Narrative Analysis In Qualitative Research
Saul Mcleod, PhD
Editor-in-Chief for Simply Psychology
BSc (Hons) Psychology, MRes, PhD, University of Manchester
Saul Mcleod, PhD., is a qualified psychology teacher with over 18 years of experience in further and higher education. He has been published in peer-reviewed journals, including the Journal of Clinical Psychology.
Learn about our Editorial Process
Olivia Guy-Evans, MSc
Associate Editor for Simply Psychology
BSc (Hons) Psychology, MSc Psychology of Education
Olivia Guy-Evans is a writer and associate editor for Simply Psychology. She has previously worked in healthcare and educational sectors.
On This Page:

What Is Narrative Analysis?
Narrative analysis is a qualitative research method used to understand how individuals create stories from their personal experiences.
There is an emphasis on understanding the context in which a narrative is constructed, recognizing the influence of historical, cultural, and social factors on storytelling.
It differs from other qualitative methods like interpretive phenomenological analysis (IPA) and discourse analysis by specifically examining how individuals use stories to make sense of their experiences and the world around them.
Narrative analysis is not applicable to all research topics; it is best suited when the focus of the analysis is narratives or stories.
Examples of topics that are well-suited to narrative analysis include: various aspects of identity, individual experiences of psychological processes, interpersonal and intimate relationships, and experiences of body, beauty and health
Assumptions of Narrative Analysis
- Stories are interpretations of the world and experiences: Narrative analysis assumes that stories are not accurate representations of reality. People use stories to explain or normalize what has occurred in their lives and make sense of why things are the way they are. People make sense of their lives through the stories they tell.
- Language is an object for close investigation: A structural analysis of a narrative focuses on the way a story is told, treating language as an object for investigation in itself, not just as it refers to content. This kind of analysis attends to the linguistic phenomena of a story and its overall composition.
- Meaning is created through narrative: Narrative inquiry is the study of how stories unfold over time and is useful for understanding how people perceive reality, make sense of their worlds, and perform social actions. Researchers and participants are co-authors of stories because they collaborate to create meaning. Narrative analysts show how the tools (e.g. its structure and style) used to build a story create the meaning of the experience being shared
- Stories do not speak for themselves: Narratives do not speak for themselves, and they require interpretation when used as data in social research. Researchers must interpret a story by deciding what constitutes a story, collecting stories, identifying stories within data, and identifying narrative themes and relationships.
Key Concepts in Narrative Analysis
Narrative analysis is concerned with more than just what is said (the content). It also considers how the story is constructed (the structure) and the context or situation in which the story is told (the performance)
- Defining “Story” and “Narrative” : A story is a structured account of events, while a narrative is a story that has been shaped and given meaning by a storyteller. The process of transforming events into a narrative involves selecting, organizing, and interpreting those events in a way that conveys a particular message or understanding.
- Content: While narrative analysis values how a story is told, the content ( what is said ) remains significant. The themes, events, and characters in a story provide insights into the storyteller’s experiences, beliefs, and values. Therefore, narrative analysis sees content as inseparable from structure and performance. All three work together to create the meaning of a story.
- Narrative Structure: Narrative analysis examines how elements like plot, setting, and characterization are used to construct a story. For example, a researcher might study how the sequence of events, the choice of words, or the use of metaphors shapes the meaning of a story.
- Narrative as Performance: Narratives are not simply neutral accounts of events but are performed and co-constructed through interactions between the storyteller and the audience. This means that understanding a narrative involves paying attention to how it is told, who is telling it, and to whom it is being told. For instance, a researcher might study how a story changes depending on who is telling it, or how the same story is received by different audiences.
Approaches to Narrative Analysis
There are different models and approaches to narrative analysis, and the type that is used depends on the research problem.
- Thematic Analysis : Thematic analysis assumes language is a direct and unambiguous route to meaning. In this approach, researchers collect many stories and then inductively create conceptual groupings from the data. One of the assumptions of thematic analysis is that everyone in the group means the same thing by what they say, even when grouped into a similar thematic category.
- Structural Analysis: This approach views language as a resource and an object for investigation, moving beyond the referential content. Structural analysis assumes the way a story is told is as important as the content of the story. Following Labov’s Narrative Model, the researcher may focus on identifying and examining the key elements of narrative structure, such as the abstract, orientation, complicating action, evaluation, resolution, and coda.
- Interactional Analysis: Interactional analysis looks at how narratives are created and understood within the context of social interactions. This approach acknowledges that narratives are not created in isolation but are shaped by the listener’s responses, the social context of the storytelling, and the relationship between the storyteller and the listener. E.g. Mishler’s Model.
- Performance Analysis : Examining the performative elements of storytelling such as the use of language, nonverbal communication, and audience engagement provides further insights into how stories are constructed and the effects they create. Researchers are interested in how the narrator positions themselves in relation to the audience.
Pratical Steps: Conducting Narrative Analysis
The steps involved in conducting narrative analysis are often iterative and non-linear, rather than following a strict sequential order.
While the steps provide a general framework and guidance for the research process, in practice, researchers may move back and forth between different stages, or engage in multiple steps simultaneously, as new insights and questions emerge from the data.
The iterative nature of narrative analysis reflects the complex and dynamic nature of human experience and meaning-making.
1. Situate the Epistemological Approach
Determine whether to use a naturalist or constructivist approach. The research questions and theoretical framework inform this decision.
Situating the epistemological approach at the outset of the study helps ensure consistency and coherence throughout the research process, guiding methodological choices and the interpretation of findings.
If the research questions focus on understanding the subjective experiences and meaning-making processes of participants, a constructivist approach may be more appropriate.
Conversely, if the research aims to identify common patterns or themes across narratives and assumes a more objective reality, a naturalist approach may be suitable.
Naturalist Approach :
- Assumes that narratives reflect an objective reality or truth
- Seeks to capture and understand the “real” experiences and perspectives of participants
- Aims to minimize the researcher’s influence on the data collection and interpretation process
- Aligns with a more positivist or realist paradigm
Constructivist Approach :
- Assumes that narratives are constructed and shaped by the interaction between the narrator and the listener (researcher)
- Acknowledges that multiple realities or truths can exist, as individuals interpret and make sense of their experiences differently
- Recognizes the researcher’s role in co-creating meaning during the data collection and analysis process
- Aligns with an interpretivist or social constructionist paradigm
2. Select the Analytical Model(s)
Decide which model(s) to use in analyzing narrative data. Different models focus on different features of narratives and raise distinct questions during analysis.
Research design, informed by the chosen epistemological approach, will guide decisions regarding the use of single or multiple models.
- Structural Model: Examines the structure of stories and the ways in which they are told. Considers elements such as plot, characters, setting, and narrative arc
- Thematic Model: Analyzes the content of stories, focusing on the themes around which stories are told. May involve coding the data to identify recurrent themes and organizing them into categories or hierarchies
- Interactional/Performative Model: Investigates the contextual features that shape the construction of narratives and how meaning is collaboratively created through interaction between storytellers and listeners.
3. Select Narratives to Analyze
In conducting narrative analysis involves selecting specific narratives to analyze within the larger dataset. Even when the aim is to analyze the data holistically, researchers often choose to focus on particular narratives for close scrutiny.
This selection process is guided by the research questions, theoretical framework, and the analytical strategy employed in the study.
When selecting narratives to analyze, researchers may consider the following:
- Representativeness : Choosing narratives that are representative of the broader dataset or the phenomena under investigation. This may involve selecting narratives that exemplify common themes, patterns, or experiences shared by multiple participants.
- Uniqueness : Identifying narratives that stand out as unique, unusual, or deviant cases. These narratives may offer valuable insights into the diversity of experiences or challenge dominant patterns or assumptions.
- Theoretical relevance : Selecting narratives that are particularly relevant to the theoretical framework or concepts guiding the study. These narratives may help illuminate or expand upon key theoretical ideas.
- Richness of data : Choosing narratives that are rich in detail, providing thick descriptions and in-depth insights into the participants’ experiences, thoughts, and emotions.
4. Identifying Narrative Blocks
A narrative block refers to a complete, self-contained story or narrative within a larger dataset, such as an interview transcript.
It is a segment of the data that has a clear beginning, middle, and end, and that conveys a specific experience, event, or perspective of the participant.
This involves looking for cues like “entrance and exit talk”, which signal the beginning and end of a distinct narrative within a conversation.
For instance, phrases like, “There was this one time…” or “Let me give you an example…” may signal the beginning of a narrative block.
Similarly, phrases like, “So that’s how that wrapped up…” or “That is a pretty classic example of…” can help researchers pinpoint the end of a narrative block
It is important to note that the selection of narratives and units of analysis is an iterative process, and researchers may revisit and refine their choices as they delve deeper into the data and their analysis progresses.
Researchers should be transparent about their selection criteria and process, and should reflect on how their choices may impact the interpretation and findings of the study.
Here’s an example of what a narrative block might look like:
“I remember when I first started college. I was so nervous and excited at the same time. I didn’t know anyone on campus, and I was worried about fitting in. But during orientation week, I met this group of people who were just as lost and nervous as I was. We bonded over our shared experiences and became fast friends. That group of friends made all the difference in my college experience. We supported each other through the ups and downs, and I don’t think I would have made it through without them.”
This narrative block has a clear beginning (starting college), middle (meeting friends during orientation week), and end (reflecting on the importance of those friendships throughout college).
It conveys a specific experience and perspective of the participant, making it a suitable unit for narrative analysis.
5. Code Narrative Blocks
In conducting narrative analysis involves coding the narrative blocks using one or multiple analytical models.
Coding is the process of assigning labels or categories to segments of data, allowing researchers to organize, retrieve, and interpret the information in a systematic manner.
The coding process may involve several rounds or iterations, as researchers refine their codes and categories based on their deepening understanding of the data.
There are two main approaches to coding narrative blocks:
It’s important to note that these classifications are not always clear-cut, and researchers may use a combination of inductive and deductive approaches in their analysis.
For example, a researcher might start with a deductive structural analysis, using a predefined model of narrative structure, but then switch to an inductive thematic analysis to identify emergent themes within each structural element.
Inductive Coding
This approach, starting with the data and allowing themes and categories to emerge from the narratives aligns with a constructivist approach, where meaning is viewed as co-created between the researcher and the participant.
Researchers using inductive coding might identify emergent themes in the narratives about “life events” and code these narrative blocks accordingly.
For example, stories about deciding to have children could be coded as “Narratives about deciding to have children”.
- Also known as “open coding” or “data-driven coding”
- Involves allowing themes and categories to emerge from the data itself, rather than imposing pre-existing frameworks or theories
- Researchers immerse themselves in the narrative data, identifying patterns, similarities, and differences across the stories
- Codes are developed based on the researcher’s interpretation of the data and are refined iteratively throughout the analysis process
- Aligns with a constructivist approach, acknowledging the researcher’s role in co-creating meaning and the possibility of multiple interpretations
Deductive Coding
This approach, using pre-existing frameworks or theories to guide the coding process, aligns with a naturalist approach, where the researcher seeks to objectively identify and categorize elements of the narratives.
One such framework is the one proposed by Labov (1997), which identifies six key elements of a story:
- Abstract : A summary or overview of the story, often provided at the beginning
- Orientation : The setting or context of the story, including information about the time, place, characters, and situation
- Complicating Action : The main plot or sequence of events that drive the story forward, often involving a problem, challenge, or conflict
- Evaluation : The storyteller’s commentary on the meaning or significance of the events, revealing their attitudes, opinions, or emotions
- Resolution : The outcome or conclusion of the story, often resolving the complicating action or providing a sense of closure
- Coda : An optional element that brings the story back to the present or reflects on its broader implications
When using this framework for deductive coding, researchers would analyze each narrative block, looking for segments that correspond to these six elements. They would then assign the appropriate code to each segment, such as “Abstract,” “Orientation,” “Complicating Action,” and so on.
Here’s an example of how this might be applied to a narrative block:
“I remember my first day at my new job [Orientation]. I was so nervous and excited at the same time [Evaluation]. As soon as I walked in, I realized I had forgotten my employee ID [Complicating Action]. I panicked and thought I would be fired on the spot [Evaluation]. But then my manager came over, laughed, and said, ‘Don’t worry, it happens to everyone. We’ll get you a new one.’ [Resolution] That moment taught me that it’s okay to make mistakes and that my new workplace was actually pretty understanding [Coda].”
By applying Labov’s story structure framework, researchers can systematically analyze the narrative data, identifying patterns in how stories are structured and told.
This can provide insights into the way individuals make sense of their experiences and construct meaning through storytelling.
Step 6: Delve into the Story Structure
This step involves a deep and systematic examination of the coded narrative data, with a focus on understanding how the narrators use story structure elements (e.g., abstract, orientation, complicating action, evaluation, resolution, and coda) to construct meaning and convey their experiences.
By delving into the story structure, researchers can identify patterns, themes, and variations across different narratives, and gain insights into the ways in which individuals make sense of their lives through storytelling.
It allows researchers to move beyond the surface level of the narratives and to gain a deeper understanding of how individuals use storytelling to make sense of their lives and multifaceted nature of human experience.
This involves:
- Researchers organize the coded narrative data by grouping together segments that belong to the same story structure element (e.g., all “orientation” segments, all “complicating action” segments, etc.).
- This allows researchers to compare and contrast how different narrators use each story structure element, and to identify patterns, themes, and variations across the narratives.
- Researchers closely examine the content of each coded segment, paying attention to the specific details, descriptions, and evaluations provided by the narrators.
- They also consider the function of each story structure element, i.e., how it contributes to the overall meaning and coherence of the narrative.
- For example, researchers might analyze how narrators use the “orientation” element to set the scene, introduce characters, and provide context for their stories, or how they use the “evaluation” element to convey their attitudes, emotions, and reflections on the events being narrated.
- Researchers seek to understand how narrators make sense of their experiences and construct meaning through the way they structure and tell their stories.
- This involves considering the interplay between story structure, content, and context, and how these elements shape the overall meaning and significance of the narratives.
- Researchers may also consider the narrator’s perspective, the audience and social context of the storytelling, and the broader cultural and historical frameworks that inform the narratives.
Throughout this process, researchers need to be aware of the challenges and complexities of interpretation, such as the fact that narrators may not always follow a linear or coherent story structure, or that different individuals may attribute different meanings to similar experiences.
Researchers should aim to provide nuanced and contextualized descriptions of their findings, supported by relevant examples and quotes from the narratives.
Step 7: Compare Across Story Structure
This step involves a comparative analysis of the narrative data, looking for patterns, similarities, and differences in how story structure elements are used across different narratives.
In the previous step (Step 6: Delve into the Story Structure), researchers examined each story structure element in depth, analyzing its content, function, and meaning within individual narratives.
In Step 7, the focus shifts to a higher-level analysis, where researchers compare and contrast the use of story structure elements across the entire dataset.
The goal is to provide a comprehensive and integrative understanding of the narrative data, one that goes beyond the analysis of individual stories and reveals the broader patterns, meanings, and significance of storytelling in human experience.
This comparative analysis can be done in several ways:
- Researchers look for similarities and differences in how different individuals use each story structure element (e.g., orientation, complicating action, resolution) to construct their narratives.
- This can reveal patterns in how people from different backgrounds, experiences, or perspectives structure and tell their stories.
- Researchers may also compare the use of story structure elements across different types of narratives, such as life stories, event narratives, or turning point narratives.
- This can help identify genre-specific patterns or conventions in how stories are structured and told.
- Researchers may consider how the social, cultural, or historical context in which narratives are produced influences the way story structure elements are used.
- For example, they may compare narratives told in different settings (e.g., interviews, social media, public speeches), or at different points in time, to see how context shapes the structure and content of stories.
Throughout this comparative analysis, researchers should remain attentive to the overarching narrative and the broader themes and meanings that emerge from the data.
While breaking down narratives into specific story structure elements can provide valuable insights, it’s important not to lose sight of the holistic nature of narratives and the way in which different elements work together to create meaning.
Researchers should also be reflexive about their own role in the analysis process, acknowledging how their own backgrounds, assumptions, and interpretive frameworks may shape their understanding of the narratives.
They should strive to provide a balanced and nuanced account of their findings, highlighting both the commonalities and the variations in how story structure elements are used across different narratives.
By comparing story structure elements across the dataset, researchers can generate new insights and theories about the ways in which individuals use storytelling to make sense of their lives and experiences.
They may identify common patterns or structures that underlie different types of narratives, or they may discover how particular social, cultural, or historical factors shape the way stories are told.
Step 8: Tell the Core Narrative
This step involves synthesizing the insights and findings from the previous steps into a coherent and compelling narrative account that captures the essence of the research participants’ experiences and the key themes and meanings that emerged from the analysis.
At this stage, researchers have thoroughly examined the narrative data, coding and analyzing it at various levels, from the specific story structure elements to the broader patterns and comparisons across narratives.
They have gained a deep understanding of how participants use storytelling to make sense of their lives and experiences, and how different factors (such as social, cultural, or historical context) shape the way stories are told.
In Step 8, researchers aim to distill this complex and multifaceted understanding into a clear and concise narrative that conveys the core insights and conclusions of the study.
The goal is to provide a powerful and insightful narrative account that captures the richness and complexity of the research participants’ experiences, and that contributes to a deeper understanding of the ways in which storytelling shapes and reflects human lives and meanings.
By telling the core narrative, researchers can communicate the significance and relevance of their findings to a wider audience, and contribute to ongoing conversations and debates in their field and beyond.
- Researchers review the findings from the previous steps and identify the most salient and significant themes and meanings that emerged from the analysis.
- These themes may relate to the content of the narratives (e.g., common experiences, challenges, or turning points), the structure of the narratives (e.g., common patterns or variations in how stories are told), or the broader social and cultural factors that shape the narratives.
- Researchers organize the key themes and findings into a logical and compelling narrative that tells the “core story” of the research participants’ experiences.
- This may involve selecting illustrative examples or quotes from the narratives to support and enrich the main points, and providing interpretive commentary to guide the reader’s understanding.
- Researchers should aim to create a narrative that is both faithful to the complexity and diversity of the participants’ experiences and clear and accessible to the intended audience.
- In telling the core narrative, researchers should also consider the broader implications and significance of their findings, both for the specific field of study and for understanding human experience more generally.
- This may involve discussing how the findings relate to existing theories or debates in the field, identifying new questions or directions for future research, or highlighting the practical applications or social relevance of the study.
Ethical Considerations in Narrative Analysis
Researchers face the challenge of balancing the need to provide faithful accounts of participant stories with the ethical obligation to interpret those stories in a way that respects the participants and avoids misrepresentation.
This requires nuance and sensitivity, acknowledging the ambiguities inherent in narrative data.
Reflexivity and Positionality
Researchers should acknowledge their role in shaping all aspects of the research process, including the interpretation of narratives.
Researchers need to be aware of their own subjectivity and how their experiences, assumptions, and perspectives could influence their interpretations of participants’ narratives.
This awareness, often referred to as reflexivity, involves critically examining one’s own assumptions and being conscious of potential biases throughout every stage of the research process.
Researchers are encouraged to maintain field journals to track their thoughts and experiences, which can provide valuable insights into their influence on the research.
- Transparency is Crucial: Researchers must be transparent about their positionality, clearly articulating how their background and perspectives have shaped their understanding of the data.
- Reflexive Journals: Researchers can utilize reflexive journals to document feelings and thoughts throughout the research process, particularly during data analysis, helping to distinguish personal biases from participant perspectives.
- Team-Based Reflexivity: In team-based research, researchers should engage in open communication with their colleagues, sharing their reflexive insights and perspectives to ensure a well-rounded understanding of the data.
Respecting Participants’ Voices
Ethical narrative analysis emphasizes the importance of representing participants’ stories in a way that is true to their experiences.
Ethical narrative analysis prioritizes representing participants’ stories in a manner that accurately reflects their lived experiences, ensuring their voices are heard and their perspectives are not misrepresented.
This can include involving participants in the interpretation of their narratives and giving them a voice in how their stories are shared.
This can involve:
- Participant Involvement: Researchers can involve participants in the interpretation of their narratives, giving them a voice in deciding how their stories are shared [VI, 15].
- Member Checking: Sharing transcripts, analyses, and publications with research participants is a common practice in narrative research, allowing for further dialogue and ensuring accurate representation.
- Collaborative Meaning-Making: Researchers should approach interviews as opportunities for collaborative meaning-making, recognizing that interviewees have their own agendas and interpretations of the interactions. Researchers should validate participant experiences without judgment, encouraging them to tell their stories authentically.
- Ethical Interviewing: Researchers must adopt ethical interviewing practices, gaining informed consent, guaranteeing anonymity, and being sensitive to potential distress caused by interview questions.
Strengths of Narrative Analysis
Narrative analysis is a powerful tool for qualitative research, offering several strengths.
- Rich Insights into Human Experience : Narrative analysis stands out for its ability to generate rich, nuanced insights into the complexities of human experience. Unlike other methods that might overlook individual perspectives, narrative analysis centers on personal stories, capturing the unique ways individuals perceive, interpret, and make sense of their lives and experiences.
- Exploring Underlying Meanings : This method enables researchers to go beyond superficial descriptions, uncovering the underlying meanings, motivations, and interpretations embedded within personal narratives. By examining the stories people tell, researchers can gain a deeper understanding of the beliefs, values, and cultural contexts that shape those experiences.
- Versatility and Broad Applications : Narrative analysis offers flexibility in its application, proving valuable for a wide range of research topics, particularly those focused on social, personal, cultural, or ideological phenomena. This approach proves particularly well-suited for exploring topics where individual perspectives and experiences are central to understanding the phenomenon under investigation.
- Democratizing Data Analysis : By focusing on the narratives of individuals, narrative analysis offers a democratizing approach to research. This method values the insights and interpretations individuals have about their own experiences, often contrasting with broader societal, cultural, and political factors. This approach acknowledges that individuals possess valuable understandings of their own lives, contributing to a more comprehensive and inclusive research process.
Let’s illustrate these strengths with a specific research example. Imagine investigating the experiences and beliefs of individuals facing social marginalization.
Narrative analysis, in this context, would allow researchers to closely examine the stories told by people within marginalized groups.
By identifying recurring patterns, symbols, or motifs within their narratives, researchers could shed light on how these individuals make sense of their experiences, revealing the often-hidden impacts of social marginalization.
Weaknesses of Narrative Analysis
- It can be time-consuming: Narrative analysis can require a significant time investment to analyze source data, especially when long-form stories are involved. Researchers must also be knowledgeable about the author’s cultural context and consider other interpretations of the narrative.
- Reliability and generalizability are limited: Because narrative analysis relies heavily on subjective interpretation of the narrative, the findings cannot usually be generalized to larger populations or empirically verified. Although conclusions about the cultural context might be drawn, they are based on anecdotal data, making them unsuitable as a basis for theory development.
- Labov’s model is not appropriate for all types of narratives: While Labov’s model can be useful for analyzing monological narratives, it is not suitable for conversational narratives, interactional discourses, or co-constructed stories. This is because the model primarily focuses on analyzing monological narratives collected through interviews like oral histories or life stories, rather than conversational interviews.
- Timelines may oversimplify life stories: While timelines can be a useful tool for organizing large amounts of narrative data, they have limitations. Summarizing and quantifying narrative data in this way risks reducing the complexity and oversimplifying the stories of individuals. Additionally, timelines may not fully capture the episodic nature of narratives, which often unfold non-linearly.
Further Information
For narrative analysis.
- Bamberg, M. (2006) Stories: Big or small. Why do we care? Narrative Inquiry, 16(1):139–147.
- Bamberg, M. (2012) Narrative analysis, in H. Cooper, P.M. Camic, D.L. Long, A.T. Panter, D. Rindskopf and K. Sher (eds), APA Handbook of Research Methods in Psychology, Vol. 2. Washington, DC: American Psychological Association, pp. 85–102.
- De Fina, A., & Georgakopoulou, A. (2012). Analyzing narrative Discourse and sociolinguistic perspectives Cambridge, UK: Cambridge University Press
- Gee, P. (2011). An introduction to discourse analysis: Theory and method (3rd ed.). New York, NY: Routledge.
- Holstein, J., & Gubrium, J. (Eds.). (2012). Varieties of narrative analysis. Thousand Oaks, CA: Sage
- Riessman, C. K. (2008). Narrative methods for the human sciences. Thousand Oaks, CA: Sage
LABOVIAN MODEL
Labov’s Narrative Model, developed by sociolinguist William Labov, is a structural approach to analyzing narratives that focuses on the formal properties and organizational features of stories.
Labov identified six key elements that he argued are present in fully-formed oral narratives: abstract, orientation, complicating action, evaluation, resolution, and coda.
- Labov, W. (1997). Further steps in narrative analysis. Journal of Narrative and Life History (7 ),395–415.
- Labov, W. and Waletzky J. (1997) Narrative analysis: Oral versions of personal experience. Journal of Narrative and Life History, 7 (1–4): 3–38.
- McCormack, C. (2004). Storying stories: a narrative approach to in-depth interview conversations. International journal of social research methodology , 7 (3), 219-236.
- Patterson, W. (2008). Narratives of events: Labovian narrative analysis and its limitations. Doing narrative research , 22-40.
POLKINGHORNE MODEL
The Polkinghorne Model, developed by psychologist Donald Polkinghorne, is a narrative approach to understanding human experience and meaning-making.
According to Polkinghorne, narratives are not simply a way of representing or communicating experience, but are the primary means through which we construct and make sense of our lives.
He argued that narratives are a fundamental form of human cognition, and that we use stories to organize and interpret our experiences, to create coherence and continuity in our sense of self, and to navigate the social and cultural worlds we inhabit.
One of the key features of the Polkinghorne Model is its emphasis on the interpretive and constructivist nature of narrative analysis.
Polkinghorne argued that narratives are not simply a reflection of an objective reality, but are always shaped by the social, cultural, and historical contexts in which they are told, as well as by the individual’s own perspective and meaning-making processes.
- Polkinghorne, D. E. (1995). Narrative configuration in qualitative analysis. International journal of qualitative studies in education , 8 (1), 5-23.
- Polkinghorne, D. (1988). Narrative knowing and the human sciences . Suny Press.
- Polkinghorne, D. E. (2007). Validity issues in narrative research. Qualitative inquiry , 13 (4), 471-486.
MISHLER MODEL
Elliot Mishler, a social psychologist and professor at Harvard Medical School, developed an influential model for analyzing narratives in the context of medical encounters.
The Mishler Model, also known as the “Narrative Functions Model,” focuses on the interactive and collaborative nature of storytelling in medical interviews, and examines how patients and healthcare providers co-construct meaning through their dialogue.
- Mishler, E. G. (1995). Models of narrative analysis: A typology. Journal of narrative and life history , 5 (2), 87-123.
- Mishler, E. G. (1986). The analysis of interview-narratives (pp. 233-255). TR Sarbin (Ed.), Narrative psychology: The storied nature of human conduct.
- Mishler, E. G. (2009). Storylines . Harvard University Press.
- Mishler, E. G. (1991). Research interviewing: Context and narrative . Harvard university press.
FOR VISUAL NARRATIVE ANALYSIS
- Bell, 5. E. (2002), Photo images: Jo Spence’s narratives of Journal for the Social Study of Health, Illness and with illness. Health An Interdisciplinary by post, 6 (1), 5-30.
- Pink, 5. (2004) Visual methods in C. Seale, G. Gobo, obrium, & D. Silverman (Eds), [Special issue) Qualitative Research Practice (pp. 361-378). London: Sage
- Adams, H. L. (2015). Insights into processes of posttraumatic growth through narrative analysis of chronic illness stories. Qualitative Psychology , 2 (2), 111.
- Ehsan, N., Riaz, M., & Khalily, T. (2019). Trauma of terror and displacement: A narrative analysis of mental health of women IDPS in KPK (Pakistan). Peace and Conflict: Journal of Peace Psychology , 25 (2), 140.
- Fewings, E., & Quinlan, E. (2023). “It hasn’t gone away after 30 years.” late-career Australian psychologists’ experience of uncertainty throughout their career . Professional Psychology: Research and Practice, 54 (3), 221–230.
- Skopp, N. A., Holland, K. M., Logan, J. E., Alexander, C. L., & Floyd, C. F. (2019). Circumstances preceding suicide in US soldiers: A qualitative analysis of narrative data. Psychological services , 16 (2), 302.

