Not logged in
- Create account

Biography:Hilary Mason (data scientist)
Page actions.
- View source
Hilary Mason is an American data scientist and the founder of technology startup Fast Forward Labs [1] [2] as well as Data Scientist in Residence at Accel Partners. [3] She was the Chief Scientist at bitly. [4] On September 7, 2017, Cloudera announced that it had acquired Fast Forward Labs, and that Mason would become Cloudera's Vice President of Research. [5]
- 1 Early life and education
- 2.1 Fast Forward Labs
- 3 References
- 4 External links
Early life and education
Mason was born in New York City , and graduated from Grinnell College with a B.A. in computer science in 2000, and attended Brown University . [4] [6] [7] [8]
Mason was the chief data scientist at bitly for four years. She is the founder and CEO of Fast Forward Labs [9] and is a consultant data scientist at Accel, evaluating and advising on data strategies regarding technologies and investment opportunities. [10] [9] In 2010, she co-founded HackNY , a non-profit that helps integrate engineering students into the startup community in New York City. She has also been a member of Mayor Bloomberg's Technology and Innovation Advisory Council. [11] In addition, Mason is a prominent member of NYCResistor, a hacker collective located in Brooklyn. [12]
She has been interviewed by TechRepublic , [13] Forbes , [14] Wall Street Journal , ProgrammableWeb [15] and others. [16] [17] [18] She has further featured in both Glamour (magazine) [19] and Scientific American [20] [21]
Fast Forward Labs
Fast Forward Labs, founded by Mason, specializes in machine learning intelligence research. [22]
On September 7, 2017 it was announced that Fast Forward Labs was being acquired by Cloudera , a US open-source software company that offers support, training and software to business clients. [23] Cloudera extended this offer due to FFL's experience and expertise in applying machine learning to practical business problems and their commitment to continual academic and industrial research surveys on new techniques. [5] Mason explained that the merger of FFL with Cloudera would enable further and accelerated research opportunities on a larger platform. [24]
Mason received the TechFellows Engineering Leadership award in 2012 worth $100,000. [25] She was also on the Fortune 40 under 40 Ones to Watch list in 2011, [26] as well as Crain's New York 40 under Forty list. [4]
She was named within the Top 100 most creative people in business by Fast Company . [27]
- ↑ Novet, Jordan (21 July 2014). "Ex-Bitly chief scientist Hilary Mason launches Fast Forward Labs as an all-in-one emerging tech consultant" . VentureBeat . https://venturebeat.com/2014/07/21/fast-forward-labs-hilary-mason/ .
- ↑ Vanian, Jonathan (21 July 2014). "Former Bit.ly data scientist Hilary Mason starts own research consulting lab" . https://gigaom.com/2014/07/21/former-bit-ly-data-scientist-hilary-mason-starts-own-research-consulting-lab/ .
- ↑ Greenfield, Rebecca (6 November 2013). "Hilary Mason Will Make Sense Of Your Company's Massive Data Sets" . Fast Company . http://www.fastcompany.com/3020908/innovation-agents/hilary-mason-will-make-sense-of-your-companys-massive-data-sets .
- ↑ 4.0 4.1 4.2 Kavanaugh, Shane Dixon (12 October 2012). "Hilary Mason - 2012 - 40 Under Forty - Crain's New York Business" . Crain's New York blog . https://www.crainsnewyork.com/awards/hilary-mason .
- ↑ 5.0 5.1 Olson, Mike (7 September 2017). "Fast Forward!" . https://vision.cloudera.com/fast-forward/ .
- ↑ "Accel" . accel.com . http://www.accel.com/team/venture-development/bay-area/hilary-mason .
- ↑ "Hilary Mason '00 featured in "Why a Liberal Education Creates Great Entrepreneurs"" . Grinnell College . http://www.grinnell.edu/news/external/hilary-mason-00-featured-why-liberal-education-creates-great-entrepreneurs .
- ↑ "Scholars' Convocation: Hilary Mason ’00" . Grinnell College . 12 February 2016 . https://www.grinnell.edu/news/scholars-convocation-hilary-mason-00 .
- ↑ 9.0 9.1 "Hilary Mason About." . https://hilarymason.com/about/ .
- ↑ "Accel: Hilary Mason." . https://www.accel.com/team/hilary-mason .
- ↑ Ruth, João-Pierre. "Mayor Bloomberg Announces New Advisory Council on Technology at NY Tech Meetup." . https://www.xconomy.com/new-york/2011/10/13/mayor-bloomberg-announces-new-advisory-council-on-technology-at-ny-tech-meetup/ .
- ↑ "NYCResistor About." . https://www.nycresistor.com/about/ .
- ↑ Carson, Erin (15 January 2016). "Hilary Mason: Fast Forward Labs CEO. One-time aspiring taxi driver. Your nerd best friend." . http://www.techrepublic.com/article/hilary-mason-fast-forward-labs-ceo-one-time-aspiring-taxi-driver-your-nerd-best-friend/ .
- ↑ Woods, Dan. "bitly's Hilary Mason on "What is a Data Scientist?"" . https://www.forbes.com/sites/danwoods/2012/03/08/hilary-mason-what-is-a-data-scientist/ .
- ↑ Ohri, Ajay (28 January 2013). "Interview Hilary Mason Chief Scientist bitly" . http://www.programmableweb.com/news/interview-hilary-mason-chief-scientist-bitly/2013/01/28 .
- ↑ Cadei, Emily (20 August 2014). "Hilary Mason Turns Big Data Into Plain English | Rising Stars | OZY" . ozy . http://www.ozy.com/rising-stars/hilary-mason-turns-big-data-into-plain-english/33324 .
- ↑ Drell, Lauren (14 May 2013). "Data Science and Why Dogs Rule the Internet" . http://mashable.com/2013/05/14/hilary-mason-data/ .
- ↑ Reddy, Elaine Ellis (23 July 2012). "Data Stories: Interview with Hilary Mason of Bit.ly" (in en-US) . https://blog.gnip.com/hilary-mason-interview/ .
- ↑ Swisher, Kara (6 October 2011). "Women in Tech: We Really Do Need More!" . https://www.glamour.com/story/women-in-tech-we-really-do-need-more .
- ↑ Fischetti, Mark (16 November 2011). "Physics or Fashion? What Science Lovers Link to Most." . https://www.scientificamerican.com/article/graphic-science-science-lovers-web-traffic/ .
- ↑ Easter, Michael (1 April 2011). "Getting to Know You: Bit.ly Chief Scientist Finds Rich Data in Shortened Links." . https://www.scientificamerican.com/article/getting-to-know-you/ .
- ↑ "Fast Forward Labs: What we do" . https://fastforwardlabs.com/#what-we-do .
- ↑ "Cloudera: About" . https://www.cloudera.com/more/about.html .
- ↑ Mason, Hilary (7 September 2017). "Fast Forward Blog: To the Future..." . http://blog.fastforwardlabs.com/2017/09/07/to-the-future.html .
- ↑ Constine, Josh (22 February 2012). "The Winners Of This Year's $100,000 TechFellow Awards Are…" . https://techcrunch.com/2012/02/22/the-winners-of-this-years-100000-techfellow-awards-are/ .
- ↑ "40 Under 40: Ones to watch - Hilary Mason (13)" . Fortune . http://archive.fortune.com/galleries/2011/news/companies/1110/gallery.40_under_40_ones_to_watch.fortune/13.html .
- ↑ Chu, Jeff (13 May 2013). "99-100. Hilary Mason, Leslie Bradshaw" (in en-US) . http://www.fastcompany.com/3009220/most-creative-people-2013/99-100-hilary-mason-leslie-bradshaw .
External links
- Official website
- cloudera.com
- Information systems researchers
- Data scientists
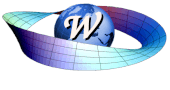
- Add a new article
- Search in all topics
- Search in namespaces
- Search in categories
- Search using prefix
- About HandWiki
- How to edit
- Citation manager
- Formatting articles
- List of categories
- Recent pages
- Recent changes
- Random page
- Support & Donate
- Special pages
- Cite this page
User page tools
- What links here
- Related changes
- Printable version
- Permanent link
- Page information
Other projects
In other languages.

- This page was last edited on 4 August 2023, at 03:19.
- Privacy policy
- Disclaimers
- Harvard Business School →
- News Story →
Ex-Bitly Chief Hilary Mason on Innovation Through Data
Post a comment.
Comments must be on-topic and civil in tone (with no name calling or personal attacks). Any promotional language or urls will be removed immediately. Your comment may be edited for clarity and length.
- 20 Mar 2024
- Harvard Business School
Free Speech and Community Values Working Group Q+A
- 18 Mar 2024
How to Face Those Middle-of-the-Night Fears for Your Company
- 17 Mar 2024
How To Find A Greater Sense Of Purpose At Work
- 15 Mar 2024
Harvard Business School Rock Center for Entrepreneurship Hosts First Annual Demo Day
→ Visit Newsroom
By clicking “Accept All Cookies,” you agree to the storing of cookies on your device to enhance site navigation and analyze site usage.
READ ABOUT OUR ACTION-PACKED YEAR! 2023 ANNUAL REPORT NOW AVAILABLE
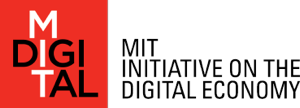
Search the site
The ide newsletter.
Get the latest from MIT IDE delivered weekly to your inbox.
MIT Initiative on the Digital Economy
MIT Sloan School of Management
245 First St, Room E94-1521
Cambridge, MA 02142-1347
617-452-3216
- share on facebook
- share on twitter
- share on linkedin
- share by email
Machine Learning Strides and Limitations: A conversation with Andrew McAfee, Hilary Mason, and Claudia Perlich
June 01, 2020
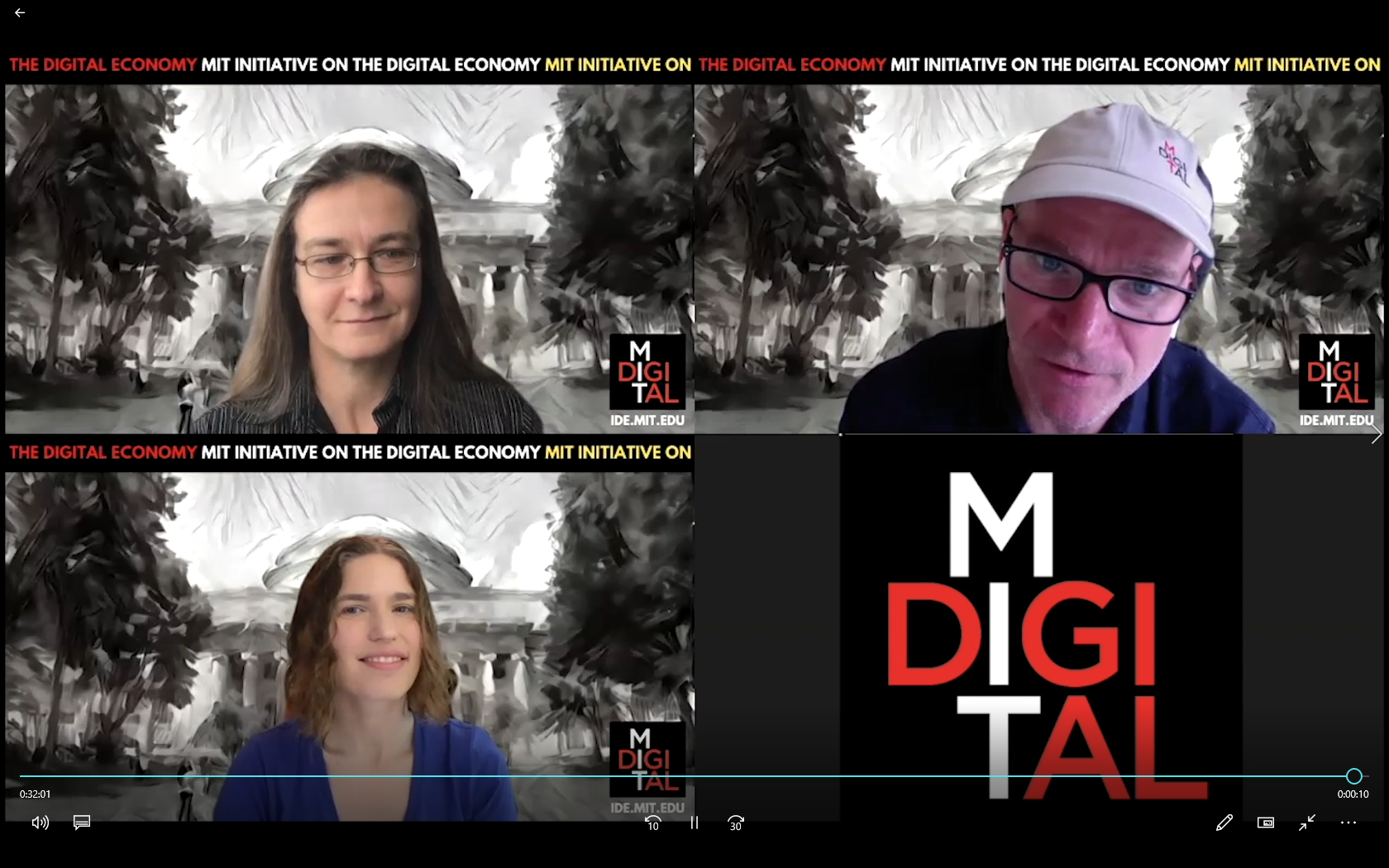
As AI and machine learning (ML) become more mainstream in business applications and more widely accepted by the public — in everything from ‘smart’ vacuum cleaners and navigation systems, to the ubiquitous Alexa and Siri — it’s important to view the strides as well as the shortcomings with a wide lens. MIT IDE Co-director and Principal Research Scientist, Andrew McAfee, did just that during a fireside chat at the recent IDE Annual Conference held virtually on May 20.
McAfee spoke with two “rock Starts in the discipline,” Hilary Mason, Data Science in Residence, at Accel (lower left in photo), and Claudia Perlich, Chief Scientist at Dstillery (upper left in photo), in a wide-ranging discussion of about machine learning’s past, present, and future.
What follows are some key excerpts and takeaways from the conversation.
Andrew McAfee: You’ve been around to watch ML go from a very niche discipline, something that was maybe used to recognize handwritten digits on checks, to this technology that appears to be taking over the economy . What was your ‘a-ha’ moment when you first realized the potential of ML?
