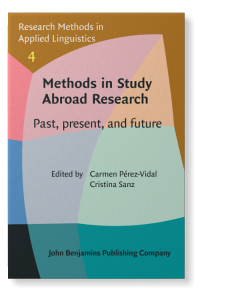

Methods in Study Abroad Research
Past, present, and future.
- Acknowledgements | pp. ix–10
- Foreword Barbara F. Freed | pp. 1–8
- Chapter 1. Research methods in study abroad : A critical state of the art account Carmen Pérez-Vidal and Cristina Sanz | pp. 9–30
- Section 1. Research designs
- Chapter 2. Quantitative approaches in L2 research abroad Janire Zalbidea and Bernard Issa | pp. 33–58
- Chapter 3. Qualitative approaches in L2 research abroad Rachel L. Shively | pp. 59–83
- Chapter 4. Mixed methods research on language learning in study abroad Celeste Kinginger and Robert W. Schrauf | pp. 85–106
- Section 2. Research instruments
- Chapter 5. Diaries and journals in study abroad research John L. Plews, Kim Misfeldt and Richard Feddersen | pp. 109–134
- Chapter 6. Narratives and identity in study abroad research Henry Tyne | pp. 135–156
- Chapter 7. Technology in cognitive research : Methods to examine second language processing in study abroad research Mandy Faretta-Stutenberg, Irene Finestrat and Kara Morgan-Short | pp. 157–179
- Section 3. Measuring L2 development
- Chapter 8. Oral fluency measures in study abroad research Joan C. Mora, Sapna Sehgal and Raquel Serrano | pp. 183–207
- Chapter 9. Complexity, fluency and accuracy in study abroad research Silvia Marijuan and Cristi Vallejos | pp. 209–238
- Chapter 10. Grammatical development during study abroad : Approaches and methods Martin Howard | pp. 239–263
- Chapter 11. Investigating pragmatic development in study abroad contexts Richard Nightingale and Eva Alcón-Soler | pp. 265–289
- Chapter 12. Phonological and phonetic development in study abroad research Alfonso Morales-Front | pp. 291–315
- Section 4. The individual learner in context
- Chapter 13. Individual variables in study abroad contexts : Concepts and measurements Ágnes Albert, Harriet Wood Bowden and Kata Csizér | pp. 319–344
- Chapter 14. Researching study abroad : Tracking social networks Rosamond Mitchell | pp. 345–372
- Afterword Barbara A. Lafford | pp. 373–382
- Index | pp. 383–393
Linguistics
Main bic subject, main bisac subject.
Methods in Study Abroad Research: Past, present, and future
About this ebook, rate this ebook, reading information, continue the series.
More by Carmen Pérez-Vidal
Similar ebooks

Study Abroad and Student Decision Making in Times of COVID: A Mixed Methods Study
- Gorka Basterretxea Santiso Georgetown University
- Cristina Sanz Georgetown University
The impacts of Covid-19 on Study abroad (SA) are deep and widespread and potentially affect the factors that guide US undergraduate students’ choices as they relate to SA. To investigate them, the present study relies on the MSA (Motivation to SA questionnaire: Anderson & Lawton, 2015) and adds two new groups of factors: language learning and health. To observe changes in the relative weight of factors before and during the pandemic, responses to two different versions of the MSA from 83 students who had participated in SA prior to the pandemic were compared. Results from statistical analyses show stability in the ranking of importance of factors pre- and during pandemic, except for health-related factors, which come second after world enlightenment. In addition, interviews show that while students view immersive SA as a significant experience for its contribution to their personal and intellectual development, they do not consider virtual SA a real SA experience.
Los impactos del Covid-19 en los programas de estudio en el extranjero son amplios y profundos y potencialmente afectan los factores que guían la decisión de los estudiantes de grado en EE.UU. a la hora de estudiar en otro país. Para poder investigarlo, el presente estudio utiliza el MSA (el cuestionario Motivation to Study Abroad : Anderson & Lawton, 2015) y añade dos nuevos grupos de factores: aprendizaje de lenguas y salud. Para observar cambios en la importancia relativa de los factores antes y durante la pandemia, se han comparado las respuestas a dos diferentes versiones del MSA de 83 estudiantes que estudiaron en el extranjero antes de la pandemia. Los resultados de los análisis estadísticos muestran estabilidad en el orden de importancia de los factores antes y durante la pandemia, con la excepción de los factores relacionados con la salud, que se encuentran en segundo lugar después de los factores relacionados con la formación global. Además, las entrevistas sugieren que mientras que los estudiantes consideran la inmersión en el extranjero como una experiencia importante para su desarrollo personal e intelectual, no consideran los programas virtuales como una experiencia real de aprendizaje en el extranjero.
Author Biographies
Gorka basterretxea santiso, georgetown university.
Gorka Basterretxea Santiso, MA, MS, is a doctoral candidate in Spanish sociolinguistics at Georgetown University. At Georgetown, he serves as Graduate Teaching Associate, Assistant Director for Intermediate Spanish, and Graduate Teaching Assistant in the Georgetown@Barcelona Summer Program. He was also co-chair for the annual graduate conference GRAPHSY 2021 at Georgetown University.
Cristina Sanz, Georgetown University
Cristina Sanz, PhD, is Professor of Spanish & Linguistics at Georgetown University, where she directs the Georgetown@Barcelona Summer Program; her term as a chair just ended. She is co-editing a volume on methods in study abroad research for John Benjamins and has co-edited the Routledge Handbook of Study Abroad Research and Practice. She received the President’s Award for Distinguished Scholars-Teachers (2019) and the AAUSC Innovation in Language Program Direction Award for Inclusion and Social Justice (2020).
Albert, A., & Csizér, K. (Forthcoming). Individual variables in study abroad contexts: Concepts and measurements. In C., Pérez-Vidal & C. Sanz (Eds.), Methods in Study Abroad Research: Past, Present and Future. Amsterdam: John Benjamins.
Allen, H. W. (2010). Language-learning motivation during short-term study abroad: An activity theory perspective. Foreign Language Annals, 43, 27-49. https://doi.org/10.1111/j.1944-9720.2010.01058.x DOI: https://doi.org/10.1111/j.1944-9720.2010.01058.x
Altbach, P., & de Wit, H. (2020, April 4). Post pandemic outlook for HE is bleakest for the poorest. University World News, The Global Window on Higher Education. https://www.universityworldnews.com/post.php?story=20200402152914362
Amoah, P., & Mok, K. H. (2020, December 7). The Covid-19 pandemic and internationalization of higher education: International students’ knowledge, experiences and wellbeing. Emerald Publishing. https://www.emeraldgrouppublishing.com/opinion-and-blog/covid-19-pandemic-and-internationalisation-higher-education-international-students
Anderson, P. H., Hubbard, A., Lawton, L. (2015). Student Motivation to Study Abroad and Their Intercultural Development. Frontiers: The Interdisciplinary Journal of Study Abroad, 26(1), 39-52. https://doi.org/10.36366/frontiers.v26i1.354 DOI: https://doi.org/10.36366/frontiers.v26i1.354
Anderson, P. H., & Lawton, L. (2015). The MSA: An Instrument for Measuring Motivation to Study Abroad. Frontiers: The Interdisciplinary Journal of Study Abroad, 26(1), 52-67. https://doi.org/10.36366/frontiers.v26i1.357 DOI: https://doi.org/10.36366/frontiers.v26i1.357
Austin, L., & Shen, L. (2016). Factors influencing Chinese students’ decisions to study in the United States. Journal of International Students, 6(3), 722-732. https://doi.org/10.32674/jis.v6i3.353 DOI: https://doi.org/10.32674/jis.v6i3.353
Bandyopadhyay, S., & Bandyopadhyay, K. (2015). Factors Influencing Student Participation in College Study Abroad Programs. Journal of International Education Research, 11(2), 87-94. https://doi.org/10.19030/jier.v11i2.9189 DOI: https://doi.org/10.19030/jier.v11i2.9189
Bilecen, B. (2020). Commentary: COVID-19 Pandemic and Higher Education: International Mobility and Students’ Social Protection. International Migration, 58(4), 263-266. https://doi.org/10.1111/imig.12749 DOI: https://doi.org/10.1111/imig.12749
Braun, V., & Clarke, V. (2006). Using thematic analysis in psychology. Qualitative Research in Psychology, 3(2), 77-101. http://dx.doi.org/10.1191/1478088706qp063oa DOI: https://doi.org/10.1191/1478088706qp063oa
Cebolla-Boado, H., Hu, Y., & Soysal, Y. N. (2018). Why study abroad? Sorting of Chinese students across British universities. British Journal of Sociology of Education, 39(3), 365-380. https://doi.org/10.1080/01425692.2017.1349649 DOI: https://doi.org/10.1080/01425692.2017.1349649
Chao, C. N., Hegarty, N., Angelidis, J., & Lu, V. F. (2017). Chinese students’ motivations for studying in the United States. Journal of International Students, 7(2), 257-269. https://doi.org/10.32674/jis.v7i2.380 DOI: https://doi.org/10.32674/jis.v7i2.380
De Jong, P., Schnusenberg, O., & Goel, L. (2010). Marketing Study Abroad Programs Effectively: What do American Business Students Think? Journal of International Education in Business, 3(1/2), 34-52. https://doi.org/10.1108/18363261011106876 DOI: https://doi.org/10.1108/18363261011106876
DeKeyser, R. M. (2014). Research on language development during study abroad: Methodological considerations and future perspectives. In C. Pérez-Vidal (Ed.), Language acquisition in study abroad and formal instruction contexts (pp. 313-327). Amsterdam/Philadelphia: John Benjamins. https://doi.org/10.1075/aals.13 DOI: https://doi.org/10.1075/aals.13.16ch13
Dennis, M. J. (2020). COVID-19 will accelerate the decline in international student enrollment. Recruiting & Retaining Adult Learners, 22, 1-7. https://doi.org/10.3390/languages6010006 DOI: https://doi.org/10.1002/nsr.30639
Dietrich, A. J. (2020). Charting a Path Forward for Education Abroad Research. Frontiers: The Interdisciplinary Journal of Study Abroad, 32(2), 1-11. https://doi.org/10.36366/frontiers.v32i2.465 DOI: https://doi.org/10.36366/frontiers.v32i2.465
Eder, J., Smith, W. W., & Pitts, R. E. (2010). Exploring factors influencing student study abroad decision choice. Journal of Teaching in Travel and Tourism, 10(3), 232-250. https://doi.org/10.1080/15313220.2010.503534 DOI: https://doi.org/10.1080/15313220.2010.503534
Fischer, K. (2020, May 22). To Keep International Students During the Pandemic, Colleges Get Creative. The Chronicle of Higher Education. https://www.chronicle.com/article/to-keep-international-students-during-the-pandemic-colleges-get-creative?cid2=gen_login_refresh&cid=gen_sign_in
Fischer, K. (2021a, April 1). The Year Without Study Abroad. Covid-19 could lead to lasting change in international learning. The Chronicle of Higher Education. https://www.chronicle.com/article/the-year-without-study-abroad
Fischer, K. (2021b). Today’s Global Campus: Strategies for reviving international enrollments and study abroad. The Chronicle of Higher Education.
Flaherty, G. T., & Nasir, N. (2020). Reiseangst: travel anxiety and psychological resilience during and beyond the COVID-19 pandemic. Journal of travel medicine, 1-2. https://doi.org/10.1093/jtm/taaa150 DOI: https://doi.org/10.1093/jtm/taaa150
Gidick, K. (2021, July 30). College students weigh risks and rewards of studying abroad in pandemic era. The Washington Post. https://www.washingtonpost.com/local/education/study-abroad-programs-covid/2021/07/30/5843a6f0-ee1e-11eb-81d2-ffae0f931b8f_story.html
Goldstein, S. B., & Kim, R. I. (2006). Predictors of U.S. College Students’ Participation in Study Abroad Programs: A Longitudinal Study. International Journal of Intercultural Relations, 30(4), 507-521. https://doi.org/10.1016/j.ijintrel.2005.10.001 DOI: https://doi.org/10.1016/j.ijintrel.2005.10.001
Helms, R. M. (2020, March 4). Can internationalization survive coronavirus? You need to see my data. Higher Education Today. https://www.higheredtoday.org/2020/03/04/can-internationalization-survive-coronavirus-need-see-data/
IIE, Institute of International Education. (2020). Internationalizing the Campus at Home. https://www.iie.org/en/Research-and-Insights/Publications/Internationalizing-the-Campus-at-Home
Leask, B., & Green, W. (2020, May 2). Is the pandemic a watershed for internationalization? University World News, The Global Window on Higher Education. https://www.universityworldnews.com/post.php?story=20200501141641136
Li, M., Olson, J. E., & Frieze, I. H. (2013). Students’ Study Abroad Plans: The Influence of Motivational and Personality Factors. Frontiers: The Interdisciplinary Journal of Study Abroad, 23, 73-89. https://doi.org/10.36366/frontiers.v23i1.330 DOI: https://doi.org/10.36366/frontiers.v23i1.330
Mercado, S. (2020, June 11). International student mobility and the impact of the pandemic. BizEd: AACSB International. https://bized.aacsb.edu/articles/2020/june/covid-19-and-the-future-of-international-student-mobility
Mok, K. H. (2020, July 4). Will Chinese students want to study abroad post-COVID-19? University World News, The Global Window on Higher Education. https://www.universityworldnews.com/post.php?story=20200703155021111
Mok, K. H., Xiong, W., Ke, G., & Cheung, J. O. W. (2020). Impact of COVID-19 Pandemic on International Higher Education and Student Mobility: Student Perspectives from Mainland China and Hong Kong. International Journal of Educational Research, 105, 1-11. https://doi.org/10.1016/j.ijer.2020.101718 DOI: https://doi.org/10.1016/j.ijer.2020.101718
Nyaupane, G. P., Paris, C. M., & Teye, V. (2011). Study abroad motivations, destination selection and pre-trip attitude formation. International Journal of Tourism Research, 13(3), 205-217. https://doi.org/10.1002/jtr.811 DOI: https://doi.org/10.1002/jtr.811
Oliveira, D. B., & Soares, A. M. (2016). Studying abroad: Developing a model for the decision process of international students. Journal of Higher Education Policy and Management, 38(2), 126-139. https://doi.org/10.1080/1360080X.2016.1150234 DOI: https://doi.org/10.1080/1360080X.2016.1150234
Oswald, F. L., & Plonsky, L. (2010). Meta-analysis in second language research: Choices and challenges. Annual Review of Applied Linguistics, 30, 85-110. https://doi.org/10.1017/S0267190510000115 DOI: https://doi.org/10.1017/S0267190510000115
Ożańska-Ponikwia, K., & Carlet, A. (2021). Should I stay or should I go? Factors that influence one’s decision to participate in a student mobility programme. In M. Howard (Ed.), Study Abroad and the Second Language Learner: Expectations, Experiences and Development (pp. 33-48). Bloomsbuty Collections. http://dx.doi.org/10.5040/9781350104228.ch-002 DOI: https://doi.org/10.5040/9781350104228.ch-002
Pedersen, E. R., Fitzke, R. E., Bouskill, K. E., & Sedano, A. (2021). A Qualitative Look at the Impact of COVID-19 Pandemic on American College Students Studying Abroad. Frontiers: The Interdisciplinary Journal of Study Abroad, 33(3), 70-100. https://doi.org/10.36366/frontiers.v33i3.602 DOI: https://doi.org/10.36366/frontiers.v33i3.602
QS. (2020). How Covid-19 is impacting prospective international students across the globe. https://www.qs.com/portfolio-items/how-covid-19-impacting-prospective-international-students-different-study-levels/
Salisbury, M. H., Umbrach, P. D., Paulsen, M. B., & Pascarella, E. T. (2009). Going Global: Understanding the Choice Process of the Intent to Study Abroad. Research in Higher Education, 50(1), 119-143. https://doi.org/10.1007/s11162-008-9111-x DOI: https://doi.org/10.1007/s11162-008-9111-x
Shively, R. L. (2018). Language socialization during study abroad: Researching social interaction outside the classroom. In S. Coffey & U. Wingate (Eds.), New directions for research in foreign language education (pp. 97-112). New York: Routledge. DOI: https://doi.org/10.4324/9781315561561-7
Stroud, A. H. (2010). Who Plans (Not) to Study Abroad? An Examination of U.S. Student Intent. Journal of Studies in International Education, 14(5), 491-507. https://doi.org/10.1177%2F1028315309357942 DOI: https://doi.org/10.1177/1028315309357942
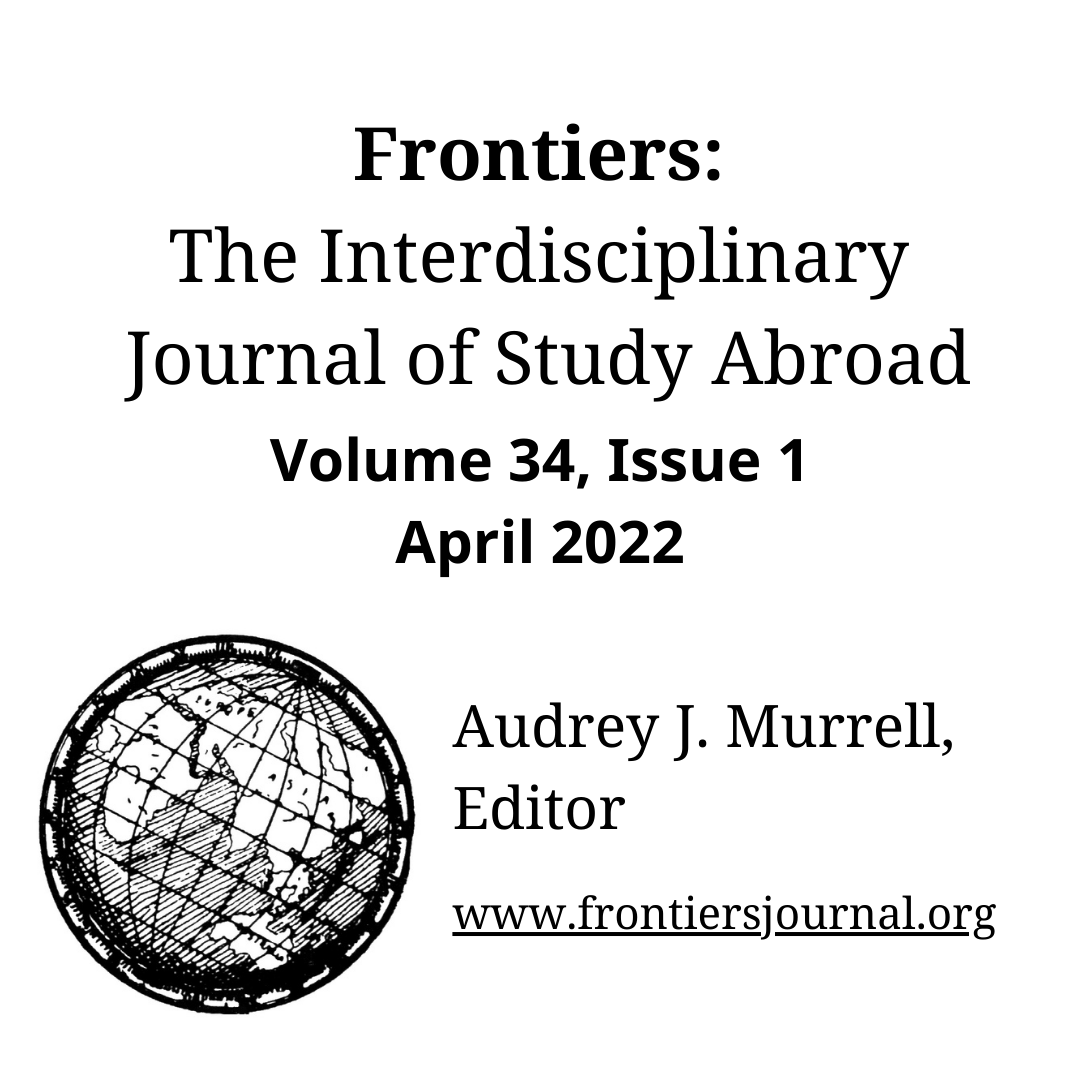
How to Cite
- Endnote/Zotero/Mendeley (RIS)
Copyright (c) 2022 Gorka Basterretxea Santiso, Cristina Sanz

This work is licensed under a Creative Commons Attribution-NonCommercial-NoDerivatives 4.0 International License .
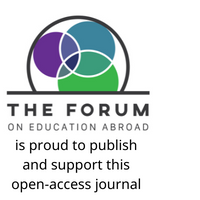
Make a Submission
Information.
