Identifiers
Linking ISSN (ISSN-L): 2754-4990
URL https://www.onlinescientificresearch.com/journal-of-ophthalmology-research-reviews-reports-home-jomrr.php
Google https://www.google.com/search?q=ISSN+%222754-4990%22
Bing https://www.bing.com/search?q=ISSN+%222754-4990%22
Yahoo https://search.yahoo.com/search?p=ISSN%20%222754-4990%22
British Library https://explore.bl.uk/primo_library/libweb/action/search.do?fn=search&ct=search&initialSearch=true&mode=Advanced&tab=local_tab&indx=1&tb=t&vl%281UIStartWith0%29=contains&vl%28freeText0%29=2754-4990&SUBMIT

Resource information
Title proper: Journal of ophthalmology research reviews & reports.
Country: United Kingdom
Medium: Online
Record information
Last modification date: 02/12/2021
Type of record: Confirmed
ISSN Center responsible of the record: ISSN National Centre for the UK
downloads requested
Discover all the features of the complete ISSN records
Display mode x.
Labelled view
MARC21 view
UNIMARC view

Journal of ophthalmology research reviews & reports
Trace this journal
Citation Count
2 citations
1 citations
Performance

An official website of the United States government
The .gov means it’s official. Federal government websites often end in .gov or .mil. Before sharing sensitive information, make sure you’re on a federal government site.
The site is secure. The https:// ensures that you are connecting to the official website and that any information you provide is encrypted and transmitted securely.
- Publications
- Account settings
Preview improvements coming to the PMC website in October 2024. Learn More or Try it out now .
- Advanced Search
- Journal List
- Front Med (Lausanne)
Hotspots and trends in ophthalmology in recent 5 years: Bibliometric analysis in 2017–2021
1 State Key Laboratory of Ophthalmology, Zhongshan Ophthalmic Center, Sun Yat-sen University, Guangzhou, China
2 Guangdong Provincial Key Laboratory of Ophthalmology and Visual Science, Guangzhou, China
3 Guangdong Provincial Clinical Research Center for Ocular Diseases, Guangzhou, China
Weining Zhu
4 Zhongshan Medical School, Sun Yat-sen University, Guangzhou, China
Yingshi Zou
Bowen zhang, guangming jin, zhenzhen liu, associated data.
Publicly available datasets were analyzed in this study. This data can be found here: https://www.webofscience.com/wos/alldb/basic-search .
The purpose of this study was to investigate the hotspots and research trends of ophthalmology research.
Ophthalmology research literature published between 2017 and 2021 was obtained in the Web of Science Core Collection database. The bibliometric analysis and network visualization were performed with the VOSviewer and CiteSpace. Publication-related information, including publication volume, citation counts, countries, journals, keywords, subject categories, and publication time, was analyzed.
A total of 10,469 included ophthalmology publications had been cited a total of 7,995 times during the past 5 years. The top countries and journals for the number of publications were the United States and the Ophthalmology. The top 25 global high-impact documents had been identified using the citation ranking. Keyword co-occurrence analysis showed that the hotspots in ophthalmology research were epidemiological characteristics and treatment modalities of ocular diseases, artificial intelligence and fundus imaging technology, COVID-19-related telemedicine, and screening and prevention of ocular diseases. Keyword burst analysis revealed that “neural network,” “pharmacokinetics,” “geographic atrophy,” “implementation,” “variability,” “adverse events,” “automated detection,” and “retinal images” were the research trends of research in the field of ophthalmology through 2021. The analysis of the subject categories demonstrated the close cooperation relationships that existed between different subject categories, and collaborations with non-ophthalmology-related subject categories were increasing over time in the field of ophthalmology research.
Conclusions
The hotspots in ophthalmology research were epidemiology, prevention, screening, and treatment of ocular diseases, as well as artificial intelligence and fundus imaging technology and telemedicine. Research trends in ophthalmology research were artificial intelligence, drug development, and fundus diseases. Knowledge from non-ophthalmology fields is likely to be more involved in ophthalmology research.
Introduction
More than 2.2 billion people worldwide were visually impaired or blind to date, with an annual economic burden of more than $269.4 billion ( 1 ). Development in ophthalmology is essential for the prevention and treatment of eye diseases, and relevant research is growing rapidly in breadth and depth and forming complex knowledge networks. Glaucoma, age-related macular degeneration, and some hereditary eye diseases were previously considered irreversible blindness-causing diseases, and progress had been made to cure or alleviate them by modulating new targets or using new technologies ( 2 – 4 ). Cataracts and posterior capsular opacification were previously thought to be treated only with surgery, but in the recent years, there had been new developments in research into drugs that inhibit cataract formation ( 5 , 6 ). With the advances in the field of ophthalmology, new hope has emerged in areas previously considered untreatable or treatable only through non-pharmaceutical interventions ( 7 – 10 ). However, it is not feasible to analyze the overall overview of the field of ophthalmology and to explore its research hotspots and trends with a traditional systematic review, which is not conducive to the development of the field.
Bibliometric analysis is the quantitative analysis of the universal scientific production data in a specific field ( 11 ). Bibliometric method obtains the history and current status of the research field development by analyzing the scientific research results and can make predictions of the research field ( 12 ). Previous studies have conducted bibliometric analysis on individual country contributions or focused only on randomized controlled studies in ophthalmology and citation patterns in ophthalmology journals ( 13 – 19 ). Unsolved questions still remain as to how to quantitatively evaluate the contribution of different global research forces (countries, journals) in ophthalmology and identify hotspots and future research trends in ophthalmology based on a wide range of research results in different subfields of ophthalmology.
This study was intended to quantitatively analyze and visualize the global ophthalmology publication from 2017 to 2021 using bibliometric methods to explore the global research forces (countries, journals), possible hotspots, and future trends of ophthalmology research and to provide insight for research development and public health policy formulation in the field of ophthalmology.
Data sources
All the data used in this study were obtained from the Web of Science Core Collection (Clarivate Analytics, Philadelphia, PA, USA). The search was conducted by searching the Topic Subject retrieval field using “ophthalmology” as the subject word. Articles published between 2017 and 2021 were included, with no restrictions on the language type or document type of the articles. Data were collected on 28 January 2022.
Data collection and processing
To describe the number of articles published per year, the number of annual citations of the articles, the number of country publications, and the number of journal publications in the field of ophthalmology, relevant data were downloaded in the Web of Science Core Collection. All ophthalmology-related articles with their corresponding references and all publication-related information were exported as plain text for country collaboration analysis, keyword co-occurrence analysis, keyword burst analysis, and subject category co-occurrence analysis. To make the results more informative, keywords that were not relevant or meaningful to the analysis were filtered and removed during the data processing.
Statistical and bibliometric analysis
Statistical descriptions of the number of annual publications, the number of annual citations, the number of country publications, and the number of journal publications were performed using Microsoft Excel 2019 (Microsoft Corporation, Redmond, WA, USA) and GraphPad Prism version 8.4.2 (GraphPad Software, La Jolla, CA, USA).
Bibliometric analysis was carried out using VOSviewer (Leiden University's Centre for Science and Technology Studies, Leiden, the Netherlands) to obtain country collaborations and research hotspots. Several clusters were formed based on the country cooperation analysis, with countries of the same color belonging to the same cluster. Countries within clusters cooperated relatively closely, whereas cooperation among countries between clusters was relatively weak. The research hotspots were obtained from the clusters formed by the co-occurrence analysis of high-frequency keywords. The common characteristics of high-frequency keywords within the same cluster revealed the research hotspots. The frequency of keyword occurrences was used to weight the size of the keywords. The larger the keyword, the higher the frequency of occurrence.
Furthermore, CiteSpace V version 5.8.R3 (Drexel University, Philadelphia, PA, USA) was used for bibliometric analysis to obtain the burst keywords and subject category cooperation. The keyword burst analysis was performed to obtain temporal trends in keywords in the field of ophthalmology. The most recent burst keywords were defined as research frontier topics, indicating the potential for continued research breakthroughs in these topics. The co-occurrence of subject categories was analyzed to obtain the collaboration of subject categories. The number of occurrences of a subject category was used to weight the subject category. The more occurrences a subject category had, the larger it was. Temporal trends in subject category occurrences were represented by temporal rings of subject categories, the thickness of which represented the number of subject category occurrences in the corresponding year. Interdisciplinary cooperation was represented by the connecting line between subject categories. The thicker the connecting line, the closer the collaboration.
Global research output distribution
A total of 139 countries contributed to the publications related to ophthalmology research, with a total of 10,469 articles, which were cited 7,995 times. The number of publications had increased year by year, but there was an inflection point in citation counts. Citation counts increased year by year from 2017, reaching 2,650 citations in 2020, whereas citations in 2021 decreased compared to 2020 ( Figure 1A ). The analysis of countries showed that the United States had the highest number of publications, more than three to four times the number of other countries, followed by the United Kingdom, India, Germany, and China ( Figure 1B ). Country collaboration analysis yielded four clusters, with close cooperation between countries within each cluster ( Figure 1C ). Publications related to ophthalmology research were distributed in 1,876 journals, and the top 10 journals in terms of the number of articles published were the Ophthalmology ( n = 1,263, 12.06%), the Ophthalmology. Retina ( n = 580, 5.54%), the BMJ Case Reports ( n = 270, 2.58%), the Journal of Neuro-Ophthalmology: the official journal of the North American Neuro-Ophthalmology Society ( n = 260, 2.48%), the Investigative Ophthalmology & Visual Science ( n = 214, 2.04%), the Ophthalmology, Glaucoma ( n = 204, 1.95%), the Journal of Current Ophthalmology ( n = 200, 1.91%), the European Journal of Ophthalmology ( n = 191, 1.82%), the Indian Journal of Ophthalmology ( n = 173, 1.65%), and Journal of Cataract and Refractive Surgery ( n = 171, 1.63%) ( Figure 1D ).

Global distribution of research output. (A) Annual publications and citations of ophthalmology research from 2017 to 2021. (B) Top 10 countries in terms of total publications. (C) Country cooperation networks. (D) Top 10 journals by total publication volume of ophthalmology research in a 5-year period.
Global high-impact documents
The top 25 high-impact articles in ophthalmology published between 2017 and 2021, ranked by total citations, are shown in Table 1 . All the articles had been cited more than 150 times, with the highest number of citations being 419. Of these articles, 10 were published in 2017, 12 in 2018, one in 2019, and two in 2020. In total, 12 of these articles were published in the Ophthalmology and three in the Progress in Retinal and Eye Research. According to the type of publication, there were 16 original research articles and 9 review articles. The keywords involved in the articles are listed in Table 1 , including 5 articles each on OCT and deep learning, 4 articles each on diabetes and macular degeneration, and other related research topics such as glaucoma, artificial intelligence, and drugs.
Top 25 most cited documents published between 2017 and 2021.
Research hotspots
Keyword co-occurrence analysis demonstrated that the three most frequent of all keywords were “glaucoma” ( n = 395), “retina” ( n = 321), and “optical coherence tomography” ( n = 230). In the past 5 years, 157 high-frequency keywords in the field of ophthalmology were identified by setting the minimum frequency of keyword occurrence at 20 times. These keywords formed four clusters: the “glaucoma” cluster (red; 86 items), the “retina” cluster (green; 47 items), the “COVID-19” cluster (blue; 13 items), and the “screening” cluster (yellow; 8 items) ( Figure 2 ). After summarizing the keyword clusters, four research hotspots were identified: epidemiological characteristics and treatment modalities of diseases such as glaucoma and diabetic retinopathy, artificial intelligence and fundus imaging technology, COVID-19-related telemedicine, and screening and prevention of eye diseases.

Ophthalmology research hotspots analysis. The keywords formed four clusters, which were differentiated by color in the diagram, with the same color being the same cluster. The keyword size indicated the number of occurrences of the keyword, whereas the thickness and distance of the connecting lines between the keywords indicated the frequency of co-occurrence between the two keywords.
Research trends
Keyword burst analysis showed that “neural network,” “pharmacokinetics,” “geographic atrophy,” “implementation,” “variability,” “adverse events,” “automated detection,” and “retinal images” were the hot topics of research in the field of ophthalmology through 2021 and displayed the potential to become the research frontiers to achieve breakthroughs shortly ( Figure 3A ).