- Privacy Policy

Home » Narrative Analysis – Types, Methods and Examples
Narrative Analysis – Types, Methods and Examples
Table of Contents

Narrative Analysis
Definition:
Narrative analysis is a qualitative research methodology that involves examining and interpreting the stories or narratives people tell in order to gain insights into the meanings, experiences, and perspectives that underlie them. Narrative analysis can be applied to various forms of communication, including written texts, oral interviews, and visual media.
In narrative analysis, researchers typically examine the structure, content, and context of the narratives they are studying, paying close attention to the language, themes, and symbols used by the storytellers. They may also look for patterns or recurring motifs within the narratives, and consider the cultural and social contexts in which they are situated.
Types of Narrative Analysis
Types of Narrative Analysis are as follows:
Content Analysis
This type of narrative analysis involves examining the content of a narrative in order to identify themes, motifs, and other patterns. Researchers may use coding schemes to identify specific themes or categories within the text, and then analyze how they are related to each other and to the overall narrative. Content analysis can be used to study various forms of communication, including written texts, oral interviews, and visual media.
Structural Analysis
This type of narrative analysis focuses on the formal structure of a narrative, including its plot, character development, and use of literary devices. Researchers may analyze the narrative arc, the relationship between the protagonist and antagonist, or the use of symbolism and metaphor. Structural analysis can be useful for understanding how a narrative is constructed and how it affects the reader or audience.
Discourse Analysis
This type of narrative analysis focuses on the language and discourse used in a narrative, including the social and cultural context in which it is situated. Researchers may analyze the use of specific words or phrases, the tone and style of the narrative, or the ways in which social and cultural norms are reflected in the narrative. Discourse analysis can be useful for understanding how narratives are influenced by larger social and cultural structures.
Phenomenological Analysis
This type of narrative analysis focuses on the subjective experience of the narrator, and how they interpret and make sense of their experiences. Researchers may analyze the language used to describe experiences, the emotions expressed in the narrative, or the ways in which the narrator constructs meaning from their experiences. Phenomenological analysis can be useful for understanding how people make sense of their own lives and experiences.
Critical Analysis
This type of narrative analysis involves examining the political, social, and ideological implications of a narrative, and questioning its underlying assumptions and values. Researchers may analyze the ways in which a narrative reflects or reinforces dominant power structures, or how it challenges or subverts those structures. Critical analysis can be useful for understanding the role that narratives play in shaping social and cultural norms.
Autoethnography
This type of narrative analysis involves using personal narratives to explore cultural experiences and identity formation. Researchers may use their own personal narratives to explore issues such as race, gender, or sexuality, and to understand how larger social and cultural structures shape individual experiences. Autoethnography can be useful for understanding how individuals negotiate and navigate complex cultural identities.
Thematic Analysis
This method involves identifying themes or patterns that emerge from the data, and then interpreting these themes in relation to the research question. Researchers may use a deductive approach, where they start with a pre-existing theoretical framework, or an inductive approach, where themes are generated from the data itself.
Narrative Analysis Conducting Guide
Here are some steps for conducting narrative analysis:
- Identify the research question: Narrative analysis begins with identifying the research question or topic of interest. Researchers may want to explore a particular social or cultural phenomenon, or gain a deeper understanding of a particular individual’s experience.
- Collect the narratives: Researchers then collect the narratives or stories that they will analyze. This can involve collecting written texts, conducting interviews, or analyzing visual media.
- Transcribe and code the narratives: Once the narratives have been collected, they are transcribed into a written format, and then coded in order to identify themes, motifs, or other patterns. Researchers may use a coding scheme that has been developed specifically for the study, or they may use an existing coding scheme.
- Analyze the narratives: Researchers then analyze the narratives, focusing on the themes, motifs, and other patterns that have emerged from the coding process. They may also analyze the formal structure of the narratives, the language used, and the social and cultural context in which they are situated.
- Interpret the findings: Finally, researchers interpret the findings of the narrative analysis, and draw conclusions about the meanings, experiences, and perspectives that underlie the narratives. They may use the findings to develop theories, make recommendations, or inform further research.
Applications of Narrative Analysis
Narrative analysis is a versatile qualitative research method that has applications across a wide range of fields, including psychology, sociology, anthropology, literature, and history. Here are some examples of how narrative analysis can be used:
- Understanding individuals’ experiences: Narrative analysis can be used to gain a deeper understanding of individuals’ experiences, including their thoughts, feelings, and perspectives. For example, psychologists might use narrative analysis to explore the stories that individuals tell about their experiences with mental illness.
- Exploring cultural and social phenomena: Narrative analysis can also be used to explore cultural and social phenomena, such as gender, race, and identity. Sociologists might use narrative analysis to examine how individuals understand and experience their gender identity.
- Analyzing historical events: Narrative analysis can be used to analyze historical events, including those that have been recorded in literary texts or personal accounts. Historians might use narrative analysis to explore the stories of survivors of historical traumas, such as war or genocide.
- Examining media representations: Narrative analysis can be used to examine media representations of social and cultural phenomena, such as news stories, films, or television shows. Communication scholars might use narrative analysis to examine how news media represent different social groups.
- Developing interventions: Narrative analysis can be used to develop interventions to address social and cultural problems. For example, social workers might use narrative analysis to understand the experiences of individuals who have experienced domestic violence, and then use that knowledge to develop more effective interventions.
Examples of Narrative Analysis
Here are some examples of how narrative analysis has been used in research:
- Personal narratives of illness: Researchers have used narrative analysis to examine the personal narratives of individuals living with chronic illness, to understand how they make sense of their experiences and construct their identities.
- Oral histories: Historians have used narrative analysis to analyze oral histories to gain insights into individuals’ experiences of historical events and social movements.
- Children’s stories: Researchers have used narrative analysis to analyze children’s stories to understand how they understand and make sense of the world around them.
- Personal diaries : Researchers have used narrative analysis to examine personal diaries to gain insights into individuals’ experiences of significant life events, such as the loss of a loved one or the transition to adulthood.
- Memoirs : Researchers have used narrative analysis to analyze memoirs to understand how individuals construct their life stories and make sense of their experiences.
- Life histories : Researchers have used narrative analysis to examine life histories to gain insights into individuals’ experiences of migration, displacement, or social exclusion.
Purpose of Narrative Analysis
The purpose of narrative analysis is to gain a deeper understanding of the stories that individuals tell about their experiences, identities, and beliefs. By analyzing the structure, content, and context of these stories, researchers can uncover patterns and themes that shed light on the ways in which individuals make sense of their lives and the world around them.
The primary purpose of narrative analysis is to explore the meanings that individuals attach to their experiences. This involves examining the different elements of a story, such as the plot, characters, setting, and themes, to identify the underlying values, beliefs, and attitudes that shape the story. By analyzing these elements, researchers can gain insights into the ways in which individuals construct their identities, understand their relationships with others, and make sense of the world.
Narrative analysis can also be used to identify patterns and themes across multiple stories. This involves comparing and contrasting the stories of different individuals or groups to identify commonalities and differences. By analyzing these patterns and themes, researchers can gain insights into broader cultural and social phenomena, such as gender, race, and identity.
In addition, narrative analysis can be used to develop interventions that address social and cultural problems. By understanding the stories that individuals tell about their experiences, researchers can develop interventions that are tailored to the unique needs of different individuals and groups.
Overall, the purpose of narrative analysis is to provide a rich, nuanced understanding of the ways in which individuals construct meaning and make sense of their lives. By analyzing the stories that individuals tell, researchers can gain insights into the complex and multifaceted nature of human experience.
When to use Narrative Analysis
Here are some situations where narrative analysis may be appropriate:
- Studying life stories: Narrative analysis can be useful in understanding how individuals construct their life stories, including the events, characters, and themes that are important to them.
- Analyzing cultural narratives: Narrative analysis can be used to analyze cultural narratives, such as myths, legends, and folktales, to understand their meanings and functions.
- Exploring organizational narratives: Narrative analysis can be helpful in examining the stories that organizations tell about themselves, their histories, and their values, to understand how they shape the culture and practices of the organization.
- Investigating media narratives: Narrative analysis can be used to analyze media narratives, such as news stories, films, and TV shows, to understand how they construct meaning and influence public perceptions.
- Examining policy narratives: Narrative analysis can be helpful in examining policy narratives, such as political speeches and policy documents, to understand how they construct ideas and justify policy decisions.
Characteristics of Narrative Analysis
Here are some key characteristics of narrative analysis:
- Focus on stories and narratives: Narrative analysis is concerned with analyzing the stories and narratives that people tell, whether they are oral or written, to understand how they shape and reflect individuals’ experiences and identities.
- Emphasis on context: Narrative analysis seeks to understand the context in which the narratives are produced and the social and cultural factors that shape them.
- Interpretive approach: Narrative analysis is an interpretive approach that seeks to identify patterns and themes in the stories and narratives and to understand the meaning that individuals and communities attach to them.
- Iterative process: Narrative analysis involves an iterative process of analysis, in which the researcher continually refines their understanding of the narratives as they examine more data.
- Attention to language and form : Narrative analysis pays close attention to the language and form of the narratives, including the use of metaphor, imagery, and narrative structure, to understand the meaning that individuals and communities attach to them.
- Reflexivity : Narrative analysis requires the researcher to reflect on their own assumptions and biases and to consider how their own positionality may shape their interpretation of the narratives.
- Qualitative approach: Narrative analysis is typically a qualitative research method that involves in-depth analysis of a small number of cases rather than large-scale quantitative studies.
Advantages of Narrative Analysis
Here are some advantages of narrative analysis:
- Rich and detailed data : Narrative analysis provides rich and detailed data that allows for a deep understanding of individuals’ experiences, emotions, and identities.
- Humanizing approach: Narrative analysis allows individuals to tell their own stories and express their own perspectives, which can help to humanize research and give voice to marginalized communities.
- Holistic understanding: Narrative analysis allows researchers to understand individuals’ experiences in their entirety, including the social, cultural, and historical contexts in which they occur.
- Flexibility : Narrative analysis is a flexible research method that can be applied to a wide range of contexts and research questions.
- Interpretive insights: Narrative analysis provides interpretive insights into the meanings that individuals attach to their experiences and the ways in which they construct their identities.
- Appropriate for sensitive topics: Narrative analysis can be particularly useful in researching sensitive topics, such as trauma or mental health, as it allows individuals to express their experiences in their own words and on their own terms.
- Can lead to policy implications: Narrative analysis can provide insights that can inform policy decisions and interventions, particularly in areas such as health, education, and social policy.
Limitations of Narrative Analysis
Here are some of the limitations of narrative analysis:
- Subjectivity : Narrative analysis relies on the interpretation of researchers, which can be influenced by their own biases and assumptions.
- Limited generalizability: Narrative analysis typically involves in-depth analysis of a small number of cases, which limits its generalizability to broader populations.
- Ethical considerations: The process of eliciting and analyzing narratives can raise ethical concerns, particularly when sensitive topics such as trauma or abuse are involved.
- Limited control over data collection: Narrative analysis often relies on data that is already available, such as interviews, oral histories, or written texts, which can limit the control that researchers have over the quality and completeness of the data.
- Time-consuming: Narrative analysis can be a time-consuming research method, particularly when analyzing large amounts of data.
- Interpretation challenges: Narrative analysis requires researchers to make complex interpretations of data, which can be challenging and time-consuming.
- Limited statistical analysis: Narrative analysis is typically a qualitative research method that does not lend itself well to statistical analysis.
About the author
Muhammad Hassan
Researcher, Academic Writer, Web developer
You may also like

Discriminant Analysis – Methods, Types and...

Histogram – Types, Examples and Making Guide

Graphical Methods – Types, Examples and Guide

Textual Analysis – Types, Examples and Guide

Inferential Statistics – Types, Methods and...

