to develop the Fuzzy Ontology for Obesity-Related Cancer (FOORC).
Ontologies have also been developed to harmonize the communication between clinicians and patients, namely by exploiting social media. Tapi Nzali et al. [ 42 ] built a Consumer Health Vocabulary (CHV) in french for breast cancer by mapping terms from forum messages and standardized medical terms. Lee et al. [ 43 ] created an ontology to understand information needs and emotions regarding cancer from social media. Myneni et al. [ 34 ] developed the Profile Ontology for Cancer Survivors (POCS) to facilitate the fast development of patient-engaging mobile apps.
Supporting the development of applications to aid diagnosis and treatment by providing a semantic representation of existing knowledge has been another major motivation for the development of new ontologies. For hepatocellular carcinoma (HCC), Messaoudi et al. [ 44 ] developed the Ontology of Hepatocellular Carcinoma (OntHCC) to support their application in the detection of nodules in medical imaging, while Gurcan et al. [ 45 ] created the Quantitative Histopathological Imaging Ontology (QHIO) to represent both data and methods used in clinical imaging and analysis. Boeker et al. [ 46 ] developed TNM-O to represent the Tumor–Node–Metastasis (TNM) classification of malignant tumors and Tagliaferri et al. [ 47 ] developed the ENT COBRA (COnsortium for BRachytherapy data Analysis) ontology to standardize data collection for head and neck cancer patients that have been specifically treated with interventional radiotherapy, while SKIN-COBRA has a similar objective for non-melanoma skin cancer patients with the same treatment [ 48 ]. With a very focused aim, Oyelade et al. [ 14 ] proposed Breast Cancer Fuzzy Ontology (BCFO) to address vagueness in the domain of this specific cancer. Mahmoodi et al. [ 49 ] manually created the Gastric Cancer Ontology (GCO) with experts to support the extraction of association rules. Gao et al. [ 50 ] constructed a treatment-based cancer ontology using a Bayesian derivation that focuses on cancer reclassification and drug inference. For lung cancer, Sesen et al. [ 51 ] constructed the LUCADA ontology to use with the clinical decision support application Lung Cancer Assistant. In the domain of bladder cancer, Barki et al. [ 52 ] developed an ontology to predict side effects caused by treatments.
Finally, ontologies have been developed for enabling data interoperability and integration, a pressing demand given the increasing volume of heterogeneous data sources available for cancer research. To study the connection between various risk factors and cancer survival, Zhang et al. [ 53 ] created the Ontology for Cancer Research Variables (OCRV) reusing some existing resources, and then linked it to a data integration pipeline. Lin et al. [ 10 ] developed the Cancer Care Treatment Outcome Ontology (CCTOO) that organizes high-level oncology treatment end points into four domains: cancer treatment, health services, physical, and psycho-social health-related concepts. To aid drug target prediction, Tao et al. [ 54 ] created the CRC ontology, reusing PharmGKB. Balasubramanian et al. [ 55 ] reused BFO and created the Ontology of Cancer Related Social-Ecological Variables (OCRSEV) to enable data integration and posterior association between Social-Economical Factors and health outcomes in cancer. Aiming to increase interoperability between data sources to allow the creation of Big Data studies that involve several treatment centers, Bibault et al. [ 56 ] created the Radiation Oncology Structures (ROS) based on FMA. To also support integrative data analysis in cancer outcomes research, Zhang et al. [ 57 ] created the Ontology for Documentation of Variable and Data Source (ODVDS) reusing BFO. Divakar et al. [ 58 ] developed CCOWL in order to analyze patient’s cytological tissue images of cervical cancer. Additionally, RiskExplorer was created by Daowd et al. [ 59 ] to represent causal associations between the incidence of breast cancer and risk factors.
Some works also report on updates or extensions to existing ontologies, motivated by some of the same objectives for creating new ontologies. Serra et al. [ 60 ] developed the Cancer Cell Ontology (CCL) as an extension of the Cell Ontology (CL), to serve as a formal representation of immunophenotyping cell types from hematologic malignancies. The Cell Line Ontology (CLO) was updated and extended by Ong et al. [ 61 ] to include NIH Common Fund Library of Integrated Network-based Cellular Signatures (LINCS) cell lines, with a subset LINCS-CLOview being generated. Campbell et al. [ 62 ] created additional concepts for Systematized Nomenclature of Medicine Clinical Terms (SNOMED CT) that unify it with Logical Observation Identifier Names and Codes (LOINC) for colorectal and breast cancer.
5. Ontologies and Knowledge Graph Applications in Cancer Research
The categorization of the reviewed works relied exclusively on the information presented in the article and no additional searches were conducted to obtain further details. The information gathered in the process of categorization is presented in Table 2 , Table 3 and Table 4 organized into columns relevant to each category.
Terminology-focused applications.
Semantic-focused applications: reasoning with ontologies.
Semantic-focused applications: mining and analyzing multimodal data with ontologies.
5.1. Terminology-Focused Applications
Table 2 describes the articles from these categories, according to the ontologies and data employed and cancer type.
5.1.1. Data Annotation
Most Data Annotation works use existing ontologies, such as NCIt, Medical Subject Headings (MeSH), and GO, among others, but there are quite a few instances where new ontologies were created to address specific needs.
In breast cancer, Zhu et al. [ 15 ] used the semantic modeling of drugs from PharmGKB to infer repositioning. As cancer care is a continuum, Myneni et al. [ 34 ] developed an ontology-driven adolescent and young adult survivor engagement framework, to aid the development of mobile apps for information dissemination about treatments and effects of cancer therapies provided through Survivorship Care Plans. Esteban-Gil et al. [ 39 ] created a semantic representation of data from a cancer registry database, that results in a model that can be reused and extended to other registries and is capable of supporting further semantic queries on patient profiles that are crucial to research. Yan et al. [ 13 ] used NLP tools and an enriched ontology from the MeSH graph to develop UDT-RF, aiming to categorize literature into the corresponding cancer hallmarks through text annotation by estimating the information of interest contained. Using the Time Event Ontology (TEO), Chen et al. [ 64 ] semantically modeled the time component of Common Data Elements (CDEs) that, in capturing clinical research data, highly benefit from a temporal dimension. For HCC, in addition to developing OntHCC, Messaoudi et al. [ 44 ] used it to help in the classification of the staging of tumors that are detected in medical imaging.
5.1.2. Data Integration
A vital part of having large amounts of data in differing repositories and/or originating from various sources is integrating them into a single cohesive semantic representation.
Salvi et al. [ 11 ] used a focused ontology to annotate their data from various sources that they have compiled in their relational database concerning Oral Squamous Cell Carcinoma (OSCC). The web-based application LncRNA Ontology was developed by Li et al. [ 65 ] from the results of their approach to predict probable functions of most human long non-coding RNAs (lncRNAs). Focusing on reusability and comparison of different sources, Milian et al. [ 66 ] developed a method that automatically structures clinical trial eligibility criteria from text. Kim et al. [ 67 ] used a graph-based framework that integrates multi-omics data with genomic knowledge in order to improve predictions of clinical outcomes. Wu et al. [ 68 ] developed a focused view of the DO from a variety of cancer datasets of various sources in order to enable pan-cancer analysis across datasets. Bona et al. [ 69 ] focused on accessibility of non-image data from the Cancer Imaging Archive (TCIA) by using ontologies to integrate it into semantic representations. In their two papers, refs. [ 53 , 70 ] also created a focused ontology, OCRV, but then used it with a data integration pipeline for data in relational databases with the aim of making the semantic relationships explicit and clear across different sources. Hasan et al. [ 71 ] developed a prototype of a KG that semantically encodes cancer registry data with the expressed aim of enabling the connection to third-party data to further enable new research. Li et al. [ 72 ], on the other hand, constructed a KG by first extracting knowledge triples from available data and then using these to construct a network for healthcare professionals that allows them to traverse this contextualized knowledge. Tao et al. [ 12 ] developed a web-based system called Interactive Mapping Interface (IMI) to first map the data dictionary in use by the North American Association of Central Cancer Registries (NAACCR) to the NCIt with the final goal of facilitating the dissemination and reuse of North American cancer registries data. Chen et al. [ 73 ] established a consensus knowledge for cancer hallmarks using functional annotations and gene set overlap, again aiming towards enabling the ability to compare data from different sources.
5.1.3. Database Interfaces
One application reported in the articles lies on ontology-based annotations to create user interfaces for databases, where labels of ontology classes and relations allow text annotation. These interfaces are notably useful in dealing with medical data, for integration and querying of different knowledge resources.
Works within this category that have already been mentioned before are Myneni et al. [ 34 ] and Esteban-Gil et al. [ 39 ] from data annotation, and Milian et al. [ 66 ], Hasan et al. [ 71 ], and Tao et al. [ 12 ] from data integration. Sesen et al. [ 51 ] used a lung ontology with the clinical decision support application Lung Cancer Assistant to categorize patients and produce treatment recommendations. González-Beltrán et al. [ 74 ] aimed to ease queries over cancer research data, by extending an existing tool, caGrid [ 75 ], with additional services, its domain metadata consisting of ontology-based annotations associated with the structural information of each incorporated data source. In lung cancer, circ2GO is a database developed by Lyu et al. [ 76 ] that holds information about the functional annotation of circular RNAs by integrating GO information for all genes in their dataset.
5.1.4. Natural Language Processing
Natural Language Processing (NLP) is also a field that can benefit from the use of a standardized organization of knowledge and terms. The works by Milian et al. [ 66 ] and Yan et al. [ 13 ] have been mentioned in previous sub-categories. In the case of Tapi Nzali et al. [ 42 ], the goal was to use their own french CHV of non-experts’ expressions for breast cancer and compare them to biomedical terms used by health care professionals. Directed toward a social scope, Lee et al. [ 43 ] created an ontology from a social media crawler and NLP, to evaluate social media data and understand information needs and emotions related to cancer.