Ophthalmology research trends analysis. (A) Keyword burst analysis. The red line indicates the year in which the burst of the corresponding keyword began and ended. (B) Subject category analysis. The larger subject categories indicate their greater frequency and importance, and the distance between subject categories indicates how closely they collaborate. The lines between subject categories indicate the collaboration between the subject categories at either end, with the color of the different lines representing the collaboration time in the different subject categories and the thickness representing the degree of collaboration closeness. The color of the temporal rings represents the occurrence of that subject category in different years, the thicker the corresponding temporal rings, the more frequently it occurs, with the time scale at the bottom right.
In terms of subject categories, the top three subject categories with the highest volume of ophthalmology-related research publications were medicine general internal ( n = 1,138, 10.87%), clinical neurology ( n = 482, 4.604%), and surgery ( n = 368, 3.515%) ( Table 2 ). The subject categories of ophthalmology research were divided into two types: one was the traditional ophthalmology-related subject categories, such as medicine general internal, clinical neurology, and surgery, and the other one was the non-ophthalmology-related subject categories, such as engineering, computer science, and chemistry. The analysis of subject category collaboration relationships indicated that over time more collaborative relationships had emerged between non-ophthalmology-related subject categories ( Figure 3B ).
Subject categories in ophthalmology from 2017 to 2021.
Research in the field of ophthalmology showed a year-on-year increase in the number of articles published in the last 5 years, with the most published country being the United States and the most prolific journal being the Ophthalmology. The top 25 high-impact articles worldwide were cited more than 150 times per article. A total of four research hotspots were identified: epidemiological characteristics and treatment modalities of diseases such as glaucoma and diabetic retinopathy, artificial intelligence and fundus imaging technology, COVID-19-related telemedicine, and screening and prevention of eye diseases. Cross-talk between different non-ophthalmology subject categories was also an important trend in ophthalmology.
The annual publication volume, country distribution, and journal distribution of the ophthalmology research articles revealed a global overview of research output in the field of ophthalmology. The output of ophthalmology research showed an increasing trend in the last 5 years, suggesting that the socioeconomic input and scientific output of the subject area were also developing ( 20 ). The individual contributions of some countries to ophthalmology research were previously reported, but there were limitations on the overall evaluation of all countries' contributions to ophthalmology research and of country collaboration ( 13 – 17 ). This study showed that the predominant countries in ophthalmology research included the United States, the United Kingdom, and India, and countries such as Germany, China, and Australia also played an important role in the contribution. Several stable collaborative networks have been formed between countries, which can facilitate cross-border research data sharing and the globalization of scientific research. The top five most published journals showed that ophthalmology research was mainly focused on clinical ophthalmology (Ophthalmology, BMJ Case Reports), basic ophthalmology research (Investigative Ophthalmology and Visual Science) and neuro-ophthalmology (Journal of Neuro-Ophthalmology, Ophthalmology Retina).
The high-impact articles in ophthalmology indicated that researchers in the field of ophthalmology were primarily concerned with ophthalmological health or disease states, as well as ophthalmological technologies and applications. In terms of health or disease conditions, age-related macular degeneration ( 21 – 24 ), glaucomatous optic neuropathy ( 25 , 26 ), corneal blindness ( 27 ), and other blinding eye diseases occupied important research positions. Research directions such as screening for diabetic retinopathy ( 28 , 29 ), preventing myopia ( 30 ), optimizing visual outcomes, and controlling complications after IOL implantation following cataract surgery were dedicated to the active identification, management, and control of disease risk factors, making the eye disease controllable and manageable ( 31 , 32 ). In addition, researchers were also concerned with the management of Behcet's syndrome ( 33 ) and COVID-19 infection prevention in ophthalmology ( 34 ). In ophthalmology-related technologies, the frontiers were artificial intelligence algorithms ( 23 , 25 , 26 , 35 – 38 ), new pathways for drug delivery ( 39 , 40 ), and new materials for therapy ( 41 ). In ophthalmology-related applications, the pioneering applications were optical coherence tomography ( 23 , 24 , 35 , 42 – 44 ), stem cell therapy, and tissue repair ( 45 ).
After clustering the high-frequency keywords in the past 5 years, four research hotspots in the field of ophthalmology were obtained. First, the epidemiological characteristics and treatment modalities of diseases such as glaucoma and diabetic retinopathy were the hot topics of ophthalmology research. The emergence of these hot topics was consistent with the increasing prevalence of systemic chronic diseases such as diabetes in the last 5 years, and several studies have revealed associations and common biomarkers of ophthalmology and systemic diseases ( 46 – 49 ). More future work needs to further focus on the diagnosis and optimal treatment strategies for blinding diseases associated with systemic conditions ( 50 ). Moreover, deep learning algorithms that could rapidly and non-invasively identify pathological features of eye diseases joined ophthalmology research ( 23 ). Deep learning algorithms could classify age-related cataract types based on slit-lamp photographs, and fully automated AI-based screening systems had been approved for the use in diabetic retinopathy ( 37 , 51 ). Furthermore, the emergence of the COVID-19 pandemic brought about an increase in the length of patient visits due to disease control and health-related problems associated with COVID-19 infections, which had a dramatic impact on ophthalmology health care. On the one hand, the close contacts physicians need when attending to patients could increase the risk of cross-infection between patients or between health care workers and patients, resulting in infection control to be optimized in ophthalmology practice. On the other hand, the need for timely intervention for patients was driving the development of telemedicine during the pandemic ( 34 , 52 ). Finally, the development of diagnostic technology has driven ophthalmology research toward early screening and disease prevention.
The keywords that were still bursting until 2021 were research trends. The keywords “neural networks,” “pharmacokinetics,” “automated detection,” and “retinal images” in this part of the keyword list were consistent with the hot research directions obtained by keyword clustering. Other keywords that had burst to 2021 could be newly emerging keywords that had not yet had time to be highly cited, were hotspots for research in ophthalmology, and were likely to continue to be of interest for some times to come. Concerning the disciplinary analysis, the analysis of this study revealed that there was extensive cross-collaboration in various basic areas of non-ophthalmology-related research. Knowledge from non-ophthalmology fields is likely to be more involved in ophthalmology research.
Strengths of the study include a global view of research forces in ophthalmology from a wide range of the literature. Additional study strengths include the revealing of highly cited documents in ophthalmology that provide useful information for researchers. Outcome measures addressed the global research force contributions, research hotspots, and research trends of ophthalmology research, providing an in-depth study of the field of ophthalmology.
Only data from the Web of Science Core Collection database were included in this study, but the Web of Science Core Collection database, as a citation database, already contained comprehensive data on the articles and corresponding citations, which was sufficient for capturing the overall development of the scientific field. In addition, the results of the analysis by the visualization software may include some repetitive and meaningless information. We tried to identify some of the hot topics that were influencing ophthalmology research, so the raw data had been further filtered to remove irrelevant or meaningless words.
In conclusion, this study provided a comprehensive analysis of ophthalmology-related research based on the Web of Science Core Collection database. The hotspots in ophthalmology research were epidemiology, prevention, screening, and treatment of ocular diseases, as well as artificial intelligence and fundus imaging technology and telemedicine. Research trends in ophthalmology research were artificial intelligence, drug development, and fundus diseases. There was an extensive cross-talk of ophthalmology-related research in various basic areas. Knowledge from non-ophthalmology fields is likely to be more involved in ophthalmology research.
Data availability statement
Author contributions.
ZL and GJ designed the study and provided a critical review for the manuscript. YT and WZ wrote the manuscript. YT, WZ, YZ, BZ, YY, and WL collected and analyzed the data. All authors contributed to the article and approved the submitted version.
This study was supported by the National Natural Science Foundation of China (81873675), the Guangdong Basic and Applied Basic Research Foundation (2022A1515011181), the Teaching Reform Research Program of Sun Yat-sen University (JX3030604024), and the Youth Project of State Key Laboratory of Ophthalmology (2021QN02).
Conflict of interest
The authors declare that the research was conducted in the absence of any commercial or financial relationships that could be construed as a potential conflict of interest.
Publisher's note
All claims expressed in this article are solely those of the authors and do not necessarily represent those of their affiliated organizations, or those of the publisher, the editors and the reviewers. Any product that may be evaluated in this article, or claim that may be made by its manufacturer, is not guaranteed or endorsed by the publisher.
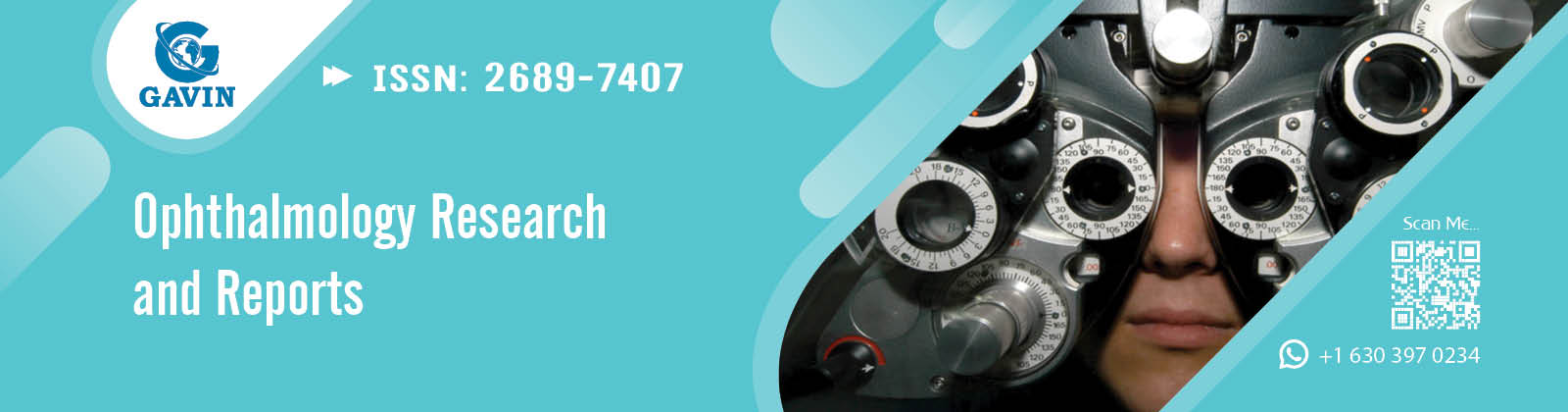
- About Journal
- Board Members
- Article In Press
- Current Issues
- Article Processing Fee
- Special Issues
Case Report

Chronic Deposits on Implantable Collamer Lens after Surgery
Xia Li, and Jinfeng Cai
- All subject areas
- Agricultural and Biological Sciences
- Arts and Humanities
- Biochemistry, Genetics and Molecular Biology
- Business, Management and Accounting
- Chemical Engineering
- Computer Science
- Decision Sciences
- Earth and Planetary Sciences
- Economics, Econometrics and Finance
- Engineering
- Environmental Science
- Health Professions
- Immunology and Microbiology
- Materials Science
- Mathematics
- Multidisciplinary
- Neuroscience
- Pharmacology, Toxicology and Pharmaceutics
- Physics and Astronomy
- Social Sciences
- All subject categories
- Acoustics and Ultrasonics
- Advanced and Specialized Nursing
- Aerospace Engineering
- Agricultural and Biological Sciences (miscellaneous)
- Agronomy and Crop Science
- Algebra and Number Theory
- Analytical Chemistry
- Anesthesiology and Pain Medicine
- Animal Science and Zoology
- Anthropology
- Applied Mathematics
- Applied Microbiology and Biotechnology
- Applied Psychology
- Aquatic Science
- Archeology (arts and humanities)
- Architecture
- Artificial Intelligence
- Arts and Humanities (miscellaneous)
- Assessment and Diagnosis
- Astronomy and Astrophysics
- Atmospheric Science
- Atomic and Molecular Physics, and Optics
- Automotive Engineering
- Behavioral Neuroscience
- Biochemistry
- Biochemistry, Genetics and Molecular Biology (miscellaneous)
- Biochemistry (medical)
- Bioengineering
- Biological Psychiatry
- Biomaterials
- Biomedical Engineering
- Biotechnology
- Building and Construction
- Business and International Management
- Business, Management and Accounting (miscellaneous)
- Cancer Research
- Cardiology and Cardiovascular Medicine
- Care Planning
- Cell Biology
- Cellular and Molecular Neuroscience
- Ceramics and Composites
- Chemical Engineering (miscellaneous)
- Chemical Health and Safety
- Chemistry (miscellaneous)
- Chiropractics
- Civil and Structural Engineering
- Clinical Biochemistry
- Clinical Psychology
- Cognitive Neuroscience
- Colloid and Surface Chemistry
- Communication
- Community and Home Care
- Complementary and Alternative Medicine
- Complementary and Manual Therapy
- Computational Mathematics
- Computational Mechanics
- Computational Theory and Mathematics
- Computer Graphics and Computer-Aided Design
- Computer Networks and Communications
- Computer Science Applications
- Computer Science (miscellaneous)
- Computer Vision and Pattern Recognition
- Computers in Earth Sciences
- Condensed Matter Physics
- Conservation
- Control and Optimization
- Control and Systems Engineering
- Critical Care and Intensive Care Medicine
- Critical Care Nursing
- Cultural Studies
- Decision Sciences (miscellaneous)
- Dental Assisting
- Dental Hygiene
- Dentistry (miscellaneous)
- Dermatology
- Development
- Developmental and Educational Psychology
- Developmental Biology
- Developmental Neuroscience
- Discrete Mathematics and Combinatorics
- Drug Discovery
- Drug Guides
- Earth and Planetary Sciences (miscellaneous)
- Earth-Surface Processes
- Ecological Modeling
- Ecology, Evolution, Behavior and Systematics
- Economic Geology
- Economics and Econometrics
- Economics, Econometrics and Finance (miscellaneous)
- Electrical and Electronic Engineering
- Electrochemistry
- Electronic, Optical and Magnetic Materials
- Emergency Medical Services
- Emergency Medicine
- Emergency Nursing
- Endocrine and Autonomic Systems
- Endocrinology
- Endocrinology, Diabetes and Metabolism
- Energy Engineering and Power Technology
- Energy (miscellaneous)
- Engineering (miscellaneous)
- Environmental Chemistry
- Environmental Engineering
- Environmental Science (miscellaneous)
- Epidemiology
- Experimental and Cognitive Psychology
- Family Practice
- Filtration and Separation
- Fluid Flow and Transfer Processes
- Food Animals
- Food Science
- Fuel Technology
- Fundamentals and Skills
- Gastroenterology
- Gender Studies
- Genetics (clinical)
- Geochemistry and Petrology
- Geography, Planning and Development
- Geometry and Topology
- Geotechnical Engineering and Engineering Geology
- Geriatrics and Gerontology
- Gerontology
- Global and Planetary Change
- Hardware and Architecture
- Health Informatics
- Health Information Management
- Health Policy
- Health Professions (miscellaneous)
- Health (social science)
- Health, Toxicology and Mutagenesis
- History and Philosophy of Science
- Horticulture
- Human Factors and Ergonomics
- Human-Computer Interaction
- Immunology and Allergy
- Immunology and Microbiology (miscellaneous)
- Industrial and Manufacturing Engineering
- Industrial Relations
- Infectious Diseases
- Information Systems
- Information Systems and Management
- Inorganic Chemistry
- Insect Science
- Instrumentation
- Internal Medicine
- Issues, Ethics and Legal Aspects
- Leadership and Management
- Library and Information Sciences
- Life-span and Life-course Studies
- Linguistics and Language
- Literature and Literary Theory
- LPN and LVN
- Management Information Systems
- Management, Monitoring, Policy and Law
- Management of Technology and Innovation
- Management Science and Operations Research
- Materials Chemistry
- Materials Science (miscellaneous)
- Maternity and Midwifery
- Mathematical Physics
- Mathematics (miscellaneous)
- Mechanical Engineering
- Mechanics of Materials
- Media Technology
- Medical and Surgical Nursing
- Medical Assisting and Transcription
- Medical Laboratory Technology
- Medical Terminology
- Medicine (miscellaneous)
- Metals and Alloys
- Microbiology
- Microbiology (medical)
- Modeling and Simulation
- Molecular Biology
- Molecular Medicine
- Nanoscience and Nanotechnology
- Nature and Landscape Conservation
- Neurology (clinical)
- Neuropsychology and Physiological Psychology
- Neuroscience (miscellaneous)
- Nuclear and High Energy Physics
- Nuclear Energy and Engineering
- Numerical Analysis
- Nurse Assisting
- Nursing (miscellaneous)
- Nutrition and Dietetics
- Obstetrics and Gynecology
- Occupational Therapy
- Ocean Engineering
- Oceanography
- Oncology (nursing)
- Ophthalmology
- Oral Surgery
- Organic Chemistry
- Organizational Behavior and Human Resource Management
- Orthodontics
- Orthopedics and Sports Medicine
- Otorhinolaryngology
- Paleontology
- Parasitology
- Pathology and Forensic Medicine
- Pathophysiology
- Pediatrics, Perinatology and Child Health
- Periodontics
- Pharmaceutical Science
- Pharmacology
- Pharmacology (medical)
- Pharmacology (nursing)
- Pharmacology, Toxicology and Pharmaceutics (miscellaneous)
- Physical and Theoretical Chemistry
- Physical Therapy, Sports Therapy and Rehabilitation
- Physics and Astronomy (miscellaneous)
- Physiology (medical)
- Plant Science
- Political Science and International Relations
- Polymers and Plastics
- Process Chemistry and Technology
- Psychiatry and Mental Health
- Psychology (miscellaneous)
- Public Administration
- Public Health, Environmental and Occupational Health
- Pulmonary and Respiratory Medicine
- Radiological and Ultrasound Technology
- Radiology, Nuclear Medicine and Imaging
- Rehabilitation
- Religious Studies
- Renewable Energy, Sustainability and the Environment
- Reproductive Medicine
- Research and Theory
- Respiratory Care
- Review and Exam Preparation
- Reviews and References (medical)
- Rheumatology
- Safety Research
- Safety, Risk, Reliability and Quality
- Sensory Systems
- Signal Processing
- Small Animals
- Social Psychology
- Social Sciences (miscellaneous)
- Social Work
- Sociology and Political Science
- Soil Science
- Space and Planetary Science
- Spectroscopy
- Speech and Hearing
- Sports Science
- Statistical and Nonlinear Physics
- Statistics and Probability
- Statistics, Probability and Uncertainty
- Strategy and Management
- Stratigraphy
- Structural Biology
- Surfaces and Interfaces
- Surfaces, Coatings and Films
- Theoretical Computer Science
- Tourism, Leisure and Hospitality Management
- Transplantation
- Transportation
- Urban Studies
- Veterinary (miscellaneous)
- Visual Arts and Performing Arts
- Waste Management and Disposal
- Water Science and Technology
- All regions / countries
- Asiatic Region
- Eastern Europe
- Latin America
- Middle East
- Northern America
- Pacific Region
- Western Europe
- ARAB COUNTRIES
- IBEROAMERICA
- NORDIC COUNTRIES
- Afghanistan
- Bosnia and Herzegovina
- Brunei Darussalam
- Czech Republic
- Dominican Republic
- Netherlands
- New Caledonia
- New Zealand
- Papua New Guinea
- Philippines
- Puerto Rico
- Russian Federation
- Saudi Arabia
- South Africa
- South Korea
- Switzerland
- Syrian Arab Republic
- Trinidad and Tobago
- United Arab Emirates
- United Kingdom
- United States
- Vatican City State
- Book Series
- Conferences and Proceedings
- Trade Journals