Hilary Mason: I’ve had a series of those moments at different times in my career but the set of problems and places where the technology can actually be impactful has radically expanded over the past 10 years. ML techniques are not new; people have been using them in financial services and for really high-value problems for a very long time. But when I was starting out, we began to answer questions that previously had been out of reach from a technical perspective. For example, it allowed coders to go beyond the very narrow edges of the problem to create huge data domains with lots of human labor involved in the classification of data. We started having more generalized approaches to solving problems, and that is one of those step-function changes in capability that’s really interesting.
Claudia Perlich: I also saw many changes over the years. For the longest time data mining, as it was called then, was this niche course. Suddenly, people realized that data mining could span many different application domains; social sciences, medical applications, and even law — and everyone wanted to take the course! I knew all these different applications existed, but my frustration before 2010 had been our limitations to fun pilots where you try to see how far you can push the technology. I was never convinced that the programs were put to their best use — not because of insufficient data, or even incorrect data; there was no technology stack or an API where you could just ping the cloud, and get the answer. Back then, you had to almost manually re-implement everything from scratch.
Today, ML is a much more relevant value proposition across domains that couldn’t previously afford the technical infrastructure and the skills needed.
In addition, personalization has created new industries where auctions and billions of decisions are made daily in real-time based on analytics. Now, there’s an economic model — an affordable infrastructure — that didn’t exist before.
AM: What areas still need work?
HM: The commoditization of the underlying platform was hugely enabling. It started happening around 2008 or 2009, but it’s still happening right now. Deployment and monitoring of machine learning systems is still something of an open question. It’s not solved. And so we will continue to see this unfold over the next five to 10 years.
CP: We don’t yet have sufficient control systems that understand when the model might be going out of whack, or whether we’re really using it for the right cause. Most ML models typically are built for a specific use case, and the person who built it understands the boundaries, but beyond that, generalization becomes questionable, at best.
HM : When you’re dealing with data collected from the real world, it will change; the real world changes. Things happen for random reasons; human behavior changes over time. All of that impacts the accuracy of a model and a system. And that’s not even getting into potential adversarial attempts to impact it deliberately.
So, while ML is easier now, there’s still a lot of work to do in order to make sure that the things we make are working accurately, and are repeatable.
On the human side of the discipline, people inside of organizations must be working together efficiently, using the right features, have some understanding of the providence of those things and what they actually mean when they’re creating systems. We do have a fairly long road ahead of us, but that’s not to diminish the incredible progress.
AM: How would you describe ML’s progress and capabilities? What can we compare it to?
HM: Machine learning is the mobile phone of making decisions. The smartphone is a piece of infrastructure that fundamentally changes the way we do even common tasks in our everyday lives or business, but it doesn’t change everything. And the fact that we all walk around holding something like this is also not the end game.
It’s absolutely a big deal. It enables us to do things ways we couldn’t do them before. Something like Google maps on a mobile device is revolutionary; we not only look at it when we don’t know where we’re going — which, by the way, has made going new places a completely different experience than it used to be — but we now look at it when we know where we’re going to figure out the best way to get there, given current conditions. That means that two levels of information are available to us instantly, and that changes the whole experience of navigating an unknown place, but also a known place.
CP: It’s a technology that gives you additional information to help you make better decisions. What you do with the information you gather should still be your choice. Just because you build a classifier to detect breast cancer doesn’t mean that the computer is telling the person what the next step should be. It means you are given some additional component that should be integrated into a decision to ultimately achieve a better outcome.
For instance, in radiology, the benefit is not only finding out if there’s cancer in an image, but supporting radiologists with reports so they actually have more time to talk to the patient — work the machine learning can’t do. So classifying images is not the value proposition: It is giving professionals additional information or making their life and workflow easier.
AM: In other words, not making very experienced, highly trained and busy people act like clerks for a lot of their working day.
CP: Exactly right.
HM: I like framing this as reducing cognitive drudgery, and the drudgery is not always separable from the core of the work.
AM: What are some caveats as move forward with adoption?
CP: There has been lots of hesitation in adopting these technologies, and a good amount of care and concern is important. Transparency isn’t the main concern; machines are not much less transparent than people. The bigger concern is the scale at which machines can execute, which means that the potential for large scale, unintended side effects is so much bigger than with human design. I would always prefer that we’re thinking a lot harder about how we make decisions with the things that the machines give us, rather than asking how the machines came up with that thing in the first place.
If you think about biases in hiring, for example, you can try to forcefully make a model that’s gender-blind, but it’s going to be really, really painful and I wonder if we should be doing it at all. If you are convinced that you want to hire an equal ratio of men and women, nothing prevents you from doing so with the data you already have. Sometimes, pushing moral questions onto ML is extremely unfair and unproductive. Let’s not focus on getting complete transparency on the machine learning system, let’s focus on greater transparency about the ground rules that we’re using to make important decisions like who should we hire? Who should we let out on parole? Things like that.
At the same time, if I trust the machine, I need to be willing to go along with its recommendations, because let’s face it, if human cognition was as good as machine learning, we probably wouldn’t need it. The value proposition of machine learning is that it can do statistical things a lot better.
HM: There are clearly applications where using ML is really not a great idea, or we need to give people a better understanding of the way those decisions are being made — things like sending someone to jail, or giving someone financial credit. But there are also many applications that don’t have that same kind of impact, and maybe we’re willing to be a little more flexible on how we make those decisions; like, do we think this temperature sensor is giving us a good reading or not?
AM: What are you optimistic about?
HM: I am most optimistic about machines being able to interface with human beings, such as much better use of natural language, of looking at our environment and making inferences about what’s going on. This goes a long way toward alleviating that cognitive drudgery and those tedious tasks — in areas like healthcare or education — and to do more meaningful work as human beings. I wish it could free us to be more human and less focused on technology, or less constrained by technology. That’s my vision.I’m also terrified, because these same technologies can be used to manipulate people. We are not spending nearly enough time on the adversarial side of machine learning and the impact that has on decision-making. So I’m both very excited and also have a lot of concerns.
CP: My concern is the extent to which we are becoming less cognizant of what’s going on in the world and more tuned into our own tastes; we can become complacent and adopt a false sense of security about what we think we know.
Podcasts keyboard_arrow_right SDS 589: Narrative A.I. with Hilary Mason
Data Science Artificial Intelligence
SDS 589: Narrative A.I. with Hilary Mason
Podcast Guest: Hilary Mason
Tuesday Jul 05, 2022
Subscribe on Website , Apple Podcasts , Spotify , Stitcher Radio or TuneIn
- How narrative A.I. can assist creativity [5:14]
- How to build ML products that have no quantitative error function to optimize [10:31]
- How to ensure creative A.I. systems do not output non-sense or explicit content [16:58]
- Hilary's OSEMN data science process [21:05]
- The emerging ML technique Hilary is most excited about [24:58]
- What it takes to be successful as CEO of an early-stage A.I. company [27:20]
- What Hilary looks for in engineering hires [32:28]
- How Hilary's hopeful A.I. will transform our lives for the better in the decades to come [38:48]

- Data Science Insider
- Hidden Door
- Computer Scientist explains Machine Learning in 5 Difficulty Levels
- Hidden Door reveals its AI-powered narrative game platform
- OSEMN data science process
- The Kaiju Preservation Society by John Scalzi
- Download The Transcript
Podcast Transcript
Related podcasts.
Artificial Intelligence • 8 mins
SDS 766: Vonnegut’s Player Piano (1952): An Eerie Novel on the Current AI Revolution
Travis oliphant.
Uncategorized • 97 mins
SDS 765: NumPy, SciPy and the Economics of Open-Source, with Dr. Travis Oliphant
Data Science • 8 mins
SDS 764: The Top 10 Episodes of 2023
To revisit this article, visit My Profile, then View saved stories .
- Backchannel
- Newsletters
- WIRED Insider
- WIRED Consulting
Computer Scientist Explains Machine Learning in 5 Levels of Difficulty
Released on 08/18/2021
Hi, I'm Hilary Mason. I'm a computer scientist.
And today, I've been asked to explain machine learning
in five levels of increasing complexity.
Machine learning gives us the ability to learn things
about the world from large amounts of data
that we as human beings can't possibly study or appreciate.
So machine learning is when we teach computers
to learn patterns from looking at examples in data,
such that they can recognize those patterns
and apply them to new things that they haven't seen before.
[playful music]
I'm Hilary, what's your name?
I'm Brynn.
Do you know what machine learning means?
Have you heard that before?
So machine learning is a way that we teach computers
to learn things about the world by looking at patterns
and looking at examples of things.
So can I show you an example
of how a machine might learn something?
[Hilary] So is this a dog or a cat?
It's a dog.
And this one?
And what makes a dog, a dog and a cat, a cat?
Well, dogs are very playful, I think, more than cats.
Cats lick themselves more than dogs, I think.
That's true.
Do you think, if we look at these pictures,
do you think maybe we could say,
Well, they both have pointy ears,
but the dogs have a different kind of body
and the cats like to stand up a little different.?
Do you think that makes sense?
Yeah. Yeah.
What about this one?
I think, a cat?
Because it's more skinny.
And also, its legs are like really tall
and its ears are a little pointy.
This one's a jackal. And it's actually a kind of dog.
But you made a good guess.
That's what machines do too. They make guesses.
Is this a cat or a dog?
[Brynn] None.
[Hilary] None. What is it?
It's humans.
And how did you know that it's not a cat or a dog?
Because cats and dogs...
Because they walk on their paws
and their ears are like right here, not right here,
and they don't wear watches.
And so, you did something pretty amazing there.
Because we asked the question, Is it a cat or a dog?
And you said, I disagree with your question. It's a human.
So machine learning is when we teach machines
to make guesses about what things are
based on looking at a lot of different examples.
And I build products that use machine learning
to learn about the world and make guesses
about things in the world.
When we try to teach machines to recognize things
like cats and dogs, it takes a lot of examples.
We have to show them tens of thousands
or even millions of examples
before they can get even close to as good at it as you are.
Do you have tests in school?
Yeah, I have.
After every unit, we have a review and then we have a test.
Are those like the practice problems
you do before the test?
Well, just like everything that's gonna be on the test
is on the review.
Which means that in the test,
you're not seeing any problems
that you don't know how to solve.
As long as you did all your practice, right?
So machines work the same way.
If you show them a lot of examples and give them practice,
they'll learn how to guess.
And then when you give them the test,
they should be able to do that.
So we looked at eight pictures
and you were able to answer really quickly.
But what would you do if I gave you 10 million examples?
Would you be able to do that so quickly?
So one of the differences between people and machines
is that people might be a little better at this,
but can't look at 10 million different things.
So now that we've been talking about machine learning,
is this something you want to learn how to do?
Because I kind of want to become a spy.
And we used to do coding,
so I may be kind of good at it.
And machine learning is a great way to use
all those math skills, all those coding skills,
and would be a super cool tool for a spy.
[quirky music]
Hi. Are you a student, Lucy?
Yes, I just finished ninth grade.
Congratulations.
Thank you. It's very exciting.
Have you ever heard of machine learning before?
I'm going to assume that it means humans being able
to teach machines or robots how to learn themselves?
That's right.
When we teach machines to learn from data,
to build a model from that data or a representation of that,
and then to make a prediction.
One of the places we often find machine learning
in the real world is in things like recommendation systems.
So do you have an artist that you really like?
Yeah, Melanie Martinez.
So I'm gonna look up Melanie Martinez.
And it says here, If you like Melanie Martinez,
one of the other songs you might like is by Au/Ra.
Do you know who that is?
So let's listen to a hint of this song.
[alternative pop music]
So why do you think Spotify might've recommended that song?
Well, I know that in Melanie Martinez's music,
she used a lot of the filtered voice
to make it sound very deep and low
and that song had that.
And that's actually a really interesting thing
to think about because that creepy vibe
is something that you can perceive and I can perceive,
but it's actually really hard to describe to a machine.
What do you think might go into that?
Pitch of the music.
If it's really low or if it's super high,
it could know that.
What can the machine understand?
It's a great question.
The machine can understand
whatever we tell it to understand.
So there might be a person thinking about things,
like the pitch or the pacing or the tone,
or sometimes machines can figure out
things about music or images or videos
that we don't tell it to discover,
but that it can learn
from looking at a lot of different examples.
Why do you think companies might use machine learning?
Well, I think things like Facebook or Instagram,
they probably use it to target ads.
Sometimes, the ads you see are really uncanny.
And I think that's because they're based on so much data.
They know where you live. They know where your device is.
It's also important to realize that people in aggregate
are actually pretty predictable.
Like when we talk to each other,
we like to talk about the novel things,
like here, we're having this conversation.
We don't do this every day.
But we probably still eat breakfast.
We're gonna eat lunch. We're gonna eat dinner.
You probably are going to the same home
you go to most of the time.
And so, they're able to take that data
that we already give them and make predictions based on that
as to what ads they should show us.
So, you're saying I give them enough data as it is
about what I might be talking about or thinking about
that they can read my mind,
[Hilary laughs]
but just use the data that I've already given them.
And it almost seems like
they're watching us. That's right.
To do machine learning, we use something called algorithms.
Have you heard of algorithms before?
A set of steps or a process
carried out to complete something?
So do you think that we've been able
to teach machines enough
so that they can do things that even we can't do?
And on the opposite side of that,
do you think there are things that we can do
that a machine might never be able to do?
So there are things that machines are really great at
that humans are actually not great at.
And imagine watching every video posted to TikTok every day.
So we just don't have enough time to do that
at the rate at which we can actually watch those videos.
But a machine can analyze all of them
and then make recommendations to us.
And then thinking about things that machines are bad at
and people are good at, people are really great
with only one or two examples of learning something new
and incorporating that into our model of the world
to make good decisions.
Whereas machines often need tens of thousands of examples,
and that's not even getting into things like good judgment
because we care about people,
because we can imagine a future that we want to live in
that doesn't exist today.
And that's something that is still uniquely human.
Machines are great at predicting
based on what they've seen in the past,
but they're not creative.
They're not going to invent.
They're not gonna, you know,
really change where we're gonna go.
That's up to us.
[serene music]
I'm Sunny.
[Hilary] And what are you majoring in?
I study Math and Computer Science.
So in your studies,
have you learned about machine learning?
So to me, machine learning is essentially
exactly what it sounds like.
It's trying to teach a machine specifics about something
by inputting a lot of data points
and slowly, the machine will build up knowledge
about it over time.
For example, my Gmail program,
I assume that there would be a lot of, like,
machine learning models happening at once, right?
Absolutely.
And that's a great example because you have models
that are operating to do things like figure out
if a new email is spam or not.
So what would you think
about if you were looking at an email
and trying to decide if it went in one category or another?
I'd probably look at certain keywords.