5.2. Semantic-Focused Applications
5.2.1. formalized definitions and axioms: reasoning with ontologies.
In the works collected, reasoning is applied to the inference of new knowledge from ontologies or error detection is also reported, as summarized in Table 3 . The most common way to access and use reasoners in the reviewed papers consisted of using Protégé, an ontology editor, while creating or editing ontologies, due to ease of access [ 80 ].
There are works that use reasoners to infer new knowledge from semantically annotated data and/or established rules. Alfonse et al. [ 81 ] used FaCT++ to determine the type and stage of a patient’s cancer in order to recommend treatments. Zhu et al. [ 15 ] used a rule-based Description Logic (DL) unnamed OWL reasoner to infer additional associations in pathways, drugs, genes and diseases for 18 breast cancer drugs from the ontological representation of the PharmGKB pathway data file. Moreover, using the same ontological representation of PharmGKB, Tao et al. [ 82 ] used Pellet to predict new targets for therapy development. Mahmoodi et al. [ 49 ] derived association rules from the GCO and patient data using a modified version of an Apriori algorithm, to establish system-wide associations between events in text through large-scale text mining. Barki et al. [ 52 ] predicted side effects of treatments for bladder cancer with Pellet. Nicholson et al. [ 83 ] used reasoners to signal rule violations in the validation of international rules for multiple primary tumors.
Reasoners can also be used to detect errors in the ontologies or models that have been built. Works by Barki et al. [ 52 ], and Nicholson et al. [ 30 , 83 ] were described above. Herrmann et al. [ 38 ] aimed at providing a generalizing pattern to classify tumors. Boeker et al. [ 46 ] used HermIT DL in their TNM Ontology to evaluate its soundness. Oyelade et al. [ 14 ] focused on addressing the issue of vagueness in breast cancer ontology (BCO).
5.2.2. Mining and Analyzing Multimodal Data with Ontologies
By far the majority of the works reviewed, fall into the category of mining and analyzing, as can be partially observed by Table 4 and the additional 72 gene set enrichment articles not present in it that belong to this category. The use of ontologies in cancer research has undoubtedly opened a new avenue in data analysis, where different methodologies (or combinations of) are used to achieve the most varied goals to derive meaning from large quantities of data.
One of the applications reported in data analysis and mining is semantic filtering [ 84 ]. The annotation of data with its semantic concepts enables the use of those same concepts to filter data. Chen et al. [ 85 ] used biomedical ontologies to guide a set of sequential filtering steps with the objective of predicting microRNAs related to the regulation of glucocorticoid resistance in the specific case of pediatric acute lymphoblastic leukemia (ALL). In another case, users can use the Semantic Web platform developed by Esteban-Gil et al. [ 39 ] to run semantic queries over the annotated data and visualize the results in different ways.
An additional use is similarity measuring [ 86 ], where the distance between items is measured by the overlap in meaning, to discern what concepts (and therefore their data) are closer or further apart. For example, Modules and Gene Ontology-based Gene Prioritization, developed by Su et al. [ 17 ], uses fuzzy similarity for cancer-related gene prioritization.
One of the main approaches used to analyze large amounts of biomedical data is the employment of ML techniques on data that has been semantically annotated. With the evolution of AI algorithms, researchers have been increasingly able to pose more complex questions and use various methodologies to obtain their answers, which is easily observed from the variety of methods and objectives in the articles reviewed. UMVMO-select is a Unsupervised Multi-View Multi-Objective clustering-based gene selection approach developed by Acharya et al. [ 87 ] that uses functional annotation to identify gene markers. Su et al. [ 88 ] used an ML method over functionally annotated genetic information to look into the immunofunctionomes of ovarian clear cell carcinoma (OCCC). Chen et al. [ 64 ] predicted drug synergy using a deep belief network over genetic expression and an ontological profile of genes built from literature (Ontology Fingerprints). For clinical decision support, Shen et al. [ 19 ] outlined an architecture that combines Case-Based Reasoning (CBR) with a Multi-Agent System (MAS) to provide treatment suggestions. [ 77 ] used the Multi-threaded Clinical Vocabulary Server (MCVS) NLP engine to mine data related to genetic markers from the New England Journal of Medicine (NEJM), with the aim of further supporting the role of inflammation in cancer. To predict drug targets, Tao et al. [ 82 ] used a combination of ontology reasoning with network-assisted gene ranking over an ontology that represents PharmGKB data. Althubaiti et al. [ 18 ] used neuro-symbolic feature learning over several ontologies to predict cancer driver genes. Deep GONet, developed by Bourgeais et al. [ 89 ], is a self-explainable deep learning model where each biological function is represented by a neuron, that can be used to predict phenotypes. Gao et al. [ 50 ] obtained drug inference results from a treatment-based cancer ontology obtained by Bayesian derivation. Comparing the same method with and without ontologies, Min et al. [ 90 ] used a rule learning system to predict patients’ ability to perform activities of daily living. Furthermore, to predict cervical cancer cells from cytological tissue images, Divakar et al. [ 58 ] used deep neural networks (DNN) on their developed ontology. Salvi et al. [ 11 ] used a variety of classifiers—Bayesian networks, artificial neural networks (ANN), support vector machines (SVMs), decision trees and random forests—in a data analysis model of their NeoMark system that holds its own semantic model. By comparing several different models, Yan et al. [ 13 ] reached an approach that outperforms the others that uses ontological features with a combined use of United Decision Trees and Random Forest algorithms. González-Beltránet al. [ 74 ] developed a system for ontology-based queries over the caGrid infrastructure than can be reused with other service-oriented and model-driven infrastructures. Xi et al. [ 91 ] leverages KG embeddings for tolerating missing data from breast cancer clinical ultrasound reports. Using graph attention networks (GAT), Zhang et al. [ 92 ] developed a method for real-time inference on a lung KG, using a new ontology.
However, in the end, the most common approach to the use of ontologies in the analysis of biomedical data was the application of GO in Gene Set Enrichment Analysis (GSEA) [ 16 , 53 , 73 , 93 , 94 , 95 , 96 , 97 , 98 , 99 , 100 , 101 , 102 , 103 , 104 , 105 , 106 , 107 , 108 , 109 , 110 , 111 , 112 , 113 , 114 , 115 , 116 , 117 , 118 , 119 , 120 , 121 , 122 , 123 , 124 , 125 , 126 , 127 , 128 , 129 , 130 , 131 , 132 , 133 , 134 , 135 , 136 , 137 , 138 , 139 , 140 , 141 , 142 , 143 , 144 , 145 , 146 , 147 , 148 , 149 , 150 , 151 , 152 , 153 , 154 , 155 , 156 , 157 , 158 , 159 ]. GSEA statistically compares set of genes that share biological characteristics and interprets their expression data in light of on whether they differ across defined phenotypes [ 160 ] and as such is commonly used in biomedical research to, for example, establish candidate genes for further studies.
Of the 141 papers selected in this systematic review, 72 employed gene set enrichment in some manner. Of these, 21 only used GO, and 48 used it in conjunction with other resources, of which Kyoto Encyclopedia of Genes and Genomes (KEGG) pathway database was more common with 45 articles, followed by REACTOME pathway database with 3. Of this application, we have the example of Tian et al. [ 131 ] that profiled the transcriptome of gastric cancer patients and used the enrichment to confirm the annotation of genes with digestive system process, secretion and digestion. She et al. [ 109 ] used GO and KEGG in an enrichment analysis with the overall objective of finding the importance of C reactive protein and its interactors in HCC. Moreover, developing research in the same cancer, Agioutantis et al. [ 16 ] also used enrichment with both GO and REACTOME in their pursuit of deciphering molecular heterogeneity and drug responsiveness by exploring the molecular diversity of tumors and drug sensitivity. No table is provided for this type of use since the methodology is standardized.
6. Conclusions
Over the last two decades, ontologies gained traction in biomedical research in general, and cancer research in particular, enabling FAIR data (findability, accessibility, interoperability and reusability) [ 162 ], supporting data integration and analysis, and facilitating data interpretation and data mining. Presently, we are witnessing the emergence of the knowledge graph paradigm, whereby large volumes of heterogeneous data are brought together under a single holistic ontological knowledge model. Yet, there are still a number of open challenges to the development and application of ontologies and knowledge graphs for cancer research.
One major challenge lies in reusing existing ontologies. With over 800 biomedical ontologies publicly available in BioPortal [ 3 ], most biomedical subjects are covered by one or more ontologies, and it might seem foolish not to reuse them. However, the fact that there are so many ontologies and many overlap in domain makes it difficult to navigate the ontology landscape and select which ones to reuse. Moreover, many ontologies were typically developed with a singular purpose in mind, and have a particular perspective on the domain they model which may be unsuited for other purposes. This means that additional care is needed when selecting ontologies to reuse, to make sure that their perspective on the domain is compatible with the new use case. Last but not least, it may be the case that existing ontologies are no longer actively maintained and kept up to date, which in a dynamic domain like biomedicine, will render them useless in a short time span. Ultimately, it may very well be that no existing ontology is compatible with or usable in the new use case, and that a new ontology must be developed, which indeed is the main reason why there are presently so many ontologies. Thus, to avoid perpetuating the problem, new ontologies should be designed circumspectly, taking into account possible other applications within their specific domain [ 30 ].
Another challenge lies in the disconnection between data and ontologies, due to the fact that, in the large majority of cases, biomedical ontologies do not include data. In fact, few biomedical ontologies were designed with the prospect of directly encoding data, as the biomedical research community has, for the most part, viewed ontologies merely as abstract knowledge models used for classification or at best annotation of data, with the data kept in relational databases or even data files. This is tied to the reusability challenge, as existing ontologies may not be reusable for use cases such as constructing knowledge graphs if they are unsuited to being instantiated. Furthermore, it means that constructing biomedical knowledge graphs to support cancer research requires (semi-)automated approaches to integrating the data with the knowledge model, which, considering the variety and heterogeneity of relevant biomedical data sources, can be burdensome [ 163 ]. However, as the knowledge graph paradigm becomes more popular, we may witness a shift in the biomedical community towards storing data in graph databases rather than relational databases.
Tied to the two previous challenges is the challenge of integrating multiple ontologies, a necessity for constructing holistic knowledge graphs for cancer research, due to the multidisciplinarity of the domain. Although there are comprehensive ontologies on cancer (e.g., NCIt), available data is often connected to more specialized ontologies (e.g., GO, MeSH), eliciting the need to integrate them. The problem is that, due to their different perspectives, overlapping ontologies may be semantically irreconcilable [ 164 ], which may impede their joint use. Thus, the costs of reusing existing ontologies may outweigh their benefits, prompting the development of an independent ontological knowledge model for a knowledge graph, ideally with mappings to existing ontologies to ensure interoperability and facilitate data integration.
The benefits of developing holistic knowledge graphs that integrate all the data relevant for cancer research are deeply tied to the potential of AI approaches to unlock knowledge conducive to better diagnostics or treatments. Knowledge graphs can serve as sources of background knowledge to AI approaches, compensating for missing values in the data, they can support image classification and NLP approaches to enrich image or textual data, which in turn can improve the performance of AI approaches relying on that data, and they provide a means to afford explainability to AI approaches [ 165 ], tackling the black-box problem of state-of-the-art AI methods.
The immense potential of ontologies and the knowledge graph paradigm to support cancer research data management and analysis is increasingly recognized by the oncology research community as an essential building block of the P4 medicine vision (preventative, predictive, personalized and participatory).
Abbreviations
The following abbreviations are used in this manuscript:
Author Contributions
Formal analysis, data curation and writing—original draft preparation, M.C.S. and P.E.; conceptualization, methodology and writing—reviewing and editing, D.F. and C.P. All authors have read and agreed to the published version of the manuscript.
This work was supported by FCT through the LASIGE Research Unit (UIDB/00408/2020 and UIDP/00408/2020). It was also partially supported by the KATY project which has received funding from the European Union’s Horizon 2020 research and innovation program under grant agreement No 101017453.
Institutional Review Board Statement
Informed consent statement, data availability statement, conflicts of interest.
The authors declare no conflict of interest.
Publisher’s Note: MDPI stays neutral with regard to jurisdictional claims in published maps and institutional affiliations.