Grounded Theory – Methods, Examples and Guide

Narrative Analysis 101
Everything you need to know to get started
By: Ethar Al-Saraf (PhD)| Expert Reviewed By: Eunice Rautenbach (DTech) | March 2023
If you’re new to research, the host of qualitative analysis methods available to you can be a little overwhelming. In this post, we’ll unpack the sometimes slippery topic of narrative analysis . We’ll explain what it is, consider its strengths and weaknesses , and look at when and when not to use this analysis method.
Overview: Narrative Analysis
- What is narrative analysis (simple definition)
- The two overarching approaches
- The strengths & weaknesses of narrative analysis
- When (and when not) to use it
- Key takeaways
What Is Narrative Analysis?
Simply put, narrative analysis is a qualitative analysis method focused on interpreting human experiences and motivations by looking closely at the stories (the narratives) people tell in a particular context.
In other words, a narrative analysis interprets long-form participant responses or written stories as data, to uncover themes and meanings . That data could be taken from interviews, monologues, written stories, or even recordings. In other words, narrative analysis can be used on both primary and secondary data to provide evidence from the experiences described.
That’s all quite conceptual, so let’s look at an example of how narrative analysis could be used.
Let’s say you’re interested in researching the beliefs of a particular author on popular culture. In that case, you might identify the characters , plotlines , symbols and motifs used in their stories. You could then use narrative analysis to analyse these in combination and against the backdrop of the relevant context.
This would allow you to interpret the underlying meanings and implications in their writing, and what they reveal about the beliefs of the author. In other words, you’d look to understand the views of the author by analysing the narratives that run through their work.
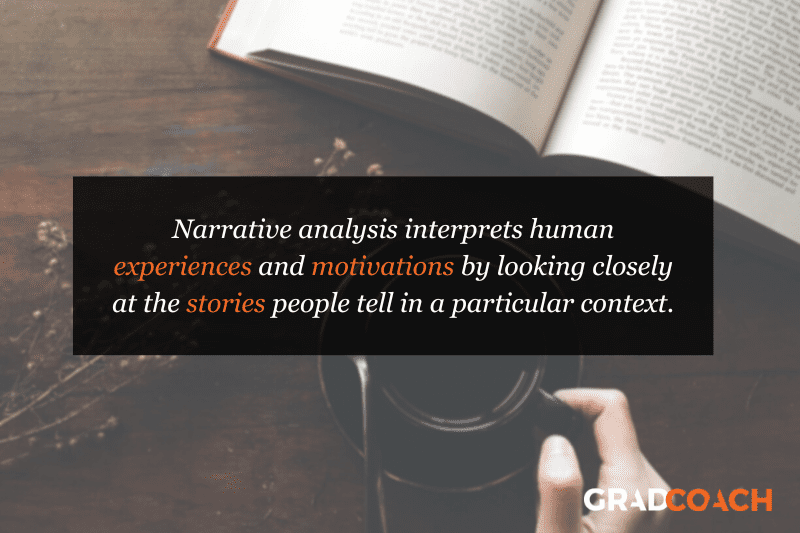
The Two Overarching Approaches
Generally speaking, there are two approaches that one can take to narrative analysis. Specifically, an inductive approach or a deductive approach. Each one will have a meaningful impact on how you interpret your data and the conclusions you can draw, so it’s important that you understand the difference.
First up is the inductive approach to narrative analysis.
The inductive approach takes a bottom-up view , allowing the data to speak for itself, without the influence of any preconceived notions . With this approach, you begin by looking at the data and deriving patterns and themes that can be used to explain the story, as opposed to viewing the data through the lens of pre-existing hypotheses, theories or frameworks. In other words, the analysis is led by the data.
For example, with an inductive approach, you might notice patterns or themes in the way an author presents their characters or develops their plot. You’d then observe these patterns, develop an interpretation of what they might reveal in the context of the story, and draw conclusions relative to the aims of your research.
Contrasted to this is the deductive approach.
With the deductive approach to narrative analysis, you begin by using existing theories that a narrative can be tested against . Here, the analysis adopts particular theoretical assumptions and/or provides hypotheses, and then looks for evidence in a story that will either verify or disprove them.
For example, your analysis might begin with a theory that wealthy authors only tell stories to get the sympathy of their readers. A deductive analysis might then look at the narratives of wealthy authors for evidence that will substantiate (or refute) the theory and then draw conclusions about its accuracy, and suggest explanations for why that might or might not be the case.
Which approach you should take depends on your research aims, objectives and research questions . If these are more exploratory in nature, you’ll likely take an inductive approach. Conversely, if they are more confirmatory in nature, you’ll likely opt for the deductive approach.
Need a helping hand?
Strengths & Weaknesses
Now that we have a clearer view of what narrative analysis is and the two approaches to it, it’s important to understand its strengths and weaknesses , so that you can make the right choices in your research project.
A primary strength of narrative analysis is the rich insight it can generate by uncovering the underlying meanings and interpretations of human experience. The focus on an individual narrative highlights the nuances and complexities of their experience, revealing details that might be missed or considered insignificant by other methods.
Another strength of narrative analysis is the range of topics it can be used for. The focus on human experience means that a narrative analysis can democratise your data analysis, by revealing the value of individuals’ own interpretation of their experience in contrast to broader social, cultural, and political factors.
All that said, just like all analysis methods, narrative analysis has its weaknesses. It’s important to understand these so that you can choose the most appropriate method for your particular research project.
The first drawback of narrative analysis is the problem of subjectivity and interpretation . In other words, a drawback of the focus on stories and their details is that they’re open to being understood differently depending on who’s reading them. This means that a strong understanding of the author’s cultural context is crucial to developing your interpretation of the data. At the same time, it’s important that you remain open-minded in how you interpret your chosen narrative and avoid making any assumptions .
A second weakness of narrative analysis is the issue of reliability and generalisation . Since narrative analysis depends almost entirely on a subjective narrative and your interpretation, the findings and conclusions can’t usually be generalised or empirically verified. Although some conclusions can be drawn about the cultural context, they’re still based on what will almost always be anecdotal data and not suitable for the basis of a theory, for example.
Last but not least, the focus on long-form data expressed as stories means that narrative analysis can be very time-consuming . In addition to the source data itself, you will have to be well informed on the author’s cultural context as well as other interpretations of the narrative, where possible, to ensure you have a holistic view. So, if you’re going to undertake narrative analysis, make sure that you allocate a generous amount of time to work through the data.
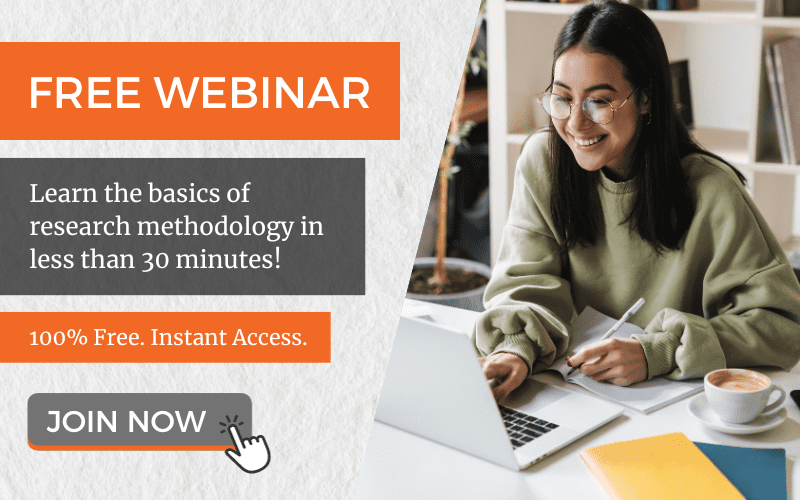
When To Use Narrative Analysis
As a qualitative method focused on analysing and interpreting narratives describing human experiences, narrative analysis is usually most appropriate for research topics focused on social, personal, cultural , or even ideological events or phenomena and how they’re understood at an individual level.
For example, if you were interested in understanding the experiences and beliefs of individuals suffering social marginalisation, you could use narrative analysis to look at the narratives and stories told by people in marginalised groups to identify patterns , symbols , or motifs that shed light on how they rationalise their experiences.
In this example, narrative analysis presents a good natural fit as it’s focused on analysing people’s stories to understand their views and beliefs at an individual level. Conversely, if your research was geared towards understanding broader themes and patterns regarding an event or phenomena, analysis methods such as content analysis or thematic analysis may be better suited, depending on your research aim .
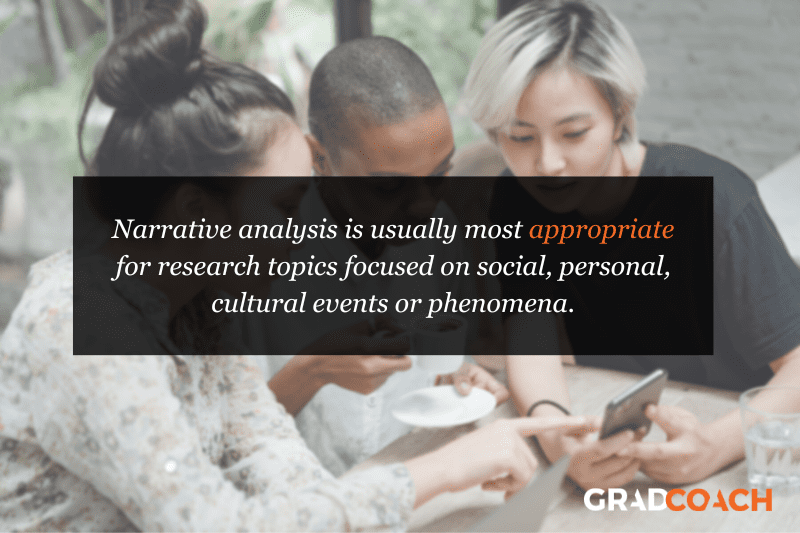
Let’s recap
In this post, we’ve explored the basics of narrative analysis in qualitative research. The key takeaways are:
- Narrative analysis is a qualitative analysis method focused on interpreting human experience in the form of stories or narratives .
- There are two overarching approaches to narrative analysis: the inductive (exploratory) approach and the deductive (confirmatory) approach.
- Like all analysis methods, narrative analysis has a particular set of strengths and weaknesses .
- Narrative analysis is generally most appropriate for research focused on interpreting individual, human experiences as expressed in detailed , long-form accounts.
If you’d like to learn more about narrative analysis and qualitative analysis methods in general, be sure to check out the rest of the Grad Coach blog here . Alternatively, if you’re looking for hands-on help with your project, take a look at our 1-on-1 private coaching service .
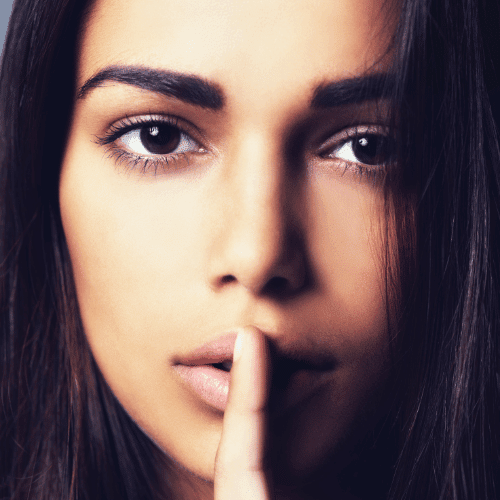
Psst... there’s more!
This post was based on one of our popular Research Bootcamps . If you're working on a research project, you'll definitely want to check this out ...
Thanks. I need examples of narrative analysis
Here are some examples of research topics that could utilise narrative analysis:
Personal Narratives of Trauma: Analysing personal stories of individuals who have experienced trauma to understand the impact, coping mechanisms, and healing processes.
Identity Formation in Immigrant Communities: Examining the narratives of immigrants to explore how they construct and negotiate their identities in a new cultural context.
Media Representations of Gender: Analysing narratives in media texts (such as films, television shows, or advertisements) to investigate the portrayal of gender roles, stereotypes, and power dynamics.
Where can I find an example of a narrative analysis table ?
Please i need help with my project,
how can I cite this article in APA 7th style?
please mention the sources as well.
My research is mixed approach. I use interview,key_inforamt interview,FGD and document.so,which qualitative analysis is appropriate to analyze these data.Thanks
Which qualitative analysis methode is appropriate to analyze data obtain from intetview,key informant intetview,Focus group discussion and document.
I’ve finished my PhD. Now I need a “platform” that will help me objectively ascertain the tacit assumptions that are buried within a narrative. Can you help?
Submit a Comment Cancel reply
Your email address will not be published. Required fields are marked *
Save my name, email, and website in this browser for the next time I comment.
- Print Friendly
Using narrative analysis in qualitative research
Last updated
7 March 2023
Reviewed by
Jean Kaluza
Short on time? Get an AI generated summary of this article instead
After spending considerable time and effort interviewing persons for research, you want to ensure you get the most out of the data you gathered. One method that gives you an excellent opportunity to connect with your data on a very human and personal level is a narrative analysis in qualitative research.
Master narrative analysis
Analyze your qualitative data faster and surface more actionable insights
- What is narrative analysis?
Narrative analysis is a type of qualitative data analysis that focuses on interpreting the core narratives from a study group's personal stories. Using first-person narrative, data is acquired and organized to allow the researcher to understand how the individuals experienced something.
Instead of focusing on just the actual words used during an interview, the narrative analysis also allows for a compilation of data on how the person expressed themselves, what language they used when describing a particular event or feeling, and the thoughts and motivations they experienced. A narrative analysis will also consider how the research participants constructed their narratives.
From the interview to coding , you should strive to keep the entire individual narrative together, so that the information shared during the interview remains intact.
Is narrative analysis qualitative or quantitative?
Narrative analysis is a qualitative research method.
Is narrative analysis a method or methodology?
A method describes the tools or processes used to understand your data; methodology describes the overall framework used to support the methods chosen. By this definition, narrative analysis can be both a method used to understand data and a methodology appropriate for approaching data that comes primarily from first-person stories.
- Do you need to perform narrative research to conduct a narrative analysis?
A narrative analysis will give the best answers about the data if you begin with conducting narrative research. Narrative research explores an entire story with a research participant to understand their personal story.
What are the characteristics of narrative research?
Narrative research always includes data from individuals that tell the story of their experiences. This is captured using loosely structured interviews . These can be a single interview or a series of long interviews over a period of time. Narrative research focuses on the construct and expressions of the story as experienced by the research participant.
- Examples of types of narratives
Narrative data is based on narratives. Your data may include the entire life story or a complete personal narrative, giving a comprehensive account of someone's life, depending on the researched subject. Alternatively, a topical story can provide context around one specific moment in the research participant's life.
Personal narratives can be single or multiple sessions, encompassing more than topical stories but not entire life stories of the individuals.
- What is the objective of narrative analysis?
The narrative analysis seeks to organize the overall experience of a group of research participants' stories. The goal is to turn people's individual narratives into data that can be coded and organized so that researchers can easily understand the impact of a certain event, feeling, or decision on the involved persons. At the end of a narrative analysis, researchers can identify certain core narratives that capture the human experience.
What is the difference between content analysis and narrative analysis?
Content analysis is a research method that determines how often certain words, concepts, or themes appear inside a sampling of qualitative data . The narrative analysis focuses on the overall story and organizing the constructs and features of a narrative.
Free AI content analysis generator
Make sense of your research by automatically summarizing key takeaways through our free content analysis tool.
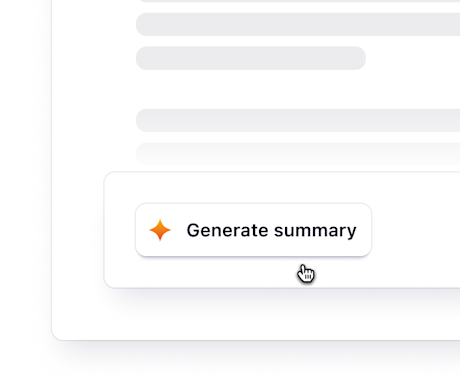
What is the difference between narrative analysis and case study in qualitative research?
A case study focuses on one particular event. A narrative analysis draws from a larger amount of data surrounding the entire narrative, including the thoughts that led up to a decision and the personal conclusion of the research participant.
A case study, therefore, is any specific topic studied in depth, whereas narrative analysis explores single or multi-faceted experiences across time.
What is the difference between narrative analysis and thematic analysis?
A thematic analysis will appear as researchers review the available qualitative data and note any recurring themes. Unlike narrative analysis, which describes an entire method of evaluating data to find a conclusion, a thematic analysis only describes reviewing and categorizing the data.
- Capturing narrative data
Because narrative data relies heavily on allowing a research participant to describe their experience, it is best to allow for a less structured interview. Allowing the participant to explore tangents or analyze their personal narrative will result in more complete data.
When collecting narrative data, always allow the participant the time and space needed to complete their narrative.
- Methods of transcribing narrative data
A narrative analysis requires that the researchers have access to the entire verbatim narrative of the participant, including not just the word they use but the pauses, the verbal tics, and verbal crutches, such as "um" and "hmm."
As the entire way the story is expressed is part of the data, a verbatim transcription should be created before attempting to code the narrative analysis.
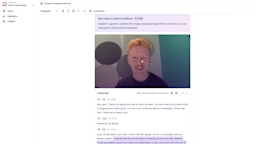
Video and audio transcription templates
- How to code narrative analysis
Coding narrative analysis has two natural start points, either using a deductive coding system or an inductive coding system. Regardless of your chosen method, it's crucial not to lose valuable data during the organization process.
When coding, expect to see more information in the code snippets.
- Types of narrative analysis
After coding is complete, you should expect your data to look like large blocks of text organized by the parts of the story. You will also see where individual narratives compare and diverge.
Inductive method
Using an inductive narrative method treats the entire narrative as one datum or one set of information. An inductive narrative method will encourage the research participant to organize their own story.
To make sense of how a story begins and ends, you must rely on cues from the participant. These may take the form of entrance and exit talks.
Participants may not always provide clear indicators of where their narratives start and end. However, you can anticipate that their stories will contain elements of a beginning, middle, and end. By analyzing these components through coding, you can identify emerging patterns in the data.
Taking cues from entrance and exit talk
Entrance talk is when the participant begins a particular set of narratives. You may hear expressions such as, "I remember when…," "It first occurred to me when…," or "Here's an example…."
Exit talk allows you to see when the story is wrapping up, and you might expect to hear a phrase like, "…and that's how we decided", "after that, we moved on," or "that's pretty much it."
Deductive method
Regardless of your chosen method, using a deductive method can help preserve the overall storyline while coding. Starting with a deductive method allows for the separation of narrative pieces without compromising the story's integrity.
Hybrid inductive and deductive narrative analysis
Using both methods together gives you a comprehensive understanding of the data. You can start by coding the entire story using the inductive method. Then, you can better analyze and interpret the data by applying deductive codes to individual parts of the story.
- How to analyze data after coding using narrative analysis
A narrative analysis aims to take all relevant interviews and organize them down to a few core narratives. After reviewing the coding, these core narratives may appear through a repeated moment of decision occurring before the climax or a key feeling that affected the participant's outcome.
You may see these core narratives diverge early on, or you may learn that a particular moment after introspection reveals the core narrative for each participant. Either way, researchers can now quickly express and understand the data you acquired.
- A step-by-step approach to narrative analysis and finding core narratives
Narrative analysis may look slightly different to each research group, but we will walk through the process using the Delve method for this article.
Step 1 – Code narrative blocks
Organize your narrative blocks using inductive coding to organize stories by a life event.
Example: Narrative interviews are conducted with homeowners asking them to describe how they bought their first home.
Step 2 – Group and read by live-event
You begin your data analysis by reading through each of the narratives coded with the same life event.
Example: You read through each homeowner's experience of buying their first home and notice that some common themes begin to appear, such as "we were tired of renting," "our family expanded to the point that we needed a larger space," and "we had finally saved enough for a downpayment."
Step 3 – Create a nested story structure
As these common narratives develop throughout the participant's interviews, create and nest code according to your narrative analysis framework. Use your coding to break down the narrative into pieces that can be analyzed together.
Example: During your interviews, you find that the beginning of the narrative usually includes the pressures faced before buying a home that pushes the research participants to consider homeownership. The middle of the narrative often includes challenges that come up during the decision-making process. The end of the narrative usually includes perspectives about the excitement, stress, or consequences of home ownership that has finally taken place.
Step 4 – Delve into the story structure
Once the narratives are organized into their pieces, you begin to notice how participants structure their own stories and where similarities and differences emerge.
Example: You find in your research that many people who choose to buy homes had the desire to buy a home before their circumstances allowed them to. You notice that almost all the stories begin with the feeling of some sort of outside pressure.
Step 5 – Compare across story structure
While breaking down narratives into smaller pieces is necessary for analysis, it's important not to lose sight of the overall story. To keep the big picture in mind, take breaks to step back and reread the entire narrative of a code block. This will help you remember how participants expressed themselves and ensure that the core narrative remains the focus of the analysis.
Example: By carefully examining the similarities across the beginnings of participants' narratives, you find the similarities in pressures. Considering the overall narrative, you notice how these pressures lead to similar decisions despite the challenges faced.
Divergence in feelings towards homeownership can be linked to positive or negative pressures. Individuals who received positive pressure, such as family support or excitement, may view homeownership more favorably. Meanwhile, negative pressures like high rent or peer pressure may cause individuals to have a more negative attitude toward homeownership.
These factors can contribute to the initial divergence in feelings towards homeownership.
Step 6 – Tell the core narrative
After carefully analyzing the data, you have found how the narratives relate and diverge. You may be able to create a theory about why the narratives diverge and can create one or two core narratives that explain the way the story was experienced.
Example: You can now construct a core narrative on how a person's initial feelings toward buying a house affect their feelings after purchasing and living in their first home.
Narrative analysis in qualitative research is an invaluable tool to understand how people's stories and ability to self-narrate reflect the human experience. Qualitative data analysis can be improved through coding and organizing complete narratives. By doing so, researchers can conclude how humans process and move through decisions and life events.
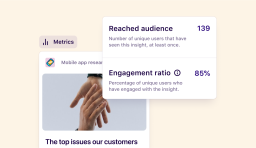
Learn more about qualitative transcription software
Should you be using a customer insights hub.
Do you want to discover previous research faster?
Do you share your research findings with others?
Do you analyze research data?
Start for free today, add your research, and get to key insights faster
Editor’s picks
Last updated: 18 April 2023
Last updated: 27 February 2023
Last updated: 6 February 2023
Last updated: 6 October 2023
Last updated: 5 February 2023
Last updated: 16 April 2023
Last updated: 9 March 2023
Last updated: 12 December 2023
Last updated: 11 March 2024
Last updated: 4 July 2024
Last updated: 6 March 2024
Last updated: 5 March 2024
Last updated: 13 May 2024
Latest articles
Related topics, .css-je19u9{-webkit-align-items:flex-end;-webkit-box-align:flex-end;-ms-flex-align:flex-end;align-items:flex-end;display:-webkit-box;display:-webkit-flex;display:-ms-flexbox;display:flex;-webkit-flex-direction:row;-ms-flex-direction:row;flex-direction:row;-webkit-box-flex-wrap:wrap;-webkit-flex-wrap:wrap;-ms-flex-wrap:wrap;flex-wrap:wrap;-webkit-box-pack:center;-ms-flex-pack:center;-webkit-justify-content:center;justify-content:center;row-gap:0;text-align:center;max-width:671px;}@media (max-width: 1079px){.css-je19u9{max-width:400px;}.css-je19u9>span{white-space:pre;}}@media (max-width: 799px){.css-je19u9{max-width:400px;}.css-je19u9>span{white-space:pre;}} decide what to .css-1kiodld{max-height:56px;display:-webkit-box;display:-webkit-flex;display:-ms-flexbox;display:flex;-webkit-align-items:center;-webkit-box-align:center;-ms-flex-align:center;align-items:center;}@media (max-width: 1079px){.css-1kiodld{display:none;}} build next, decide what to build next.

Users report unexpectedly high data usage, especially during streaming sessions.

Users find it hard to navigate from the home page to relevant playlists in the app.

It would be great to have a sleep timer feature, especially for bedtime listening.

I need better filters to find the songs or artists I’m looking for.
Log in or sign up
Get started for free
- Skip to main content
- Skip to primary sidebar
- Skip to footer
- QuestionPro

- Solutions Industries Gaming Automotive Sports and events Education Government Travel & Hospitality Financial Services Healthcare Cannabis Technology Use Case NPS+ Communities Audience Contactless surveys Mobile LivePolls Member Experience GDPR Positive People Science 360 Feedback Surveys
- Resources Blog eBooks Survey Templates Case Studies Training Help center

Home Market Research
Qualitative Data Analysis: What is it, Methods + Examples
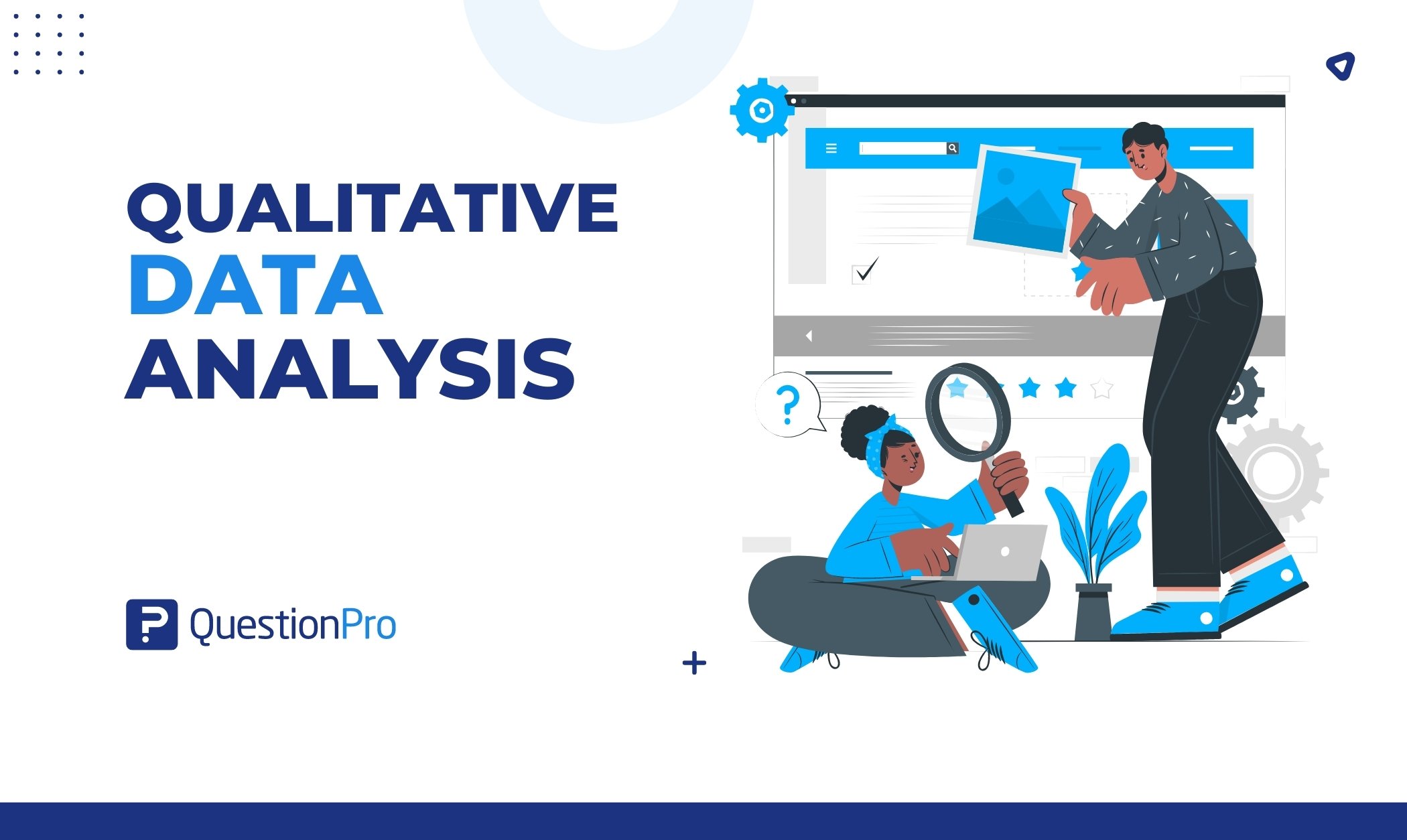
In a world rich with information and narrative, understanding the deeper layers of human experiences requires a unique vision that goes beyond numbers and figures. This is where the power of qualitative data analysis comes to light.
In this blog, we’ll learn about qualitative data analysis, explore its methods, and provide real-life examples showcasing its power in uncovering insights.
What is Qualitative Data Analysis?
Qualitative data analysis is a systematic process of examining non-numerical data to extract meaning, patterns, and insights.
In contrast to quantitative analysis, which focuses on numbers and statistical metrics, the qualitative study focuses on the qualitative aspects of data, such as text, images, audio, and videos. It seeks to understand every aspect of human experiences, perceptions, and behaviors by examining the data’s richness.
Companies frequently conduct this analysis on customer feedback. You can collect qualitative data from reviews, complaints, chat messages, interactions with support centers, customer interviews, case notes, or even social media comments. This kind of data holds the key to understanding customer sentiments and preferences in a way that goes beyond mere numbers.
Importance of Qualitative Data Analysis
Qualitative data analysis plays a crucial role in your research and decision-making process across various disciplines. Let’s explore some key reasons that underline the significance of this analysis:
In-Depth Understanding
It enables you to explore complex and nuanced aspects of a phenomenon, delving into the ‘how’ and ‘why’ questions. This method provides you with a deeper understanding of human behavior, experiences, and contexts that quantitative approaches might not capture fully.
Contextual Insight
You can use this analysis to give context to numerical data. It will help you understand the circumstances and conditions that influence participants’ thoughts, feelings, and actions. This contextual insight becomes essential for generating comprehensive explanations.
Theory Development
You can generate or refine hypotheses via qualitative data analysis. As you analyze the data attentively, you can form hypotheses, concepts, and frameworks that will drive your future research and contribute to theoretical advances.
Participant Perspectives
When performing qualitative research, you can highlight participant voices and opinions. This approach is especially useful for understanding marginalized or underrepresented people, as it allows them to communicate their experiences and points of view.
Exploratory Research
The analysis is frequently used at the exploratory stage of your project. It assists you in identifying important variables, developing research questions, and designing quantitative studies that will follow.
Types of Qualitative Data
When conducting qualitative research, you can use several qualitative data collection methods , and here you will come across many sorts of qualitative data that can provide you with unique insights into your study topic. These data kinds add new views and angles to your understanding and analysis.
Interviews and Focus Groups
Interviews and focus groups will be among your key methods for gathering qualitative data. Interviews are one-on-one talks in which participants can freely share their thoughts, experiences, and opinions.
Focus groups, on the other hand, are discussions in which members interact with one another, resulting in dynamic exchanges of ideas. Both methods provide rich qualitative data and direct access to participant perspectives.
Observations and Field Notes
Observations and field notes are another useful sort of qualitative data. You can immerse yourself in the research environment through direct observation, carefully documenting behaviors, interactions, and contextual factors.
These observations will be recorded in your field notes, providing a complete picture of the environment and the behaviors you’re researching. This data type is especially important for comprehending behavior in their natural setting.
Textual and Visual Data
Textual and visual data include a wide range of resources that can be qualitatively analyzed. Documents, written narratives, and transcripts from various sources, such as interviews or speeches, are examples of textual data.
Photographs, films, and even artwork provide a visual layer to your research. These forms of data allow you to investigate what is spoken and the underlying emotions, details, and symbols expressed by language or pictures.
When to Choose Qualitative Data Analysis over Quantitative Data Analysis
As you begin your research journey, understanding why the analysis of qualitative data is important will guide your approach to understanding complex events. If you analyze qualitative data, it will provide new insights that complement quantitative methodologies, which will give you a broader understanding of your study topic.
It is critical to know when to use qualitative analysis over quantitative procedures. You can prefer qualitative data analysis when:
- Complexity Reigns: When your research questions involve deep human experiences, motivations, or emotions, qualitative research excels at revealing these complexities.
- Exploration is Key: Qualitative analysis is ideal for exploratory research. It will assist you in understanding a new or poorly understood topic before formulating quantitative hypotheses.
- Context Matters: If you want to understand how context affects behaviors or results, qualitative data analysis provides the depth needed to grasp these relationships.
- Unanticipated Findings: When your study provides surprising new viewpoints or ideas, qualitative analysis helps you to delve deeply into these emerging themes.
- Subjective Interpretation is Vital: When it comes to understanding people’s subjective experiences and interpretations, qualitative data analysis is the way to go.
You can make informed decisions regarding the right approach for your research objectives if you understand the importance of qualitative analysis and recognize the situations where it shines.
Qualitative Data Analysis Methods and Examples
Exploring various qualitative data analysis methods will provide you with a wide collection for making sense of your research findings. Once the data has been collected, you can choose from several analysis methods based on your research objectives and the data type you’ve collected.
There are five main methods for analyzing qualitative data. Each method takes a distinct approach to identifying patterns, themes, and insights within your qualitative data. They are:
Method 1: Content Analysis
Content analysis is a methodical technique for analyzing textual or visual data in a structured manner. In this method, you will categorize qualitative data by splitting it into manageable pieces and assigning the manual coding process to these units.
As you go, you’ll notice ongoing codes and designs that will allow you to conclude the content. This method is very beneficial for detecting common ideas, concepts, or themes in your data without losing the context.
Steps to Do Content Analysis
Follow these steps when conducting content analysis:
- Collect and Immerse: Begin by collecting the necessary textual or visual data. Immerse yourself in this data to fully understand its content, context, and complexities.
- Assign Codes and Categories: Assign codes to relevant data sections that systematically represent major ideas or themes. Arrange comparable codes into groups that cover the major themes.
- Analyze and Interpret: Develop a structured framework from the categories and codes. Then, evaluate the data in the context of your research question, investigate relationships between categories, discover patterns, and draw meaning from these connections.
Benefits & Challenges
There are various advantages to using content analysis:
- Structured Approach: It offers a systematic approach to dealing with large data sets and ensures consistency throughout the research.
- Objective Insights: This method promotes objectivity, which helps to reduce potential biases in your study.
- Pattern Discovery: Content analysis can help uncover hidden trends, themes, and patterns that are not always obvious.
- Versatility: You can apply content analysis to various data formats, including text, internet content, images, etc.
However, keep in mind the challenges that arise:
- Subjectivity: Even with the best attempts, a certain bias may remain in coding and interpretation.
- Complexity: Analyzing huge data sets requires time and great attention to detail.
- Contextual Nuances: Content analysis may not capture all of the contextual richness that qualitative data analysis highlights.
Example of Content Analysis
Suppose you’re conducting market research and looking at customer feedback on a product. As you collect relevant data and analyze feedback, you’ll see repeating codes like “price,” “quality,” “customer service,” and “features.” These codes are organized into categories such as “positive reviews,” “negative reviews,” and “suggestions for improvement.”
According to your findings, themes such as “price” and “customer service” stand out and show that pricing and customer service greatly impact customer satisfaction. This example highlights the power of content analysis for obtaining significant insights from large textual data collections.
Method 2: Thematic Analysis
Thematic analysis is a well-structured procedure for identifying and analyzing recurring themes in your data. As you become more engaged in the data, you’ll generate codes or short labels representing key concepts. These codes are then organized into themes, providing a consistent framework for organizing and comprehending the substance of the data.
The analysis allows you to organize complex narratives and perspectives into meaningful categories, which will allow you to identify connections and patterns that may not be visible at first.
Steps to Do Thematic Analysis
Follow these steps when conducting a thematic analysis:
- Code and Group: Start by thoroughly examining the data and giving initial codes that identify the segments. To create initial themes, combine relevant codes.
- Code and Group: Begin by engaging yourself in the data, assigning first codes to notable segments. To construct basic themes, group comparable codes together.
- Analyze and Report: Analyze the data within each theme to derive relevant insights. Organize the topics into a consistent structure and explain your findings, along with data extracts that represent each theme.
Thematic analysis has various benefits:
- Structured Exploration: It is a method for identifying patterns and themes in complex qualitative data.
- Comprehensive knowledge: Thematic analysis promotes an in-depth understanding of the complications and meanings of the data.
- Application Flexibility: This method can be customized to various research situations and data kinds.
However, challenges may arise, such as:
- Interpretive Nature: Interpreting qualitative data in thematic analysis is vital, and it is critical to manage researcher bias.
- Time-consuming: The study can be time-consuming, especially with large data sets.
- Subjectivity: The selection of codes and topics might be subjective.
Example of Thematic Analysis
Assume you’re conducting a thematic analysis on job satisfaction interviews. Following your immersion in the data, you assign initial codes such as “work-life balance,” “career growth,” and “colleague relationships.” As you organize these codes, you’ll notice themes develop, such as “Factors Influencing Job Satisfaction” and “Impact on Work Engagement.”
Further investigation reveals the tales and experiences included within these themes and provides insights into how various elements influence job satisfaction. This example demonstrates how thematic analysis can reveal meaningful patterns and insights in qualitative data.
Method 3: Narrative Analysis
The narrative analysis involves the narratives that people share. You’ll investigate the histories in your data, looking at how stories are created and the meanings they express. This method is excellent for learning how people make sense of their experiences through narrative.
Steps to Do Narrative Analysis
The following steps are involved in narrative analysis:
- Gather and Analyze: Start by collecting narratives, such as first-person tales, interviews, or written accounts. Analyze the stories, focusing on the plot, feelings, and characters.
- Find Themes: Look for recurring themes or patterns in various narratives. Think about the similarities and differences between these topics and personal experiences.
- Interpret and Extract Insights: Contextualize the narratives within their larger context. Accept the subjective nature of each narrative and analyze the narrator’s voice and style. Extract insights from the tales by diving into the emotions, motivations, and implications communicated by the stories.
There are various advantages to narrative analysis:
- Deep Exploration: It lets you look deeply into people’s personal experiences and perspectives.
- Human-Centered: This method prioritizes the human perspective, allowing individuals to express themselves.
However, difficulties may arise, such as:
- Interpretive Complexity: Analyzing narratives requires dealing with the complexities of meaning and interpretation.
- Time-consuming: Because of the richness and complexities of tales, working with them can be time-consuming.
Example of Narrative Analysis
Assume you’re conducting narrative analysis on refugee interviews. As you read the stories, you’ll notice common themes of toughness, loss, and hope. The narratives provide insight into the obstacles that refugees face, their strengths, and the dreams that guide them.
The analysis can provide a deeper insight into the refugees’ experiences and the broader social context they navigate by examining the narratives’ emotional subtleties and underlying meanings. This example highlights how narrative analysis can reveal important insights into human stories.
Method 4: Grounded Theory Analysis
Grounded theory analysis is an iterative and systematic approach that allows you to create theories directly from data without being limited by pre-existing hypotheses. With an open mind, you collect data and generate early codes and labels that capture essential ideas or concepts within the data.
As you progress, you refine these codes and increasingly connect them, eventually developing a theory based on the data. Grounded theory analysis is a dynamic process for developing new insights and hypotheses based on details in your data.
Steps to Do Grounded Theory Analysis
Grounded theory analysis requires the following steps:
- Initial Coding: First, immerse yourself in the data, producing initial codes that represent major concepts or patterns.
- Categorize and Connect: Using axial coding, organize the initial codes, which establish relationships and connections between topics.
- Build the Theory: Focus on creating a core category that connects the codes and themes. Regularly refine the theory by comparing and integrating new data, ensuring that it evolves organically from the data.
Grounded theory analysis has various benefits:
- Theory Generation: It provides a one-of-a-kind opportunity to generate hypotheses straight from data and promotes new insights.
- In-depth Understanding: The analysis allows you to deeply analyze the data and reveal complex relationships and patterns.
- Flexible Process: This method is customizable and ongoing, which allows you to enhance your research as you collect additional data.
However, challenges might arise with:
- Time and Resources: Because grounded theory analysis is a continuous process, it requires a large commitment of time and resources.
- Theoretical Development: Creating a grounded theory involves a thorough understanding of qualitative data analysis software and theoretical concepts.
- Interpretation of Complexity: Interpreting and incorporating a newly developed theory into existing literature can be intellectually hard.
Example of Grounded Theory Analysis
Assume you’re performing a grounded theory analysis on workplace collaboration interviews. As you open code the data, you will discover notions such as “communication barriers,” “team dynamics,” and “leadership roles.” Axial coding demonstrates links between these notions, emphasizing the significance of efficient communication in developing collaboration.
You create the core “Integrated Communication Strategies” category through selective coding, which unifies new topics.
This theory-driven category serves as the framework for understanding how numerous aspects contribute to effective team collaboration. This example shows how grounded theory analysis allows you to generate a theory directly from the inherent nature of the data.
Method 5: Discourse Analysis
Discourse analysis focuses on language and communication. You’ll look at how language produces meaning and how it reflects power relations, identities, and cultural influences. This strategy examines what is said and how it is said; the words, phrasing, and larger context of communication.
The analysis is precious when investigating power dynamics, identities, and cultural influences encoded in language. By evaluating the language used in your data, you can identify underlying assumptions, cultural standards, and how individuals negotiate meaning through communication.
Steps to Do Discourse Analysis
Conducting discourse analysis entails the following steps:
- Select Discourse: For analysis, choose language-based data such as texts, speeches, or media content.
- Analyze Language: Immerse yourself in the conversation, examining language choices, metaphors, and underlying assumptions.
- Discover Patterns: Recognize the dialogue’s reoccurring themes, ideologies, and power dynamics. To fully understand the effects of these patterns, put them in their larger context.
There are various advantages of using discourse analysis:
- Understanding Language: It provides an extensive understanding of how language builds meaning and influences perceptions.
- Uncovering Power Dynamics: The analysis reveals how power dynamics appear via language.
- Cultural Insights: This method identifies cultural norms, beliefs, and ideologies stored in communication.
However, the following challenges may arise:
- Complexity of Interpretation: Language analysis involves navigating multiple levels of nuance and interpretation.
- Subjectivity: Interpretation can be subjective, so controlling researcher bias is important.
- Time-Intensive: Discourse analysis can take a lot of time because careful linguistic study is required in this analysis.