Maybe if the recipient and the sender
had exchanged emails before
and generally, those fell into in the past.
So these are things we would call features.
And we go through a process where we do feature engineering,
where somebody looks at the example and says,
Okay, these are the things that I think might allow us
to statistically tell the difference
from something in one category versus another.
So for example, perhaps you don't speak Russian,
you start getting a lot of email in Russian.
Obviously, like the features that you just described
are features which a person would have had to think about.
Are there features
which, like, the machine itself could learn?
This is a great question
because it really gets to the difference
between some of our different tools
in our machine learning tool belt
in addressing problems like this.
So if we were to use a supervised learning classic
classification approach,
a person would need to think about those features
and creatively come up with them
in approach we call the kitchen sink approach,
which is just try everything you can possibly think of
and see what works.
Unsupervised learning, where we don't have labeled data
and we're trying to infer some structure out of the data
is you're projecting that data into a space
and looking for things like clusters.
And there's a bunch of really fun math
about how you do that, how you think about distance
and by distance, I mean that if we have two data points
in space, how do we decide if they're similar or not?
And how do the algorithms themselves usually differ
between unsupervised and supervised learning.
Supervised learning, we have our labels
and we're trying to figure out what statistically indicates
if something matches one label or another label.
Unsupervised learning,
we don't necessarily have those labels.
That's the thing we're trying to discover.
So reinforcement learning is another technique
that we use sometimes.
You can think about it like a turn in a game
and you can play, you know, millions and millions of trials
so that you're able to develop a system
that by experimenting with reinforcement learning
can eventually learn to play these games
pretty successfully.
Deep learning, which is essentially using neural networks
and very large amounts of data to eventually iterate
on a network structure that can make predictions.
With reinforcement learning versus deep learning,
it seems to me that reinforcement learning,
is it sort of like the kitchen sink approach
that you were talking about earlier,
where you're just kind of trying everything?
It is, but it also thrives in environments
where you have a decision point,
a pallet of actions to choose from.
And it actually comes historically
from trying to train a robot to navigate a room.
If it bonks into this chair, it can't go forward anymore.
And if it falls into that pit,
you know, it's not going to succeed.
But if it keeps exploring, it'll eventually get to the goal.
Oh, like roombas?
[Hilary] Yes.
[both laugh]
Oh, I didn't realize it was that deep, almost.
Is there a situation which you'd want to use
a deep learning algorithm
over a reinforcement learning algorithm?
So typically, you would choose deep learning
if you have sufficient high quality data,
hopefully labeled in a useful way.
If you really are happy not to necessarily understand
or be able to interpret what your system is doing
or you're willing to invest
in another set of work afterwards to understand
what the system is doing once you've already trained it.
And this also comes down to the fact that some things
are actually really easy to solve with linear regression
or simple statistical approaches.
And some things are impossible.
What would be the outcome if you were to choose
the, quote-unquote, wrong approach?
You build a system that could actually be useless.
So years ago, I had a client that was a big telecom company
and they had a data scientist
who built a deep learning system to predict customer churn.
It actually was very accurate, but it wasn't useful
because nobody knew why the prediction was what it was.
So they could say, you know,
Sunny, you're likely to quit next month.
But they had no idea what to do about it.
And so, I think there are a bunch of failure modes.
Would that be an example of, like, linear regression
where the regression is accurate, but,
you know, for marketing purposes, it's like,
if you don't know why I'm quitting the service,
then how can we fix this?
This is actually a good example of a very real world
kind of machine learning problem where the solution to this
was to build an interpretable system
on top of the accurate predictions not to throw it away,
but to do a bunch more work to figure out the why.
How can we improve machine learning algorithms?
It's actually fairly new
that we're able to solve all of these problems
and start to build these products and apply it in businesses
and apply it, you know, everywhere.
And so, we're still developing good practices
and what it means to be a professional in machine learning.
We're really developing a notion of what good looks like.
I'm in my first year of a PhD in Computer Science
and I'm studying natural language processing
and machine learning.
So would you mind telling me a bit about
what you've been working on or interested in lately?
I've been looking at understanding persuasion
in online text and the ways that we might be able to
automatically detect the intent behind that persuasion
or who it's targeted at
and what makes effective persuasive techniques.
So what are some of the techniques you're applying
to look at that debate data?
Something I'm interested in exploring
is how well it works to use deep learning
and sort of automatically extracted features from this text
versus using some of the more traditional techniques
that we have, things like lexicons
or some sort of template matching techniques
for extracting features from texts.
That's a question I'm just interested in, in general.
When do we really need deep learning
versus when can we use something
that's a little bit more interpretable,
something that's been around for a while?
Do you think there are going to be general principles
that guide those decisions?
Because right now, it's generally
up to the machine learning engineer to decide
what tools they want to apply.
I definitely think there is,
but I also, sort of, see it varying a lot
based on the use case,
something that, kind of, works out of the box
and maybe works a little bit more automatically
might be better.
And in other cases, you do, sort of, kind of,
you want a lot of fine grain control.
So is that where some of that frustration
around the lack of controllability
and interpretability comes from?
Yeah, if you're building a model
that just predicts the next thing
based off of everything it's seen from texts online,
then yeah, you're really gonna be replicating
whatever that distribution online is.
If you train a model off of language off the internet,
it sometimes says uncomfortable things
or inappropriate things and sometimes really biased things.
Have you ever run into this yourself?
And then how do you think about that problem
of potentially even measuring the bias
in a model that we've trained?
Yeah, it's a really tricky question.
As you said, these models are trained to, sort of, predict
the next sequence of words,
given a certain sequence of words.
So we could start with just, sort of, prompts
like the woman was versus the man was,
and, kind of, pull out common words
that are, sort of, more used
with one phrase versus the other.
So that's, sort of, a qualitative way of looking at it.
It's not ever kind of a guarantee of how the model
is gonna behave in one particular instance.
And I think that's what's really tricky
and that's why I, sort of, think it's really good
for creators of systems to just be honest
about, This is, sort of, what we have seen.
And so then, someone can make their own judgment about,
Is this gonna be too high risk
for, sort of, my particular use case?
I imagine in the last few years,
we've seen a lot of changes and improvements
in the capabilities of NLP systems.
So is there anything in that
that you're particularly excited about exploring further?
I'm really interested in, sort of, the creative potential
that we've started to see from NLP systems
with things like GPT-3
and other really powerful language models.
It's really easy to write long grammatical passages
thinking about the way that we can then harness, like,
the human ability to actually give meaning to those words
and, sort of, provide structure
and how we can combine those things with the, kind of like,
generative capabilities of these models now
is really interesting.
Yeah, I agree.
So, hi Claudia. It's so great to see you.
It has been far too long.
You know, we first met 10, 11 years ago
and machine learning has changed a lot since then.
Tooling that we now have, the capacity,
and also, an elevation of the problem sets
that we're dealing with and how to frame the problem.
And I'm almost struggling to figure out
whether it's a blessing or curse that it has become
as accessible and as democratized and as easy to execute
and you just build another new company from scratch.
And so, what's been, kind of, your reflection on that?
Well, you're absolutely right that the attention
machine learning gets has grown dramatically.
20 years ago, going to gatherings
and telling people what I was working on
and seeing the blank face or the like,
Where's the turn? and walk away.
Like, Oh, no.
The accessibility of the tooling,
like, we can now do in, like, five lines of code
something that would have taken 500 lines
of very mathematical, messy, gnarly code
even, you know, five years ago.
And that's not an exaggeration.
And there are tools that mean that pretty much anyone
can pick this up and start playing with it
and start to build with it.
And that is also really exciting.
In contrast, what I'm struggling with,
the friend of mine who asked me
to look at some health care data for him.
And despite the capabilities that we're having
in all of the, kind of, bigger societal problems
alongside with data collection engineering,
all the gnarly stuff,
that is actually not the machine learning itself,
it's the rest of it where certain data isn't available.
And to me, it's staggering how difficult it is
to get it off the ground and actually use.
And part of the challenge of it
is not the mathematics of building models,
but the challenge is making sure that the data
is sufficiently representative, potentially high quality.
And how transparent do I need to build it
for it to be adopted at some point?
What types of biases in the data collection,
and then also in the usage?
We now call it the bias, but we're still struggling
with the society not really living up to its expectations
and then machine learning, bringing it to the forefront.
And so, to say that another way,
when you're collecting data from the real world
and then building machine learning systems
that automate decisions based on that data,
all of the biases and problems
that are already in the real world then can be magnified
through that machine learning system.
And so, it can make many of these problems much worse.
Feeling increasingly challenged
that my skillset of being very good at programming
has become somewhat secondary.
And it's feeling...
It's really the bigger picture understanding
of Who would be using that?
How transparent do I need to build it
What types of biases in the data collection
I think, in certain areas, we have societal expectations
as to what is fair and what isn't.
And so, it's not just the provenance of that data,
but it's, sort of, deeply understanding,
Why does it look the way it looks?
Why was it collected this way?
What are the limitations of it?
We need to think about that
in entire process, how we document that process.
This is an issue in companies
where somebody might create something
that even their peers can't recreate.
What have you seen in terms of which industries,
where they stand, like who is adopting now?
Who is ready to utilize it?
Where would you maybe wish that didn't even try?
These are great questions.
So things like actuarial science, operations research,
where they actually are not using machine learning
as much as you might think.
And then you have other sorts of companies
or on the FinTech side, or even the ad tech side of things
where they perhaps are using machine learning
to the point of even absurdity.
So I spent about eight years working in ad tech.
And the motivation was really
because it was such an amazingly exciting playground
to push that technology
that used to largely live in academia, really,
out in the world and see, kind of, what it can achieve.
It has created such a hunger for data
that now everything is being collected.
I'm curious, when are we going to
make a foray into things like agriculture
about smart production of the things we eat?
You see and hear these interesting stories,
but I feel like we're not ready yet
to put that into a economically viable situation.
So when we think about the next five to 10 years,
the things that are really still holding us back
are these uneven applications of resources to problems
because the problems that get attention
are the high value ones
in terms of how much money you can make
or the things that are fashionable enough
that you can publish a paper on it.
So what do you think is holding us back?
I fully agree on the steps you pointed out
and the processes.
I think there is a chicken and egg problem,
like your former example,
that these areas that need to wait for data,
the value of the data collection
is then also slightly less apparent.
And so, it gets delayed further
and you'll see that happening.
But what my experience has been,
there's unfortunately, I feel a drifting apart
between academia
and the uses of AI,
but I am somewhat frustrated with a generation of students
who have standard data sets that they never think about
what the model needs to be used for,
that they never have to think about
how the data was collected.
So with all of these challenges ahead of us,
how optimistic are you about this world
that I deeply believe we can create
and the steps towards it?
I am incredibly optimistic and not...
Perhaps it's a personality flaw, but I can't help but look
at the potential of the technology to reduce harm,
to give us information that help us make better decisions.
And to think that we would choose
to address the big problems ahead of us.
I don't think we have a hope of addressing them
without figuring out the role
that machine learning will play.
And to think that we would then choose not to do that
is just unthinkable.
Despite that the rightfully raised concerns
about the challenges ahead,
but I think they also make us as a society better.
They challenge us to be a lot clearer
of what fairness means to all of us.
So with all of the setbacks,
I think we have exciting years to come.
And I am looking forward to a world where a lot more of that
is used for the right purposes.
[gentle upbeat music]
I hope you learned something about machine learning.
There has never been a better time to study machine learning
because you're now able to build products
that have tremendous potential and impact
across any industry or area that you might be excited about.
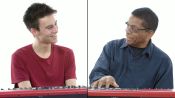
Musician Explains One Concept in 5 Levels of Difficulty
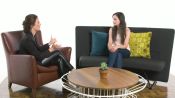
Expert Explains One Concept in 5 Levels of Difficulty - Blockchain
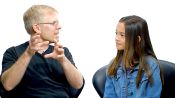
Oculus' John Carmack Explains Virtual Reality in 5 Levels of Difficulty
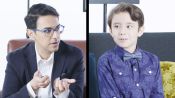
Biologist Explains One Concept in 5 Levels of Difficulty - CRISPR
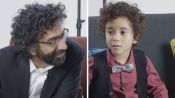
Neuroscientist Explains One Concept in 5 Levels of Difficulty
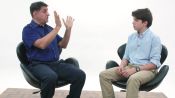
Astronomer Explains One Concept in 5 Levels of Difficulty
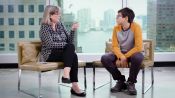
Laser Expert Explains One Concept in 5 Levels of Difficulty
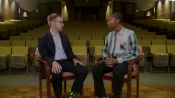
Sleep Scientist Explains One Concept in 5 Levels of Difficulty
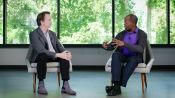
Physicist Explains One Concept in 5 Levels of Difficulty
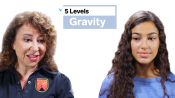
Astrophysicist Explains One Concept in 5 Levels of Difficulty
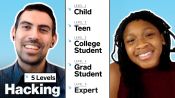
Hacker Explains One Concept in 5 Levels of Difficulty
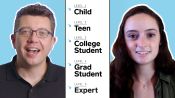
Nanotechnology Expert Explains One Concept in 5 Levels of Difficulty
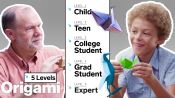
Physicist Explains Origami in 5 Levels of Difficulty
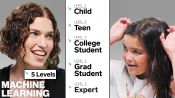
Neuroscientist Explains Memory in 5 Levels of Difficulty
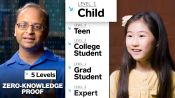
Computer Scientist Explains One Concept in 5 Levels of Difficulty
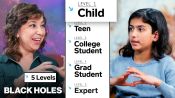
Astrophysicist Explains Black Holes in 5 Levels of Difficulty
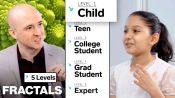
Computer Scientist Explains Fractals in 5 Levels of Difficulty
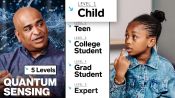

College Professor Explains One Concept in 5 Levels of Difficulty
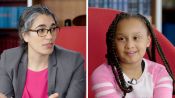
Quantum Computing Expert Explains One Concept in 5 Levels of Difficulty
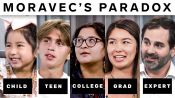
UMass Professor Explains the Internet in 5 Levels of Difficulty
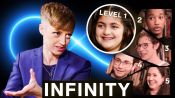
Mathematician Explains Infinity in 5 Levels of Difficulty
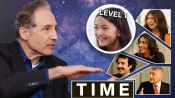
Theoretical Physicist Explains Time in 5 Levels of Difficulty
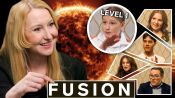
MIT Professor Explains Nuclear Fusion in 5 Levels of Difficulty
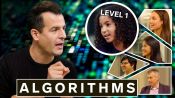
Harvard Professor Explains Algorithms in 5 Levels of Difficulty
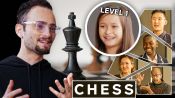
Chess Pro Explains Chess in 5 Levels of Difficulty (ft. GothamChess)
Trending video.