Current Materials Science
Editor-in-Chief : Ram Gupta Department of Chemistry Pittsburg State University Pittsburg KS USA
ISSN (Print): 2666-1454 ISSN (Online): 2666-1462
A Comprehensive Overview of Ontology: Fundamental and Research Directions
- School of Law, Forensic Justice, and Policy Studies, National Forensic Sciences University, Gandhinagar, Gujarat, India
- Department of Software Engineering, Eastern International University, Binh Duong, Vietnam
Volume 17, Issue 1, 2024
Published on: 20 October, 2022
Page: [2 - 20] Pages: 19
DOI: 10.2174/2666145415666220914114301
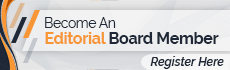
Knowledge representation and reasoning is a field of ‘Artificial Intelligence’ that encodes knowledge, beliefs, actions, feelings, goals, desires, preferences, and all other mental states in the machine. An ontology is prominently used to represent knowledge and offers the richest machine-interpretable (rather than just machine-processable) and explicit semantics. Ontology does not only provide sharable and reusable knowledge, but it also provides a common understanding of the knowledge; as a result, the interoperability and interconnectedness of the model make it priceless for addressing the issues of querying data. Ontology work with concepts and relations that are very close to the working of the human brain. Ontological engineering provides the methods and methodologies for the development of ontology. Nowadays, ontologies are used in almost every field, and a lot of much research is being done on this topic. The paper aims to elaborate on the need of ontology (from data to knowledge), how does for ontology (from data to knowledge), how semantics come from logic, the ontological engineering field, history from hypertext to linked data, and further possible research directions of the ontology. This paper benefit reader who wishes to embark on ontology-based research and application development.
Keywords: Ontology , semantic , logic , OWL , knowledge , linked data.
Graphical Abstract

Title: A Comprehensive Overview of Ontology: Fundamental and Research Directions
Volume: 17 Issue: 1
Author(s): Archana Patel*Narayan C. Debnath
Abstract: Knowledge representation and reasoning is a field of ‘Artificial Intelligence’ that encodes knowledge, beliefs, actions, feelings, goals, desires, preferences, and all other mental states in the machine. An ontology is prominently used to represent knowledge and offers the richest machine-interpretable (rather than just machine-processable) and explicit semantics. Ontology does not only provide sharable and reusable knowledge, but it also provides a common understanding of the knowledge; as a result, the interoperability and interconnectedness of the model make it priceless for addressing the issues of querying data. Ontology work with concepts and relations that are very close to the working of the human brain. Ontological engineering provides the methods and methodologies for the development of ontology. Nowadays, ontologies are used in almost every field, and a lot of much research is being done on this topic. The paper aims to elaborate on the need of ontology (from data to knowledge), how does for ontology (from data to knowledge), how semantics come from logic, the ontological engineering field, history from hypertext to linked data, and further possible research directions of the ontology. This paper benefit reader who wishes to embark on ontology-based research and application development.
Export Options
About this article.
Cite this article as:
Patel Archana*, Debnath C. Narayan, A Comprehensive Overview of Ontology: Fundamental and Research Directions, Current Materials Science 2024; 17 (1) . https://dx.doi.org/10.2174/2666145415666220914114301
Call for Papers in Thematic Issues
Trends in modeling and characterization of advanced materials: tools and techniques.
Over the last decade, there is exponential growth in the function, dynamics and design of advanced materials and thus sustain enhanced functional and structural properties such as electrical, biological, thermal, magnetic, mechanical, optical at various scales. Materials modeling and characterization provide significant information on design principles and methods for fabrication ... read more
Related Journals
Current Catalysis
Current Nanomaterials
Journal of Photocatalysis
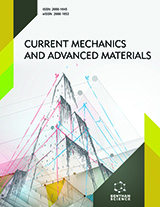
Current Mechanics and Advanced Materials

Current Applied Materials
Related Books

Metal Matrix Composites: A Modern Approach to Manufacturing
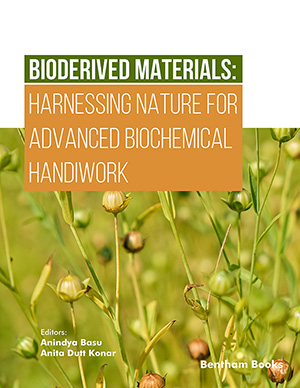
Bioderived Materials: Harnessing Nature for Advanced Biochemical Handiwork
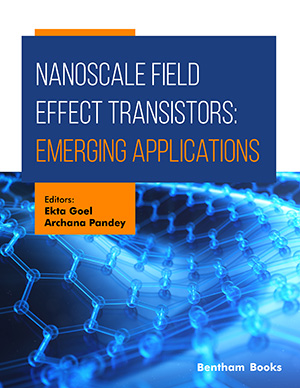
Nanoscale Field Effect Transistors: Emerging Applications

Biocarbon Polymer Composites
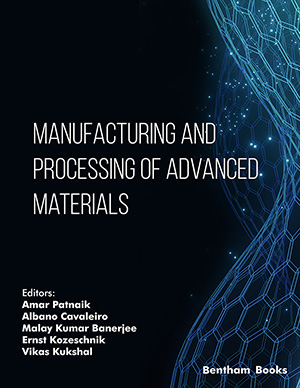
Manufacturing and Processing of Advanced Materials
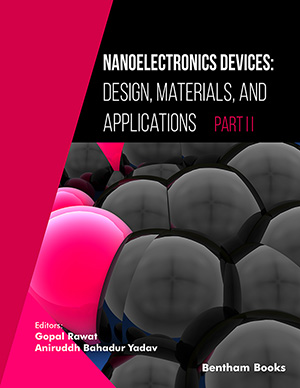
Nanoelectronics Devices: Design, Materials, and Applications Part II

Nanoelectronics Devices: Design, Materials, and Applications (Part I)
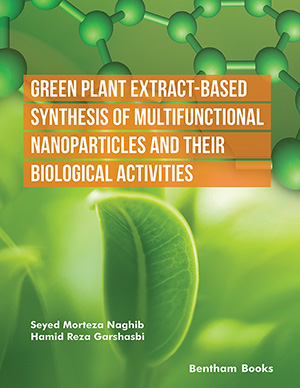
Green Plant Extract-Based Synthesis of Multifunctional Nanoparticles and their Biological Activities

Industrial Applications of Polymer Composites
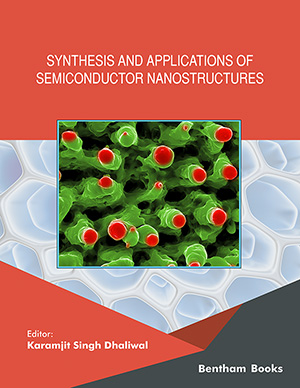
Synthesis and Applications of Semiconductor Nanostructures