- Citable Docs. (3years)
- Total Cites (3years)

Follow us on @ScimagoJR Scimago Lab , Copyright 2007-2024. Data Source: Scopus®

Cookie settings
Cookie Policy
Legal Notice
Privacy Policy
Risk of Corneal Transplant Rejection Following COVID-19 Vaccination: A Systematic Review and Meta-analysis
- ORIGINAL RESEARCH
- Open access
- Published: 16 April 2024
Cite this article
You have full access to this open access article
- Parul Chawla Gupta 1 na1 ,
- Bijaya K. Padhi 2 ,
- Hashem Abu Serhan 3 ,
- Arkadiusz Dziedzic 4 ,
- Mahalaqua Nazli Khatib 5 na1 ,
- Shilpa Gaidhane 6 ,
- Quazi Syed Zahiruddin 7 ,
- Abhay M. Gaidhane 8 ,
- Neelima Kukreti 9 ,
- Sarvesh Rustagi 10 &
- Prakasini Satapathy 11 , 12
Introduction
The COVID-19 pandemic has initiated an unparalleled global vaccination campaign, raising concerns about the vaccine's effects on various health conditions, including the risk of corneal transplant rejection. This systematic review aimed to identify the relationship between COVID-19 vaccination and rejection of corneal transplant, filling a significant gap in the existing medical literature.
A literature search was performed across multiple databases up to February 12, 2024, to identify studies evaluating the risk of corneal transplant rejection post-COVID-19 vaccination. Eligible studies were original research that reported outcomes of corneal graft rejection following vaccination. Nested Knowledge web software facilitated screening and data extraction. The Newcastle–Ottawa Scale was employed for quality assessment. A meta-analysis was conducted to calculate the aggregated relative risk (RR) utilizing R software version 4.3.
Six studies were included in the qualitative synthesis, with four meeting the criteria for meta-analysis. These studies varied in geographic location, surgical techniques, and types of vaccines used. The pooled RR for corneal transplant rejection following COVID-19 vaccination was 0.816 (95% CI 0.178–1.453), indicating no significant risk of rejection. No statistical heterogeneity was observed among the studies ( I 2 = 0%).
Conclusions
This review and meta-analysis found no significant evidence that COVID-19 vaccination increases the risk of corneal graft rejection. However, the current evidence is insufficient to conclusively determine the vaccine's safety for corneal transplant recipients. These findings underscore the need for additional research to confirm these preliminary results and investigate the long-term effects of COVID-19 vaccination on corneal transplants, aiming to provide evidence-based guidance to healthcare providers and patients.
Avoid common mistakes on your manuscript.
During the unparalleled worldwide health emergency induced by the COVID-19 pandemic, vaccination has risen as a cornerstone in the collective effort to control the spread of the SARS-CoV-2 virus and alleviate its profound impact on worldwide public health infrastructure [ 1 , 2 , 3 ]. The swift development, regulatory approval, and extensive administration of COVID-19 vaccines have played an instrumental role in decreasing transmission rates, reducing the severity of cases, and lowering mortality rates attributed to the virus [ 4 ]. As the vaccination initiative has expanded, there has been an escalating interest in discerning the potential ramifications of COVID-19 vaccines on various health conditions and medical interventions, particularly concerning organ transplantation procedures [ 5 , 6 , 7 , 8 ].
Corneal transplantation, or keratoplasty, represents a critical surgical intervention for restoring vision in individuals affected by corneal opacities, dystrophies, or scarring [ 9 , 10 , 11 ]. Despite the procedure's notable success rates, the postoperative period is fraught with the challenge of immune-mediated graft rejection, a significant cause of graft failure and subsequent vision loss [ 11 ]. The introduction of the COVID-19 vaccine has sparked a debate over its potential impact on the incidence or intensity of corneal transplant rejection [ 12 ]. This speculation is rooted in the notion that the vaccine's systemic immune activation could amplify the immune response to the transplanted corneal tissue, thereby elevating the risk of graft rejection [ 13 ].
The importance of addressing this concern is twofold; not only does it bear significance for patient care but it also has implications for the formulation of vaccination guidelines for individuals awaiting or having undergone corneal transplantation. Consequently, there is an urgent need to thoroughly examine the existing evidence to determine the relationship between COVID-19 vaccination and the risk of corneal transplant rejection [ 14 , 15 , 16 , 17 ]. While previous systematic reviews have delved into the characteristics and outcomes of corneal transplant rejection in the context of COVID-19 vaccination [ 18 , 19 ], there still needs to be a gap in the literature regarding a comprehensive assessment of the risk associated with corneal graft rejection post-vaccination.
This systematic review and meta-analysis seeks to bridge this gap by aggregating and scrutinizing data from various studies investigating the occurrence of corneal graft rejection after COVID-19 vaccination. The objective is to determine whether a statistically significant association exists between vaccination and an increased risk of rejection. This endeavor is vital for informing clinical decision-making processes, guiding vaccination policy for transplant recipients, and optimizing patient outcomes during the pandemic. By providing a detailed analysis of the evidence, this review aimed to contribute valuable insights to the body of knowledge on the efficacy and safety profile of COVID-19 vaccines in the context of corneal transplantation, thereby aiding healthcare professionals, policymakers, and patients in navigating the complexities of transplantation and vaccination during these challenging times.
We followed the Preferred Reporting Items for Systematic Reviews and Meta-Analyses (PRISMA) to conduct the systematic review (Table S1 ). For the processes of screening and extracting data, we employed the Nested-Knowledge (Nested-Knowledge, Saint Paul, MN, USA) software. The protocol has been registered in PROSPERO.
Ethical Approval
Ethical approval is not required since this is a systematic review. This study was performed in accordance with the Helsinki Declaration of 1964 and its later amendments.
Eligibility Criteria
Studies were eligible for inclusion if they involved individuals who had undergone corneal transplantation and subsequently received a COVID-19 vaccine, reported outcomes specifically related to corneal graft rejection, and fell into the categories of either observational studies or clinical trials. On the other hand, exclusions were made for studies that did not explicitly address the incidence or outcomes of corneal transplant rejection after receiving the COVID-19 vaccine. Narrative reviews, case reports, editorial comments, and studies lacking primary data were also excluded from the analysis. The criteria for inclusion are detailed in Table S2.
Search Strategy
A literature search was performed across multiple electronic databases such as EMBASE, PubMed, and Web of Science, from their inception until February 12, 2024, to identify studies assessing the risk of corneal transplant rejection after receiving the COVID-19 vaccine. The search strategy was crafted to encompass a combination of keywords and MeSH terms related to "corneal transplantation," "keratoplasty," "COVID-19," "SARS-CoV-2," "vaccination," and "graft rejection." No language restrictions were imposed to ensure the inclusion of a wide range of relevant studies. Furthermore, we conducted a manual examination of the reference lists from the identified articles to discover additional studies that met our eligibility criteria. Google Scholar was also manually explored for additional relevant publications to enhance the search comprehensively. The details of the full search strategy are provided in Table S3.
For the screening process, we utilized the advanced capabilities of Nested Knowledge software, a specialized tool for managing and streamlining literature. The screening involved two steps: title and abstract, followed by full-text screening. Two independent reviewers screened the articles. A third reviewer resolved any discrepancies.
Data Extraction and Quality Assessment
Data from the included studies was collected by two separate reviewers using a uniform data collection template. The tagging function of Nested Knowledge software was used for data extraction. Data extracted included study design, participant characteristics, type of COVID-19 vaccine administered, incidence of graft rejection, and outcomes of interest, such as Relative Risk (RR)/Odds Ratio (OR)/Hazard Ratio (HR) for graft rejection. Disagreements among reviewers were settled via discussion, or when required, by seeking the opinion of a third reviewer. The quality assessment of the studies incorporated in this review was performed utilizing the Newcastle–Ottawa Scale (NOS) [ 20 , 21 ].
Statistical Analysis
Meta-analytic techniques were employed to estimate the pooled RR of corneal transplant rejection after receiving the COVID-19 vaccine. Heterogeneity among studies was quantified using the I 2 statistic, with values greater than 50% accounting for substantial heterogeneity [ 22 , 23 ]. A random-effects model (REM) was utilized to accommodate the expected variability among studies. The tau-squared ( τ 2 ) statistic, calculated using the maximum likelihood estimation method, served as an additional measure of heterogeneity [ 24 ]. A p value of less than 0.05 was established as the criterion for statistical significance. The analyses were conducted using the "Meta" and "Metaphor" packages within R statistical software, Version 4.3 [ 20 ].
Search Result
The search across various databases resulted in 229 records initially. Upon the removal of 99 duplicates prior to screening, we proceeded to screen 130 records. Out of these, 115 records were excluded for various reasons [ 25 , 26 ], leaving 15 full-text articles to be assessed for eligibility. Additionally, records identified through citation searching and Google Scholar amounted to seven more articles retrieved for potential inclusion, of which four studies were included. Upon full-text review, 13 articles were excluded from the analysis: two were case reports, and 11 did not report the risk of graft rejection. Consequently, six studies were included in the systematic review. Of these, four studies provided data suitable for meta-analysis (Fig. 1 ).
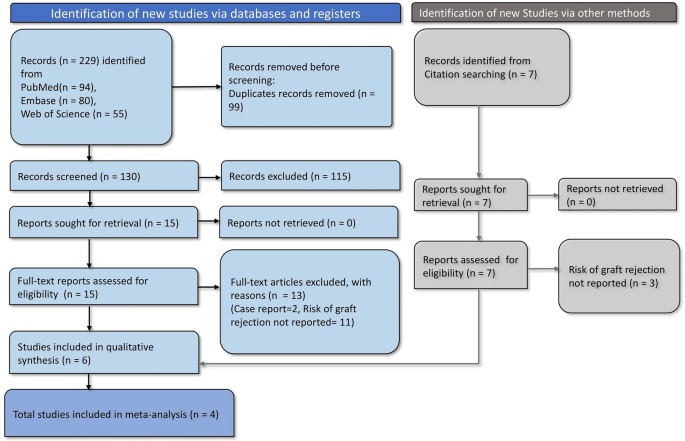
PRISMA flowchart depicting study selection and screening process
Characteristics of Included Studies
Table 1 presents a summary of the characteristics of the studies included in the analysis. Three studies were from Japan [ 27 , 28 , 29 ], two from Italy [ 8 , 30 ], and one from the USA [ 31 ]. All of the studies employed a retrospective design. The surgical techniques utilized across the studies included conventional penetrating keratoplasty (PK), deep anterior lamellar keratoplasty (DALK), Descemet stripping automated endothelial keratoplasty (DSAEK), Descemet membrane endothelial keratoplasty (DMEK), and mushroom PK. The type of COVID-19 vaccine was not reported in two of the studies. In contrast, one study exclusively involved an mRNA vaccine, and another study included a variety of vaccines such as BNT162b2, Moderna, Pfizer COVID-19 Vaccine, Johnson & Johnson's Janssen Vaccine, AZD1222, and Ad26.COV2.S. The quality assessment of the studies is presented in Table S4.
Risk of Corneal Transplant Rejection After Receiving COVID-19 Vaccine
The transplant rejection risk varied between studies. In Japan, Igarashi et al. (2023) [ 27 ] conducted a retrospective cohort study involving 74 vaccinated patients with an average age of 73.3 years who underwent DMEK. Although the specific type of vaccine was not reported, the study observed an HR of 25.5 (95% CI 2.10–309) for graft rejection over a 474-day follow-up period. From Italy, Busin et al. (2022) [ 30 ] analyzed 785 vaccinated patients with a mean age of 58.6 years. Various surgical techniques were utilized, such as DALK, DMEK, DSAEK, and different forms of PK. Vaccinations included BNT162b2 (79.2%), mRNA-based vaccines (15.7%), AZD1222 (3.2%), and Ad26.COV2.S (1.9%). The study reported an HR of 0.75 (95% CI 0.10–5.52) and an incidence rate ratio (IRR) of 0.56 (95% CI 0.13–2.28) following 60 days after the last vaccine dose. In another retrospective study, Roberts et al. (2023) reported that An IRR of 0.53 with a p value of 0.71 was reported, suggesting no significant association with graft rejection. Fujimoto et al. (2021) presented findings from a Japanese study with 29 patients, averaging 72 years old, who received various corneal transplants, including PK, DSAEK, ALK or DALK, and LT. The BNT162b2 vaccine yielded an OR of 5.57 (95% CI 1.18–25.57) and an RR of 4.47 (95% CI 1.15–18.34) within a 74-day follow-up. In the USA, Culp et al. (2022) included 784 patients in a retrospective study that did not disclose mean age or surgical techniques. The vaccines administered were Moderna, Pfizer COVID-19, or Johnson & Johnson's Janssen Vaccine, with a reported RR of 0.92 (95% CI 0.42–2.01), indicating no significant risk of rejection post-vaccination. Fujimoto et al. (2024) conducted a retrospective cohort study in Japan with an average patient age of 67.95 years. Though the number of vaccinated patients was not detailed, the study utilized PK, DSAEK, DALK, and LT with SARS-CoV-2 mRNA vaccines and reported an RR of 5.74 over an extensive 804-day follow-up.
- Meta-analysis
We performed a meta-analysis of four studies that reported the RR for corneal transplant rejection following COVID-19 vaccination (Fig. 2 ). The studies included in the analysis were Busin 2022, Culp 2022, Fujimoto 2021, and Igarashi 2023. The pooled RR was estimated using a REM, accounting for variation within and between studies. The pooled RR for the meta-analysis was 0.816 (95% CI 0.178–1.453), indicating no significant risk of corneal transplant rejection after receiving COVID-19 vaccine across the included studies. The prediction interval, which provides a range where the true effect is expected to lie in similar studies, was – 0.584 to 2.215. There was no observed heterogeneity among the studies (Tau 2 = 0; Chi 2 = 1.16, ( p = 0.76); I 2 = 0%).
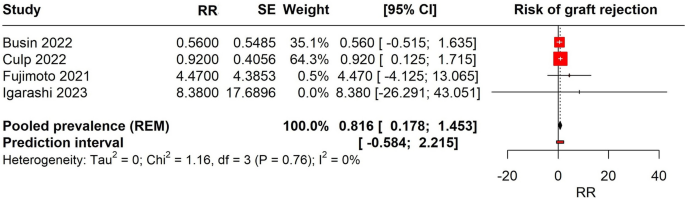
Forest plot depicting the risk of corneal transplant rejection with COVID-19 vaccination
Publication Bias
Our ability to evaluate the presence of publication bias was constrained by the limited number of studies included in our meta-analysis. Typically, assessment methods such as funnel plots or Egger's test require more studies to provide reliable results. Due to this limitation, we cannot draw definitive conclusions about the potential for publication bias in this body of research.
Our study represents the first attempt to perform a meta-analysis assessing the risk of corneal transplant rejection after COVID-19 vaccine administration. Our analysis revealed a pooled RR of 0.816 (95% CI 0.178–1.453) for corneal transplant rejection after receiving the COVID-19 vaccine. This finding suggests no significant increase in the risk of graft rejection among vaccinated individuals. The studies analyzed varied in geographic location, surgical techniques, and types of COVID-19 vaccines administered, reflecting a broad spectrum of clinical practices and patient experiences. Despite these variations, the overall lack of a significant association between COVID-19 vaccination and increased risk of corneal graft rejection may suggest a favorable safety profile for the vaccines in the context of corneal transplantation. Nonetheless, the available evidence still needs to provide a definitive conclusion.
While previous systematic reviews have broached the subject by collating case reports, our study provides a risk analysis of corneal transplant rejection with COVID-19 vaccination. For instance, a previous review [ 19 ] documented various types of corneal transplants, including 12 cases of penetrating keratoplasty, six of DMEK, four of DSAEK, and one instance of living-related conjunctival-limbal allograft. The onset of graft rejection post-vaccination ranged from as short as 1 day to up to 6 weeks. The leading clinical presentation was corneal edema, occurring in 20 eyes. Other notable symptoms included keratic precipitates and conjunctival or ciliary injection, each observed in 14 eyes. The primary treatment involved the frequent application of topical corticosteroids in 12 eyes and a regimen combining both topical and oral corticosteroids in four eyes. Corneal edema was identified as the most prevalent clinical ocular manifestation post-vaccination, affecting 87% of the cases, with keratic precipitates and conjunctival injection also being significant findings. Another systematic review has also reported similar findings [ 32 ].
Implications of this study are significant for clinical practice, particularly for the management of corneal transplant recipients in the era of COVID-19 vaccination. Our findings suggest that COVID-19 vaccines do not increase the risk of corneal graft rejection, which may alleviate concerns among healthcare providers and patients about the potential impact of vaccination on transplant outcomes. This could potentially support vaccination campaigns within this patient population, emphasizing the importance of protection against COVID-19 without compromising graft survival. Studies with larger cohorts are essential to validate our results and enable a more robust analysis of publication bias. Studies that are longitudinal in nature and include extended periods of follow-up could offer valuable insights into the enduring impacts of COVID-19 vaccination on the survival of corneal grafts. Further research should also aim to include diverse populations to ensure the applicability of the results across different patient demographics. Investigations into the relationship between different types of COVID-19 vaccines and corneal transplant rejection could uncover vaccine-specific risks or benefits. Additionally, mechanistic studies exploring the immunological responses to vaccination in the context of corneal transplantation could further our understanding of graft rejection. Comparative effectiveness research could situate the safety profile of COVID-19 vaccines within the broader context of vaccines administered to transplant recipients. Utilizing data from transplant registries might offer a comprehensive view of the post-vaccination outcomes on a global scale, providing valuable data for healthcare policy and practice. Addressing these research directions will be crucial in advancing our knowledge and ensuring the well-being of corneal transplant recipients during and beyond the pandemic.
Our review is subject to several limitations. The number of available studies that reported an association between the COVID-19 vaccine and corneal transplant rejection was very limited. The inherent biases of retrospective study designs, which constituted all the included studies, may affect the reliability of the results. Additionally, the variability in reporting the type of COVID-19 vaccines and the lack of detailed immunological profiles of the recipients represent gaps in the data. More studies are needed in the future for a better understanding of the condition.
Our analysis has revealed no significant evidence to suggest that COVID-19 vaccination increases the risk of corneal graft rejection. However, the evidence currently available is insufficient to conclusively determine the safety of COVID-19 vaccines on corneal transplant recipients. Further research is necessary to verify these findings and investigate the long-term effects of COVID-19 vaccination on corneal transplant outcomes. This will enable healthcare providers to offer evidence-based advice to their patients.
Data Availability
The data are with the authors and available on request.
Agyarko R, Al Slail F, Garrett DO, Gentry B, Gresham L, Underwood MLK, et al. The imperative for global cooperation to prevent and control pandemics. In: Modernizing global health security to prevent, detect, and respond. NY: Elsevier; 2024. p. 53–69.
Chapter Google Scholar
Dzinamarira T, Tungwarara N, Chitungo I, Chimene M, Iradukunda PG, Mashora M, et al. Unpacking the implications of SARS-CoV-2 breakthrough infections on COVID-19 vaccination programs. Vaccines. 2022;10(2):252.
Article CAS PubMed PubMed Central Google Scholar
Adu P, Popoola T, Medvedev ON, Collings S, Mbinta J, Aspin C, Simpson CR. Implications for COVID-19 vaccine uptake: a systematic review. J Infect Public Health. 2023;16(3):441–66.
Article PubMed PubMed Central Google Scholar
Liu H, Han X, Lin X, Zhu X, Wei Y. Impact of vaccine measures on the transmission dynamics of COVID-19. PLoS ONE. 2023;18(8): e0290640.
Chen X, Luo D, Mei B, Du J, Liu X, Xie H, et al. Immunogenicity of COVID-19 vaccines in solid organ transplant recipients: a systematic review and meta-analysis. Clin Microbiol Infect. 2023;29(4):441–56.
Article CAS PubMed Google Scholar
Luo D, Chen X, Du J, Mei B, Wang A, Kuang F, et al. Immunogenicity of COVID-19 vaccines in chronic liver disease patients and liver transplant recipients: a systematic review and meta-analysis. Liver Int. 2023;43(1):34–48.
Article PubMed Google Scholar
Akbulut S, Yagin FH, Sahin TT, Garzali IU, Tuncer A, Akyuz M, et al. Effect of COVID-19 pandemic on patients who have undergone liver transplantation: retrospective cohort study. J Clin Med. 2023;12(13):4466.
Roberts HW, Wilkins MR, Malik M, Talachi-Langroudi M, Myerscough J, Pellegrini M, et al. A lack of an association between COVID-19 vaccination and corneal graft rejection: results of a large multi-country population based study. Eye. 2023;37(11):2316–9.
Zhou Y, Wang T, Tuli SS, Steigleman WA, Shah AA. Overview of corneal transplantation for the nonophthalmologist. Transplant Direct. 2023;9(2): e1434.
Hemaya M, Hemaya M, Habeeb A. Evaluating keratoplasty for Fuchs’ endothelial corneal dystrophy: a literature review. Cureus. 2023. https://doi.org/10.7759/cureus.33639 .
Wajima H, Hayashi T, Kobayashi A, Nishino T, Mori N, Yokogawa H, et al. Graft rejection episodes after keratoplasty in Japanese eyes. Sci Rep. 2023;13(1):2635.
Allen NE, Zhang J, McGhee CN. COVID-19 vaccination and corneal allograft rejection-a review. Front Cell Infect Microbiol. 2023. https://doi.org/10.3389/fcimb.2023.1307655 .
Singh RB, Li J, Parmar UPS, Jeng BH, Jhanji V. Vaccine-associated corneal graft rejection following SARS-CoV-2 vaccination: a CDC-VAERS database analysis. Br J Ophthalmol. 2024;108(1):17–22.
Article Google Scholar
Raiker R, Akosman S, Foos W, Pakhchanian H, Mishra S, Geist C, Belyea DA. Examining the influence of COVID-19 infection and pandemic restrictions on the risk of corneal transplant rejection or failure: a multicenter study. Semin Ophthalmol. 2023;38(8):777–83.
Kuziez L, Eleiwa TK, Chauhan MZ, Sallam AB, Elhusseiny AM, Saeed HN. Corneal adverse events associated with SARS-CoV-2/COVID-19 vaccination: a systematic review. Vaccines. 2023;11(1):166.
Wasai Y, Yamada N, Ariyoshi N, Haraguchi A, Funatsu M, Mikuni M, et al. SARS-CoV-2 infection status in corneal preservation solution and COVID-19 prevalence after corneal transplantation. Sci Rep. 2024;14(1):3766.
Bushi G, Shabil M, Padhi BK, Ahmed M, Pandey P, Satapathy P, et al. Prevalence of acute kidney injury among dengue cases: a systematic review and meta-analysis. Trans R Soc Trop Med Hyg. 2024;118(1):1–11.
Moura-Coelho N, Cunha JP, Papa-Vettorazzi R, Gris Ó, Güell JL. Acute corneal allograft rejection following SARS-CoV-2 vaccination: a systematic review. Acta Ophthalmol. 2023;101(1):e1–13.
Fujio K, Sung J, Nakatani S, Yamamoto K, Iwagami M, Fujimoto K, et al. Characteristics and clinical ocular manifestations in patients with acute corneal graft rejection after receiving the COVID-19 vaccine: a systematic review. J Clin Med. 2022;11(15):4500.
Bushi G, Padhi BK, Shabil M, Satapathy P, Rustagi S, Pradhan KB, et al. Cardiovascular disease outcomes associated with obstructive sleep apnea in diabetics: a systematic review and meta-analysis. Diseases. 2023;11(3):103.
Shabil M, Bushi G, Beig MA, Rais MA, Ahmed M, Padhi BK. Cardiovascular manifestation in tuberculosis cases: a systematic review and meta-analysis. Curr Probl Cardiol. 2023;48(7): 101666.
Gandhi AP, Satapathy P, Rustagi S, Hermis AH, Sah R, Padhi BK. Comments on" Shigellosis in Southeast Asia: a systematic review and meta-analysis. Travel Med Infect Dis. 2023;54: 102593.
Swarup SS, Padhi BK, Satapathy P, Shabil M, Bushi G, Gandhi AP, et al. Cardiovascular consequences of financial stress: a systematic review and meta-analysis. Curr Probl Cardiol. 2023;49: 102153.
Shabil M, Bushi G, Yadav A, Ahmed M, Kishore J, Lekamwasam S, Joshi A. Effect of metformin on cardiovascular outcomes: a systematic review and meta-analysis of observational studies and RCTs. The Evidence. 2023;1(1):23–34.
Google Scholar
Shabil M, Kumar VU, Dhingra S, Ravichandiran V, Parihar VK, Kumar N, et al. Current scenario and strategies to tackle cardiovascular disease risk in HIV geriatrics. Curr Pharmacol Rep. 2023;9(6):523–39.
Shabil M, Murti K, Kumar VU, Kumar R, Kumar N, Dhingra S, et al. Older PLHIV are at higher cardiovascular risk with poor quality of life. Curr HIV Res. 2023;21(6):354–60.
Igarashi A, Shimizu T, Takeda M, Ida Y, Ishida A, Yuda K, et al. Incidence of graft rejection in Descemet membrane endothelial keratoplasty after COVID-19 mRNA vaccination. Cornea. 2022;13:10–97.
Fujimoto H, Kiryu J. Incidence of corneal transplant rejection following BNT162b2 SARS-CoV-2 messenger RNA vaccination. J Ophthalmol Res. 2021;4(3):279–88.
Fujimoto H. Rejection of corneal transplant after administration of SARS-CoV-2 messenger RNA vaccine. J Ophthalmol Res. 2024;7:13–5.
Busin M, Zauli G, Pellegrini M, Virgili G, Yu AC. COVID-19 vaccination may not increase rates of corneal graft rejection. Cornea. 2022;41(12):1536–8.
Culp CJ, Pakhchanian H, Raiker R, Chan C, Foos WF, Belyea D. Risk of corneal graft rejection in COVID-19 vaccinated patients in the 120-day postoperative period: a multi-healthcare system analysis. Investig Ophthalmol Vis Sci. 2022;63(7):4351-A0288.
Huang L-Y, Chiang C-C, Li Y-L, Lai H-Y, Hsieh Y-C, Wu Y-H, Tsai Y-Y. Corneal complications after COVID-19 vaccination: a systemic review. J Clin Med. 2022;11(22):6828.
Download references
Acknowledgements
The authors acknowledge the Nested-Knowledge, Saint Paul, MN, USA for providing access to the software. Qatar National Library funded the publication of this article.
This author received no funding for conducting the study. The journal’s Rapid Service Fee was funded by Qatar National Library through the author Hashem Abu Serhan.
Author information
Parul Chawla Gupta and Mahalaqua Nazli Khatib contributed equally to this work.

