Introduction to Digital Systems: Modeling, Synthesis, and Simulation Using VHDL by
Get full access to Introduction to Digital Systems: Modeling, Synthesis, and Simulation Using VHDL and 60K+ other titles, with a free 10-day trial of O'Reilly.
There are also live events, courses curated by job role, and more.

9.6 ONE-HOT ENCODING METHOD
One-hot encoding is an alternative state assignment method which attempts to minimize the combinational logic by increasing the number of flip-flops. The goal of the method is to try to reduce the number of connections between the logic gates in the combinational circuit of the FSM. The presence of more gate interconnections results into longer propagation delays and a slower FSM. Since the propagation delay through the flip-flops is faster, FSMs require fewer logic gates but not necessarily fewer flip-flops.
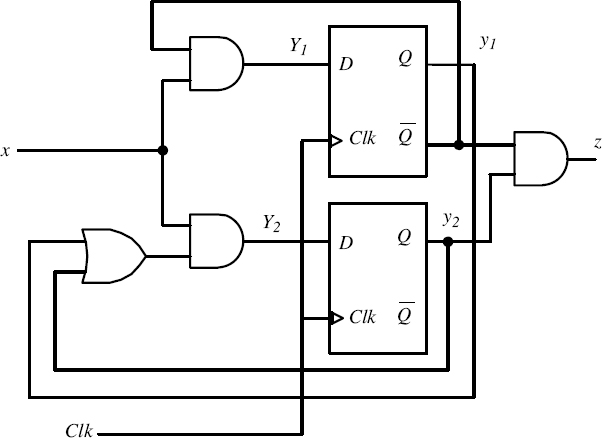
Figure 9.26 Logic Implementation of the FSM in Figure 9.24
One-hot encoding assigns one flip-flop for each state. For example, a finite-state machine with N states requires N flip-flops. The states are assigned N -bit binary numbers; where only the corresponding bit position is equal to 1, the remaining bits are equal to0. For example, in a finite-state machine with four states S 0 , S 1 , S 2 , and S 3 , the states are assigned the binary values 0001, 0010, 0100, and 1000, respectively. Notice that only one bit position is equal to 1; the other bits are all equal to 0. The reamaining 12 binary combinations are assigned to don't-care states. Consider the Mealy-type finite-state machine described by the state diagram shown in Figure 9.19 . The state diagram has three states: S 0 , S 1 , and S 2 . One-hot encoding assigns the binary number values 001, 010, and 100 ...
Get Introduction to Digital Systems: Modeling, Synthesis, and Simulation Using VHDL now with the O’Reilly learning platform.
O’Reilly members experience books, live events, courses curated by job role, and more from O’Reilly and nearly 200 top publishers.
Don’t leave empty-handed
Get Mark Richards’s Software Architecture Patterns ebook to better understand how to design components—and how they should interact.
It’s yours, free.
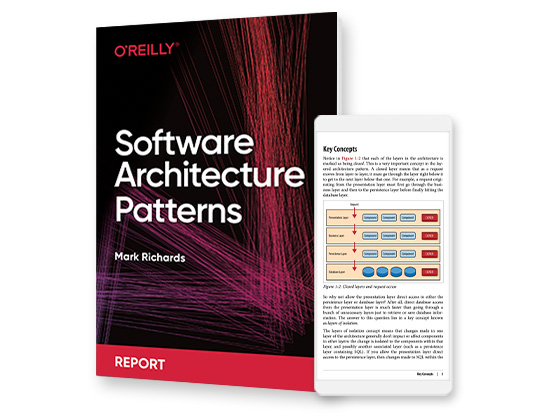
Check it out now on O’Reilly
Dive in for free with a 10-day trial of the O’Reilly learning platform—then explore all the other resources our members count on to build skills and solve problems every day.
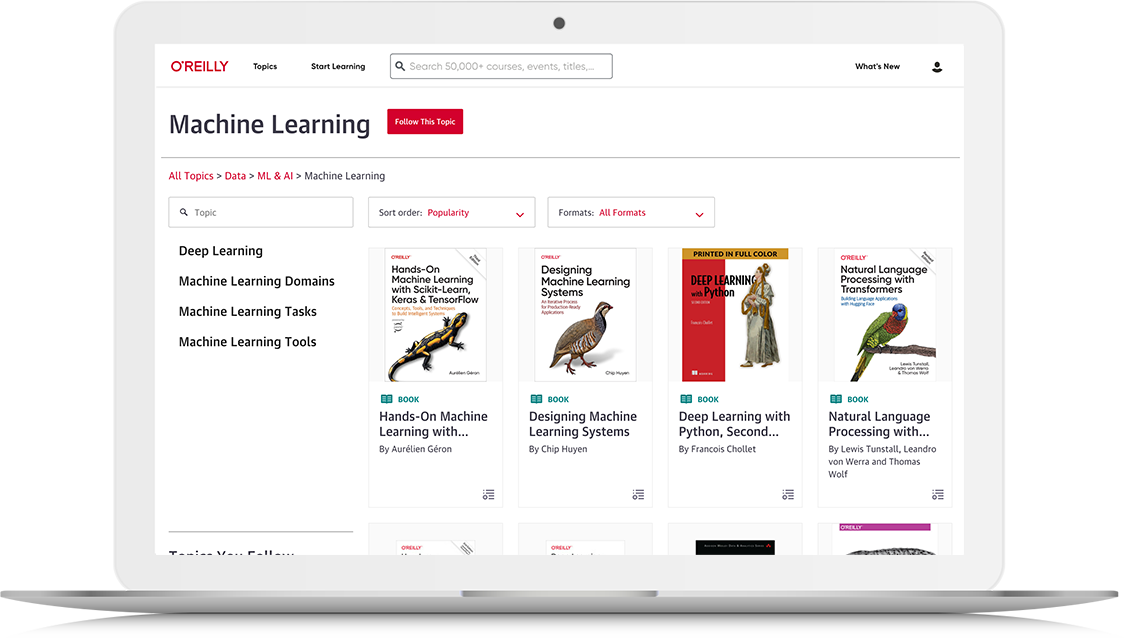
Verilog Pro
One-hot State Machine in SystemVerilog – Reverse Case Statement
Finite state machine (FSM) is one of the first topics taught in any digital design course, yet coding one is not as easy as first meets the eye. There are Moore and Mealy state machines, encoded and one-hot state encoding, one or two or three always block coding styles. Recently I was reviewing a coworker’s RTL code and came across a SystemVerilog one-hot state machine coding style that I was not familiar with. Needless to say, it became a mini research topic resulting in this blog post.
When coding state machines in Verilog or SystemVerilog, there are a few general guidelines that can apply to any state machine:
- If coding in Verilog, use parameters to define state encodings instead of ‘define macro definition. Verilog ‘define macros have global scope; a macro defined in one module can easily be redefined by a macro with the same name in a different module compiled later, leading to macro redefinition warnings and unexpected bugs.
- If coding in SystemVerilog, use enumerated types to define state encodings.
- Always define a parameter or enumerated type value for each state so you don’t leave it to the synthesis tool to choose a value for you. Otherwise it can make for a very difficult ECO when it comes time to reverse engineer the gate level netlist.