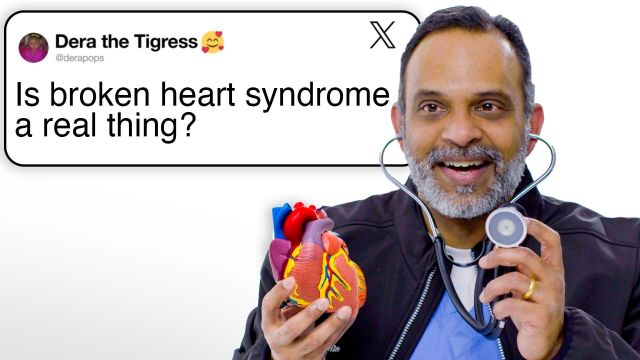
Cardiologist Answers Heart Questions From Twitter | Tech Support
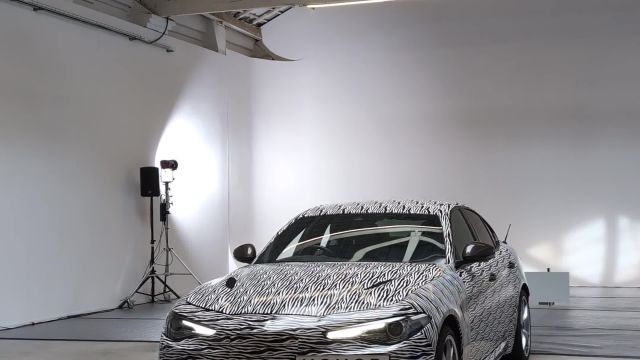
Honor Eye Tracking 1
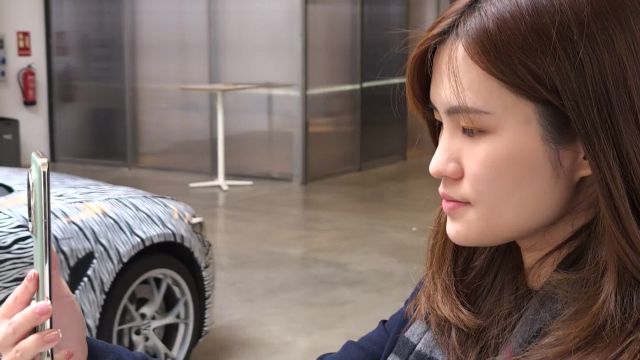
Honor Eye Tracking 2
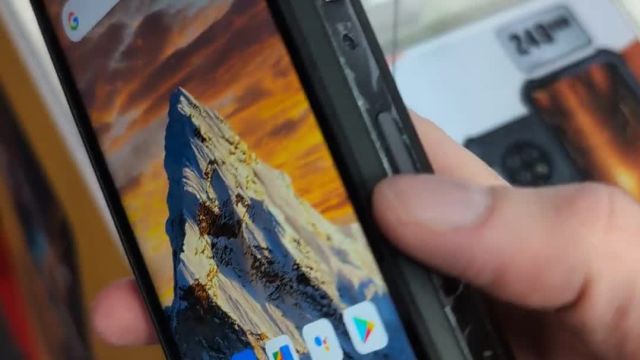
Energizer’s Latest Android Phone Has a Stupidly Big Battery

Hilary Mason
Hilary mason's career is defined by pushing the limits of what big data and machine learning can achieve. she is the co-founder and ceo of hidden door, a new gameplay platform that uses generative ai and llms to allow users to play in the world of their favorite book, movie, or tv show. she is also the founder of fast forward labs, a machine intelligence research company. a genuinely charming speaker who resonates with both tech and nonspecialist audiences, hilary speaks about the challenges and the rewards of working with big data, with a special focus on machine learning., in her former role as the data scientist in residence at accel, a leading silicon valley venture capital firm, she advised companies large and small on their data strategy. in her four-year tenure as chief scientist for bitly, the popular link-shortening service, she led a team that studied attention on the internet in realtime, doing a mix of research, exploration, and engineering., hilary is the co-founder of hackny, a non-profit that helps talented engineering students find their way into the startup community of creative technologists in new york city; co-host of datagotham, an annual event for professionals involved in data science; and member of nycresistor. she advises a number of companies, including mortar, knod.es, collective[i], datakind, betaspring, and techstars new york. she also served on mayor bloomberg’s technology and innovation advisory council., hilary is on fast company 's 100 most creative people in business 2013 and 1000 most creative people in business 2014. other honors include the techfellows engineering leadership award, the fortune 40 under 40 ones to watch list and the crain’s new york business 40 under forty., the secret life of links.
What we've learned from analysis of social media data.
How Big Data Is Changing the Way We Work, Live, and Dream
More and more data is being generated by people engaging in everyday activities. The resulting data exhaust is an amazing source of insight about human behavior — insights based on actual behaviors, not samples. This talk will review the evolution of big data technologies; explore why this shift is happening now; and discuss is likely to change in the near future. Mason will share stories of big data and analytics done well by different types of organizations. She will also explain practical tools and techniques attendees can consider when thinking about how to use their own data — as well as how to best use their companies' data.
Engineering Our Data-driven Future
We're seeing a shift from developing systems that generate data as a side-effect to designing products that help people make better decisions from data. Hilary explains what this means for technologists, why it's happening now, and what the opportunities look like, with examples from her work at bitly and others.
Data science: What is data science?
How do you train data scientists? And how do you build data teams?
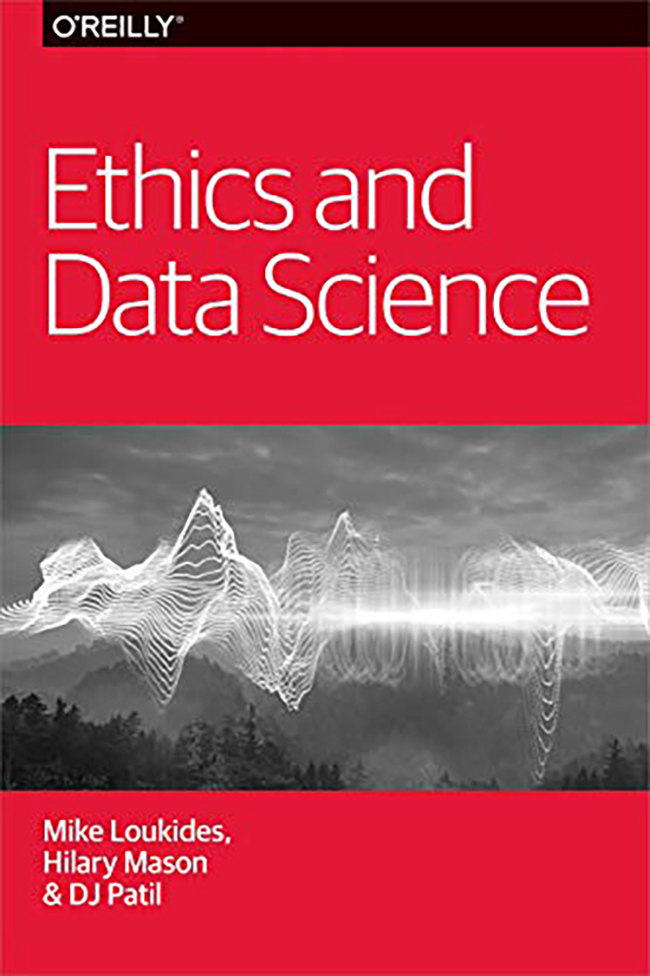
Testimonials
Hilary did a great job, audience responded well. [She] 'rocked it'.
Thanks Hilary. You were fantastic!!! You made a somewhat boring topic so interesting and applicable to work and life. Th
Related speakers
People also viewed.
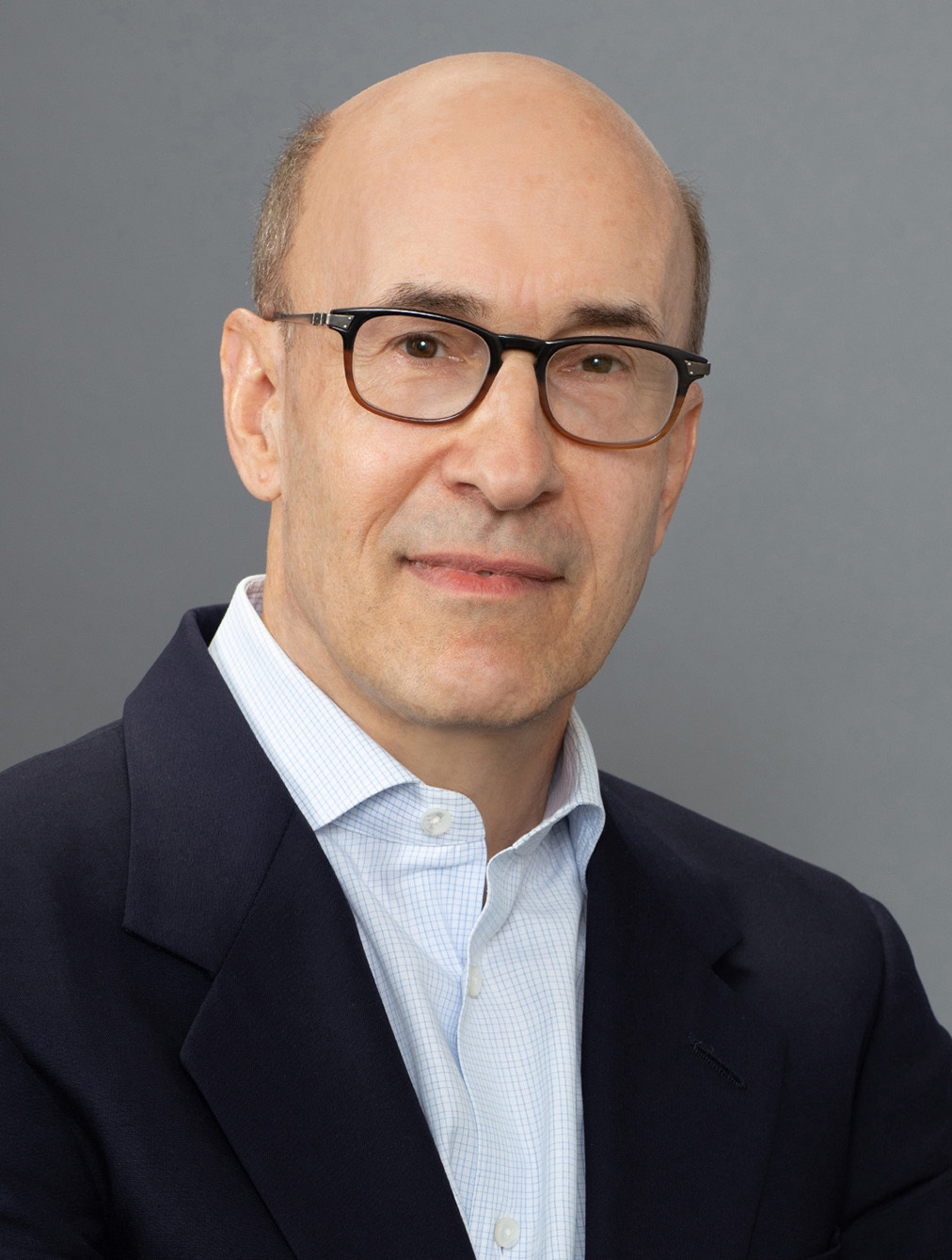
Our team will get back to you with availability and fees
*We will use the information provided above to contact you in relation to your inquiry. You may contact us at any time to unsubscribe from our communications. For further details on how your data is used and stored, please see our privacy policy.
Building at the intersection of AI and games
Established in 2020, Hidden Door is building a new kind of story game experience, powered by a proprietary generative AI platform, that can adapt any existing work of fiction into an online social roleplaying game.
We're excited to collaborate with storytellers from around the world, to create immersive fan-focused story experiences for everyone.
We're an intersectional mix of game professionals and AI experts, and a wide range of perspectives, that we’ve recruited to invent a delightful & safe experience for players.
Our Investors
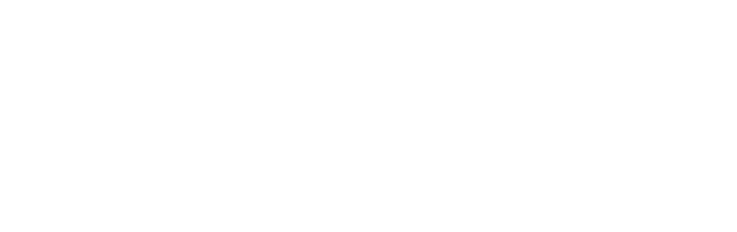
Support our work
Hilary Mason
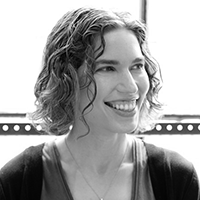
Hilary Mason is the Founder of Fast Forward Labs, a machine intelligence research company, and the Data Scientist in Residence at Accel. Previously, Hilary was the Chief Scientist at bitly. She also co-founded of HackNY, co-host DataGotham, and is a member of NYCResistor.
- Select Type
- Academic Article
- Announcement
- Book or Chapter
- Installation
- Policy Brief
- Press Coverage
- video Data Driven NYC A Process for Discovery Hilary Mason D&S advisor Hilary Mason presents her process for discovering changes in markets. Read on Data Driven NYC December 2016
- podcast ScienceFriday.com Will Our Smartphones Know Us Better Than Ourselves? Hilary Mason "On Monday, Apple revealed a more inquisitive Siri for iOS 9—a direct competitor to Android’s Google Now. Each smartphone feature seeks to anticipate your next move by offering up suggestions, directions, and the like. [D&S... Read on ScienceFriday.com June 2015

06 April 2016

Hilary Mason: Geek of the Week
Founder of machine intelligence research company Fast Forward Labs, Hilary Mason is the Data-Scientist-in-residence at Accel, and the former Chief Scientist at bitly. She is famous for proving that social media users like to share breaking news and current events, but are more likely to read inane gossip, watch sneezing pandas, or play online games.