- About Journal
- Editorial Board
- Current Issue
- Volumes /Issues
- Author Guidelines
- Graphical Abstracts
- Fabricating and Stating False Information
- Research Misconduct
- Post Publication Discussions and Corrections
- Publishing Ethics and Rectitude
- Increase Visibility of Your Article
- Archiving Policies
- Peer Review Workflow
- Order Your Article Before Print
- Promote Your Article
- Manuscript Transfer Facility
- Editorial Policies
- Allegations from Whistleblowers
- Forthcoming Thematic Issues
- Guest Editor Guidelines
- Editorial Management
- Ethical Guidelines for New Editors
- Reviewer Guidelines
- Abstract Ahead of Print 4
- Article(s) in Press 65
- Free Online Copy
- Most Cited Articles
- Most Accessed Articles
- Highlighted Article
- Most Popular Articles
- Editor's Choice
- Thematic Issues
- Open Access Articles
- Open Access Funding
- Library Recommendation
- Trial Requests
- Advertise With Us
- Meet the Executive Guest Editor(s)
- Brand Ambassador
- Author's Comment & Reviews
- New Journals 2023
- New Journals 2024
- Alert Subscription
Restricted Access Panel
Thank you for visiting nature.com. You are using a browser version with limited support for CSS. To obtain the best experience, we recommend you use a more up to date browser (or turn off compatibility mode in Internet Explorer). In the meantime, to ensure continued support, we are displaying the site without styles and JavaScript.
- View all journals
Gene ontology articles from across Nature Portfolio
The Gene Ontology (GO) project is a bioinformatics initiative that provides an ontology (shared vocabulary) of defined terms to represent specific gene product properties. The use of controlled terms from the GO means that computers can be used to analyse relationships between gene products, which in turn can reveal previously unknown functions.
Latest Research and Reviews
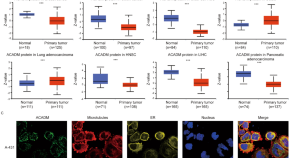
Low ACADM expression predicts poor prognosis and suppressive tumor microenvironment in clear cell renal cell carcinoma
- Huimin Long
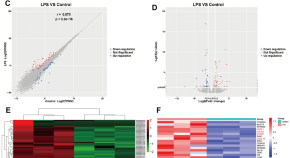
Insights from bioinformatics analysis reveal that lipopolysaccharide induces activation of chemokine-related signaling pathways in human nasal epithelial cells
- Shaolin Tan
- Weitian Zhang
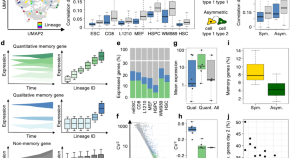
Gene-expression memory-based prediction of cell lineages from scRNA-seq datasets
Combining experimental lineage tracing with single cell transcriptomics is technically demanding. Here, authors present GEMLI, a computational tool to annotate cell lineages in single cell RNA sequencing data solely based on gene expression.
- A. S. Eisele
- D. M. Suter
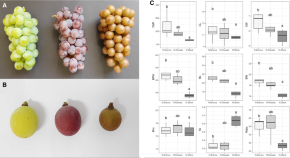
Uncovering the molecular mechanisms of russet skin formation in Niagara grapevine ( Vitis vinifera × Vitis labrusca )
- Guilherme Francio Niederauer
- Geovani Luciano de Oliveira
- Anete Pereira de Souza
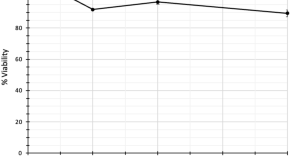
Transcriptomic screening of novel targets of sericin in human hepatocellular carcinoma cells
- Jiraporn Jantaravinid
- Napatara Tirawanchai
- Pornanong Aramwit
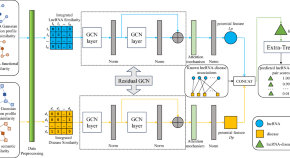
Prediction of lncRNA and disease associations based on residual graph convolutional networks with attention mechanism
- Shengchang Wang
- Jiaqing Qiao
News and Comment
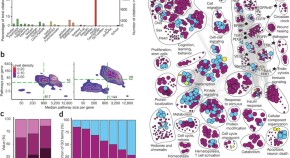
Impact of outdated gene annotations on pathway enrichment analysis
- Jüri Reimand
miRWalk2.0: a comprehensive atlas of microRNA-target interactions
- Harsh Dweep
- Norbert Gretz
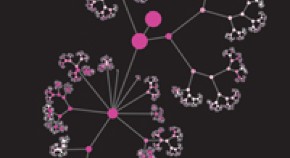
A next-gen ontology
An approach to cluster and organize systems biology data yields NeXO, a data-driven ontology.
- Natalie de Souza
Automating the construction of gene ontologies
Manual curation of biological ontologies is recapitulated by an algorithmic approach, supplementing the Gene Ontology and enabling the discovery of relationships among genes and proteins.
- Kara Dolinski
- David Botstein
Ontology engineering
- Gil Alterovitz
- Michael Xiang
- Marco F Ramoni
Quick links
- Explore articles by subject
- Guide to authors
- Editorial policies

A Comprehensive Overview of Ontology: Fundamental and Research Directions
Archana Patel , N. Debnath
Sep 14, 2022
Influential Citations
Current Materials Science
Key Takeaway : Ontology is a key tool in AI that represents knowledge, provides sharable, reusable knowledge, and enhances data querying by providing common understanding and interoperability.
Knowledge representation and reasoning is a field of ‘Artificial Intelligence’ that encodes knowledge, beliefs, actions, feelings, goals, desires, preferences, and all other mental states in the machine. An ontology is prominently used to represent knowledge and offers the richest machine-interpretable (rather than just machine-processable) and explicit semantics. Ontology does not only provide sharable and reusable knowledge, but it also provides a common understanding of the knowledge; as a result, the interoperability and interconnectedness of the model make it priceless for addressing the issues of querying data. Ontology work with concepts and relations that are very close to the working of the human brain. Ontological engineering provides the methods and methodologies for the development of ontology. Nowadays, ontologies are used in almost every field, and a lot of research is being done on this topic. The paper aims to elaborate the need of ontology (from data to knowledge), how does semantics come from logic, the ontological engineering field, history from hypertext to linked data, and further possible research directions of the ontology. This paper benefit reader who wishes to embark on ontology-based research and application development.

Integration and Implementation Insights
A community blog and repository of resources for improving research impact on complex real-world problems
A guide to ontology, epistemology, and philosophical perspectives for interdisciplinary researchers
By Katie Moon and Deborah Blackman

How can understanding philosophy improve our research? How can an understanding of what frames our research influence our choices? Do researchers’ personal thoughts and beliefs shape research design, outcomes and interpretation?
These questions are all important for social science research. Here we present a philosophical guide for scientists to assist in the production of effective social science (adapted from Moon and Blackman, 2014).

Understanding philosophy is important because social science research can only be meaningfully interpreted when there is clarity about the decisions that were taken that affect the research outcomes. Some of these decisions are based, not always knowingly, on some key philosophical principles, as outlined in the figure below.
Philosophy provides the general principles of theoretical thinking, a method of cognition, perspective and self-awareness, all of which are used to obtain knowledge of reality and to design, conduct, analyse and interpret research and its outcomes. The figure below shows three main branches of philosophy that are important in the sciences and serves to illustrate the differences between them.