- Make curr_state and next_state declarations right after the parameter or enumerated type assignments. This is simply clean coding style.
- Code all sequential always block using nonblocking assignments (<=). This helps guard against simulation race conditions.
- Code all combinational always block using blocking assignments (=). This helps guard against simulation race conditions.
SystemVerilog enumerated types are especially useful for coding state machines. An example of using an enumerated type as the state variable is shown below.
Notice that enumerated types allow X assignments. Enumerated types can be displayed as names in simulator waveforms, which eliminates the need of a Verilog trick to display the state name in waveform as a variable in ASCII encoding.
One-hot refers to how each of the states is encoded in the state vector. In a one-hot state machine, the state vector has as many bits as number of states. Each bit represents a single state, and only one bit can be set at a time— one-hot . A one-hot state machine is generally faster than a state machine with encoded states because of the lack of state decoding logic.
SystemVerilog and Verilog has a unique (pun intended) and efficient coding style for coding one-hot state machines. This coding style uses what is called a reverse case statement to test if a case item is true by using a case header of the form case (1’b1) . Example code is shown below:
In this one-hot state machine coding style, the state parameters or enumerated type values represent indices into the state and next vectors. Synthesis tools interpret this coding style efficiently and generates output assignment and next state logic that does only 1-bit comparison against the state vectors. Notice also the use of always_comb and always_ff SystemVerilog always statements, and unique case to add some run-time checking.
An alternate one-hot state machine coding style to the “index-parameter” style is to completely specify the one-hot encoding for the state vectors, as shown below:
According to Cliff Cummings’ 2003 paper , this coding style yields poor performance because the Design Compiler infers a full 4-bit comparison against the state vector, in effect defeating the speed advantage of a one-hot state machine. However, the experiments conducted in this paper were done in 2003, and I suspect synthesis tools have become smarter since then.
State machines may look easy on paper, but are often not so easy in practice. Given how frequently state machines appear in designs, it is important for every RTL designer to develop a consistent and efficient style for coding them. One-hot state machines are generally preferred in applications that can trade-off area for a speed advantage. This article demonstrated how they can be coded in Verilog and SystemVerilog using a unique and very efficient “reverse case statement” coding style. It is a technique that should be in every RTL designer’s arsenal.
What are your experiences with coding one-hot state machines? Do you have another coding style or synthesis results to share? Leave a comment below!
- Synthesizable Finite State Machine Design Techniques Using the New SystemVerilog 3.0 Enhancements
Share this:
- Click to share on LinkedIn (Opens in new window)
- Click to share on Twitter (Opens in new window)
- Click to share on Facebook (Opens in new window)
- Click to share on Pocket (Opens in new window)
- Click to email a link to a friend (Opens in new window)
- Click to print (Opens in new window)
14 thoughts on “One-hot State Machine in SystemVerilog – Reverse Case Statement”
I am enjoying reading your blog, but I believe you are missing something from your one-hot reverse-case example above. In Cliff’s paper, the enumerated type is used as an index to a vector – you neglected to copy this part over. I believe the correct code would look like this:
enum { IDLE = 0, READ = 1, DLY = 2, DONE = 3 } state_index_t;
logic [3:0] state, next;
with the vector declaration in place the rest of the example above should work OK.
Hi John, thanks for your comment! You’re correct that in Cliff’s paper the enumerated type is used as an index into the one-hot vector. In my original code above I was sloppy and mixed the value of the enumerated type (0, 1, 2, 3) with the type itself. While I did test the original code and it simulated correctly, I’m sure a linting tool would give a warning about the improper usage. I have made a correction to the code above.
Great post. I have seen improved performance with one hot reverse case statements as well. One comment I would have is that the default assignment for “next” should be a valid case especially since we don’t have a default case statement. In the case of a bit being flipped because of some rare condition you’ll be able to recover. Something like this:
// Combinational next state logic always_comb begin next = ‘0; next[IDLE] = 1’b1; // ADDED unique case (1’b1) state[IDLE] : begin if (go) begin next = ‘0; // ADDED next[READ] = 1’b1; end else begin next = ‘0; // ADDED next[IDLE] = 1’b1; end end
Thanks, Amol, for your suggestion. I understand your reasoning to have a valid case (like IDLE) be the default transition for safety. Personally I don’t code that way as I think a bit flip anywhere in the chip is considered fatal, and even if the affected state machine returns to IDLE, it will likely have become out of sync with other logic and state machines. However, I have coworkers who code in the way you suggested as well.
There is a subtle point that may cause your code to not behave in the way intended. Due to the use of “unique case” in the code, I think for any unspecified cases (e.g. if a bit flip causes the state vector to become 4’b0000; 4’b0000 is not a case that is listed in the case statement), the synthesis tool is free to infer any logic (specifically to the “next” vector), so there’s no guarantee the default “next[IDLE]=1’b1” wouldn’t be overridden by other logic the synthesis tool inferred. See my post on SystemVerilog unique keyword.
Interesting. I had a “typo” in my code recently that caused the case_expression to be one not listed in the case statement and I did observe odd behavior from what I would have expected. So I guess having the default “next[IDLE]=1’b1” is probably not that useful here. 🙂
This is a synthetic example I would assume but want to get your feedback on preserving X-propagation. Bugs in the state machine next state logic or outside the state machine driving the input signals “go” and “ws” can be hidden by the current way of coding (using if-else and also default assigned to 0). Killing x-prop can cause “simulation vs. synthesis mismatch”, which can be pretty fatal. Consider this improvement:
// Combinational next state logic always_comb begin //next = ‘0; // Comment out; see added default in case statement instead unique case (1’b1) state[IDLE] : begin // Ensures x-prop if go === x next[READ] = go == 1’b1; next[IDLE] = go == 1’b0; end state[READ] : next[ DLY] = 1’b1; state[ DLY] : begin // Ensures x-prop if ws === x next[DONE] = ws == 1’b0; next[READ] = ws == 1’b1; end state[DONE] : next[IDLE] = 1’b1; // Add default to propagate Xs for unhandled states or if state reg went X itself default: next <= 'x; endcase end
Hi VK, thanks for your comment! When you say the code “kills x-prop”, I think you mean that if “ws” or “go” input has the value of X, then the “if(x)-else” coding style will take on the “else” case, rather than also corrupting the outputs (in this case the “next” vector)? Yes you’re right, and I’ll admit I’ve coded this kind of bug before! Recently our team has turned on the Synopsys VCS x-propagation feature to detect this kind of problem. It corrupts the output even with this “if-else” coding style (see my post on x-prop ). If that’s not available, then yes, the code you propose will also do the job. Previously I also coded a “default: next <= 'x" in my state machines, but the RTL linting tool we use complains about this, so I've moved away from this style. VCS x-prop will also catch this kind of problem.
I just realized you are not driving every bit of “next” in the case statement, so you would need to have the default next = ‘0 at the beginning as you did originally. That would not kill x-prop anyways since the default in the case statement would override it if needed.
