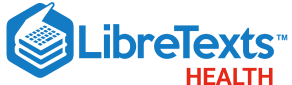
- school Campus Bookshelves
- menu_book Bookshelves
- perm_media Learning Objects
- login Login
- how_to_reg Request Instructor Account
- hub Instructor Commons
- Download Page (PDF)
- Download Full Book (PDF)
- Periodic Table
- Physics Constants
- Scientific Calculator
- Reference & Cite
- Tools expand_more
- Readability
selected template will load here
This action is not available.
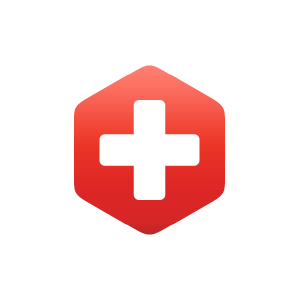

10.2: Exercises
- Last updated
- Save as PDF
- Page ID 52772
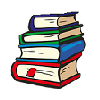
Lab 10 Exercise \(\PageIndex{1}\)
Identify the pats of a "neuron".
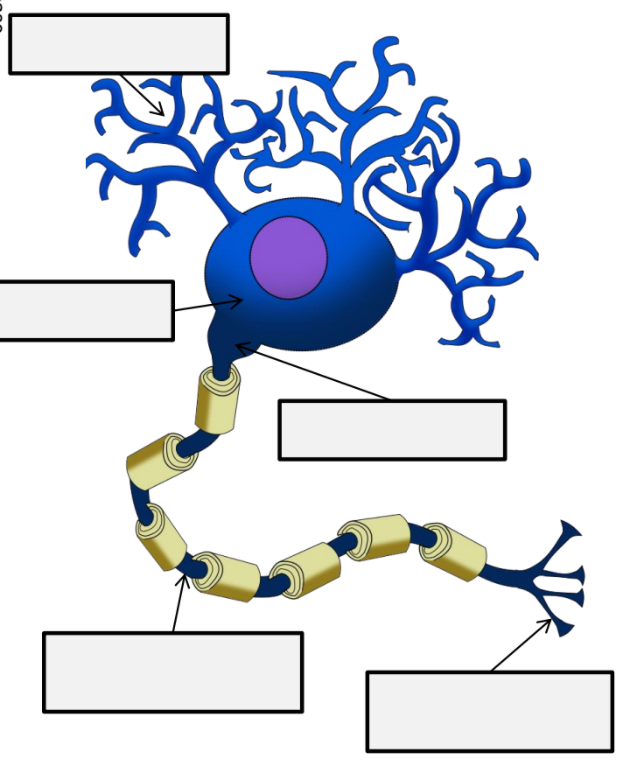
Lab 10 Exercise \(\PageIndex{2}\)
Label the following:, lab 10 exercise \(\pageindex{3}\), match these items or actions to their locations on a neuron: graded potentials, action.
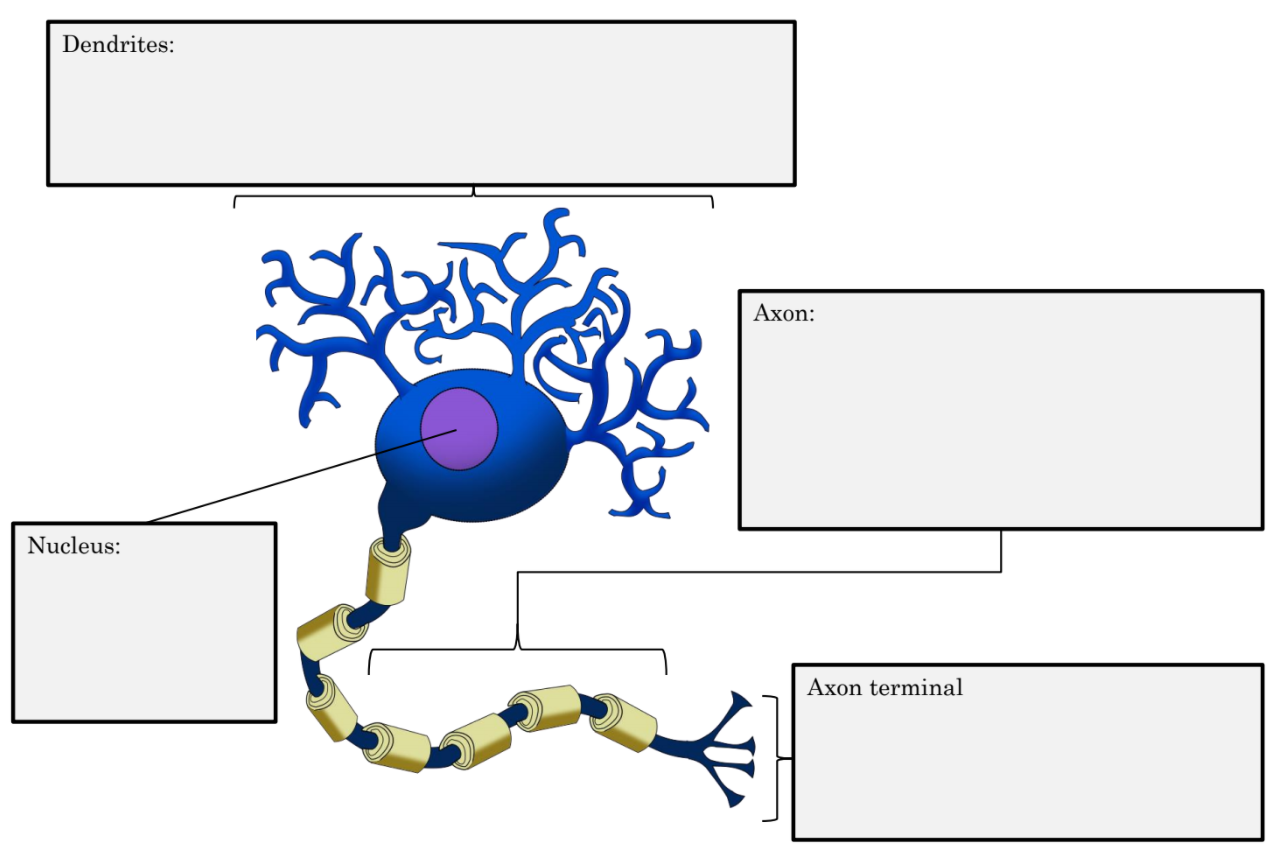
Lab 10 Exercise \(\PageIndex{4}\)
Label the following glia & related items: .