Authors and Affiliations
Department of Ophthalmology, Postgraduate Institute of Medical Education and Research, Chandigarh, 160012, India
Parul Chawla Gupta
Department of Community Medicine and School of Public Health, Postgraduate Institute of Medical Education and Research, Chandigarh, 160012, India
Bijaya K. Padhi
Department of Ophthalmology, Hamad Medical Corporation, Doha, Qatar
Hashem Abu Serhan
Department of Conservative Dentistry with Endodontics, Medical University of Silesia, 40-055, Katowice, Poland
Arkadiusz Dziedzic
Division of Evidence Synthesis, Global Consortium of Public Health and Research, Datta Meghe Institute of Higher Education, Wardha, India
Mahalaqua Nazli Khatib
One Health Centre (COHERD), Jawaharlal Nehru Medical College, Datta Meghe Institute of Higher Education, Wardha, India
Shilpa Gaidhane
Division of Evidence Synthesis, Global Consortium of Public Health and Research, South Asia Infant Feeding Research Network (SAIFRN), Datta Meghe Institute of Higher Education, Wardha, India
Quazi Syed Zahiruddin
School of Epidemiology and Public Health, Jawaharlal Nehru Medical College, and Global Health Academy, Datta Meghe Institute of Higher Education, Wardha, India
Abhay M. Gaidhane
School of Pharmacy, Graphic Era Hill University, Dehradun, India
Neelima Kukreti
School of Applied and Life Sciences, Uttaranchal University, Dehradun, Uttarakhand, India
Sarvesh Rustagi
Center for Global Health Research, Saveetha Medical College and Hospital, Saveetha Institute of Medical and Technical Sciences, Saveetha University, Chennai, India
Prakasini Satapathy
Medical Laboratories Techniques Department, AL-Mustaqbal University, Hillah, Babil, 51001, Iraq
You can also search for this author in PubMed Google Scholar
Contributions
Conceptualization: Bijaya K Padhi, Mahalaqua Nazli Khatib, Parul Chawla Gupta; Data curation: Mahalaqua Nazli Khatib, Quazi Syed Zahiruddin, Arkadiusz Dziedzic; Formal analysis: Shilpa Gaidhane, Arkadiusz Dziedzic; Investigation: Shilpa Gaidhane, Prakasini Satapathy, Parul Chawla Gupta; Methodology: Bijaya K Padhi, Arkadiusz Dziedzic, Neelima Kukreti; Project administration: Abhay M Gaidhane; Resources: Quazi Syed Zahiruddin, Prakasini Satapathy; Software: Sarvesh Rustagi; Supervision: Abhay M Gaidhane; Validation: Neelima Kukreti, Sarvesh Rustagi; Visualization: Hashem Abu Serhan; Writing – original draft: Mahalaqua Nazli Khatib; Writing – review & editing: Hashem Abu Serhan, Prakasini Satapathy.
Corresponding author
Correspondence to Hashem Abu Serhan .
Ethics declarations
Conflict of interest.
All named authors confirm that they have no conflicts of interest to report.
Ethical approval is not required since it is a systematic review. This study was performed in accordance with the Helsinki Declaration of 1964 and its later amendments.
Supplementary Information
Below is the link to the electronic supplementary material.
Supplementary file1 (PDF 233 KB)
Rights and permissions.
Open Access This article is licensed under a Creative Commons Attribution-NonCommercial 4.0 International License, which permits any non-commercial use, sharing, adaptation, distribution and reproduction in any medium or format, as long as you give appropriate credit to the original author(s) and the source, provide a link to the Creative Commons licence, and indicate if changes were made. The images or other third party material in this article are included in the article's Creative Commons licence, unless indicated otherwise in a credit line to the material. If material is not included in the article's Creative Commons licence and your intended use is not permitted by statutory regulation or exceeds the permitted use, you will need to obtain permission directly from the copyright holder. To view a copy of this licence, visit http://creativecommons.org/licenses/by-nc/4.0/ .
Reprints and permissions
About this article
Gupta, P.C., Padhi, B.K., Abu Serhan, H. et al. Risk of Corneal Transplant Rejection Following COVID-19 Vaccination: A Systematic Review and Meta-analysis. Ophthalmol Ther (2024). https://doi.org/10.1007/s40123-024-00941-y
Download citation
Received : 05 March 2024
Accepted : 25 March 2024
Published : 16 April 2024
DOI : https://doi.org/10.1007/s40123-024-00941-y
Share this article
Anyone you share the following link with will be able to read this content:
Sorry, a shareable link is not currently available for this article.
Provided by the Springer Nature SharedIt content-sharing initiative
- COVID-19 vaccine
- Corneal transplantation
- Systematic review
- Ophthalmology
- Find a journal
- Publish with us
- Track your research
- Download PDF
- Share X Facebook Email LinkedIn
- Permissions
Ocular Pentastomiasis in Human Hosts
- 1 Department of Ophthalmology, Cliniques Universitaires Saint-Luc, UCLouvain, Bruxelles, Belgium
- 2 Department of Ophthalmology, CHU UCL NAMUR, UCLouvain, Yvoir, Belgium
- 3 National Reference Center for Tropical Diseases, Bernhard Nocht Institute for Tropical Medicine, Hamburg, Germany
- 4 St Raphael Ophthalmological Center, Ophthalmological Ambulance, Mbuji Mayi, Democratic Republic of Congo
Pentastomiasis is a rare zoonotic disease caused by the larval stages of pentastomid parasites. 1 , 2 Most human infections occur in the tropics and subtropics. In Africa and Asia, the disease is often caused by Armillifer species, which have snakes as their final host, whereas Linguatula infections are cosmopolitan, with dogs as final hosts. 1 , 2
Read More About
Van Acker G , Ates E , Levecq L , Tappe D , Hardi R. Ocular Pentastomiasis in Human Hosts. JAMA Ophthalmol. Published online April 11, 2024. doi:10.1001/jamaophthalmol.2024.0685
Manage citations:
© 2024
Artificial Intelligence Resource Center
Ophthalmology in JAMA : Read the Latest
Browse and subscribe to JAMA Network podcasts!
Others Also Liked
Select your interests.
Customize your JAMA Network experience by selecting one or more topics from the list below.
- Academic Medicine
- Acid Base, Electrolytes, Fluids
- Allergy and Clinical Immunology
- American Indian or Alaska Natives
- Anesthesiology
- Anticoagulation
- Art and Images in Psychiatry
- Artificial Intelligence
- Assisted Reproduction
- Bleeding and Transfusion
- Caring for the Critically Ill Patient
- Challenges in Clinical Electrocardiography
- Climate and Health
- Climate Change
- Clinical Challenge
- Clinical Decision Support
- Clinical Implications of Basic Neuroscience
- Clinical Pharmacy and Pharmacology
- Complementary and Alternative Medicine
- Consensus Statements
- Coronavirus (COVID-19)
- Critical Care Medicine
- Cultural Competency
- Dental Medicine
- Dermatology
- Diabetes and Endocrinology
- Diagnostic Test Interpretation
- Drug Development
- Electronic Health Records
- Emergency Medicine
- End of Life, Hospice, Palliative Care
- Environmental Health
- Equity, Diversity, and Inclusion
- Facial Plastic Surgery
- Gastroenterology and Hepatology
- Genetics and Genomics
- Genomics and Precision Health
- Global Health
- Guide to Statistics and Methods
- Hair Disorders
- Health Care Delivery Models
- Health Care Economics, Insurance, Payment
- Health Care Quality
- Health Care Reform
- Health Care Safety
- Health Care Workforce
- Health Disparities
- Health Inequities
- Health Policy
- Health Systems Science
- History of Medicine
- Hypertension
- Images in Neurology
- Implementation Science
- Infectious Diseases
- Innovations in Health Care Delivery
- JAMA Infographic
- Law and Medicine
- Leading Change
- Less is More
- LGBTQIA Medicine
- Lifestyle Behaviors
- Medical Coding
- Medical Devices and Equipment
- Medical Education
- Medical Education and Training
- Medical Journals and Publishing
- Mobile Health and Telemedicine
- Narrative Medicine
- Neuroscience and Psychiatry
- Notable Notes
- Nutrition, Obesity, Exercise
- Obstetrics and Gynecology
- Occupational Health
- Ophthalmology
- Orthopedics
- Otolaryngology
- Pain Medicine
- Palliative Care
- Pathology and Laboratory Medicine
- Patient Care
- Patient Information
- Performance Improvement
- Performance Measures
- Perioperative Care and Consultation
- Pharmacoeconomics
- Pharmacoepidemiology
- Pharmacogenetics
- Pharmacy and Clinical Pharmacology
- Physical Medicine and Rehabilitation
- Physical Therapy
- Physician Leadership
- Population Health
- Primary Care
- Professional Well-being
- Professionalism
- Psychiatry and Behavioral Health
- Public Health
- Pulmonary Medicine
- Regulatory Agencies
- Reproductive Health
- Research, Methods, Statistics
- Resuscitation
- Rheumatology
- Risk Management
- Scientific Discovery and the Future of Medicine
- Shared Decision Making and Communication
- Sleep Medicine
- Sports Medicine
- Stem Cell Transplantation
- Substance Use and Addiction Medicine
- Surgical Innovation
- Surgical Pearls
- Teachable Moment
- Technology and Finance
- The Art of JAMA
- The Arts and Medicine
- The Rational Clinical Examination
- Tobacco and e-Cigarettes
- Translational Medicine
- Trauma and Injury
- Treatment Adherence
- Ultrasonography
- Users' Guide to the Medical Literature
- Vaccination
- Venous Thromboembolism
- Veterans Health
- Women's Health
- Workflow and Process
- Wound Care, Infection, Healing
- Register for email alerts with links to free full-text articles
- Access PDFs of free articles
- Manage your interests
- Save searches and receive search alerts
This paper is in the following e-collection/theme issue:
Published on 12.4.2024 in Vol 26 (2024)
Application of AI in in Multilevel Pain Assessment Using Facial Images: Systematic Review and Meta-Analysis
Authors of this article:

- Jian Huo 1 * , MSc ;
- Yan Yu 2 * , MMS ;
- Wei Lin 3 , MMS ;
- Anmin Hu 2, 3, 4 , MMS ;
- Chaoran Wu 2 , MD, PhD
1 Boston Intelligent Medical Research Center, Shenzhen United Scheme Technology Company Limited, Boston, MA, United States
2 Department of Anesthesia, Shenzhen People's Hospital, The First Affiliated Hospital of Southern University of Science and Technology, Shenzhen Key Medical Discipline, Shenzhen, China
3 Shenzhen United Scheme Technology Company Limited, Shenzhen, China
4 The Second Clinical Medical College, Jinan University, Shenzhen, China
*these authors contributed equally
Corresponding Author:
Chaoran Wu, MD, PhD
Department of Anesthesia
Shenzhen People's Hospital, The First Affiliated Hospital of Southern University of Science and Technology
Shenzhen Key Medical Discipline
No 1017, Dongmen North Road
Shenzhen, 518020
Phone: 86 18100282848
Email: [email protected]
Background: The continuous monitoring and recording of patients’ pain status is a major problem in current research on postoperative pain management. In the large number of original or review articles focusing on different approaches for pain assessment, many researchers have investigated how computer vision (CV) can help by capturing facial expressions. However, there is a lack of proper comparison of results between studies to identify current research gaps.
Objective: The purpose of this systematic review and meta-analysis was to investigate the diagnostic performance of artificial intelligence models for multilevel pain assessment from facial images.
Methods: The PubMed, Embase, IEEE, Web of Science, and Cochrane Library databases were searched for related publications before September 30, 2023. Studies that used facial images alone to estimate multiple pain values were included in the systematic review. A study quality assessment was conducted using the Quality Assessment of Diagnostic Accuracy Studies, 2nd edition tool. The performance of these studies was assessed by metrics including sensitivity, specificity, log diagnostic odds ratio (LDOR), and area under the curve (AUC). The intermodal variability was assessed and presented by forest plots.
Results: A total of 45 reports were included in the systematic review. The reported test accuracies ranged from 0.27-0.99, and the other metrics, including the mean standard error (MSE), mean absolute error (MAE), intraclass correlation coefficient (ICC), and Pearson correlation coefficient (PCC), ranged from 0.31-4.61, 0.24-2.8, 0.19-0.83, and 0.48-0.92, respectively. In total, 6 studies were included in the meta-analysis. Their combined sensitivity was 98% (95% CI 96%-99%), specificity was 98% (95% CI 97%-99%), LDOR was 7.99 (95% CI 6.73-9.31), and AUC was 0.99 (95% CI 0.99-1). The subgroup analysis showed that the diagnostic performance was acceptable, although imbalanced data were still emphasized as a major problem. All studies had at least one domain with a high risk of bias, and for 20% (9/45) of studies, there were no applicability concerns.
Conclusions: This review summarizes recent evidence in automatic multilevel pain estimation from facial expressions and compared the test accuracy of results in a meta-analysis. Promising performance for pain estimation from facial images was established by current CV algorithms. Weaknesses in current studies were also identified, suggesting that larger databases and metrics evaluating multiclass classification performance could improve future studies.
Trial Registration: PROSPERO CRD42023418181; https://www.crd.york.ac.uk/prospero/display_record.php?RecordID=418181
Introduction
The definition of pain was revised to “an unpleasant sensory and emotional experience associated with, or resembling that associated with, actual or potential tissue damage” in 2020 [ 1 ]. Acute postoperative pain management is important, as pain intensity and duration are critical influencing factors for the transition of acute pain to chronic postsurgical pain [ 2 ]. To avoid the development of chronic pain, guidelines were promoted and discussed to ensure safe and adequate pain relief for patients, and clinicians were recommended to use a validated pain assessment tool to track patients’ responses [ 3 ]. However, these tools, to some extent, depend on communication between physicians and patients, and continuous data cannot be provided [ 4 ]. The continuous assessment and recording of patient pain intensity will not only reduce caregiver burden but also provide data for chronic pain research. Therefore, automatic and accurate pain measurements are necessary.
Researchers have proposed different approaches to measuring pain intensity. Physiological signals, for example, electroencephalography and electromyography, have been used to estimate pain [ 5 - 7 ]. However, it was reported that current pain assessment from physiological signals has difficulties isolating stress and pain with machine learning techniques, as they share conceptual and physiological similarities [ 8 ]. Recent studies have also investigated pain assessment tools for certain patient subgroups. For example, people with deafness or an intellectual disability may not be able to communicate well with nurses, and an objective pain evaluation would be a better option [ 9 , 10 ]. Measuring pain intensity from patient behaviors, such as facial expressions, is also promising for most patients [ 4 ]. As the most comfortable and convenient method, computer vision techniques require no attachments to patients and can monitor multiple participants using 1 device [ 4 ]. However, pain intensity, which is important for pain research, is often not reported.
With the growing trend of assessing pain intensity using artificial intelligence (AI), it is necessary to summarize current publications to determine the strengths and gaps of current studies. Existing research has reviewed machine learning applications for acute postoperative pain prediction, continuous pain detection, and pain intensity estimation [ 10 - 14 ]. Input modalities, including facial recordings and physiological signals such as electroencephalography and electromyography, were also reviewed [ 5 , 8 ]. There have also been studies focusing on deep learning approaches [ 11 ]. AI was applied in children and infant pain evaluation as well [ 15 , 16 ]. However, no study has focused on pain intensity measurement, and no comparison of test accuracy results has been made.
Current AI applications in pain research can be categorized into 3 types: pain assessment, pain prediction and decision support, and pain self-management [ 14 ]. We consider accurate and automatic pain assessment to be the most important area and the foundation of future pain research. In this study, we performed a systematic review and meta-analysis to assess the diagnostic performance of current publications for multilevel pain evaluation.
This study was registered with PROSPERO (International Prospective Register of Systematic Reviews; CRD42023418181) and carried out strictly following the PRISMA (Preferred Reporting Items for Systematic Reviews and Meta-Analyses) guidelines [ 17 ] .
Study Eligibility
Studies that reported AI techniques for multiclass pain intensity classification were eligible. Records including nonhuman or infant participants or 2-class pain detection were excluded. Only studies using facial images of the test participants were accepted. Clinically used pain assessment tools, such as the visual analog scale (VAS) and numerical rating scale (NRS), and other pain intensity indicators, were rejected in the meta-analysis. Textbox 1 presents the eligibility criteria.
Study characteristics and inclusion criteria
- Participants: children and adults aged 12 months or older
- Setting: no restrictions
- Index test: artificial intelligence models that measure pain intensity from facial images
- Reference standard: no restrictions for systematic review; Prkachin and Solomon pain intensity score for meta-analysis
- Study design: no need to specify
Study characteristics and exclusion criteria
- Participants: infants aged 12 months or younger and animal subjects
- Setting: no need to specify
- Index test: studies that use other information such as physiological signals
- Reference standard: other pain evaluation tools, e.g., NRS, VAS, were excluded from meta-analysis
- Study design: reviews
Report characteristics and inclusion criteria
- Year: published between January 1, 2012, and September 30, 2023
- Language: English only
- Publication status: published
- Test accuracy metrics: no restrictions for systematic reviews; studies that reported contingency tables were included for meta-analysis
Report characteristics and exclusion criteria
- Year: no need to specify
- Language: no need to specify
- Publication status: preprints not accepted
- Test accuracy metrics: studies that reported insufficient metrics were excluded from meta-analysis
Search Strategy
In this systematic review, databases including PubMed, Embase, IEEE, Web of Science, and the Cochrane Library were searched until December 2022, and no restrictions were applied. Keywords were “artificial intelligence” AND “pain recognition.” Multimedia Appendix 1 shows the detailed search strategy.
Data Extraction
A total of 2 viewers screened titles and abstracts and selected eligible records independently to assess eligibility, and disagreements were solved by discussion with a third collaborator. A consentient data extraction sheet was prespecified and used to summarize study characteristics independently. Table S5 in Multimedia Appendix 1 shows the detailed items and explanations for data extraction. Diagnostic accuracy data were extracted into contingency tables, including true positives, false positives, false negatives, and true negatives. The data were used to calculate the pooled diagnostic performance of the different models. Some studies included multiple models, and these models were considered independent of each other.
Study Quality Assessment
All included studies were independently assessed by 2 viewers using the Quality Assessment of Diagnostic Accuracy Studies 2 (QUADAS-2) tool [ 18 ]. QUADAS-2 assesses bias risk across 4 domains, which are patient selection, index test, reference standard, and flow and timing. The first 3 domains are also assessed for applicability concerns. In the systematic review, a specific extension of QUADAS-2, namely, QUADAS-AI, was used to specify the signaling questions [ 19 ].
Meta-Analysis
Meta-analyses were conducted between different AI models. Models with different algorithms or training data were considered different. To evaluate the performance differences between models, the contingency tables during model validation were extracted. Studies that did not report enough diagnostic accuracy data were excluded from meta-analysis.
Hierarchical summary receiver operating characteristic (SROC) curves were fitted to evaluate the diagnostic performance of AI models. These curves were plotted with 95% CIs and prediction regions around averaged sensitivity, specificity, and area under the curve estimates. Heterogeneity was assessed visually by forest plots. A funnel plot was constructed to evaluate the risk of bias.
Subgroup meta-analyses were conducted to evaluate the performance differences at both the model level and task level, and subgroups were created based on different tasks and the proportion of positive and negative samples.
All statistical analyses and plots were produced using RStudio (version 4.2.2; R Core Team) and the R package meta4diag (version 2.1.1; Guo J and Riebler A) [ 20 ].
Study Selection and Included Study Characteristics
A flow diagram representing the study selection process is shown in ( Figure 1 ). After removing 1039 duplicates, the titles and abstracts of a total of 5653 papers were screened, and the percentage agreement of title or abstract screening was 97%. After screening, 51 full-text reports were assessed for eligibility, among which 45 reports were included in the systematic review [ 21 - 65 ]. The percentage agreement of the full-text review was 87%. In 40 of the included studies, contingency tables could not be made. Meta-analyses were conducted based on 8 AI models extracted from 6 studies. Individual study characteristics included in the systematic review are provided in Tables 1 and 2 . The facial feature extraction method can be categorized into 2 classes: geometrical features (GFs) and deep features (DFs). One typical method of extracting GFs is to calculate the distance between facial landmarks. DFs are usually extracted by convolution operations. A total of 20 studies included temporal information, but most of them (18) extracted temporal information through the 3D convolution of video sequences. Feature transformation was also commonly applied to reduce the time for training or fuse features extracted by different methods before inputting them into the classifier. For classifiers, support vector machines (SVMs) and convolutional neural networks (CNNs) were mostly used. Table 1 presents the model designs of the included studies.