Hello Jason, Thanks for the post. It really helped me to understand more about OneHot FSMs. The statement on Cliff’s paper in 2003 is not entirely true. we can handle that case by adding a unique key word. In the meantime concern raised by VK is great and worth looking into. with both of this I would like you to consider the following code which will give you the same synthesis result. Advantages being 1) state vector is enum, hence waveform visualization is better 2) does propagate x, in case FSM assignment is buggy 3) simpler and easy to read
typedef enum logic [3:0] { IDLE = 4’b0001, READ = 4’b0010, DLY = 4’b0100, DONE = 4’b1000, SX = 4’x } state_t; state_t state, next;
// Sequential state transition always_ff @(posedge clk or negedge rst_n) if (!rst_n) state <= IDLE; else state <= next;
// Combinational next state logic always_comb begin next = SX; unique case (state) IDLE : begin if (go) next = READ; else next = IDLE; end READ : next = DLY; DLY : begin if (!ws) next = DONE; else next = READ; end DONE : next = IDLE; endcase end
// Make output assignments always_ff @(posedge clk or negedge rst_n) …
Thanks for your comments! Yes I had been intending to make an update to this page for while, especially about your first point on waveform display of the state vector. After simulating my coworker’s code in the original coding style, I also realized simulators do not visualize state vectors written this way very well. I would agree that specifying the one-hot state encoding in the enum type should be equivalent and will display better. Your proposed code is indeed how I would write a one-hot state machine using SystemVerilog today!
Hi Jason Reffering to the last comment by shailesh, do you still suggests using “reverse case method” for FSM ? is it worth the less-readable code? or that shailesh’s code will do the same w/o infers the full bit comparison? this code is much more initiuative, but what about performence?
I have not had a chance to look closely at the synthesized netlist of a one-hot encoded state machine written with enumerated type and regular case statement. I believe with today’s compiler technology it should synthesize the same as the reverse case statement method (i.e. optimized to do single state bit comparisons). I coded my most recent design this way based on this belief. I’ll let you know when I can verify exactly the gates that Design Compiler synthesized 🙂
Thanks for the article, Jason. Your articles have helped answer many questions I’ve had. My colleague and I were discussing about this recently and a question came up – Are reverse case statements useful at all in plain Verilog (not System Verilog) if one isn’t allowed to use synthesis pragmas such as ‘parallel_case’? Unlike System Verilog, Verilog doesn’t have the ‘unique’ keyword.
Put in another way, the question is what really happens when one codes a reverse case statement in Verilog with no ‘parallel_case’ directive. Does synthesis assume that the input to the case-statement is not parallel and hence not one-hot and hence infer priority logic? I’d love to hear your thoughts.
I think if coded correctly, the reverse case statement FSM coding style should automatically create a case statement that is parallel, without requiring the ‘parallel_case’ directive. Since each state is represented by a unique number, each case expression is effectively comparing 1 unique register bit against 1’b1. Therefore no overlap between the different case expressions should be possible, which matches the definition of parallel case.
Leave a Comment Cancel reply
Notify me of follow-up comments by email.
Notify me of new posts by email.
This site uses Akismet to reduce spam. Learn how your comment data is processed .
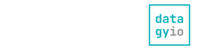
- Learn Python
- Python Lists
- Python Dictionaries
- Python Strings
- Python Functions
- Learn Pandas & NumPy
- Pandas Tutorials
- Numpy Tutorials
- Learn Data Visualization
- Python Seaborn
- Python Matplotlib
One-Hot Encoding in Scikit-Learn with OneHotEncoder
- February 23, 2022 April 14, 2024
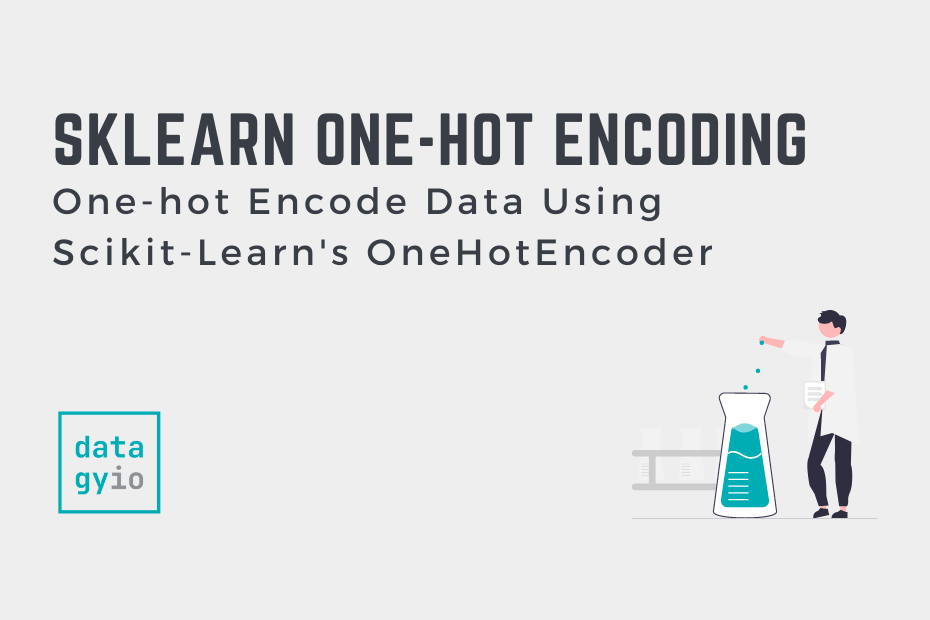
In this tutorial, you’ll learn how to use the OneHotEncoder class in Scikit-Learn to one hot encode your categorical data in sklearn . One-hot encoding is a process by which categorical data (such as nominal data) are converted into numerical features of a dataset. This is often a required preprocessing step since machine learning models require numerical data.
By the end of this tutorial, you’ll have learned:
- What one-hot encoding is and why it’s important in machine learning
- How to use sklearn’s OneHotEncoder class to one-hot encode categorical data
- How to one-hot encode multiple columns
- How to use the ColumnTransformer class to manage multiple transformations
Are you looking to one-hot encode data in Pandas? You can also use the pd.get_dummies() function for this!
Table of Contents
What is One-Hot Encoding?
One-hot encoding is the process by which categorical data are converted into numerical data for use in machine learning. Categorical features are turned into binary features that are “one-hot” encoded, meaning that if a feature is represented by that column, it receives a 1 . Otherwise, it receives a 0 .