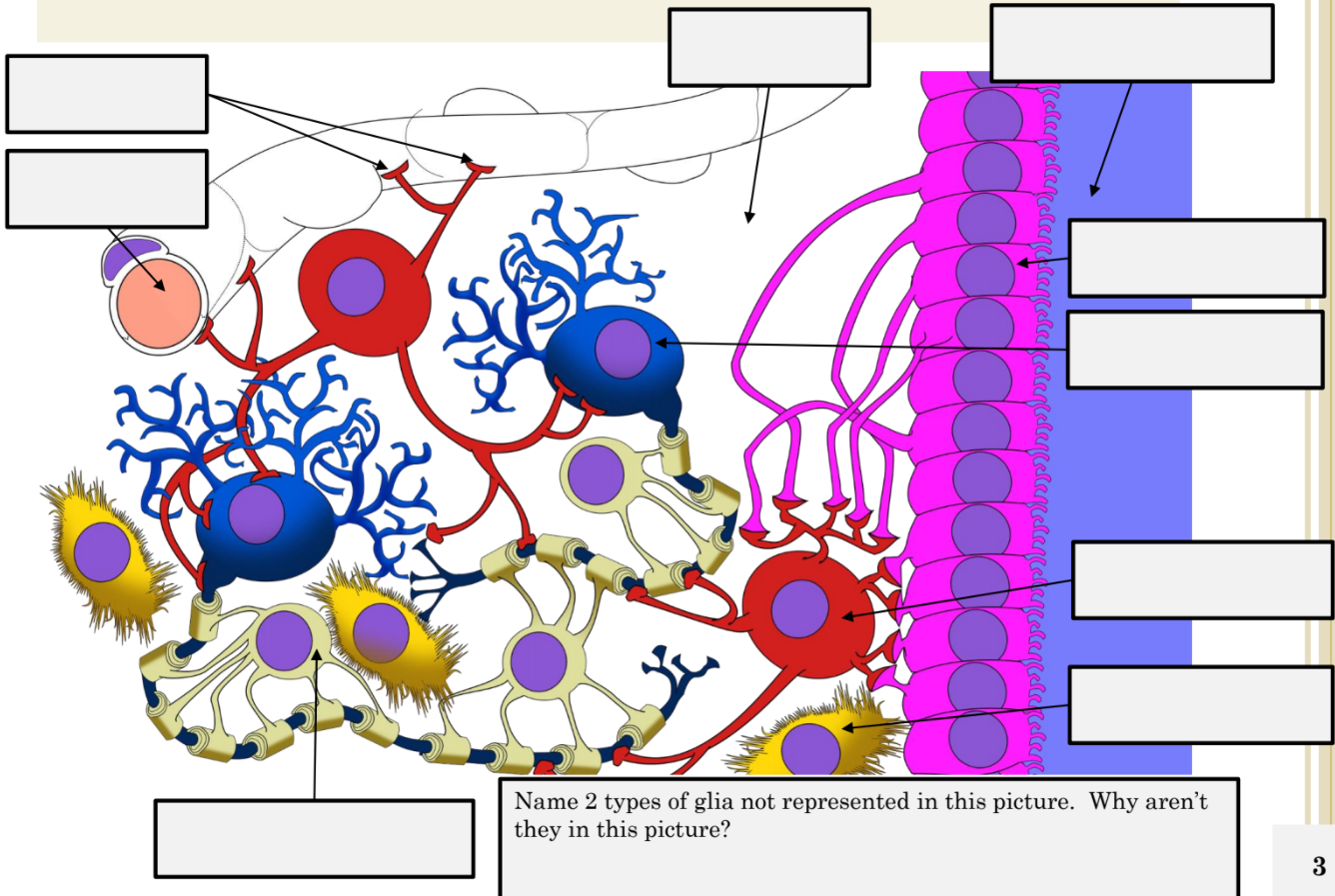
Lab 10 Exercise \(\PageIndex{5}\)
Label the histological sections.