- For Readers
- For Authors
- For Librarians
advertisement
Other Titles by our Publisher:
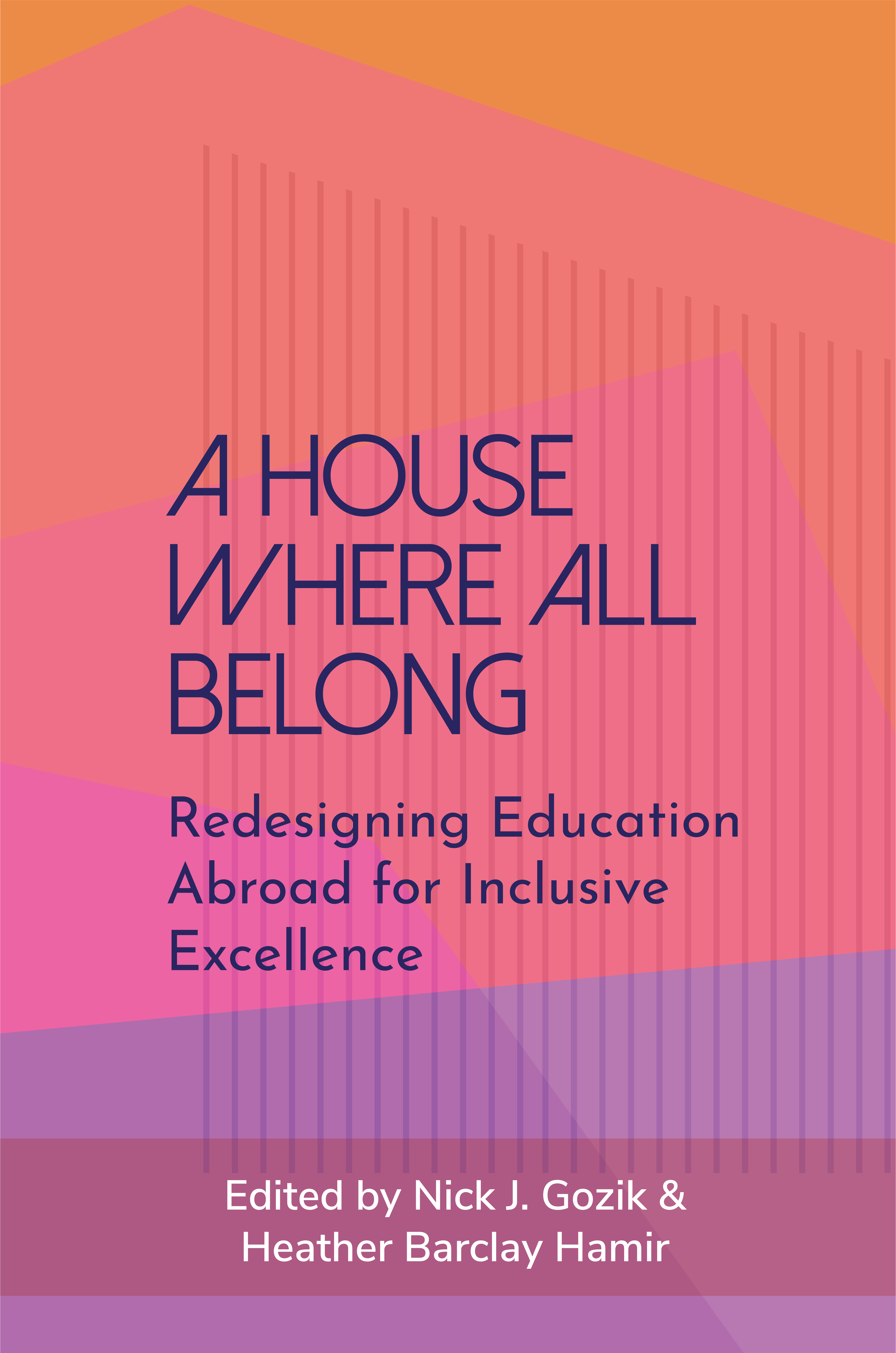
DIGITAL ISSN 2380-8144 | PRINT ISSN (pre-2013) 1085-4568
© 2022 The Forum on Education Abroad
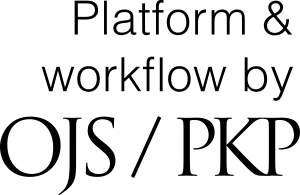
- New York Times Bestsellers
- Publishers Weekly Bestsellers
- E-book Bestsellers
- Taylor and Francis eBooks
- Oxford University Press e-products
- Ebook Central ebooks platform
- Springer eBooks
- Wiley Digital Books
- Eesti keeles
- Lietuviškai
- Grāmatas angļu valodā Grāmatas angļu valodā Grāmatas vācu valodā Grāmatas krievu valodā Estonian Books E-grāmatas angļu valodā ELT grāmatas Mūzikas grāmatas
- Grāmatas » 0 skaits 0,00 €
- E-Books » 0 skaits 0,00 €
- E-dāvanu kartes » 0 skaits 0,00 €
- ELT Books » 0 skaits 0,00 €
- Giftbooks » 0 skaits 0,00 €
- Grozs ir tukšs
- Vēlmju saraksts
- Ienākt Jauns konts
- Jūsu profils
- Nomainīt datus
- Nomainīt paroli
- Dāvanu kartes
- Personīgie iestatījumi
- Saņemtas noliktavā no izdevēja
- Pasūtījumu saraksts
- Atceltie pasūtījumi
- Iegādātās e-dāvanu kartes
- Grāmatas »
- Methods in Study Abroad Research: Past, present, and future
Methods in Study Abroad Research: Past, present, and future [Hardback]
- Hardback 118,34 €
- Ebook - EPUB+DRM 98,74 €
- Ebook - PDF+DRM 98,74 €
- Formāts: Hardback, 393 pages, weight: 855 g
- Sērija : Research Methods in Applied Linguistics 4
- Izdošanas datums: 03-Feb-2023
- Izdevniecība: John Benjamins Publishing Co
- ISBN-10: 9027212864
- ISBN-13: 9789027212863
- Language acquisition - (Noliktavā: 2 punkts )
- Cena: 118,34 €*
- * ši ir gala cena, t.i., netiek piemērotas nekādas papildus atlaides
- Grāmatu piegādes laiks ir 3-4 nedēļas, ja grāmata ir uz vietas izdevniecības noliktavā. Ja izdevējam nepieciešams publicēt jaunu tirāžu, grāmatas piegāde var aizkavēties.
- Ielikt grozā
- Piegādes laiks - 4-6 nedēļas
- Pievienot vēlmju sarakstam

- Language and languages - Study and teaching Higher
- Foreign study
- Second language acquisition
- Goodreads reviews
- Help and Support
Konts un iestatījumi
- Iepirkumu grozs
- Meklēt datubāzē
Tēmas Grāmatas angļu valodā
- Arts and Architecture (1026485)
- Biography & True Stories (764226)
- Children's, young adult & educational (2505487)
- Computing & information technology (380558)
- Earth sciences, geography, environment, planning (272279)
- Economics, finance, business & management (1186505)
- English language teaching (ELT) (59128)
- Fiction & related items (3482607)
- Health & personal development (1306296)
- Humanities (3565857)
- Language (540460)
- Law (647929)
- Lifestyle, sport & leisure (2161938)
- Literature & literary studies (2547772)
- Mathematics & science (781938)
- Medicine (723835)
- Reference, information & interdisciplinary subjects (555596)
- Society & social sciences (2550966)
- Technology, engineering, agriculture (608053)
- --> Apakšgrupa
Izvēlieties iepirkumu grozu
- E-dāvanu karšu pasūtījumi
- Iet uz iepirkumu grozu
Ignorēt un rādīt lapu

An official website of the United States government
The .gov means it’s official. Federal government websites often end in .gov or .mil. Before sharing sensitive information, make sure you’re on a federal government site.
The site is secure. The https:// ensures that you are connecting to the official website and that any information you provide is encrypted and transmitted securely.
- Publications
- Account settings
Preview improvements coming to the PMC website in October 2024. Learn More or Try it out now .
- Advanced Search
- Journal List
- HHS Author Manuscripts

Methods in Microbiome Research: Past, Present and Future
Emily c. gotschlich.
NIH/NIAMS, Building 4 Room 228, 4 Memorial Drive, Bethesda, MD 20892
Robert A. Colbert
Pediatric Translational Research Branch, 10 Center Drive, Bldg. 10, Rm 12N240E, Bethesda, MD 20892
Tejpal Gill
Oregon Health and Science University, 3215 SW Pavilion Loop, Lamfrom Biomedical Research Building 253E, Portland, OR 97239
The human microbiome is impressively immense and participates in many aspects of our health and wellness, particularly involving development and maintenance of a healthy immune system. Not only do our microbes teach the immune system to fight infection, they also teach immune tolerance and help maintain homeostasis. From this knowledge, we have learned that the loss of tolerance to microbiota in both innate and adaptive processes play an important role in immune-mediated and autoimmune disease. In this chapter, we will discuss methods used to study the microbiome, both old and new, fundamental concepts that have taken hold within the field, and how these principles relate to rheumatology including thoughts on how microbiome research may be focused in the next decade.
1) Introduction: Historical Perspective
Microbiome refers to the community of microbes that exist on and in a species as well their collective genes. The terms ‘microbiota’ and ‘microbiome’ are often used interchangeably but some argue that the microbiota are the organisms themselves and microbiome includes the organisms and their collective genomes. These microbes live on our skin, along our gastrointestinal tract, at other mucosal surfaces, and sometimes even inside our cells. The majority are bacteria, but microbiota also includes fungi, archaea and viruses ( 1 ). Human intestinal tracts have been estimated to contain up to 100 trillion (10^14) microbes which is about ten times the number of cells in the human body and it has been suggested that these microbes encode 100 times more genes than the human genome ( 1 – 3 ). For most of the earth’s 4.5-billion-year history, microbes were the only living organisms in existence. They have been around long before humans existed and as a result, we have evolved among them – and even from them. Therefore, though the term ‘microbiome’ is a relatively recent one, it is not surprising that the concept of the microbiome is centuries old.
The first microscopes were developed as early as 1675 when scientists discovered the existence of the microscopic creatures that we now call microbes. As these tools improved over the next 200 years, scientists like Pasteur and Koch taught us about the importance of these creatures as ‘germs’ or pathogens discovering the causes of leprosy, gonorrhea, typhoid, tuberculosis, cholera, diphtheria, tetanus and plague. Nevertheless, even Pasteur thought that bacteria were important to life ( 4 , 5 ). Around the turn of the 20 th century the idea of symbiosis was coined with newspapers at the time reporting on ‘good germs’ that could nourish soil as well as make alcohol and yogurt ( 4 ). However, most of the early 20 th century was spent trying to kill microbes with the discovery of antibiotics.
In the 1960s Carl Woese began to analyze 16S ribosomal RNA (16S rRNA) genes, which encode a component of the 30S small subunit of ribosomes in prokaryotes. These genes have both highly conserved as well as variable regions and therefore can be used to accurately determine microbial phylogeny. Woese profiled 16S rRNA genes from many different bacteria, changing the field of microbiology as we know it, and creating the field of molecular phylogenetics ( 4 , 6 , 7 ). This paved the way for the idea of metagenomics, or the genomics of communities, by direct extraction and cloning of DNA to capture the yet-uncultured microorganisms present among us and provide insight into their function within the community ( 8 ).
Characterizing the diversity and complexity of the microbes to which we play host is daunting and, at present, cataloging this diversity remains an incomplete task. However, recent technological innovations that we will discuss here have enabled us to begin to understand the role of microbiome in human health and disease. While gut microbiota are required for host immune education and colonic nutrition, microbial ‘dysbiosis’ or maladaptation, has been linked with complex genetic immune-mediated disorders such as inflammatory bowel disease (IBD) and spondyloarthritis (SpA). In this chapter, we will discuss the advancements in gut microbial profiling and its impact on understanding disease development.
2) Bacterial Bar Codes – 16S rRNA Sequencing
In 1977, Woese and Fox suggested that the 16S rRNA gene could be used as a marker in taxonomic identification ( 6 , 7 ). Profiling microbial communities in a culture-independent manner enabled the identification of gut microbiota that are highly refractory to culture-based assays. This method utilizes selective binding of universal primer pairs to highly conserved sequences within the hypervariable regions of the 16S rRNA gene (such as V3–V5), enabling amplification and sequencing of a region that contains sufficient phylogenetic information for taxonomic classification. This information is used to determine operational taxonomic units (OTUs) having 97% sequence identity with a bacterial taxa.
Sequence-based clustering is often guided by bacterial reference genomes; however, common methods include de novo clustering to identify previously unknown species ( 9 ). Importantly, natural genetic variations are not easily distinguished from sequencing errors, which affect about 0.1% of all sequenced nucleotides, despite the use of the mentioned highly accurate sequencing platforms ( 9 ). Therefore, it is important to consider and account for incorrect base calls in analyses. Statistical denoising methods such as Deblur or Dada2 are available and implemented in QIIME2, an open-source software for 16S rRNA sequence analysis ( 10 , 11 ).
The 16S rRNA gene sequencing approach is the most utilized method in the field of microbiome research to date. Costs are moderate, enabling large-scale microbiota analyses on relatively modest budgets ( 9 ). In addition to low costs, there are advantages to 16S rRNA gene sequencing. First, 16S genes are universally distributed with a high abundance exceeding other bacterial genes, thus facilitating sequencing and measuring relationships across taxa ( 12 ). Further, there is a low risk of horizontal 16S rRNA gene transfer. Disadvantages of using the 16S rRNA approach include the inability to detect variation among some strains and sub-strains; copy numbers per genome can vary in a taxon-specific fashion. There is also a risk of PCR amplification bias resulting in overinflated or inaccurate diversity estimates. Because 16S rRNA gene variation is sometimes not sufficient to differentiate closely related species, it provides limited information compared to whole bacterial genome sequencing or metagenomic sequencing ( 12 , 13 ).
When considering microbiome sequencing studies, it is important to note the spatial heterogeneity of community profiling along the intestinal tract ( 14 ). Using fecal samples to provide a community profile delivers a valuable picture of the diversity of the gut microbiota, however focusing on abundance measurements within the feces neglects the importance of mucus and tissue-associated organisms ( 14 ). Bacteria can access and adhere to the epithelium only if they have the ability to cross the mucus layer and bacterial adhesion influences the composition of the gut microbiota, particularly in the small intestine ( 14 ). Futhermore, sampling only the feces does not account for spatial distributions along the GI tract due to the variety of distinct microbial habitats ( 14 ). The cecum and colon contain the most diverse and dense microbial communities within the entire body ( 14 ). Whereas, the small intestine, which has more oxygen, lower pH and more antimicrobials than the colon, is host to fast-growing facultative anaerobes that can tolerate these conditions ( 14 ). Future work considering the microhabitats within the gut will have an essential impact on our understanding of microbial function as it relates to health and disease.
3) Bacterial Genome Sequencing – Metagenomics
Metagenomic sequencing, which refers to random sequencing of sheared DNA fragments that are then realigned, generally affords a more comprehensive taxonomic and functional analysis of the entire genomes of viral, bacterial, and eukaryotic microbiota ( 9 ). This method captures the entire genome of organisms instead of focusing on fragments of 16S rRNA genes ( 9 ). Therefore, shotgun metagenomic approaches provide information about phages, viruses, archaea, fungi and other eukaryotes, as well as plasmids and other extra-chromosomal elements in addition to host, chloroplast and mitochondrial DNA. As a result, this method requires much more sequencing reads to obtain the depth necessary to characterize rare microbiota. The data generated often reaches several terabases per study, which adds to the costs and increases bioinformatic demands on sequence assembly, mapping and analysis ( 9 , 15 ). However, due to the possibility of characterizing a microbiome with taxonomic resolution down to individual strains, this method is growing in popularity.
Since shotgun metagenomic sequencing provides complete genetic information about the microbes, it can reveal a functional profile of the microbe as well as specific taxonomic information. The microbes in our gut are regularly undergoing transcription and translation and interacting with each other as well as the human intestine. Therefore, sequencing of their genetic material can focus on phylogenetic markers (e.g., 16S rRNA) or expression of specific traits like enzyme activity ( 8 ). Using functional metagenomic techniques, the distribution and redundancy of functions in a community can be inferred and insights can be made into how microorganisms form symbioses, how they compete and communicate with other microbes, and how they use and produce energy ( 8 ).
Metagenomic data are analyzed by either comparing reads individually to reference databases, or after de novo assembly ( 8 , 15 , 16 ). One major disadvantage is that using a pre-existing database does not allow for characterization of previously unknown microbiota. And, de novo assembly requires substantial sequencing depth; therefore, this is restricted to only highly abundant species or strains within the microbial community ( 15 , 16 ). This is particularly challenging if the sample being analyzed does not have a large microbial load. Therefore, the major disadvantages of metagenomics include cost and the risk of missing previously uncharacterized species or strains of bacteria ( 9 ).
Interestingly, there are statistical methods which use the more affordable 16S rRNA sequencing data to predict metagenomics and provide functional information from taxonomic and phylogenetic data. These statistical tools (phylogenetic investigation of communities by reconstruction of unobserved states, or PICRUSt) offer predictive inferences as opposed to measured data but have been found to produce accurate data ( 17 ). PICRUSt uses an ancestral-state reconstruction algorithm in order to predict which gene families are present and then uses this predicted information to estimate the metagenome ( 17 ). This method has been recently used by Gill and colleagues in an HLA-B27 induced experimental spondyloarthritis model in rats linking specific microbes to dysregulated cytokine pathways ( 18 ).
4) Metabolomics
Microbiota produce and consume metabolites that serve as a crucial link between microbial function and host physiology, in both health and disease states. Metabolomics, as applied to microbiome research, aims to comprehensively identify and quantify the metabolic consequences of microbe-host interactions ( 19 ). Nuclear magnetic resonance (NMR) spectroscopy and mass spectrometry are the main technologies utilized in this field. Metabolic profiling can be targeted or non-targeted for discovery purposes. Targeted analysis of a disease state determines perturbation of metabolites associated with disease and will focus on a specific class of compounds (e.g., amino acids, fatty acids, lipids, carbohydrates, or bile acids). Non-targeted discovery approaches provide a greater overview of metabolic differences that may be a consequence of quantitative or functional differences between microbes in a community and can lead to discovery of unexpected biomarkers or therapeutic targets ( 19 ). Best practice recommendations for microbiome analysis, including laboratory and bioinformatic procedures are available, for example, from the U.S. Microbiome Quality Control project ( 20 ).
5) Host Response to the gut microbiome
A. barrier function & innate immune reactivity.
Our understanding of how the immune system relates and responds to both commensal and pathogenic bacteria in the gut in healthy individuals is incomplete but has grown significantly over the last two decades. The conventional view has been that the gut barrier prevents dissemination of nearly all bacteria from the intestine and this view has been supported by rare detection of live commensal bacteria in extra-intestinal organs in an immunocompetent host ( 21 ). However, we now know that the gut and the microbiota within it also play a critical role in the development of a healthy immune system ( 22 ). In fact, the host response to microbes is directed in part by the gut barrier. The gut epithelial barrier serves as the interface between the microbes within the gut lumen and the host. The degree of epithelial permeability is regulated by the integrity of the epithelial cell layer and the basement membrane, the surface mucus layer, the autonomic nervous system, and by the secretion of host factors, such as defensins. Toll-like Receptors (TLRs), in addition to their significant role in defense against microbial infection, also play a role in prevention of epithelial injury and permeability ( 23 ). TLRs encompass a family of pattern-recognition receptors that detect molecular products of microbes, or microbe-associated molecular patterns (MAMPs) including lipopolysaccharide (LPS) and lipoteichoic acid (LTA) as well as muramic acid, capsular polysaccharides, flagellin and unmethylated bacterial DNA ( 24 ). By recognition of these MAMPs, TLRs sense the presence of microbes in order to initiate an inflammatory response ( 23 ). Following TLR stimulation, a cascade of signals is initiated which then leads to the release of nuclear factor kappa-light-chain-enhancer of activated B cells (NF-κB) which then activates transcription of many host genes including chemokines, cytokines and acute phase reactants ( 24 ). However, the molecular products recognized by TLRs, like LPS and other MAMPS, are not unique to pathogens but rather are shared by entire classes of bacteria, including commensals. TLR activation by commensal MAMPS will in some cases signal inhibition of inflammatory reactions which is crucial to maintain intestinal epithelial homeostasis and symbiosis between the host and its commensals ( 23 – 25 ). Some bacteria increase gut permeability, like Escherichia coli , whereas others can decrease permeability, like lactobacilli ( 26 ) potentially due to the different MAMPs they express, like LPS. Furthermore, there exist LPS subtypes which exhibit various levels of endotoxicity or capacities to elicit an innate immune response ( 27 ). For example, LPS from E. coli is a more potent immune activator than LPS from Bacteroides species due to subtle structural differences ( 28 ).
There is some correlative evidence for TLR activation by MAMPs playing a role in the pathogenesis of autoimmune disease ( 28 ). A study in 2016 characterized the development of the infant gut microbiome in populations from Finland, Estonia and Russia – who have different rates of autoimmune disease – and identified significant differences between populations of intestinal microbes with different LPS subtypes ( 28 ). This group proposes that early microbial communities producing different LPS subtypes might contribute to immune modulation and alter the course of autoimmunity by their differing abilities to initiate tolerance ( 28 ). The importance of TLRs in both gut homeostasis and immune defense underscores both the delicate balance the immune system must achieve in maintaining gut health and the potential role of the microbiome in development of autoimmune disease.
Some systemic autoimmune diseases, like inflammatory bowel disease (IBD), are characterized by increased gut permeability which then allows for mucosal penetration of MAMPS and antigens and may trigger an aberrant immune response as a result. However, altered intestinal permeability has been documented in healthy relatives of patients with Crohn’s Disease (CD) ( 29 ), which may suggest that increased permeability is only one factor that predisposes an individual to disease. Overproduction of IgG directed against commensals occurs in patients with CD which could either reflect an increase in gut permeability or a state of hyperresponsiveness to normally harmless bacteria ( 30 ). Whether these antibodies are a result of nonspecific bacterial translocation through a damaged and epithelial layer or reflect a primary pathogenic process is currently not known ( 26 ) and is an important question that is currently being explored in other autoimmune diseases as well. These immunologic observations, among many others, highlight the potential relevance of the microbiome in immune-related disease.
b. Host response to bacterial metabolites
An important concept in relating the microbiome to host health is the role of bacteria in digestion, creation of nutrients, and the resulting byproducts of their metabolism that influence our health. Human co-evolution with symbiotic gut microbes has provided us with beneficial microbial metabolites, required for the degradation of indigestible components including plant-derived pectin, cellulose, and resistant starches ( 31 , 32 ). The anaerobic microbial communities of the mammalian intestine digest dietary fiber which is, in turn, used by the gut microbiota of the colon as their main source of energy ( 33 , 34 ). Fibrolytic bacteria degrade polysaccharides into smaller carbohydrates, which are then fermented into short-chain fatty acids (SCFAs) such as acetate, propionate, and butyrate. These SCFAs are carried in the bloodstream to a variety of different organs, where they are used as substrates for oxidation, lipid synthesis, and energy metabolism particularly by hepatocytes, which use propionate for gluconeogenesis ( 35 , 36 ). Additionally, butyrate can inhibit cell growth or promote cell differentiation, and it can induce apoptosis in tumor cells ( 34 ). And butyrate is a major source of energy for colonocytes and transported preferentially across the epithelium. However, all three SCFAs have demonstrated important immunomodulatory properties ( 33 ).