This is perhaps better explained by an image:
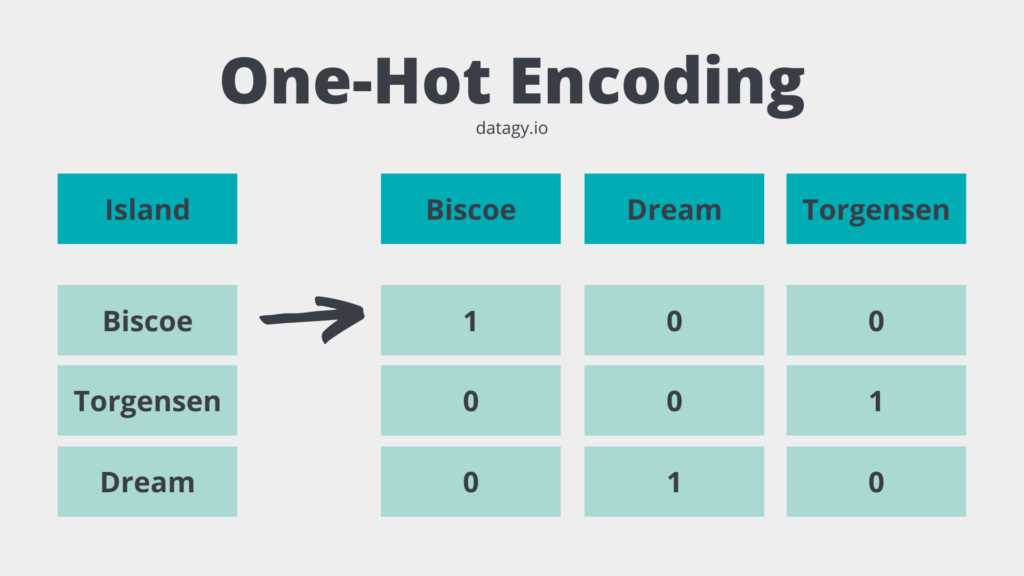
You may be wondering why we didn’t simply turn the values in the column to, say, {'Biscoe': 1, 'Torgensen': 2, 'Dream': 3} . This would presume a larger difference between Biscoe and Dream than between Biscoe and Torgensen.
While this difference may exist, it isn’t specified in the data and shouldn’t be imagined.
However, if your data is ordinal , meaning that the order matters, then this approach may be appropriate. For example, when comparing shirt sizes, the difference between a Small and a Large is , in fact, bigger than between a Medium and a Large.
Why is One-Hot Encoding Important to Machine Learning?
Now that you understand the basic mechanics of one-hot encoding, you may be wondering how this all relates to machine learning. Because machine learning algorithms assume (and require) your data to be numeric, categorical data must be pre-processed in order for it to be accepted .
Following the example of the Island above – if we were to ask any classification or regression model to be built using the categorical data, an error would be raised. This is because machine learning algorithms cannot work with non-numerical data.
How to Use Sklearn’s OneHotEncoder
Sklearn comes with a one-hot encoding tool built-in: the OneHotEncoder class. The OneHotEncoder class takes an array of data and can be used to one-hot encode the data.
Let’s take a look at the different parameters the class takes:
Let’s see how we can create a one-hot encoded array using a categorical data column. For this, we’ll use the penguins dataset provided in the Seaborn library . We can load this using the load_dataset() function:
Let’s break down what we did here:
- We loaded the dataset into a Pandas DataFrame, df
- We initialized a OneHotEncoder object and assigned it to ohe
- We fitted and transformed our data using the .fit_transform() method
- We returned the array version of the transformed data using the .toarray() method
We can see that each of the resulting three columns are binary values. There are three columns in the array, because there are three unique values in the Island column. The columns are returned alphabetically.
We can access the column labels using the .categories_ attribute of the encoder:
If we wanted to build these columns back into the DataFrame, we could add them as separate columns:
In the next section, you’ll learn how to use the ColumnTransformer class to streamline the way in which you can one-hot encode data.
How to Use ColumnTransformer with OneHotEncoder
The process outlined above demonstrates how to one-hot encode a single column. It’s not the most intuitive approach, however. Sklearn comes with a helper function, make_column_transformer() which aids in the transformations of columns. The function generates ColumnTransformer objects for you and handles the transformations.
This allows us to simply pass in a list of transformations we want to do and the columns to which we want to apply them. It also handles the process of adding the data back into the original dataset. Let’s see how this works:
Let’s see what we did here:
- We imported the make_column_transformer() function
- The function took a tuple containing the transformer we want to apply and the column to which to apply to. In this case, we wanted to use the OneHotEncoder() transformer and apply it to the 'island' column.
- We used the remainder='passthrough' parameter to specify that all other columns should be left untouched.
- We then applied the .fit_transform() method to our DataFrame.
- Finally, we reconstructed the DataFrame
In the next section, you’ll learn how to use the make_column_transformer() function to one-hot encode multiple columns with sklearn.
How to One-Hot Encode Multiple Columns with Scikit-Learn
The make_column_transformer() function makes it easy to one-hot encode multiple columns. In the argument where we specify which columns we want to apply transformations to, we can simply provide a list of additional columns.
Let’s reduce our DataFrame a bit to see what this result will look like:
In this tutorial, you learned how to one-hot encode data using Scikit-Learn’s OneHotEncoder class. You learned what one-hot encoding is and why it matters in machine learning. You then learned how to use the OneHotEncoder class in sklearn to one-hot encode data. Finally, you learned how to use the make_column_transformer helper function for the ColumnTransformer class to one-hot encode multiple columns.
Additional Resources
To learn more about related topics, check out the tutorials below:
- Introduction to Scikit-Learn (sklearn) in Python
- Pandas get dummies (One-Hot Encoding) Explained
- K-Nearest Neighbor (KNN) Algorithm in Python
- Introduction to Random Forests in Scikit-Learn (sklearn)
- Official Documentation: OneHotEncoder
Nik Piepenbreier
Nik is the author of datagy.io and has over a decade of experience working with data analytics, data science, and Python. He specializes in teaching developers how to use Python for data science using hands-on tutorials. View Author posts
4 thoughts on “One-Hot Encoding in Scikit-Learn with OneHotEncoder”
This was very helpful. Thanks!
Thanks so much, Lealdo!
When I used all the imports from the code listings I still got unresolved functions:
AttributeError: ‘ColumnTransformer’ object has no attribute ‘get_feature_names’
Is this just because older versions of ColumTransformer had this function or is there some import not listed?
Sorry for the late reply, Louis! I have fixed the code to what is shown below. Thanks for catching this!
columns=transformer.get_feature_names_out()
Leave a Reply Cancel reply
Your email address will not be published. Required fields are marked *
Save my name, email, and website in this browser for the next time I comment.
Bayes Classifier VS Regression Function #
Consider the two-class classification problem with a label set given by $\mathcal{Y}=\{-1,1\}$, without loss of generality. The regression function for the binary variable $Y$ is given by $$\begin{align*} \mu(x)=&\mathbb{E}[Y\mid X=x]\\=&\mathbb{P}(Y=1\mid X=x)\cdot 1\\&+\mathbb{P}(Y=-1\mid X=x)\cdot (-1)\\=&\mathbb{P}(Y=1\mid X=x)\\&-\mathbb{P}(Y=-1\mid X=x).\end{align*} $$
The Bayes classifier becomes nothing else but the sign of the regression function
$$ \underset{y\in\{-1,1\}}{\operatorname{argmax}}~\mathbb{P}(Y=y\mid X=x) =\operatorname{sign}(\mu(x)) $$
except for the feature values at the decision boundary $\{x:\mu(x)=0\}$ for which we can arbitrarily assign the labels.
Multiple-Class Classification #
What if we have multiple labels, say, with $\mathcal{Y}=\{\mathcal{C}_1,\ldots,\mathcal{C}_K\}$ for some finite $K\geq 2$? Unfortunately, the sign trick above is insufficient to distinguish more than two classes. To handle more classes, we need to encode the categorical target using a vector of dummy variables.