(Source: Moon and Blackman 2014)
The first branch is ontology, or the ‘study of being’, which is concerned with what actually exists in the world about which humans can acquire knowledge. Ontology helps researchers recognize how certain they can be about the nature and existence of objects they are researching. For instance, what ‘truth claims’ can a researcher make about reality? Who decides the legitimacy of what is ‘real’? How do researchers deal with different and conflicting ideas of reality?
To illustrate, realist ontology relates to the existence of one single reality which can be studied, understood and experienced as a ‘truth’; a real world exists independent of human experience. Meanwhile, relativist ontology is based on the philosophy that reality is constructed within the human mind, such that no one ‘true’ reality exists. Instead, reality is ‘relative’ according to how individuals experience it at any given time and place.
Epistemology
The second branch is epistemology, the ‘study of knowledge’. Epistemology is concerned with all aspects of the validity, scope and methods of acquiring knowledge, such as a) what constitutes a knowledge claim; b) how can knowledge be acquired or produced; and c) how the extent of its transferability can be assessed. Epistemology is important because it influences how researchers frame their research in their attempts to discover knowledge.
By looking at the relationship between a subject and an object we can explore the idea of epistemology and how it influences research design. Objectivist epistemology assumes that reality exists outside, or independently, of the individual mind. Objectivist research is useful in providing reliability (consistency of results obtained) and external validity (applicability of the results to other contexts).
Constructionist epistemology rejects the idea that objective ‘truth’ exists and is waiting to be discovered. Instead, ‘truth’, or meaning, arises in and out of our engagement with the realities in our world. That is, a ‘real world’ does not preexist independently of human activity or symbolic language. The value of constructionist research is in generating contextual understandings of a defined topic or problem.
Subjectivist epistemology relates to the idea that reality can be expressed in a range of symbol and language systems, and is stretched and shaped to fit the purposes of individuals such that people impose meaning on the world and interpret it in a way that makes sense to them. For example, a scuba diver might interpret a shadow in the water according to whether they were alerted to a shark in the area (the shark), waiting for a boat (the boat), or expecting a change in the weather (clouds). The value of subjectivist research is in revealing how an individual’s experience shapes their perception of the world.
Philosophical perspectives
Stemming from ontology (what exists for people to know about) and epistemology (how knowledge is created and what is possible to know) are philosophical perspectives, a system of generalized views of the world, which form beliefs that guide action.
Philosophical perspectives are important because, when made explicit, they reveal the assumptions that researchers are making about their research, leading to choices that are applied to the purpose, design, methodology and methods of the research, as well as to data analysis and interpretation. At the most basic level, the mere choice of what to study in the sciences imposes values on one’s subject.
Understanding the philosophical basis of science is critical in ensuring that research outcomes are appropriately and meaningfully interpreted. With an increase in interdisciplinary research, an examination of the points of difference and intersection between the philosophical approaches can generate critical reflection and debate about what we can know, what we can learn and how this knowledge can affect the conduct of science and the consequent decisions and actions.
How does your philosophical standpoint affect your research? What are your experiences of clashing philosophical perspectives in interdisciplinary research? How did you become aware of them and resolve them? Do you think that researchers need to recognize different philosophies in interdisciplinary research teams?
To find out more : Moon, K., and Blackman, D. (2014). A Guide to Understanding Social Science Research for Natural Scientists. Conservation Biology , 28 : 1167-1177. Online: http://onlinelibrary.wiley.com/doi/10.1111/cobi.12326/full
Biography: Katie Moon is a Post Doctoral Research Fellow at the University of New South Wales, Canberra. She is also an adjunct at the Institute for Applied Ecology at the University of Canberra. She has worked in the environmental policy arena for 17 years within Australia and Europe, in government, the private sector and academia. Her research focuses on how the right policy instruments can be paired to the right people; the role of evidence in policy development and implementation; and how to increase policy implementation success .
Biography: Deborah Blackman is a Professor in Public Sector Management Strategy and Deputy Director of the Public Service Research Group at the University of New South Wales, Canberra. She researches knowledge transfer in a range of applied, real world contexts. The common theme of her work is creating new organisational conversations in order to improve organisational effectiveness. This has included strengthening the performance management framework in the Australian Public Service; the role of social capital in long-term disaster recovery; and developing a new diagnostic model to support effective joined-up working in whole of government initiatives .
Related posts:
A guide for interdisciplinary researchers: Adding axiology alongside ontology and epistemology by Peter Deane https://i2insights.org/2018/05/22/axiology-and-interdisciplinarity/
Epistemological obstacles to interdisciplinary research by Evelyn Brister https://i2insights.org/2017/10/31/epistemology-and-interdisciplinarity/
Transforming transdisciplinarity: Interweaving the philosophical with the pragmatic to move beyond either/or thinking by Katie Ross and Cynthia Mitchell https://i2insights.org/2018/11/13/transdisciplinarity-and-either-or-thinking/
What is the role of theory in transdisciplinary research? by Workshop Group on Theory at 2015 Basel International Transdisciplinary Conference http://i2insights.org/2016/02/17/role-of-theory-in-transdisciplinary-research/
Share this:
13 thoughts on “a guide to ontology, epistemology, and philosophical perspectives for interdisciplinary researchers”.
Hi Katie and Deborah, First of all want to thank you for such incredible synthesis! Then I want to ask you, how can we situate a paradigm or an school or though in this map? For example, where do you think we can situate the complex paradigm of Edgar Morin? in between the relativistic ontology? or critical theory? thanks in advance.
- Pingback: creative drift: on being natural - bob's thoughts, images, feelings
The table summary is admirable. All your write is very nice
- Pingback: A guide to ontology, epistemology, and philosophical perspectives for interdisciplinary researchers | Learning Research Methods
- Pingback: Week 2 – Ontology and Epistemology – Research Methods
Great post! I really like the table and find it a very helpful illustration!
Hi Kate, thank you very much for helping out. I understand the subject matter more now than before Olushola
- Pingback: RES 701, Week 2, Ontology & Epistemology – RES701 Research Methods
Thanks so much for the debate and discussion around the blog post. Machiel is right in pointing out that the blog post (and the article it is based on) was intended as a conversation piece, and we’re pleased that a useful conversation is taking place. The resources and links are very helpful, philosophy is a fascinating discipline and the opportunity to learn and expand our thinking is endless.
We tried to make it clear in the article the blog post is based on that we wanted to bring attention to philosophy; it was obviously impossible to do the discipline of philosophy any real justice within 6,000 words. We wanted to start a conversation: “The purpose of the guide is to open the door to social science research and thus demonstrate that scientists can bring different and legitimate principles, assumptions, and interpretations to their research.”
As Jessica and Melissa point out, it can be challenging to offer social research to a natural science community that typically adopts a narrow philosophical position (e.g. objectivist). The paper was intended to encourage natural scientists to consider alternative ways of generating knowledge, particularly about the human, as opposed to natural, world.
We accept unequivocally that the framework does not get close to accommodating the depth and diversity of philosophy. Adam, we agree that the approach we have taken may not resonate with some philosophers, but we wanted to communicate with a particular audience (conservation scientists) and so we defined ontologies and epistemologies (and posited them relative to one another) that are most commonly observed within this discipline and that might be best understood by the audience. We tried to identify points of difference between ontologies, epistemologies and philosophical perspectives in an attempt to explain how they can influence research design. In the article, we use a case of deforestation in rainforests to demonstrate how different positions can influence the nature of the research questions and outcomes, including the assumptions that will be made.
We did explain in the introduction to our paper the limitations of our approach: “The multifaceted nature and interpretation of each of the concepts we present in our guide means they can be combined in a diversity of ways (see also Lincoln & Guba 2000; Schwandt 2000; Evely et al. 2008; H¨oijer 2008; Cunliffe 2011; Tang 2011). Therefore, our guide represents just one example of how the elements (i.e., different positions within the main branches of philosophy) of social research can apply specifically to conservation science. We recognize that by distilling and defining the elements in a simplified way we have necessarily constrained argument and debate surrounding each element. Furthermore, the guide had to have some structure. In forming this structure, we do not suggest that researchers must consider first their ontological and then their epistemological position and so on; they may well begin by exploring their philosophical perspective.”
This point comes back to Bruce’s comment, about pragmatic approaches to research. Often researchers pick and choose between a range of options that will allow them to define and answer their research questions in a way that makes most sense to them. We make this point in the paper: “Each perspective is characterized by an often wide ranging pluralism, which reflects the complex evolution of philosophy and the varied contributions of philosophers through time (Crotty 1998). All ontologies, epistemologies, and philosophical perspectives are characterized by this pluralism, including the prevailing (post) positivist approach of the natural sciences. It is common for more than one philosophical perspective to resonate with researchers and for researchers to change their perspective (and thus epistemological and ontological positions) toward their research over time (Moses & Knutsen 2012). Thus, scientists do not necessarily commit to one philosophical perspective and all associated characteristics (Bietsa 2010).”
We tried to anticipate concerns that scholars of philosophy might have with our rather reductionist approach, but felt that the more important contribution to make was to bring attention to alternative worldviews, and highlight the importance of philosophy in generating any type of knowledge.
With respect to the characterization of epistemologies, we adopted a continuum provided by Crotty (1998) that focuses on the relationship between the subject and the object. Again, this choice was made on the basis of our audience, to demonstrate that different types of relationship can exist between subject and object
This blog post has generated an interesting discussion on the Association for Interdisciplinary Studies listserv ([email protected]). Selected excerpts below.
Adam Potthast: I hate to make one of my first posts to this list critical without the time to correct some of the errors, but I don’t think you’d see many philosophers agreeing with the characterizations of philosophical views in this post. The infographic strongly mischaracterizes a lot of these positions, and the section on epistemology doesn’t map on to any of the standard understandings of epistemology in the discipline of philosophy. I’d caution against thinking of it as a reliable source to the philosophy behind science.
Gabriele Bammer: Thanks Adam for raising the alarm. It would be great if you and/or others who have problems with this post would spell out your criticisms – not only via this listserv, but (more importantly from my perspective) in a comment on the blog itself. Non-philosophers are hungry for a version of epistemology, ontology etc that they can understand and use and this blog post (and the paper it is based on) address this need. If it is seriously misleading though, that’s obviously a problem. It’s important that this is pointed out and that better alternatives are offered. I appreciate that time is an issue for everyone – anything you can do will be appreciated.
Stuart Henry: Well a good start, so we don’t reinvent the wheel again is James Welch’s article: https://oakland.edu/Assets/upload/docs/AIS/Issues-in-Interdisciplinary-Studies/2009-Volume-27/05_Vol_27_pp_35_69_Interdisciplinarity_and_the_History_of_Western_Epistemology_(James_Welch_IV) .pdf
Gabriele Bammer: Thanks Stuart, I may be missing something, but it seems to me that Welch’s article covers different terrain, being more about the philosophy underpinning interdisciplinarity. What Moon and Blackman provide is a quick guide to understanding people’s different philosophical positions, so that if you are working in a team, for example, you can better understand why someone sees the world differently. The Toolbox developed by Eigenbrode, O’Rourke and others provides a practical way of uncovering these differences.
Julie Thompson Klein: Good point Gabriele about the value of the Toolbox, though people still need the kind of background you’re aiming to provide.
Machiel Keestra: Although I agree that the blog post should perhaps not so much be taken to offer a current representation of the main positions in philosophy of science or about the interconnections between epistemological and ontological positions, I think it does a nice job in offering a conversation piece: what are relevant positions and options that people might -implicitly– take and how are they different from other positions. Given the modest ambitions of the authors, I think that is a fair result.
In addition to the interesting approach offered by the Toolbox Project, an alternative is presented in Jan Schmidt’s Towards a philosophy of interdisciplinarity: http://link.springer.com/article/10.1007%2Fs10202-007-0037-8 In our Introduction to interdisciplinary research, I’ve inserted an all-too brief philosophy of science which should help to raise some understanding of this difficult issue as well: https://www.academia.edu/22420234/An_Introduction_to_Interdisciplinary_Research._Theory_and_Practice
Lovely work! Thank you. I am also initially trained as a natural scientist, and now consider myself a ‘social-ecological researcher’ and have had to do a lot of learning about ontologies, epistemologies etc. I think I might use this paper as a discussion paper in our department as I think it is crucial for interdisciplinarians to understand these issues.
Kia ora Katie and Debbie, great post! I am a biophysical scientist who has come to social science and one of the struggles is being able to place the new and relevant concepts about questions that we don’t necessarily ask as biophysical scientists. Your table is a really useful aid to this – I immediately sent it to all my colleagues! It also makes it clearer to me how I can use the concept of triangulation that Bruce alluded to in his reply. So thank you for explaining so concisely. Thanks, Melissa
Hi Katie and Deborah,
Thank you for that discussion. I think that you have created a really useful table showing the philosophical continuums/polarities, how the various ontological and epistemological positions relate to each other, and the importance for researchers to be aware of them. In my own research practice, I am not committed to any one particular philosophical theory or perspective. They all appear to be true to some degree, that is, in some conceivable context – even though some of the concepts and philosophical positions appear, in the extreme form of their statement, to be contradictory, that is, if one end of a continuum/polarity is true then by implication it seems the other must be false – thus creating a quandary of research perspective. Hence the attraction, for me, of the application of a multiplicity of methods, approaches and philosophical perspectives – as and when they seem able to give ontological or epistemological insight – with triangulation between the results of the disparate approaches as the temporary arbiter of an evolving meaning and truth. This might be considered a pragmatic, perhaps even an opportunistic, approach to conducting science. However, as the old adage goes “the proof is in the pudding” – how useful is the knowledge obtained?
cheers Bruce
Leave a Reply Cancel reply
This site uses Akismet to reduce spam. Learn how your comment data is processed .
Discover more from Integration and Implementation Insights
Subscribe now to keep reading and get access to the full archive.
Type your email…
Continue reading
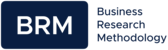
Ontology and epistemology are two different ways of viewing the research philosophy . Ontology in business research can be defined as “the science or study of being” [1] and it deals with the nature of reality. Ontology is a system of belief that reflects an interpretation of an individual about what constitutes a fact. In simple terms, ontology is associated with what we consider as reality.
Table 1 below illustrates main questions that key philosophical concepts attempt to answer:
Table 1 Key philosophical concepts and questions
Ontology relates to a central question of whether social entities need to be perceived as objective or subjective. In other words, within the scope of your research you need to decide whether the world is external to social world or the perceptions and actions of social actors create social phenomena. [2]
Accordingly, objectivism (or positivism ) and subjectivism can be specified as two important aspects of ontology.
Objectivism “portrays the position that social entities exist in reality external to social actors concerned with their existence” [3] . Alternatively, objectivism “is an ontological position that asserts that social phenomena and their meanings have an existence that is independent of social actors” [4] .
Subjectivism (also known as constructionism or interpretivism ) on the contrary, perceives that social phenomena are created from perceptions and consequent actions of those social actors concerned with their existence. Formally, constructionism can be defined as “ontological position which asserts that social phenomena and their meanings are continually being accomplished by social actors”. [5]
The Table 2 below illustrates the ontology of four major research philosophies related to business studies:
Table 2 Ontology of research philosophies
Identification of ontology at the start of the research process is critically important as it determines the choice of the research design . The figure below illustrates the consequent impact of ontology on the choice of research methods via epistemology, research approach, research strategy and methods of data collection and data analysis.
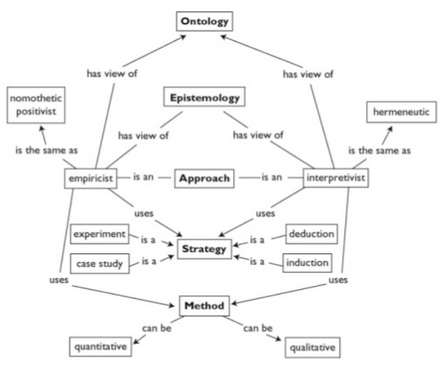
Impact of research philosophy on the choice of research method
Ontology in business studies
You don’t have to discuss ontology in great depth when writing a dissertation in business studies. Several paragraphs to one page will suffice for a dissertation on Bachelor’s or Master’s level, whereas you can devote about two pages to ontology on a research at a PhD level.
You can address ontology part of methodology chapter of your dissertation in the following manner:
Firstly, you can provide a formal definition of ontology with proper referencing. When providing definitions, it is better to use and reference books rather than internet web pages. This can be followed by explanation of ontology in simple terms, in your own words.
Secondly, you have to specify whether you are adopting objectivism or constructivism view. This should be followed by explanation of rationale for your choice by referring to your research aims and objectives.
Thirdly, you have to discuss implications of your ontological choice on the choice of epistemology, research approach, and research strategy and data collection methods.
My e-book, The Ultimate Guide to Writing a Dissertation in Business Studies: a step by step assistance contains discussions of theory and application of research philosophy. The e-book also explains all stages of the research process starting from the selection of the research area to writing personal reflection. Important elements of dissertations such as research philosophy , research approach , research design , methods of data collection and data analysis are explained in this e-book in simple words.
John Dudovskiy
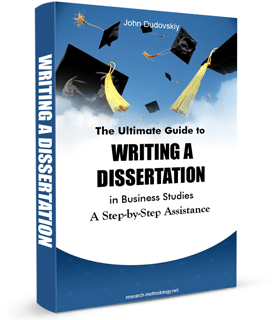
[1] Blaikie, N. (2010) “Designing Social Research” Polity Press
[2] Wilson, J. (2010) “Essentials of Business Research: A Guide to Doing Your Research Project” SAGE
[3] Saunders, M., Lewis, P. & Thornhill, A. (2012) “Research Methods for Business Students” 6 th edition, Pearson Education Limited
[4] Bryman, A. (2012) “Social Research Methods” 4 th edition, Oxford University Press
[5] Bryman, A. (2012) “Social Research Methods” 4 th edition, Oxford University Press
Our approach
- Responsibility
- Infrastructure
- Try Meta AI
COMPUTER VISION
Imagine flash: accelerating emu diffusion models with backward distillation.
April 18, 2024
Diffusion models are a powerful generative framework, but come with expensive inference. Existing acceleration methods often compromise image quality or fail under complex conditioning when operating in an extremely low-step regime. In this work, we propose a novel distillation framework tailored to enable high-fidelity, diverse sample generation using just one to three steps. Our approach comprises three key components: (i) Backward Distillation, which mitigates training-inference discrepancies by calibrating the student on its own backward trajectory; (ii) Shifted Reconstruction Loss that dynamically adapts knowledge transfer based on the current time step; and (iii) Noise Correction, an inference time technique that enhances sample quality by addressing singularities in noise prediction. Through extensive experiments, we demonstrate that our method outperforms existing competitors in quantitative metrics and human evaluations. Remarkably, it achieves performance comparable to the teacher model using only three denoising steps, enabling efficient high-quality generation.
Jonas Kohler
Albert Pumarola
Edgar Schoenfeld
Artsiom Sanakoyeu
Roshan Sumbaly
Peter Vajda
Research Topics
Computer Vision
Related Publications
March 20, 2024
SceneScript: Reconstructing Scenes With An Autoregressive Structured Language Model
Armen Avetisyan , Chris Xie , Henry Howard-Jenkins , Tsun-Yi Yang , Samir Aroudj , Suvam Patra , Fuyang Zhang , Duncan Frost , Luke Holland , Campbell Orme , Jakob Julian Engel , Edward Miller , Richard Newcombe , Vasileios Balntas