Example of Discourse Analysis
Consider doing discourse analysis on media coverage of a political event. You notice repeating linguistic patterns in news articles that depict the event as a conflict between opposing parties. Through deconstruction, you can expose how this framing supports particular ideologies and power relations.
You can illustrate how language choices influence public perceptions and contribute to building the narrative around the event by analyzing the speech within the broader political and social context. This example shows how discourse analysis can reveal hidden power dynamics and cultural influences on communication.
How to do Qualitative Data Analysis with the QuestionPro Research suite?
QuestionPro is a popular survey and research platform that offers tools for collecting and analyzing qualitative and quantitative data. Follow these general steps for conducting qualitative data analysis using the QuestionPro Research Suite:
- Collect Qualitative Data: Set up your survey to capture qualitative responses. It might involve open-ended questions, text boxes, or comment sections where participants can provide detailed responses.
- Export Qualitative Responses: Export the responses once you’ve collected qualitative data through your survey. QuestionPro typically allows you to export survey data in various formats, such as Excel or CSV.
- Prepare Data for Analysis: Review the exported data and clean it if necessary. Remove irrelevant or duplicate entries to ensure your data is ready for analysis.
- Code and Categorize Responses: Segment and label data, letting new patterns emerge naturally, then develop categories through axial coding to structure the analysis.
- Identify Themes: Analyze the coded responses to identify recurring themes, patterns, and insights. Look for similarities and differences in participants’ responses.
- Generate Reports and Visualizations: Utilize the reporting features of QuestionPro to create visualizations, charts, and graphs that help communicate the themes and findings from your qualitative research.
- Interpret and Draw Conclusions: Interpret the themes and patterns you’ve identified in the qualitative data. Consider how these findings answer your research questions or provide insights into your study topic.
- Integrate with Quantitative Data (if applicable): If you’re also conducting quantitative research using QuestionPro, consider integrating your qualitative findings with quantitative results to provide a more comprehensive understanding.
Qualitative data analysis is vital in uncovering various human experiences, views, and stories. If you’re ready to transform your research journey and apply the power of qualitative analysis, now is the moment to do it. Book a demo with QuestionPro today and begin your journey of exploration.
LEARN MORE FREE TRIAL
MORE LIKE THIS
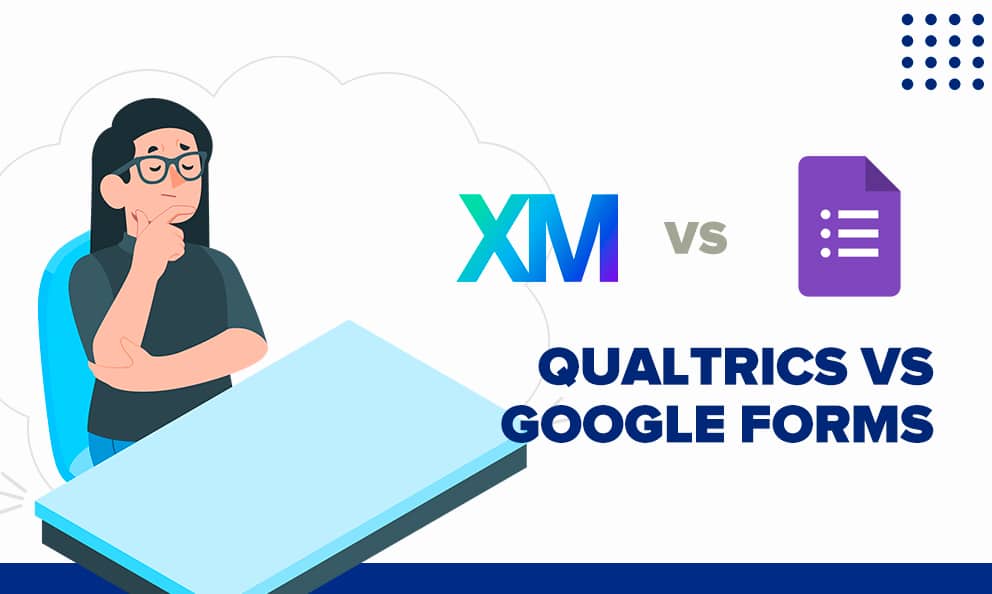
Qualtrics vs Google Forms: Which is the Best Platform?
Jul 24, 2024
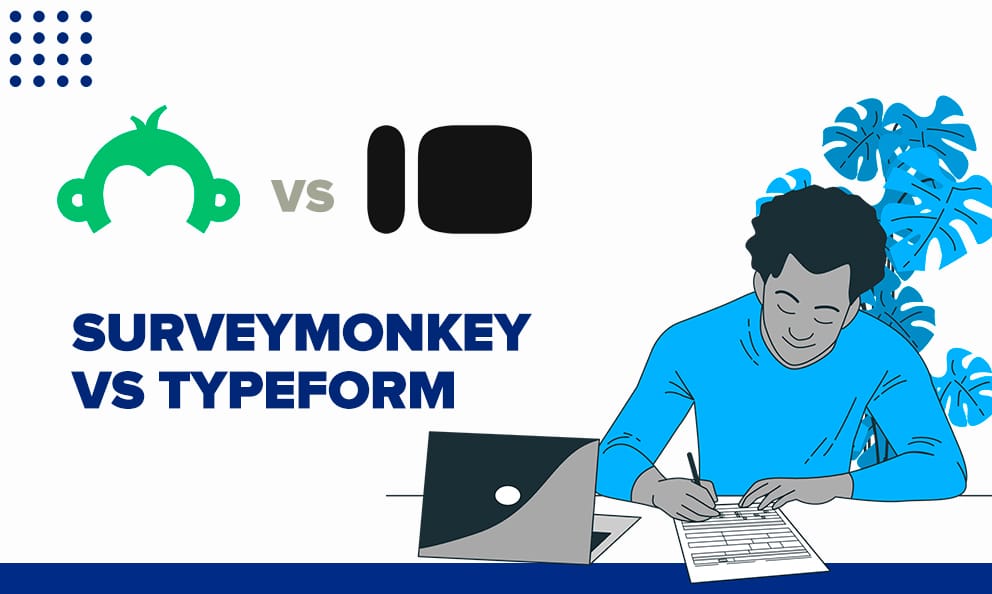
TypeForm vs. SurveyMonkey: Which is Better in 2024?
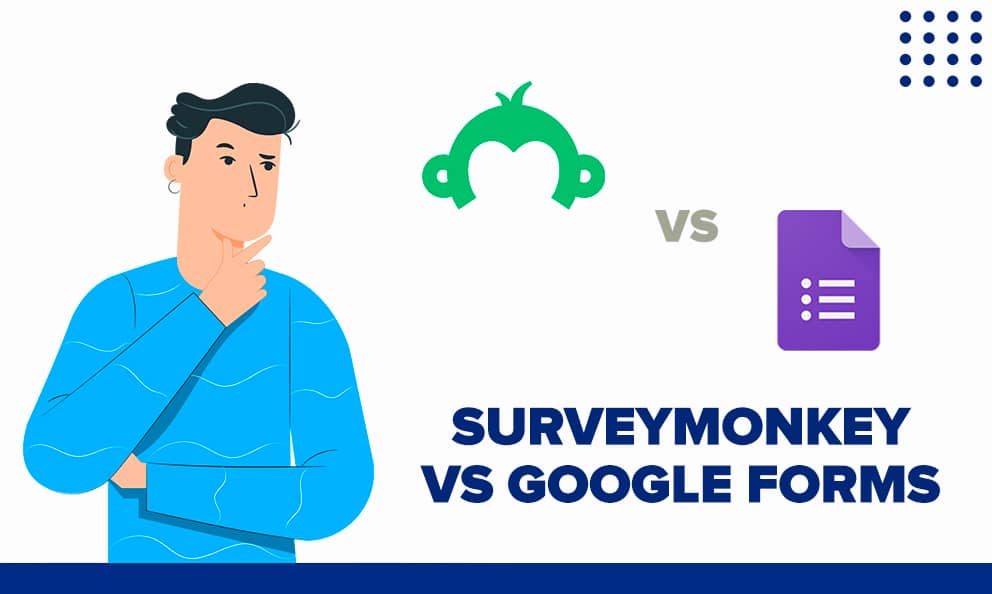
SurveyMonkey vs Google Forms: A Detailed Comparison
Jul 23, 2024
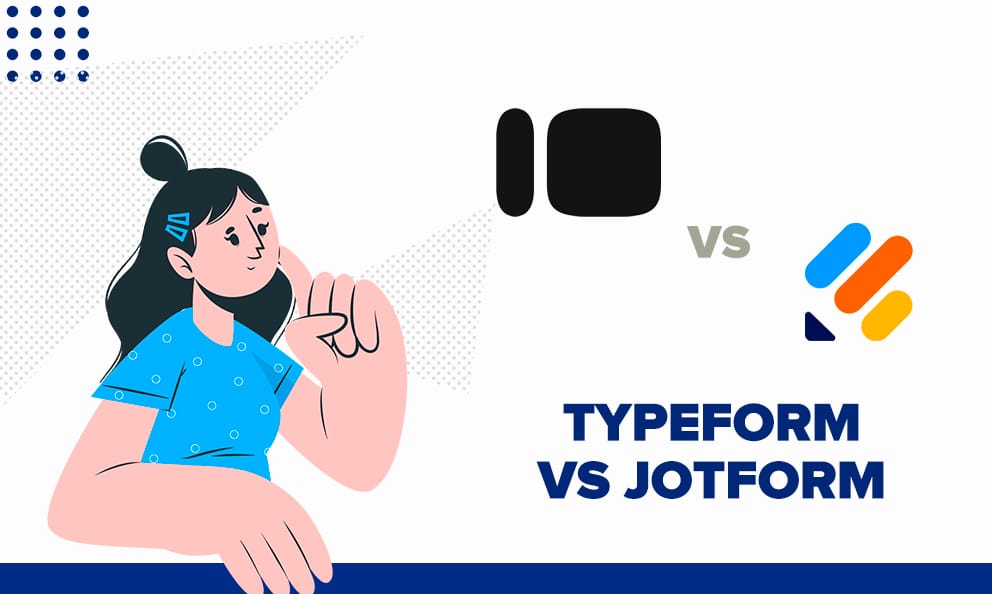
Jotform vs Typeform: Which is the Best Option? Comparison (2024)
Other categories.
- Academic Research
- Artificial Intelligence
- Assessments
- Brand Awareness
- Case Studies
- Communities
- Consumer Insights
- Customer effort score
- Customer Engagement
- Customer Experience
- Customer Loyalty
- Customer Research
- Customer Satisfaction
- Employee Benefits
- Employee Engagement
- Employee Retention
- Friday Five
- General Data Protection Regulation
- Insights Hub
- Life@QuestionPro
- Market Research
- Mobile diaries
- Mobile Surveys
- New Features
- Online Communities
- Question Types
- Questionnaire
- QuestionPro Products
- Release Notes
- Research Tools and Apps
- Revenue at Risk
- Survey Templates
- Training Tips
- Tuesday CX Thoughts (TCXT)
- Uncategorized
- What’s Coming Up
- Workforce Intelligence
Narrative Research
- In book: https://link.springer.com/referencework/10.1007/978-981-10-2779-6 (pp.1-13)
- Edition: eds
- Publisher: Springer

- University of Eswatini
Discover the world's research
- 25+ million members
- 160+ million publication pages
- 2.3+ billion citations

- Elizabeth Emily Watson

- Bambang Pratama

- Gloria Hongyee Chan

- Mohammad Hassan Naderi
- Josie Leonard
- EUR J ONCOL NURS
- Dimas Ria Angga Pribadi

- Hung-Ru Lin

- Sun Kyung Kim
- Myonghwa Park
- Fereshteh Heidari
- Zinat Amiri

- W. Bryce Hagedorn

- David Herman

- F. Michael Connelly
- Denise Lillian Appel
- Maria Papaikonomou
- Recruit researchers
- Join for free
- Login Email Tip: Most researchers use their institutional email address as their ResearchGate login Password Forgot password? Keep me logged in Log in or Continue with Google Welcome back! Please log in. Email · Hint Tip: Most researchers use their institutional email address as their ResearchGate login Password Forgot password? Keep me logged in Log in or Continue with Google No account? Sign up
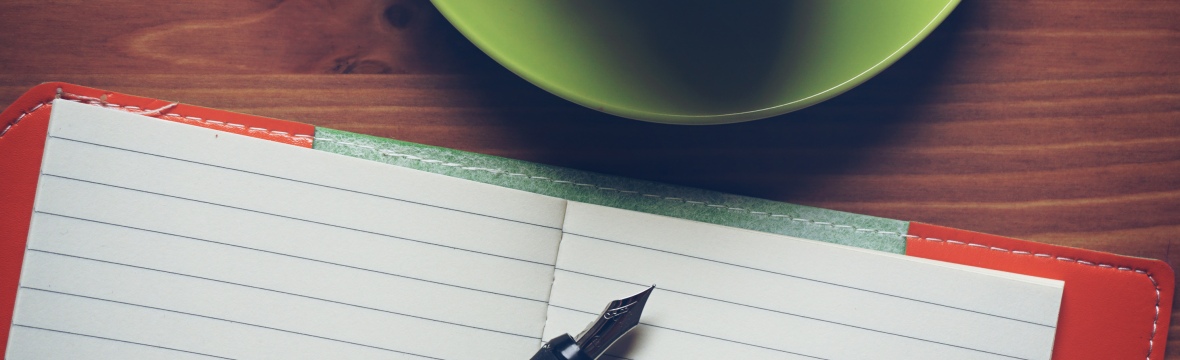
Dr Karen Lumsden
trainer / coach / consultant / researcher
Narrative Analysis
Narrative analysis is a valuable data analysis technique in qualitative research. It is typically used in those studies which have already employed narrative inquiry as a qualitative method. Narrative knowledge is created and constructed through the stories of lived experience and sense-making, the meanings people afford to them, and therefore offers valuable insight into the complexity of human lives, cultures, and behaviours. Narrative analysis uses the ‘story’ as the unit of analysis, in contrast to thematic and other forms of qualitative analysis.
Narrative analysis is useful for practitioners and researchers who wish to focus on individual experiences, e.g. via collected stories and unstructured interviews. Examples of possible applications include case studies; patients’ experiences of health care services or illness; life stories and experiences of social care clients; victims’ experiences of the criminal justice system.
This training benefits participants who wish to advance their knowledge of qualitative research methods. It explores the opportunities that narrative analysis offers in a range of applied and policy settings and contexts. It is relevant to researchers who have narrative data (or plan to collect narrative data) ready for analysis.
Share this:
Qualitative Research: Narrative
- Qualitative Research Texts
- Interviewing
What is Narrative Analysis?
Narrative research is a term that subsumes a group of approaches that in turn rely on the written or spoken words or visual representation of individuals. These approaches typically focus on the lives of individuals as told through their own stories. Clandinin and Connelly define it as "a way of understanding and inquiring into experience through “collaboration between researcher and participants, over time, in a place or series of places, and in social interaction with milieus” ( Clandinin & Connelly, 2000, p. 20)."
Books available at CSL
Books available through MOBIUS
Helpful Articles
- Narrative Analysis Survey of the science of Narrative Analysis by Catherine Kohler Riessman, a leading voice in the field.
- The state of the art in Narrative Inquiry Reflections on narrative inquiry and the status of the field.
- Stories of Experience and Narrative Inquiry This paper briefly surveys forms of narrative inquiry in educational studies and outline certain criteria, methods, and writing forms, which are described in terms of beginning the story, living the story, and selecting stories to construct and reconstruct narrative plots.
- Validity in Issues of Narrative Research Examines the question of validity in narrative studies.
- << Previous: Interviewing
- Last Updated: Mar 10, 2023 2:59 PM
- URL: https://csl.libguides.com/qualitative

An official website of the United States government
The .gov means it’s official. Federal government websites often end in .gov or .mil. Before sharing sensitive information, make sure you’re on a federal government site.
The site is secure. The https:// ensures that you are connecting to the official website and that any information you provide is encrypted and transmitted securely.
- Publications
- Account settings
Preview improvements coming to the PMC website in October 2024. Learn More or Try it out now .
- Advanced Search
- Journal List
- Malays Fam Physician
- v.3(1); 2008
Data Analysis in Qualitative Research: A Brief Guide to Using Nvivo
MSc, PhD, Faculty of Medicine, University of Malaya, Kuala Lumpur, Malaysia
Qualitative data is often subjective, rich, and consists of in-depth information normally presented in the form of words. Analysing qualitative data entails reading a large amount of transcripts looking for similarities or differences, and subsequently finding themes and developing categories. Traditionally, researchers ‘cut and paste’ and use coloured pens to categorise data. Recently, the use of software specifically designed for qualitative data management greatly reduces technical sophistication and eases the laborious task, thus making the process relatively easier. A number of computer software packages has been developed to mechanise this ‘coding’ process as well as to search and retrieve data. This paper illustrates the ways in which NVivo can be used in the qualitative data analysis process. The basic features and primary tools of NVivo which assist qualitative researchers in managing and analysing their data are described.
QUALITATIVE RESEARCH IN MEDICINE
Qualitative research has seen an increased popularity in the last two decades and is becoming widely accepted across a wide range of medical and health disciplines, including health services research, health technology assessment, nursing, and allied health. 1 There has also been a corresponding rise in the reporting of qualitative research studies in medical and health related journals. 2
The increasing popularity of qualitative methods is a result of failure of quantitative methods to provide insight into in-depth information about the attitudes, beliefs, motives, or behaviours of people, for example in understanding the emotions, perceptions and actions of people who suffer from a medical condition. Qualitative methods explore the perspective and meaning of experiences, seek insight and identify the social structures or processes that explain people”s behavioural meaning. 1 , 3 Most importantly, qualitative research relies on extensive interaction with the people being studied, and often allows researchers to uncover unexpected or unanticipated information, which is not possible in the quantitative methods. In medical research, it is particularly useful, for example, in a health behaviour study whereby health or education policies can be effectively developed if reasons for behaviours are clearly understood when observed or investigated using qualitative methods. 4
ANALYSING QUALITATIVE DATA
Qualitative research yields mainly unstructured text-based data. These textual data could be interview transcripts, observation notes, diary entries, or medical and nursing records. In some cases, qualitative data can also include pictorial display, audio or video clips (e.g. audio and visual recordings of patients, radiology film, and surgery videos), or other multimedia materials. Data analysis is the part of qualitative research that most distinctively differentiates from quantitative research methods. It is not a technical exercise as in quantitative methods, but more of a dynamic, intuitive and creative process of inductive reasoning, thinking and theorising. 5 In contrast to quantitative research, which uses statistical methods, qualitative research focuses on the exploration of values, meanings, beliefs, thoughts, experiences, and feelings characteristic of the phenomenon under investigation. 6
Data analysis in qualitative research is defined as the process of systematically searching and arranging the interview transcripts, observation notes, or other non-textual materials that the researcher accumulates to increase the understanding of the phenomenon. 7 The process of analysing qualitative data predominantly involves coding or categorising the data. Basically it involves making sense of huge amounts of data by reducing the volume of raw information, followed by identifying significant patterns, and finally drawing meaning from data and subsequently building a logical chain of evidence. 8
Coding or categorising the data is the most important stage in the qualitative data analysis process. Coding and data analysis are not synonymous, though coding is a crucial aspect of the qualitative data analysis process. Coding merely involves subdividing the huge amount of raw information or data, and subsequently assigning them into categories. 9 In simple terms, codes are tags or labels for allocating identified themes or topics from the data compiled in the study. Traditionally, coding was done manually, with the use of coloured pens to categorise data, and subsequently cutting and sorting the data. Given the advancement of software technology, electronic methods of coding data are increasingly used by qualitative researchers.
Nevertheless, the computer does not do the analysis for the researchers. Users still have to create the categories, code, decide what to collate, identify the patterns and draw meaning from the data. The use of computer software in qualitative data analysis is limited due to the nature of qualitative research itself in terms of the complexity of its unstructured data, the richness of the data and the way in which findings and theories emerge from the data. 10 The programme merely takes over the marking, cutting, and sorting tasks that qualitative researchers used to do with a pair of scissors, paper and note cards. It helps to maximise efficiency and speed up the process of grouping data according to categories and retrieving coded themes. Ultimately, the researcher still has to synthesise the data and interpret the meanings that were extracted from the data. Therefore, the use of computers in qualitative analysis merely made organisation, reduction and storage of data more efficient and manageable. The qualitative data analysis process is illustrated in Figure 1 .