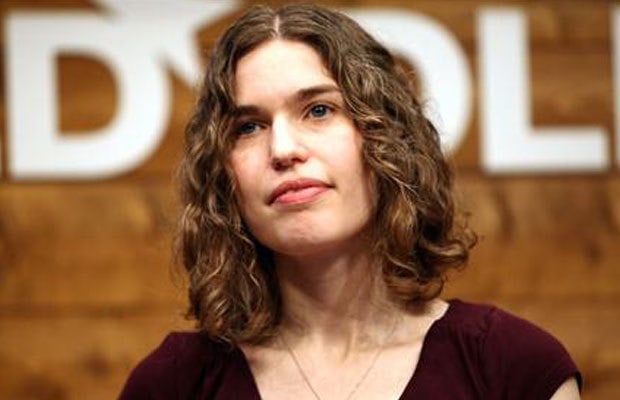
At the time bitly launched it seemed a little frothy, we already had TinyURL. Was this what the world needed: a URL shortener so when Web surfers shared their endless musings through social-media they could shorten the links to their content?
After all, TinyURL allowed you to paste in a very long web address then provided you with a short code that redirected to it.
In the years since its birth, bitly has more than proved its business model. People used to ask what is the value of a service that takes a long Web address and makes it shorter? The answer was several million dollars.
The main activity bitly added to life online was and is URL tracking: in other words, we get a number for how many people clicked each link and it also keeps a copy of each page.
Hilary Mason’s job, as Chief Scientist at bitly for four years, was to play with this information, looking at data about what people click on, trying to figure out what it says about human behaviour and communication.
A third of her time was spent looking for interesting events, trends or visualizations. The other two thirds she focused on translating models and equations into functional systems.
She discovered the rather arcane fact that iPad, and other tablet owners are mostly likely to use their computers most often before bed, and while people like to share breaking news and current events, they’re more likely to read inane gossip, watch sneezing pandas or play online games.
Inane maybe but this data has its adherents who are willing to pay a lot of money for information.
She has also received a few awards including the TechFellows Engineering Leadership award, she was profiled for Forbes magazine’s 40 under 40 Ones to Watch list and has been featured in numerous glossy magazines including Glamour, Fast Company and Scientific American.
A former member of Mayor Michael Bloomberg’s Technology and Innovation Advisory Council not surprisingly she features prominently as one of the most important people in the New York Tech Industry.
After graduate school, Hilary joined Johnson & Wales University in Rhode Island as an assistant professor, but continued to program in addition to teaching and working on research and built a program that crawled job boards to determine which skills employers value, which helped Johnson & Wales explore ways to improve its curriculum.
Hilary is the founder of Fast Forward Labs, a machine intelligence research company, and the Data Scientist in Residence at Accel. She co-founded of HackNY is a member of NYCResistor, a non-profit company which helps talented engineering students find their way into the start-up community of creative technologists in New York City.
Are you able to untangle the web of information for me here and tell me what the central role of a data scientist is? Can anyone who is able to understand a little math, has plenty of logic and communication skills able to become a data scientist? HM: A data scientist does three things; first, they can build mathematical models of a system from messy data. Second, they can code. And third, they can ask good questions and communicate the answers back to non-technical people.
I think of ‘data science’ as a flag that was planted at the intersection of several different disciplines that have not always existed in the same place. Statistics, computer science, domain expertise, and what I usually call ‘hacking,’ though I don’t mean the ‘evil’ kind of hacking. I mean the ability to take all those statistics and computer science, mash them together and actually make something work.
And I think that last one is by far the most important. If you are the kind of person who can say, “I have some cool data. I really am curious about some questions about that data. I’m going to figure this out.” Then yes, you can do it. RM: Is data science one of those techniques which has out there for some time just waiting to be applied? Is it a branch of dynamic programming? HM: Data science is not a technique, it is a profession. It deserves a new and distinct title because it requires multiple skills in the same professional that have never existed together before.
Think of the job of the data scientist is asking the right questions, so if I ask a question like ‘how many clicks did this link get?’ which is something we look at all the time, that’s not a data science question. It is an analytics question. If I ask a question like, ‘based on the previous history of links on this publisher’s site, can I predict how many people from France will read this in the next three hours?’ that is more of a data science question.
Modern data science also bridges disciplines in a unique way, bringing together academia, the startup community, and, to a limited but growing degree, the corporate world. RM: What was it that made you think that ‘Wow, this is something I really need to explore and get into more’? Was it just intuition that this was the career path for you? HM: I am a computer scientist and have always had a keen interest in both algorithms and databases. It became clear to me in the last decade that the most interesting algorithms were those that worked on real data. I am curious, and I like understanding things.
The chance to work on a system that allows me to learn things about human behaviour that have never been understood before, with hard and interesting engineering challenges, was too good to pass up. RM: I understand that one of the products you developed was a real-time search engine which crunches data from its users is then able to spit back out again to searchers. As well as its computational relationship can it also pull answers from a range of approved references, databases and documents? Can it also visualize data and say, predict how many clicks a certain document will receive in the future? HM: We’ve did very successful predictive models of how many clicks a link will ever get, but didn’t found a use for that in any of bitly’s products.
One of the great things of the new crop of data-exploration software is that the inflection point of value in the data chain moves toward the right-out of heavy-duty processing systems that are expensive, complicated and must be maintained by IT, and into lightweight solutions almost anyone can use. Thus, the “priesthood,” ivory-tower academics or deep programmers, didn’t need to be consulted about every data-related question going forward.
The tools we created restricted the kind of analysis you’re able to do immediately, but they opened up a capability for analysis to a much wider population.The amount of learning you need to do to be effective does decrease from a multi-year process to a multi-hour process. RM: When modern computing started 50 years ago, people thought they would be able to ask a computer any question, and have it compute the answer. But it didn’t work out that way. Are we nearer that goal with what you are developing for business and will it get better as the platform is developed and more data sets are added? HM: I think we’re still fairly fair away from true AI. That said, there are people building tools that answer structured questions very efficiently, and there are even more opportunities in this space. RM: How much do you think you can sit down and figure out how something should work, assuming it is not something you have built before? Do you need to start writing code to understand what the problem is? HM: Personally, I like to think through a problem on paper or a whiteboard first, and then to write code to try it out, generally in ipython, then possibly to go back to paper and do some math, and then to re-write code, this time more robustly.
Python is my current programming language of choice, though I am not averse to C. RM: In general do you think data science is the next phenomenon? Regardless of this, what is the great promise of this data-exploration software for corporates and other organisations? HM: Data science offers a better picture of the truth of the world around us, which means that people can make more robust decisions. I don’t think it’s magic, just a cheap capability that everyone now has access to. RM: I’ve read that you see a lot of self-education with data science so where someone has two of the three essential components for conducting a productive inquiry and is able to teach themselves the third on their own. Is there any essential skill needed to do this? Is there a commonality you can highlight? HM: The essential commonalities are curiosity (motivation) and resourcefulness (ability to do things). If you have those two qualities, you can learn almost anything. RM: You mentioned that data science will enable people not having to consult the ‘priesthood’ or the ivory-tower academics and deep programmers about every data-related question. You obviously feel that academic computer science, the tech industry and industrial programming fail to meet in the right place? HM: In academia, you work on problems that will get you published. In industry, you work on problems that will make you money. Some questions are better explored in one of these contexts, and some
Startups are ahead of many large enterprises on this front, because many large corporations have heavy technology legacies and multiple layers of “priesthood” to negotiate.
The gaps between these communities’ take on data science can be characterized by their degree of openness. On the one hand, academia is known for its propensity to share information and conduct peer reviews. Startups are commercial in nature, but are just as likely to share findings with the open-source world as they are to consume open-source solutions.
Corporations, unsurprisingly, tend to be the most locked-down and opaque about their use of tools and their findings. RM: There have been a great number of tech start-ups in New York over the last few years do you think the NYC will soon outrun Silicon Valley for innovation? HM: The NYC versus Silicon Valley question is a false dichotomy. New York is a fantastic place to work in tech startups, and our culture is different than the valley. I think having more than one city with a strong tech culture will just lead to more creative projects in general.
Subscribe for more articles
Fortnightly newsletters help sharpen your skills and keep you ahead, with articles, ebooks and opinion to keep you informed.
Rate this article

Richard Morris
Richard Morris is a journalist, author and public relations/public affairs consultant. He has written for a number of UK and US newspapers and magazines and has offered strategic advice to numerous tech companies including Digital Island, Sony and several ISPs. He now specialises in social enterprise and is, among other things, a member of the Big Issue Invest advisory board. Big Issue Invest is the leading provider to high-performing social enterprises & has a strong brand name based on its parent company The Big Issue, described by McKinsey & Co as the most well known and trusted social brand in the UK.
Follow Richard Morris via
View all articles by Richard Morris
Load comments
Related articles
Martin odersky: geek of the week.
- Geek of the Week
- Skip to main content
- My Subscription Renewal
WHERE IT HAPPENS
Join Music Director Esa-Pekka Salonen and the San Francisco Symphony for a vibrant new season!
COMPOSE YOUR OWN SERIES
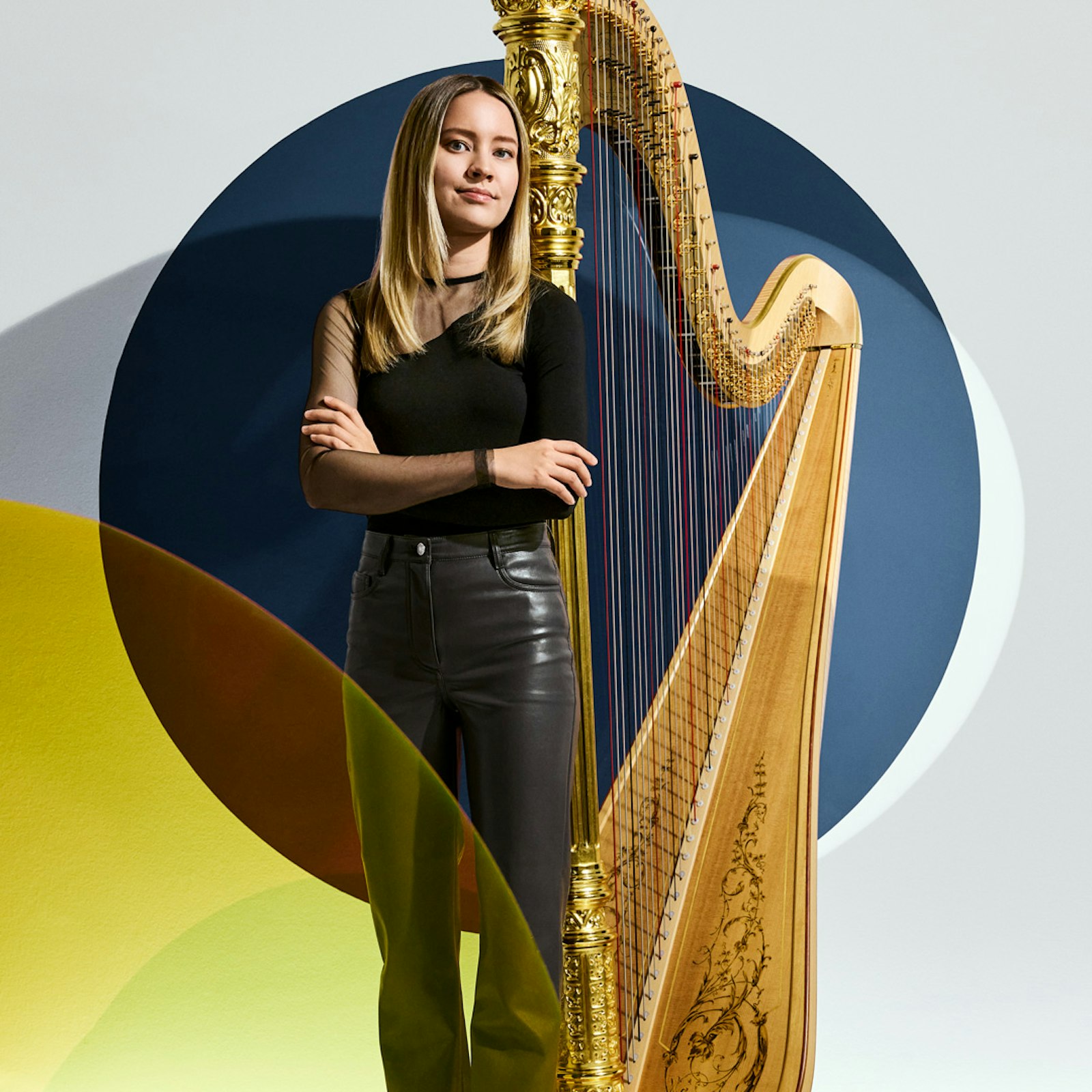
Design your own concert package from our complete lineup or be inspired by the suggested groupings below.