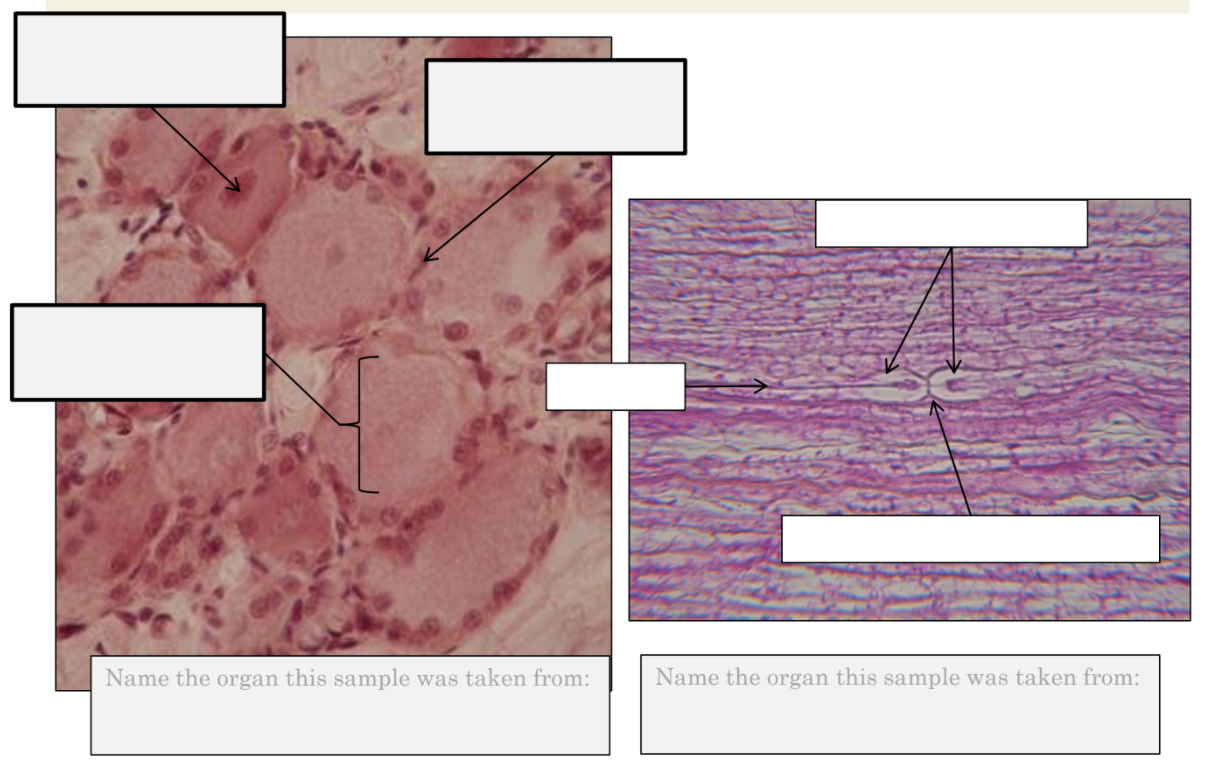
Lab 10 Exercise \(\PageIndex{6}\)
Obtain slides of each of the following tissues, observe them, draw and label the significant features. SLIDES: Spinal cord, cerebral cortex, cerebellum, dorsal root ganglion, peripheral nerve, neuromuscular junction:
1. Obtain a slide of nervous tissue from the slide box. Use any nervous tissue except peripheral nerve, there are no nerve cell bodies in a peripheral nerve section.
2. View the slide on the second-highest objective. Search carefully until you find a clear, representative neuron in your field of view.
3. In the circle below, draw the neuron you found. Only draw the single neuron. Do not draw any of the other material. Draw your structures proportionately to their size in your microscope’s field of view.
4. Label any neural parts you can clearly recognize.
3.2 Determining Empirical and Molecular Formulas
Learning objectives.
By the end of this section, you will be able to:
- Compute the percent composition of a compound
- Determine the empirical formula of a compound
- Determine the molecular formula of a compound
The previous section discussed the relationship between the bulk mass of a substance and the number of atoms or molecules it contains (moles). Given the chemical formula of the substance, one may determine the amount of the substance (moles) from its mass, and vice versa. But what if the chemical formula of a substance is unknown? In this section, these same principles will be applied to derive the chemical formulas of unknown substances from experimental mass measurements.
Percent Composition
The elemental makeup of a compound defines its chemical identity, and chemical formulas are the most succinct way of representing this elemental makeup. When a compound’s formula is unknown, measuring the mass of each of its constituent elements is often the first step in the process of determining the formula experimentally. The results of these measurements permit the calculation of the compound’s percent composition , defined as the percentage by mass of each element in the compound. For example, consider a gaseous compound composed solely of carbon and hydrogen. The percent composition of this compound could be represented as follows:
If analysis of a 10.0-g sample of this gas showed it to contain 2.5 g H and 7.5 g C, the percent composition would be calculated to be 25% H and 75% C:
Example 3.9
Calculation of percent composition.
The analysis results indicate that the compound is 61.0% C, 15.4% H, and 23.7% N by mass.
Check Your Learning
12.1% C, 16.1% O, 71.79% Cl
Determining Percent Composition from Molecular or Empirical Formulas
Percent composition is also useful for evaluating the relative abundance of a given element in different compounds of known formulas. As one example, consider the common nitrogen-containing fertilizers ammonia (NH 3 ), ammonium nitrate (NH 4 NO 3 ), and urea (CH 4 N 2 O). The element nitrogen is the active ingredient for agricultural purposes, so the mass percentage of nitrogen in the compound is a practical and economic concern for consumers choosing among these fertilizers. For these sorts of applications, the percent composition of a compound is easily derived from its formula mass and the atomic masses of its constituent elements. A molecule of NH 3 contains one N atom weighing 14.01 amu and three H atoms weighing a total of (3 × × 1.008 amu) = 3.024 amu. The formula mass of ammonia is therefore (14.01 amu + 3.024 amu) = 17.03 amu, and its percent composition is:
This same approach may be taken considering a pair of molecules, a dozen molecules, or a mole of molecules, etc. The latter amount is most convenient and would simply involve the use of molar masses instead of atomic and formula masses, as demonstrated Example 3.10 . As long as the molecular or empirical formula of the compound in question is known, the percent composition may be derived from the atomic or molar masses of the compound's elements.
Example 3.10
Determining percent composition from a molecular formula.
Note that these percentages sum to equal 100.00% when appropriately rounded.