Qualitative data analysis flowchart
USING NVIVO IN QUALITATIVE DATA ANALYSIS
NVivo is one of the computer-assisted qualitative data analysis softwares (CAQDAS) developed by QSR International (Melbourne, Australia), the world’s largest qualitative research software developer. This software allows for qualitative inquiry beyond coding, sorting and retrieval of data. It was also designed to integrate coding with qualitative linking, shaping and modelling. The following sections discuss the fundamentals of the NVivo software (version 2.0) and illustrates the primary tools in NVivo which assist qualitative researchers in managing their data.
Key features of NVivo
To work with NVivo, first and foremost, the researcher has to create a Project to hold the data or study information. Once a project is created, the Project pad appears ( Figure 2 ). The project pad of NVivo has two main menus: Document browser and Node browser . In any project in NVivo, the researcher can create and explore documents and nodes, when the data is browsed, linked and coded. Both document and node browsers have an Attribute feature, which helps researchers to refer the characteristics of the data such as age, gender, marital status, ethnicity, etc.

Project pad with documents tab selected
The document browser is the main work space for coding documents ( Figure 3 ). Documents in NVivo can be created inside the NVivo project or imported from MS Word or WordPad in a rich text (.rtf) format into the project. It can also be imported as a plain text file (.txt) from any word processor. Transcripts of interview data and observation notes are examples of documents that can be saved as individual documents in NVivo. In the document browser all the documents can be viewed in a database with short descriptions of each document.

Document browser with coder and coding stripe activated
NVivo is also designed to allow the researcher to place a Hyperlink to other files (for example audio, video and image files, web pages, etc.) in the documents to capture conceptual links which are observed during the analysis. The readers can click on it and be taken to another part of the same document, or a separate file. A hyperlink is very much like a footnote.
The second menu is Node explorer ( Figure 4 ), which represents categories throughout the data. The codes are saved within the NVivo database as nodes. Nodes created in NVivo are equivalent to sticky notes that the researcher places on the document to indicate that a particular passage belongs to a certain theme or topic. Unlike sticky notes, the nodes in NVivo are retrievable, easily organised, and give flexibility to the researcher to either create, delete, alter or merge at any stage. There are two most common types of node: tree nodes (codes that are organised in a hierarchical structure) and free nodes (free standing and not associated with a structured framework of themes or concepts). Once the coding process is complete, the researcher can browse the nodes. To view all the quotes on a particular Node, select the particular node on the Node Explorer and click the Browse button ( Figure 5 ).

Node explorer with a tree node highlighted

Browsing a node
Coding in NVivo using Coder
Coding is done in the document browser. Coding involves the desegregation of textual data into segments, examining the data similarities and differences, and grouping together conceptually similar data in the respective nodes. 11 The organised list of nodes will appear with a click on the Coder button at the bottom of document browser window.
To code a segment of the text in a project document under a particular node, highlight the particular segment and drag the highlighted text to the desired node in the coder window ( Figure 3 ). The segments that have been coded to a particular node are highlighted in colours and nodes that have attached to a document turns bold. Multiple codes can be assigned to the same segment of text using the same process. Coding Stripes can be activated to view the quotes that are associated with the particular nodes. With the guide of highlighted text and coding stripes, the researcher can return to the data to do further coding or refine the coding.
Coding can be done with pre-constructed coding schemes where the nodes are first created using the Node explorer followed by coding using the coder. Alternatively, a bottom-up approach can be used where the researcher reads the documents and creates nodes when themes arise from the data as he or she codes.
Making and using memos
In analysing qualitative data, pieces of reflective thinking, ideas, theories, and concepts often emerge as the researcher reads through the data. NVivo allows the user the flexibility to record ideas about the research as they emerge in the Memos . Memos can be seen as add-on documents, treated as full status data and coded like any other documents. 12 Memos can be placed in a document or at a node. A memo itself can have memos (e.g. documents or nodes) linked to it, using DocLinks and NodeLinks .
Creating attributes
Attributes are characteristics (e.g. age, marital status, ethnicity, educational level, etc.) that the researcher associates with a document or node. Attributes have different values (for example, the values of the attribute for ethnicity are ‘Malay’, ‘Chinese’ and ‘Indian’). NVivo makes it possible to assign attributes to either document or node. Items in attributes can be added, removed or rearranged to help the researcher in making comparisons. Attributes are also integrated with the searching process; for example, linking the attributes to documents will enable the researcher to conduct searches pertaining to documents with specified characteristics ( Figure 6 ).

Document attribute explorer
Search operation
The three most useful types of searches in NVivo are Single item (text, node, or attribute value), Boolean and Proximity searches. Single item search is particularly important, for example, if researchers want to ensure that every mention of the word ‘cure’ has been coded under the ‘Curability of cervical cancer’ tree node. Every paragraph in which this word is used can be viewed. The results of the search can also be compiled into a single document in the node browser and by viewing the coding stripe. The researcher can check whether each of the resulting passages has been coded under a particular node. This is particularly useful for the researcher to further determine whether conducting further coding is necessary.
Boolean searches combine codes using the logical terms like ‘and’, ‘or’ and ‘not’. Common Boolean searches are ‘or’ (also referred to as ‘combination’ or ‘union’) and ‘and’ (also called ‘intersection’). For example, the researcher may wish to search for a node and an attributed value, such as ‘ever screened for cervical cancer’ and ‘primary educated’. Search results can be displayed in matrix form and it is possible for the researcher to perform quantitative interpretations or simple counts to provide useful summaries of some aspects of the analysis. 13 Proximity searches are used to find places where two items (e.g. text patterns, attribute values, nodes) appear near each other in the text.
Using models to show relationships
Models or visualisations are an essential way to describe and explore relationships in qualitative research. NVivo provides a Modeler designated for visual exploration and explanation of relationships between various nodes and documents. In Model Explorer, the researcher can create, label and connect ideas or concepts. NVivo allows the user to create a model over time and have any number of layers to track the progress of theory development to enable the researcher to examine the stages in the model-building over time ( Figure 7 ). Any documents, nodes or attributes can be placed in a model and clicking on the item will enable the researcher to inspect its properties.

Model explorer showing the perceived risk factors of cervical cancer
NVivo has clear advantages and can greatly enhance research quality as outlined above. It can ease the laborious task of data analysis which would otherwise be performed manually. The software certainly removes the tremendous amount of manual tasks and allows more time for the researcher to explore trends, identify themes, and make conclusions. Ultimately, analysis of qualitative data is now more systematic and much easier. In addition, NVivo is ideal for researchers working in a team as the software has a Merge tool that enables researchers that work in separate teams to bring their work together into one project.
The NVivo software has been revolutionised and enhanced recently. The newly released NVivo 7 (released March 2006) and NVivo 8 (released March 2008) are even more sophisticated, flexible, and enable more fluid analysis. These new softwares come with a more user-friendly interface that resembles the Microsoft Windows XP applications. Furthermore, they have new data handling capacities such as to enable tables or images embedded in rich text files to be imported and coded as well. In addition, the user can also import and work on rich text files in character based languages such as Chinese or Arabic.
To sum up, qualitative research undoubtedly has been advanced greatly by the development of CAQDAS. The use of qualitative methods in medical and health care research is postulated to grow exponentially in years to come with the further development of CAQDAS.
More information about the NVivo software
Detailed information about NVivo’s functionality is available at http://www.qsrinternational.com . The website also carries information about the latest versions of NVivo. Free demonstrations and tutorials are available for download.
ACKNOWLEDGEMENT
The examples in this paper were adapted from the data of the study funded by the Ministry of Science, Technology and Environment, Malaysia under the Intensification of Research in Priority Areas (IRPA) 06-02-1032 PR0024/09-06.
TERMINOLOGY
Attributes : An attribute is a property of a node, case or document. It is equivalent to a variable in quantitative analysis. An attribute (e.g. ethnicity) may have several values (e.g. Malay, Chinese, Indian, etc.). Any particular node, case or document may be assigned one value for each attribute. Similarities within or differences between groups can be identified using attributes. Attribute Explorer displays a table of all attributes assigned to a document, node or set.
CAQDAS : Computer Aided Qualitative Data Analysis. The CAQDAS programme assists data management and supports coding processes. The software does not really analyse data, but rather supports the qualitative analysis process. NVivo is one of the CAQDAS programmes; others include NUDIST, ATLAS-ti, AQUAD, ETHNOGRAPH and MAXQDA.
Code : A term that represents an idea, theme, theory, dimension, characteristic, etc., of the data.
Coder : A tool used to code a passage of text in a document under a particular node. The coder can be accessed from the Document or Node Browser .
Coding : The action of identifying a passage of text in a document that exemplifies ideas or concepts and connecting it to a node that represents that idea or concept. Multiple codes can be assigned to the same segment of text in a document.
Coding stripes : Coloured vertical lines displayed at the right-hand pane of a Document ; each is named with title of the node at which the text is coded.
DataLinks : A tool for linking the information in a document or node to the information outside the project, or between project documents. DocLinks , NodeLinks and DataBite Links are all forms of DataLink .
Document : A document in an NVivo project is an editable rich text or plain text file. It may be a transcription of project data or it may be a summary of such data or memos, notes or passages written by the researcher. The text in a document can be coded, may be given values of document attributes and may be linked (via DataLinks ) to other related documents, annotations, or external computer files. The Document Explorer shows the list of all project documents.
Memo : A document containing the researcher”s commentary flagged (linked) on any text in a Document or Node. Any files (text, audio or video, or picture data) can be linked via MemoLink .
Model : NVivo models are made up of symbols, usually representing items in the project, which are joined by lines or arrows, designed to represent the relationship between key elements in a field of study. Models are constructed in the Modeller .
Node : Relevant passages in the project”s documents are coded at nodes. A Node represents a code, theme, or idea about the data in a project. Nodes can be kept as Free Nodes (without organisation) or may be organised hierarchically in Trees (of categories and subcategories). Free nodes are free-standing and are not associated to themes or concepts. Early on in the project, tentative ideas may be stored in the Free Nodes area. Free nodes can be kept in a simple list and can be moved to a logical place in the Tree Node when higher levels of categories are discovered. Nodes can be given values of attributes according to the features of what they represent, and can be grouped in sets. Nodes can be organised (created, edited) in Node Explorer (a window listing all the project nodes and node sets). The Node Browser displays the node”s coding and allow the researcher to change the coding.
Project : Collection of all the files, documents, codes, nodes, attributes, etc. associated with a research project. The Project pad is a window in NVivo when a project is open which gives access to all the main functions of the programme.
Sets : Sets in NVivo hold shortcuts to any nodes or documents, as a way of holding those items together without actually combining them. Sets are used primarily as a way of indicating items that in some way are related conceptually or theoretically. It provides different ways of sorting and managing data.
Tree Node : Nodes organised hierarchically into trees to catalogue categories and subcategories.
- Advanced search
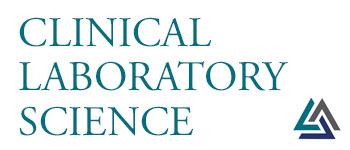
Advanced Search
A Narrative Approach to Qualitative Inquiry
- Find this author on Google Scholar
- Find this author on PubMed
- Search for this author on this site
- For correspondence: [email protected]
- Info & Metrics
This article requires a subscription to view the full text. If you have a subscription you may use the login form below to view the article. Access to this article can also be purchased.
- University of Kentucky, College of Health Sciences, Lexington, KY
- Address for Correspondence: Michelle Butina, PhD, MLS(ASCP) CM , Assistant Professor and Program Director, Medical Laboratory Science, University of Kentucky, College of Health Sciences, 900 South Limestone Street, CTW Bldg. Rm. 126E, Lexington, KY 40536-0200, (859) 218-0852, Michelle.Butina{at}uky.edu
Provide an example of when narrative inquiry would be the most appropriate qualitative research approach.
Identify the activities involved in data collection.
Define and describe narrative thematic data analysis.
Discuss data verification and validation strategies used in qualitative research approaches.
INTRODUCTION When selecting a research design, such as quantitative, qualitative, or mixed methods, Patton (2002) suggested reflecting on the purpose of the inquiry and the types of answers you seek. 1 Qualitative methods allow the researcher to study issues in depth with data collection often occurring through open-ended questions permitting “one to understand and capture the points of view of other people without predetermining those points of view through prior selection of questionnaire categories”. 1 Results include a wealth of detailed information about a small number of people; therefore, leading to an increase in the depth of understanding of these select individuals.
As Creswell (2013) stated, “Those undertaking qualitative studies have a baffling number of choices of approaches”. 2 Qualitative approaches of inquiry are diverse and can be numerous depending upon how they are classified as some classification schemes include 20 plus approaches. 2 Regardless of how they are classified, the traditional 5 approaches are represented, in some fashion, in all classification schemes. These include case studies, ethnography, grounded theory, narrative, and phenomenology. This article will provide the reader with a better understanding of narrative approach or narrative inquiry.
Narrative inquiry is the approach of which I have the most knowledge and the most experience with as it was the approach I selected for my doctoral research study. Narrative inquiry is a form of qualitative research in which the stories themselves become the raw data. 3 This approach has been used in many disciplines to learn more about the culture, historical experiences, identity, and lifestyle…
- Data coding
- data validation
- narrative inquiry
- professional identity
- qualitative research methods
- © Copyright 2015 American Society for Clinical Laboratory Science Inc. All rights reserved.
In this issue
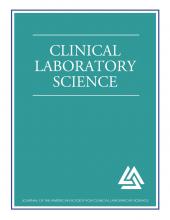
- Table of Contents
- Table of Contents (PDF)
- Index by author
- Front Matter (PDF)
Thank you for your interest in spreading the word on American Society for Clinical Laboratory Science.
NOTE: We only request your email address so that the person you are recommending the page to knows that you wanted them to see it, and that it is not junk mail. We do not capture any email address.
Citation Manager Formats
- EndNote (tagged)
- EndNote 8 (xml)
- RefWorks Tagged
- Ref Manager

- Tweet Widget
- Facebook Like
- Google Plus One
Jump to section
Related articles.
- No related articles found.
- Google Scholar
Cited By...
- Impact of defocus incorporated multiple segments (DIMS) spectacle lenses for myopia control on quality of life of the children: a qualitative study
- Practices and challenges of community engagement in health research in Ethiopia: a qualitative study
- The experiences of Indigenous people with cancer in Saskatchewan: a patient-oriented qualitative study using a sharing circle
More in this TOC Section
- Conducting Qualitative Research Introduction
- Conducting Case Study Research
Similar Articles
- data coding

Narrative Analysis in Qualitative Research
First-person interviews are a regular part of the qualitative research process. In order to interpret and examine the content of those interviews, it can be useful to take advantage of narrative analysis in qualitative research. Simply put, it’s a way for researchers to examine how and why participants share their stores in certain ways.
Are you looking to use narrative analysis to code your data? Check out our quick guide to learn how to get started.