The main producers of butyrate belong to the classes Clostridia, Eubacteria and Roseburia ( 35 ). Butyrate has anti-inflammatory effects due to inhibition of NF-kappaB transcription factor activation which consequently reduces the formation of pro-inflammatory cytokines ( 34 ). Acetate, propionate, and butyrate inhibit TNFalpha-mediated activation of the NF-kappaB pathway and inhibit LPS-induced TNFalpha release from neutrophils ( 37 ). Furthermore, SCFAs can regulate neutrophil functions and migration, inhibit expression of vascular cell adhesions molecule-1 and increase expression of tight junction proteins in the colonic epithelia ( 35 ). Regulatory T cells (Tregs) expressing transcription factor Foxp3 have an important role in modulating inflammatory responses in the intestine and it has also been found that both butyrate and propionate facilitate extrathymic differentiation of Treg cells in mice ( 38 ). SCFAs help to regulate the size and function of the Treg pool within the colon and protect against colitis in mice ( 39 ). Accordingly, the notion that dysbiosis may be related to a disrupted ratio of fermenter versus non-fermenters (i.e., bacteria that do and don’t ferment polysaccharides to create SCFAs) as part of the pathogenesis of IBD has been proposed. Due to these anti-inflammatory properties, SCFAs have been explored as options for treatment in IBD and colon cancer ( 40 ). Recent rodent models of inflammatory disease, like experimental autoimmune encephalomyelitis and allergic airway disease, have revealed evidence that SCFA administration can modulate or inhibit disease by increasing Tregs ( 41 , 42 ). Ultimately, the immunomodulatory properties of these microbial and dietary metabolites might be exploited for the treatment of inflammatory disease in humans.
c. Adaptive Immune Reactivity
I. t cell responses.
The adaptive immune response is crucial in the maintenance of intestinal immune homeostasis. Germ-free mice fail to develop a normal immune system, but rather develop lymphoid architecture with abnormalities of multiple cell lineages including B cells, T cells (Th17, Treg, CD8+ T), and Th1/Th2 balance ( 43 ). The gastrointestinal tract microbiota have been shown to affect differentiation of T cells into various T helper cell types, like Th1, Th2, Th17 or Tregs ( 24 ). The Th17 population secretes cytokines, like IL-22 and IL-17A, which have an impact on immune homeostasis and gut epithelial inflammation ( 24 ). The purified capsular polysaccharide of the commensal Bacteroides fragilis suppresses production of IL-17 and stimulates T cell production of IL-10, thereby protecting colonic epithelium from inflammation due to bacterial antigens ( 24 ). The colon also promotes expansion of Tregs, mediators of immune tolerance, (in part due to SCFA production as previously discussed) which limits an inappropriately high inflammatory response ( 24 ).
CD4+ T cells in particular, discriminate between harmless microbes (e.g., commensals) versus toxic ones (e.g., pathogens) ( 44 , 45 ). CD4+ T cells establish a well-honed crosstalk with the gut microenvironment to maintain tolerance yet simultaneously and efficiently eradicate local and systemic infections ( 44 , 45 ). In order to generate tolerance to commensal antigens, CD4+ Tregs dampen inflammation through release of anti-inflammatory cytokines, like IL-10 and TGFβ ( 44 , 45 ). To this end, they orchestrate the immune response via release of either pro- or anti-inflammatory cytokines and expression of co-stimulatory molecules as well as driving or repressing a response of macrophages, CD8+ T cells or B cells ( 44 , 45 ). Importantly, the breakdown of these mechanisms may play a role in chronic inflammatory disease.
ii. Sequencing IgA-Targeted Microbes (IgA-Seq)
The gut microbiota also induce local secretory immunoglobulin A (sIgA) which contributes to gut homeostasis and protection from enteric pathogens ( 46 ). IgA is the most abundant immunoglobulin isotype produced in mammals, largely secreted across mucous membranes and by extension is a critical mediator of intestinal immunity ( 46 ). Secretory IgA production is induced by antigen capture within Peyer’s patches M cells, subsequent activation of T cells and then B cell class switch recombination within mesenteric lymph nodes and gut-associated lymphoid tissue (GALT). Various cytokines, including IL-10, stimulate sIgA production and are crucial in maintaining mucosal tolerance, providing a link between sIgA, immunity and intestinal homeostasis ( 24 ). A T cell-independent IgA response to commensal bacteria is also locally induced in the intestine under homeostatic conditions ( 47 , 48 ).
IgA is also an effective marker of potentially inflammatory microbiota, not just commensals. The intestinal immune system will recognize enteric pathogens and thereby produce high-affinity, T-cell-dependent, pathogen-specific IgA, which is transcytosed into the intestinal lumen. In the lumen, IgA can then bind and ‘‘coat’’ offending pathogens and provide protection against infection through neutralization and exclusion ( 49 ). Due to the overwhelming diversity of the mammalian microbiome and difficulty in associating specific microbes with disease, another experimental methodology was identified which has been termed “IgA-Seq”. The IgA-Seq method takes advantage of these differences in IgA binding between different microbes. Using flow cytometry, one can identify fluorescence intensity shifts of IgA-bound microbes compared to those not bound to IgA and thereby identify a specific taxon or group of taxa that is potentially related to dysbiosis. The microbes in the high-binding gate and low-binding gate are separated using flow cytometry sorting or magnetic-activated cell sorting (MACS) and the two different fractions are analyzed for taxonomic differences using either 16S rRNA sequencing or shotgun metagenomics. This approach has identified inflammatory taxa in various disease states in both mice and humans ( 49 ).
However, despite IgA being the predominant antibody isotype produced at mucosal surfaces it only interacts with a fraction of the intestinal microbiota ( 50 – 52 ). Furthermore, normal flora within the intestinal microbiota, or commensals, also can stimulate IgA production and can become coated with IgA ( 50 , 51 , 53 ). As compared to pathogen-induced IgA, commensal-induced IgA is thought to be of low affinity and specificity ( 50 , 51 ). Nevertheless, the commensal bacteria bound by IgA are poorly characterized and the type of humoral immunity they elicit remains elusive. One study, using bacterial flow cytometry coupled with 16S rRNA gene sequencing (IgA-Seq) in murine models of immunodeficiency, identified IgA-bound bacteria to elucidate mechanisms of commensal IgA targeting. They found that residence in the small intestine, rather than bacterial identity, dictated induction of specific IgA ( 52 ). They reported that commensals elicited strong humoral T-independent immune responses that originated from the B1b lineage in particular. Atypical commensals including segmented filamentous bacteria and Mucispirillum evaded T-independent responses but elicited T-dependent IgA. These data demonstrate targeting of distinct commensal bacteria by multiple layers of humoral immunity, and suggest IgA “coating” may be more suggestive of bacterial location along the gastrointestinal (GI) tract rather than indicate a role in pathogenesis of disease ( 52 ). Nevertheless, the use of IgA-Seq has helped isolate potential colitogenic bacteria in IBD. Noah Palm and colleagues aimed to characterize taxa-specific coating of gut microbes with IgA with the hypothesis that IgA coating can identify potentially inflammatory commensals that drive intestinal disease ( 49 ). Using this method, Palm and other groups have shown particular bacterial taxa as uniquely potent drivers of intestinal disease. However, it is important to note that associations between species, or even phyla, and dysbiosis in the setting of disease states are often unable to be replicated; this fact may suggest that the role of the microbiome in driving disease is complex and is likely related to multiple microbial factors and an ecological model of dysbiosis.
iii. Sequencing IgG-Targeted Microbes (IgG-Seq)
Immunoglobulin G (IgG) is not native to the gut and it is not clear whether human gut microbiota are capable of inducing a systemic IgG immune response under healthy homeostatic conditions. However, circulating IgG against commensals has been reported in healthy humans and at higher concentrations in those with Crohn’s disease and diabetes ( 21 , 54 – 56 ). This higher IgG response to symbiotic bacteria in patients with disease may be due to increased gut permeability leading to increased bacterial translocation. However, there is also evidence from mouse studies that under healthy homeostatic conditions (in the absence of a “leaky gut”) some symbiotic gram-negative bacteria (Salmonella spp. and E. Coli) are able to disseminate systemically and induce an IgG response. This IgG was found to directly coat the bacteria to promote killing by phagocytosis, and thus confer protection against systemic infection. This suggests a possible compensatory protective role for gut bacterial translocation ( 21 ). Recent work suggests that mice generate broadly reactive T-independent IgG to commensals with the help of TLRs ( 57 ). Using a similar approach to IgA-Seq to characterize the antibody response to the microbiota, this group used murine feces as the microbial source and stained the bacteria with paired sera (from the same mouse). This allows profiling of the complete antibody response rather than restricting analysis to antibodies present within the lumen that are bound to commensals. Using isotype-specific secondary antibodies, you can distinguish between IgA, IgM and IgG binding. These mouse models have shown that fecal samples with paired serum demonstrate IgG binding of the microbiota without IgG ever entering the gut, suggesting that the microbes or a portion of the microbes have translocated the gut barrier ( 57 , 58 ). However, whether this approach will be useful in humans is not yet known.
6) Important Concepts in Microbiome Research
A. richness, diversity & redundancy.
The human microbiome is both vast and diverse. The gut is dominated by four major bacterial phyla: Bacteroidetes, Firmicutes, Proteobacteria and Actinobacteria ( 33 ). However, an individual’s gastrointestinal tract likely contains on the order of 10 14 microorganisms with over 2,000 species and 12 different phyla represented ( 3 , 24 , 59 , 60 ). Any given fecal sample, for example, contains at least 1,000 species of bacteria; these species vary among individuals. In one of the largest studies of 16S rRNA sequence diversity in a gut community, investigators revealed more than 395 bacterial phylotypes in samples from 3 subjects ( 61 ). As described by Jeff Gordon and his group, the gut microbiota can be visualized as a grove of eight palm trees (or divisions) with deeply divergent lineages represented by the fans of closely related bacteria at the top of each trunk ( 31 ). This diversity is often measured two ways: alpha and beta diversity. Alpha diversity, when described by an OTU count, provides information about how many different species are present in one ecosystem, or species richness. The Shannon alpha diversity index also takes into account relative abundance, and therefore it measures diversity and evenness, or whether the microbes within the ecosystem are balanced or instead if there are species that dominate. Beta diversity provides information on how different one environment’s microbial community is as compared to another environment and generally focuses on taxonomic abundance between samples. The question of whether increased or decreased alpha diversity is associated with inflammatory disease has not yet been resolved. The data are mixed; differences may be due to divergent pathogenesis between diseases and/or alpha diversity may be too simplistic of a measure to characterize disease or wellness.
In order to characterize this profound richness and diversity, an integrated catalog of microbiota from various cohorts in Europe and the United States has been created (the Integrated Gene Catalog) which describes almost 10 million sequenced genes ( 1 ). While we do not yet have a global comprehensive microbial database, regional microbiome projects that are linked as a single global network, such as the International Human Microbiome Consortium, the European Commission’s Metagenomics of the Human Intestinal Tract project, the US National Institutes of Health’s Human Microbiome Project (HMP), and the Canadian Microbiome Initiative, are a step in this direction.
The HMP Consortium as well as other work has addressed the question of whether, despite the significant diversity among individual microbiomes, healthy individuals all share a core microbiome. Insights from these studies suggest that we do share many of the same microbes, but the relative quantities of species vary greatly among individuals ( 3 , 59 , 62 ). Qin et al demonstrated that 57 bacterial species were present in >90% and 75 species in > 50% of the individuals in their study ( 3 , 63 ). But, despite these interindividual similarities, the idea of a universal healthy gut microbiome or a core microbiome is likely too simplistic in light of the interindividual differences observed in many other studies ( 64 ). Therefore, it is essential to sample a broad population of healthy individuals over time to uncover features of the microbiome unique to geography, age and cultural traditions.
Additionally, despite the significant diversity between individual microbiomes, some data suggest that the functional profile, or the relative abundance of different metabolic pathways, is quite even ( 62 ). This provides evidence for a functional core microbiome (ecological model) and suggests that microbial function is redundant ( 62 , 63 ). However, despite these data, our knowledge of the genetic and functional diversity of the gut microbiota remains incomplete.
b. Inter-individual differences
I. the role of genetics.
It is clear that both host genetics and the gut microbiome can influence metabolic and immunologic phenotypes in animals and humans ( 65 ). However, the relative influence of host genetics on the host microbiome is not known ( 65 ). The gut microbiome may function as an environmental factor that interacts with host genetics to shape a phenotype, or the microbiome itself may be genetically determined and subsequently influence the host (or both). Gut microbiomes differ significantly across adults( 3 , 59 ) but family members have more similar microbiota than individuals who are not related ( 62 , 66 ). These familial similarities are often attributed to similar environments (e.g., cohabitation & diet). However, when these factors are controlled for, family members have more similar microbiota profiles than unrelated individuals; therefore, genetics does appear to play a role ( 62 ). Animal and twin studies provide an interesting way to examine this question. One study providing evidence for a role of genetics reported that the concordance rate for carriage of the methanogen Methanobrevibacter smithii is higher for monozygotic (MZ) twins than dizygotic (DZ) twins ( 67 ). A highly powered twin study comparing MZ and DZ twins found certain taxa that were likely “heritable” compared to other taxa that were more likely to be shaped by environment ( 65 ). They found that the most highly heritable taxon was Christensenellaceae , a member of the Firmicute phylum. Members of the Bacteroidetes phylum were not found to be heritable and likely more influenced by environment ( 65 ). Other studies have compared known genetic loci differences between humans (e.g., different NOD2 risk alleles) and shown differences in relative frequencies of bacterial taxa between these groups ( 68 – 70 ).
In a similar study design to Goodrich and colleagues ( 65 ), albeit less powered, other groups have reported, in contrast, that characterization of the fecal microbiota of adult female monozygotic and dizygotic twins using 16S rRNA sequencing has shown that an individual’s GI microbial community will vary in specific bacterial lineages present but the degree of variation between adult twin pairs is comparable between monozygotic and dizygotic twins. Therefore, they suggest that the overall heritability of the microbiome is low, emphasizing the importance of environment( 62 , 66 ).
ii. The importance of environment
There are many studies that underscore the importance of environment on the gut microbiota and several population-based studies have shown diet is a key determinant of microbiota variation among individuals ( 71 – 73 ). Studies of hunter-gatherer populations with seasonal variation in diet have shown changes in their gut microbial communities that parallel these cyclical dietary changes ( 71 ). For example, in seasons where more fiber is consumed, bacteria that specialize in complex carbohydrate digestion (Bacteroidetes) become more abundant ( 74 ). Mouse studies have shown that changing their diet to a high fat chow (a “Western” diet) will dramaticall alter the gut microbiome within hours ( 75 ).
As the prevalence of metabolic diseases, like diabetes and obesity, continue to rise and the complexity of these diseases are slowly unveiled, the role of the gut microbiota has been offered as an explanation for part of their pathophysiologic intricacy. Because the gut microbiota can affect energy balance, and as a result have an impact on body mass and metabolism, by extension, the GI microbiota can influence the efficiency of calorie harvest from the diet and how this energy is used and stored ( 75 ). In early seminal microbiome studies, Jeff Gordon and his group found that when germ-free mice are colonized with the microbial community of conventionally raised mice, they have a dramatic increase in body fat within two weeks despite decreased caloric intake ( 32 ). They also note that it is repeatedly found that obesity is associated with a significant decrease in the level of diversity of the microbiome as compared to non-obese individuals ( 75 ). Though their work highlights the influence the microbiota may have on development of obesity, it also demonstrates how diet dramatically affects the composition of one’s microbiome.
Both diet and geographical environment are two major determinants of the microbial structure of the gut and comparisons of rural indigenous populations versus industrialized populations shows significant differences in microbial community makeup ( 66 , 76 , 77 ). In a recent study from Vangay et al, the effects of immigration to the United States from Thailand on the gut microbiome was explored( 78 ). They collected stool from individuals both before and after immigration and found that moving from a non-Western country to the United States is associated with loss of gut microbiome diversity with replacement of bacterial strains for local ones( 78 ).
2. Cohabitation
In addition to diet, a major environmental factor that influences the microbiome is cohabitation. Song et al. compared microbiota of humans and dogs in 60 families and found by comparing human pairs, dog pairs and human-dog pairs, that co-habitation resulted in more similar microbiomes, particularly of the skin ( 79 ). They also found that the skin microbiome was similar between people of different ages (whereas this is not the case in the gut). Similarly, genetically unrelated couples living together are microbially more similar to one another than family members living apart ( 66 , 80 ). One study found in analysis of spouse and sibling pairs that spouses have more similar microbiota to one another than to their siblings even after accounting for dietary factors ( 80 ). The phenomenon of the “cage effect” in murine studies is a pronounced example of this, where mice housed in the same cage will share microbiota due to coprophagia ( 81 ).
iii. What’s more important, genetics or environment?
The evidence clearly shows there are many factors that shape our microbial composition, but it is not clear which factor or factors dominate. Many studies support a greater role for the environment than genetics. In a 2018 study looking at over 1,000 healthy individuals of distinct ancestries in a relatively common environment, it was concluded that over 20% of the variability between individual microbiomes is associated with diet, drugs and body size, and that genetic ancestry played a minor role in gut microbial composition ( 82 ).
1. HLA-B27 models demonstrate dual role of genetics and environment
The initial studies linking microbiota to a rheumatic disease phenotype came from HLA-B27, human β 2 -microglobulin (hβ 2 m) transgenic (HLA-B27 Tg) rats in the early 1990s ( 83 , 84 ). These animals develop joint, gut, and skin inflammation, and males develop epididymoorchitis, thus resembling a spondyloarthritis phenotype. However, in germ-free facilities neither gut nor joint inflammation is seen ( 84 ). Yet, re-colonization with normal gut flora, especially containing Bacteroides spp. , was permissive for the gastrointestinal phenotype, whereas E. coli was not ( 85 ). These studies established the importance of environmental triggers, particularly gut microbiota, acting in the context of genetic predisposition to cause spondyloarthritis-like disease.
Subsequent studies in related strains of HLA-B27 Tg rats demonstrated that this allele along with hβ 2 m can alter the composition of gut microbiota, raising the possibility that this mechanism might be involved in disease predisposition ( 86 ). These studies also revealed a greater abundance of Bacteroides vulgatus in HLA-B27 Tg rats compared to wild-type (WT) animals ( 85 , 86 ). Since this work was performed using low copy number HLA-B27 Tg rats that do not develop disease, this suggested that changes in gut microbiota did not require the presence of overt gut inflammation ( 86 ). They also suggest that changes in Bacteroides vulgatus (and other microbiota) are not sufficient to elicit the inflammatory phenotype. A recent study has shown that effects of HLA-B27 on gut microbiota in experimental SpA in rats are highly dependent on host genetics and/or environment. They showed that while HLA-B27-induced microbial dysbiosis varied between strains housed in different facilities, the dysregulated immune pathways and cytokines were background independent ( 87 ). This suggests there may be inter-individual differences in patterns of dysbiosis in humans that are nevertheless disease-related and important.
c. Other variables
I. early life microbial acquisition and delivery conditions.
Infants begin to acquire their microbiota at birth, with the mode of delivery impacting which microbes will colonize their gut and skin ( 88 ). The gastrointestinal microbiota of infants delivered by C-section is significantly less similar to their mothers than vaginally delivered infants ( 64 , 88 ). In contrast to vaginally delivered infants, the fecal microbiome of infants delivered via C-section, who lack vaginal exposure, was enriched in bacteria commonly found on the skin like Staphylococcus aureus and other Staphylococcus species as well as species of Streptococcus , among others, suggesting that these newborns are first colonized by microbes in the surrounding environment during delivery. Vaginally born infants are enriched in genera including Bacteroides , Bifidobacterium and Escherichia which are seen in the vaginal and perineal milieu of their mothers. These differences gradually decrease over the first year of life ( 64 ) but may have long-lasting consequences on susceptibility to certain pathogens, like methicillin-resistant staphylococcus aureus (MRSA), or on the risk of obesity ( 88 ). Recent findings also suggest that microbial colonization of the gut may even start before birth ( 24 , 89 , 90 ). The placenta demonstrates a unique microbiome profile with Firmicutes , Proteobacteria , Tenericutes , Bacteroidetes and Fusobacteria ( 90 ). Additionally, meconium of full-term infants has been determined to play host to multiple genera often found in the amniotic fluid, vagina, and oral cavity ( 89 ).
i. Longitudinal variation & development
Human colonizing microbiota are established early in life but can shift with changes in age, diet, geographical location, intake of food supplements and drugs, and likely other causes as well ( 64 ). Bacterial communities are structured primarily by their habitat, meaning the oral, gut, skin and vaginal regions have a distinct community ( 88 ). Longitudinal studies of the human gut microbiota have suggested that the adult human bacterial community is stable over time in the absence of perturbations (e.g., antibiotics). In a longitudinal study of five years, it was shown that the microbial community of an adult individual was largely stable and persistent ( 91 , 92 ). However, the gut microbiota does undergo significant changes as humans move from infancy to old age.