Determination of Empirical Formulas
As previously mentioned, the most common approach to determining a compound’s chemical formula is to first measure the masses of its constituent elements. However, keep in mind that chemical formulas represent the relative numbers , not masses, of atoms in the substance. Therefore, any experimentally derived data involving mass must be used to derive the corresponding numbers of atoms in the compound. This is accomplished using molar masses to convert the mass of each element to a number of moles. These molar amounts are used to compute whole-number ratios that can be used to derive the empirical formula of the substance. Consider a sample of compound determined to contain 1.71 g C and 0.287 g H. The corresponding numbers of atoms (in moles) are:
Thus, this compound may be represented by the formula C 0.142 H 0.284 . Per convention, formulas contain whole-number subscripts, which can be achieved by dividing each subscript by the smaller subscript:
(Recall that subscripts of “1” are not written but rather assumed if no other number is present.)
The empirical formula for this compound is thus CH 2 . This may or may not be the compound’s molecular formula as well; however, additional information is needed to make that determination (as discussed later in this section).
Consider as another example a sample of compound determined to contain 5.31 g Cl and 8.40 g O. Following the same approach yields a tentative empirical formula of:
In this case, dividing by the smallest subscript still leaves us with a decimal subscript in the empirical formula. To convert this into a whole number, multiply each of the subscripts by two, retaining the same atom ratio and yielding Cl 2 O 7 as the final empirical formula.
In summary, empirical formulas are derived from experimentally measured element masses by:
- Deriving the number of moles of each element from its mass
- Dividing each element’s molar amount by the smallest molar amount to yield subscripts for a tentative empirical formula
- Multiplying all coefficients by an integer, if necessary, to ensure that the smallest whole-number ratio of subscripts is obtained
Figure 3.11 outlines this procedure in flow chart fashion for a substance containing elements A and X.
Example 3.11
Determining a compound’s empirical formula from the masses of its elements.
Next, derive the iron-to-oxygen molar ratio by dividing by the lesser number of moles:
The ratio is 1.000 mol of iron to 1.500 mol of oxygen (Fe 1 O 1.5 ). Finally, multiply the ratio by two to get the smallest possible whole number subscripts while still maintaining the correct iron-to-oxygen ratio:
The empirical formula is Fe 2 O 3 .
Link to Learning
For additional worked examples illustrating the derivation of empirical formulas, watch the brief video clip.
Deriving Empirical Formulas from Percent Composition
Finally, with regard to deriving empirical formulas, consider instances in which a compound’s percent composition is available rather than the absolute masses of the compound’s constituent elements. In such cases, the percent composition can be used to calculate the masses of elements present in any convenient mass of compound; these masses can then be used to derive the empirical formula in the usual fashion.
Example 3.12
Determining an empirical formula from percent composition.
The molar amounts of carbon and oxygen in a 100-g sample are calculated by dividing each element’s mass by its molar mass:
Coefficients for the tentative empirical formula are derived by dividing each molar amount by the lesser of the two:
Since the resulting ratio is one carbon to two oxygen atoms, the empirical formula is CO 2 .
Derivation of Molecular Formulas
Recall that empirical formulas are symbols representing the relative numbers of a compound’s elements. Determining the absolute numbers of atoms that compose a single molecule of a covalent compound requires knowledge of both its empirical formula and its molecular mass or molar mass. These quantities may be determined experimentally by various measurement techniques. Molecular mass, for example, is often derived from the mass spectrum of the compound (see discussion of this technique in the previous chapter on atoms and molecules). Molar mass can be measured by a number of experimental methods, many of which will be introduced in later chapters of this text.
Molecular formulas are derived by comparing the compound’s molecular or molar mass to its empirical formula mass . As the name suggests, an empirical formula mass is the sum of the average atomic masses of all the atoms represented in an empirical formula. If the molecular (or molar) mass of the substance is known, it may be divided by the empirical formula mass to yield the number of empirical formula units per molecule ( n ):
The molecular formula is then obtained by multiplying each subscript in the empirical formula by n , as shown by the generic empirical formula A x B y :
For example, consider a covalent compound whose empirical formula is determined to be CH 2 O. The empirical formula mass for this compound is approximately 30 amu (the sum of 12 amu for one C atom, 2 amu for two H atoms, and 16 amu for one O atom). If the compound’s molecular mass is determined to be 180 amu, this indicates that molecules of this compound contain six times the number of atoms represented in the empirical formula:
Molecules of this compound are then represented by molecular formulas whose subscripts are six times greater than those in the empirical formula:
Note that this same approach may be used when the molar mass (g/mol) instead of the molecular mass (amu) is used. In this case, one mole of empirical formula units and molecules is considered, as opposed to single units and molecules.
Example 3.13
Determination of the molecular formula for nicotine.
Next, calculate the molar ratios of these elements relative to the least abundant element, N.
The C-to-N and H-to-N molar ratios are adequately close to whole numbers, and so the empirical formula is C 5 H 7 N. The empirical formula mass for this compound is therefore 81.13 amu/formula unit, or 81.13 g/mol formula unit.
Calculate the molar mass for nicotine from the given mass and molar amount of compound:
Comparing the molar mass and empirical formula mass indicates that each nicotine molecule contains two formula units:
Finally, derive the molecular formula for nicotine from the empirical formula by multiplying each subscript by two:
C 8 H 10 N 4 O 2
As an Amazon Associate we earn from qualifying purchases.
This book may not be used in the training of large language models or otherwise be ingested into large language models or generative AI offerings without OpenStax's permission.
Want to cite, share, or modify this book? This book uses the Creative Commons Attribution License and you must attribute OpenStax.