a No temporal features are shown by – symbol, time information extracted from 2 images at different time by +, and deep temporal features extracted through the convolution of video sequences by ++.
b SVM: support vector machine.
c GF: geometric feature.
d GMM: gaussian mixture model.
e TPS: thin plate spline.
f DML: distance metric learning.
g MDML: multiview distance metric learning.
h AAM: active appearance model.
i RVR: relevance vector regressor.
j PSPI: Prkachin and Solomon pain intensity.
k I-FES: individual facial expressiveness score.
l LSTM: long short-term memory.
m HCRF: hidden conditional random field.
n GLMM: generalized linear mixed model.
o VLAD: vector of locally aggregated descriptor.
p SVR: support vector regression.
q MDS: multidimensional scaling.
r ELM: extreme learning machine.
s Labeled to distinguish different architectures of ensembled deep learning models.
t DCNN: deep convolutional neural network.
u GSM: gaussian scale mixture.
v DOML: distance ordering metric learning.
w LIAN: locality and identity aware network.
x BiLSTM: bidirectional long short-term memory.
a UNBC: University of Northern British Columbia-McMaster shoulder pain expression archive database.
b LOSO: leave one subject out cross-validation.
c ICC: intraclass correlation coefficient.
d CT: contingency table.
e AUC: area under the curve.
f MSE: mean standard error.
g PCC: Pearson correlation coefficient.
h RMSE: root mean standard error.
i MAE: mean absolute error.
j ICC: intraclass coefficient.
k CCC: concordance correlation coefficient.
l Reported both external and internal validation results and summarized as intervals.
Table 2 summarizes the characteristics of model training and validation. Most studies used publicly available databases, for example, the University of Northern British Columbia-McMaster shoulder pain expression archive database [ 57 ]. Table S4 in Multimedia Appendix 1 summarizes the public databases. A total of 7 studies used self-prepared databases. Frames from video sequences were the most used test objects, as 37 studies output frame-level pain intensity, while few measure pain intensity from video sequences or photos. It was common that a study redefined pain levels to have fewer classes than ground-truth labels. For model validation, cross-validation and leave-one-subject-out validation were commonly used. Only 3 studies performed external validation. For reporting test accuracies, different evaluation metrics were used, including sensitivity, specificity, mean absolute error (MAE), mean standard error (MSE), Pearson correlation coefficient (PCC), and intraclass coefficient (ICC).
Methodological Quality of Included Studies
Table S2 in Multimedia Appendix 1 presents the study quality summary, as assessed by QUADAS-2. There was a risk of bias in all studies, specifically in terms of patient selection, caused by 2 issues. First, the training data are highly imbalanced, and any method to adjust the data distribution may introduce bias. Next, the QUADAS-AI correspondence letter [ 19 ] specifies that preprocessing of images that changes the image size or resolution may introduce bias. However, the applicability concern is low, as the images properly represent the feeling of pain. Studies that used cross-fold validation or leave-one-out cross-validation were considered to have a low risk of bias. Although the Prkachin and Solomon pain intensity (PSPI) score was used by most of the studies, its ability to represent individual pain levels was not clinically validated; as such, the risk of bias and applicability concerns were considered high when the PSPI score was used as the index test. As an advantage of computer vision techniques, the time interval between the index tests was short and was assessed as having a low risk of bias. Risk proportions are shown in Figure 2 . For all 315 entries, 39% (124) were assessed as high-risk. In total, 5 studies had the lowest risk of bias, with 6 domains assessed as low risk [ 26 , 27 , 31 , 32 , 59 ].

Pooled Performance of Included Models
In 6 studies included in the meta-analysis, there were 8 different models. The characteristics of these models are summarized in Table S1 in Multimedia Appendix 2 [ 23 , 24 , 26 , 32 , 41 , 57 ]. Classification of PSPI scores greater than 0, 2, 3, 6, and 9 was selected and considered as different tasks to create contingency tables. The test performance is shown in Figure 3 as hierarchical SROC curves; 27 contingency tables were extracted from 8 models. The sensitivity, specificity, and LDOR were calculated, and the combined sensitivity was 98% (95% CI 96%-99%), the specificity was 98% (95% CI 97%-99%), the LDOR was 7.99 (95% CI 6.73-9.31) and the AUC was 0.99 (95% CI 0.99-1).

Subgroup Analysis
In this study, subgroup analysis was conducted to investigate the performance differences within models. A total of 8 models were separated and summarized as a forest plot in Multimedia Appendix 3 [ 23 , 24 , 26 , 32 , 41 , 57 ]. For model 1, the pooled sensitivity, specificity, and LDOR were 95% (95% CI 86%-99%), 99% (95% CI 98%-100%), and 8.38 (95% CI 6.09-11.19), respectively. For model 2, the pooled sensitivity, specificity, and LDOR were 94% (95% CI 84%-99%), 95% (95% CI 88%-99%), and 6.23 (95% CI 3.52-9.04), respectively. For model 3, the pooled sensitivity, specificity, and LDOR were 100% (95% CI 99%-100%), 100% (95% CI 99%-100%), and 11.55% (95% CI 8.82-14.43), respectively. For model 4, the pooled sensitivity, specificity, and LDOR were 83% (95% CI 43%-99%), 94% (95% CI 79%-99%), and 5.14 (95% CI 0.93-9.31), respectively. For model 5, the pooled sensitivity, specificity, and LDOR were 92% (95% CI 68%-99%), 94% (95% CI 78%-99%), and 6.12 (95% CI 1.82-10.16), respectively. For model 6, the pooled sensitivity, specificity, and LDOR were 94% (95% CI 74%-100%), 94% (95% CI 78%-99%), and 6.59 (95% CI 2.21-11.13), respectively. For model 7, the pooled sensitivity, specificity, and LDOR were 98% (95% CI 90%-100%), 97% (95% CI 87%-100%), and 8.31 (95% CI 4.3-12.29), respectively. For model 8, the pooled sensitivity, specificity, and LDOR were 98% (95% CI 93%-100%), 97% (95% CI 88%-100%), and 8.65 (95% CI 4.84-12.67), respectively.
Heterogeneity Analysis
The meta-analysis results indicated that AI models are applicable for estimating pain intensity from facial images. However, extreme heterogeneity existed within the models except for models 3 and 5, which were proposed by Rathee and Ganotra [ 24 ] and Semwal and Londhe [ 32 ]. A funnel plot is presented in Figure 4 . A high risk of bias was observed.