In the TEDDY study in 2018, stool samples from 903 children were collected longitudinally over a few years revealing rapid changes in three phases: the “developmental” phase from ages 3–14 months, the “transitional” phase from ages 15–30 months, and the “stable” phase from ages 31–46 months ( 93 ). Breastmilk was the most significant factor influencing microbial structure, with breastfeeding associated with higher levels of Bifidobacterium species ( 93 ). The cessation of breastmilk was associated with more rapid maturation of the gut microbiome as marked by presence of Firmicutes ( 93 ). They also found the birth mode significantly influenced the microbiome of the developmental phase.
Given hormonal differences between sexes as well as differences in body fat percentages, sex has been considered as another possible factor influencing the microbiome. One group used a mouse model of type 1 diabetes (NOD mouse) to examine the relationship of microbial exposures and sex hormones to development of autoimmune disease ( 94 ). They found that transfer of adult male gut microbiota to immature females not only altered the microbiota of the host, but resulted in elevated testosterone levels, reduced pancreatic islet inflammation, reduced autoantibody production and protection from development of type 1 diabetes ( 94 ). There is not much known in humans about the sex-microbiome relationship but recent data has suggested that gut microbial diversity may be inversely associated with the android fat ratio (central adiposity) ( 95 ). This group suggested that certain taxa may be associated with fat distribution in men and women, helping to link obesity to the gut microbiome ( 95 ).
d. Dysbiosis
Dysbiosis is a condition where the normal microbiome population structure is disturbed, generally due to extrinsic factors like medications, diet, and disease ( 96 ), or even genetic variants, and is in contrast to eubiosis. The composition and functional characteristics of a “healthy” microbiome, or eubiosis, have yet to be defined, but in theory could be described in many ways ( 63 ). For example, a “healthy” microbiome may be defined by stability, the ability to resist community structure change under stress, or how quickly the community returns to baseline after a stressor. It could be defined by an idealized, health-associated, composition. And additionally, it may be defined by a desirable functional profile ( 63 ). Dysbiosis is then the loss of these characteristics due to a stressor, like acute or chronic illness, or perhaps loss or gain-of-function of critical genes.
In individuals with dysbiosis, the host may become more susceptible to infection from both exogenous and endogenous sources, or immunotolerance may be affected leading to development of autoimmunity, allergy, and/or chronic inflammation ( 24 ). Dysbiosis can therefore be a cause or an effect of disease. In dysbiosis, immune responsiveness could be up- or down-regulated to promote the return to eubiosis. This could be achieved through specific effects of sIgA, less specific effects of innate immune effectors (such as defensins) or local environment changes (i.e., diarrhea) ( 24 ).
i. Ecological & single organism models
The observation that the gut microbiota play a role in conditions like IBD, specifically Crohn’s disease and ulcerative colitis, was proposed as early as the 19 th and early 20 th century with descriptions of multiple potential infectious agents as triggers ( 97 , 98 ). Early evidence for this hypothesis includes the use of oral antibiotics to treat IBD, epidemiologic studies that demonstrate IBD clustering similar to the way some infectious diseases cluster, mouse models of colitis requiring the presence of bacteria, and diversion of the fecal stream from inflamed bowel associated with improvement in IBD ( 26 , 99 ). Additionally, in contrast to healthy subjects, colonic biopsy samples from subjects with Crohn’s disease reveal a higher concentration and thicker mucosal layer of bacteria that associate with intestinal surface, suggestive of a dysbiosis ( 26 ). Despite significant evidence supporting the role of the microbiome in IBD, identifying bacteria that “cause” dysbiosis has been a challenge. While many microbes have been postulated to play a role, there is no definitive evidence or consensus that microorganisms are the cause ( 26 ).
Altered gut microbial composition, or dysbiosis, is also implicated in the pathogenesis of several chronic immune-mediated rheumatic diseases, from ankylosing spondylitis (AS) and other forms of spondyloarthritis (SpA) including psoriatic arthritis (PsA), to rheumatoid arthritis (RA) and systemic lupus erythematosus (SLE) ( 100 – 102 ). Similar to IBD, these studies have found dysbiosis characterized by perturbations in many species of bacteria rather than single or small groups of organisms. These studies are reviewed in greater depth in other sections of this issue, and will not be detailed here. However, it is interesting to note that dysbiosis in HLA-B27 transgenic rats that serve as an experimental system for studying SpA, conforms to an ecological model ( 87 ). In other words, the effect of HLA-B27 is very much dependent on the strain of animal used and the housing environment. The effect of HLA-B27 expression in different rodent strains is to cause substantial shifts in the composition of gut microbiota associated with gut inflammation. The changes in gut microbiota caused by HLA-B27 on three different genetic backgrounds (DA, Lewis and Fischer) are almost distinct – there is very little overlap – despite the immunological and inflammatory changes being almost entirely overlapping and dominated by activation of inflammatory pathways involving IL-23/IL-17, IFNγ, IL-1, and TNF). This study would suggest that different HLA-B27 positive individuals in the human population with different communities of gut microbiota are likely to exhibit different patterns of dysbiosis. By focusing on microbiota that are associated with HLA-B27 and disease across a large population may ignore important individual differences. These results implicate a model of dysbiosis involving multiple microbes contributing to the aberrant immune response, rather than a single or small number of microbes driving pathogenesis ( 18 , 87 ).
While changes in communities of microbes are of importance, there is also evidence that a particular genus or species may be important. For example, Dialister prevalence in ileal biopsies correlates with disease activity in spondyloarthritis ( 103 ) in one study, while R. gnavus is over-represented in fecal samples from patients with spondyloarthritis, particularly in a sub-group with coexisting IBD ( 104 ). These and many other studies are laying the groundwork for evidence of the influence of the microbiota on disease by providing associations of microbial communities or species with disease states. However, association does not provide causation.
ii. Association studies evolve to mechanistic studies
Over the past few decades, clinical association studies are moving closer to the root of pathogenesis as the experimental techniques change. Methods previously discussed, like sequencing of IgG targeted microbes and metagenomics, used together, get us closer to pathogenesis than simply categorizing community structures and making associations between groups. However, even these methods still provide correlations. We are now in need of future investigations that will provide a deeper and more mechanistic understanding of how microbial dysbiosis influences disease pathogenesis.
In the past few decades we have made leaps in our knowledge about the human interaction with and dependence upon the microbes that live within us. Though some of the current data appear contradictory, with various different microbes, from species to entire phyla, associated with pathology from study to study, as we develop our understanding of the functional microbiome, we are learning that these differences may not be contradictory at all. In fact, the ecological model of dysbiosis implicating multiple microbes at once may explain these differences. Multiple different microbes may not only contribute to an aberrant immune response but may also provide similar functions to the host and when these functions are disrupted in some way may precipitate disease. The ecological model of dysbiosis requires further study as it is currently simply a hypothesis, but we are now entering an era of microbiome research where we have more and more tools at our disposal. As we move toward examining which microbes activate the immune system using IgA- and IgG-coated microbe sequencing and toward a more functional exploration of the disrupted microbes associated with disease, we will be more able to fully understand how the microbiome impacts disease.
Practice Points:
- 16S rRNA gene sequencing is the most cost-effective sequencing approach and currently the most commonly used methodology to explore the microbiome; certain statistical analyses of 16S rRNA data (PICRUSt) can be used to obtain predictive inferences about metagenomics in addition to phylogenetic data
- Metagenomic sequencing is a more comprehensive and informative method of microbiome analysis with increased capture of species and strains as well as providing a functional profile of the microbes’ potential activities
- The gut epithelial barrier is highly regulated by various components of the immune system in order to maintain gut homeostasis and symbiosis between the host and its commensals while also recognizing pathogens
- The gut is a major site for development of immune tolerance, but it is not yet clear how much of a role the microbiome plays in breaking tolerance during the development and propagation of autoimmune disease
- Our growing understanding of how the immune system interacts with microbes in the gut has allowed for newer methodologies for microbiome analysis, like sequencing IgA- or IgG-targeted microbes, which may provide more functional information than 16S rRNA gene and even metagenome sequencing
- The human microbiome of the GI tract is rich, diverse and also redundant, and is affected by innate factors like genetics and sex but even more so by our environment, including diet, geographical location, antibiotic use, birth method and other factors
- The redundancy of the microbiome in addition to the different types of dysbiosis associated with disease in different studies suggests that dysbiosis is likely not related to perturbation of a small number of microbes but instead implicates an ecological model where multiple microbes with important functions contribute to an aberrant immune response
Research Agenda:
- The promising newer methodologies of microbiome analysis which implicate function over taxonomy must be further explored in human studies with an emphasis on pathogenesis and establishing cause and effect relationships rather than association studies
- We must work to develop multidimensional, interdisciplinary approaches to scrutinize the complexities of the relationship of the host to its microbiota
- Larger, better powered and controlled studies with improved control group matching are needed. Increased sampling along the GI tract due to the spatial heterogeneity of the gut microbiome is important
- We must work to continue to grow our current understanding of bacterial metabolites, like SCFAs, with the ultimate goal of using these metabolites to understand their role in development of or protection from disease and then further manipulate them to use as treatments for disease
Acknowledgments
Funding Support: ECG and RAC were supported by the NIAMS Intramural Research Program (Z01 AR041184)
Publisher's Disclaimer: This is a PDF file of an unedited manuscript that has been accepted for publication. As a service to our customers we are providing this early version of the manuscript. The manuscript will undergo copyediting, typesetting, and review of the resulting proof before it is published in its final form. Please note that during the production process errors may be discovered which could affect the content, and all legal disclaimers that apply to the journal pertain.
Conflicts of Interest: The authors have no conflicts of interest to disclose.
Contributor Information
Emily C. Gotschlich, NIH/NIAMS, Building 4 Room 228, 4 Memorial Drive, Bethesda, MD 20892.
Robert A. Colbert, Pediatric Translational Research Branch, 10 Center Drive, Bldg. 10, Rm 12N240E, Bethesda, MD 20892.
Tejpal Gill, Oregon Health and Science University, 3215 SW Pavilion Loop, Lamfrom Biomedical Research Building 253E, Portland, OR 97239.
References:
Researching L2 Motivation: Past, Present and Future
- First Online: 12 January 2020
Cite this chapter
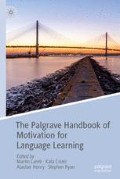
- Ema Ushioda 5
5552 Accesses
7 Citations
This concluding chapter examines the ‘how’ of language learning motivation research–that is, the methodological approaches, research designs, and tools of inquiry used to investigate motivation for language learning. The purpose of the chapter is to survey the diverse range of approaches to researching L2 (second language) motivation, with reference to particular types of study and particular research traditions. The chapter does not provide a practical “how to” guide for specific methods of inquiry, but aims instead to offer a critical analysis of strengths, limitations and challenges associated with different approaches to investigating language learning motivation. As reflected in its title, the chapter takes a broadly historical perspective and reviews how research designs have evolved and diversified over the decades in line with theoretical developments, broader research trends, and advances in technology. It discusses recent methodological innovations in the field, identifies current research challenges and issues, and outlines considerations for researching language learning motivation in the future.
This is a preview of subscription content, log in via an institution to check access.
Access this chapter
- Available as PDF
- Read on any device
- Instant download
- Own it forever
- Available as EPUB and PDF
- Compact, lightweight edition
- Dispatched in 3 to 5 business days
- Free shipping worldwide - see info
- Durable hardcover edition
Tax calculation will be finalised at checkout
Purchases are for personal use only
Institutional subscriptions
Al-Hoorie, A. H. (2016a). Unconscious motivation. Part I: Implicit attitudes towards L2 speakers. Studies in Second Language Learning and Teaching, 6 (3), 423–454.
Article Google Scholar
Al-Hoorie, A. H. (2016b). Unconscious motivation. Part II: Implicit attitudes and L2 achievement. Studies in Second Language Learning and Teaching, 6 (4), 619–649.
Banegas, D. (2013). The integration of content and language as a driving force in the EFL lesson. In E. Ushioda (Ed.), International perspectives on motivation: Language learning and professional challenges (pp. 82–97). Basingstoke, UK: Palgrave Macmillan.
Chapter Google Scholar
Barkhuizen, G. (Ed.). (2013). Narrative research in applied linguistics . Cambridge, UK: Cambridge University Press.
Google Scholar
Boo, Z., Dörnyei, Z., & Ryan, S. (2015). L2 motivation research 2005–2014: Understanding a publication surge and a changing landscape. System, 55 , 145–157.
Brewer, J., & Hunter, A. (2006). Foundations of multimethod research: Synthesizing styles . Thousand Oaks, CA: Sage.
Book Google Scholar
Bryman, Z. (2016). Social research methods (5th ed.). Oxford, UK: Oxford University Press.
de Bot, K., & Larsen-Freeman, D. (2011). Researching second language development from a dynamic systems theory perspective. In M. H. Verspoor, K. de Bot, & W. Lowie (Eds.), A dynamic approach to second language development: Methods and techniques (pp. 5–23). Amsterdam, The Netherlands: John Benjamins.
Chan, L., Dörnyei, Z., & Henry, A. (2015). Learner archetypes and signature dynamics in the language classroom: A retrodictive qualitative modelling approach to studying L2 motivation. In Z. Dörnyei, P. D. MacIntyre, & A. Henry (Eds.), Motivational dynamics in language learning (pp. 238–259). Bristol, UK: Multilingual Matters.
Chen, J. F., Warden, C. A., & Chang, H. T. (2005). Motivators that do not motivate: The case of Chinese EFL learners and the influence of culture on motivation. TESOL Quarterly, 39 (4), 609–633.
Chik, A., & Breidbach, S. (2011). Identity, motivation and autonomy: A tale of two cities. In G. Murray, X. Gao, & T. Lamb (Eds.), Identity, motivation and autonomy in language learning (pp. 145–159). Bristol, UK: Multilingual Matters.
Creswell, J. W., & Plano-Clark, V. L. (2011). Designing and conducting mixed methods research (2nd ed.). Thousand Oaks, CA: Sage.
Coffey, S. J. (2016). Choosing to study modern foreign languages: Discourses of value as forms of cultural capital. Applied Linguistics . https://doi.org/10.1093/applin/amw019
Denscombe, M. (2014). The good research guide: For small-scale social research projects (5th ed.). Maidenhead, UK: Open University Press.
Dörnyei, Z. (2001). New themes and approaches in second language motivation research. Annual Review of Applied Linguistics, 21 , 43–59.
Dörnyei, Z. (2007). Research methods in applied linguistics . Oxford, UK: Oxford University Press.
Dörnyei, Z. (2009). Individual differences: Interplay of learner characteristics and learning environment. In N. C. Ellis & D. Larsen-Freeman (Eds.), Language as a complex adaptive system (pp. 237–255). Oxford, UK: Wiley-Blackwell.
Dörnyei, Z. (2014). Researching complex dynamic systems: ‘Retrodictive qualitative modelling’ in the language classroom. Language Teaching, 47 (1), 80–91.
Dörnyei, Z., Csizér, K., & Németh, N. (2006). Motivation, language attitudes and globalisation: A Hungarian perspective . Clevedon, UK: Multilingual Matters.
Dörnyei, Z., MacIntyre, P. D., & Henry, A. (2015a). Introduction: Complex dynamic systems principles to empirical research on L2 motivation. In Z. Dörnyei, P. D. MacIntyre, & A. Henry (Eds.), Motivational dynamics in language learning (pp. 1–7). Bristol, UK: Multilingual Matters.
Dörnyei, Z., MacIntyre, P. D., & Henry, A. (Eds.). (2015b). Motivational dynamics in language learning. Bristol . UK: Multilingual Matters.
Dörnyei, Z., & Ushioda, E. (2011). Teaching and researching motivation (2nd ed.). Harlow, UK: Longman.
Gao, X. (2013). Motivated by visions: Stories from Chinese contexts. In E. Ushioda (Ed.), International perspectives on motivation: Language learning and professional challenges (pp. 176–191). Basingstoke, UK: Palgrave Macmillan.
Gardner, R. C. (1985). Social psychology and second language learning: The role of attitudes and motivation . London, UK: Edward Arnold.
Gardner, R. C., Masgoret, A.-M., Tennant, J., & Mihic, L. (2004). Integrative motivation: Changes during a year-long intermediate-level language course. Language Learning, 54 (1), 1–34.
Gu, M. (2009). The discursive construction of second language learners’ motivation: A multi-level perspective . Bern, Switzerland: Peter Lang.
Gu, M., & Qu, X. (2017). The discursive construction of university language learners in China. In M. T. Apple, D. Da Silva, & T. Fellner (Eds.), L2 selves and motivations in Asian contexts (pp. 134–150). Bristol, UK: Multilingual Matters.
Guillemin, M., & Gillam, L. (2004). Ethics, reflexivity, and ‘ethically important moments’ in research. Qualitative Inquiry, 10 , 261–280.
Harvey, L. (2017). Language learning motivation as ideological becoming. System, 65 , 69–77.
Henry, A. (2015). The dynamics of L3 motivation: A longitudinal interview/observation-based study. In Z. Dörnyei, P. D. MacIntyre, & A. Henry (Eds.), Motivational dynamics in language learning (pp. 315–342). Bristol, UK: Multilingual Matters.
Henry, A., & Thorsen, C. (2018). Teacher-student relationships and L2 motivation. Modern Language Journal, 102 (1), 218–241.
Hiver, P., & Al-Hoorie, A. H. (2016). A dynamic ensemble for second language research: Putting complexity theory into practice. Modern Language Journal, 100 (4), 741–756.
Holliday, A. (1999). Small cultures. Applied Linguistics, 20 (2), 237–264.
Irie, K. (2014). Q methodology for post-social-turn research in SLA. Studies in Second Language Learning and Teaching, 4 (1), 13–32.
Irie, K., & Ryan, S. (2015). Study abroad and the dynamics of change in learner L2 self-concept. In Z. Dörnyei, P. D. MacIntyre, & A. Henry (Eds.), Motivational dynamics in language learning (pp. 343–366). Bristol, UK: Multilingual Matters.
Kim, T.-Y. (2009). The sociocultural interface between ideal self and ought-to self: A case study of two Korean students’ ESL motivation. In Z. Dörnyei & E. Ushioda (Eds.), Motivation, language identity and the L2 self (pp. 274–294). Bristol, UK: Multilingual Matters.
Kormos, J., & Csizér, K. (2014). The interaction of motivation, self-regulatory strategies and autonomous learner behaviour in different learner groups. TESOL Quarterly, 48 (2), 275–299.
Lamb, M. (2004). Integrative motivation in a globalizing world. System, 32 (1), 3–19.
Lamb, M. (2009). Situating the L2 self: Two Indonesian school learners of English. In Z. Dörnyei & E. Ushioda (Eds.), Motivation, language identity and the L2 self (pp. 229–247). Bristol, UK: Multilingual Matters.
Lamb, M. (2016). When motivation research motivates: Issues in long-term empirical investigations. Innovation in Language Learning and Teaching . https://doi.org/10.1080/17501229.2016.1251438
Larsen-Freeman, D. (2016). Classroom-oriented research from a complex systems perspective. Studies in Second Language Learning and Teaching, 6 (3), 377–393.
Larsen-Freeman, D., & Cameron, L. (2008). Complex systems and applied linguistics . Oxford, UK: Oxford University Press.
MacIntyre, P. D. (2012). The idiodynamic method: A closer look at the dynamics of communication traits. Communication Research Reports, 29 (4), 361–367.
MacIntyre, P. D., Baker, S., & Sparling, H. (2017). Heritage passions, heritage convictions and the rooted L2 self: Music and Gaelic language learning in cape Breton, Nova Scotia. Modern Language Journal, 101 (3), 501–516.
MacIntyre, P. D., & Legatto, L. L. (2011). A dynamic system approach to willingness to communicate: Developing an idiodynamic method to capture rapidly changing affect. Applied Linguistics, 32 (2), 149–171.
MacIntyre, P. D., Mackinnon, S., & Clément, R. (2009). Towards the development of a scale to assess possible selves as a source of language learning motivation. In Z. Dörnyei & E. Ushioda (Eds.), Motivation, language identity and the L2 self (pp. 193–214). Bristol, UK: Multilingual Matters.
Mackey, A., & Gass, S. M. (Eds.). (2012). Research methods in second language acquisition: A practical guide . Chichester, UK: Wiley-Blackwell.
Magid, M. (2014). Applications and implications of the L2 motivational self-system. In K. Csizér & M. Magid (Eds.), The impact of self-concept on language learning (pp. 333–356). Bristol, UK: Multilingual Matters.
Magid, M., & Chan, L. (2012). Motivating English learners by helping them visualise their ideal L2 self: Lessons from two motivational programmes. Innovation in Language Learning and Teaching, 6 (2), 113–125.
Marsden, E., & Kasprowicz, R. (2017). Foreign language educators’ exposure to research: Reported experiences, exposure via citations, and a proposal for action. Modern Language Journal, 101 (4), 613–642.
Masgoret, A.-M., & Gardner, R. C. (2003). Attitudes, motivation, and second language learning: A meta-analysis of studies conducted by Gardner and his associates. Language Learning, 53 (1), 123–163.
Mercer, N. (2004). Sociocultural discourse analysis: Analysing classroom talk as a social mode of thinking. Journal of Applied Linguistics, 1 (2), 137–168.