February 13, 2024
IM-3D: Iterative Multiview Diffusion and Reconstruction for High-Quality 3D Generation
Luke Melas-Kyriazi , Iro Laina , Christian Rupprecht , Natalia Neverova , Andrea Vedaldi , Oran Gafni , Filippos Kokkinos
January 25, 2024
LRR: Language-Driven Resamplable Continuous Representation against Adversarial Tracking Attacks
Felix Xu , Di Lin , Jianjun Zhao , Jianlang Chen , Lei Ma , Qing Guo , Wei Feng , Xuhong Ren
December 08, 2023
Learning Fine-grained View-Invariant Representations from Unpaired Ego-Exo Videos via Temporal Alignment
Sherry Xue , Kristen Grauman

Help Us Pioneer The Future of AI
We share our open source frameworks, tools, libraries, and models for everything from research exploration to large-scale production deployment..
Product experiences
Foundational models
Latest news
Meta © 2024

latest in US News

Environmentalist smashes world record by hugging 1,123 trees in...

Beloved New York Post photographer dead at 78

Anti-Israel protesters drape massive Palestinian flag over hotel...

Michigan teen warned best friend to slow down before fatal...

Human skeleton unearthed from Hudson Valley home sparking mystery...

Tornadoes level towns in Nebraska, Iowa: harrowing video

Victim stood up to thugs who tried to rob him at gunpoint in...

Anti-Israel protesters sue Columbia, claiming they're the real...
Yale professor accuses columbia prez shafik of plagiarism, ‘intellectual theft’ in resurfaced 1994 research paper.
- View Author Archive
- Get author RSS feed
- Email the Author
- Follow on Twitter
Contact The Author
Thanks for contacting us. We've received your submission.
Thanks for contacting us. We've received your submission.
Embattled Columbia University president Nemat “Minouche” Shafik screwed a former underling out of credit on a research paper published 30 years ago, a Yale University professor claims.
Ahmed Mushfiq Mobarak posted the bombshell allegations in a blistering thread on X early Friday, juxtaposing images of a 1992 report Shafik co-authored for World Bank with researcher Sushenjit Bandyopadhyay, along with a journal published in Oxford Economic Papers two years later in which Bandyopadhyay’s name was removed.

Mobarak, an economics and management professor at Yale, told The Post the findings and research cited in both papers are pretty much equal.
“It got rewritten, but fundamentally it’s the same paper,” he alleged.

“We can’t get in the room and [learn] what sentences did he write and what sentences she wrote, but what we do know is his contribution was sufficient to warrant co-authorship [in 1992],” he added. “What is not common is for someone to be a co-author and then suddenly their name is taken off.”
Instead, Bandyopadhyay is only “thanked” in an acknowledgement section in the back of the 1994 published journal — which screams of “power asymmetry” considering Shafik was then Bandyopadhyay’s boss, alleged Mobarak.
Bandyopadhyay declined comment when asked whether he felt slighted.
However, Mobarak, also a former World Bank consultant and University of Maryland graduate, said he spoke to Bandyopadhyay about the issue and that Bandyopadhyay believes he should have been credited as a co-author in the second paper. The professor conceded Bandyopadhyay never said anything “negative” about the Columbia president.

“This [1994] paper is lifted almost entirely from a 1992 report coauthored with consultant not credited in the publication,” wrote Mobarak on X. “This is wholesale intellectual theft, not subtle plagiarism.”
At the time both papers were written, Shafik was a vice president for World Bank and Bandyopadhyay, a consultant who also attended the University of Maryland.

Mobarak’s allegations echo plagiarism accusations leveled against former Harvard University president Claudine Gay, who eventually resigned in disgrace in January .
Columbia University spokesperson Ben Chang shot down the Yale professor’s claims, saying “this is an absurd attempt at running a well-known playbook, and it has no credibility.”
Share this article:

Advertisement
Suggestions or feedback?
MIT News | Massachusetts Institute of Technology
- Machine learning
- Social justice
- Black holes
- Classes and programs
Departments
- Aeronautics and Astronautics
- Brain and Cognitive Sciences
- Architecture
- Political Science
- Mechanical Engineering
Centers, Labs, & Programs
- Abdul Latif Jameel Poverty Action Lab (J-PAL)
- Picower Institute for Learning and Memory
- Lincoln Laboratory
- School of Architecture + Planning
- School of Engineering
- School of Humanities, Arts, and Social Sciences
- Sloan School of Management
- School of Science
- MIT Schwarzman College of Computing
Researchers detect a new molecule in space
Press contact :.
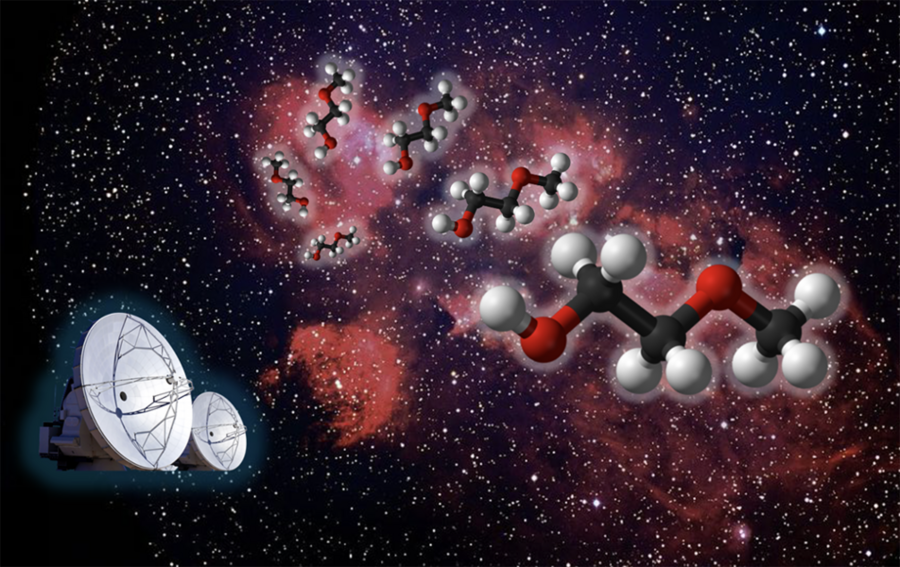
Previous image Next image
New research from the group of MIT Professor Brett McGuire has revealed the presence of a previously unknown molecule in space. The team's open-access paper, “ Rotational Spectrum and First Interstellar Detection of 2-Methoxyethanol Using ALMA Observations of NGC 6334I ,” appears in April 12 issue of The Astrophysical Journal Letters .
Zachary T.P. Fried , a graduate student in the McGuire group and the lead author of the publication, worked to assemble a puzzle comprised of pieces collected from across the globe, extending beyond MIT to France, Florida, Virginia, and Copenhagen, to achieve this exciting discovery.
“Our group tries to understand what molecules are present in regions of space where stars and solar systems will eventually take shape,” explains Fried. “This allows us to piece together how chemistry evolves alongside the process of star and planet formation. We do this by looking at the rotational spectra of molecules, the unique patterns of light they give off as they tumble end-over-end in space. These patterns are fingerprints (barcodes) for molecules. To detect new molecules in space, we first must have an idea of what molecule we want to look for, then we can record its spectrum in the lab here on Earth, and then finally we look for that spectrum in space using telescopes.”
Searching for molecules in space
The McGuire Group has recently begun to utilize machine learning to suggest good target molecules to search for. In 2023, one of these machine learning models suggested the researchers target a molecule known as 2-methoxyethanol.
“There are a number of 'methoxy' molecules in space, like dimethyl ether, methoxymethanol, ethyl methyl ether, and methyl formate, but 2-methoxyethanol would be the largest and most complex ever seen,” says Fried. To detect this molecule using radiotelescope observations, the group first needed to measure and analyze its rotational spectrum on Earth. The researchers combined experiments from the University of Lille (Lille, France), the New College of Florida (Sarasota, Florida), and the McGuire lab at MIT to measure this spectrum over a broadband region of frequencies ranging from the microwave to sub-millimeter wave regimes (approximately 8 to 500 gigahertz).
The data gleaned from these measurements permitted a search for the molecule using Atacama Large Millimeter/submillimeter Array (ALMA) observations toward two separate star-forming regions: NGC 6334I and IRAS 16293-2422B. Members of the McGuire group analyzed these telescope observations alongside researchers at the National Radio Astronomy Observatory (Charlottesville, Virginia) and the University of Copenhagen, Denmark.
“Ultimately, we observed 25 rotational lines of 2-methoxyethanol that lined up with the molecular signal observed toward NGC 6334I (the barcode matched!), thus resulting in a secure detection of 2-methoxyethanol in this source,” says Fried. “This allowed us to then derive physical parameters of the molecule toward NGC 6334I, such as its abundance and excitation temperature. It also enabled an investigation of the possible chemical formation pathways from known interstellar precursors.”
Looking forward
Molecular discoveries like this one help the researchers to better understand the development of molecular complexity in space during the star formation process. 2-methoxyethanol, which contains 13 atoms, is quite large for interstellar standards — as of 2021, only six species larger than 13 atoms were detected outside the solar system , many by McGuire’s group, and all of them existing as ringed structures.
“Continued observations of large molecules and subsequent derivations of their abundances allows us to advance our knowledge of how efficiently large molecules can form and by which specific reactions they may be produced,” says Fried. “Additionally, since we detected this molecule in NGC 6334I but not in IRAS 16293-2422B, we were presented with a unique opportunity to look into how the differing physical conditions of these two sources may be affecting the chemistry that can occur.”
Share this news article on:
Related links.
- McGuire Lab
- Department of Chemistry
Related Topics
- Space, astronomy and planetary science
- Astrophysics
Related Articles
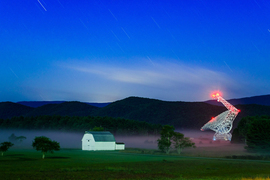
Found in space: Complex carbon-based molecules
Previous item Next item
More MIT News
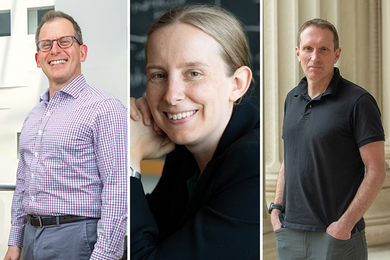
Three from MIT awarded 2024 Guggenheim Fellowships
Read full story →
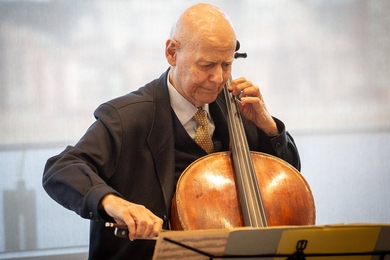
A musical life: Carlos Prieto ’59 in conversation and concert
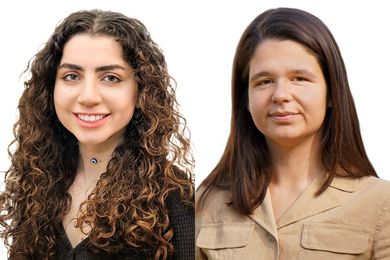
Two from MIT awarded 2024 Paul and Daisy Soros Fellowships for New Americans
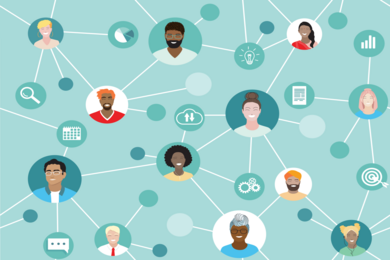
MIT Emerging Talent opens pathways for underserved global learners
The MIT Edgerton Center’s third annual showcase dazzles onlookers
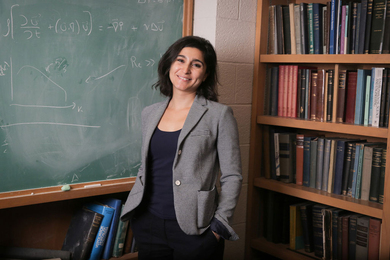
3 Questions: A shared vocabulary for how infectious diseases spread
- More news on MIT News homepage →
Massachusetts Institute of Technology 77 Massachusetts Avenue, Cambridge, MA, USA
- Map (opens in new window)
- Events (opens in new window)
- People (opens in new window)
- Careers (opens in new window)
- Accessibility
- Social Media Hub
- MIT on Facebook
- MIT on YouTube
- MIT on Instagram
share this!
April 23, 2024
This article has been reviewed according to Science X's editorial process and policies . Editors have highlighted the following attributes while ensuring the content's credibility:
fact-checked
peer-reviewed publication
trusted source
Researchers detect a new molecule in space
by Danielle Randall Doughty, Massachusetts Institute of Technology
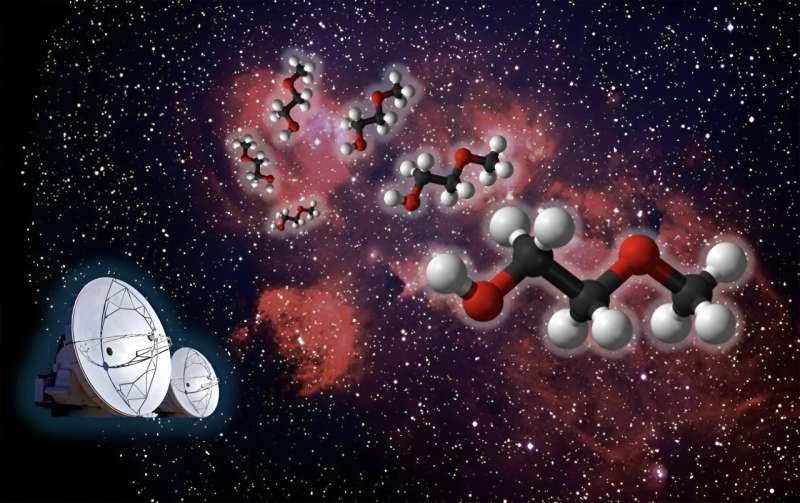
New research from the group of MIT Professor Brett McGuire has revealed the presence of a previously unknown molecule in space. The team's open-access paper, "Rotational Spectrum and First Interstellar Detection of 2-Methoxyethanol Using ALMA Observations of NGC 6334I," was published in the April 12 issue of The Astrophysical Journal Letters .
Zachary T.P. Fried, a graduate student in the McGuire group and the lead author of the publication, worked to assemble a puzzle comprised of pieces collected from across the globe, extending beyond MIT to France, Florida, Virginia, and Copenhagen, to achieve this exciting discovery.
"Our group tries to understand what molecules are present in regions of space where stars and solar systems will eventually take shape," explains Fried. "This allows us to piece together how chemistry evolves alongside the process of star and planet formation. We do this by looking at the rotational spectra of molecules, the unique patterns of light they give off as they tumble end-over-end in space.
"These patterns are fingerprints (barcodes) for molecules. To detect new molecules in space, we first must have an idea of what molecule we want to look for, then we can record its spectrum in the lab here on Earth, and then finally we look for that spectrum in space using telescopes."
Searching for molecules in space
The McGuire Group has recently begun to utilize machine learning to suggest good target molecules to search for. In 2023, one of these machine learning models suggested the researchers target a molecule known as 2-methoxyethanol.
"There are a number of 'methoxy' molecules in space, like dimethyl ether, methoxymethanol, ethyl methyl ether, and methyl formate, but 2-methoxyethanol would be the largest and most complex ever seen," says Fried.
To detect this molecule using radio telescope observations, the group first needed to measure and analyze its rotational spectrum on Earth. The researchers combined experiments from the University of Lille (Lille, France), the New College of Florida (Sarasota, Florida), and the McGuire lab at MIT to measure this spectrum over a broadband region of frequencies ranging from the microwave to sub-millimeter wave regimes (approximately 8 to 500 gigahertz).
The data gleaned from these measurements permitted a search for the molecule using Atacama Large Millimeter/submillimeter Array (ALMA) observations toward two separate star-forming regions: NGC 6334I and IRAS 16293-2422B. Members of the McGuire group analyzed these telescope observations alongside researchers at the National Radio Astronomy Observatory (Charlottesville, Virginia) and the University of Copenhagen, Denmark.
"Ultimately, we observed 25 rotational lines of 2-methoxyethanol that lined up with the molecular signal observed toward NGC 6334I (the barcode matched), thus resulting in a secure detection of 2-methoxyethanol in this source," says Fried. "This allowed us to then derive physical parameters of the molecule toward NGC 6334I, such as its abundance and excitation temperature. It also enabled an investigation of the possible chemical formation pathways from known interstellar precursors."
Looking forward
Molecular discoveries like this one help the researchers to better understand the development of molecular complexity in space during the star formation process. 2-methoxyethanol, which contains 13 atoms, is quite large for interstellar standards—as of 2021, only six species larger than 13 atoms were detected outside the solar system, many by McGuire's group, and all of them existing as ringed structures.
"Continued observations of large molecules and subsequent derivations of their abundances allows us to advance our knowledge of how efficiently large molecules can form and by which specific reactions they may be produced," says Fried.
"Additionally, since we detected this molecule in NGC 6334I but not in IRAS 16293-2422B, we were presented with a unique opportunity to look into how the differing physical conditions of these two sources may be affecting the chemistry that can occur."
Journal information: Astrophysical Journal Letters
Provided by Massachusetts Institute of Technology
This story is republished courtesy of MIT News ( web.mit.edu/newsoffice/ ), a popular site that covers news about MIT research, innovation and teaching.
Explore further
Feedback to editors

Global study shows a third more insects come out after dark
10 hours ago

Cicada-palooza! Billions of bugs to blanket America
12 hours ago

Getting dynamic information from static snapshots

Ancient Maya blessed their ballcourts: Researchers find evidence of ceremonial offerings in Mexico

Optical barcodes expand range of high-resolution sensor
Apr 26, 2024

Ridesourcing platforms thrive on socio-economic inequality, say researchers

Did Vesuvius bury the home of the first Roman emperor?