Access for free at https://openstax.org/books/chemistry-2e/pages/1-introduction
- Authors: Paul Flowers, Klaus Theopold, Richard Langley, William R. Robinson, PhD
- Publisher/website: OpenStax
- Book title: Chemistry 2e
- Publication date: Feb 14, 2019
- Location: Houston, Texas
- Book URL: https://openstax.org/books/chemistry-2e/pages/1-introduction
- Section URL: https://openstax.org/books/chemistry-2e/pages/3-2-determining-empirical-and-molecular-formulas
© Jan 8, 2024 OpenStax. Textbook content produced by OpenStax is licensed under a Creative Commons Attribution License . The OpenStax name, OpenStax logo, OpenStax book covers, OpenStax CNX name, and OpenStax CNX logo are not subject to the Creative Commons license and may not be reproduced without the prior and express written consent of Rice University.
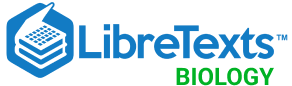
- school Campus Bookshelves
- menu_book Bookshelves
- perm_media Learning Objects
- login Login
- how_to_reg Request Instructor Account
- hub Instructor Commons
- Download Page (PDF)
- Download Full Book (PDF)
- Periodic Table
- Physics Constants
- Scientific Calculator
- Reference & Cite
- Tools expand_more
- Readability
selected template will load here
This action is not available.
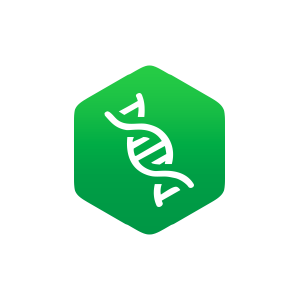
10.1: General Characteristics of Viruses
- Last updated
- Save as PDF
- Page ID 3232
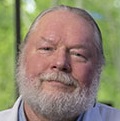
- Gary Kaiser
- Community College of Baltimore Country (Cantonsville)
Learning Objectives
- State 2 living and 2 nonliving characteristics of viruses.
- List 3 criteria used to define a virus.
- Discuss why bacteria can be cultivated on synthetic media such as nutrient broth whereas viruses cannot.
- Define bacteriophage.
Viruses are infectious agents with both living and nonliving characteristics. They can infect animals, plants, and even other microorganisms. Viruses that infect only bacteria are called bacteriophages and those that infect only fungi are termed mycophages . There are even some viruses called virophages that infect other viruses.
Recently, viruses have been declared as living entities based on the large number of protein folds encoded by viral genomes that are shared with the genomes of cells. This indicates that viruses likely arose from multiple ancient cells.
The vast majority of viruses contain only one type of nucleic acid: DNA or RNA, but not both. Virus are totally dependent on a host cell for replication (i.e., they are strict intracellular parasites.) Furthermore, v iral components must assemble into complete viruses (virions) to go from one host cell to another. Since viruses lack metabolic machinery of their own and are totally dependent on their host cell for replication, they cannot be grown in synthetic culture media. Animal viruses are normally grown in animals, embryonated eggs, or in cell cultures where in animal host cells are grown in a synthetic medium and the viruses are then grown in these cells.
- Viruses are infectious agents with both living and nonliving characteristics.
- Living characteristics of viruses include the ability to reproduce – but only in living host cells – and the ability to mutate.
- Nonliving characteristics include the fact that they are not cells, have no cytoplasm or cellular organelles, and carry out no metabolism on their own and therefore must replicate using the host cell's metabolic machinery.
- Viruses can infect animals, plants, and even other microorganisms.
- Since viruses lack metabolic machinery of their own and are totally dependent on their host cell for replication, they cannot be grown in synthetic culture media.

- school Campus Bookshelves
- menu_book Bookshelves
- perm_media Learning Objects
- login Login
- how_to_reg Request Instructor Account
- hub Instructor Commons
- Download Page (PDF)
- Download Full Book (PDF)
- Periodic Table
- Physics Constants
- Scientific Calculator
- Reference & Cite
- Tools expand_more
- Readability
selected template will load here
This action is not available.
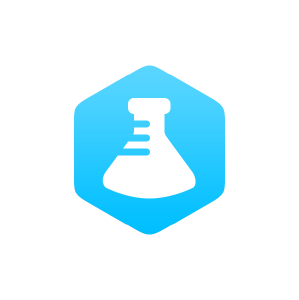
10: The Mole
- Last updated
- Save as PDF
- Page ID 53764
- 10.1: Avogadro's Number
- 10.2: Conversions Between Moles and Atoms
- 10.3: Molar Mass
- 10.4: Conversions Between Moles and Mass
- 10.5: Conversions Between Mass and Number of Particles
- 10.6: Avogadro's Hypothesis and Molar Volume
- 10.7: Conversions Between Moles and Gas Volume
- 10.8: Gas Density As you know, density is defined as the mass per unit volume of a substance. Since gases all occupy the same volume on a per mole basis, the density of a particular gas is dependent on its molar mass. A gas with a small molar mass will have a lower density than a gas with a large molar mass. Gas densities are typically reported in g/L . Gas density can be calculated from molar mass and molar volume.
- 10.8: Mole Road Map
- 10.10: Percent Composition
- 10.11: Percent of Water in a Hydrate
- 10.12: Determining Empirical Formulas
- 10.13: Determining Molecular Formulas
Applied Data Science with Python
Free Applied Data Science with Python Course
Embark on a data-driven journey with our free Applied Data Science with Python course. Master Python for data manipulation and analysis, tackle real-world challenges and showcase your skills in a hands-on final project. Join us to unleash the potential of data science and propel your career forward.
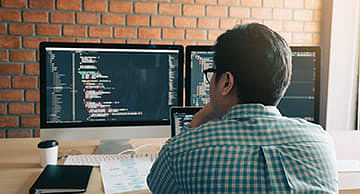
- 8 Hours Of self-paced video lessons
- Completion Certificate awarded on course completion
- 90 Days of Access To your Free Course
Data Science with Python Skills you will learn
- Data wrangling
- Data exploration
- Data visualization
- Mathematical computing
- Web scraping
- Hypothesis building
- Python programming concepts
- NumPy and SciPy package
- ScikitLearn package for Natural Language Processing
Who should learn this Data Science with Python free course?