What is narrative analysis?
Narrative analysis is a means of interpreting how the participants in a research study tell the stories of their lives. In many qualitative research studies, participants are encouraged to make sense of the world around them through a first-person narrative structure.
In turn, researchers can analyze those narratives to better understand how the participants in question experienced these events. They can look at everything from the words used in those narratives to how those narratives are structured. Ultimately, by coding the qualitative data on hand, researchers are able to get a better understanding of the world around them.
How do you conduct narrative analysis?
In order to gather meaningful data for this type of analysis, you’ll want to keep a couple of key factors in mind:
- Ask open-ended questions : Participants should feel free to tell their narratives in whatever way feels most natural to them. In order to encourage this sort of behavior, researchers should ask questions that allow for more free-form responses.
- Give participants lots of time: Similarly, researchers will want to make sure their participants have enough time to share everything that’s on their mind. This includes any asides or tangents that come up naturally throughout the course of the conversation. Researchers should avoid interrupting participants or cutting them off.
- Transcribe all collected data: When the interview ends, it’s important to transcribe the collected data as accurately as possible. This includes keeping any verbal tics like “um” or “uh”. All of it is relevant for the analysis, since it works together to give a sense of a participant’s state of mind. LiGRE’s audio transcription tool is a great way to make sure you don’t miss out on any key details.
While this advice is more specific to narrative inquiries, you can also check out our more general advice on how to conduct a guided intervie w .
What is an example of narrative analysis in qualitative research?
To get a better understanding of narrative inquiries, let’s dig into a step-by-step example of how it could work in practice.
Step One: Identify your research question
Every study needs a research question to define the parameters of its investigation. This allows researchers to identify what information they need to gather from their research participants.
As an example, let’s consider a study of a group of married people. A researcher might design a study around their decision to get married, as well as its influence on the participants’ lives.
Step Two: Collect your narratives
Researchers may find enough information through pre-existing biographies or collected life stories from their participants. However, it’s much more likely that they’ll need to conduct first-person interviews as part of their research process.
Returning to our example, a researcher might ask a participant to talk more broadly about the story of their life. The circumstances surrounding their marriage would likely come up naturally, given that it is a significant life event. A researcher could also ask the participant to speak more specifically about the marriage instead.
Step Three: Code the collected data
Next, it’s time to analyze the collected data. Coding is the most effective means of identifying core narratives and themes within a block of qualitative data. When it comes to this type of analysis, you’ll want to start out by identifying specific narratives within the text.
In order to identify the starting and ending points of these particular stories, it can be useful to look for certain phrases within the transcript. For example, participants may launch into a story with phrases like “It all started when…” or “At the start…”. At a story’s conclusion, you may see the use of phrases like “…and that’s how it happened” or “…that’s the end of that.”
Step Four: Break it down using narrative structure
Within these stories, researchers will also want to identify the various components of narrative structure. Different methods will work better for different researchers, but to start, it can be helpful to rely on more basic story-structure concepts like beginning, middle, and end.
A researcher will likely notice certain patterns as they begin to code their collected narratives. For example, a researcher may notice that many participants begin the story of their marriage with details on how they met their spouse. They may discuss certain factors that led to their decision to get married, such as love, finances, the desire to start a family, or social pressure from their community. Also, their narratives may include information about the impact of the marriage on their social, emotional, and financial well-being.
Step Five: Analyze your findings
The patterns that arise throughout the coding process are at the heart of the analytic process. From there, researchers can consider how their specific conclusions relate to broader societal patterns and trends. How do those findings relate to your research question? Do they confirm your hypothesis? Does it challenge your expectations in a meaningful way?
Also, researchers will want to use this opportunity to consider how their own biases, backgrounds, and beliefs might factor into their understanding. This is especially true if the participant group represents a different race, ethnicity, or social status than the research team. In that case, a practice of reflexivity could be especially useful.
Properly transcribing, organizing, and coding qualitative data isn’t always a simple task—but it is an essential one if you’re looking to perform an effective narrative inquiry.
LiGRE offers qualitative analysis software that’s designed to help researchers with these tasks and a whole lot more. To get started, sign up today!
- QUICK LINKS
- How to enroll
- Career services
Narrative Inquiry Research Design Essentials
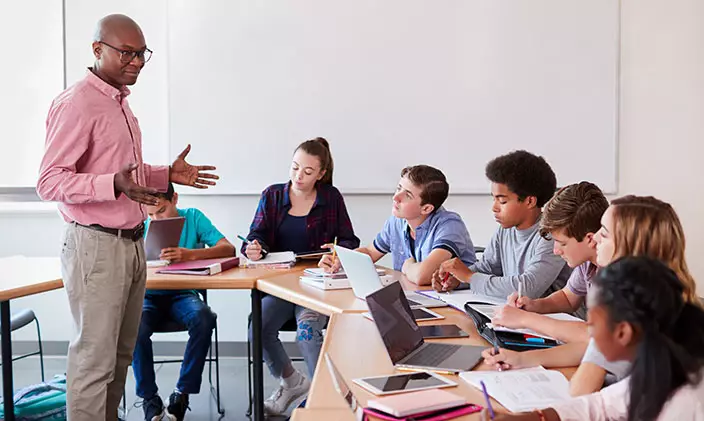
By Dr. Jim Lane
Introduction
More specifically than other qualitative study designs, Narrative inquiry presents strategies for researchers to “study the stories people tell about their experiences” (Clandinin, 2007, xiv). On February 20, 2024, the Research Methodology Group hosted a webinar focused on Narrative Inquiry Essentials. In this blog post, we delve into the essence of Narrative inquiry design, exploring its principles, applications, and significance in contemporary research. We end with some suggestions for how to learn more about narrative inquiry.
What is Narrative Inquiry?
Jean Clandinin and Michael Connelly are early and noted practitioners of the narrative inquiry design. Any discussion of narrative inquiry musty include them. They explain, “Narrative inquiry, the study of experience as story, is first and foremost a way of thinking about experience. Narrative enquiry as a methodology entails a view of the phenomenon. To use narrative inquiry methodology is to adopt a particular narrative view of experience as phenomena under study” (Connelly & Clandinin, 2006, in Kim, 2016, p. 18).
Narrative inquiry, the study of experience as story, is first and foremost a way of thinking about experience
Jean Clandinin and Michael Connelly
Essential Components of Narrative Inquiry
Narrative inquiry is the analysis of a person’s story, of an experience they have had. The goal of researcher is to draw out the story from the participant.
Clandinin and Connelly (2000) describe a model they call a “metaphorical three-dimensional space” to describe their approach to narrative inquiry. They explain the model is based on
Dewey’s notion of interaction, by focusing on what we call four directions in any inquiry: Inward and outward, backward and forward. By inward, we mean toward the internal conditions, such as feelings, hope, aesthetic reactions, and moral dispositions. By outward we mean toward the existential conditions, that is, the environment. By backward and forward, we refer to temporality – past, present, and future (p. 50).
A story can be told many ways, including through visual means. Researchers may create vignettes of each story, create one overarching meta-narrative that includes the main aspects of all shared stories, depict the story through imagery, create a fictitious story/play demonstrating main gist of narratives, or even identify main themes found within the narratives.
Building Appropriate Research Questions and Purpose Statement
From the College of Doctoral Studies Dissertation Guide:
The research questions should focus on temporality, sociality, and spatiality. For example:
R1: What were the participants’ feelings about the experience (name specific experience)? (temporality)
R2: What were the cultural factors and what were the interrelationships with other people involved in the situation (name specific situation)? (sociality)
R3: What was the physical environment during the experience and how did the environment impact the experience (name specific experience)? (spatiality)
A person may use different types of data to tell their story:
Artifacts: “Personal journals, diaries, letters, books, photographs, paintings, personal belongings, formal and informal documents, or any other objects the storytellers would like to share that are related to the telling of their story” (Kim, 2016, p. 177)
Visual data: Websites, photos, advertisements, signs, etc. “We don’t always notice the importance of what we see, nor do we attempt to systematically analyze it. It is the paying attention, the looking and the taking note of what we see that makes images especially important” (Weber, 2008, in Kim, 2016, p. 178).
Digital archival data: Personal stories, folk tales, audio files, video files, etc.
Fieldwork: Living in the research field, collecting data through observations (Connelly & Clandinin, 2006).
The purpose statement for narrative inquiry studies must include the objective of examining participants’ experiences as understood through their stories. The elements of temporality, sociality, and spatiality should be included in the stated study objectives.
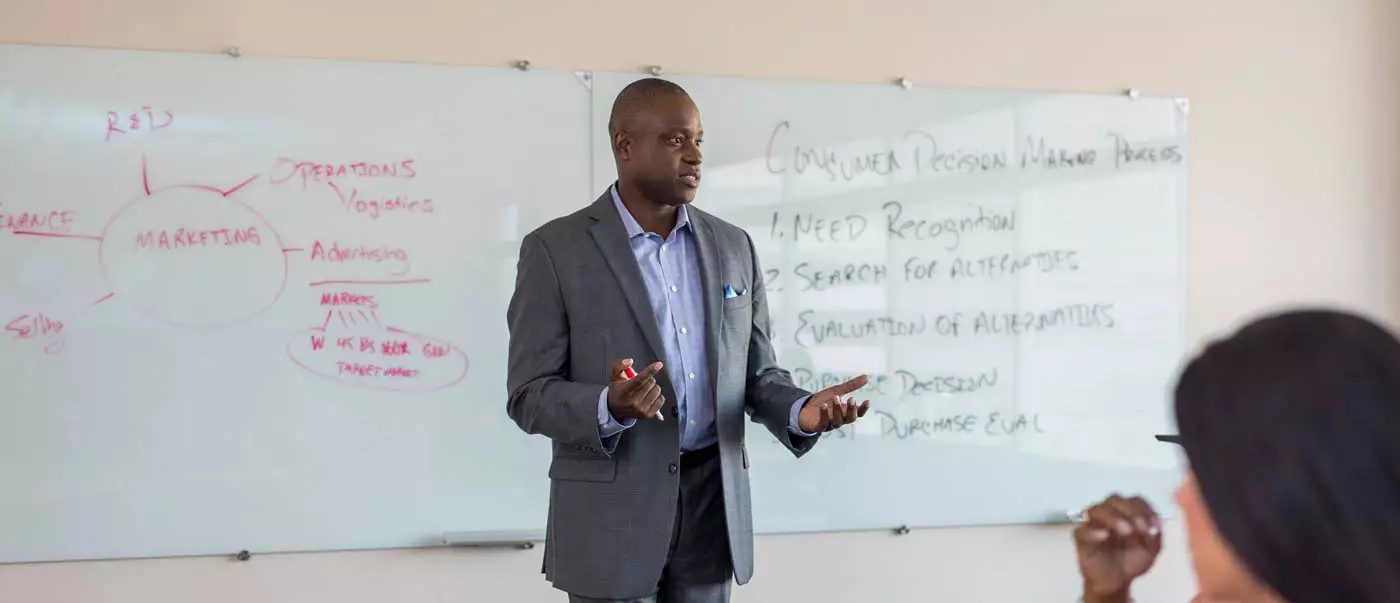
Appropriate Interview Questions
Semi-structured interviews use “general questions … to guide the interview, helping you maintain its focus rather than dictate its direction. … They allow you to ask different but relevant questions depending on the interviewee’s responses” (Kim, 2016, p. 164).
In unstructured, open-ended interviews the researcher may have no set agenda. Rather, the researcher plays an active role through asking probing or clarifying questions (Kim, 2016, p. 164) Kim notes, “We have to think narratively about how we can generate interview data that will help us accomplish our research purpose … while leaving room for surprises that might come out of our narrative data” (Kim, 2016, p. 169).
Applications of Narrative Inquiry
Creswell explains, “The focus of a narrative is on the life of an individual” (2013, p. 121). “Within the story may be epiphanies, turning points, or disruptions in which the story line changes direction dramatically. In the end, the narrative study tells the story of individuals unfolding in a chronology of their experiences, set within their personal, social, and historical context, and including the important themes in those lived experiences” (2013, p. 75).
Cole and Knowles argue, “To understand some of the complexities, complications, and confusions within the life of just one member of a community is to gain insights into the collective . . . every in-depth exploration of an individual life-in-context brings us that much closer to understanding the complexities of lives in communities” (2001, p. 11).
Significance of Narrative Inquiry
Narrative inquiry is linked to Schon’s concept of “reflection-in-action,” through which professionals learn the practical application of theory (Schon, 1983). In effect, professionals learn by doing and applying academic knowledge. Schon’s description mirrors the Scholar-Practitioner-Leader Model applied in the University of Phoenix College of Dissertation Studies , which also recognizes the importance of both cognitive learning and the application of that knowledge in practical terms.
Narrative & Theoretical Lens/Prism
Narrative cannot exist in a vacuum. We cannot make sense of the stories told without the help of a theory or the intersection of multiple theories. However, theories should inform or guide us to understand a story but not to diminish our ability to listen. Theories are there to be used, not to dictate to us or shape our stories (Kim, 2016).
For example, critical theory “Examines relationships of domination and subordination that create social inequality in society. … For critical theorists, the ability to look at the contradictions inherent in a society is a starting point to develop forms of social inquiry that interrogate ‘what is in reality’ and ‘what should be?’” (Kim, 2016, p. 36).
Some Common Critical Paradigms
Critical Race Theory focuses on the impact of race and racism (Crenshaw, 1995; Delgado & Stefanic, 2023).
Feminist Theory focuses on “situations of patriarchy, the unequal ways people are treated due to their gender and sexuality, and the meanings we construct or that are constructed by the prevailing value system that assigns gender roles” (Kim, 2016, p. 48).
Queer Theory examines “the homo-heterosexual binary in everyday life, … investigating the way group boundaries are created, negotiated, and changed” (Denzin & Lincoln, 2018, p. 105).
Cultural studies examine cultural practices and subjectivities (Denzin & Lincoln, 2016).
Challenges of Narrative Inquiry
Narrative researchers must anticipate several challenges. First, they must be willing to spend time to read key researchers in the field to understand both theory and practice. Next, they must be willing to spend quality time with the participants. They must also be willing to probe to gather, describe, and understand their participants’ stories. They must be prepared for epiphanies, insights, significant quotes. And finally, narrative researchers must be able to write, not only grammatically, but empathetically, capturing both cognitively and affectively the stories of their participants.
Important Issues to Consider
First, the narrative researcher wants to understand stories that chronicle experience.
This is broader than phenomenology and more focused than a case study. The researcher should also be prepared to keep a journal, including reflective memos to self. This facilitates the reflective process of reflecting on the stories of one’s participants to understand how they apply to the researcher’s problem, purpose, and research questions.
Want to know more?
- Check out the full webinar on Narrative Inquiry that is uploaded to the Research and Methodology Group Teams site.
- Schedule an office hours appointment with a methodologist to discuss your Narrative Inquiry design.
References
Bochner, A.P. (2014). Coming to narrative: A personal history of paradigm change in the human sciences . Left Coast Press.
Braun, V. & Clarke, V. (2013). Successful qualitative research: A practical guide for beginners . SAGE.
Clandinin, D.J. & Connelly, F.M. (2000). Narrative inquiry: Experience and story in qualitative research . Jossey-Bass.
Clandinen, J.C., Huber, J., Huber, M., Murphy, M.S., Orr, A.M., Pearce, M., & Steeves, P. (2006). Composing diverse identities: Narrative inquiries into the interwoven lives of children and teachers . Routledge.
Clandinin, D.J. (Ed.) (2007). Handbook of narrative inquiry: Mapping a methodology . SAGE.
Clandinin, D.J. (2013). Engaging in narrative inquiry . Left Coast Press
Clandinin, D.J., Caine, V., Lessard, S. & Huber, J. (2016). Engaging in narrative inquiries with children and youth . Routledge.
College of Doctoral Studies Dissertation Guide (2024, April 1). University of Phoenix College of Doctoral Studies.
Cole, A.L. & Knowles, J.G. (2001). Lives in context: The art of life history research . Alta Mira Press.
Dewey, J. (1934). Art as experience. Penguin.
Dewey, J. (2015). Experience and education . Free Press (Original work published 1938 by Kappa Delta Pi).
Denzin, N.K., Canella, G.S., Giardina, M.D., & Lincoln, Y.S.(eds.) (2024). The SAGE handbook of qualitative research, 6 th ed. SAGE
Kim, J.H. (2016). Understanding narrative inquiry. SAGE.
Riessman, C.K. (2008). Narrative methods for the human sciences. SAGE.
Rose, G. (2016). Visual methodologies. An introduction to researching with visual materials, 4rth ed . SAGE.
Saldana, J.(2015). Thinking qualitatively . SAGE
Saldana, J. & Omasta, M. (2018). Qualitative research: Analyzing life . SAGE.
Schon, D. A. (1983). The reflective practitioner: How professionals think in action . Basic Books, Inc
Additional Resources
- Narrative Inquiry
- Qualitative Inquiry
- SAGE Methods https://methods.sagepub.com/Search/Results
- Jean Clandinin Video
- Handbook of Narrative Inquiry: Mapping a Methodology
- Oxford Handbook of Qualitative Inquiry
- Dissertations of the Year
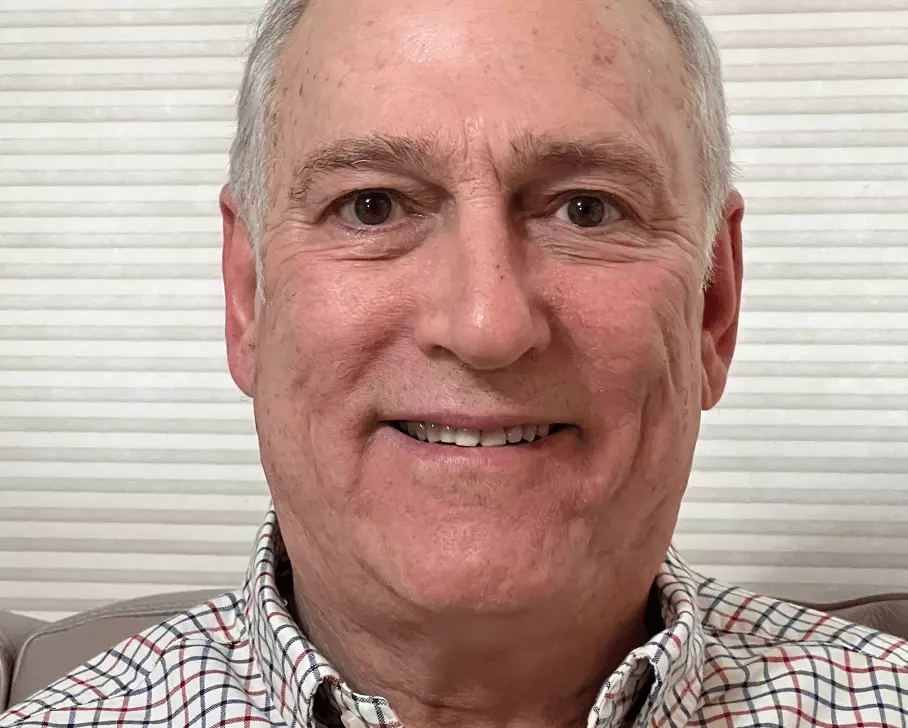
Jim Lane, Ed.D.
ABOUT THE AUTHOR
Jim Lane, Ed.D. served as a public school educator for 37 years as an English teacher, district language arts supervisor, and middle school assistant principal and principal. He holds a BA in English-Mass Communications Education, an MA in English, and an MEd and EdD in Educational Leadership. He has worked with the University of Phoenix since 2006 in various roles, including the associate research chair of the Center for Professional Responsibility in Education, research fellow in the Center for Education and Instructional Technology, reviewer for the Dissertation to Publication workshop, and member of the Research Methodology SIG. He is a faculty member in the ACCESS program, facilitating doctoral courses and serving dissertation committees as panel validator.
- Open access
- Published: 22 July 2024
Characteristics and quality of reporting qualitative nursing research related to the COVID-19 pandemic: a systematic search and critical review
- Ian-In Vong 1 ,
- Monique Rothan-Tondeur 1 , 2 &
- Rita Georges Nohra 1
BMC Nursing volume 23 , Article number: 498 ( 2024 ) Cite this article
132 Accesses
Metrics details
The COVID-19 (Coronavirus disease of 2019) pandemic caused major disruption to nursing research, especially qualitative research. Researchers had to overcome numerous challenges that potentially impacted the quality of the studies carried out.
The aim of this study is to assess the characteristics and quality of reporting qualitative nursing articles on the COVID-19 pandemic.
A systematic search and critical review using content analysis was conducted on published nurse-led articles using a qualitative approach related to the COVID-19 pandemic. A combination of the Consolidated Criteria for Reporting Qualitative Research (COREQ) and Standards for Reporting Qualitative Research (SRQR) checklists and additional items identified from the literature were used to assess the characteristics and overall quality of reporting of qualitative research.
Out of 63,494 articles screened, 444 met the inclusion criteria. Most studies were published in high-impact, Quartile 1 journals, with the majority originating from the USA. Common themes included workforce experiences and the impact of pandemic restrictions. Methodological quality varied, with a notable underuse of standardized reporting checklists. Despite pandemic-induced challenges in data collection, interviews remained the predominant method. However, the adoption of remote research methods and analysis software was limited.
The findings underscore the resilience and adaptability of nursing researchers during the pandemic. High-quality publications in top-tier journals indicate rigorous academic standards. However, the low utilization of reporting checklists suggests a need for greater emphasis on methodological transparency and adherence to established quality guidelines. This review highlights the importance of enhancing qualitative research practices to improve the rigor and reliability of studies, particularly in crisis contexts.
Peer Review reports
Introduction
The COVID-19 pandemic has threatened the health and well-being of global citizens which has led to a significant change in the attitude, lifestyle, and behavior of people from diverse professions [ 1 ]. Nurses have been and remain central to the pandemic––nurses are central to preventative, curative and palliative activities associated with COVID-19, and have taken these roles on in addition to their usual roles [ 2 ]. Nurses reported low job satisfaction, high levels of burnout, stress, and anxiety [ 3 ]. Researchers have experienced a decline in research motivation [ 4 ]. Scientific productivity, particularly among female academics, has suffered due to increased childcare responsibilities and psychological distress [ 5 ]. And parent researchers struggled to balance work and family responsibilities during the pandemic [ 6 ].
On the other hand, the travel restrictions and lockdown during the pandemic have undoubtedly affected all aspects of research, including qualitative research [ 7 , 8 ]. Qualitative nursing research is essential and important for understanding patient experiences, exploring complex healthcare phenomena, and guiding patient-centered care [ 9 ]. It provides insights into the subjective experiences, perceptions, and emotions of patients, families, and providers [ 10 ], bringing a holistic perspective to understanding the phenomena under study [ 11 ]. With qualitative methodologies, insight can be gained regarding the social responses to this pandemic, they are also the best methods to help explain, address, and plan for emergencies and pandemics, such as COVID-19 [ 7 , 12 , 13 ]. Restrictions during the pandemics made traditional data collection methods challenging [ 1 , 14 ]. Nurse researchers had to adapt to perform data collection in a virtual environment, shifting from face-to-face interviews to telephone or online meetings [ 1 ]; research participants were unwilling to show their faces at virtual meetings, and face-to-face interviews were only allowed with masks on [ 7 , 8 ]. These changes affected the quality and richness of data collection, missing important non-verbal elements such as attitude, gesture, and context [ 15 , 16 ].
Given the disruptive impact of the COVID-19 pandemic on nursing qualitative research activities, and deleterious effects on nurses, like emotional exhaustion [ 17 ], psychological distress [ 18 ], and burnout [ 3 , 19 ], but nurse researchers have also been very responsive to the pandemic, the Journal of Advanced Nursing has received hundreds of manuscripts focused on the pandemic, and more than 200 papers published on the COVID-19 pandemic in 2 years [ 2 ]. We doubted the quality of the publication. Scholarly journals are the most important media source for the dissemination of such research findings and information related to connecting this new evidence to practice [ 20 ] and nursing publication plays an essential role in improving nurses’ knowledge of new information and interesting this knowledge into nursing practice [ 21 ]. Together these phenomena might run the risk of producing poor quality qualitative research. Current literature provides two bibliometric analyses of COVID-19 research published in nursing journal, these provide the readers with only objective information on nursing publication related to COVID-19. The existing literature lacks comprehensive reviews that specifically focus on the characteristics and reporting quality of qualitative nursing research related to COVID-19. This study addresses this gap by providing a thorough analysis, which is crucial for guiding future research efforts and improving the overall quality of qualitative studies in nursing. By emphasizing the importance of maintaining high research quality, this study aims to contribute valuable insights that can inform future research, policymaking, and practice in nursing.
Providing a critical review of COVID-19 qualitative nursing research is an unmet need. To achieve this goal, we designed a systematic literature search including all available COVID-19 nursing qualitative articles using a large task force dedicated to the analysis of high-volume articles. We aimed at investigating the characteristics and the methodological quality assessment of reporting COVID-19 qualitative nursing publications.
We conducted a systematic literature search and a critical review using content analysis. This type of content analysis was to enable the production of measurements, occurrences, or comparisons through statistical or quantitative methods [ 22 ]. This review builds upon the methods utilized in two similar reviews [ 23 , 24 ], which assessed the characteristics of articles and described the methodological quality of the articles by presenting the percentage of compliance with each item of a standardized methodological reporting quality checklist. Our study adopted a pre-established checklist which was designed based on the Consolidated Criteria for Reporting Qualitative Research (COREQ) [ 25 ] and the Standards for Reporting Qualitative Research (SRQR) [ 26 ], along with other items identified in the literature to examine the quality of reporting in qualitative research.
This study is an ancillary study that extracted articles related to COVID-19 from the database of a large study aims to assess the characteristics and reporting quality using a qualitative approach in the field of nursing from 2012 to January 2023.
Search strategy
Several databases were consulted to ensure the inclusion of relevant studies in the field of nursing. The main databases are academic and medical databases, such as PubMed, CINAHL (Cumulative Index to Nursing and Allied Health Literature), Cairn, Embase, Web of science and Scopus. Document search strategies are developed using the MeSH thesaurus (Medical subject headings) and related keywords. The MEDLINE strategy has been developed and tested by the research team: “nursing research“[MH] OR “nursing research“[TW] OR (“nursing research“[Title/Abstract:~2]) OR nurs*[affiliation]) AND (“qualitative research“[MH] OR “qualitative research“[TIAB] OR “qualitative study“[TIAB] OR “qualitative studies“[TIAB] OR “grounded theory“[TIAB] OR “phenomenology“[TIAB] OR “ethnography“[TIAB] OR (“qualitative study“[Title/Abstract:~2] OR “qualitative studies“[Title/Abstract:~2] OR “qualitative research“[Title/Abstract:~2] OR “qualitative theory“[Title/Abstract:~2] OR “qualitative theories“[Title/Abstract:~2] OR “grounded study“[Title/Abstract:~2] OR “grounded studies“[Title/Abstract:~2] OR “grounded theory“[Title/Abstract:~2] OR “grounded theories“[Title/Abstract:~2] OR “grounded research“[Title/Abstract:~2] OR “ethnological study“[Title/Abstract:~2] OR “ethnological studies“[Title/Abstract:~2] OR “ethnological theory“[Title/Abstract:~2] OR “ethnological theories“[Title/Abstract:~2] OR “ethnological research“[Title/Abstract:~2] OR “phenomenological study“[Title/Abstract:~2] OR “phenomenological studies“[Title/Abstract:~2] OR “phenomenological theory“[Title/Abstract:~2] OR “phenomenological theories“[Title/Abstract:~2] OR “phenomenological research“[Title/Abstract:~2]. Then, a hand search was conducted to identified articles related to COVID-19. The literature search was performed between June 2023 to August 2023.
Inclusion and exclusion criteria
Any qualitative nursing research related to COVID-19 was included. The first authors must be nurses. The language was limited to English and French. Both peer-reviewed and pre-prints articles were included.
Articles related to non-human samples and full-text unavailable were excluded.
Article screening
We followed the PRISMA 2020 (Preferred Reporting Items for Systematic Reviews and Meta-Analyses) for article selection. All articles yielded through an initial search from the databases were exported into Rayyan Software, a web-based tool designed to conduct and coordinate systematic literature reviews. Hand search was performed to identify articles related to COVID-19, and duplicates were removed. Next, affiliations were examined to determine if the first author was a nurse, and then titles and abstracts were reviewed to determine if the publication met inclusion and exclusion criteria. Two researchers finished the screening independently. Any discrepant result was discussed by the two reviewers and resolved by consensus, or where necessary, a third researcher was involved. Finally, the articles that met the inclusion and exclusion criteria were selected for full-text reading.
Data extraction and data analysis
We used the pre-established checklist combining items from the SRQR and COREQ checklists and adding other items identified in the literature to answer the objective of this study. The checklist included 33 items seen in Tables 1 and 9 items regarding characteristics of the articles, and 24 items regarding methodological quality assessment. The checklist was pilot-tested and revised. Revisions were made after discussion among the researchers and included clarification of checklist items and the response of researchers to each item. For items of the characteristics of the articles, data were extracted to Excel (Excel 2020, Microsoft Excel, Redmond, WA, USA) for categorization. For items of methodological quality assessment, ATLAS.ti software (version 23.2.1) was used. All identified articles were imported into the software for content analysis with the use of a coding function, codes were created according to the items on the data extraction checklist, researcher read the content of the full-text articles one by one, then identified and coded the phrases according to the codes. For example, the code “field note” was created, and the researcher identified and coded the content if it is mentioned in the article. The frequency of each code was calculated to identify the methodological quality of the included articles.
Ethical considerations
This study is a review based on published articles; ethical approval was not required.
A total of 63,494 articles were registered in Rayyan software. Of these, 918 articles (1.44%) were related to COVID-19. After the exclusion of 56 articles due to duplication, the titles, and abstracts of all the articles were examined and 393 articles were excluded due to affiliations in which the first author was not a nurse. The remaining studies were reviewed in full-text. There were 20 articles excluded due to articles with a non-qualitative approach, 4 articles written in a foreign language were excluded, and 1 article was excluded because of full-texted unavailable. A total of 444 full-text articles related to COVID-19 were analyzed. The flowchart is presented in Fig. 1 .
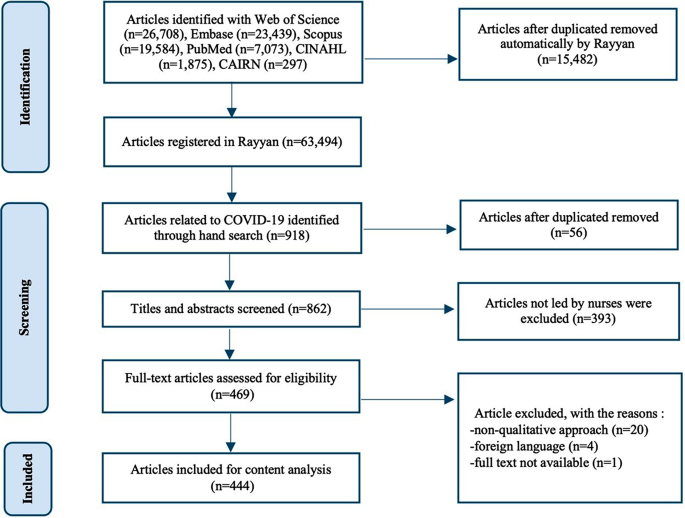
Flowchart of articles screening
Characteristics of the COVID-19 qualitative nursing research
The 444 included articles were published in 196 different journals, one of which was published on MedRxiv, an online pre-print platform for non-peer-reviewed research, with the most articles being published in the International Journal of Environmental Research and Public Health ( n = 28, 14.3%).
Table 2 shows the most productive journals in terms of COVID-19 nursing qualitative publications. With regard to the quartile of the journals, the studies were published most frequently in Q1 journals ( n = 260, 58.6%), followed by Q2 ( n = 118, 26.6%), Q3 ( n = 49, 11.0%), Q4 ( n = 12, 2.7%). The impact factors for each journal are grouped into 6 categories: Of the 444 articles, impact factor below 1 ( n = 54, 12.2%), impact factor between 1 and 1.999 ( n = 72, 16.2%). In addition, impact factor between 2 and 2.999 ( n = 107, 24.1%), impact factor between 3 and 3.999 ( n = 92, 20.7%), impact factor between 4 and 4.999 ( n = 87, 19.6%), and impact factor of 5 or higher ( n = 29, 6.5%). And 3 articles published in journals with an impact factor which is not applicable.
We then assessed the distribution of countries among all the included publications. The top 10 publishing countries were the United States ( n = 64, 14.4%), Iran ( n = 57, 12.8%), China ( n = 35, 7.9%), Turkey ( n = 33, 7.4%), Spain ( n = 32, 7.2%), Canada ( n = 22, 5.0%), Indonesia ( n = 19, 4.3%), Italy ( n = 16, 3.6%) and the United Kingdom ( n = 16, 3.6%) respectively, and South Korea ( n = 14, 3.2%), see Fig. 2 . Regarding the year of publication, 27 articles (6.1%) were published in 2020, 170 articles (38.3%) in 2021, and 240 articles (54.1%) in 2022.
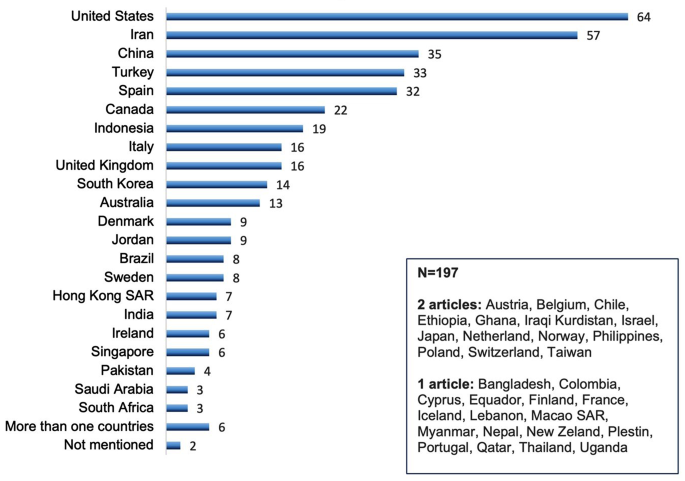
Distribution of countries of COVID-19 related qualitative nursing research published
The academic qualifications of the first authors were reported in 150 (33.8%) of the 444 articles. Of these, 113 (75.3%) first authors have a Ph.D degree ( n = 113, 75.3%), Ph.D. candidates ( n = 3, 2.0%), Ph.D. students ( n = 7, 4.7%), Master degree ( n = 23, 15.3%), Master students ( n = 2, 1.3%), and Bachelor degree ( n = 2, 1.3%). The affiliations of the first author were the universities ( n = 395, 89.7%), the hospitals ( n = 34, 7.7%), research centers ( n = 12, 2.7%), and independent researchers ( n = 1, 0.2%).
The focuses on COVID-19 qualitative nursing publications were categorized into 7 groups: workforce experience ( n = 213, 48.0%), pandemic restrictions experience ( n = 100, 22.5%), learning experience ( n = 44, 9.9%), infected COVID-19 experience ( n = 32, 7.2%), hospitalized experience ( n = 30, 6.8%), psychological perception ( n = 24, 5.4%), and guideline analysis ( n = 1, 0.2%) during the COVID-19 pandemic, see Fig. 3 . The population was mainly clinical nurses ( n = 197, 44.4%), nursing managers ( n = 15, 3.4%), nurse educators ( n = 5, 1.1%), nursing students ( n = 50, 11.3%), other healthcare professionals ( n = 18, 4.1%), COVID-19 patients ( n = 31, 7.0%), other patients ( n = 36, 8.1%), family members / caregivers ( n = 24, 5.4%), and public ( n = 68, 15.3%). Figures 4 and 5 shows the population distribution of the included articles.
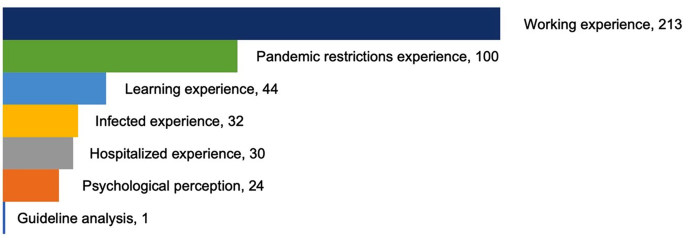
Focuses of COVID-19 qualitative nursing research
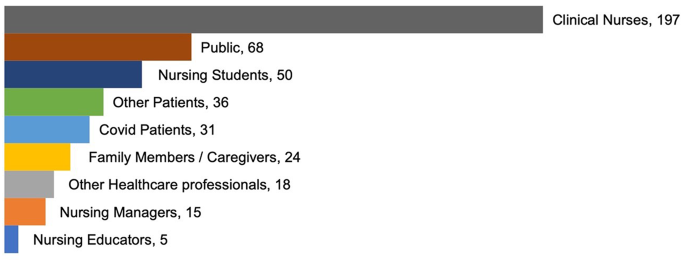
Target population of COVID-19 qualitative nursing research
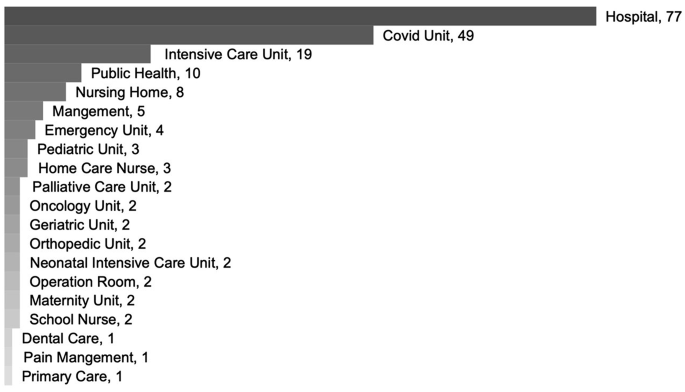
Distribution of clinical nurses
Methodological quality assessment of COVID-19 qualitative nursing research
Table 3 shows the prevalence of the items for reporting the methodological quality assessment of the included articles.
Methodological orientation
Of the 444 articles, the most adopted approach was the descriptive approach ( n = 165, 37.1%), Fig. 6 shows the types of approach adopted. Additionally, 84 (18.9%) of the articles only mentioned “qualitative study” without specifying which approach was being adopted.
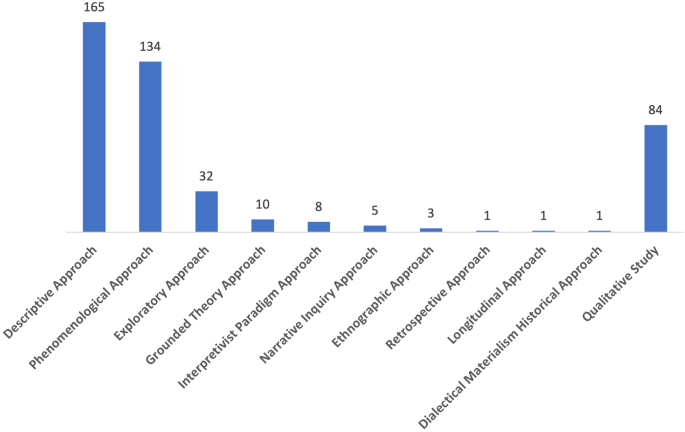
Types of approach adopted
Only one-third of the analyzed articles ( n = 135, 30.4%) mentioned employing standardized reporting quality checklists. Among these, the COREQ checklist was the most utilized ( n = 119, 26.8%), followed by the SRQR checklist ( n = 16, 3.6%).
Data collection
Among the articles included, 382 (86%) used interviews/discussions as a data collection method, 20 articles (4.5%) used mixed methods for data collection, and 42 articles (9.5%) that used methods other than interviews/discussions, 2.7% used surveys with open questions, 2.03% carried out document analysis, 1.8% examined diaries, 1.6% analyzed comments on social media, 0.5% used the photovoice method, and finally 0.2% carried out an analysis of audio-newspapers, an analysis of video diaries, an analysis of media interviews, only 1 article used observation as data collection method.