CHOOSE YOUR CONCERTS
FIXED PACKAGES
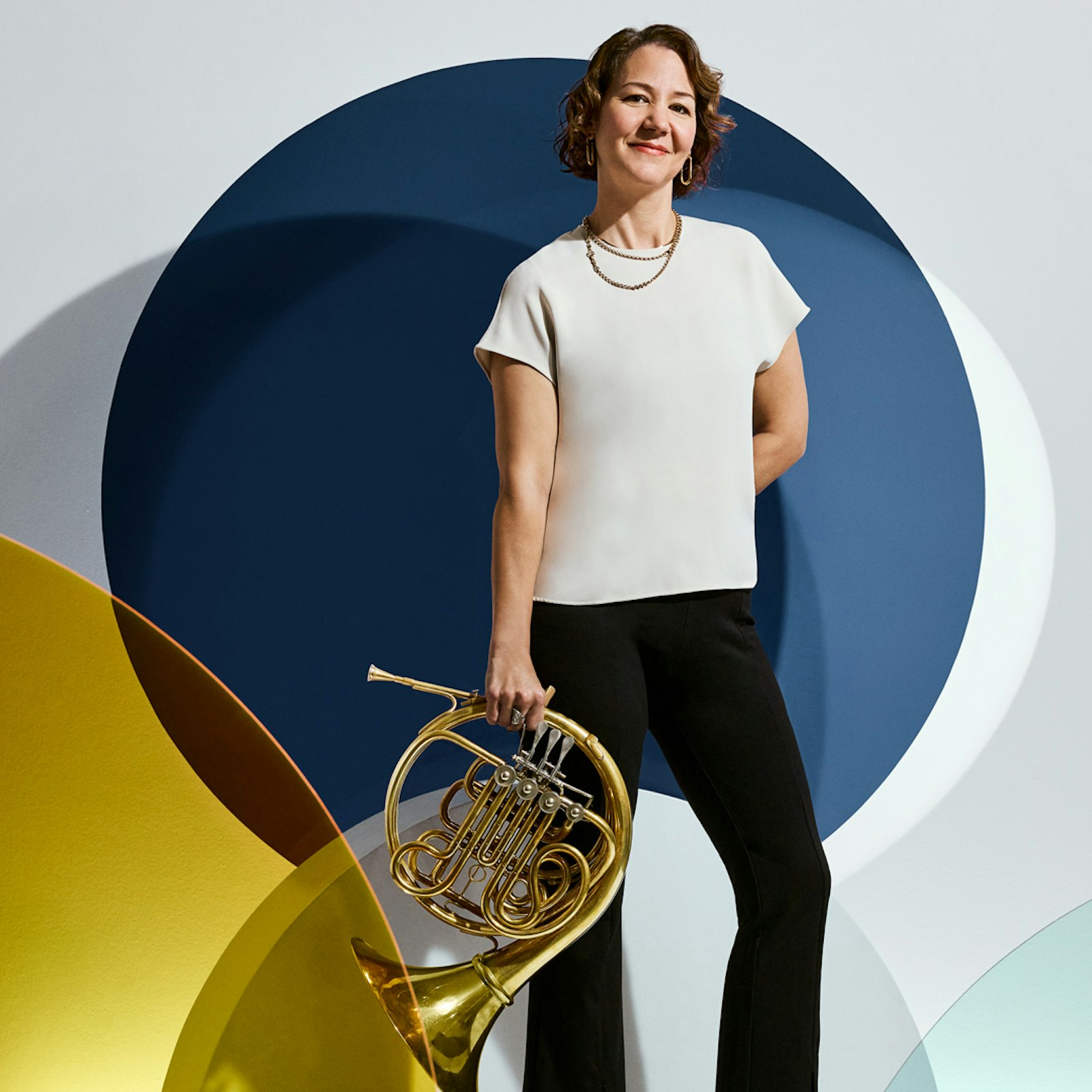
Select from a wide array of curated ticket packages designed around day of the week, concert experience, musical taste, and more.
RECEIVE MORE OF WHAT YOU LOVE*
Purchase four or more concerts by April 19 and get free SF Symphony Orchestral Series concerts.
SUBSCRIBER BENEFITS
ESA-PEKKA SALONEN
A little Stravinsky magic. Works so new the ink is barely dry. Ear-opening journeys. Experience the musical world of Esa-Pekka Salonen in his final season as San Francisco Symphony Music Director.
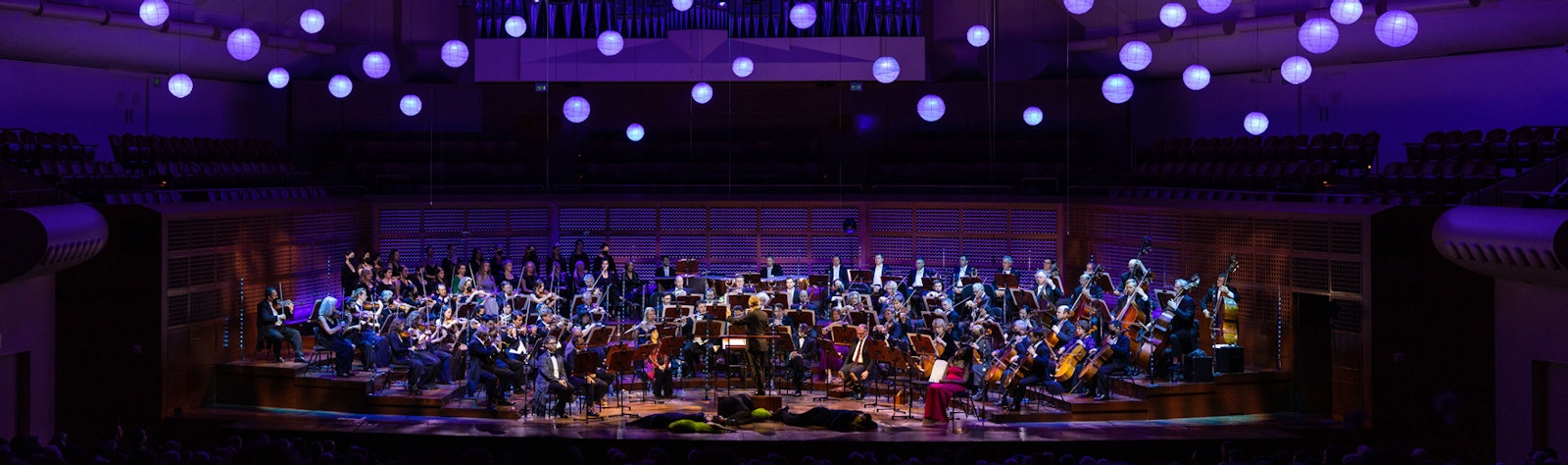
September 27–28
SALONEN CONDUCTS NICO MUHLY
October 18–20
SALONEN CONDUCTS BEETHOVEN’S PASTORAL
February 13–16
ESA-PEKKA SALONEN & YUJA WANG
SALONEN CONDUCTS SIBELIUS 7
BUCKET-LIST BRILLIANCE
The world’s most electrifying artists and ensembles converge at Davies Symphony Hall.
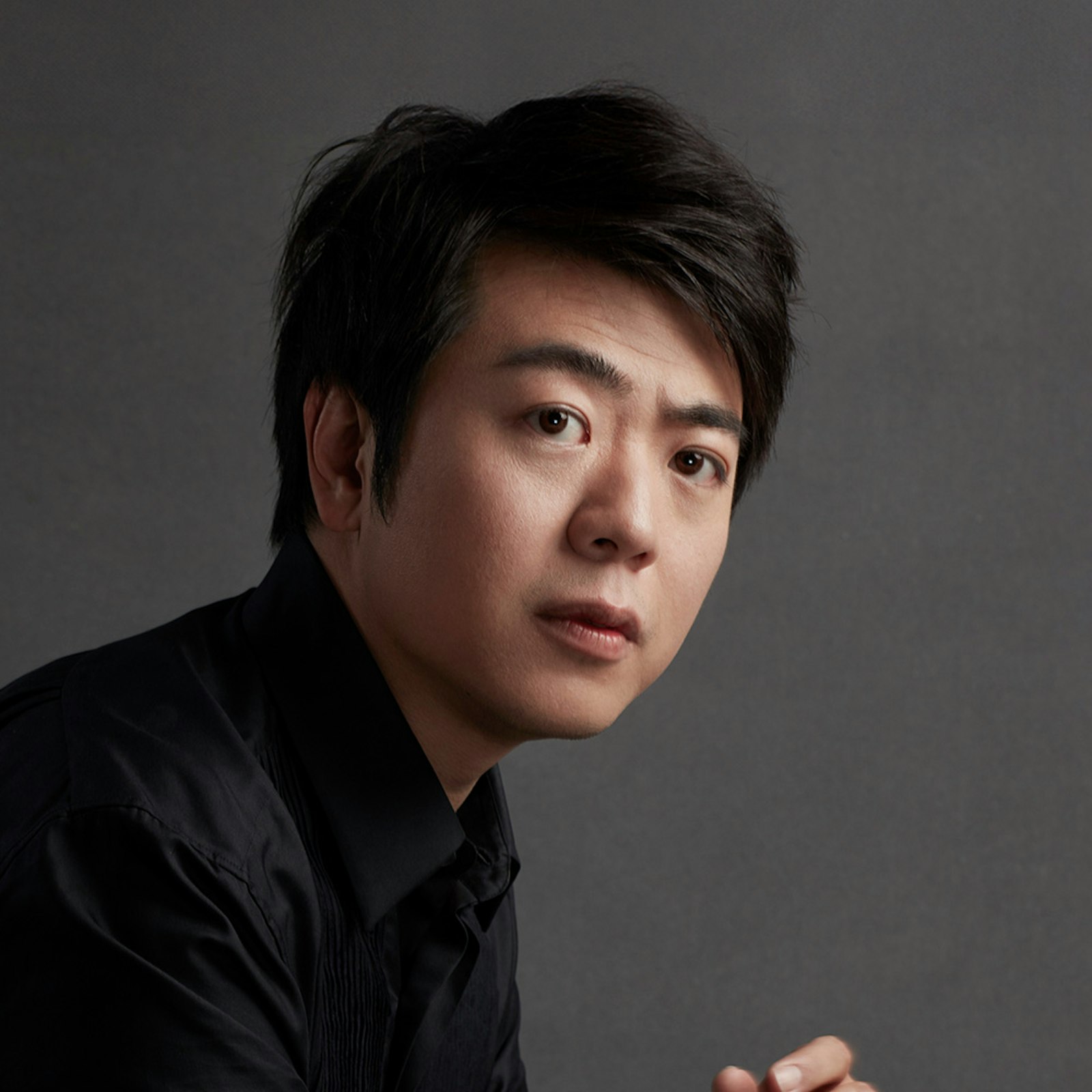
September 25
OPENING GALA WITH LANG LANG
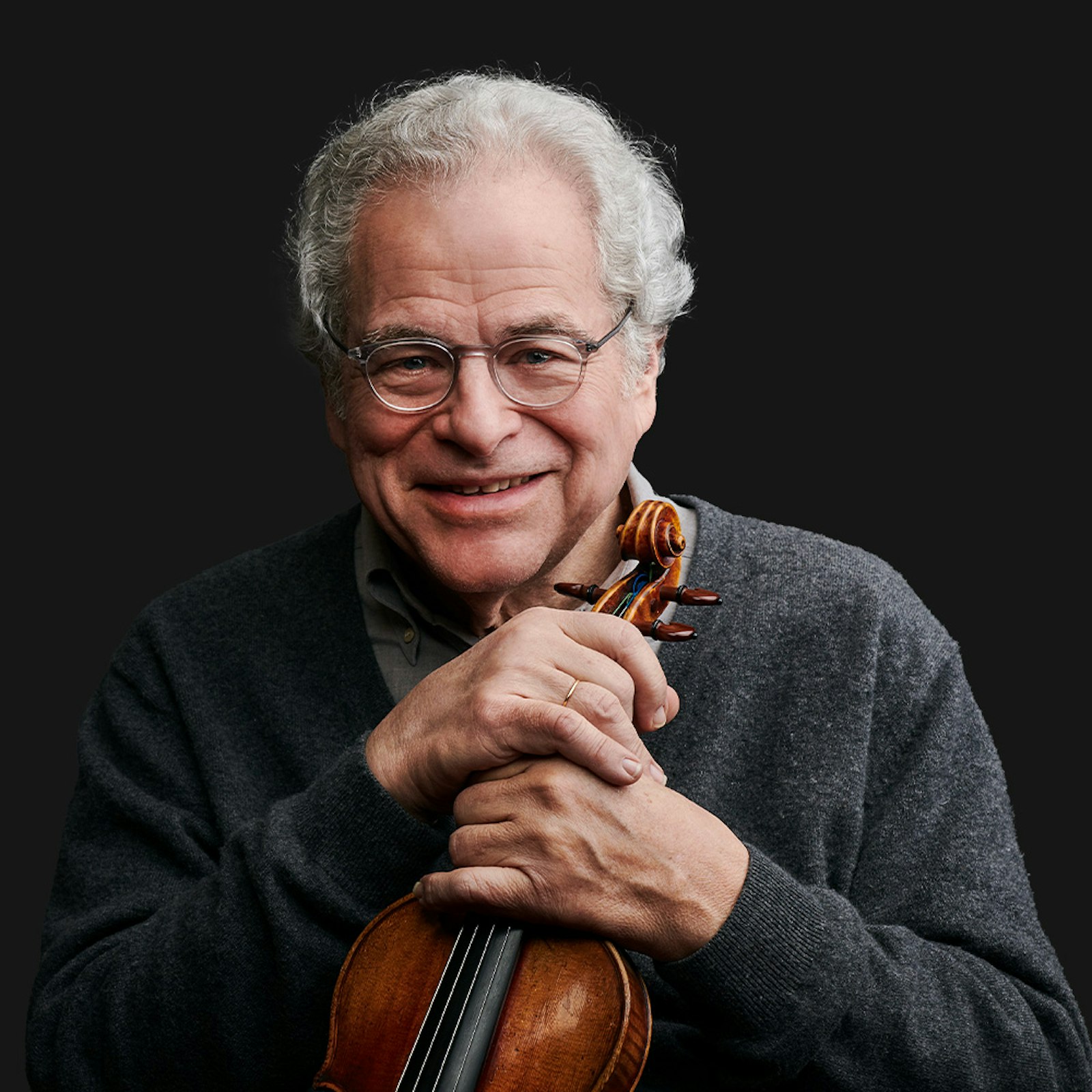
November 10
ITZHAK PERLMAN & FRIENDS
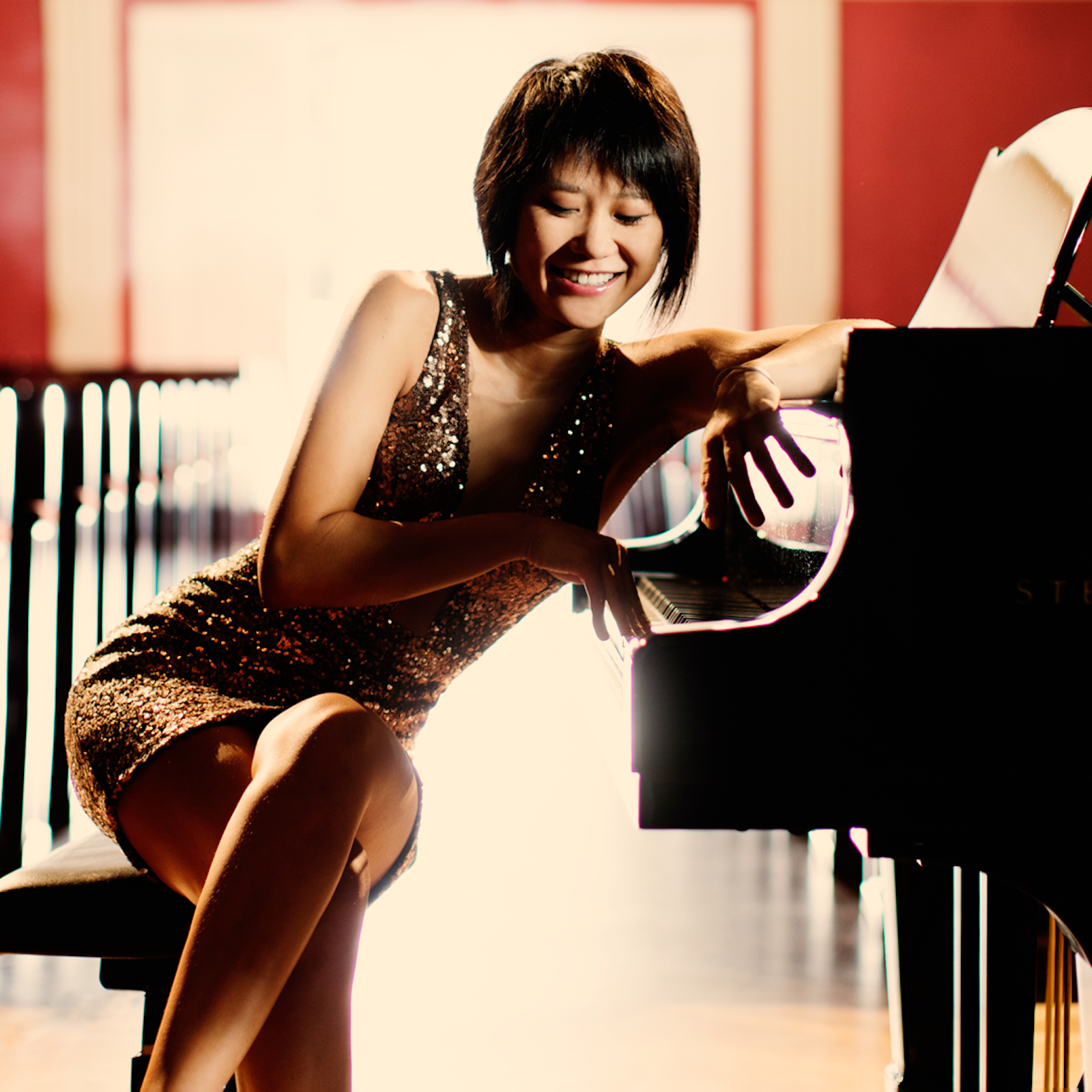
SHEKU & ISATA KANNEH-MASON DUO RECITAL
BIG SCREEN, BIG SCORES
Films and music with live orchestra.