- Analytics Professionals
- Software Professionals
- IT Professionals
- Data Scientist
- Data Analyst

What you will learn in this Data Science with Python free course?
Data science with python, lesson 01 - course introduction, 1.01 course introduction, 1.02 what you will learn, lesson 02 - introduction to data science, 2.01 introduction, 2.02 data science and its applications, 2.03 the data science process: part 1, 2.04 the data science process: part 2, lesson 03 - essentials of python programming, 3.01 introduction, 3.02 setting up jupyter notebook: part 1, 3.03 setting up jupyter notebook: part 2, 3.04 python functions, 3.05 python types and sequences, 3.06 python strings deep dive, 3.07 python demo: reading and writing csv files, 3.08 date and time in python, 3.09 objects in python map, 3.10 lambda and list comprehension, 3.11 why python for data analysis, 3.12 python packages for data science, 3.13 statsmodels package: part 1, 3.14 statsmodels package: part 2, 3.15 scipy package, 3.17 spotlight, lesson 04 - numpy, 4.01 introduction, 4.02 fundamentals of numpy, 4.03 array shapes and axes in numpy: part a, 4.04 numpy array shapes and axes: part b, 4.05 arithmetic operations, 4.06 conditional logic, 4.07 common mathematical and statistical functions in numpy, 4.08 indexing and slicing: part 1, 4.09 indexing and slicing: part 2, 4.10 file handling, lesson 05 - linear algebra, 5.01 introduction, 5.02 introduction to linear algebra, 5.03 scalars and vectors, 5.04 dot product of two vectors, 5.05 linear independence of vectors, 5.06 norm of a vector, 5.07 matrix, 5.08 matrix operations, 5.09 transpose of a matrix, 5.10 rank of a matrix, 5.11 determinant of a matrix and identity matrix or operator, 5.12 inverse of a matrix and eigenvalues and eigenvectors, 5.13 calculus in linear algebra, lesson 06 - statistics fundamentals, 6.01 introduction, 6.02 importance of statistics with respect to data science, 6.03 common statistical terms, 6.04 types of statistics, 6.05 data categorization and types, 6.06 levels of measurement, 6.07 measures of central tendency, 6.08 measures of central tendency, 6.09 measures of central tendency, 6.10 measures of dispersion, 6.11 random variables, 6.13 measures of shape (skewness), 6.14 measures of shape (kurtosis), 6.15 covariance and correlation, lesson 07 - probability distribution, 7.01 introduction, 7.02 probability,its importance, and probability distribution, 7.03 probability distribution : binomial distribution, 7.04 probability distribution: poisson distribution, 7.05 probability distribution: normal distribution, 7.06 probability distribution: uniform distribution, 7.07 probability distribution: bernoulli distribution, 7.08 probability density function and mass function, 7.09 cumulative distribution function, 7.10 central limit theorem, 7.11 estimation theory, lesson 08 - advanced statistics, 8.01 introduction, 8.02 distribution, 8.03 kurtosis skewness and student's t-distribution, 8.04 hypothesis testing and mechanism, 8.05 hypothesis testing outcomes: type i and ii errors, 8.06 null hypothesis and alternate hypothesis, 8.07 confidence intervals, 8.08 margins of error, 8.09 confidence level, 8.10 t - test and p - values (lab), 8.11 z - test and p - values, 8.12 comparing and contrasting t test and z test, 8.13 bayes theorem, 8.14 chi sqare distribution, 8.15 chi square distribution : demo, 8.16 chi square test and goodness of fit, 8.17 analysis of variance or anova, 8.18 anova termonologies, 8.19 assumptions and types of anova, 8.20 partition of variance using python, 8.21 f - distribution, 8.22 f - distribution using python, 8.23 f - test, 8.25 spotlight, lesson 09 - pandas, 9.01 introduction, 9.02 introduction to pandas, 9.03 pandas series, 9.04 querying a series, 9.05 pandas dataframes, 9.06 pandas panel, 9.07 common functions in pandas, 9.08 pandas functions data statistical function, windows function, 9.09 pandas function data and timedelta, 9.10 io tools explain all the read function, 9.11 categorical data, 9.12 working with text data, 9.13 iteration, 9.14 sorting, 9.15 plotting with pandas, lesson 10 - data analysis, 10.01 introduction, 10.02 understanding data, 10.03 types of data structured unstructured messy etc, 10.04 working with data choosing appropriate tools, data collection, data wrangling, 10.05 importing and exporting data in python, 10.06 regular expressions in python, 10.07 manipulating text with regular expressions, 10.08 accessing databases in python, 10.09 recap, 10.10 spotlight, lesson 11 - data wrangling, 11.01 introduction, 11.02 pandorable or idiomatic pandas code, 11.03 loading indexing and reindexing, 11.04 merging, 11.05 memory optimization in python, 11.06 data pre processing: data loading and dropping null values, 11.07 data pre-processing filling null values, 11.08 data binning formatting and normalization, 11.09 data binning standardization, 11.10 describing data, 11.11 recap, lesson 12 - data visualization, 12.01 introduction, 12.02 principles of information visualization, 12.03 visualizing data using pivot tables, 12.04 data visualization libraries in python matplotlib, 12.05 graph types, 12.06 data visualization libraries in python seaborn, 12.07 data visualization libraries in python seaborn, 12.08 data visualization libraries in python plotly, 12.09 data visualization libraries in python plotly, 12.10 data visualization libraries in python bokeh, 12.11 data visualization libraries in python bokeh, 12.12 using matplotlib to plot graphs, 12.13 plotting 3d graphs for multiple columns using matplotlib, 12.14 using matplotlib with other python packages, 12.15 using seaborn to plot graphs, 12.16 using seaborn to plot graphs, 12.17 plotting 3d graphs for multiple columns using seaborn, 12.18 introduction to plotly, 12.19 introduction to bokeh, 12.20 recap, lesson 13 - end to end statistics application with python, 13.01 introduction, 13.02 basic statistics with python problem statement, 13.03 basic statistics with python solution, 13.04 scipy for statistics problem statement, 13.05 scipy for statistics solution, 13.06 advanced statistics python, 13.07 advanced statistics with python solution, 13.08 recap, 13.09 spotlight, get a completion certificate.