Pain management has long been a critical problem in clinical practice, and the use of AI may be a solution. For acute pain management, automatic measurement of pain can reduce the burden on caregivers and provide timely warnings. For chronic pain management, as specified by Glare et al [ 2 ], further research is needed, and measurements of pain presence, intensity, and quality are one of the issues to be solved for chronic pain studies. Computer vision could improve pain monitoring through real-time detection for clinical use and data recording for prospective pain studies. To our knowledge, this is the first meta-analysis dedicated to AI performance in multilevel pain level classification.
In this study, one model’s performance at specific pain levels was described by stacking multiple classes into one to make each task a binary classification problem. After careful selection in both the medical and engineering databases, we observed promising results of AI in evaluating multilevel pain intensity through facial images, with high sensitivity (98%), specificity (98%), LDOR (7.99), and AUC (0.99). It is reasonable to believe that AI can accurately evaluate pain intensity from facial images. Moreover, the study quality and risk of bias were evaluated using an adapted QUADAS-2 assessment tool, which is a strength of this study.
To investigate the source of heterogeneity, it was assumed that a well-designed model should have familiar size effects regarding different levels, and a subgroup meta-analysis was conducted. The funnel and forest plots exhibited extreme heterogeneity. The model’s performance at specific pain levels was described and summarized by a forest plot. Within-model heterogeneity was observed in Multimedia Appendix 3 [ 23 , 24 , 26 , 32 , 41 , 57 ] except for 2 models. Models 3 and 5 were different in many aspects, including their algorithms and validation methods, but were both trained with a relatively small data set, and the proportion of positive and negative classes was relatively close to 1. Because training with imbalanced data is a critical problem in computer vision studies [ 66 ], for example, in the University of Northern British Columbia-McMaster pain data set, fewer than 10 frames out of 48,398 had a PSPI score greater than 13. Here, we emphasized that imbalanced data sets are one major cause of heterogeneity, resulting in the poorer performance of AI algorithms.
We tentatively propose a method to minimize the effect of training with imbalanced data by stacking multiple classes into one class, which is already presented in studies included in the systematic review [ 26 , 32 , 42 , 57 ]. Common methods to minimize bias include resampling and data augmentation [ 66 ]. This proposed method is used in the meta-analysis to compare the test results of different studies as well. The stacking method is available when classes are only different in intensity. A disadvantage of combined classes is that the model would be insufficient in clinical practice when the number of classes is low. Commonly used pain evaluation tools, such as VAS, have 10 discrete levels. It is recommended that future studies set the number of pain levels to be at least 10 for model training.
This study is limited for several reasons. First, insufficient data were included because different performance metrics (mean standard error and mean average error) were used in most studies, which could not be summarized into a contingency table. To create a contingency table that can be included in a meta-analysis, the study should report the following: the number of objects used in each pain class for model validation, and the accuracy, sensitivity, specificity, and F 1 -score for each pain class. This table cannot be created if a study reports the MAE, PCC, and other commonly used metrics in AI development. Second, a small study effect was observed in the funnel plot, and the heterogeneity could not be minimized. Another limitation is that the PSPI score is not clinically validated and is not the only tool that assesses pain from facial expressions. There are other clinically validated pain intensity assessment methods, such as the Faces Pain Scale-revised, Wong-Baker Faces Pain Rating Scale, and Oucher Scale [ 3 ]. More databases could be created based on the above-mentioned tools. Finally, AI-assisted pain assessments were supposed to cover larger populations, including incommunicable patients, for example, patients with dementia or patients with masked faces. However, only 1 study considered patients with dementia, which was also caused by limited databases [ 50 ].
AI is a promising tool that can help in pain research in the future. In this systematic review and meta-analysis, one approach using computer vision was investigated to measure pain intensity from facial images. Despite some risk of bias and applicability concerns, CV models can achieve excellent test accuracy. Finally, more CV studies in pain estimation, reporting accuracy in contingency tables, and more pain databases are encouraged for future studies. Specifically, the creation of a balanced public database that contains not only healthy but also nonhealthy participants should be prioritized. The recording process would be better in a clinical environment. Then, it is recommended that researchers report the validation results in terms of accuracy, sensitivity, specificity, or contingency tables, as well as the number of objects for each pain class, for the inclusion of a meta-analysis.
Acknowledgments
WL, AH, and CW contributed to the literature search and data extraction. JH and YY wrote the first draft of the manuscript. All authors contributed to the conception and design of the study, the risk of bias evaluation, data analysis and interpretation, and contributed to and approved the final version of the manuscript.
Data Availability
The data sets generated during and analyzed during this study are available in the Figshare repository [ 67 ].
Conflicts of Interest
None declared.
PRISMA checklist, risk of bias summary, search strategy, database summary and reported items and explanations.
Study performance summary.
Forest plot presenting pooled performance of subgroups in meta-analysis.
- Raja SN, Carr DB, Cohen M, Finnerup NB, Flor H, Gibson S, et al. The revised International Association for the Study of Pain definition of pain: concepts, challenges, and compromises. Pain. 2020;161(9):1976-1982. [ FREE Full text ] [ CrossRef ] [ Medline ]
- Glare P, Aubrey KR, Myles PS. Transition from acute to chronic pain after surgery. Lancet. 2019;393(10180):1537-1546. [ CrossRef ] [ Medline ]
- Chou R, Gordon DB, de Leon-Casasola OA, Rosenberg JM, Bickler S, Brennan T, et al. Management of postoperative pain: a clinical practice guideline from the American Pain Society, the American Society of Regional Anesthesia and Pain Medicine, and the American Society of Anesthesiologists' Committee on Regional Anesthesia, Executive Committee, and Administrative Council. J Pain. 2016;17(2):131-157. [ FREE Full text ] [ CrossRef ] [ Medline ]
- Hassan T, Seus D, Wollenberg J, Weitz K, Kunz M, Lautenbacher S, et al. Automatic detection of pain from facial expressions: a survey. IEEE Trans Pattern Anal Mach Intell. 2021;43(6):1815-1831. [ CrossRef ] [ Medline ]
- Mussigmann T, Bardel B, Lefaucheur JP. Resting-State Electroencephalography (EEG) biomarkers of chronic neuropathic pain. A systematic review. Neuroimage. 2022;258:119351. [ FREE Full text ] [ CrossRef ] [ Medline ]
- Moscato S, Cortelli P, Chiari L. Physiological responses to pain in cancer patients: a systematic review. Comput Methods Programs Biomed. 2022;217:106682. [ FREE Full text ] [ CrossRef ] [ Medline ]
- Thiam P, Hihn H, Braun DA, Kestler HA, Schwenker F. Multi-modal pain intensity assessment based on physiological signals: a deep learning perspective. Front Physiol. 2021;12:720464. [ FREE Full text ] [ CrossRef ] [ Medline ]
- Rojas RF, Brown N, Waddington G, Goecke R. A systematic review of neurophysiological sensing for the assessment of acute pain. NPJ Digit Med. 2023;6(1):76. [ FREE Full text ] [ CrossRef ] [ Medline ]
- Mansutti I, Tomé-Pires C, Chiappinotto S, Palese A. Facilitating pain assessment and communication in people with deafness: a systematic review. BMC Public Health. 2023;23(1):1594. [ FREE Full text ] [ CrossRef ] [ Medline ]
- El-Tallawy SN, Ahmed RS, Nagiub MS. Pain management in the most vulnerable intellectual disability: a review. Pain Ther. 2023;12(4):939-961. [ FREE Full text ] [ CrossRef ] [ Medline ]
- Gkikas S, Tsiknakis M. Automatic assessment of pain based on deep learning methods: a systematic review. Comput Methods Programs Biomed. 2023;231:107365. [ FREE Full text ] [ CrossRef ] [ Medline ]
- Borna S, Haider CR, Maita KC, Torres RA, Avila FR, Garcia JP, et al. A review of voice-based pain detection in adults using artificial intelligence. Bioengineering (Basel). 2023;10(4):500. [ FREE Full text ] [ CrossRef ] [ Medline ]
- De Sario GD, Haider CR, Maita KC, Torres-Guzman RA, Emam OS, Avila FR, et al. Using AI to detect pain through facial expressions: a review. Bioengineering (Basel). 2023;10(5):548. [ FREE Full text ] [ CrossRef ] [ Medline ]
- Zhang M, Zhu L, Lin SY, Herr K, Chi CL, Demir I, et al. Using artificial intelligence to improve pain assessment and pain management: a scoping review. J Am Med Inform Assoc. 2023;30(3):570-587. [ FREE Full text ] [ CrossRef ] [ Medline ]
- Hughes JD, Chivers P, Hoti K. The clinical suitability of an artificial intelligence-enabled pain assessment tool for use in infants: feasibility and usability evaluation study. J Med Internet Res. 2023;25:e41992. [ FREE Full text ] [ CrossRef ] [ Medline ]
- Fang J, Wu W, Liu J, Zhang S. Deep learning-guided postoperative pain assessment in children. Pain. 2023;164(9):2029-2035. [ FREE Full text ] [ CrossRef ] [ Medline ]
- Page MJ, McKenzie JE, Bossuyt PM, Boutron I, Hoffmann TC, Mulrow CD, et al. The PRISMA 2020 statement: an updated guideline for reporting systematic reviews. BMJ. 2021;372:n71. [ FREE Full text ] [ CrossRef ] [ Medline ]
- Whiting PF, Rutjes AWS, Westwood ME, Mallett S, Deeks JJ, Reitsma JB, et al. QUADAS-2: a revised tool for the quality assessment of diagnostic accuracy studies. Ann Intern Med. 2011;155(8):529-536. [ FREE Full text ] [ CrossRef ] [ Medline ]
- Sounderajah V, Ashrafian H, Rose S, Shah NH, Ghassemi M, Golub R, et al. A quality assessment tool for artificial intelligence-centered diagnostic test accuracy studies: QUADAS-AI. Nat Med. 2021;27(10):1663-1665. [ FREE Full text ] [ CrossRef ] [ Medline ]
- Guo J, Riebler A. meta4diag: Bayesian bivariate meta-analysis of diagnostic test studies for routine practice. J Stat Soft. 2018;83(1):1-31. [ CrossRef ]
- Hammal Z, Cohn JF. Automatic detection of pain intensity. Proc ACM Int Conf Multimodal Interact. 2012;2012:47-52. [ FREE Full text ] [ CrossRef ] [ Medline ]
- Adibuzzaman M, Ostberg C, Ahamed S, Povinelli R, Sindhu B, Love R, et al. Assessment of pain using facial pictures taken with a smartphone. 2015. Presented at: 2015 IEEE 39th Annual Computer Software and Applications Conference; July 01-05, 2015;726-731; Taichung, Taiwan. [ CrossRef ]
- Majumder A, Dutta S, Behera L, Subramanian VK. Shoulder pain intensity recognition using Gaussian mixture models. 2015. Presented at: 2015 IEEE International WIE Conference on Electrical and Computer Engineering (WIECON-ECE); December 19-20, 2015;130-134; Dhaka, Bangladesh. [ CrossRef ]
- Rathee N, Ganotra D. A novel approach for pain intensity detection based on facial feature deformations. J Vis Commun Image Represent. 2015;33:247-254. [ CrossRef ]
- Sikka K, Ahmed AA, Diaz D, Goodwin MS, Craig KD, Bartlett MS, et al. Automated assessment of children's postoperative pain using computer vision. Pediatrics. 2015;136(1):e124-e131. [ FREE Full text ] [ CrossRef ] [ Medline ]
- Rathee N, Ganotra D. Multiview distance metric learning on facial feature descriptors for automatic pain intensity detection. Comput Vis Image Und. 2016;147:77-86. [ CrossRef ]
- Zhou J, Hong X, Su F, Zhao G. Recurrent convolutional neural network regression for continuous pain intensity estimation in video. 2016. Presented at: 2016 IEEE Conference on Computer Vision and Pattern Recognition Workshops (CVPRW); June 26-July 01, 2016; Las Vegas, NV. [ CrossRef ]
- Egede J, Valstar M, Martinez B. Fusing deep learned and hand-crafted features of appearance, shape, and dynamics for automatic pain estimation. 2017. Presented at: 2017 12th IEEE International Conference on Automatic Face & Gesture Recognition (FG 2017); May 30-June 03, 2017;689-696; Washington, DC. [ CrossRef ]
- Martinez DL, Rudovic O, Picard R. Personalized automatic estimation of self-reported pain intensity from facial expressions. 2017. Presented at: 2017 IEEE Conference on Computer Vision and Pattern Recognition Workshops (CVPRW); July 21-26, 2017;2318-2327; Honolulu, HI. [ CrossRef ]
- Bourou D, Pampouchidou A, Tsiknakis M, Marias K, Simos P. Video-based pain level assessment: feature selection and inter-subject variability modeling. 2018. Presented at: 2018 41st International Conference on Telecommunications and Signal Processing (TSP); July 04-06, 2018;1-6; Athens, Greece. [ CrossRef ]
- Haque MA, Bautista RB, Noroozi F, Kulkarni K, Laursen C, Irani R. Deep multimodal pain recognition: a database and comparison of spatio-temporal visual modalities. 2018. Presented at: 2018 13th IEEE International Conference on Automatic Face & Gesture Recognition (FG 2018); May 15-19, 2018;250-257; Xi'an, China. [ CrossRef ]
- Semwal A, Londhe ND. Automated pain severity detection using convolutional neural network. 2018. Presented at: 2018 International Conference on Computational Techniques, Electronics and Mechanical Systems (CTEMS); December 21-22, 2018;66-70; Belgaum, India. [ CrossRef ]
- Tavakolian M, Hadid A. Deep binary representation of facial expressions: a novel framework for automatic pain intensity recognition. 2018. Presented at: 2018 25th IEEE International Conference on Image Processing (ICIP); October 07-10, 2018;1952-1956; Athens, Greece. [ CrossRef ]
- Tavakolian M, Hadid A. Deep spatiotemporal representation of the face for automatic pain intensity estimation. 2018. Presented at: 2018 24th International Conference on Pattern Recognition (ICPR); August 20-24, 2018;350-354; Beijing, China. [ CrossRef ]
- Wang J, Sun H. Pain intensity estimation using deep spatiotemporal and handcrafted features. IEICE Trans Inf & Syst. 2018;E101.D(6):1572-1580. [ CrossRef ]
- Bargshady G, Soar J, Zhou X, Deo RC, Whittaker F, Wang H. A joint deep neural network model for pain recognition from face. 2019. Presented at: 2019 IEEE 4th International Conference on Computer and Communication Systems (ICCCS); February 23-25, 2019;52-56; Singapore. [ CrossRef ]
- Casti P, Mencattini A, Comes MC, Callari G, Di Giuseppe D, Natoli S, et al. Calibration of vision-based measurement of pain intensity with multiple expert observers. IEEE Trans Instrum Meas. 2019;68(7):2442-2450. [ CrossRef ]
- Lee JS, Wang CW. Facial pain intensity estimation for ICU patient with partial occlusion coming from treatment. 2019. Presented at: BIBE 2019; The Third International Conference on Biological Information and Biomedical Engineering; June 20-22, 2019;1-4; Hangzhou, China.
- Saha AK, Ahsan GMT, Gani MO, Ahamed SI. Personalized pain study platform using evidence-based continuous learning tool. 2019. Presented at: 2019 IEEE 43rd Annual Computer Software and Applications Conference (COMPSAC); July 15-19, 2019;490-495; Milwaukee, WI. [ CrossRef ]
- Tavakolian M, Hadid A. A spatiotemporal convolutional neural network for automatic pain intensity estimation from facial dynamics. Int J Comput Vis. 2019;127(10):1413-1425. [ FREE Full text ] [ CrossRef ]
- Bargshady G, Zhou X, Deo RC, Soar J, Whittaker F, Wang H. Ensemble neural network approach detecting pain intensity from facial expressions. Artif Intell Med. 2020;109:101954. [ CrossRef ] [ Medline ]
- Bargshady G, Zhou X, Deo RC, Soar J, Whittaker F, Wang H. Enhanced deep learning algorithm development to detect pain intensity from facial expression images. Expert Syst Appl. 2020;149:113305. [ CrossRef ]
- Dragomir MC, Florea C, Pupezescu V. Automatic subject independent pain intensity estimation using a deep learning approach. 2020. Presented at: 2020 International Conference on e-Health and Bioengineering (EHB); October 29-30, 2020;1-4; Iasi, Romania. [ CrossRef ]
- Huang D, Xia Z, Mwesigye J, Feng X. Pain-attentive network: a deep spatio-temporal attention model for pain estimation. Multimed Tools Appl. 2020;79(37-38):28329-28354. [ CrossRef ]
- Mallol-Ragolta A, Liu S, Cummins N, Schuller B. A curriculum learning approach for pain intensity recognition from facial expressions. 2020. Presented at: 2020 15th IEEE International Conference on Automatic Face and Gesture Recognition (FG 2020); November 16-20, 2020;829-833; Buenos Aires, Argentina. [ CrossRef ]
- Peng X, Huang D, Zhang H. Pain intensity recognition via multi‐scale deep network. IET Image Process. 2020;14(8):1645-1652. [ FREE Full text ] [ CrossRef ]
- Tavakolian M, Lopez MB, Liu L. Self-supervised pain intensity estimation from facial videos via statistical spatiotemporal distillation. Pattern Recognit Lett. 2020;140:26-33. [ CrossRef ]
- Xu X, de Sa VR. Exploring multidimensional measurements for pain evaluation using facial action units. 2020. Presented at: 2020 15th IEEE International Conference on Automatic Face and Gesture Recognition (FG 2020); November 16-20, 2020;786-792; Buenos Aires, Argentina. [ CrossRef ]
- Pikulkaew K, Boonchieng W, Boonchieng E, Chouvatut V. 2D facial expression and movement of motion for pain identification with deep learning methods. IEEE Access. 2021;9:109903-109914. [ CrossRef ]
- Rezaei S, Moturu A, Zhao S, Prkachin KM, Hadjistavropoulos T, Taati B. Unobtrusive pain monitoring in older adults with dementia using pairwise and contrastive training. IEEE J Biomed Health Inform. 2021;25(5):1450-1462. [ CrossRef ] [ Medline ]
- Semwal A, Londhe ND. S-PANET: a shallow convolutional neural network for pain severity assessment in uncontrolled environment. 2021. Presented at: 2021 IEEE 11th Annual Computing and Communication Workshop and Conference (CCWC); January 27-30, 2021;0800-0806; Las Vegas, NV. [ CrossRef ]
- Semwal A, Londhe ND. ECCNet: an ensemble of compact convolution neural network for pain severity assessment from face images. 2021. Presented at: 2021 11th International Conference on Cloud Computing, Data Science & Engineering (Confluence); January 28-29, 2021;761-766; Noida, India. [ CrossRef ]
- Szczapa B, Daoudi M, Berretti S, Pala P, Del Bimbo A, Hammal Z. Automatic estimation of self-reported pain by interpretable representations of motion dynamics. 2021. Presented at: 2020 25th International Conference on Pattern Recognition (ICPR); January 10-15, 2021;2544-2550; Milan, Italy. [ CrossRef ]
- Ting J, Yang YC, Fu LC, Tsai CL, Huang CH. Distance ordering: a deep supervised metric learning for pain intensity estimation. 2021. Presented at: 2021 20th IEEE International Conference on Machine Learning and Applications (ICMLA); December 13-16, 2021;1083-1088; Pasadena, CA. [ CrossRef ]
- Xin X, Li X, Yang S, Lin X, Zheng X. Pain expression assessment based on a locality and identity aware network. IET Image Process. 2021;15(12):2948-2958. [ FREE Full text ] [ CrossRef ]
- Alghamdi T, Alaghband G. Facial expressions based automatic pain assessment system. Appl Sci. 2022;12(13):6423. [ FREE Full text ] [ CrossRef ]
- Barua PD, Baygin N, Dogan S, Baygin M, Arunkumar N, Fujita H, et al. Automated detection of pain levels using deep feature extraction from shutter blinds-based dynamic-sized horizontal patches with facial images. Sci Rep. 2022;12(1):17297. [ FREE Full text ] [ CrossRef ] [ Medline ]
- Fontaine D, Vielzeuf V, Genestier P, Limeux P, Santucci-Sivilotto S, Mory E, et al. Artificial intelligence to evaluate postoperative pain based on facial expression recognition. Eur J Pain. 2022;26(6):1282-1291. [ CrossRef ] [ Medline ]
- Hosseini E, Fang R, Zhang R, Chuah CN, Orooji M, Rafatirad S, et al. Convolution neural network for pain intensity assessment from facial expression. 2022. Presented at: 2022 44th Annual International Conference of the IEEE Engineering in Medicine & Biology Society (EMBC); July 11-15, 2022;2697-2702; Glasgow, Scotland. [ CrossRef ]
- Huang Y, Qing L, Xu S, Wang L, Peng Y. HybNet: a hybrid network structure for pain intensity estimation. Vis Comput. 2021;38(3):871-882. [ CrossRef ]
- Islamadina R, Saddami K, Oktiana M, Abidin TF, Muharar R, Arnia F. Performance of deep learning benchmark models on thermal imagery of pain through facial expressions. 2022. Presented at: 2022 IEEE International Conference on Communication, Networks and Satellite (COMNETSAT); November 03-05, 2022;374-379; Solo, Indonesia. [ CrossRef ]
- Swetha L, Praiscia A, Juliet S. Pain assessment model using facial recognition. 2022. Presented at: 2022 6th International Conference on Intelligent Computing and Control Systems (ICICCS); May 25-27, 2022;1-5; Madurai, India. [ CrossRef ]
- Wu CL, Liu SF, Yu TL, Shih SJ, Chang CH, Mao SFY, et al. Deep learning-based pain classifier based on the facial expression in critically ill patients. Front Med (Lausanne). 2022;9:851690. [ FREE Full text ] [ CrossRef ] [ Medline ]
- Ismail L, Waseem MD. Towards a deep learning pain-level detection deployment at UAE for patient-centric-pain management and diagnosis support: framework and performance evaluation. Procedia Comput Sci. 2023;220:339-347. [ FREE Full text ] [ CrossRef ] [ Medline ]
- Vu MT, Beurton-Aimar M. Learning to focus on region-of-interests for pain intensity estimation. 2023. Presented at: 2023 IEEE 17th International Conference on Automatic Face and Gesture Recognition (FG); January 05-08, 2023;1-6; Waikoloa Beach, HI. [ CrossRef ]
- Kaur H, Pannu HS, Malhi AK. A systematic review on imbalanced data challenges in machine learning: applications and solutions. ACM Comput Surv. 2019;52(4):1-36. [ CrossRef ]
- Data for meta-analysis of pain assessment from facial images. Figshare. 2023. URL: https://figshare.com/articles/dataset/Data_for_Meta-Analysis_of_Pain_Assessment_from_Facial_Images/24531466/1 [accessed 2024-03-22]
Abbreviations
Edited by A Mavragani; submitted 26.07.23; peer-reviewed by M Arab-Zozani, M Zhang; comments to author 18.09.23; revised version received 08.10.23; accepted 28.02.24; published 12.04.24.
©Jian Huo, Yan Yu, Wei Lin, Anmin Hu, Chaoran Wu. Originally published in the Journal of Medical Internet Research (https://www.jmir.org), 12.04.2024.
This is an open-access article distributed under the terms of the Creative Commons Attribution License (https://creativecommons.org/licenses/by/4.0/), which permits unrestricted use, distribution, and reproduction in any medium, provided the original work, first published in the Journal of Medical Internet Research, is properly cited. The complete bibliographic information, a link to the original publication on https://www.jmir.org/, as well as this copyright and license information must be included.
assets/design/img/gavin-journals.png">