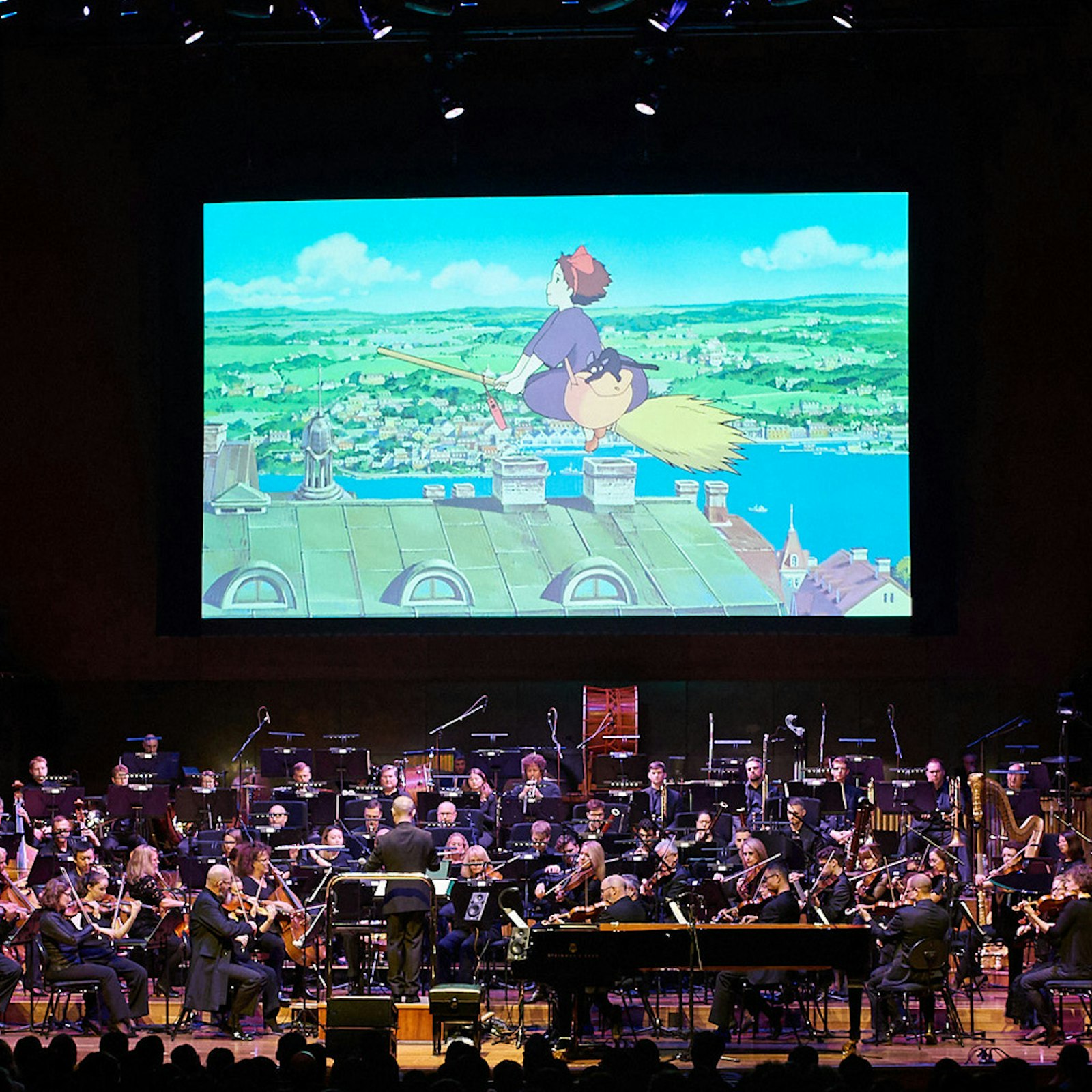
September 5–8
MUSIC FROM THE STUDIO GHIBLI FILMS OF HAYAO MIYAZAKI
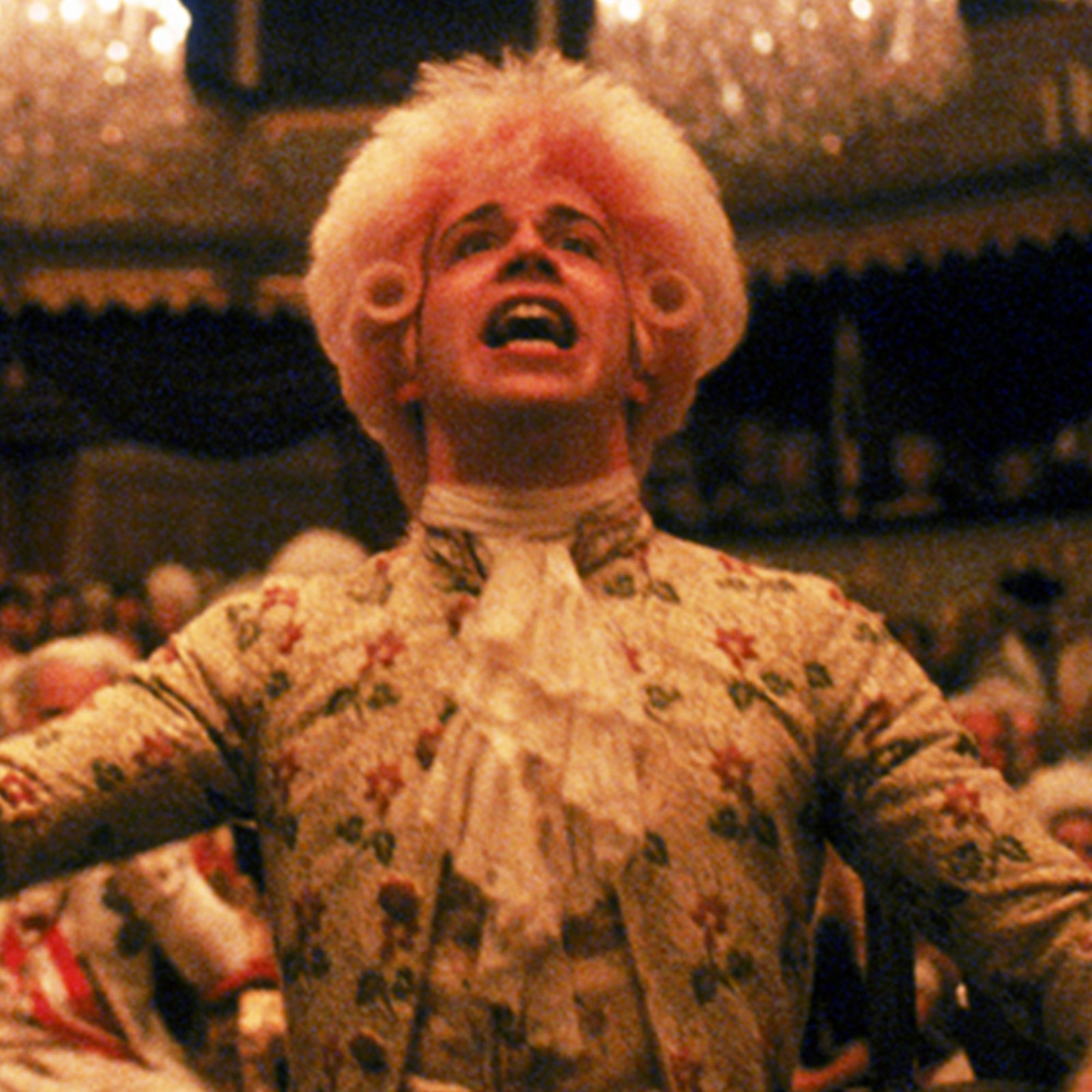
November 29–30
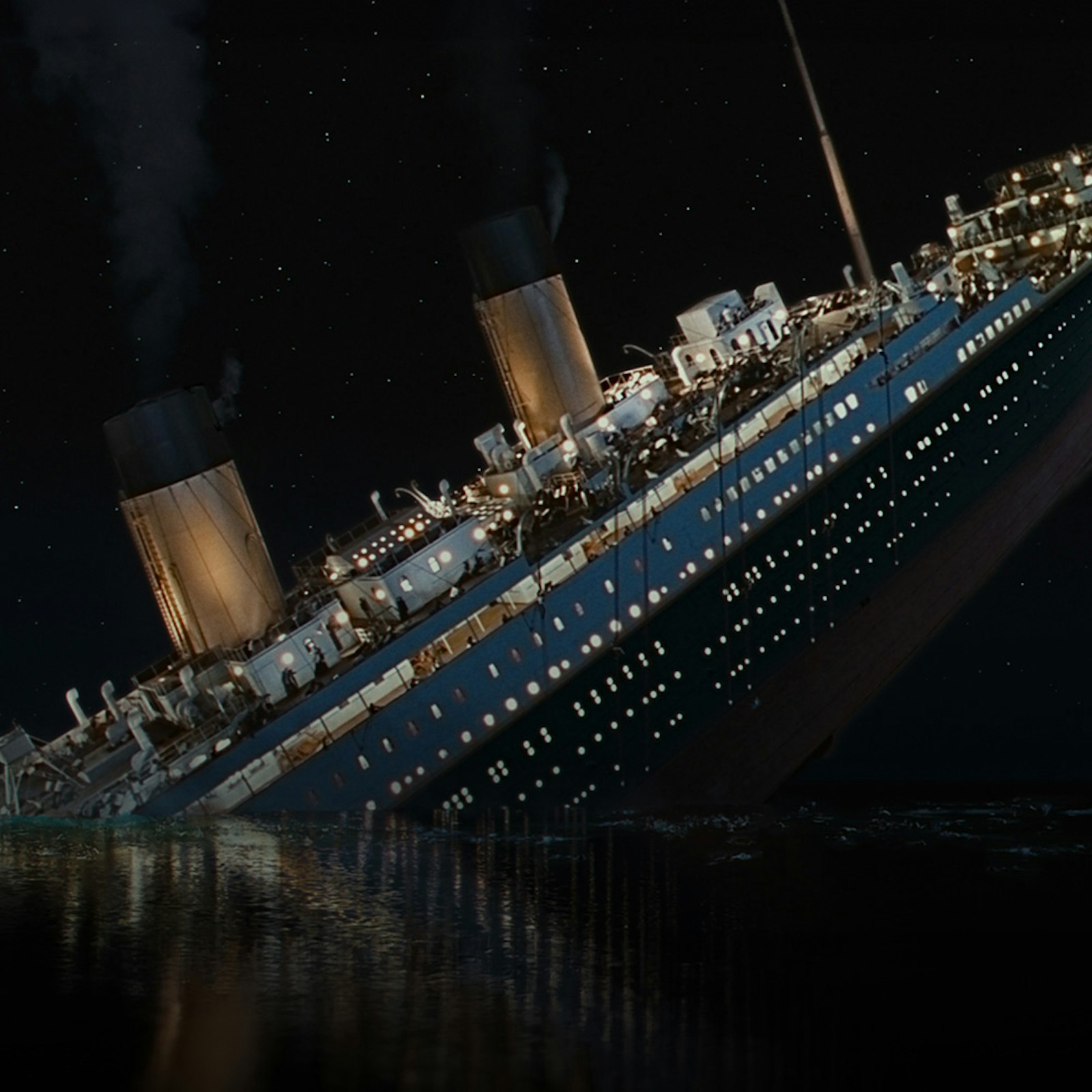
THE LORD OF THE RINGS: THE TWO TOWERS
FAVORITES IN FOCUS
Your ultimate playlist awaits in an enchanting assortment of symphonic essentials.