Mercer, S. (2015). Dynamics of the self: A multilevel nested systems approach. In Z. Dörnyei, P. D. MacIntyre, & A. Henry (Eds.), Motivational dynamics in language learning (pp. 139–163). Bristol, UK: Multilingual Matters.
Miyahara, M. (2014). Emerging self-identities of second language learners: Emotions and the experiential profile of identity construction. In K. Csizér & M. Magid (Eds.), The impact of self-concept on language learning (pp. 206–231). Bristol, UK: Multilingual Matters.
Murphy, L. (2011). ‘Why am I doing this?’ Maintaining motivation in distance language learning. In G. Murray, X. Gao, & T. Lamb (Eds.), Identity, motivation and autonomy in language learning (pp. 107–124). Bristol, UK: Multilingual Matters.
Nikolov, M. (2001). A study of unsuccessful language learners. In Z. Dörnyei & R. Schmidt (Eds.), Motivation and second language acquisition (pp. 149–169). Honolulu, HI: University of Hawaii Press.
Norton, B. (2000). Identity and language learning: Gender, ethnicity and educational change . Harlow, UK: Longman.
Ortega, L. (2005). For what and for whom is our research? The ethical as transformative lens in instructed SLA. Modern Language Journal, 89 (3), 427–443.
Paltridge, B., & Phakiti, A. (Eds.). (2015). Research methods in applied linguistics: A practical resource . London, UK: Bloomsbury Academic.
Papi, M., & Teimouri, Y. (2014). Language learner motivational types: A cluster analysis study. Language Learning, 64 (3), 493–525.
Pavlenko, A. (2007). Autobiographic narratives as data in applied linguistics. Applied Linguistics, 28 (2), 163–188.
Pemberton, R., & Cooker, L. (2012). Self-directed learning: Concepts, practice, and a novel research methodology. In S. Mercer, S. Ryan, & M. Williams (Eds.), Psychology for language learning: Insights from research, theory and practice (pp. 304–327). Basingstoke, UK: Palgrave Macmillan.
Piniel, K., & Csizér, K. (2015). Changes in motivation, anxiety and self-efficacy during the course of an academic writing seminar. In Z. Dörnyei, P. D. MacIntyre, & A. Henry (Eds.), Motivational dynamics in language learning (pp. 164–194). Bristol, UK: Multilingual Matters.
Pinter, A. (2013). Child participant roles in applied linguistics research. Applied Linguistics, 35 (2), 168–183.
Preston, A. E. (2009). The contribution of interaction to learner motivation in the MFL classroom (unpublished doctoral dissertation). University of Southampton, UK.
Punch, K. F. (2014). Introduction to social research: Quantitative and qualitative approaches (3rd ed.). Thousand Oaks, CA: Sage.
Ryan, S. (2009a). Self and identity in L2 motivation in Japan: The ideal L2 self and Japanese learners of English. In Z. Dörnyei & E. Ushioda (Eds.), Motivation, language identity and the L2 self (pp. 120–143). Bristol, UK: Multilingual Matters.
Ryan, S. (2009b). Ambivalence and commitment, liberation and challenge: Investigating the attitudes of young Japanese people towards the learning of English. Journal of Multilingual and Multicultural Development, 30 (5), 405–420.
Sampson, R. (2016). Complexity in classroom foreign language learning motivation: A practitioner perspective from Japan . Bristol, UK: Multilingual Matters.
Sasaki, M., Kozaki, Y., & Ross, S. J. (2017). The impact of normative environments on learner motivation and L2 reading ability growth. Modern Language Journal, 101 (1), 163–178.
Seedhouse, P. (2004). The interactional architecture of the language classroom: A conversation analysis perspective . Oxford, UK: Blackwell.
Sugita McEown, M., Sawaki, Y., & Harada, T. (2017). Foreign language learning motivation in the Japanese context: Social and political influences on self. Modern Language Journal, 101 (3), 533–547.
Taguchi, T., Magid, M., & Ryan, S. (2009). The L2 motivational self system among Japanese, Chinese and Iranian learners of English: A comparative study. In Z. Dörnyei & E. Ushioda (Eds.), Motivation, language identity and the L2 self (pp. 66–97). Bristol, UK: Multilingual Matters.
Teddlie, C., & Tashakkori, A. (2009). Foundations of mixed methods research: Integrating quantitative and qualitative approaches in the social and behavioral sciences . Thousand Oaks, CA: Sage.
Thompson, A. S., & Erdil-Moody, Z. (2016). Operationalizing multilingualism: Language learning motivation in Turkey. International Journal of Bilingual Education and Bilingualism, 19 (3), 314–331.
Ueki, M., & Takeuchi, O. (2017). The impact of studying abroad experience on the affective changes related to L2 motivation: A qualitative study of the processes of change. In M. T. Apple, D. Da Silva, & T. Fellner (Eds.), L2 selves and motivations in Asian contexts (pp. 119–133)). Bristol, UK: Multilingual Matters.
Ushioda, E. (1994). L2 motivation as a qualitative construct. Teanga, 14 , 76–84.
Ushioda, E. (2001). Language learning at university: Exploring the role of motivational thinking. In Z. Dörnyei & R. Schmidt (Eds.), Motivation and second language acquisition (pp. 93–125). Honolulu, HI: University of Hawaii Press.
Ushioda, E. (2009). A person-in-context relational view of emergent motivation, self and identity. In Z. Dörnyei & E. Ushioda (Eds.), Motivation, language identity and the L2 self (pp. 215–228). Bristol, UK: Multilingual Matters.
Ushioda, E. (2015). Context and complex dynamic systems theory. In Z. Dörnyei, P. D. MacIntyre, & A. Henry (Eds.), Motivational dynamics in language learning (pp. 47–54). Bristol, UK: Multilingual Matters.
Ushioda, E. (2016). Language learning motivation through a small lens: A research agenda. Language Teaching, 49 (4), 564–577.
Ushioda, E., & Chen, S.-A. (2011). Researching motivation and possible selves among learners of English: The need to integrate qualitative inquiry. Anglistik: International Journal of English Studies, 22 (1), 43–61.
Vauras, M., Kinnunen, R., Kajamies, A., & Lehtinen, E. (2013). Interpersonal regulation in instructional interaction: A dynamic systems analysis of scaffolding. In S. Volet & M. Vauras (Eds.), Interpersonal regulation of learning and motivation: Methodological advances (pp. 125–146). Abingdon, UK: Routledge.
Watts, D. S., & Stenner, D. P. (2012). Doing Q methodological research: Theory, method and interpretation . Thousand Oaks, CA: Sage.
Wengraf, T. (2001). Qualitative research interviewing: Biographic narratives and semi-structured methods . London, UK: Sage.
You, C. J., & Chan, L. (2015). The dynamics of L2 imagery in future motivational self-guides. In Z. Dörnyei, P. D. MacIntyre, & A. Henry (Eds.), Motivational dynamics in language learning (pp. 397–418). Bristol, UK: Multilingual Matters.
You, C. J., & Dörnyei, Z. (2016). Language learning motivation in China: Results of a large-scale stratified survey. Applied Linguistics, 37 (4), 495–519.
Download references
Author information
Authors and affiliations.
University of Warwick, Coventry, UK
Ema Ushioda
You can also search for this author in PubMed Google Scholar
Corresponding author
Correspondence to Ema Ushioda .
Editor information
Editors and affiliations.
School of Education, University of Leeds, Leeds, UK
Martin Lamb
Faculty of Humanities, Eötvös Loránd University, Budapest, Hungary
Kata Csizér
Department of Social and Behavioural Studies, University West, Trollhättan, Sweden
Alastair Henry
School of Culture, Media and Society, Waseda University, Tokyo, Japan
Stephen Ryan

Rights and permissions
Reprints and permissions
Copyright information
© 2019 The Author(s)
About this chapter
Ushioda, E. (2019). Researching L2 Motivation: Past, Present and Future. In: Lamb, M., Csizér, K., Henry, A., Ryan, S. (eds) The Palgrave Handbook of Motivation for Language Learning. Palgrave Macmillan, Cham. https://doi.org/10.1007/978-3-030-28380-3_32
Download citation
DOI : https://doi.org/10.1007/978-3-030-28380-3_32
Published : 12 January 2020
Publisher Name : Palgrave Macmillan, Cham
Print ISBN : 978-3-030-28379-7
Online ISBN : 978-3-030-28380-3
eBook Packages : Education Education (R0)
Share this chapter
Anyone you share the following link with will be able to read this content:
Sorry, a shareable link is not currently available for this article.
Provided by the Springer Nature SharedIt content-sharing initiative
- Publish with us
Policies and ethics
- Find a journal
- Track your research
Methods in microbiome research: Past, present, and future
Affiliations.
- 1 NIH/NIAMS, Building 4 Room 228, 4 Memorial Drive, Bethesda, MD 20892, USA. Electronic address: [email protected].
- 2 Pediatric Translational Research Branch, 10 Center Drive, Bldg. 10, Rm 12N240E, Bethesda, MD 20892, USA. Electronic address: [email protected].
- 3 Oregon Health and Science University, 3215 SW Pavilion Loop, Lamfrom Biomedical Research Building 253E, Portland, OR 97239, USA. Electronic address: [email protected].
- PMID: 32340923
- PMCID: PMC7299794
- DOI: 10.1016/j.berh.2020.101498
The human microbiome is impressively immense and participates in many aspects of our health and wellness, particularly involving the development and maintenance of a healthy immune system. Not only do our microbes teach the immune system to fight infection, they also teach immune tolerance and help maintain homeostasis. From this knowledge, we have learned that the loss of tolerance to microbiota in both innate and adaptive processes plays an important role in immune-mediated and autoimmune disease. In this chapter, we will be discussing about methods used to study the microbiome, both old and new methods, fundamental concepts that have taken hold within the field, and how these principles relate to rheumatology, including thoughts on how microbiome research may be focused in the next decade.
Keywords: 16S rRNA gene sequencing; Dysbiosis; Ecological model; Metabolomics; Metagenomics; Microbiome; Mucosal immunity; Tolerance.
Copyright © 2020 Elsevier Ltd. All rights reserved.
Publication types
- Research Support, N.I.H., Intramural
- Microbiota*
- Research / trends
Grants and funding
- ZIA AR041184/ImNIH/Intramural NIH HHS/United States
Thank you for visiting nature.com. You are using a browser version with limited support for CSS. To obtain the best experience, we recommend you use a more up to date browser (or turn off compatibility mode in Internet Explorer). In the meantime, to ensure continued support, we are displaying the site without styles and JavaScript.
- View all journals
- My Account Login
- Explore content
- About the journal
- Publish with us
- Sign up for alerts
- Open access
- Published: 17 April 2024
The economic commitment of climate change
- Maximilian Kotz ORCID: orcid.org/0000-0003-2564-5043 1 , 2 ,
- Anders Levermann ORCID: orcid.org/0000-0003-4432-4704 1 , 2 &
- Leonie Wenz ORCID: orcid.org/0000-0002-8500-1568 1 , 3
Nature volume 628 , pages 551–557 ( 2024 ) Cite this article
14k Accesses
1983 Altmetric
Metrics details
- Environmental economics
- Environmental health
- Interdisciplinary studies
- Projection and prediction
Global projections of macroeconomic climate-change damages typically consider impacts from average annual and national temperatures over long time horizons 1 , 2 , 3 , 4 , 5 , 6 . Here we use recent empirical findings from more than 1,600 regions worldwide over the past 40 years to project sub-national damages from temperature and precipitation, including daily variability and extremes 7 , 8 . Using an empirical approach that provides a robust lower bound on the persistence of impacts on economic growth, we find that the world economy is committed to an income reduction of 19% within the next 26 years independent of future emission choices (relative to a baseline without climate impacts, likely range of 11–29% accounting for physical climate and empirical uncertainty). These damages already outweigh the mitigation costs required to limit global warming to 2 °C by sixfold over this near-term time frame and thereafter diverge strongly dependent on emission choices. Committed damages arise predominantly through changes in average temperature, but accounting for further climatic components raises estimates by approximately 50% and leads to stronger regional heterogeneity. Committed losses are projected for all regions except those at very high latitudes, at which reductions in temperature variability bring benefits. The largest losses are committed at lower latitudes in regions with lower cumulative historical emissions and lower present-day income.
Similar content being viewed by others
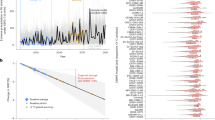
Climate damage projections beyond annual temperature
Paul Waidelich, Fulden Batibeniz, … Sonia I. Seneviratne
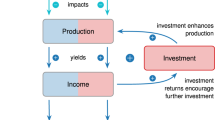
Investment incentive reduced by climate damages can be restored by optimal policy
Sven N. Willner, Nicole Glanemann & Anders Levermann
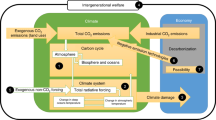
Climate economics support for the UN climate targets
Martin C. Hänsel, Moritz A. Drupp, … Thomas Sterner
Projections of the macroeconomic damage caused by future climate change are crucial to informing public and policy debates about adaptation, mitigation and climate justice. On the one hand, adaptation against climate impacts must be justified and planned on the basis of an understanding of their future magnitude and spatial distribution 9 . This is also of importance in the context of climate justice 10 , as well as to key societal actors, including governments, central banks and private businesses, which increasingly require the inclusion of climate risks in their macroeconomic forecasts to aid adaptive decision-making 11 , 12 . On the other hand, climate mitigation policy such as the Paris Climate Agreement is often evaluated by balancing the costs of its implementation against the benefits of avoiding projected physical damages. This evaluation occurs both formally through cost–benefit analyses 1 , 4 , 5 , 6 , as well as informally through public perception of mitigation and damage costs 13 .
Projections of future damages meet challenges when informing these debates, in particular the human biases relating to uncertainty and remoteness that are raised by long-term perspectives 14 . Here we aim to overcome such challenges by assessing the extent of economic damages from climate change to which the world is already committed by historical emissions and socio-economic inertia (the range of future emission scenarios that are considered socio-economically plausible 15 ). Such a focus on the near term limits the large uncertainties about diverging future emission trajectories, the resulting long-term climate response and the validity of applying historically observed climate–economic relations over long timescales during which socio-technical conditions may change considerably. As such, this focus aims to simplify the communication and maximize the credibility of projected economic damages from future climate change.
In projecting the future economic damages from climate change, we make use of recent advances in climate econometrics that provide evidence for impacts on sub-national economic growth from numerous components of the distribution of daily temperature and precipitation 3 , 7 , 8 . Using fixed-effects panel regression models to control for potential confounders, these studies exploit within-region variation in local temperature and precipitation in a panel of more than 1,600 regions worldwide, comprising climate and income data over the past 40 years, to identify the plausibly causal effects of changes in several climate variables on economic productivity 16 , 17 . Specifically, macroeconomic impacts have been identified from changing daily temperature variability, total annual precipitation, the annual number of wet days and extreme daily rainfall that occur in addition to those already identified from changing average temperature 2 , 3 , 18 . Moreover, regional heterogeneity in these effects based on the prevailing local climatic conditions has been found using interactions terms. The selection of these climate variables follows micro-level evidence for mechanisms related to the impacts of average temperatures on labour and agricultural productivity 2 , of temperature variability on agricultural productivity and health 7 , as well as of precipitation on agricultural productivity, labour outcomes and flood damages 8 (see Extended Data Table 1 for an overview, including more detailed references). References 7 , 8 contain a more detailed motivation for the use of these particular climate variables and provide extensive empirical tests about the robustness and nature of their effects on economic output, which are summarized in Methods . By accounting for these extra climatic variables at the sub-national level, we aim for a more comprehensive description of climate impacts with greater detail across both time and space.
Constraining the persistence of impacts
A key determinant and source of discrepancy in estimates of the magnitude of future climate damages is the extent to which the impact of a climate variable on economic growth rates persists. The two extreme cases in which these impacts persist indefinitely or only instantaneously are commonly referred to as growth or level effects 19 , 20 (see Methods section ‘Empirical model specification: fixed-effects distributed lag models’ for mathematical definitions). Recent work shows that future damages from climate change depend strongly on whether growth or level effects are assumed 20 . Following refs. 2 , 18 , we provide constraints on this persistence by using distributed lag models to test the significance of delayed effects separately for each climate variable. Notably, and in contrast to refs. 2 , 18 , we use climate variables in their first-differenced form following ref. 3 , implying a dependence of the growth rate on a change in climate variables. This choice means that a baseline specification without any lags constitutes a model prior of purely level effects, in which a permanent change in the climate has only an instantaneous effect on the growth rate 3 , 19 , 21 . By including lags, one can then test whether any effects may persist further. This is in contrast to the specification used by refs. 2 , 18 , in which climate variables are used without taking the first difference, implying a dependence of the growth rate on the level of climate variables. In this alternative case, the baseline specification without any lags constitutes a model prior of pure growth effects, in which a change in climate has an infinitely persistent effect on the growth rate. Consequently, including further lags in this alternative case tests whether the initial growth impact is recovered 18 , 19 , 21 . Both of these specifications suffer from the limiting possibility that, if too few lags are included, one might falsely accept the model prior. The limitations of including a very large number of lags, including loss of data and increasing statistical uncertainty with an increasing number of parameters, mean that such a possibility is likely. By choosing a specification in which the model prior is one of level effects, our approach is therefore conservative by design, avoiding assumptions of infinite persistence of climate impacts on growth and instead providing a lower bound on this persistence based on what is observable empirically (see Methods section ‘Empirical model specification: fixed-effects distributed lag models’ for further exposition of this framework). The conservative nature of such a choice is probably the reason that ref. 19 finds much greater consistency between the impacts projected by models that use the first difference of climate variables, as opposed to their levels.
We begin our empirical analysis of the persistence of climate impacts on growth using ten lags of the first-differenced climate variables in fixed-effects distributed lag models. We detect substantial effects on economic growth at time lags of up to approximately 8–10 years for the temperature terms and up to approximately 4 years for the precipitation terms (Extended Data Fig. 1 and Extended Data Table 2 ). Furthermore, evaluation by means of information criteria indicates that the inclusion of all five climate variables and the use of these numbers of lags provide a preferable trade-off between best-fitting the data and including further terms that could cause overfitting, in comparison with model specifications excluding climate variables or including more or fewer lags (Extended Data Fig. 3 , Supplementary Methods Section 1 and Supplementary Table 1 ). We therefore remove statistically insignificant terms at later lags (Supplementary Figs. 1 – 3 and Supplementary Tables 2 – 4 ). Further tests using Monte Carlo simulations demonstrate that the empirical models are robust to autocorrelation in the lagged climate variables (Supplementary Methods Section 2 and Supplementary Figs. 4 and 5 ), that information criteria provide an effective indicator for lag selection (Supplementary Methods Section 2 and Supplementary Fig. 6 ), that the results are robust to concerns of imperfect multicollinearity between climate variables and that including several climate variables is actually necessary to isolate their separate effects (Supplementary Methods Section 3 and Supplementary Fig. 7 ). We provide a further robustness check using a restricted distributed lag model to limit oscillations in the lagged parameter estimates that may result from autocorrelation, finding that it provides similar estimates of cumulative marginal effects to the unrestricted model (Supplementary Methods Section 4 and Supplementary Figs. 8 and 9 ). Finally, to explicitly account for any outstanding uncertainty arising from the precise choice of the number of lags, we include empirical models with marginally different numbers of lags in the error-sampling procedure of our projection of future damages. On the basis of the lag-selection procedure (the significance of lagged terms in Extended Data Fig. 1 and Extended Data Table 2 , as well as information criteria in Extended Data Fig. 3 ), we sample from models with eight to ten lags for temperature and four for precipitation (models shown in Supplementary Figs. 1 – 3 and Supplementary Tables 2 – 4 ). In summary, this empirical approach to constrain the persistence of climate impacts on economic growth rates is conservative by design in avoiding assumptions of infinite persistence, but nevertheless provides a lower bound on the extent of impact persistence that is robust to the numerous tests outlined above.
Committed damages until mid-century
We combine these empirical economic response functions (Supplementary Figs. 1 – 3 and Supplementary Tables 2 – 4 ) with an ensemble of 21 climate models (see Supplementary Table 5 ) from the Coupled Model Intercomparison Project Phase 6 (CMIP-6) 22 to project the macroeconomic damages from these components of physical climate change (see Methods for further details). Bias-adjusted climate models that provide a highly accurate reproduction of observed climatological patterns with limited uncertainty (Supplementary Table 6 ) are used to avoid introducing biases in the projections. Following a well-developed literature 2 , 3 , 19 , these projections do not aim to provide a prediction of future economic growth. Instead, they are a projection of the exogenous impact of future climate conditions on the economy relative to the baselines specified by socio-economic projections, based on the plausibly causal relationships inferred by the empirical models and assuming ceteris paribus. Other exogenous factors relevant for the prediction of economic output are purposefully assumed constant.