Florida dolphin found with highly pathogenic avian flu: Report

A new way to study and help prevent landslides

New algorithm cuts through 'noisy' data to better predict tipping points
Relevant physicsforums posts, need help simplifying standard error formula for redshift.
13 hours ago
Our Beautiful Universe - Photos and Videos
Apr 25, 2024
Solar Activity and Space Weather Update thread
'devil' comet visible tonight 21.04.24, waves in space, documenting the setup of my new telescope.
Apr 24, 2024
More from Astronomy and Astrophysics
Related Stories

ALMA's highest frequency receiver produces its first scientific result on massive star formation
Nov 22, 2018

GBT detection unlocks exploration of 'aromatic' interstellar chemistry
Jan 11, 2018

Astronomers discover largest molecule yet in a planet-forming disc
Mar 8, 2022

Found in space: Complex carbon-based molecules
Mar 18, 2021

First science with ALMA's highest-frequency capabilities
Aug 17, 2018

ALMA finds new molecular signposts in starburst galaxy
Mar 28, 2024
Recommended for you

Research investigates radio emission of the rotating radio transient RRAT J1854+0306

Researchers advance detection of gravitational waves to study collisions of neutron stars and black holes

Recently discovered black hole is part of a nearby disrupted star cluster, study finds

International team detects eruption of mega-magnetic star in nearby galaxy

Black hole 'traffic jams' discovered in galactic centers by astronomers

New evidence found for Planet 9
Apr 23, 2024
Let us know if there is a problem with our content
Use this form if you have come across a typo, inaccuracy or would like to send an edit request for the content on this page. For general inquiries, please use our contact form . For general feedback, use the public comments section below (please adhere to guidelines ).
Please select the most appropriate category to facilitate processing of your request
Thank you for taking time to provide your feedback to the editors.
Your feedback is important to us. However, we do not guarantee individual replies due to the high volume of messages.
E-mail the story
Your email address is used only to let the recipient know who sent the email. Neither your address nor the recipient's address will be used for any other purpose. The information you enter will appear in your e-mail message and is not retained by Phys.org in any form.
Newsletter sign up
Get weekly and/or daily updates delivered to your inbox. You can unsubscribe at any time and we'll never share your details to third parties.
More information Privacy policy
Donate and enjoy an ad-free experience
We keep our content available to everyone. Consider supporting Science X's mission by getting a premium account.
E-mail newsletter

IMAGES
VIDEO
COMMENTS
between ontology research and bibliographic classification, fostering coop eration, as for exa mple Vickery (1997). Emphasizing the importance of the procedural aspect of ontology, as a study of ...
New Delhi: Primus Books, 2017, 351 pp., ₹ 1,195 (hardback). ISBN: 978-93-86552-15-. The review attempts to place the contributions of Ananta Kumar Giri, the editor of the three volumes, and the writers who contributed to the volumes in the debate on the relations between epistemology and ontology that has been going on in social sciences ...
This paper outlines the. links among ontology, epistemology and research methodology by exploring. ontological, epistemological and methodological perspectives in the research. It discusses how ...
The COVID-19 pandemic prompted immense work on the investigation of the SARS-CoV-2 virus. Rapid, accurate, and consistent interpretation of generated data is thereby of fundamental concern. Ontologies-structured, controlled, vocabularies-are designed to support consistency of interpretation, and thereby to prevent the development of data silos. This paper describes how ontologies are ...
In addition, plenty of information is presented in scientific research in textual form, e.g., research papers by many domain experts. Those research papers contain a high number of domain-specific vocabulary. Using techniques from Natural Language Processing ... 3.3 Extension of an ontology by new classes based on text dataset.
1 Introduction. Unprecedented amounts of clinically relevant data are now available for clinical and research use, including Electronic Health Records (EHRs), laboratory reports, imaging, clinical instrument outputs, drugs and drug doses, genomic investigations, and dynamic data from wearable devices 1.Machine Learning (ML) is increasingly being applied to these data sources for predictive ...
Introduction Ontologies are a formal way to represent knowledge in a particular field and have the potential to transform the field of health promotion and digital interventions. However, few researchers in physical activity (PA) are familiar with ontologies, and the field can be difficult to navigate. This systematic review aims to (1) identify ontologies in the field of PA, (2) assess their ...
They summarized the contents of ontology learning papers in perspective of methodologies used for ontology extraction, evaluation methods and challenges of various real life application scenarios. ... This survey considers the latest trends in different tasks of ontology learning layer cake. ... We found 200 research papers using Google Scholar ...
3. Ontology-based information retrieval. This section reviews the state of the art in ontology-based database information retrieval. Here, a historical overview of information retrieval approaches is first presented, followed by a detailed analysis of existing ontology-based query systems and data search strategies in relation to three different key aspects that guided the review of such work.
This paper by Nathan Andrew Baker discusses the design and development of an ontology relating to the preparation, chemical composition, and characterization of nanomaterials involved in cancer research. While the ontology is in part developed within the framework of the BFO, it does not yet satisfy all of the associated principles.
Nucleic Acids Research, Volume 47, Issue D1, 08 January 2019, Pages D330-D338, ... papers with annotations will have a link labeled 'Gene Ontology annotations from this paper - Gene Ontology' (see, ... One major advantage of the new ontology management process is that the work can be parallelized among multiple editors, thus increasing ...
This survey is presented in two parts. The first part reviews the state-of-the-art techniques and work done on semi-automatic and automatic ontology generation, as well as the problems facing such ...
2.1. Ontologies. The term "ontology" was borrowed from philosophy to computer science to signify a machine-readable formalization of a conceptualization pertaining to a particular domain of knowledge [].That is to say, an ontology is a digital artifact that can be interpreted by both humans and computers and which encodes the terminology and the semantic relations between concepts in a ...
Ontology is an important emerging discipline that has the huge potential to improve information organization, management and understanding. It has a crucial role to play in enabling content-based access, interoperability, communications, and providing qualitatively new levels of services on the next wave of web transformation in the form of the Semantic Web.
With new articles being added to these collections on a daily basis, the collections serve as an ideal tool to keep researchers updated with new developments in the respective fields. ... and further possible research directions of the ontology. This paper benefit reader who wishes to embark on ontology-based research and application ...
SUBMIT PAPER. Close Add email alerts. You are adding the following journal to your email alerts ... Story as link between nursing practice, ontology, epistemology. Image, 23 (4), 245-248. Google Scholar. Cash, K. (1997). Social epistemology, gender and nursing theory. ... Sage Knowledge Multimedia learning resources opens in new tab; Sage ...
The Gene Ontology (GO) project is a bioinformatics initiative that provides an ontology (shared vocabulary) of defined terms to represent specific gene product properties. ... Latest Research and ...
Ontology work with concepts and relations that are very close to the working of the human brain. Ontological engineering provides the methods and methodologies for the development of ontology. Nowadays, ontologies are used in almost every field, and a lot of research is being done on this topic. The paper aims to elaborate the need of ontology ...
The issue of ontology learning is divided into nine sub-issues according to the structured degree of source data and learning objects and the characteristics, major approaches, and the latest research progress of the nineSub-issues are summarized. Recently, ontology learning is emerging as a new hotspot of research in computer science. In this paper the issue of ontology learning is divided ...
The proposed S&TO can promote the discovery of new research areas and collaborations across disciplines. The ontology is constructed by applying BERTopic to a dataset of 393,991 scientific articles collected from Semantic Scholar from October 2021 to August 2022, covering four fields of science. Currently, S&TO includes 5,153 topics and 13,155 ...
proc eedin gs. Proceeding Paper. Ontology and AI Paradigms †. Roman Krzanowski and Pawel Polak *. Faculty of Philosophy, The Pontifical University of John Paul II in Krakow, Kanonicza Street 9 ...
research paradigm that aligns with their methodological choice for the study. In addressing this challenge, this paper discusses various paradigms of research and the two dominant epistemological assumptions; positivism, constructivism or interpretivism often debated in business and management research from an ontological perspective.
Social science research guide consisting of ontology, epistemology, and philosophical perspectives. When read from left to right, elements take on a more multidimensional nature (eg., epistemology: objectivism to subjectivism). ... Katie Moon is a Post Doctoral Research Fellow at the University of New South Wales, Canberra. She is also an ...
Ontology in business research can be defined as "the science or study of being" [1] and it deals with the nature of reality. Ontology is a system of belief that reflects an interpretation of an individual about what constitutes a fact. In simple terms, ontology is associated with what we consider as reality.
Abstract. Diffusion models are a powerful generative framework, but come with expensive inference. Existing acceleration methods often compromise image quality or fail under complex conditioning when operating in an extremely low-step regime.
Yale management and economics professor Ahmed Mushfiq Mobarak accused Columbia University president Minouche Shafik of "intellectual theft" over a 30-year-old research paper he says fails to ...
New research from the group of MIT Professor Brett McGuire has revealed the presence of a previously unknown molecule in space. The team's open-access paper, "Rotational Spectrum and First Interstellar Detection of 2-Methoxyethanol Using ALMA Observations of NGC 6334I," appears in April 12 issue of The Astrophysical Journal Letters. Zachary T.P. Fried, a graduate student in the McGuire ...
New research from the group of MIT Professor Brett McGuire has revealed the presence of a previously unknown molecule in space. The team's open-access paper, "Rotational Spectrum and First ...