One-Hot Encoding # The one-hot encoding of categorical target $Y\in \{\mathcal{C}_1,\ldots,\mathcal{C}_K\}$ is the vector given by $$(Y^{(1)},\ldots,Y^{(K)})\in \{0,1\}^K$$ with $$Y^{(k)}=\mathbf{1}[Y=\mathcal{C}_k],~1\leq k\leq K$$ where $\mathbf{1}[A]$ denotes the indicator function that equals to 1 if condition $A$ is satisfied and equals to 0 otherwise.
In this example, there are $K=3$ teams with labels A, B, and C. We convert each observation of the label A/B/C into a vector of 3 dummies.
The $K$-dimensional regression function for the one-hot encoded target is given by
$$\mu(x)=(\mu_1(x),\ldots,\mu_K(x))$$ where $$\begin{align*}\mu_k(x)=&\mathbb{E}[Y^{(k)}\mid X=x]\\=&\mathbb{P}\left( Y=\mathcal{C}_k\mid X=x\right).\end{align*}$$
With one-hot encoding, one can now estimate the Bayes classifier using a two-step procedure:
- First estimate the multivariate regression function $\mu(x)$
- Then choose the label $h(x)\in\{\mathcal{C}_1,\ldots,\mathcal{C}_K\}$ with largest regression value.
Best Practices for One-Hot State Machine, coding in Verilog
There are 3 main points to making high speed state machines by one-hot encoding:
- Use 'parallel_case' and 'full_case' directives on a 'case (1'b1)' statement
- Use state[3] style to represent the current state
- Assign next state by:
- default assignment of 0 to state vector
- fully specify all conditions of next state assignments, including staying in current state.
Also, these points are also recommended:
- Separate the code for next state assignment from output logic/assignments.
- Use parameters to assign state encoding (or `define)
- use continuous assignments,
- set/reset at specific conditions, holding value at all other times
(the designer should choose based on complexity of generated logic)
Simple example:
reg [2:0] state ; parameter IDLE=0, RUN=1, DONE=2 ; always @ (posedge clock or negedge resetl) if ( ! resetl) begin state <= 3'b001 ; out1 <= 0 ; end else begin state <= 3'b000 ; case (1'b1) // synthesis parallel_case full_case state[IDLE]: if (go) state[RUN] <= 1 ; else state[IDLE] <= 1 ;
state[RUN]:
if (finished) state[DONE] <= 1 ; else state[RUN] <= 1 ;
state[DONE]:
state[IDLE] <= 1 ;
out1 <= state[RUN] & ! finished ;
If you want to read more in depth about all of these points, including why one-hot is useful for high speed, read this longer writeup .
I arrived at the conclusions here on my own, but around the same time, Cliff Cummings presented a paper at SNUG San Jose 2003 that included these same points: Cliff's paper Cliff's website
Chapter: Digital Logic Circuits : Asynchronous Sequential Circuits and Programmable Logic Devices
Using a one hot state assignment.
USING A ONE HOT STATE ASSIGNMENT
When designing with PGAS4, we should keep in mind that each logic cell contains two flip flops. This means that it may not be important to minimize the number of flip flops used in the design. Instead, we should try to reduce the number of logic cells used and try to reduce the interconnections between cells. In order to design faster logic, we should try to reduce the number of cells required to realize each equation. Using one hot state assigning will often help to accomplish this.
The one hot assignment uses one flip flop for each state, so a state machine with N states requires N flip flop. Exactly one flip flop is set to l in each state. For example a system with four (To T 1 , T 2 and T 3 ) could use four flip flop (O 0 , Q 1 , Q 2 and Q 3 ) with the following state assignment.

The other 12 combinations are not used.
We can write next state and output equations by inspection of the graph or by tracking link parts on an SM chart consider the partial state graph . The next state equation for flip flop Q3 could be written as.

However since Q=1 implies Q1=Q2=Q3=0 the Q1=Q2=Q3 term is redundant and can be eliminated, similarly, all the primed state variables can be eliminated from the other terms, so the next state equation reduces to.

Note that each contains exactly one state variable. similarly, each term in each output equation contains exactly one state variable.

When a one hot assignment is used, the next state equation for each flip flop will contain one term for each are leading into the corresponding state (or for each link path leading into the state). In general, each term in every next state equation and in every output equation will contain exactly one state variable. The one hot state assignment for asynchronous networks is similar to that described above, but a “holding term” is required for each next state equation.
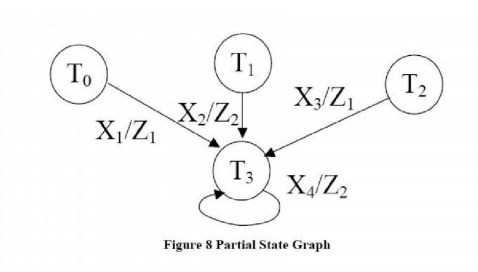
When a one hot assignment is used, resetting the system requires that one flip flop be set to 1 instead of resetting all flip flops to 0. If the flip flops used do not have a preset input (as in the case for the xilnx 3000 series), then we can modify the one hot assignment by replacing Q 0 and Q 0 ’ throughout. For the assignment, the modification is

And the modified equations are:
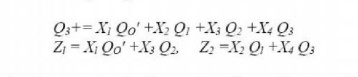
Another way to solve the reset problem without modifying the one hot assignment is to add an extra term to the equation for the flip flop, which should be 1 in the starting state. As an example, we use the one hot assignment given in (6-6) for the main dice game control. The next state equation for Q O is

If the system is reset to state 0000 after power-up, we can add the term Q 0 ’Q 1 ’Q 2 ’Q 3 ’ to the equation for Q 0 . Then, after the first clock the state will change from 0000 and 1000 (T0), Which is the correct starting state.
In general, both an assignment with a minimum number of state variables and a one hot assignment should be tried to see which one leads to a design with the smallest number of logic cells. Alternatively, if speed of operation is important, the design that leads to the fastest logic should be chosen. When a one hot assignment is used, more next state equations are required, but in general both the next state and output equation will contain fewer variables. An equation with fewer variable generally requires fewer logic cells to realize. Equations with five or fewer variables require a single cell. As seen in figure, an equation with six variables require cascading two cells, an equation with seven variables may require cascading three cells etc. The more cells cascaded, the longer the propagation delay, and the slower the operation.
STAT ASSIGNMENT RULES: A set of heuristic rules that attempts to reduce the cost of the combinational logic in a finite state machine.
ONE HOT STATE ASSIGNMENT: State assignment uses one flip flop for each state, so a state machine with N states requires N flip flops.