A Monte Carlo procedure that samples from climate model projections, empirical models with different numbers of lags and model parameter estimates (obtained by 1,000 block-bootstrap resamples of each of the regressions in Supplementary Figs. 1 – 3 and Supplementary Tables 2 – 4 ) is used to estimate the combined uncertainty from these sources. Given these uncertainty distributions, we find that projected global damages are statistically indistinguishable across the two most extreme emission scenarios until 2049 (at the 5% significance level; Fig. 1 ). As such, the climate damages occurring before this time constitute those to which the world is already committed owing to the combination of past emissions and the range of future emission scenarios that are considered socio-economically plausible 15 . These committed damages comprise a permanent income reduction of 19% on average globally (population-weighted average) in comparison with a baseline without climate-change impacts (with a likely range of 11–29%, following the likelihood classification adopted by the Intergovernmental Panel on Climate Change (IPCC); see caption of Fig. 1 ). Even though levels of income per capita generally still increase relative to those of today, this constitutes a permanent income reduction for most regions, including North America and Europe (each with median income reductions of approximately 11%) and with South Asia and Africa being the most strongly affected (each with median income reductions of approximately 22%; Fig. 1 ). Under a middle-of-the road scenario of future income development (SSP2, in which SSP stands for Shared Socio-economic Pathway), this corresponds to global annual damages in 2049 of 38 trillion in 2005 international dollars (likely range of 19–59 trillion 2005 international dollars). Compared with empirical specifications that assume pure growth or pure level effects, our preferred specification that provides a robust lower bound on the extent of climate impact persistence produces damages between these two extreme assumptions (Extended Data Fig. 3 ).
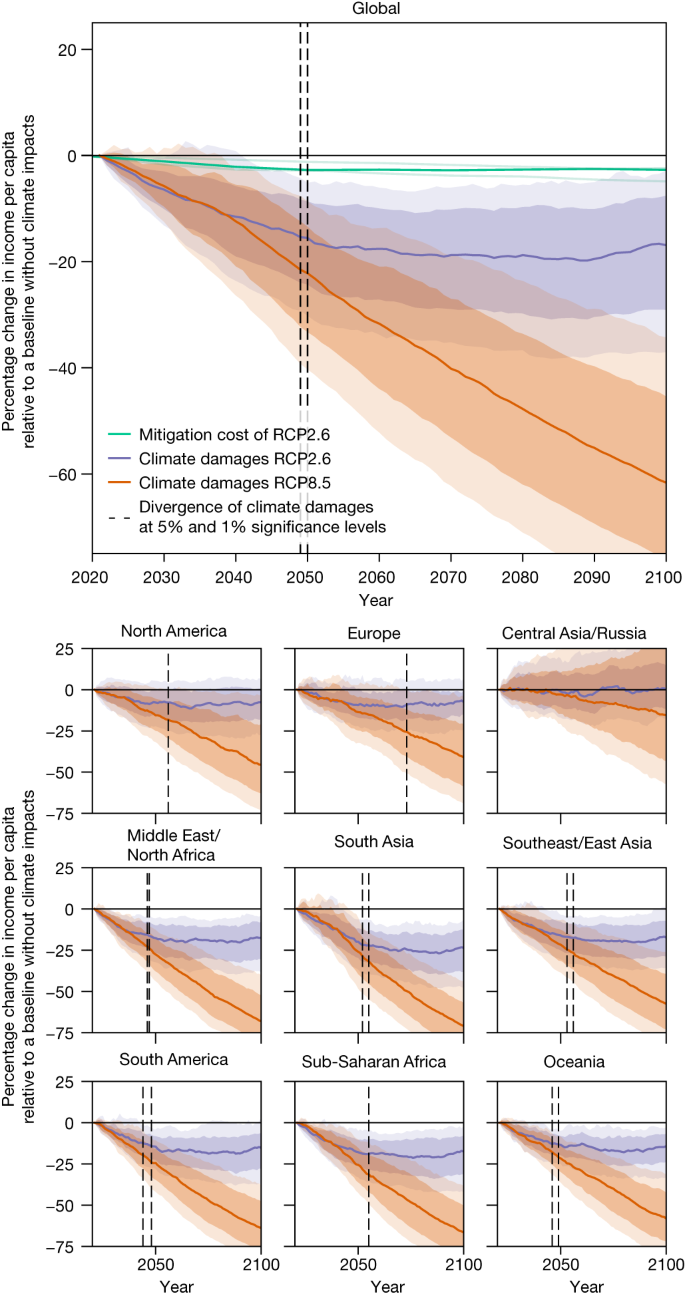
Estimates of the projected reduction in income per capita from changes in all climate variables based on empirical models of climate impacts on economic output with a robust lower bound on their persistence (Extended Data Fig. 1 ) under a low-emission scenario compatible with the 2 °C warming target and a high-emission scenario (SSP2-RCP2.6 and SSP5-RCP8.5, respectively) are shown in purple and orange, respectively. Shading represents the 34% and 10% confidence intervals reflecting the likely and very likely ranges, respectively (following the likelihood classification adopted by the IPCC), having estimated uncertainty from a Monte Carlo procedure, which samples the uncertainty from the choice of physical climate models, empirical models with different numbers of lags and bootstrapped estimates of the regression parameters shown in Supplementary Figs. 1 – 3 . Vertical dashed lines show the time at which the climate damages of the two emission scenarios diverge at the 5% and 1% significance levels based on the distribution of differences between emission scenarios arising from the uncertainty sampling discussed above. Note that uncertainty in the difference of the two scenarios is smaller than the combined uncertainty of the two respective scenarios because samples of the uncertainty (climate model and empirical model choice, as well as model parameter bootstrap) are consistent across the two emission scenarios, hence the divergence of damages occurs while the uncertainty bounds of the two separate damage scenarios still overlap. Estimates of global mitigation costs from the three IAMs that provide results for the SSP2 baseline and SSP2-RCP2.6 scenario are shown in light green in the top panel, with the median of these estimates shown in bold.
Damages already outweigh mitigation costs
We compare the damages to which the world is committed over the next 25 years to estimates of the mitigation costs required to achieve the Paris Climate Agreement. Taking estimates of mitigation costs from the three integrated assessment models (IAMs) in the IPCC AR6 database 23 that provide results under comparable scenarios (SSP2 baseline and SSP2-RCP2.6, in which RCP stands for Representative Concentration Pathway), we find that the median committed climate damages are larger than the median mitigation costs in 2050 (six trillion in 2005 international dollars) by a factor of approximately six (note that estimates of mitigation costs are only provided every 10 years by the IAMs and so a comparison in 2049 is not possible). This comparison simply aims to compare the magnitude of future damages against mitigation costs, rather than to conduct a formal cost–benefit analysis of transitioning from one emission path to another. Formal cost–benefit analyses typically find that the net benefits of mitigation only emerge after 2050 (ref. 5 ), which may lead some to conclude that physical damages from climate change are simply not large enough to outweigh mitigation costs until the second half of the century. Our simple comparison of their magnitudes makes clear that damages are actually already considerably larger than mitigation costs and the delayed emergence of net mitigation benefits results primarily from the fact that damages across different emission paths are indistinguishable until mid-century (Fig. 1 ).
Although these near-term damages constitute those to which the world is already committed, we note that damage estimates diverge strongly across emission scenarios after 2049, conveying the clear benefits of mitigation from a purely economic point of view that have been emphasized in previous studies 4 , 24 . As well as the uncertainties assessed in Fig. 1 , these conclusions are robust to structural choices, such as the timescale with which changes in the moderating variables of the empirical models are estimated (Supplementary Figs. 10 and 11 ), as well as the order in which one accounts for the intertemporal and international components of currency comparison (Supplementary Fig. 12 ; see Methods for further details).
Damages from variability and extremes
Committed damages primarily arise through changes in average temperature (Fig. 2 ). This reflects the fact that projected changes in average temperature are larger than those in other climate variables when expressed as a function of their historical interannual variability (Extended Data Fig. 4 ). Because the historical variability is that on which the empirical models are estimated, larger projected changes in comparison with this variability probably lead to larger future impacts in a purely statistical sense. From a mechanistic perspective, one may plausibly interpret this result as implying that future changes in average temperature are the most unprecedented from the perspective of the historical fluctuations to which the economy is accustomed and therefore will cause the most damage. This insight may prove useful in terms of guiding adaptation measures to the sources of greatest damage.
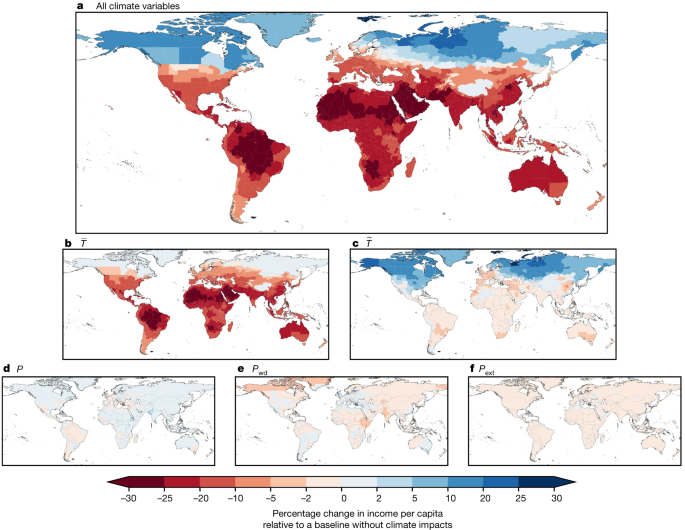
Estimates of the median projected reduction in sub-national income per capita across emission scenarios (SSP2-RCP2.6 and SSP2-RCP8.5) as well as climate model, empirical model and model parameter uncertainty in the year in which climate damages diverge at the 5% level (2049, as identified in Fig. 1 ). a , Impacts arising from all climate variables. b – f , Impacts arising separately from changes in annual mean temperature ( b ), daily temperature variability ( c ), total annual precipitation ( d ), the annual number of wet days (>1 mm) ( e ) and extreme daily rainfall ( f ) (see Methods for further definitions). Data on national administrative boundaries are obtained from the GADM database version 3.6 and are freely available for academic use ( https://gadm.org/ ).
Nevertheless, future damages based on empirical models that consider changes in annual average temperature only and exclude the other climate variables constitute income reductions of only 13% in 2049 (Extended Data Fig. 5a , likely range 5–21%). This suggests that accounting for the other components of the distribution of temperature and precipitation raises net damages by nearly 50%. This increase arises through the further damages that these climatic components cause, but also because their inclusion reveals a stronger negative economic response to average temperatures (Extended Data Fig. 5b ). The latter finding is consistent with our Monte Carlo simulations, which suggest that the magnitude of the effect of average temperature on economic growth is underestimated unless accounting for the impacts of other correlated climate variables (Supplementary Fig. 7 ).
In terms of the relative contributions of the different climatic components to overall damages, we find that accounting for daily temperature variability causes the largest increase in overall damages relative to empirical frameworks that only consider changes in annual average temperature (4.9 percentage points, likely range 2.4–8.7 percentage points, equivalent to approximately 10 trillion international dollars). Accounting for precipitation causes smaller increases in overall damages, which are—nevertheless—equivalent to approximately 1.2 trillion international dollars: 0.01 percentage points (−0.37–0.33 percentage points), 0.34 percentage points (0.07–0.90 percentage points) and 0.36 percentage points (0.13–0.65 percentage points) from total annual precipitation, the number of wet days and extreme daily precipitation, respectively. Moreover, climate models seem to underestimate future changes in temperature variability 25 and extreme precipitation 26 , 27 in response to anthropogenic forcing as compared with that observed historically, suggesting that the true impacts from these variables may be larger.
The distribution of committed damages
The spatial distribution of committed damages (Fig. 2a ) reflects a complex interplay between the patterns of future change in several climatic components and those of historical economic vulnerability to changes in those variables. Damages resulting from increasing annual mean temperature (Fig. 2b ) are negative almost everywhere globally, and larger at lower latitudes in regions in which temperatures are already higher and economic vulnerability to temperature increases is greatest (see the response heterogeneity to mean temperature embodied in Extended Data Fig. 1a ). This occurs despite the amplified warming projected at higher latitudes 28 , suggesting that regional heterogeneity in economic vulnerability to temperature changes outweighs heterogeneity in the magnitude of future warming (Supplementary Fig. 13a ). Economic damages owing to daily temperature variability (Fig. 2c ) exhibit a strong latitudinal polarisation, primarily reflecting the physical response of daily variability to greenhouse forcing in which increases in variability across lower latitudes (and Europe) contrast decreases at high latitudes 25 (Supplementary Fig. 13b ). These two temperature terms are the dominant determinants of the pattern of overall damages (Fig. 2a ), which exhibits a strong polarity with damages across most of the globe except at the highest northern latitudes. Future changes in total annual precipitation mainly bring economic benefits except in regions of drying, such as the Mediterranean and central South America (Fig. 2d and Supplementary Fig. 13c ), but these benefits are opposed by changes in the number of wet days, which produce damages with a similar pattern of opposite sign (Fig. 2e and Supplementary Fig. 13d ). By contrast, changes in extreme daily rainfall produce damages in all regions, reflecting the intensification of daily rainfall extremes over global land areas 29 , 30 (Fig. 2f and Supplementary Fig. 13e ).
The spatial distribution of committed damages implies considerable injustice along two dimensions: culpability for the historical emissions that have caused climate change and pre-existing levels of socio-economic welfare. Spearman’s rank correlations indicate that committed damages are significantly larger in countries with smaller historical cumulative emissions, as well as in regions with lower current income per capita (Fig. 3 ). This implies that those countries that will suffer the most from the damages already committed are those that are least responsible for climate change and which also have the least resources to adapt to it.
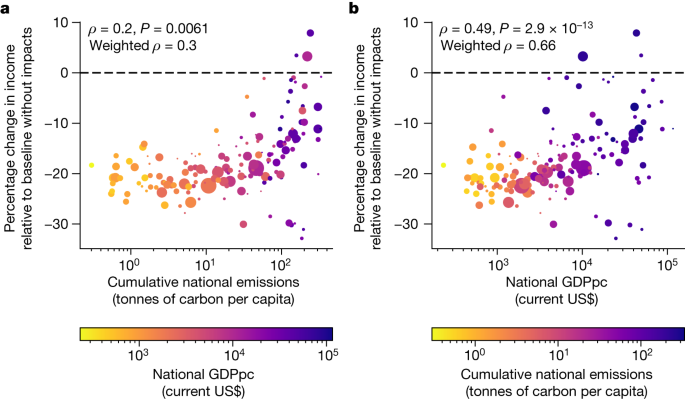
Estimates of the median projected change in national income per capita across emission scenarios (RCP2.6 and RCP8.5) as well as climate model, empirical model and model parameter uncertainty in the year in which climate damages diverge at the 5% level (2049, as identified in Fig. 1 ) are plotted against cumulative national emissions per capita in 2020 (from the Global Carbon Project) and coloured by national income per capita in 2020 (from the World Bank) in a and vice versa in b . In each panel, the size of each scatter point is weighted by the national population in 2020 (from the World Bank). Inset numbers indicate the Spearman’s rank correlation ρ and P -values for a hypothesis test whose null hypothesis is of no correlation, as well as the Spearman’s rank correlation weighted by national population.
To further quantify this heterogeneity, we assess the difference in committed damages between the upper and lower quartiles of regions when ranked by present income levels and historical cumulative emissions (using a population weighting to both define the quartiles and estimate the group averages). On average, the quartile of countries with lower income are committed to an income loss that is 8.9 percentage points (or 61%) greater than the upper quartile (Extended Data Fig. 6 ), with a likely range of 3.8–14.7 percentage points across the uncertainty sampling of our damage projections (following the likelihood classification adopted by the IPCC). Similarly, the quartile of countries with lower historical cumulative emissions are committed to an income loss that is 6.9 percentage points (or 40%) greater than the upper quartile, with a likely range of 0.27–12 percentage points. These patterns reemphasize the prevalence of injustice in climate impacts 31 , 32 , 33 in the context of the damages to which the world is already committed by historical emissions and socio-economic inertia.
Contextualizing the magnitude of damages
The magnitude of projected economic damages exceeds previous literature estimates 2 , 3 , arising from several developments made on previous approaches. Our estimates are larger than those of ref. 2 (see first row of Extended Data Table 3 ), primarily because of the facts that sub-national estimates typically show a steeper temperature response (see also refs. 3 , 34 ) and that accounting for other climatic components raises damage estimates (Extended Data Fig. 5 ). However, we note that our empirical approach using first-differenced climate variables is conservative compared with that of ref. 2 in regard to the persistence of climate impacts on growth (see introduction and Methods section ‘Empirical model specification: fixed-effects distributed lag models’), an important determinant of the magnitude of long-term damages 19 , 21 . Using a similar empirical specification to ref. 2 , which assumes infinite persistence while maintaining the rest of our approach (sub-national data and further climate variables), produces considerably larger damages (purple curve of Extended Data Fig. 3 ). Compared with studies that do take the first difference of climate variables 3 , 35 , our estimates are also larger (see second and third rows of Extended Data Table 3 ). The inclusion of further climate variables (Extended Data Fig. 5 ) and a sufficient number of lags to more adequately capture the extent of impact persistence (Extended Data Figs. 1 and 2 ) are the main sources of this difference, as is the use of specifications that capture nonlinearities in the temperature response when compared with ref. 35 . In summary, our estimates develop on previous studies by incorporating the latest data and empirical insights 7 , 8 , as well as in providing a robust empirical lower bound on the persistence of impacts on economic growth, which constitutes a middle ground between the extremes of the growth-versus-levels debate 19 , 21 (Extended Data Fig. 3 ).
Compared with the fraction of variance explained by the empirical models historically (<5%), the projection of reductions in income of 19% may seem large. This arises owing to the fact that projected changes in climatic conditions are much larger than those that were experienced historically, particularly for changes in average temperature (Extended Data Fig. 4 ). As such, any assessment of future climate-change impacts necessarily requires an extrapolation outside the range of the historical data on which the empirical impact models were evaluated. Nevertheless, these models constitute the most state-of-the-art methods for inference of plausibly causal climate impacts based on observed data. Moreover, we take explicit steps to limit out-of-sample extrapolation by capping the moderating variables of the interaction terms at the 95th percentile of the historical distribution (see Methods ). This avoids extrapolating the marginal effects outside what was observed historically. Given the nonlinear response of economic output to annual mean temperature (Extended Data Fig. 1 and Extended Data Table 2 ), this is a conservative choice that limits the magnitude of damages that we project. Furthermore, back-of-the-envelope calculations indicate that the projected damages are consistent with the magnitude and patterns of historical economic development (see Supplementary Discussion Section 5 ).
Missing impacts and spatial spillovers
Despite assessing several climatic components from which economic impacts have recently been identified 3 , 7 , 8 , this assessment of aggregate climate damages should not be considered comprehensive. Important channels such as impacts from heatwaves 31 , sea-level rise 36 , tropical cyclones 37 and tipping points 38 , 39 , as well as non-market damages such as those to ecosystems 40 and human health 41 , are not considered in these estimates. Sea-level rise is unlikely to be feasibly incorporated into empirical assessments such as this because historical sea-level variability is mostly small. Non-market damages are inherently intractable within our estimates of impacts on aggregate monetary output and estimates of these impacts could arguably be considered as extra to those identified here. Recent empirical work suggests that accounting for these channels would probably raise estimates of these committed damages, with larger damages continuing to arise in the global south 31 , 36 , 37 , 38 , 39 , 40 , 41 , 42 .
Moreover, our main empirical analysis does not explicitly evaluate the potential for impacts in local regions to produce effects that ‘spill over’ into other regions. Such effects may further mitigate or amplify the impacts we estimate, for example, if companies relocate production from one affected region to another or if impacts propagate along supply chains. The current literature indicates that trade plays a substantial role in propagating spillover effects 43 , 44 , making their assessment at the sub-national level challenging without available data on sub-national trade dependencies. Studies accounting for only spatially adjacent neighbours indicate that negative impacts in one region induce further negative impacts in neighbouring regions 45 , 46 , 47 , 48 , suggesting that our projected damages are probably conservative by excluding these effects. In Supplementary Fig. 14 , we assess spillovers from neighbouring regions using a spatial-lag model. For simplicity, this analysis excludes temporal lags, focusing only on contemporaneous effects. The results show that accounting for spatial spillovers can amplify the overall magnitude, and also the heterogeneity, of impacts. Consistent with previous literature, this indicates that the overall magnitude (Fig. 1 ) and heterogeneity (Fig. 3 ) of damages that we project in our main specification may be conservative without explicitly accounting for spillovers. We note that further analysis that addresses both spatially and trade-connected spillovers, while also accounting for delayed impacts using temporal lags, would be necessary to adequately address this question fully. These approaches offer fruitful avenues for further research but are beyond the scope of this manuscript, which primarily aims to explore the impacts of different climate conditions and their persistence.
Policy implications
We find that the economic damages resulting from climate change until 2049 are those to which the world economy is already committed and that these greatly outweigh the costs required to mitigate emissions in line with the 2 °C target of the Paris Climate Agreement (Fig. 1 ). This assessment is complementary to formal analyses of the net costs and benefits associated with moving from one emission path to another, which typically find that net benefits of mitigation only emerge in the second half of the century 5 . Our simple comparison of the magnitude of damages and mitigation costs makes clear that this is primarily because damages are indistinguishable across emissions scenarios—that is, committed—until mid-century (Fig. 1 ) and that they are actually already much larger than mitigation costs. For simplicity, and owing to the availability of data, we compare damages to mitigation costs at the global level. Regional estimates of mitigation costs may shed further light on the national incentives for mitigation to which our results already hint, of relevance for international climate policy. Although these damages are committed from a mitigation perspective, adaptation may provide an opportunity to reduce them. Moreover, the strong divergence of damages after mid-century reemphasizes the clear benefits of mitigation from a purely economic perspective, as highlighted in previous studies 1 , 4 , 6 , 24 .