Share your certificate with prospective employers and your professional network on LinkedIn.
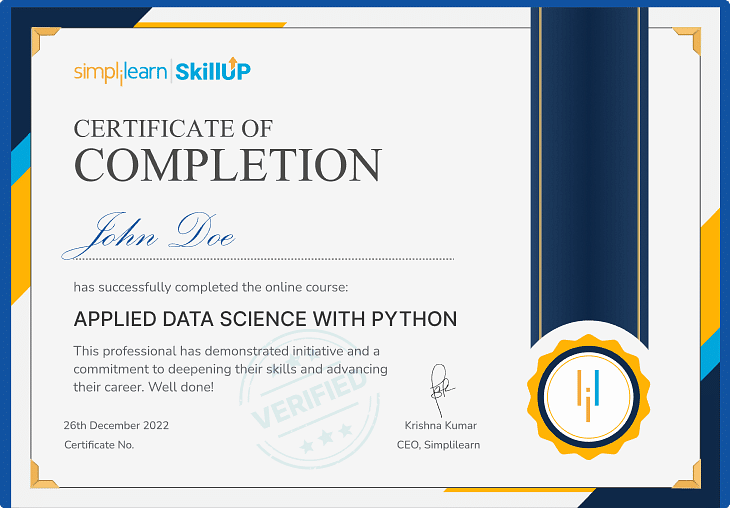
$100K - $217K Per Annum

Why is Python popular in data science?
Python's popularity in data science may be attributed to its ease of use, readability, and abundance of tools that facilitate data handling.
Is there a cost associated with this free Applied Data Science with Python course?
No, this course is free and has no hidden charges or registration fees.
What are the prerequisites to learn this free course?
There are no prerequisites to learning this course; the only requirement is your interest in learning.
When can I expect to receive my certificate?
You'll receive your certificate as soon as you complete your course.
What is the duration of my access to the course?
You will have access to the course for 90 Days.
Can I learn data science only with Python?
You can learn data science only with Python.
How challenging is this free course?
The course is easy.
Who can benefit from a data science with Python course?
Anyone from aspiring data scientists and analysts to programmers, business professionals, students, and career changers can benefit from data science with a Python course. It's a versatile skill set applicable across various industries and career stages.
Do I need a strong programming background to learn data science with Python?
No, you don't need a strong programming background to learn data science with Python.
Learner Review

Ashish KC Khatri
I learned some new interesting python content, from Simplilearn's Data Science course. Looking forward to learn more.

Abhimanyu Chandgude
Thank you Simplilearn for providing such an amazing and valuable course!

The Data Science with Python courses helped me a lot in improving my understanding of Python skills. I really enjoyed learning it.

Kipngetich Evans
The course is well-structured. I loved learning this course because it introduced me to a whole new world of Data Science.

Pooja Rohiwal
The entire syllabus for this course was explained well. The best part was the exercises which helped a lot in understanding python better.
Related Courses
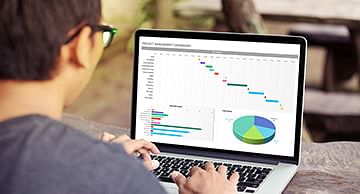
Business Analytics with Excel
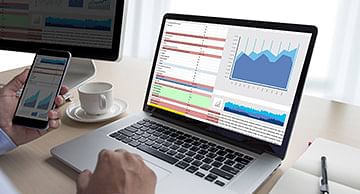
Introduction to Data Analytics Course
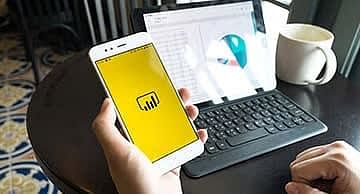
Power BI for Beginners
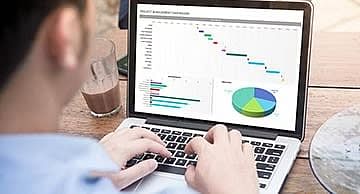
Introduction to MS Excel
- PMP, PMI, PMBOK, CAPM, PgMP, PfMP, ACP, PBA, RMP, SP, and OPM3 are registered marks of the Project Management Institute, Inc.

- school Campus Bookshelves
- menu_book Bookshelves
- perm_media Learning Objects
- login Login
- how_to_reg Request Instructor Account
- hub Instructor Commons
- Download Page (PDF)
- Download Full Book (PDF)
- Periodic Table
- Physics Constants
- Scientific Calculator
- Reference & Cite
- Tools expand_more
- Readability
selected template will load here
This action is not available.
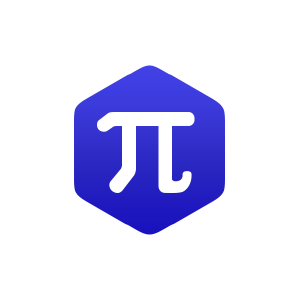
2.10: Exercises
- Last updated
- Save as PDF
- Page ID 118492

COMMENTS
Fill in the Blank Exercise 10.02. 5.0 (5 reviews) A #20 knife blade fits on a # _____knife handle. Click the card to flip 👆. 4. Click the card to flip 👆. 1 / 5.