1. Reduce the following state table to the minimum number of states using successive partitioning method.
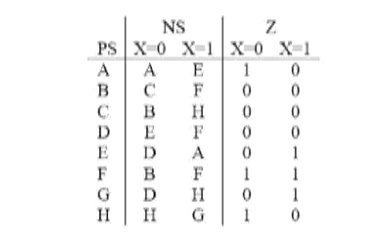
2. Reduce the following state table to the minimum number of states using implication chart method.
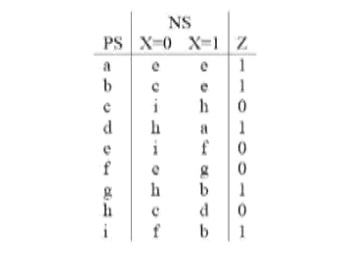
3. Use the heuristic rule on page 4 to make compact state assignment. Assign state A to “000”.
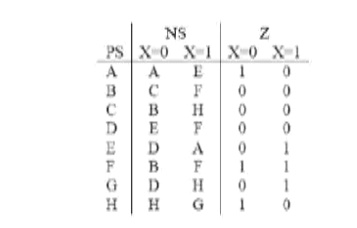
4. Implement the following state using D flip flop and gates. Use a one hot assignment and write down the logic equations by inspecting the state table. Let S 0 =001, S 1 =010, and S 2 =1000.
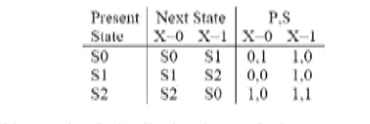
5. Repeat problem 1 using the implication chart.
6. Repeat problem 2 using the successive partitioning method.
7. Implement the state of problem 3 using one hot state assignment. Assume A=00000001, B=00000010, through H=10000000.
Related Topics
Privacy Policy , Terms and Conditions , DMCA Policy and Compliant
Copyright © 2018-2024 BrainKart.com; All Rights Reserved. Developed by Therithal info, Chennai.

- FPGA Families
TABLE OF CONTENTS
- INTRODUCTION
- THE MASKED GATE ARRAY ASIC
- Programmable Read Only Memories (PROMs)
- Programmable Logic Arrays (PLAs)
- Programmable Array Logic (PALs)
- CPLDs and FPGAs
- Complex Programmable Logic Devices (CPLDs)
- Field Programmable Gate Arrays (FPGAs)
- Choosing Between CPLDs and FPGAs
- Writing a Specification
- Designing the chip
- Simulating - design review
- Place and Route
- Resimulating - final review
- Top-Down Design
- Keep the Architecture in Mind
- Synchronous Design
- Floating Nodes
- Bus Contention
One-Hot State Encoding
- Testing Redundant Logic
- Initializing State Machines
- Observable Nodes
- Scan Techniques
- Built-In Self Test
- Signature Analysis
- Functional Simulation
- Static Timing Analysis
- Timing Simulation
FONT SIZE : A A A
For large grain FPGAs, which are the majority of architectures available, the normal method of designing state machines is not optimal. This is because the each CLB in an FPGA has one or more flip-flops, making for an abundance of flip-flops. For large combinatorial logic terms, however, many CLBs are often involved which means connecting these CLBs through slow interconnect. A typical state machine design, like the one shown in Figure 31, uses few flip-flops and much combinatorial logic. This is good for ASICs, bad for FPGAs.
The better method of designing state machines for FPGAs is known as one-hot encoding, seen in Figure 32. Using this method, each state is represented by a single flip-flop, rather than encoded from several flip-flop outputs. This greatly reduces the combinatorial logic, since only one bit needs to be checked to see if the state machine is in a particular state. It is important to note that each state bit flip-flop needs to be reset when initialized, except for the IDLE state flip-flop which needs to be set so that the state machine begins in the IDLE state.
< PREVIOUS
XC3S250E-4VQ100I
Manufacturer: Xilinx
- FPGA Spartan-3E Family 250K Gates 5508 Cells 572MHz 90nm Technology 1.2V 100-Pin VTQFP
Product Categories: FPGAs
Lifecycle: Active Active
RoHS: No RoHS
- Request Quote
XC3S250E-5TQG144C
- FPGA Spartan-3E Family 250K Gates 5508 Cells 657MHz 90nm Technology 1.2V 144-Pin TQFP EP
Product Categories: FPGAs (Field Programmable Gate Array)
XC2018-100TQ100C
- Field Programmable Gate Array (FPGA)
Product Categories:
Lifecycle: Any -
XC4020E-4HQ240C
- FPGA XC4000E Family 20K Gates 1862 Cells 0.35um Technology 5V 240-Pin HSPQFP EP
Lifecycle: Obsolete -
XC4VSX25-10FF668I
- FPGA Virtex-4 SX Family 23040 Cells 90nm Technology 1.2V 668-Pin FCBGA
Product Categories: Disjoncteur
If you have any questions about the product and related issues, Please contact us.
- Data Science
- Data Analysis
- Data Visualization
- Machine Learning
- Deep Learning
- Computer Vision
- Artificial Intelligence
- AI ML DS Interview Series
- AI ML DS Projects series
- Data Engineering
- Web Scrapping
- Machine Learning Tutorial
Getting Started with Machine Learning
- An introduction to Machine Learning
- Getting started with Machine Learning
- What is Machine Learning?
- Types of Machine Learning
- Best Python libraries for Machine Learning
- Difference Between Machine Learning and Artificial Intelligence
- General steps to follow in a Machine Learning Problem
- Machine Learning Mathematics

Data Preprocessing
- ML | Introduction to Data in Machine Learning
- ML | Understanding Data Processing
- Python | Create Test DataSets using Sklearn
- Generate Test Datasets for Machine learning
- ML | Overview of Data Cleaning
One Hot Encoding in Machine Learning
- ML | Dummy variable trap in Regression Models
- What is Exploratory Data Analysis ?
- ML | Feature Scaling - Part 1
- Feature Engineering: Scaling, Normalization, and Standardization
- Label Encoding in Python
- ML | Handling Imbalanced Data with SMOTE and Near Miss Algorithm in Python
Classification & Regression
- Ordinary Least Squares (OLS) using statsmodels
- Linear Regression (Python Implementation)
- ML | Multiple Linear Regression using Python
- Polynomial Regression ( From Scratch using Python )
- Implementation of Bayesian Regression
- How to Perform Quantile Regression in Python
- Isotonic Regression in Scikit Learn
- Stepwise Regression in Python
- Least Angle Regression (LARS)
- Logistic Regression in Machine Learning
- Understanding Activation Functions in Depth
- Regularization in Machine Learning
- Implementation of Lasso Regression From Scratch using Python
- Implementation of Ridge Regression from Scratch using Python
K-Nearest Neighbors (KNN)
- K-Nearest Neighbor(KNN) Algorithm
- Implementation of Elastic Net Regression From Scratch
- Brute Force Approach and its pros and cons
- ML | Implementation of KNN classifier using Sklearn
- Regression using k-Nearest Neighbors in R Programming
Support Vector Machines
- Support Vector Machine (SVM) Algorithm
- Classifying data using Support Vector Machines(SVMs) in Python
- Support Vector Regression (SVR) using Linear and Non-Linear Kernels in Scikit Learn
- Major Kernel Functions in Support Vector Machine (SVM)
Decision Tree
- Python | Decision tree implementation
- CART (Classification And Regression Tree) in Machine Learning
- Decision Tree Classifiers in R Programming
- Python | Decision Tree Regression using sklearn
Ensemble Learning
- Ensemble Methods in Python
- Random Forest Regression in Python
- ML | Extra Tree Classifier for Feature Selection
- Implementing the AdaBoost Algorithm From Scratch
- Gradient Boosting in ML
- CatBoost in Machine Learning
- LightGBM (Light Gradient Boosting Machine)
- Stacking in Machine Learning
Generative Model
- ML | Naive Bayes Scratch Implementation using Python
- Applying Multinomial Naive Bayes to NLP Problems
- Gaussian Process Classification (GPC) on the XOR Dataset in Scikit Learn
- Gaussian Discriminant Analysis
- Quadratic Discriminant Analysis
- Basic Understanding of Bayesian Belief Networks
- Hidden Markov Model in Machine learning
Time Series Forecasting
- Components of Time Series Data
- AutoCorrelation
- How to Check if Time Series Data is Stationary with Python?