With the articles using interview/discussion methods, 261 articles (64.9%) specified who conducted the interviews. And 78 of them (19.4%) provided detailed information on their professional profiles. Most articles ( n = 327, 81.3%) mentioned the setting of data collection, with 65.4% ( n = 214) conducted remotely, 28.4% ( n = 93) conducted face-to-face, and 6.1% ( n = 20) indicated that the interviews were conducted whether remotely or face-to-face depending on participants’ wishes. The remoted interviews were conducted by teleconference ( n = 134, 57.3%), by telephone ( n = 66, 28.2%), and by teleconference or telephone ( n = 34, 14.5%), depending on the choice of participants. The software commonly used for teleconferencing was Zoom (44.5%), WhatsApp (11%), and Microsoft Teams (9.2%), while 35% did not mention which software was used, Fig. 7 shows the characteristics of data collection. Most articles ( n = 340, 84.8%) specified the duration of the interviews, they were described in two ways: mean duration ( n = 87, 25.6) or minimum and maximum duration ( n = 253, 74.4%). Audio recording was most used (86.7%), followed by visual recording (13.0%), and a few (0.3%) mentioned whether audio/visual recording was used. Most articles ( n = 351, 87.3%) provided interview guidelines, while only 16.9% ( n = 68) pre-tested them.
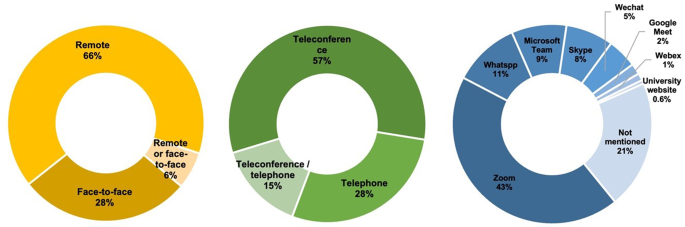
Characteristics of data collection
Participants
Most articles ( n = 434, 97.7%) mentioned the number of samples. Almost all the articles ( n = 443, 99.9%) provided a detailed description of the samples. The most common sampling method was purposive sampling ( n = 244, 66.8%), followed by convenience sampling ( n = 34, 9.3%) and snowball sampling ( n = 33, 9.0%). Some articles ( n = 54, 14.8%) used mixed sampling methods.
Data analysis
The commonly used methods of analysis were content analysis ( n = 149, 36.0%) and thematic analysis ( n = 143, 34.4%) (Fig. 8 ). And some articles did not specify which method was used ( n = 14, 3.4%). And the most common software chosen by the authors were NVivo (46.9%), MAXQDA (26.7%), and ATLAS.ti (16.0%).
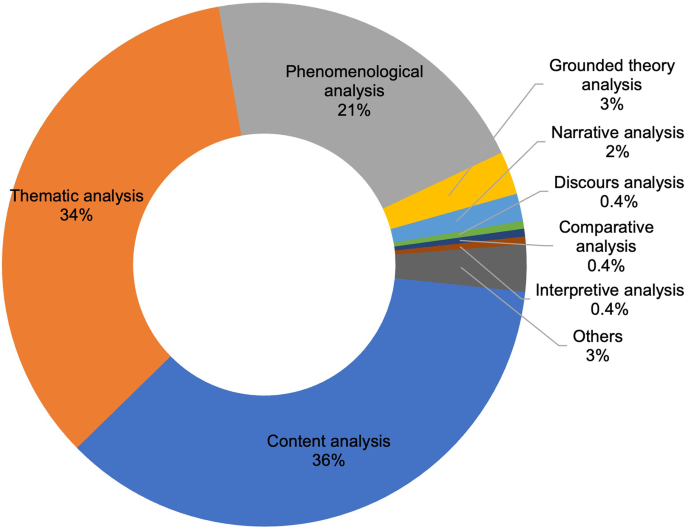
Methods of data analysis
Presentation of results
All the articles (100%) presented their results in narrative form. The majority (90.3%) presented quotations in their results. Only 4.7% presented code recurrence. 18.7% used graphics to present their results, and 9.2% mentioned participants checking reports.
This study focused on the identification of the characteristics and reporting quality of qualitative nursing published research related to COVID-19 pandemic. We used a systematic search approach to identify qualitative nursing studies published related to the COVID-19 and then carried out a critical review with the use of content analysis of the identified articles, relying on a checklist created based on two standardized checklists (SRQR and COREQ). A total of 444 published studies were included and critically reviewed. The most productive country was the USA, which corresponds with a bibliometric analysis of COVID-19 research published in a nursing journal. This can be explained by the fact that the USA is one of the most impacted countries by COVID-19 [ 27 ] and is one of the most prolific countries regarding nursing research [ 28 ]. A significant finding of our study is that the majority of articles were published in journals ranked within Quartile 1. This suggests that the research produced during this period not only addressed urgent topics but also met high academic standards.
In addition, the findings revealed that the most represented topics and target population were related to the workforce experience and clinical nurses respectively, this is consistent with an article that focused on the reflections on nursing research during the pandemic COVID-19 [ 2 ]. Interestingly, clinical nurses were the predominant target population of the articles reviewed, this is possibly attributable to the challenge of conducting research with patients and the public due to pandemic-related restrictions. This thematic focus is likely driven by the critical challenge and changes by clinical nurses during the pandemic, highlighting their significant role in the frontline response and the need to understand and support them.
It was surprising to see that the use of standardized checklists to guide research studies by the researchers was notably low, with only 30.4% mentioning the use of standardized checklists. This finding is particularly noteworthy in the context of qualitative nursing research during the COVID-19 pandemic, a period that demanded high-quality evidence to inform rapidly changing clinical practices. The low adoption rate of standardized checklists may reflect gaps in researchers’ awareness or accessibility to these tools, or perhaps a broader issue in the research culture that undervalues structured guidance in study design and reporting, as these checklists aim to improve the quality of reporting these study types and allow readers to better understand the design, conduct, analysis and findings of published studies [ 25 ].
Traditional qualitative research data collection methods like interviews and discussions were supposed to be most impacted by the pandemic. Surprisingly, 86% ( n = 382) of the included articles used interviews or discussions as the data collection methods, and 28% of the researchers remained choosing the face-to-face interview method. We questioned how communication and facial expression were observed if facemasks were worn during the interview. Among the included studies that used the interview method in data collection, a significant proportion with 66% of these interviews were conducted remotely, either by telephone or online. These findings align with the literature reviewed, where nursing researchers in the USA reported an increase in the use of online platforms, as well as sending emails and phone calls for data collection, a trend which has seen a significant increase [ 8 ]. Researchers in Japan also reported having to adapt their research methods according to changes in the research environment, moving from in-person interviews to remote telephone interviews, collecting data while maintaining the social distancing, and online data collection [ 8 ]. A randomized research study comparing online interviews to in-person interviews person to assess health conditions was conducted in Australia. The results of this study showed that online interviews were preferred by a greater proportion of participants than in-person interviews, and then those assigned to the online group had a lower dropout rate. Additionally, the use of online interviews did not result in a loss of data quality [ 29 ]. Another study also indicated that online modalities for conducting qualitative research did not lead to substantially different thematic findings than in-person data collection [ 30 ]. These suggest that remote data collection methods would be a good choice for researchers, especially in situations where face-to-face interactions are challenging or not possible. The success of remote interviews in maintaining data quality, participant engagement, and lower dropout rates indicates their viability as a robust alternative to traditional methods. This shift not only ensures the continuity of research during crises like the COVID-19 pandemic but also offers a flexible and efficient approach for future qualitative studies. Embracing remote data collection can enhance the adaptability of research designs and potentially broaden the reach and inclusivity of participant recruitment, making it a valuable methodological option for qualitative nursing researchers.
The adoption of software tools in data analysis was surprisingly low, with only 37% of studies utilizing such resources. This finding suggests a potential area for further development in qualitative research practices, particularly to enhance efficiency and collaboration, especially in scenarios necessitating remote work and data sharing, especially during the pandemic when social contact was limited. In addition, there are other benefits of using qualitative data analysis software, including freedom from manual and administrative tasks, saving time, greater flexibility, and improved validity and reliability, and traceability of qualitative research [ 31 ].
In summary, this study carried out an in-depth analysis of data relating to the journals, articles, researchers, and methods used, identifying both strengths and areas requiring improvement. It highlighted the editorial quality of the publications and the methodological diversity observed in qualitative nursing studies linked to the COVID-19 pandemic. We found that many articles demonstrated commendable transparency in explicitly detailing their research approach, data collection processes, sampling methods, and data analysis techniques. However, some areas need improvement. A key aspect is the insufficient representation of strategies to ensure study rigor, such as triangulation and validation by respondents. It is essential to include critical reflection on the role of researchers, potential biases and their influence during the analysis and selection of data for presentation. Additionally, discussions about data saturation and sequential analysis can significantly strengthen the quality of qualitative research reporting. It is important that authors not only explain the methods or techniques they used but also provide clear and detailed justifications for their choices.
The effective translation of nursing research into clinical practice is critical, especially as healthcare professionals heavily depend on the latest research to guide their practices and decisions. The variability in the quality and reliability of research articles can lead to the adoption of clinical practices that may not be supported by strong evidence, potentially affecting patient care and hindering the advancement of nursing practice [ 32 ]. Therefore, improving the transparency and rigor of research methodology reporting is essential to ensure that clinical practices are based on reliable and robust evidence. Our study highlights the importance of methodological clarity and the use of standardized checklists in guiding research, This is increasingly relevant as nursing research evolves to meet global health challenges. By ensuring the high quality of reporting qualitative research, we can better bridge the gap between research and clinical practice, leading to improved patient outcomes and more effective healthcare delivery.
Limitations
It is also essential to recognize that our research method may have some limitations. The diversity of qualitative research methods restricted our assessment to an overview of overall research reporting quality. Additionally, our inclusion criterion based on the first author as a nurse may have excluded studies conducted by nurse-led teams, but where academic conventions led to a different first author. The time limit of the database prevented us from including articles published after January 2023. Finally, we excluded articles not published in English or French, meaning that relevant articles in other languages may have been omitted.
Conclusions
In conclusion, we urge researchers to provide detailed information in their articles, thereby allowing audiences to carefully evaluate the effectiveness and adequacy of the methods and materials used to produce credible and useful results. We also recommend researchers to adopt validated critical appraisal checklists when conducting their studies. This study highlights the importance of continued reflection on qualitative research practices with a view to improving the reporting quality of future studies in the field of nursing, especially during the special period of a pandemic. Additionally, we plan to compare these results with ancillary studies to assess the characteristics and reporting quality of qualitative nursing research before the COVID-19 pandemic. In the future, we wish to open the way for future studies aimed at exploring the relationships between the different criteria identified and each qualitative approach.
Data availability
The data as well as detailed descriptions of the literature search and search outcome (including excluded articles) are available from the corresponding author upon request.
Abbreviations
Coronavirus disease of 2019
Consolidated Criteria for Reporting Qualitative Research
Standards for Reporting Qualitative Research
Medical Subject Heading
Cumulative Index to Nursing and Allied Health Literature
Preferred Reporting Items for Systematic Reviews and Meta-Analyses
United States of America
Sah LK, Singh DR, Sah RK. Conducting qualitative interviews using virtual communication tools amid COVID-19 pandemic: a learning opportunity for Future Research. J Nepal Med Association. 2020;58(232):1103–6. https://doi.org/10.31729/jnma.5738 .
Article Google Scholar
Jackson D. (2022). Reflections on nursing research focusing on the COVID-19 pandemic. Journal of advanced nursing. 2022;78(7);e84–e86; https://doi.org/10.1111/jan.15281 .
Galanis P, Vraka I, Fragkou D, Bilali A, Kaitelidou D. Nurses’ burnout and associated risk factors during the COVID-19 pandemic: a systematic review and meta-analysis. J Adv Nurs. 2021;77(8):3286–302. https://doi.org/10.1111/jan.14839 .
Article PubMed PubMed Central Google Scholar
Takeuchi A, Yokota S, Tomotaki A, Fukahori H, Shimpuku Y, Yoshinaga N. Relationship between research activities and individual factors among Japanese nursing researchers during the COVID-19 pandemic. PLoS ONE. 2022;17(8):e0271001. https://doi.org/10.1371/journal.pone.0271001 .
Article PubMed PubMed Central CAS Google Scholar
Gabster BP, Van Daalen K, Dhatt R, Barry M. Challenges for the female academic during the COVID-19 pandemic. Lancet (London England). 2020;395(10242):1968–70. https://doi.org/10.1016/S0140-6736(20)31412-4 .
Article PubMed CAS Google Scholar
Abshire DA, McDonnell KK, Donevant SB, Corbett CF, Tavakoli AS, Felder TM, Pinto BM. Pivoting Nursing Research and Scholarship during the COVID-19 pandemic. Nurs Res. 2021;70(3):165–72. https://doi.org/10.1097/NNR.0000000000000493 .
Webber-Ritchey KJ, Simonovich SD, Spurlark RS. COVID-19: qualitative research with vulnerable populations. Nurs Sci Q. 2021;34(1):13–9. https://doi.org/10.1177/0894318420965225 .
Article PubMed Google Scholar
Im EO, Sakashita R, Oh EG, Tsai HM, Chen CM, Lin CC, McCauley L. COVID-19 and nursing research across five countries/regions: commonalities and recommendations. Res Nurs Health. 2021;44(5):758–66. https://doi.org/10.1002/nur.22171 .
Polit DF, Beck CT. Nursing research: Generating and assessing evidence for nursing practice. 11th ed. Wolters Kluwer; 2020.
Sandelowski M. Whatever happened to qualitative description ? Res Nurs Health. 2020;23(4):4. https://doi.org/10.1002/1098-240x(200008)23 .
Creswell JW, Poth CN. Qualitative inquiry & research design: choosing among five approaches. 4th ed. SAGE; 2018.
Miles MB, Huberman AM. Qualitative data analysis: an expanded sourcebook. Sage; 1994.
Teti M, Schatz E, Liebenberg L. Methods in the Time of COVID-19: the vital role of qualitative inquiries. Int J Qualitative Methods. 2020;19. https://doi.org/10.1177/1609406920920962 .
Abdul Rashid N, Lee K, Jamil NA. Conducting qualitative research in the new norms: are we ready? Nurs Health Sci. 2021;23(4):967–73. https://doi.org/10.1111/nhs.12872 .
Prasad P. Crafting qualitative research: working in the postpositivist traditions : working in the postpositivist traditions. Routledge; 2015.
Presado MH, Baixinho CL, Oliveira ESF. Qualitative research in pandemic times. Investigação qualitativa em tempos de pandemia. Revista brasileira de enfermagem. 2021;74. https://doi.org/10.1590/0034-7167.202174Suppl101 . Suppl 1:e74Suppl101.
Sarabia-Cobo C, Pérez V, De Lorena P, Hermosilla-Grijalbo C, Sáenz-Jalón M, Fernández-Rodríguez A, Alconero-Camarero AR. Experiences of geriatric nurses in nursing home settings across four countries in the face of the COVID-19 pandemic. J Adv Nurs. 2021;77(2):869–78. https://doi.org/10.1111/jan.14626 .
Hamama L, Marey-Sarwan I, Hamama-Raz Y, Nakad B, Asadi A. Psychological distress and perceived job stressors among hospital nurses and physicians during the COVID-19 outbreak. J Adv Nurs. 2022;78(6):1642–52. https://doi.org/10.1111/jan.15041 .
Manzano-García G, Ayala-Calvo JC. The threat of COVID-19 and its influence on nursing staff burnout. J Adv Nurs. 2021;77(2):832–44. https://doi.org/10.1111/jan.14642 .
Daly J, Jackson D. Contexts of nursing: an introduction. Elsevier Health Sciences; 2020.
Oh J, Kim JA. A bibliometric analysis of COVID-19 research published in nursing journals. Sci Editing. 2020;7(2):118–24. https://doi.org/10.6087/kcse.205 .
Ezzy D. Qualitative analysis: practice and innovation. Taylor & Francis; 2002.
Raynaud M, Zhang H, Louis K, Goutaudier V, Wang J, Dubourg Q, Wei Y, Demir Z, Debiais C, Aubert O, Bouatou Y, Lefaucheur C, Jabre P, Liu L, Wang C, Jouven X, Reese P, Empana JP, Loupy A. COVID-19-related medical research: a meta-research and critical appraisal. BMC Med Res Methodol. 2021;21(1). https://doi.org/10.1186/s12874-020-01190-w .
Younas A, Pedersen M, Tayaben JL. Review of Mixed-Methods Research in nursing. Nurs Res. 2019;68(6):464–72. https://doi.org/10.1097/NNR.0000000000000372 .
Tong A, Sainsbury P, Craig J. Consolidated criteria for reporting qualitative research (COREQ): a 32-item checklist for interviews and focus groups. Int J Qual Health Care. 2007;19(6):349–57. https://doi.org/10.1093/intqhc/mzm042 .
O’Brien BC, Harris IB, Beckman TJ, Reed DA, Cook DA. Standards for reporting qualitative research: a synthesis of recommendations. Acad Med. 2014;89(9):1245–51. https://doi.org/10.1097/ACM.0000000000000388 .
Chahrour M, Assi S, Bejjani M, Nasrallah AA, Salhab H, Fares M, Khachfe HH. A bibliometric analysis of COVID-19 Research Activity: a call for increased output. Cureus. 2020;12(3):e7357. https://doi.org/10.7759/cureus.7357 .
Giménez-Espert MDC, Prado-Gascó V, Soto-Rubio A, Psychosocial, Risks. Work Engagement, and job satisfaction of nurses during COVID-19 pandemic. Front Public Health. 2020. https://doi.org/10.3389/fpubh.2020.566896 . 8;566896.
Peasgood T, Bourke M, Devlin N, Yang Y, Dalziel K. Randomised comparison of online interviews versus face-to-face interviews to value health states. Soc Sci Med. 2023. https://doi.org/10.1016/j.socscimed.2023.115818 . 1982(323);115818.
Guest G, Namey E, Chen M. A simple method to assess and report thematic saturation in qualitative research. PLoS ONE. 2020;15(5):e0232076. https://doi.org/10.1371/journal.pone.0232076 .
Cypress BS. Qualitative research: challenges and dilemmas. Dimens Crit Care Nurs. 2019;38(5):264–70. https://doi.org/10.1097/DCC.0000000000000374 .
Gaglio B, Henton M, Barbeau A, Evans E, Hickam D, Newhouse R, Zickmund S. Methodological standards for qualitative and mixed methods patient centered outcomes research. BMJ (Clinical Res ed). 2020;371:m4435. https://doi.org/10.1136/bmj.m4435 .
Download references
Acknowledgements
Not applicable.
This study received no specific funding from any funder.
Author information
Authors and affiliations.
Nursing Sciences Research Chair, Laboratory Educations and Health Promotion (LEPS), University Sorbonne Paris Nord, UFR SMBH, Villetaneuse, EA, 3412, F-93430, France
Ian-In Vong, Monique Rothan-Tondeur & Rita Georges Nohra
Nursing Sciences Research Chair, Assistance Publique-Hôpitaux de Paris (AP-HP), Paris, F- 75005, France
Monique Rothan-Tondeur
You can also search for this author in PubMed Google Scholar
Contributions
Ian-In Vong (I.I.V.) and Rita Georges Nohra (R.G.N.) independently conducted the article screening. Monique Rothan-Tondeur (M.R.T.) was consulted to resolve any discrepancies that arose during the screening process. I.I.V. and R.G.N. were primarily responsible for writing the main manuscript text. M.R.T. provided critical revisions and contributed intellectual content to the manuscript draft. All authors have read and approved the final manuscript.
Corresponding author
Correspondence to Ian-In Vong .
Ethics declarations
Ethic approval and consent to participate, consent for publication, competing interests.
The authors declare no competing interests.
Additional information
Publisher’s note.
Springer Nature remains neutral with regard to jurisdictional claims in published maps and institutional affiliations.
Rights and permissions
Open Access This article is licensed under a Creative Commons Attribution 4.0 International License, which permits use, sharing, adaptation, distribution and reproduction in any medium or format, as long as you give appropriate credit to the original author(s) and the source, provide a link to the Creative Commons licence, and indicate if changes were made. The images or other third party material in this article are included in the article’s Creative Commons licence, unless indicated otherwise in a credit line to the material. If material is not included in the article’s Creative Commons licence and your intended use is not permitted by statutory regulation or exceeds the permitted use, you will need to obtain permission directly from the copyright holder. To view a copy of this licence, visit http://creativecommons.org/licenses/by/4.0/ . The Creative Commons Public Domain Dedication waiver ( http://creativecommons.org/publicdomain/zero/1.0/ ) applies to the data made available in this article, unless otherwise stated in a credit line to the data.
Reprints and permissions
About this article
Cite this article.
Vong, II., Rothan-Tondeur, M. & Nohra, R.G. Characteristics and quality of reporting qualitative nursing research related to the COVID-19 pandemic: a systematic search and critical review. BMC Nurs 23 , 498 (2024). https://doi.org/10.1186/s12912-024-02138-x
Download citation
Received : 12 April 2024
Accepted : 01 July 2024
Published : 22 July 2024
DOI : https://doi.org/10.1186/s12912-024-02138-x
Share this article
Anyone you share the following link with will be able to read this content:
Sorry, a shareable link is not currently available for this article.
Provided by the Springer Nature SharedIt content-sharing initiative
- Nursing research
- Qualitative research
- Methodological quality
- Critical review
BMC Nursing
ISSN: 1472-6955
- General enquiries: [email protected]
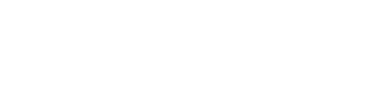
Verify originality of an essay
Get ideas for your paper
Find top study documents
What is qualitative research? Approaches, methods, and examples
Updated 23 Jul 2024
Students in social sciences frequently seek to understand how people feel, think, and behave in specific situations or relationships that evolve over time. To achieve this, they employ various techniques and data collection methods in qualitative research allowing for a deeper exploration of human experiences. Participant observation, in-depth interviews, and other qualitative methods are commonly used to gather rich, detailed data to uncover key aspects of social behavior and relationships. What is qualitative research? This article will answer this question and guide you through the essentials of this methodology, including data collection techniques and analytical approaches.
Qualitative research definition and significance
This inquiry method is helpful for learners interested in how to conduct research . It focuses on understanding human behavior, experiences, and social phenomena from the perspective of those involved. What does qualitative mean? It uses non-numerical data, such as interviews, observations, and textual analysis, to understand people’s feelings, thoughts, and actions.
Where and when is it used?
Qualitative analysis is crucial in education, healthcare, social sciences, marketing, and business. It helps gain detailed insights into behaviors, experiences, and cultural phenomena. This approach is fundamental during exploratory phases, for understanding complex issues, and when context-specific insights are required. By focusing on depth over breadth, this approach is often employed when researchers seek to explore complex issues, understand the context of a phenomenon, or investigate things that are not easily quantifiable. It uncovers rich, nuanced data essential for developing theories and evaluating programs.
Why is qualitative research important in academia?
- It sheds light on complex phenomena and human experiences that quantitative methods may overlook.
- This method offers contextual understanding by studying subjects in their natural environments, which is crucial for grasping real-world complexities.
- It adapts flexibly to evolving study findings and allows for adjusting approaches as new ideas emerge.
- It collects rich, detailed data through interviews, observations, and analysis, offering a comprehensive view of the exploration topic.
- Qualitative research studies focus on new or less explored areas, helping to identify key variables and generate hypotheses for further study.
- This approach focuses on understanding individuals' perspectives, motivations, and emotions, essential in fields like sociology, psychology, and education.
- It supports theory development by providing empirical data that can create new theories and frameworks (you may read about “What is a conceptual framework?” and learn about other frameworks on the EduBirdie website).
- It improves practices in fields such as education and healthcare by offering insights into practitioners' and clients' needs and experiences.
The difference between qualitative and quantitative studies
Now that you know the answer to “Why is qualitative data important?”, let’s consider how this method differs from quantitative. Both studies represent two main types of research methods. The qualitative approach focuses on understanding behaviors, experiences, and perspectives using interviews, observations, and analyzing texts. These studies are based on reflexivity and aim to explore complexities and contexts, often generating new ideas or theories. Researchers analyze data to find patterns and themes, clarifying the details. However, findings demonstrated in the results section of a research paper may not apply broadly because they often use small, specific groups rather than large, random samples.
Quantitative studies, on the other hand, emphasize numerical data and statistical analysis to measure variables and relationships. They use methods such as surveys, experiments, or analyzing existing data to collect structured information. The goal is quantifying phenomena, testing hypotheses, and determining correlations or causes. Statistical methods are used to analyze data, identifying patterns and significance. Quantitative studies produce results that can be applied to larger populations, providing generalizable findings. However, they may lack the detailed context that qualitative methods offer.
The approaches to qualitative research
To better understand the answer to “What is qualitative research?”, it’s necessary to consider various approaches within this methodology, each with its unique focus, implications, and functions.
1. Phenomenology.
This theory aims to understand and describe the lived experiences of individuals regarding a particular phenomenon.
Peculiarities:
- Focuses on personal experiences and perceptions.
- Seeks to uncover the essence of a phenomenon.
- Uses in-depth interviews and first-person accounts.
Example: Studying the experiences of people living with chronic illness to understand how it affects their daily lives.
2. Ethnography.
The approach involves immersive, long-term observation and participation in particular cultural or social contexts.
- Provides a deep understanding of cultural practices and social interactions.
- Involves participant observation and fieldwork.
- Researchers often live within the community they are studying.
Example: Observing and participating in the daily life of a rural village to understand its social structure and cultural practices.
3. Grounded theory.
This approach seeks to develop a research paper problem statement and theories based on participant data.
- Focuses on creating new theories rather than analyzing existing ones.
- Uses a systematic process of data collection and analysis.
- Involves constant comparison and coding of data.
Example: Developing a theory on how people cope with job loss by interviewing and analyzing the experiences of unemployed individuals.
4. Case study.
Case studies involve an in-depth examination of a single case or a small number of cases.
- Provides detailed, holistic insights.
- Can involve individuals, groups, organizations, or events.
- Uses multiple data sources such as interviews, observations, and documents.
Example: One of the qualitative research examples is analyzing a specific company’s approach to innovation to understand its success factors.
5. Narrative research.
This methodology focuses on the stories and personal interpretations of individuals.
- Emphasizes the chronological sequence and context of events.
- Seeks to understand how people make sense of their experiences.
- Uses interviews, diaries, and autobiographies.
Example: Collecting and analyzing the life stories of veterans to understand their experiences during and after military service.
6. Action research.
This theoretical model involves a collaborative approach in which researchers and participants work together to solve a problem or improve a situation.
- Aims for practical outcomes and improvements.
- Involves cycles of planning, acting, observing, and reflecting.
- Often used in educational, organizational, and community settings.
Example: Teachers collaborating with researchers to develop and test new teaching approaches to improve student engagement.
7. Discourse analysis.
It examines language use in texts, conversations, and other forms of communication.
- Focuses on how language shapes social reality and power dynamics.
- Analyzes speech, written texts, and media content.
- Explores the underlying meanings and implications of language.
Example: Analyzing political speeches to understand how leaders construct and convey their messages to the public.
Each of these examples of qualitative research offers unique tools and perspectives, enabling researchers to delve deeply into complex issues and gain a rich understanding of the issue they study.
Qualitative research methods
Various techniques exist to explore phenomena in depth and understand the complexities of human behavior, experiences, and social interactions. Some key methodologies that are commonly used in different sciences include several approaches.
Unstructured interviews;
These are informal and open-ended, designed to capture detailed narratives without imposing preconceived notions. Researchers typically start with a broad question and encourage interviewees to share their stories freely.
Semi-structured interviews;
They involve a core set of questions that allow researchers to explore topics deeply, adapting their inquiries based on responses received. This method of qualitative research design aims to gather rich, descriptive information, such as understanding what qualities make a good teacher.
Open questionnaire surveys;
They differ from closed-ended surveys in that they seek opinions and descriptions through open-ended questions. They allow for gathering diverse viewpoints from a larger group than one-on-one interviews would permit.
Observation;
It relies on researchers' skills to observe and interpret unbiased behaviors or activities. For instance, in education research, observation might track how students stay focused and manage distractions, recorded through field notes taken during or shortly after the observation.
Keeping logs and diaries;
This involves participants or researchers documenting daily activities or study contexts. Participants might record their social interactions or exercise routines, giving detailed data for later analysis. Researchers may also maintain diaries to document study contexts, helping to explain findings and other information sources.
All types of qualitative research have their strengths for gathering detailed information and exploring the social, cultural, and psychological aspects of exploration topics. Learners often use several methods (triangulation) to confirm their findings and deepen their understanding of complex subjects. If you need assistance choosing the most appropriate method to explore, feel free to contact our website, as we offer essays for sale and support with academic papers.
Advantages and disadvantages of the qualitative research methodology
This approach has unique strengths, making it valuable in many sciences. One of the primary advantages of qualitative research is its ability to capture participants' voices and perspectives accurately. It is highly adaptable, allowing researchers to modify the technique as new questions and ideas arise. This flexibility allows researchers to investigate new ideas and trends without being limited to set methods from the start. While this approach has many strengths, it also has significant drawbacks. A research paper writer faces practical and theoretical limitations when analyzing and interpreting data. Let’s consider all the pros and cons of this methodology in detail.
Strengths of qualitative research:
- Adaptability: Data gathering and analysis can be adjusted as new patterns or ideas develop, ensuring the study remains relevant and responsive.
- Real-world contexts: Research often occurs in natural conditions, providing a more authentic understanding of phenomena and describing the particularities of human behavior and interactions.
- Rich insights: Detailed analysis of people’s feelings, perceptions, and experiences can be useful for designing, testing, or developing systems, products, and services.
- Innovation: Open-ended responses allow experts to discover new problems or opportunities, leading to innovative ideas and approaches.
Limitations of qualitative research:
- Unpredictability: Real-world conditions often introduce uncontrolled factors, making this approach less reliable and difficult to replicate.
- Bias: The qualitative method relies heavily on the researcher’s viewpoint, leading to subjective interpretations. This makes it challenging to replicate studies and achieve consistent results.
- Limited applicability: Small, specific samples give detailed information but limit the ability to generalize findings to a broader population. Conclusions about the qualitative research topics may be biased and not representative of the wider population.
- Time and effort: Analyzing qualitative data is time-consuming and labor-intensive. While software can help, much of the analysis must be done manually, requiring significant effort and expertise.
So, qualitative methodology offers significant benefits, such as adaptability, real-world context, rich insights, and fostering innovation. However, it also presents challenges like unpredictability, bias, limited applicability, or time- and labor-intensive. Understanding these pros and cons helps researchers make informed decisions about when and how to effectively utilize various types of qualitative research designs in their studies.
Final thoughts
Qualitative research provides a valuable understanding of complicated human experiences and social situations, making it a strong tool in various areas of study. Despite its challenges, such as unreliability, subjectivity, and limited generalizability, its strengths in flexibility, natural settings, and generating meaningful insights make it an essential approach. If you are one of the students looking to incorporate qualitative methodology into their academic papers, EduBirdie is here to help. Our experts can guide you through the process, ensuring your work is thorough, credible, and impactful.
Was this helpful?
Thanks for your feedback.