Fill in the Blank Exercise 10.02. 5 terms. lan3nita. Preview. Surgical Technology Chapter 10 WS. Teacher 155 terms. jose_lerma4. Preview. Ortega_Hailey_Lección4. 59 terms. Hailey_Ortega1. ... Matching Exercise 10.04: Match the type of material used with the situation described. 10 terms. lan3nita. Preview. Chapter 2 Spanish vocab quiz 1. 10 ...
Identify the pats of a "neuron". Lab 10 Exercise 10.2.2 10.2. 2. Label the following: Lab 10 Exercise 10.2.3 10.2. 3. Match these items or actions to their locations on a neuron: graded potentials, action. Lab 10 Exercise 10.2.4 10.2. 4. Label the following glia & related items: Lab 10 Exercise 10.2.5 10.2. 5. Label the histological sections.
In exercises 1 - 4, justify your answer with a proof or a counterexample. 1) If the radius of convergence for a power series ∑n=0∞ anxn ∑ n = 0 ∞ a n x n is 5 5, then the radius of convergence for the series ∑n=1∞ nanxn−1 ∑ n = 1 ∞ n a n x n − 1 is also 5 5. Answer. 2) Power series can be used to show that the derivative of ...
The measure of one angle of a triangle is twice the measure of the smallest angle. The measure of the third angle is three times the measure of the smallest angle. Find the measures of all three angles. 652. The length of a rectangle is five feet more than four times the width. The perimeter is 60 feet.
Exercises 11 - 16 explore applications of annuities. 11) Calculate the present values \(P\) of an annuity in which $10,000 is to be paid out annually for a period of 20 years, assuming interest rates of \(r=0.03,\, r=0.05\), and \(r=0.07\). Answer
In this lab, use the 192.168.33.128/25 network address to develop an address scheme for the network displayed in the topology diagram. VLSM is used to meet the IPv4 addressing requirements. After you have designed the VLSM address scheme, you will configure the interfaces on the routers with the appropriate IP address information.
Figure 10.3.1 10.3. 1: (a) Ribonucleotides contain the pentose sugar ribose instead of the deoxyribose found in deoxyribonucleotides. (b) RNA contains the pyrimidine uracil in place of thymine found in DNA. The RNA-specific pyrimidine uracil forms a complementary base pair with adenine and is used instead of the thymine used in DNA.
The ratio is 1.000 mol of iron to 1.500 mol of oxygen (Fe 1 O 1.5 ). Finally, multiply the ratio by two to get the smallest possible whole number subscripts while still maintaining the correct iron-to-oxygen ratio: 2(Fe1O1.5) = Fe2O3 2 ( Fe 1 O 1.5) = Fe 2 O 3. The empirical formula is Fe 2 O 3.
Fill in the Blank Exercise 14.02: identify the incisions in the figure. A. Click the card to flip 👆. Right upper paramedial incision. Click the card to flip 👆. 1 / 10.
Figure 10.6.1: Dalton's Law. The total pressure of a mixture of gases is the sum of the partial pressures of the individual gases. For a mixture of two ideal gases, A and B, we can write an expression for the total pressure: Ptot = PA + PB = nA(RT V) + nB(RT V) = (nA + nB)(RT V) More generally, for a mixture of n component gases, the total ...
Summary. Viruses are infectious agents with both living and nonliving characteristics. Living characteristics of viruses include the ability to reproduce - but only in living host cells - and the ability to mutate. Nonliving characteristics include the fact that they are not cells, have no cytoplasm or cellular organelles, and carry out no ...
The Rectangular Coordinate Systems and Graphs. For the following exercises, find the x -intercept and the y -intercept without graphing. 1 . 4x − 3y = 12 4 x − 3 y = 12. 2. 2y − 4 = 3x 2 y − 4 = 3 x. For the following exercises, solve for y in terms of x, putting the equation in slope-intercept form. 3 .
It is defined as the amount of a chemical substance that contains as many elementary entities (e.g., atoms, molecules, ions, electrons, or photons). This number is expressed by the Avogadro constant, which has a value of 6.022140857 ×1023 mol−1 6.022140857 × 10 23 m o l − 1. The mole is one of the base units of the SI, and has the unit ...
Terms in this set (20) Study with Quizlet and memorize flashcards containing terms like Classify the instruments using the following designations: # 3 knife handle, Classify the instruments using the following designations: Allis tissue forceps, Classify the instruments using the following designations: Babcock tissue forceps and more.
This problem has been solved! You'll get a detailed solution from a subject matter expert that helps you learn core concepts. See Answer See Answer See Answer done loading
4.5 359411 Learners EnrolledAdvanced Level. Embark on a data-driven journey with our free Applied Data Science with Python course. Master Python for data manipulation and analysis, tackle real-world challenges and showcase your skills in a hands-on final project. Join us to unleash the potential of data science and propel your career forward.
3/30/24 iii 10.4 Disciplinary Action. 24 Article 11 Conduct and Employment of Athletics Personnel 11.01 General Principles. 25 11.02 Definitions and Applications. 25
Download Full Book (PDF) Resources expand_more. Periodic Table. Physics Constants. Scientific Calculator. Reference expand_more. Reference & Cite. Tools expand_more. Help expand_more.
Minnesota Rules of Criminal Procedure - Minnesota Judicial Branch ... rule. ...
10. _____ lap sponges come in a single package. 5. Kitner sponges are used to aid the surgeon in ____. blunt dissection. A one-layer dressing is used to cover a small incision from which drainage is expected to be. minimal. _____ is a brand name for a one-layer dressing made of polyurethane film. Bioclusive.
Study with Quizlet and memorize flashcards containing terms like Helicopters can help rescue people in _____., A night of rain is enough to _____ the fields., Do you think that human beings will one day _____ on the Moon? and more.