- How to Perform an Augmented Dickey-Fuller Test in R
- How to calculate MOVING AVERAGE in a Pandas DataFrame?
- Exponential Smoothing in R Programming
- Python | ARIMA Model for Time Series Forecasting
Clustering Algorithm
- K means Clustering - Introduction
- Hierarchical Clustering in Machine Learning
- Principal Component Analysis(PCA)
- ML | T-distributed Stochastic Neighbor Embedding (t-SNE) Algorithm
- DBSCAN Clustering in ML | Density based clustering
- Spectral Clustering in Machine Learning
- Gaussian Mixture Model
- ML | Mean-Shift Clustering
Convolutional Neural Networks
- Introduction to Convolution Neural Network
- Image Classification using CNN
- What is Transfer Learning?
Recurrent Neural Networks
- Introduction to Recurrent Neural Network
- Introduction to Natural Language Processing
- NLP Sequencing
- Bias-Variance Trade Off - Machine Learning
Reinforcement Learning
- Reinforcement learning
- Markov Decision Process
- Q-Learning in Python
- Deep Q-Learning
- Deep Learning Tutorial
- Computer Vision Tutorial
- Natural Language Processing (NLP) Tutorial
Model Deployment and Productionization
- Python | Build a REST API using Flask
- How To Use Docker for Machine Learning?
- Cloud Deployment Models
Advanced Topics
- What is AutoML in Machine Learning?
- Generative Adversarial Network (GAN)
- Explanation of BERT Model - NLP
- What is a Large Language Model (LLM)
- Variational AutoEncoders
- Transfer Learning with Fine-tuning
- 100 Days of Machine Learning - A Complete Guide For Beginners
- 100+ Machine Learning Projects with Source Code [2024]
- Machine Learning Interview Questions
Most real-life datasets we encounter during our data science project development have columns of mixed data type. These datasets consist of both categorical as well as numerical columns. However, various Machine Learning models do not work with categorical data and to fit this data into the machine learning model it needs to be converted into numerical data. For example, suppose a dataset has a Gender column with categorical elements like Male and Female . These labels have no specific order of preference and also since the data is string labels, machine learning models misinterpreted that there is some sort of hierarchy in them.
One approach to solve this problem can be label encoding where we will assign a numerical value to these labels for example Male and Female mapped to 0 and 1 . But this can add bias in our model as it will start giving higher preference to the Female parameter as 1>0 but ideally, both labels are equally important in the dataset. To deal with this issue we will use the One Hot Encoding technique.
One Hot Encoding
One hot encoding is a technique that we use to represent categorical variables as numerical values in a machine learning model.
The advantages of using one hot encoding include:
- It allows the use of categorical variables in models that require numerical input.
- It can improve model performance by providing more information to the model about the categorical variable.
- It can help to avoid the problem of ordinality, which can occur when a categorical variable has a natural ordering (e.g. “small”, “medium”, “large”).
The disadvantages of using one hot encoding include:
- It can lead to increased dimensionality, as a separate column is created for each category in the variable. This can make the model more complex and slow to train.
- It can lead to sparse data, as most observations will have a value of 0 in most of the one-hot encoded columns.
- It can lead to overfitting, especially if there are many categories in the variable and the sample size is relatively small.
- One-hot-encoding is a powerful technique to treat categorical data, but it can lead to increased dimensionality, sparsity, and overfitting. It is important to use it cautiously and consider other methods such as ordinal encoding or binary encoding.
One Hot Encoding Examples
In One Hot Encoding , the categorical parameters will prepare separate columns for both Male and Female labels. So, wherever there is a Male, the value will be 1 in the Male column and 0 in the Female column, and vice-versa. Let’s understand with an example: Consider the data where fruits, their corresponding categorical values, and prices are given.
The output after applying one-hot encoding on the data is given as follows,
One-Hot Encoding Using Python
Creating dataframe.
Creating a dataframe to implement one hot encoding from CSV file.
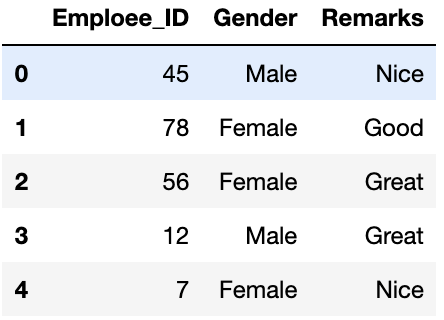
First five rows of Dataframe
Unique Elements in Categorical Column
we can use the unique() function from the pandas library to get unique elements from the column of the dataframe.
Count of Elements in the Column
We can use value_counts() function from pandas to get the counts of each element in the dataframe.
We have two methods available to us for performing one-hot encoding on the categorical column.
One-Hot Encoding of Categorical Column Using Pandas library
We can use pd.get_dummies() function from pandas to one-hot encode the categorical columns. This Function
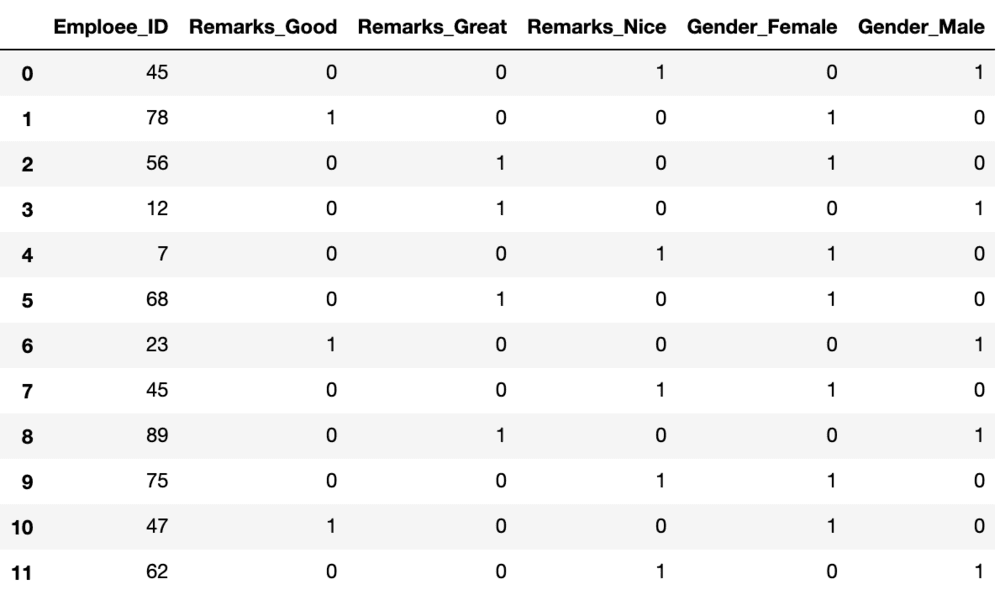
One-Hot encoded columns of the dataset
We can observe that we have 3 Remarks and 2 Gender columns in the data. However, you can just use n-1 columns to define parameters if it has n unique labels. For example, if we only keep the Gender_Female column and drop the Gender_Male column, then also we can convey the entire information as when the label is 1, it means female and when the label is 0 it means male. This way we can encode the categorical data and reduce the number of parameters as well.