Historical climate data
Historical daily 2-m temperature and precipitation totals (in mm) are obtained for the period 1979–2019 from the W5E5 database. The W5E5 dataset comes from ERA-5, a state-of-the-art reanalysis of historical observations, but has been bias-adjusted by applying version 2.0 of the WATCH Forcing Data to ERA-5 reanalysis data and precipitation data from version 2.3 of the Global Precipitation Climatology Project to better reflect ground-based measurements 49 , 50 , 51 . We obtain these data on a 0.5° × 0.5° grid from the Inter-Sectoral Impact Model Intercomparison Project (ISIMIP) database. Notably, these historical data have been used to bias-adjust future climate projections from CMIP-6 (see the following section), ensuring consistency between the distribution of historical daily weather on which our empirical models were estimated and the climate projections used to estimate future damages. These data are publicly available from the ISIMIP database. See refs. 7 , 8 for robustness tests of the empirical models to the choice of climate data reanalysis products.
Future climate data
Daily 2-m temperature and precipitation totals (in mm) are taken from 21 climate models participating in CMIP-6 under a high (RCP8.5) and a low (RCP2.6) greenhouse gas emission scenario from 2015 to 2100. The data have been bias-adjusted and statistically downscaled to a common half-degree grid to reflect the historical distribution of daily temperature and precipitation of the W5E5 dataset using the trend-preserving method developed by the ISIMIP 50 , 52 . As such, the climate model data reproduce observed climatological patterns exceptionally well (Supplementary Table 5 ). Gridded data are publicly available from the ISIMIP database.
Historical economic data
Historical economic data come from the DOSE database of sub-national economic output 53 . We use a recent revision to the DOSE dataset that provides data across 83 countries, 1,660 sub-national regions with varying temporal coverage from 1960 to 2019. Sub-national units constitute the first administrative division below national, for example, states for the USA and provinces for China. Data come from measures of gross regional product per capita (GRPpc) or income per capita in local currencies, reflecting the values reported in national statistical agencies, yearbooks and, in some cases, academic literature. We follow previous literature 3 , 7 , 8 , 54 and assess real sub-national output per capita by first converting values from local currencies to US dollars to account for diverging national inflationary tendencies and then account for US inflation using a US deflator. Alternatively, one might first account for national inflation and then convert between currencies. Supplementary Fig. 12 demonstrates that our conclusions are consistent when accounting for price changes in the reversed order, although the magnitude of estimated damages varies. See the documentation of the DOSE dataset for further discussion of these choices. Conversions between currencies are conducted using exchange rates from the FRED database of the Federal Reserve Bank of St. Louis 55 and the national deflators from the World Bank 56 .
Future socio-economic data
Baseline gridded gross domestic product (GDP) and population data for the period 2015–2100 are taken from the middle-of-the-road scenario SSP2 (ref. 15 ). Population data have been downscaled to a half-degree grid by the ISIMIP following the methodologies of refs. 57 , 58 , which we then aggregate to the sub-national level of our economic data using the spatial aggregation procedure described below. Because current methodologies for downscaling the GDP of the SSPs use downscaled population to do so, per-capita estimates of GDP with a realistic distribution at the sub-national level are not readily available for the SSPs. We therefore use national-level GDP per capita (GDPpc) projections for all sub-national regions of a given country, assuming homogeneity within countries in terms of baseline GDPpc. Here we use projections that have been updated to account for the impact of the COVID-19 pandemic on the trajectory of future income, while remaining consistent with the long-term development of the SSPs 59 . The choice of baseline SSP alters the magnitude of projected climate damages in monetary terms, but when assessed in terms of percentage change from the baseline, the choice of socio-economic scenario is inconsequential. Gridded SSP population data and national-level GDPpc data are publicly available from the ISIMIP database. Sub-national estimates as used in this study are available in the code and data replication files.
Climate variables
Following recent literature 3 , 7 , 8 , we calculate an array of climate variables for which substantial impacts on macroeconomic output have been identified empirically, supported by further evidence at the micro level for plausible underlying mechanisms. See refs. 7 , 8 for an extensive motivation for the use of these particular climate variables and for detailed empirical tests on the nature and robustness of their effects on economic output. To summarize, these studies have found evidence for independent impacts on economic growth rates from annual average temperature, daily temperature variability, total annual precipitation, the annual number of wet days and extreme daily rainfall. Assessments of daily temperature variability were motivated by evidence of impacts on agricultural output and human health, as well as macroeconomic literature on the impacts of volatility on growth when manifest in different dimensions, such as government spending, exchange rates and even output itself 7 . Assessments of precipitation impacts were motivated by evidence of impacts on agricultural productivity, metropolitan labour outcomes and conflict, as well as damages caused by flash flooding 8 . See Extended Data Table 1 for detailed references to empirical studies of these physical mechanisms. Marked impacts of daily temperature variability, total annual precipitation, the number of wet days and extreme daily rainfall on macroeconomic output were identified robustly across different climate datasets, spatial aggregation schemes, specifications of regional time trends and error-clustering approaches. They were also found to be robust to the consideration of temperature extremes 7 , 8 . Furthermore, these climate variables were identified as having independent effects on economic output 7 , 8 , which we further explain here using Monte Carlo simulations to demonstrate the robustness of the results to concerns of imperfect multicollinearity between climate variables (Supplementary Methods Section 2 ), as well as by using information criteria (Supplementary Table 1 ) to demonstrate that including several lagged climate variables provides a preferable trade-off between optimally describing the data and limiting the possibility of overfitting.
We calculate these variables from the distribution of daily, d , temperature, T x , d , and precipitation, P x , d , at the grid-cell, x , level for both the historical and future climate data. As well as annual mean temperature, \({\bar{T}}_{x,y}\) , and annual total precipitation, P x , y , we calculate annual, y , measures of daily temperature variability, \({\widetilde{T}}_{x,y}\) :
the number of wet days, Pwd x , y :
and extreme daily rainfall:
in which T x , d , m , y is the grid-cell-specific daily temperature in month m and year y , \({\bar{T}}_{x,m,{y}}\) is the year and grid-cell-specific monthly, m , mean temperature, D m and D y the number of days in a given month m or year y , respectively, H the Heaviside step function, 1 mm the threshold used to define wet days and P 99.9 x is the 99.9th percentile of historical (1979–2019) daily precipitation at the grid-cell level. Units of the climate measures are degrees Celsius for annual mean temperature and daily temperature variability, millimetres for total annual precipitation and extreme daily precipitation, and simply the number of days for the annual number of wet days.
We also calculated weighted standard deviations of monthly rainfall totals as also used in ref. 8 but do not include them in our projections as we find that, when accounting for delayed effects, their effect becomes statistically indistinct and is better captured by changes in total annual rainfall.
Spatial aggregation
We aggregate grid-cell-level historical and future climate measures, as well as grid-cell-level future GDPpc and population, to the level of the first administrative unit below national level of the GADM database, using an area-weighting algorithm that estimates the portion of each grid cell falling within an administrative boundary. We use this as our baseline specification following previous findings that the effect of area or population weighting at the sub-national level is negligible 7 , 8 .
Empirical model specification: fixed-effects distributed lag models
Following a wide range of climate econometric literature 16 , 60 , we use panel regression models with a selection of fixed effects and time trends to isolate plausibly exogenous variation with which to maximize confidence in a causal interpretation of the effects of climate on economic growth rates. The use of region fixed effects, μ r , accounts for unobserved time-invariant differences between regions, such as prevailing climatic norms and growth rates owing to historical and geopolitical factors. The use of yearly fixed effects, η y , accounts for regionally invariant annual shocks to the global climate or economy such as the El Niño–Southern Oscillation or global recessions. In our baseline specification, we also include region-specific linear time trends, k r y , to exclude the possibility of spurious correlations resulting from common slow-moving trends in climate and growth.
The persistence of climate impacts on economic growth rates is a key determinant of the long-term magnitude of damages. Methods for inferring the extent of persistence in impacts on growth rates have typically used lagged climate variables to evaluate the presence of delayed effects or catch-up dynamics 2 , 18 . For example, consider starting from a model in which a climate condition, C r , y , (for example, annual mean temperature) affects the growth rate, Δlgrp r , y (the first difference of the logarithm of gross regional product) of region r in year y :
which we refer to as a ‘pure growth effects’ model in the main text. Typically, further lags are included,
and the cumulative effect of all lagged terms is evaluated to assess the extent to which climate impacts on growth rates persist. Following ref. 18 , in the case that,
the implication is that impacts on the growth rate persist up to NL years after the initial shock (possibly to a weaker or a stronger extent), whereas if
then the initial impact on the growth rate is recovered after NL years and the effect is only one on the level of output. However, we note that such approaches are limited by the fact that, when including an insufficient number of lags to detect a recovery of the growth rates, one may find equation ( 6 ) to be satisfied and incorrectly assume that a change in climatic conditions affects the growth rate indefinitely. In practice, given a limited record of historical data, including too few lags to confidently conclude in an infinitely persistent impact on the growth rate is likely, particularly over the long timescales over which future climate damages are often projected 2 , 24 . To avoid this issue, we instead begin our analysis with a model for which the level of output, lgrp r , y , depends on the level of a climate variable, C r , y :
Given the non-stationarity of the level of output, we follow the literature 19 and estimate such an equation in first-differenced form as,
which we refer to as a model of ‘pure level effects’ in the main text. This model constitutes a baseline specification in which a permanent change in the climate variable produces an instantaneous impact on the growth rate and a permanent effect only on the level of output. By including lagged variables in this specification,
we are able to test whether the impacts on the growth rate persist any further than instantaneously by evaluating whether α L > 0 are statistically significantly different from zero. Even though this framework is also limited by the possibility of including too few lags, the choice of a baseline model specification in which impacts on the growth rate do not persist means that, in the case of including too few lags, the framework reverts to the baseline specification of level effects. As such, this framework is conservative with respect to the persistence of impacts and the magnitude of future damages. It naturally avoids assumptions of infinite persistence and we are able to interpret any persistence that we identify with equation ( 9 ) as a lower bound on the extent of climate impact persistence on growth rates. See the main text for further discussion of this specification choice, in particular about its conservative nature compared with previous literature estimates, such as refs. 2 , 18 .
We allow the response to climatic changes to vary across regions, using interactions of the climate variables with historical average (1979–2019) climatic conditions reflecting heterogenous effects identified in previous work 7 , 8 . Following this previous work, the moderating variables of these interaction terms constitute the historical average of either the variable itself or of the seasonal temperature difference, \({\hat{T}}_{r}\) , or annual mean temperature, \({\bar{T}}_{r}\) , in the case of daily temperature variability 7 and extreme daily rainfall, respectively 8 .
The resulting regression equation with N and M lagged variables, respectively, reads:
in which Δlgrp r , y is the annual, regional GRPpc growth rate, measured as the first difference of the logarithm of real GRPpc, following previous work 2 , 3 , 7 , 8 , 18 , 19 . Fixed-effects regressions were run using the fixest package in R (ref. 61 ).
Estimates of the coefficients of interest α i , L are shown in Extended Data Fig. 1 for N = M = 10 lags and for our preferred choice of the number of lags in Supplementary Figs. 1 – 3 . In Extended Data Fig. 1 , errors are shown clustered at the regional level, but for the construction of damage projections, we block-bootstrap the regressions by region 1,000 times to provide a range of parameter estimates with which to sample the projection uncertainty (following refs. 2 , 31 ).
Spatial-lag model
In Supplementary Fig. 14 , we present the results from a spatial-lag model that explores the potential for climate impacts to ‘spill over’ into spatially neighbouring regions. We measure the distance between centroids of each pair of sub-national regions and construct spatial lags that take the average of the first-differenced climate variables and their interaction terms over neighbouring regions that are at distances of 0–500, 500–1,000, 1,000–1,500 and 1,500–2000 km (spatial lags, ‘SL’, 1 to 4). For simplicity, we then assess a spatial-lag model without temporal lags to assess spatial spillovers of contemporaneous climate impacts. This model takes the form:
in which SL indicates the spatial lag of each climate variable and interaction term. In Supplementary Fig. 14 , we plot the cumulative marginal effect of each climate variable at different baseline climate conditions by summing the coefficients for each climate variable and interaction term, for example, for average temperature impacts as:
These cumulative marginal effects can be regarded as the overall spatially dependent impact to an individual region given a one-unit shock to a climate variable in that region and all neighbouring regions at a given value of the moderating variable of the interaction term.
Constructing projections of economic damage from future climate change
We construct projections of future climate damages by applying the coefficients estimated in equation ( 10 ) and shown in Supplementary Tables 2 – 4 (when including only lags with statistically significant effects in specifications that limit overfitting; see Supplementary Methods Section 1 ) to projections of future climate change from the CMIP-6 models. Year-on-year changes in each primary climate variable of interest are calculated to reflect the year-to-year variations used in the empirical models. 30-year moving averages of the moderating variables of the interaction terms are calculated to reflect the long-term average of climatic conditions that were used for the moderating variables in the empirical models. By using moving averages in the projections, we account for the changing vulnerability to climate shocks based on the evolving long-term conditions (Supplementary Figs. 10 and 11 show that the results are robust to the precise choice of the window of this moving average). Although these climate variables are not differenced, the fact that the bias-adjusted climate models reproduce observed climatological patterns across regions for these moderating variables very accurately (Supplementary Table 6 ) with limited spread across models (<3%) precludes the possibility that any considerable bias or uncertainty is introduced by this methodological choice. However, we impose caps on these moderating variables at the 95th percentile at which they were observed in the historical data to prevent extrapolation of the marginal effects outside the range in which the regressions were estimated. This is a conservative choice that limits the magnitude of our damage projections.
Time series of primary climate variables and moderating climate variables are then combined with estimates of the empirical model parameters to evaluate the regression coefficients in equation ( 10 ), producing a time series of annual GRPpc growth-rate reductions for a given emission scenario, climate model and set of empirical model parameters. The resulting time series of growth-rate impacts reflects those occurring owing to future climate change. By contrast, a future scenario with no climate change would be one in which climate variables do not change (other than with random year-to-year fluctuations) and hence the time-averaged evaluation of equation ( 10 ) would be zero. Our approach therefore implicitly compares the future climate-change scenario to this no-climate-change baseline scenario.
The time series of growth-rate impacts owing to future climate change in region r and year y , δ r , y , are then added to the future baseline growth rates, π r , y (in log-diff form), obtained from the SSP2 scenario to yield trajectories of damaged GRPpc growth rates, ρ r , y . These trajectories are aggregated over time to estimate the future trajectory of GRPpc with future climate impacts:
in which GRPpc r , y =2020 is the initial log level of GRPpc. We begin damage estimates in 2020 to reflect the damages occurring since the end of the period for which we estimate the empirical models (1979–2019) and to match the timing of mitigation-cost estimates from most IAMs (see below).
For each emission scenario, this procedure is repeated 1,000 times while randomly sampling from the selection of climate models, the selection of empirical models with different numbers of lags (shown in Supplementary Figs. 1 – 3 and Supplementary Tables 2 – 4 ) and bootstrapped estimates of the regression parameters. The result is an ensemble of future GRPpc trajectories that reflect uncertainty from both physical climate change and the structural and sampling uncertainty of the empirical models.
Estimates of mitigation costs
We obtain IPCC estimates of the aggregate costs of emission mitigation from the AR6 Scenario Explorer and Database hosted by IIASA 23 . Specifically, we search the AR6 Scenarios Database World v1.1 for IAMs that provided estimates of global GDP and population under both a SSP2 baseline and a SSP2-RCP2.6 scenario to maintain consistency with the socio-economic and emission scenarios of the climate damage projections. We find five IAMs that provide data for these scenarios, namely, MESSAGE-GLOBIOM 1.0, REMIND-MAgPIE 1.5, AIM/GCE 2.0, GCAM 4.2 and WITCH-GLOBIOM 3.1. Of these five IAMs, we use the results only from the first three that passed the IPCC vetting procedure for reproducing historical emission and climate trajectories. We then estimate global mitigation costs as the percentage difference in global per capita GDP between the SSP2 baseline and the SSP2-RCP2.6 emission scenario. In the case of one of these IAMs, estimates of mitigation costs begin in 2020, whereas in the case of two others, mitigation costs begin in 2010. The mitigation cost estimates before 2020 in these two IAMs are mostly negligible, and our choice to begin comparison with damage estimates in 2020 is conservative with respect to the relative weight of climate damages compared with mitigation costs for these two IAMs.
Data availability
Data on economic production and ERA-5 climate data are publicly available at https://doi.org/10.5281/zenodo.4681306 (ref. 62 ) and https://www.ecmwf.int/en/forecasts/datasets/reanalysis-datasets/era5 , respectively. Data on mitigation costs are publicly available at https://data.ene.iiasa.ac.at/ar6/#/downloads . Processed climate and economic data, as well as all other necessary data for reproduction of the results, are available at the public repository https://doi.org/10.5281/zenodo.10562951 (ref. 63 ).
Code availability
All code necessary for reproduction of the results is available at the public repository https://doi.org/10.5281/zenodo.10562951 (ref. 63 ).
Glanemann, N., Willner, S. N. & Levermann, A. Paris Climate Agreement passes the cost-benefit test. Nat. Commun. 11 , 110 (2020).
Article ADS CAS PubMed PubMed Central Google Scholar
Burke, M., Hsiang, S. M. & Miguel, E. Global non-linear effect of temperature on economic production. Nature 527 , 235–239 (2015).
Article ADS CAS PubMed Google Scholar
Kalkuhl, M. & Wenz, L. The impact of climate conditions on economic production. Evidence from a global panel of regions. J. Environ. Econ. Manag. 103 , 102360 (2020).
Article Google Scholar
Moore, F. C. & Diaz, D. B. Temperature impacts on economic growth warrant stringent mitigation policy. Nat. Clim. Change 5 , 127–131 (2015).
Article ADS Google Scholar
Drouet, L., Bosetti, V. & Tavoni, M. Net economic benefits of well-below 2°C scenarios and associated uncertainties. Oxf. Open Clim. Change 2 , kgac003 (2022).
Ueckerdt, F. et al. The economically optimal warming limit of the planet. Earth Syst. Dyn. 10 , 741–763 (2019).
Kotz, M., Wenz, L., Stechemesser, A., Kalkuhl, M. & Levermann, A. Day-to-day temperature variability reduces economic growth. Nat. Clim. Change 11 , 319–325 (2021).
Kotz, M., Levermann, A. & Wenz, L. The effect of rainfall changes on economic production. Nature 601 , 223–227 (2022).
Kousky, C. Informing climate adaptation: a review of the economic costs of natural disasters. Energy Econ. 46 , 576–592 (2014).
Harlan, S. L. et al. in Climate Change and Society: Sociological Perspectives (eds Dunlap, R. E. & Brulle, R. J.) 127–163 (Oxford Univ. Press, 2015).
Bolton, P. et al. The Green Swan (BIS Books, 2020).
Alogoskoufis, S. et al. ECB Economy-wide Climate Stress Test: Methodology and Results European Central Bank, 2021).
Weber, E. U. What shapes perceptions of climate change? Wiley Interdiscip. Rev. Clim. Change 1 , 332–342 (2010).
Markowitz, E. M. & Shariff, A. F. Climate change and moral judgement. Nat. Clim. Change 2 , 243–247 (2012).
Riahi, K. et al. The shared socioeconomic pathways and their energy, land use, and greenhouse gas emissions implications: an overview. Glob. Environ. Change 42 , 153–168 (2017).
Auffhammer, M., Hsiang, S. M., Schlenker, W. & Sobel, A. Using weather data and climate model output in economic analyses of climate change. Rev. Environ. Econ. Policy 7 , 181–198 (2013).
Kolstad, C. D. & Moore, F. C. Estimating the economic impacts of climate change using weather observations. Rev. Environ. Econ. Policy 14 , 1–24 (2020).
Dell, M., Jones, B. F. & Olken, B. A. Temperature shocks and economic growth: evidence from the last half century. Am. Econ. J. Macroecon. 4 , 66–95 (2012).
Newell, R. G., Prest, B. C. & Sexton, S. E. The GDP-temperature relationship: implications for climate change damages. J. Environ. Econ. Manag. 108 , 102445 (2021).
Kikstra, J. S. et al. The social cost of carbon dioxide under climate-economy feedbacks and temperature variability. Environ. Res. Lett. 16 , 094037 (2021).
Article ADS CAS Google Scholar
Bastien-Olvera, B. & Moore, F. Persistent effect of temperature on GDP identified from lower frequency temperature variability. Environ. Res. Lett. 17 , 084038 (2022).
Eyring, V. et al. Overview of the Coupled Model Intercomparison Project Phase 6 (CMIP6) experimental design and organization. Geosci. Model Dev. 9 , 1937–1958 (2016).
Byers, E. et al. AR6 scenarios database. Zenodo https://zenodo.org/records/7197970 (2022).
Burke, M., Davis, W. M. & Diffenbaugh, N. S. Large potential reduction in economic damages under UN mitigation targets. Nature 557 , 549–553 (2018).
Kotz, M., Wenz, L. & Levermann, A. Footprint of greenhouse forcing in daily temperature variability. Proc. Natl Acad. Sci. 118 , e2103294118 (2021).
Article CAS PubMed PubMed Central Google Scholar
Myhre, G. et al. Frequency of extreme precipitation increases extensively with event rareness under global warming. Sci. Rep. 9 , 16063 (2019).
Min, S.-K., Zhang, X., Zwiers, F. W. & Hegerl, G. C. Human contribution to more-intense precipitation extremes. Nature 470 , 378–381 (2011).
England, M. R., Eisenman, I., Lutsko, N. J. & Wagner, T. J. The recent emergence of Arctic Amplification. Geophys. Res. Lett. 48 , e2021GL094086 (2021).
Fischer, E. M. & Knutti, R. Anthropogenic contribution to global occurrence of heavy-precipitation and high-temperature extremes. Nat. Clim. Change 5 , 560–564 (2015).