Written by Elizabeth Miller
Seasoned academic writer, nurturing students' writing skills. Expert in citation and plagiarism. Contributing to EduBirdie since 2019. Aspiring author and dedicated volunteer. You will never have to worry about plagiarism as I write essays 100% from scratch. Vast experience in English, History, Ethics, and more.
Related Blog Posts
Discover how to compose acknowledgements in research paper.
This post will help you learn about the use of acknowledgements in research paper and determine how they are composed and why they must be present ...
How to conduct research: best tips from experienced EduBirdie writers
Have you ever got your scholarly task and been unsure how to start research? Are you a first-year student beginning your project? Whatever the case...
Research Papers on Economics: Writing Tips
More and more students nowadays are faced with the problem: How to write an economics paper. Research papers are one of the main results of researc...
Join our 150K of happy users
- Get original papers written according to your instructions
- Save time for what matters most

- Section 2: Home
- Developing the Quantitative Research Design
- Qualitative Descriptive Design
- Design and Development Research (DDR) For Instructional Design
- Qualitative Narrative Inquiry Research
What is a Qualitative Narrative Inquiry Design?
Tips for using narrative inquiry in an applied manuscript, summary of the elements of a qualitative narrative inquiry design, sampling and data collection, resource videos.
- Action Research Resource
- Case Study Design in an Applied Doctorate
- SAGE Research Methods
- Research Examples (SAGE) This link opens in a new window
- Dataset Examples (SAGE) This link opens in a new window
- IRB Resource Center This link opens in a new window
Narrative inquiry is relatively new among the qualitative research designs compared to qualitative case study, phenomenology, ethnography, and grounded theory. What distinguishes narrative inquiry is it beings with the biographical aspect of C. Wright Mills’ trilogy of ‘biography, history, and society’(O’Tolle, 2018). The primary purpose for a narrative inquiry study is participants provide the researcher with their life experiences through thick rich stories. Narrative inquiry was first used by Connelly and Calandinin as a research design to explore the perceptions and personal stories of teachers (Connelly & Clandinin, 1990). As the seminal authors, Connelly & Clandinin (1990), posited:
Although narrative inquiry has a long intellectual history both in and out of education, it is increasingly used in studies of educational experience. One theory in educational research holds that humans are storytelling organisms who, individually and socially, lead storied lives. Thus, the study of narrative is the study of the ways humans experience the world. This general concept is refined into the view that education and educational research is the construction and reconstruction of personal and social stories; learners, teachers, and researchers are storytellers and characters in their own and other's stories. In this paper we briefly survey forms of narrative inquiry in educational studies and outline certain criteria, methods, and writing forms, which we describe in terms of beginning the story, living the story, and selecting stories to construct and reconstruct narrative plots.
Attribution: Reprint Policy for Educational Researcher: No written or oral permission is necessary to reproduce a tale, a figure, or an excerpt fewer that 500 words from this journal, or to make photocopies for classroom use. Copyright (1990) by the American Educational Research Association; reproduced with permission from the publisher.
- Example Qualitative Narrative Inquiry Design
First, the applied doctoral manuscript narrative inquiry researcher should recognize that they are earning a practical/professional based doctorate (Doctor of Education), rather than a research doctorate such as a Ph.D. Unlike a traditional Ph.D. dissertation oral defense where the candidates focus is on theory and research, the NU School of Education applied doctoral candidate presents their finding and contributions to practice to their doctoral committee as a conceptual professional conference level presentation that centers on how their study may resolve a complex problem or issue in the profession. When working on the applied doctoral manuscript keep the focus on the professional and practical benefits that could arise from your study. If the Applied Doctoral Experience (ADE) student is unsure as to whether the topic fits within the requirements of the applied doctoral program (and their specialization, if declared) they should reach out to their research course professor or dissertation chair for guidance. This is known as alignment to the topic and program, and is critical in producing a successful manuscript. Also, most applied doctoral students doing an educational narrative inquiry study will want to use a study site to recruit their participants. For example, the study may involve teachers or college faculty that the researcher will want to interview in order to obtain their stories. Permission may be need from not only the NU Institutional Review Board (IRB), but also the study site. For example, conducting interviews on campus, procuring private school district or college email lists, obtaining archival documents, etc.
The popularity of narrative inquiry in education is increasing as a circular and pedagogical strategy that lends itself to the practical application of research (Kim, 2016). Keep in mind that by and large practical and professional benefits that arise from a narrative inquiry study revolve around exploring the lived experiences of educators, education administrators, students, and parents or guardians. According to Dunne (2003),
Research into teaching is best served by narrative modes of inquiry since to understand the teacher’s practice (on his or her own part or on the part of an observer) is to find an illuminating story (or stories) to tell of what they have been involved with their student” (p. 367).
- Temporality – the time of the experiences and how the experiences could influence the future;
- Sociality – cultural and personal influences of the experiences; and;
- Spatiality – the environmental surroundings during the experiences and their influence on the experiences.
From Haydon and van der Riet (2017)
- Narrative researchers collect stories from individuals retelling of their life experiences to a particular phenomenon.
- Narrative stories may explore personal characteristics or identities of individuals and how they view themselves in a personal or larger context.
- Chronology is often important in narrative studies, as it allows participants to recall specific places, situations, or changes within their life history.
Sampling and Sample Size
- Purposive sampling is the most often used in narrative inquiry studies. Participants must meet a form of requirement that fits the purpose, problem, and objective of the study
- There is no rule for the sample size for narrative inquiry study. For a dissertation the normal sample size is between 6-10 participants. The reason for this is sampling should be terminated when no new information is forthcoming, which is a common strategy in qualitative studies known as sampling to the point of redundancy.
Data Collection (Methodology)
- Participant and researcher collaborate through the research process to ensure the story told and the story align.
- Extensive “time in the field” (can use Zoom) is spent with participant(s) to gather stories through multiple types of information including, field notes, observations, photos, artifacts, etc.
- Field Test is strongly recommended. The purpose of a field study is to have a panel of experts in the profession of the study review the research protocol and interview questions to ensure they align to the purpose statement and research questions.
- Member Checking is recommended. The trustworthiness of results is the bedrock of high-quality qualitative research. Member checking, also known as participant or respondent validation, is a technique for exploring the credibility of results. Data or results are returned to participants to check for accuracy and resonance with their experiences. Member checking is often mentioned as one in a list of validation techniques (Birt, et al., 2016).
Narrative Data Collection Essentials
- Restorying is the process of gathering stories, analyzing themes for key elements (e.g., time, place, plot, and environment) and then rewriting the stories to place them within a chronological sequence (Ollerenshaw & Creswell, 2002).
- Narrative thinking is critical in a narrative inquiry study. According to Kim (2016), the premise of narrative thinking comprises of three components, the storyteller’s narrative schema, his or her prior knowledge and experience, and cognitive strategies-yields a story that facilitates an understanding of the others and oneself in relation to others.
Instrumentation
- In qualitative research the researcher is the primary instrument.
- In-depth, semi-structured interviews are the norm. Because of the rigor that is required for a narrative inquiry study, it is recommended that two interviews with the same participant be conducted. The primary interview and a follow-up interview to address any additional questions that may arise from the interview transcriptions and/or member checking.
Birt, L., Scott, S., Cavers, D., Campbell, C., & Walter, F. (2016). Member checking: A tool to enhance trustworthiness or merely a nod to validation? Qualitative Health Research, 26 (13), 1802-1811. http://dx.doi.org./10.1177/1049732316654870
Cline, J. M. (2020). Collaborative learning for students with learning disabilities in inclusive classrooms: A qualitative narrative inquiry study (Order No. 28263106). Available from ProQuest Dissertations & Theses Global. (2503473076).
Connelly, F. M., & Clandinin, D. J. (1990). Stories of Experience and Narrative Inquiry. Educational Researcher, 19 (5), 2–14. https://doi.org/10.1080/03323315.2018.1465839
Dunne, J. (2003). Arguing for teaching as a practice: A reply to Alasdair Macintyre. Journal of Philosophy of Education . https://doi.org/10.1111/1467-9752.00331
Haydon, G., & der Riet, P. van. (2017). Narrative inquiry: A relational research methodology suitable to explore narratives of health and illness. Nordic Journal of Nursing Research , 37(2), 85–89. https://doi.org/10.1177/2057158516675217
Kim, J. H. (2016). Understanding Narrative Inquiry: The crafting and analysis of stories as research. Sage Publications.
Kim J. H. (2017). Jeong-Hee Kim discusses narrative methods [Video]. SAGE Research Methods Video https://www-doi-org.proxy1.ncu.edu/10.4135/9781473985179
O’ Toole, J. (2018). Institutional storytelling and personal narratives: reflecting on the value of narrative inquiry. Institutional Educational Studies, 37 (2), 175-189. https://doi.org/10.1080/03323315.2018.1465839
Ollerenshaw, J. A., & Creswell, J. W. (2002). Narrative research: A comparison of two restorying data analysis approaches. Qualitative Inquiry, 8 (3), 329–347.
- << Previous: Design and Development Research (DDR) For Instructional Design
- Next: Action Research Resource >>
- Last Updated: Jul 28, 2023 8:05 AM
- URL: https://resources.nu.edu/c.php?g=1013605

© Copyright 2024 National University. All Rights Reserved.
Privacy Policy | Consumer Information

IMAGES
VIDEO
COMMENTS
Narrative analysis is a qualitative research method used to understand how individuals create stories from their personal experiences. There is an emphasis on understanding the context in which a narrative is constructed, recognizing the influence of historical, cultural, and social factors on storytelling.
Narrative analysis is a qualitative research methodology that involves examining and interpreting the stories or narratives people tell in order to gain insights into the meanings, experiences, and perspectives that underlie them. Narrative analysis can be applied to various forms of communication, including written texts, oral interviews, and ...
Simply put, narrative analysis is a qualitative analysis method focused on interpreting human experiences and motivations by looking closely at the stories (the narratives) people tell in a particular context. In other words, a narrative analysis interprets long-form participant responses or written stories as data, to uncover themes and meanings.
Narrative analysis in qualitative research is an invaluable tool to understand how people's stories and ability to self-narrate reflect the human experience. Qualitative data analysis can be improved through coding and organizing complete narratives. By doing so, researchers can conclude how humans process and move through decisions and life ...
Qualitative data analysis is a systematic process of examining non-numerical data to extract meaning, patterns, and insights. In contrast to quantitative analysis, which focuses on numbers and statistical metrics, the qualitative study focuses on the qualitative aspects of data, such as text, images, audio, and videos.
Narrative analysis is a method with a particular history and epistemology, and it is designed to answer certain types of research questions. As part of the growing recognition of the value and legitimacy of qualitative inquiry in psychology, narrative analysis is becoming increasingly articulated and refined.
Hence, understanding qualitative research analysis will greatly inform us, who are about to go into the details of narrative data analysis. I assume that you have taken one or two (or more) qualitative research courses as part of your program of study. As you know, data analysis in qualitative research in general is comprised of: examining raw ...
The quest for knowledge in this qualitative research ... narrative research requires personal involvement in how the researcher understands the stories of participants related to time and context, in a manner that establishes coherence and is connected to knowledge of existence through the systematic process of data collection, analysis, and ...
Even though narrative research shares features of other qualitative research approaches such as the social focus in ethnography and the focus on experience in phenomenology, it is the simultaneous ...
What is narrative research. In addition to narrative analysis, you can also practice narrative research, which is a type of study that seeks to understand and encapsulate the human experience by using in depth methods to explore the meanings associated to people's lived experiences.
Oct 5, 2023. Qualitative data analysis varies by methodology, so there is no one approach that fits across different types of studies. Narrative research is focused on the elicitation and interpretation of people's narrative accounts of their experience. A method based on the use of diaries which have been created for the purposes of research.
Narrative analysis in research. Narrative analysis is an approach to qualitative research that involves the documentation of narratives both for the purpose of understanding events and phenomena and understanding how people communicate stories. Collecting narrative data means focusing on individual research participants to understand particular ...
narrative identity research.11,12 One of the first narrative identity researchers was McAdams (2008), who defined ... Qualitative data analysis is simply "the process of making sense out of the data".10 To do this one immerses oneself in the data and consolidates the data focusing on those segments that may provide insight ...
Narrative research has many forms, uses a variety of analytic practices, and is rooted in different social and humanities disciplines (Daiute & ... Thus, the qualitative data analysis may be a description of both the story and themes that emerge from it. A postmodern narrative writer, such as Czarniawska (2004), would add another element to the
Narrative analysis is a valuable data analysis technique in qualitative research. It is typically used in those studies which have already employed narrative inquiry as a qualitative method. Narrative knowledge is created and constructed through the stories of lived experience and sense-making, the meanings people afford to them, and therefore offers valuable insight into the…
Several examples of narrative analysis illustrating when it is best used in qualitative research. Chapter 1: Narrative Analysis in Qualitative Research icon angle down
A comprehensive, thought-provoking introduction to narrative inquiry in the social and human sciences that guides readers through the entire narrative inquiry process--from locating narrative inquiry in the interdisciplinary context, through the philosophical and theoretical underpinnings, to narrative research design, data collection (excavating stories), data analysis and interpretation, and ...
Doing qualitative research is not easy and may require a complete rethink of how research is conducted, particularly for researchers who are more familiar with quantitative approaches. There are many ways of conducting qualitative research, and this paper has covered some of the practical issues regarding data collection, analysis, and management.
Abstract. Narrative research methodology is evolving, and we contend that the notion of emergent design is vital if narrative inquiry (NI) is to continue flourishing in generating new knowledge. We situate the discussion within the narrative turn in qualitative research while drawing on experiences of conducting a longitudinal narrative study.
Data analysis in qualitative research is defined as the process of systematically searching and arranging the interview transcripts, observation notes, or other non-textual materials that the researcher accumulates to increase the understanding of the phenomenon.7 The process of analysing qualitative data predominantly involves coding or ...
It enables researchers to draw nuanced conclusions and generate new insights. A narrative systematic review focuses on providing a detailed, qualitative synthesis of research findings on a specific topic. It begins with a clearly defined research question and employs a structured and thorough search strategy to identify all relevant studies ...
Narrative inquiry is relatively new among the qualitative research designs compared to qualitative case study, phenomenology, ethnography, and grounded theory. What distinguishes narrative inquiry is it beings with the biographical aspect of C. Wright Mills' trilogy of 'biography, history, and society' (O'Tolle, 2018).
Narrative inquiry is a form of qualitative research in which the stories themselves become the raw data. 3 This approach has been used in many disciplines to learn more about the culture, historical experiences, identity, and lifestyle…. Provide an example of when narrative inquiry would be the most appropriate qualitative research approach.
example, the Standards for Reporting Qualitative Research (known as SRQR) break qualitative research reports into 21 sections and provide detailed explanations of each section's components. See O'Brien et al. (2014) for more details. Equity Considerations . In reporting qualitative findings, consider all audiences, not just the funder. C ...
Narrative analysis is a means of interpreting how the participants in a research study tell the stories of their lives. In many qualitative research studies, participants are encouraged to make sense of the world around them through a first-person narrative structure.
Qualitative research is a type of research that aims to gather and analyse non-numerical (descriptive) data in order to gain an understanding of individuals' social reality, including understanding their attitudes, beliefs, and motivation. This type of research typically involves in-depth interviews, focus groups, or observations in order to collect data that is rich in detail and context.
Narrative inquiry is the analysis of a person's story, of an experience they have had. The goal of researcher is to draw out the story from the participant. ... Living in the research field, collecting data through observations (Connelly & Clandinin, 2006). ... Successful qualitative research: A practical guide for beginners. SAGE. Clandinin ...
The COVID-19 (Coronavirus disease of 2019) pandemic caused major disruption to nursing research, especially qualitative research. Researchers had to overcome numerous challenges that potentially impacted the quality of the studies carried out. The aim of this study is to assess the characteristics and quality of reporting qualitative nursing articles on the COVID-19 pandemic.
Example: One of the qualitative research examples is analyzing a specific company's approach to innovation to understand its success factors. 5. Narrative research. This methodology focuses on the stories and personal interpretations of individuals. Peculiarities: Emphasizes the chronological sequence and context of events.
Narrative inquiry is relatively new among the qualitative research designs compared to qualitative case study, phenomenology, ethnography, and grounded theory. What distinguishes narrative inquiry is it beings with the biographical aspect of C. Wright Mills' trilogy of 'biography, history, and society' (O'Tolle, 2018).