One Hot Encoding using Sci-kit Learn Library
Scikit-learn(sklearn) is a popular machine-learning library in Python that provide numerous tools for data preprocessing. It provides a OneHotEncoder function that we use for encoding categorical and numerical variables into binary vectors.
Please Login to comment...
Similar reads.
- AI-ML-DS With Python
Improve your Coding Skills with Practice
What kind of Experience do you want to share?

IMAGES
VIDEO
COMMENTS
One-hot. In digital circuits and machine learning, a one-hot is a group of bits among which the legal combinations of values are only those with a single high (1) bit and all the others low (0). [1] A similar implementation in which all bits are '1' except one '0' is sometimes called one-cold. [2] In statistics, dummy variables represent a ...
9.6 ONE-HOT ENCODING METHOD One-hot encoding is an alternative state assignment method which attempts to minimize the combinational logic by increasing the number of flip-flops. The goal of the method … - Selection from Introduction to Digital Systems: Modeling, Synthesis, and Simulation Using VHDL [Book]
About Press Copyright Contact us Creators Advertise Developers Terms Privacy Policy & Safety How YouTube works Test new features NFL Sunday Ticket Press Copyright ...
SystemVerilog and Verilog has a unique (pun intended) and efficient coding style for coding one-hot state machines. This coding style uses what is called a reverse case statement to test if a case item is true by using a case header of the form case (1'b1). Example code is shown below: IDLE = 0, READ = 1, DLY = 2,
With one-hot encoding, each state has its own flip flop. Note: 'A' is the name of a state. It is also the name of the wire coming out from the flip flop for state 'A'. The same holds true for
One-Hot State Machines I One hot encoding uses the reversed case statement I In this style, case expression and case item are swapped I In one-hot encoding: I case expression is the literal (1'b1) to match against I case items are single bits in the present state vector case (1'b1) present_state[bit0]: next_state_assignment; present_state[bit1]: next_state_assignment;
There is another state assignment method, namely, one-hot encoding, which can simplify the "Logic to Generate the Outputs" and "Logic to Generate the Next State" blocks in Figure 2. With these two blocks simplified, we can generate the FSM outputs and next state faster. The next section discusses this encoding in more detail. One-Hot Encoding
One-hot encoding! One-hot: Encode n states using n flip-flops " Assign a single fi1fl for each state #Example: 0001, 0010, 0100, 1000 " Propagate a single fi1fl from one flip-flop to the next #All other flip-flop outputs are fi0fl! The inverse: One-cold encoding " Assign a single fi0fl for each state #Example: 1110, 1101, 1011, 0111
One-Hot Encoding CSC411: Machine Learning and Data Mining, Winter 2017 Michael Guerzhoy Slides from Geoffrey Hinton 0 1 0 0 0 0 0 0 0 0 0 1 1
One-hot state assignment. Simple easy to encode easy to debug Small logic functions each state function requires only predecessor state bits as input Good for programmable devices lots of flip-flops readily available simple functions with small support (signals its dependent upon) ... Many slight variations to one-hot one-hot + all-0.
Visit https://sites.google.com/view/daolam/teaching for extra study notesUniversity of California, San DiegoCSE 140 - Digital System Design
January 05, 2021 by Eduardo Corpeño. This article shows a comparison of the implementations that result from using binary, Gray, and one-hot encodings to implement state machines in an FPGA. These encodings are often evaluated and applied by the synthesis and implementation tools, so it's important to know why the software makes these decisions.
One-hot encoding is the process by which categorical data are converted into numerical data for use in machine learning. Categorical features are turned into binary features that are "one-hot" encoded, meaning that if a feature is represented by that column, it receives a 1. Otherwise, it receives a 0. This is perhaps better explained by an ...
One important decision in state encoding is the choice between binary encoding and one-hot encoding.With binary encoding, as was used in the traffic light controller example, each state is represented as a binary number.Because K binary numbers can be represented by log 2 K bits, a system with K states needs only log 2 K bits of state.
With one-hot encoding, one can now estimate the Bayes classifier using a two-step procedure: First estimate the multivariate regression function. μ ( x) \mu (x) μ(x) Then choose the label. h ( x) ∈ { C 1, …, C K } h (x)\in\ {\mathcal {C}_1,\ldots,\mathcal {C}_K\} h(x) ∈ {C 1. .
Best Practices for One-Hot State Machine, coding in Verilog. There are 3 main points to making high speed state machines by one-hot encoding: fully specify all conditions of next state assignments, including staying in current state. Also, these points are also recommended: Separate the code for next state assignment from output logic/assignments.
The one hot assignment uses one flip flop for each state, so a state machine with N states requires N flip flop. Exactly one flip flop is set to l in each state. For example a system with four (To T 1, T 2 and T 3) could use four flip flop (O 0, Q 1, Q 2 and Q 3) with the following state assignment. The other 12 combinations are not used.
One Hot Encoding is probably the most commonly utilised pre-processing method for independent categorical data, ensuring that the model can interpret the input data fairly, and without bias. This article will explore the three most common methods of encoding categorical data using the one-hot method, and discuss why you would want to use this ...
One-Hot Encoding. 1. Integer Encoding. As a first step, each unique category value is assigned an integer value. For example, " red " is 1, " green " is 2, and " blue " is 3. This is called a label encoding or an integer encoding and is easily reversible. For some variables, this may be enough.
About Press Copyright Contact us Creators Advertise Developers Terms Privacy Policy & Safety How YouTube works Test new features NFL Sunday Ticket Press Copyright ...
One-Hot Encoding: One-hot encoding is the process of turning categorical factors into a numerical structure that machine learning algorithms can readily process. It functions by representing each category in a feature as a binary vector of 1s and 0s, with the vector's size equivalent to the number of potential categories.
This is good for ASICs, bad for FPGAs. The better method of designing state machines for FPGAs is known as one-hot encoding, seen in Figure 32. Using this method, each state is represented by a single flip-flop, rather than encoded from several flip-flop outputs. This greatly reduces the combinatorial logic, since only one bit needs to be ...
One Hot Encoding. One hot encoding is a technique that we use to represent categorical variables as numerical values in a machine learning model. The advantages of using one hot encoding include: It allows the use of categorical variables in models that require numerical input. It can improve model performance by providing more information to ...
Bogus state transitions are obvi- ous, and current state display is trivial. One-hot state machine design for FPGAs. Steve Golson Trilobyte Systems, 33 Sunset Road, Carlisle MA 01741 Phone: 508/369-9669 Email: [email protected]. Page 2 -- March 30, 1993 -- 3rd PLD Design Conference, Santa Clara CA. Example design.