Gavin Journals
Manuscript preparation and submission guidelines, manuscript preparation guidelines.
To ensure the highest quality publication of your manuscript, please adhere to the following preparation guidelines:
General Formatting: Use a standard font (e.g., Times New Roman or Arial) in 12-point size. Include page numbers and Use 1-inch margins on all sides.
Sections: Organize your manuscript into the following sections: title page, abstract, introduction, materials and methods / case presentation, results, discussion, acknowledgments, references, tables, and figures.
Title Page: Include the title of the manuscript, the names and affiliations of all authors, the corresponding author's contact information, and a short running title. Read More
Abstract Provide an abstract of no more than 250 words that summarizes the purpose, methods, results, and conclusions of the study.
Keywords: Provide a list of 4 to 6 keywords that best represent the content of the manuscript.
Introduction: Clearly state the purpose and objectives of the study, provide relevant background information, and explain the significance of the research.
Materials and Methods: Describe the materials & methods used in the study in sufficient detail to allow others to replicate the research.
If Your Paper is Case Report
Case Presentation: The case presentation should include the patient's demographic information, medical history, presenting symptoms, and physical examination findings. Any relevant laboratory, radiographic or other diagnostic test results should also be included.
Results: Present the results of the study in a logical and concise manner, using appropriate statistical analyses.
Discussion: Interpret and discuss the results, and relate them to the study objectives and the existing literature.
Conclusions: The conclusion section of a manuscript serves as a final summary and reflection on the main findings and implications of the study. It allows the authors to highlight the significance of their work and provide a closing statement.
Acknowledgments: Acknowledge individuals or organizations that provided assistance or funding for the study.
Ethical Guidelines: Provide a statement of compliance with relevant ethical guidelines, including any necessary approvals from institutional review boards or ethics committees.
Conflict of Interest: Disclose any potential conflicts of interest that may influence the results or interpretations of the manuscript.
References: Use a standard citation format and follow our journal's citation style guidelines. Please ensure that all references are cited in the text and that the reference list is complete.
Tables and Figures: Present data in tables and figures as needed, and ensure that they are clear, concise, and easy to understand.
Supplementary Materials: Provide any supplementary materials such as data sets, videos, or images as separate files.
Proofreading: Ensure that your manuscript has been proofread for grammar, spelling, and punctuation errors.
Note: Format Preferably in Word Document (DOC, DOCX) Like Sample Manuscript Paper
By following these manuscript preparation guidelines, you can ensure that your manuscript is ready for submission to our journal. If you have any questions or concerns, please don't hesitate to contact us.
Article Type Manuscript Guidelines
- ● Editorial
- ● Research Article
- ● Case Report
- ● Review Article
- ● Opinion
- ● Short Communication
- ● Mini Review
- ● Letter to Editor
Manuscript Submission Guidelines
Thank you for your interest in submitting your manuscript to our journal. We welcome original research articles, reviews, case reports, and other types of articles related to our scope.
To ensure a smooth and efficient review process, please follow these manuscript submission guidelines:
Format: Please submit your manuscript in Microsoft Word or a compatible format. Use 12-point font, 1-inch margins, and Include page numbers. Figures and tables should be embedded within the text, or provided as separate files.
Cover Letter: Include a cover letter that explains the significance and originality of the study, and highlights the key findings of the manuscript.
Title Page: The title page should include the title of the manuscript, the names and affiliations of all authors, corresponding author's contact information, and a short running title.
Abstract: Provide an abstract of no more than 250 words that summarizes the purpose, materials & methods / case presentaion, results, and conclusions of the study.
Main Manuscript: Your manuscript should be structured with an introduction, materials & methods / case presentaion, results, and discussion sections. Acknowledgments, references, and any additional information should be provided at the end of the manuscript.
Supplementary Materials: Supplementary materials such as data sets, videos, or images should be provided as separate files.
Submitting Your Manuscript: Submit your manuscript through our Online Submission system. Please ensure that you have included all required documents and that your manuscript adheres to our journal's formatting and ethical guidelines.
If You have Images and Tables in Seperatly Archive all files in .zip Format By Using ZIP Converter and then Submit.
We look forward to receiving your manuscript and will ensure that it is reviewed promptly and fairly. If you have any questions or concerns, please don't hesitate to contact us at: [email protected]
Author Corrections: Suggestions from the authors, before or after the publication process, are acceptable and ensure that the corrections are clearly legible.
Manuscript Publication Process
Manuscript Preparation: Write your research/case report/review/other type manuscript following the guidelines provided by the target journal, ensuring proper formatting and adherence to specific requirements.
Journal Selection: Identify the most appropriate journal for your manuscript based on scope, audience, impact factor, and relevance to your research field.
Submission: Submit your manuscript to the chosen journal via our Online Submission system, providing author information, abstract, keywords, and supporting materials or data.
Editorial Review: The manuscript undergoes an initial review by the journal's editorial office to check adherence to guidelines, perform a plagiarism check, and evaluate its suitability for the journal.
Plagiarism Check: The submitted manuscript is screened for potential plagiarism using sophisticated software to ensure that proper credit is given to all sources.
Peer Review: If the manuscript passes the initial review, it is sent for peer review. Independent experts in the field (reviewers) evaluate the quality, methodology, significance, and validity of the research and provide feedback and recommendations.
Decision: The editor makes a decision based on the peer reviewers' feedback, which may include acceptance, minor/major revision, or rejection.
Revision and Resubmission: If revisions are requested, address the reviewers' comments and submit a revised version along with a cover letter explaining the changes made.
Peer Review (Revised Manuscript): The revised manuscript may go through another round of peer review to ensure the addressed issues have been appropriately resolved.
Acceptance and Production: Once the revised manuscript is deemed satisfactory, the editor accepts it for publication. The manuscript undergoes copyediting, typesetting, and proofreading by the journal's production team.
Article Processing Charges (APCs): After acceptance, authors may be required to pay an Article Processing Charge to cover the costs associated with publishing the article. APCs vary depending on the journal and country.
Author Approval: Authors may be asked to review and approve the final version of the manuscript before publication.
Publication: The manuscript is published online or in print, assigned a digital object identifier (DOI), and made available to the public through the journal's website or other platforms.
Post-Publication: After publication, the article may receive further attention, citations, and feedback from the research community.
It's important to note that the publication process can vary between different journals and disciplines, and the specific details of each step may vary.
Manuscript Withdrawal Policy
Authors may wish to withdraw their manuscript after submitting it to Gavin Publishers. We understand that circumstances can arise that necessitate the withdrawal of a manuscript and we will work with authors to facilitate this process.
To withdraw a manuscript, the corresponding author must send a written request to the editorial office via email. The request should include the following information:
- ● The title of the manuscript
- ● The name of the corresponding author
- ● The reason for withdrawing the manuscript
Once the request has been received, the editorial office will confirm the withdrawal with the corresponding author and all other authors listed on the manuscript. If the manuscript has already been assigned to an editor or reviewers, they will be notified of the withdrawal.
If a manuscript is withdrawn after acceptance and before publication, the corresponding author will be asked to pay 50% of the invoice amount. Each and every manuscript submission costs us (Gavin Publishers) a certain amount along with overhead cost during processing. Once a manuscript's peer review process is completed (which can often happen within a week), and it has been worked into a journal issue, we have incurred costs on our end that need to be reimbursed.
Please note that authors are responsible for ensuring that their manuscript is ready for submission and has met all relevant ethical and publishing standards before submitting it for consideration. Withdrawal requests based on disputes among authors or between authors and their institutions regarding authorship, data ownership, or other issues cannot be considered.
We understand that withdrawing a manuscript can be a difficult decision for authors and we will do our best to accommodate their needs while also maintaining the integrity of our publication process. If you have any questions or concerns about manuscript withdrawal or article processing fees, please don't hesitate to contact us at: [email protected] .
Publication Proccess

Online Payments
We use cookies for a better experience. Read privacy policy . Manage your consent settings:
Manage Your Consent:

IMAGES
VIDEO
COMMENTS
Journal of Ophthalmology Research Reviews & Reports is an open access peer-reviewed journal from the publishers of Scientific Research and Community (SRC) which publishes articles in the form of written, video and power-point presentations from the arena of Ophthalmology Research Reviews & Reportsis. The journal focuses to build up accessing the complete content of articles freely from online ...
Result: A total of 12,516 patients attended the Ophthalmology out-patient clinic of the hospital during the 30- month study period (May 2014- Oct. 2016), 1,760 old and new patients (14.1%) were ...
Journal of Ophthalmology publishes original research articles and review articles related to the anatomy, physiology and diseases of the eye. ... See full report. Acceptance rate 10%. Submission to final decision 129 days. Acceptance to publication 18 days. CiteScore 3.400.
Eye Injuries in Children: About 199 Cases Collected to the Aristide Le Dantec Hospital in Dakar. Journal of Ophthalmology Research and Reports is a peer-reviewed open access journal that aims to publish original research articles, review articles and clinical studies related to ophthalmology, anatomy, physiology and eye diseases.
Journal of Ophthalmology Research Reviews & Reports. Archive. Year: 2024. Volume 5, Issue 4. Volume 5, Issue 3. Volume 5, Issue 2. Volume 5, Issue 1. Year: 2023. Volume 4, Issue 6. Volume 4, Issue 5. ... Journal Menu Journal Home Aims and Scope Call for Papers Editorial Board Inpress Current Issue Archive Journal Guidelines Submit Manuscript.
URL https://www.onlinescientificresearch.com/journal-of-ophthalmology-research-reviews-reports-home-jomrr.php. URL https://www.onlinescientificresearch.com/journal-of ...
Ophthalmology-focused publications accounted for less than 0.5 percent of the total COVID-19-related articles. Most of the articles were published in the Indian Journal of Ophthalmology, and the main publication type was "original article." Almost 88% of the publications were in English.
Journal of Ophthalmology Research and Reports is a peer-reviewed open access journal that aims to publish original research articles, review articles and clinical studies related to ophthalmology, anatomy, physiology and eye diseases.
About: Journal of ophthalmology research reviews & reports is an academic journal published by Scientific Research and Community Ltd. The journal publishes majorly in the area(s): Medicine & Ophthalmology. It has an ISSN identifier of 2754-4990. Over the lifetime, 15 publications have been published receiving 4 citations.
Volume - 1 (2016) Issue 1. Issue 2. Journal of Ophthalmology Research and Reports is a peer-reviewed open access journal that aims to publish original research articles, review articles and clinical studies related to ophthalmology, anatomy, physiology and eye diseases.
The LitCovid database was systematically reviewed for ophthalmology-focused COVID-19 articles. The quality of the evidence was assessed for articles investigating conjunctivitis in COVID-19 patients. There were 21,364 articles in LitCovid on June 12, 2020, of which 215 (1%) were ophthalmology-focused.
Ophthalmology Research and Reports is a peer-reviewed journal dedicated to advancing the field of ophthalmology by disseminating high-quality research and scholarly contributions. Our aim is to serve as a global platform for ophthalmologists, researchers, and healthcare professionals to share their expertise, insights, and innovations in the ...
Current Ophthalmology Reports is a source for expert review articles on the most significant recent developments in the field of ophthalmology, providing clear, insightful, balanced contributions to benefit those who diagnose, treat, manage, and prevent ocular conditions and diseases.. International authorities in ophthalmology serve as Section Editors, choosing key topics for which leading ...
Retina (n = 580, 5.54%), the BMJ Case Reports (n = 270, 2.58%), the Journal of Neuro-Ophthalmology: ... there were 16 original research articles and 9 review articles. The keywords involved in the articles are listed in Table 1, ... The subject categories of ophthalmology research were divided into two types: one was the traditional ...
Journal of Ophthalmology Research and Reports is a peer-reviewed open access journal that aims to publish original research articles, review articles and clinical studies related to ophthalmology, anatomy, physiology and eye diseases. ... Ophthalmology Research and Reports . ISSN: 2689-7407 Impact Factor: 2.58 ...
International Scientific Journal & Country Ranking. SCImago Institutions Rankings SCImago Media Rankings SCImago Iber SCImago Research Centers Ranking SCImago Graphica Ediciones Profesionales de la Información
Impact Factor: 1.023* Classification: Q4- H indexed. Citations Value: 84.5%. Acceptance Rate: 90%. Editor - in - Chief: Wenjuan Luo. Journal of Ophthalmology Research and Reports is an open access peer-reviewed journal from the publishers of Scientific Research and Community (SRC) which publishes articles in the form of written, video and power-point presentations from the arena of ...
Journal of Ophthalmology Research and Reports is a peer-reviewed open access journal that aims to publish original research articles, review articles and clinical studies related to ophthalmology, anatomy, physiology and eye diseases. ... Our dedicated team of reviewers ensures that each manuscript undergoes a rigorous peer-review process to ...
Journal of Ophthalmology Research Reviews & Reports Open Access Volume 3(1): 1-5 Keywords: Macula, Poison, Retina, Hydrogen, Energy ... Journal of Ophthalmology Research Reviews & Reports. SRC ...
Introduction The COVID-19 pandemic has initiated an unparalleled global vaccination campaign, raising concerns about the vaccine's effects on various health conditions, including the risk of corneal transplant rejection. This systematic review aimed to identify the relationship between COVID-19 vaccination and rejection of corneal transplant, filling a significant gap in the existing medical ...
Glaucoma is a progressive optic neuropathy and it is the main cause of irreversible blindness worldwide. Intraocular pressure (IOP) is the only modifiable one and trabeculectomy is commonly considered the surgical "gold standard" to decrease IOP.
Pentastomiasis is a rare zoonotic disease caused by the larval stages of pentastomid parasites. 1, 2 Most human infections occur in the tropics and subtropics. In Africa and Asia, the disease is often caused by Armillifer species, which have snakes as their final host, whereas Linguatula infections are cosmopolitan, with dogs as final hosts. 1, 2.
April 16, 2024. 0. TOPLINE: Botulinum toxin (BTX) and dermal fillers are safe as cosmetic and antiaging treatments in individuals with skin of color (SOC), with the largest amount of data ...
Ophthalmology Research Journals. Journal of Ophthalmology Research and Reports is a peer-reviewed open access journal that aims to publish original research articles, review articles and clinical studies related to ophthalmology, anatomy, physiology and eye diseases.
Journal of Ophthalmology Research Reviews & Reports Open Access ABSTRACT Diabetes mellitus is a group of metabolic diseases which are typified by the development of hyperglycaemia which do emanate from defects in insulin secretion, insulin action, or both.
Conclusions: This review summarizes recent evidence in automatic multilevel pain estimation from facial expressions and compared the test accuracy of results in a meta-analysis. ... Results: A total of 45 reports were included in the systematic review. The reported test accuracies ranged from 0.27-0.99, and the other metrics, including the mean ...
Manuscript Preparation: Write your research/case report/review/other type manuscript following the guidelines provided by the target journal, ensuring proper formatting and adherence to specific requirements. Journal Selection: Identify the most appropriate journal for your manuscript based on scope, audience, impact factor, and relevance to your research field.
Feature papers represent the most advanced research with significant potential for high impact in the field. A Feature Paper should be a substantial original Article that involves several techniques or approaches, provides an outlook for future research directions and describes possible research applications. ... Case Report and Review of ...