Pfahl, S., O’Gorman, P. A. & Fischer, E. M. Understanding the regional pattern of projected future changes in extreme precipitation. Nat. Clim. Change 7 , 423–427 (2017).
Callahan, C. W. & Mankin, J. S. Globally unequal effect of extreme heat on economic growth. Sci. Adv. 8 , eadd3726 (2022).
Diffenbaugh, N. S. & Burke, M. Global warming has increased global economic inequality. Proc. Natl Acad. Sci. 116 , 9808–9813 (2019).
Callahan, C. W. & Mankin, J. S. National attribution of historical climate damages. Clim. Change 172 , 40 (2022).
Burke, M. & Tanutama, V. Climatic constraints on aggregate economic output. National Bureau of Economic Research, Working Paper 25779. https://doi.org/10.3386/w25779 (2019).
Kahn, M. E. et al. Long-term macroeconomic effects of climate change: a cross-country analysis. Energy Econ. 104 , 105624 (2021).
Desmet, K. et al. Evaluating the economic cost of coastal flooding. National Bureau of Economic Research, Working Paper 24918. https://doi.org/10.3386/w24918 (2018).
Hsiang, S. M. & Jina, A. S. The causal effect of environmental catastrophe on long-run economic growth: evidence from 6,700 cyclones. National Bureau of Economic Research, Working Paper 20352. https://doi.org/10.3386/w2035 (2014).
Ritchie, P. D. et al. Shifts in national land use and food production in Great Britain after a climate tipping point. Nat. Food 1 , 76–83 (2020).
Dietz, S., Rising, J., Stoerk, T. & Wagner, G. Economic impacts of tipping points in the climate system. Proc. Natl Acad. Sci. 118 , e2103081118 (2021).
Bastien-Olvera, B. A. & Moore, F. C. Use and non-use value of nature and the social cost of carbon. Nat. Sustain. 4 , 101–108 (2021).
Carleton, T. et al. Valuing the global mortality consequences of climate change accounting for adaptation costs and benefits. Q. J. Econ. 137 , 2037–2105 (2022).
Bastien-Olvera, B. A. et al. Unequal climate impacts on global values of natural capital. Nature 625 , 722–727 (2024).
Malik, A. et al. Impacts of climate change and extreme weather on food supply chains cascade across sectors and regions in Australia. Nat. Food 3 , 631–643 (2022).
Article ADS PubMed Google Scholar
Kuhla, K., Willner, S. N., Otto, C., Geiger, T. & Levermann, A. Ripple resonance amplifies economic welfare loss from weather extremes. Environ. Res. Lett. 16 , 114010 (2021).
Schleypen, J. R., Mistry, M. N., Saeed, F. & Dasgupta, S. Sharing the burden: quantifying climate change spillovers in the European Union under the Paris Agreement. Spat. Econ. Anal. 17 , 67–82 (2022).
Dasgupta, S., Bosello, F., De Cian, E. & Mistry, M. Global temperature effects on economic activity and equity: a spatial analysis. European Institute on Economics and the Environment, Working Paper 22-1 (2022).
Neal, T. The importance of external weather effects in projecting the macroeconomic impacts of climate change. UNSW Economics Working Paper 2023-09 (2023).
Deryugina, T. & Hsiang, S. M. Does the environment still matter? Daily temperature and income in the United States. National Bureau of Economic Research, Working Paper 20750. https://doi.org/10.3386/w20750 (2014).
Hersbach, H. et al. The ERA5 global reanalysis. Q. J. R. Meteorol. Soc. 146 , 1999–2049 (2020).
Cucchi, M. et al. WFDE5: bias-adjusted ERA5 reanalysis data for impact studies. Earth Syst. Sci. Data 12 , 2097–2120 (2020).
Adler, R. et al. The New Version 2.3 of the Global Precipitation Climatology Project (GPCP) Monthly Analysis Product 1072–1084 (University of Maryland, 2016).
Lange, S. Trend-preserving bias adjustment and statistical downscaling with ISIMIP3BASD (v1.0). Geosci. Model Dev. 12 , 3055–3070 (2019).
Wenz, L., Carr, R. D., Kögel, N., Kotz, M. & Kalkuhl, M. DOSE – global data set of reported sub-national economic output. Sci. Data 10 , 425 (2023).
Article PubMed PubMed Central Google Scholar
Gennaioli, N., La Porta, R., Lopez De Silanes, F. & Shleifer, A. Growth in regions. J. Econ. Growth 19 , 259–309 (2014).
Board of Governors of the Federal Reserve System (US). U.S. dollars to euro spot exchange rate. https://fred.stlouisfed.org/series/AEXUSEU (2022).
World Bank. GDP deflator. https://data.worldbank.org/indicator/NY.GDP.DEFL.ZS (2022).
Jones, B. & O’Neill, B. C. Spatially explicit global population scenarios consistent with the Shared Socioeconomic Pathways. Environ. Res. Lett. 11 , 084003 (2016).
Murakami, D. & Yamagata, Y. Estimation of gridded population and GDP scenarios with spatially explicit statistical downscaling. Sustainability 11 , 2106 (2019).
Koch, J. & Leimbach, M. Update of SSP GDP projections: capturing recent changes in national accounting, PPP conversion and Covid 19 impacts. Ecol. Econ. 206 (2023).
Carleton, T. A. & Hsiang, S. M. Social and economic impacts of climate. Science 353 , aad9837 (2016).
Article PubMed Google Scholar
Bergé, L. Efficient estimation of maximum likelihood models with multiple fixed-effects: the R package FENmlm. DEM Discussion Paper Series 18-13 (2018).
Kalkuhl, M., Kotz, M. & Wenz, L. DOSE - The MCC-PIK Database Of Subnational Economic output. Zenodo https://zenodo.org/doi/10.5281/zenodo.4681305 (2021).
Kotz, M., Wenz, L. & Levermann, A. Data and code for “The economic commitment of climate change”. Zenodo https://zenodo.org/doi/10.5281/zenodo.10562951 (2024).
Dasgupta, S. et al. Effects of climate change on combined labour productivity and supply: an empirical, multi-model study. Lancet Planet. Health 5 , e455–e465 (2021).
Lobell, D. B. et al. The critical role of extreme heat for maize production in the United States. Nat. Clim. Change 3 , 497–501 (2013).
Zhao, C. et al. Temperature increase reduces global yields of major crops in four independent estimates. Proc. Natl Acad. Sci. 114 , 9326–9331 (2017).
Wheeler, T. R., Craufurd, P. Q., Ellis, R. H., Porter, J. R. & Prasad, P. V. Temperature variability and the yield of annual crops. Agric. Ecosyst. Environ. 82 , 159–167 (2000).
Rowhani, P., Lobell, D. B., Linderman, M. & Ramankutty, N. Climate variability and crop production in Tanzania. Agric. For. Meteorol. 151 , 449–460 (2011).
Ceglar, A., Toreti, A., Lecerf, R., Van der Velde, M. & Dentener, F. Impact of meteorological drivers on regional inter-annual crop yield variability in France. Agric. For. Meteorol. 216 , 58–67 (2016).
Shi, L., Kloog, I., Zanobetti, A., Liu, P. & Schwartz, J. D. Impacts of temperature and its variability on mortality in New England. Nat. Clim. Change 5 , 988–991 (2015).
Xue, T., Zhu, T., Zheng, Y. & Zhang, Q. Declines in mental health associated with air pollution and temperature variability in China. Nat. Commun. 10 , 2165 (2019).
Article ADS PubMed PubMed Central Google Scholar
Liang, X.-Z. et al. Determining climate effects on US total agricultural productivity. Proc. Natl Acad. Sci. 114 , E2285–E2292 (2017).
Desbureaux, S. & Rodella, A.-S. Drought in the city: the economic impact of water scarcity in Latin American metropolitan areas. World Dev. 114 , 13–27 (2019).
Damania, R. The economics of water scarcity and variability. Oxf. Rev. Econ. Policy 36 , 24–44 (2020).
Davenport, F. V., Burke, M. & Diffenbaugh, N. S. Contribution of historical precipitation change to US flood damages. Proc. Natl Acad. Sci. 118 , e2017524118 (2021).
Dave, R., Subramanian, S. S. & Bhatia, U. Extreme precipitation induced concurrent events trigger prolonged disruptions in regional road networks. Environ. Res. Lett. 16 , 104050 (2021).
Download references
Acknowledgements
We gratefully acknowledge financing from the Volkswagen Foundation and the Deutsche Gesellschaft für Internationale Zusammenarbeit (GIZ) GmbH on behalf of the Government of the Federal Republic of Germany and Federal Ministry for Economic Cooperation and Development (BMZ).
Open access funding provided by Potsdam-Institut für Klimafolgenforschung (PIK) e.V.
Author information
Authors and affiliations.
Research Domain IV, Research Domain IV, Potsdam Institute for Climate Impact Research, Potsdam, Germany
Maximilian Kotz, Anders Levermann & Leonie Wenz
Institute of Physics, Potsdam University, Potsdam, Germany
Maximilian Kotz & Anders Levermann
Mercator Research Institute on Global Commons and Climate Change, Berlin, Germany
Leonie Wenz
You can also search for this author in PubMed Google Scholar
Contributions
All authors contributed to the design of the analysis. M.K. conducted the analysis and produced the figures. All authors contributed to the interpretation and presentation of the results. M.K. and L.W. wrote the manuscript.
Corresponding author
Correspondence to Leonie Wenz .
Ethics declarations
Competing interests.
The authors declare no competing interests.
Peer review
Peer review information.
Nature thanks Xin-Zhong Liang, Chad Thackeray and the other, anonymous, reviewer(s) for their contribution to the peer review of this work. Peer reviewer reports are available.
Additional information
Publisher’s note Springer Nature remains neutral with regard to jurisdictional claims in published maps and institutional affiliations.
Extended data figures and tables
Extended data fig. 1 constraining the persistence of historical climate impacts on economic growth rates..
The results of a panel-based fixed-effects distributed lag model for the effects of annual mean temperature ( a ), daily temperature variability ( b ), total annual precipitation ( c ), the number of wet days ( d ) and extreme daily precipitation ( e ) on sub-national economic growth rates. Point estimates show the effects of a 1 °C or one standard deviation increase (for temperature and precipitation variables, respectively) at the lower quartile, median and upper quartile of the relevant moderating variable (green, orange and purple, respectively) at different lagged periods after the initial shock (note that these are not cumulative effects). Climate variables are used in their first-differenced form (see main text for discussion) and the moderating climate variables are the annual mean temperature, seasonal temperature difference, total annual precipitation, number of wet days and annual mean temperature, respectively, in panels a – e (see Methods for further discussion). Error bars show the 95% confidence intervals having clustered standard errors by region. The within-region R 2 , Bayesian and Akaike information criteria for the model are shown at the top of the figure. This figure shows results with ten lags for each variable to demonstrate the observed levels of persistence, but our preferred specifications remove later lags based on the statistical significance of terms shown above and the information criteria shown in Extended Data Fig. 2 . The resulting models without later lags are shown in Supplementary Figs. 1 – 3 .
Extended Data Fig. 2 Incremental lag-selection procedure using information criteria and within-region R 2 .
Starting from a panel-based fixed-effects distributed lag model estimating the effects of climate on economic growth using the real historical data (as in equation ( 4 )) with ten lags for all climate variables (as shown in Extended Data Fig. 1 ), lags are incrementally removed for one climate variable at a time. The resulting Bayesian and Akaike information criteria are shown in a – e and f – j , respectively, and the within-region R 2 and number of observations in k – o and p – t , respectively. Different rows show the results when removing lags from different climate variables, ordered from top to bottom as annual mean temperature, daily temperature variability, total annual precipitation, the number of wet days and extreme annual precipitation. Information criteria show minima at approximately four lags for precipitation variables and ten to eight for temperature variables, indicating that including these numbers of lags does not lead to overfitting. See Supplementary Table 1 for an assessment using information criteria to determine whether including further climate variables causes overfitting.
Extended Data Fig. 3 Damages in our preferred specification that provides a robust lower bound on the persistence of climate impacts on economic growth versus damages in specifications of pure growth or pure level effects.
Estimates of future damages as shown in Fig. 1 but under the emission scenario RCP8.5 for three separate empirical specifications: in orange our preferred specification, which provides an empirical lower bound on the persistence of climate impacts on economic growth rates while avoiding assumptions of infinite persistence (see main text for further discussion); in purple a specification of ‘pure growth effects’ in which the first difference of climate variables is not taken and no lagged climate variables are included (the baseline specification of ref. 2 ); and in pink a specification of ‘pure level effects’ in which the first difference of climate variables is taken but no lagged terms are included.
Extended Data Fig. 4 Climate changes in different variables as a function of historical interannual variability.
Changes in each climate variable of interest from 1979–2019 to 2035–2065 under the high-emission scenario SSP5-RCP8.5, expressed as a percentage of the historical variability of each measure. Historical variability is estimated as the standard deviation of each detrended climate variable over the period 1979–2019 during which the empirical models were identified (detrending is appropriate because of the inclusion of region-specific linear time trends in the empirical models). See Supplementary Fig. 13 for changes expressed in standard units. Data on national administrative boundaries are obtained from the GADM database version 3.6 and are freely available for academic use ( https://gadm.org/ ).
Extended Data Fig. 5 Contribution of different climate variables to overall committed damages.
a , Climate damages in 2049 when using empirical models that account for all climate variables, changes in annual mean temperature only or changes in both annual mean temperature and one other climate variable (daily temperature variability, total annual precipitation, the number of wet days and extreme daily precipitation, respectively). b , The cumulative marginal effects of an increase in annual mean temperature of 1 °C, at different baseline temperatures, estimated from empirical models including all climate variables or annual mean temperature only. Estimates and uncertainty bars represent the median and 95% confidence intervals obtained from 1,000 block-bootstrap resamples from each of three different empirical models using eight, nine or ten lags of temperature terms.
Extended Data Fig. 6 The difference in committed damages between the upper and lower quartiles of countries when ranked by GDP and cumulative historical emissions.
Quartiles are defined using a population weighting, as are the average committed damages across each quartile group. The violin plots indicate the distribution of differences between quartiles across the two extreme emission scenarios (RCP2.6 and RCP8.5) and the uncertainty sampling procedure outlined in Methods , which accounts for uncertainty arising from the choice of lags in the empirical models, uncertainty in the empirical model parameter estimates, as well as the climate model projections. Bars indicate the median, as well as the 10th and 90th percentiles and upper and lower sixths of the distribution reflecting the very likely and likely ranges following the likelihood classification adopted by the IPCC.
Supplementary information
Supplementary information, peer review file, rights and permissions.
Open Access This article is licensed under a Creative Commons Attribution 4.0 International License, which permits use, sharing, adaptation, distribution and reproduction in any medium or format, as long as you give appropriate credit to the original author(s) and the source, provide a link to the Creative Commons licence, and indicate if changes were made. The images or other third party material in this article are included in the article’s Creative Commons licence, unless indicated otherwise in a credit line to the material. If material is not included in the article’s Creative Commons licence and your intended use is not permitted by statutory regulation or exceeds the permitted use, you will need to obtain permission directly from the copyright holder. To view a copy of this licence, visit http://creativecommons.org/licenses/by/4.0/ .
Reprints and permissions
About this article
Cite this article.
Kotz, M., Levermann, A. & Wenz, L. The economic commitment of climate change. Nature 628 , 551–557 (2024). https://doi.org/10.1038/s41586-024-07219-0
Download citation
Received : 25 January 2023
Accepted : 21 February 2024
Published : 17 April 2024
Issue Date : 18 April 2024
DOI : https://doi.org/10.1038/s41586-024-07219-0
Share this article
Anyone you share the following link with will be able to read this content:
Sorry, a shareable link is not currently available for this article.
Provided by the Springer Nature SharedIt content-sharing initiative
By submitting a comment you agree to abide by our Terms and Community Guidelines . If you find something abusive or that does not comply with our terms or guidelines please flag it as inappropriate.
Quick links
- Explore articles by subject
- Guide to authors
- Editorial policies
Sign up for the Nature Briefing newsletter — what matters in science, free to your inbox daily.


IMAGES
VIDEO
COMMENTS
The present volume contributes an update on and a systematic critical appraisal of the methods employed in study abroad research to identify strengths and weaknesses and to look ahead and point towards new directions. ... Methods in Study Abroad Research Past, present, and future. Editors. Carmen Pérez-Vidal ...
Study abroad research has become an established area of inquiry with theoretical impact and methodological sophistication. The field has incorporated the different approaches and methodological changes that have characterized SLA scholarship, including technological advances and new designs.
Study abroad research has become an established area of inquiry with theoretical impact and methodological sophistication. The field has incorporated the different approaches and methodological changes that have characterized SLA scholarship, including technological advances and new designs. The present volume contributes an update on and a systematic critical appraisal of the methods employed ...
Affiliations: 1: Universitat Pompeu Fabra; 2: Georgetown University. The chapter summarizes the evolution of SA research from the 90s to the present, a field that has become an established area of inquiry with theoretical impact and methodological sophistication. SA research has incorporated the different approaches and methodological changes ...
Buy Methods in Study Abroad Research: Past, Present, and Future by Carmen P?rez-Vidal (Editor), Cristina Sanz (Editor) online at Alibris. We have new and used copies available, in 2 editions - starting at $63.00. ... All Editions of Methods in Study Abroad Research: Past, Present, and Future . 2023, Trade paperback. ISBN-13: 9789027212856. 2023 ...
Buy Methods in Study Abroad Research: Past, present, and future: 4 (Research Methods in Applied Linguistics) by Pérez-Vidal, Carmen, Sanz, Cristina (ISBN: 9789027212856) from Amazon's Book Store. Everyday low prices and free delivery on eligible orders.
Methods in Study Abroad Research: Past Present and Future. Amsterdam, Netherlands: John Benjamins, 2020. | Find, read and cite all the research you need on ResearchGate Home
Methods in Study Abroad Research: Past, present, and future - Ebook written by Carmen Pérez-Vidal, Cristina Sanz. Read this book using Google Play Books app on your PC, android, iOS devices. Download for offline reading, highlight, bookmark or take notes while you read Methods in Study Abroad Research: Past, present, and future.
Buy Methods in Study Abroad Research: Past, present, and future by Pérez-Vidal, Carmen, Sanz, Cristina online on Amazon.ae at best prices. Fast and free shipping free returns cash on delivery available on eligible purchase.
Methods in Study Abroad Research: Past, present, and future: 4 : Pérez-Vidal, Carmen, Sanz, Cristina: Amazon.com.au: Books
Amazon.in - Buy Methods in Study Abroad Research: Past, present, and future: 4 (Research Methods in Applied Linguistics) book online at best prices in India on Amazon.in. Read Methods in Study Abroad Research: Past, present, and future: 4 (Research Methods in Applied Linguistics) book reviews & author details and more at Amazon.in. Free delivery on qualified orders.
Study abroad research has become an established area of inquiry with theoretical impact and methodological sophistication. The field has incorporated the different approaches and methodological changes that have characterized SLA scholarship, including technological advances and new designs. The...
Introduction. Over the past two decades, there has been a steady shift in study abroad programming away from year- and semester-long sojourns toward short-term study abroad (STSA), generally defined as programs lasting eight weeks or less (Chieffo and Griffiths, 2004, Donnelly-Smith, 2009).This trend can be seen in sending institutions across the globe (e.g., Erasmus, 2021; Institute of ...
She is co-editing a volume on methods in study abroad research for John Benjamins and has co-edited the Routledge Handbook of Study Abroad Research and ... (Eds.), Methods in Study Abroad Research: Past, Present and Future. Amsterdam: John Benjamins. Allen, H. W. (2010). Language-learning motivation during short-term study abroad: An activity ...
Methods in Study Abroad Research: Past, present, and future - Carmen Pérez-Vidal, Cristina Sanz - ISBN: 9789027212863. Study abroad research has become an established area of inquiry with theoretical impact and methodological sophistication. The field has incorporated the different approaches and methodological changes that have characterized SLA scholarship, including technological advances ...
(1) Research question. A research question typically guides a study (Bryman, 1992; Patton, 1990).Methodological fit is often assessed in terms of the research question, which in turn is likely to be influenced by the goal of the research, the background, skills and expertise of the researcher, interests of the stakeholders, and available time, data and other resources (Brannen, 2005; Flood ...
Qin et al demonstrated that 57 bacterial species were present in >90% and 75 species in > 50% of the individuals in their study (3, 63). But, despite these interindividual similarities, the idea of a universal healthy gut microbiome or a core microbiome is likely too simplistic in light of the interindividual differences observed in many other ...
The purpose of the chapter is to survey the diverse range of approaches to researching L2 (second language) motivation, with reference to particular types of study and particular research traditions. The chapter does not provide a practical "how to" guide for specific methods of inquiry, but aims instead to offer a critical analysis of ...
to shed light on the past, present and future of research methodology in. this field. 3. Method: systematic review ... quently adopt a case study method (in 116 articles, 53% of the papers ...
Methods in microbiome research: Past, present, and future Best Pract Res Clin Rheumatol. 2019 Dec;33(6):101498. doi: 10.1016/j.berh.2020.101498. Epub 2020 Apr 24. ... In this chapter, we will be discussing about methods used to study the microbiome, both old and new methods, fundamental concepts that have taken hold within the field, and how ...
Face recognition is one of the most active research fields of computer vision and pattern recognition, with many practical and commercial applications including identification, access control, forensics, and human-computer interactions. However, identifying a face in a crowd raises serious questions about individual freedoms and poses ethical issues. Significant methods, algorithms, approaches ...
Analysis of projected sub-national damages from temperature and precipitation show an income reduction of 19% of the world economy within the next 26 years independent of future emission choices.