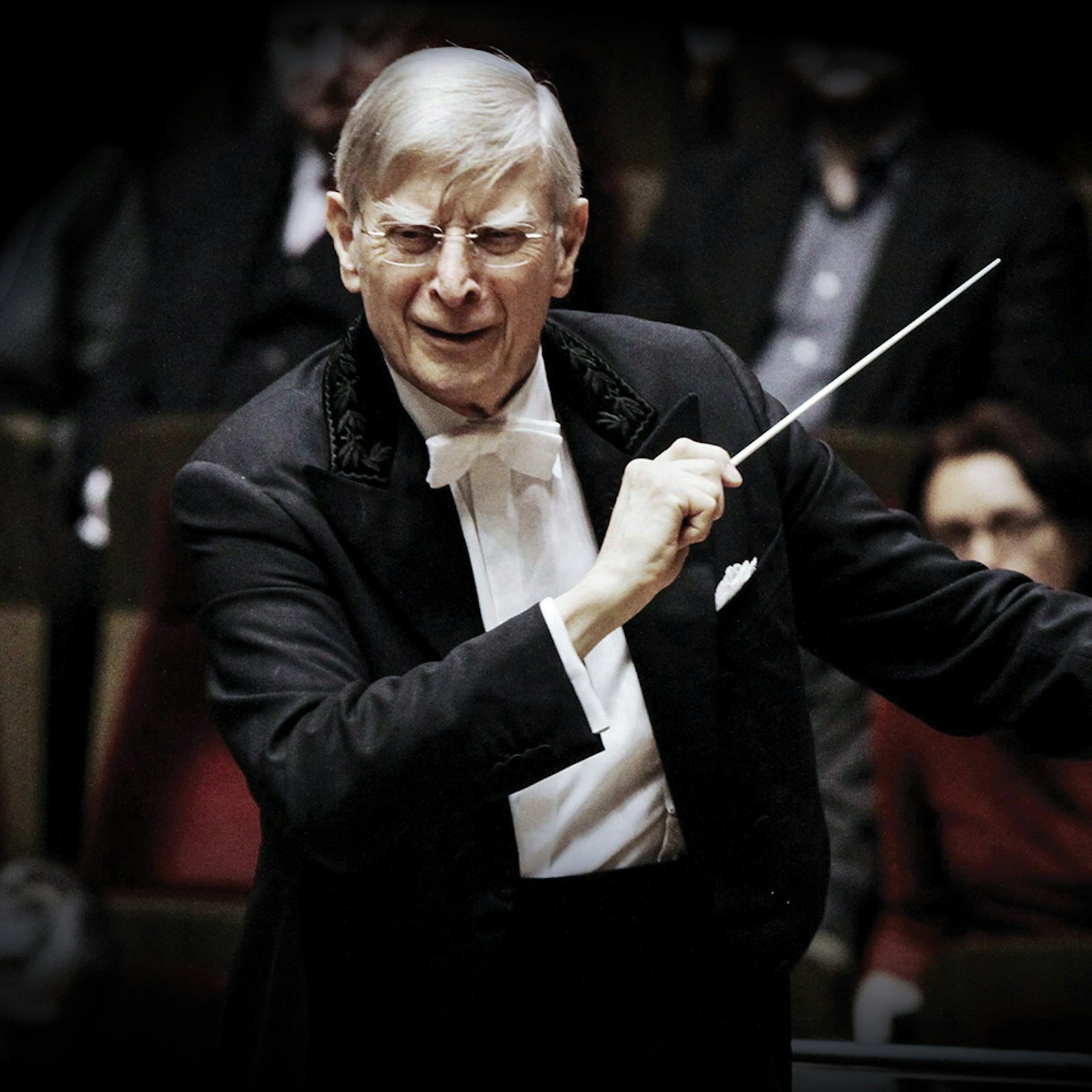
January 30–February 1
BLOMSTEDT CONDUCTS SCHUBERT & BRAHMS
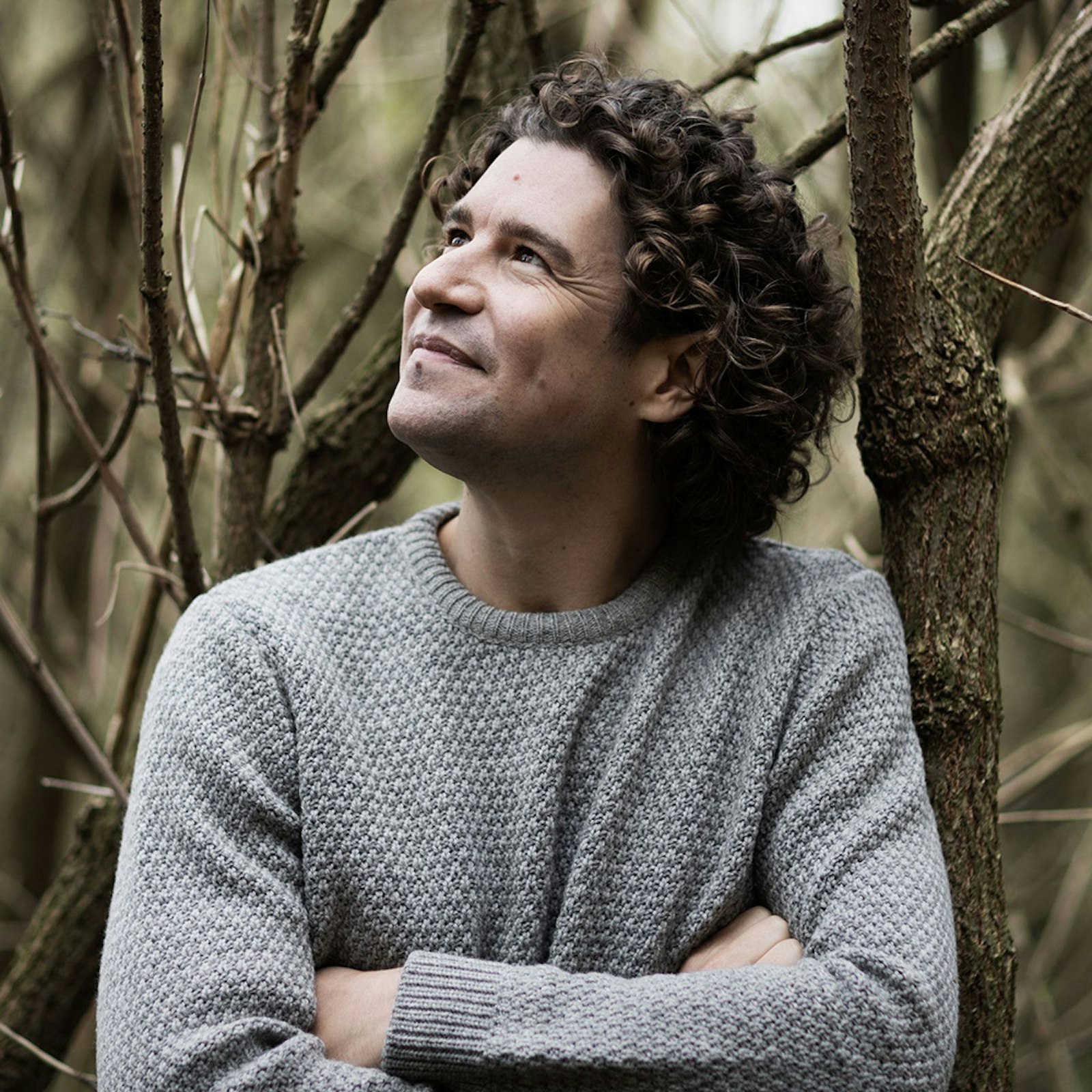
February 28–March 2
TICCIATI CONDUCTS RACHMANINOFF 2
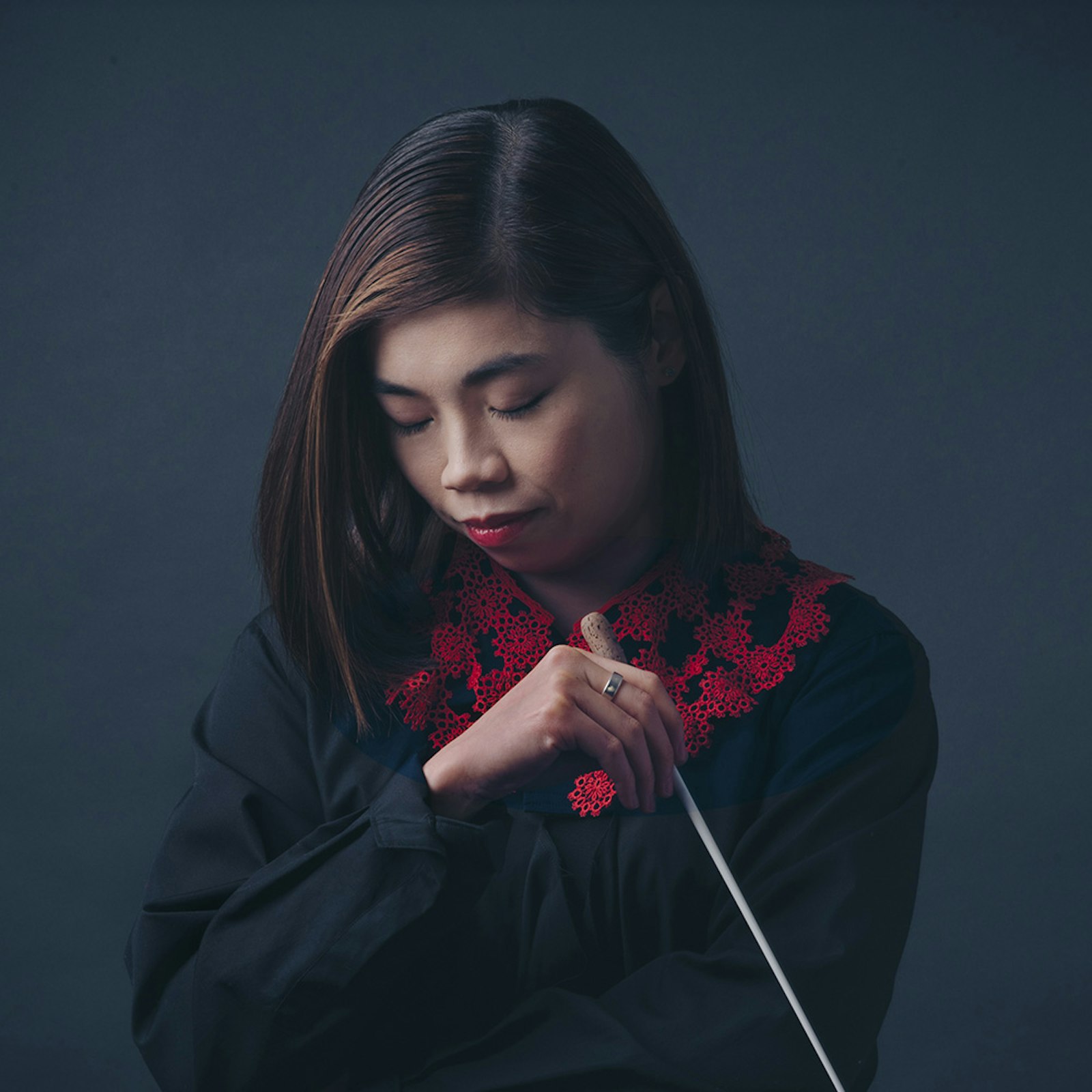
March 13–15
CHAN CONDUCTS ALL-TCHAIKOVSKY
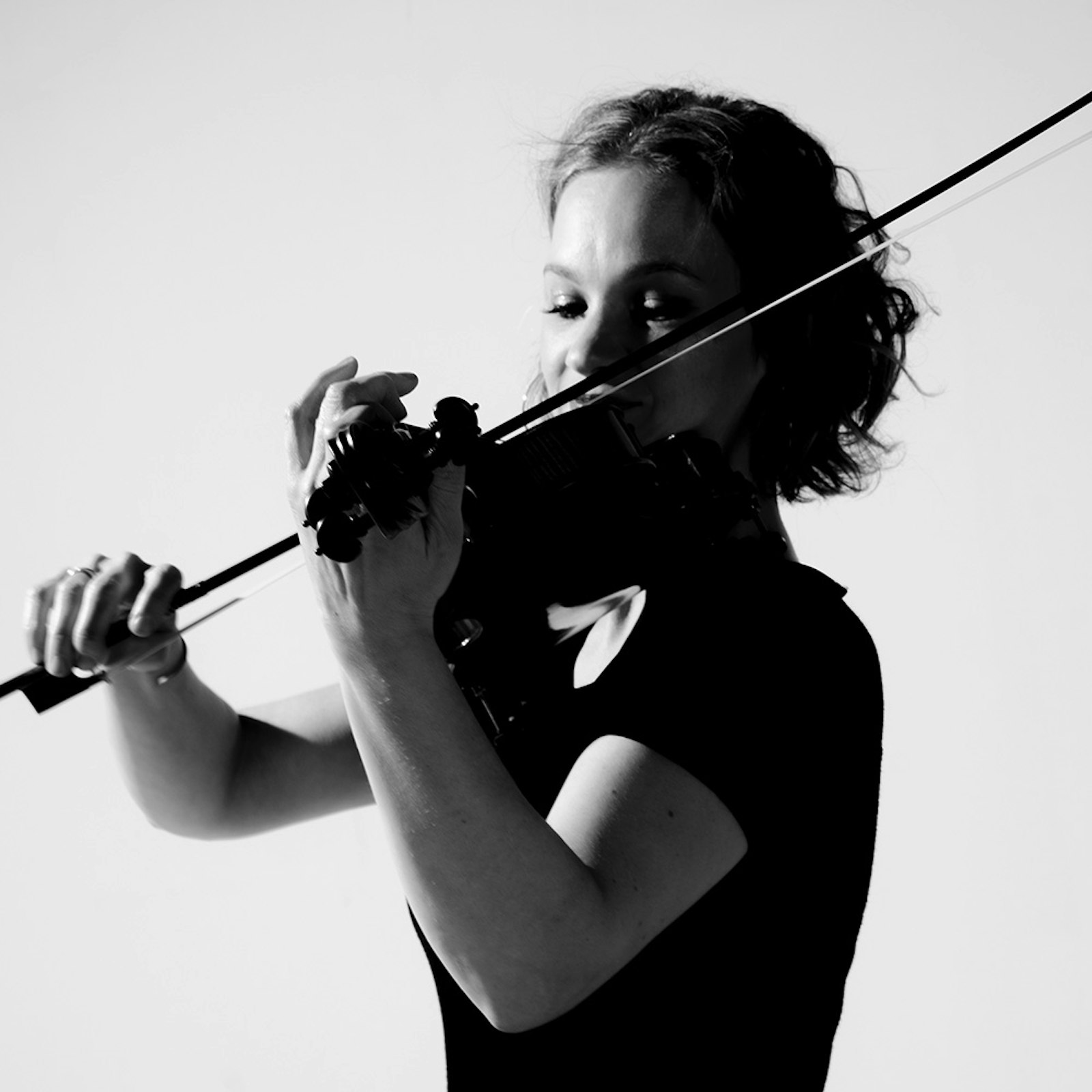
May 29–June 1
ESA-PEKKA SALONEN & HILARY HAHN
FIXED PACKAGES: ORCHESTRAL SERIES
Secure the best seats at the best price by purchasing your season tickets today!
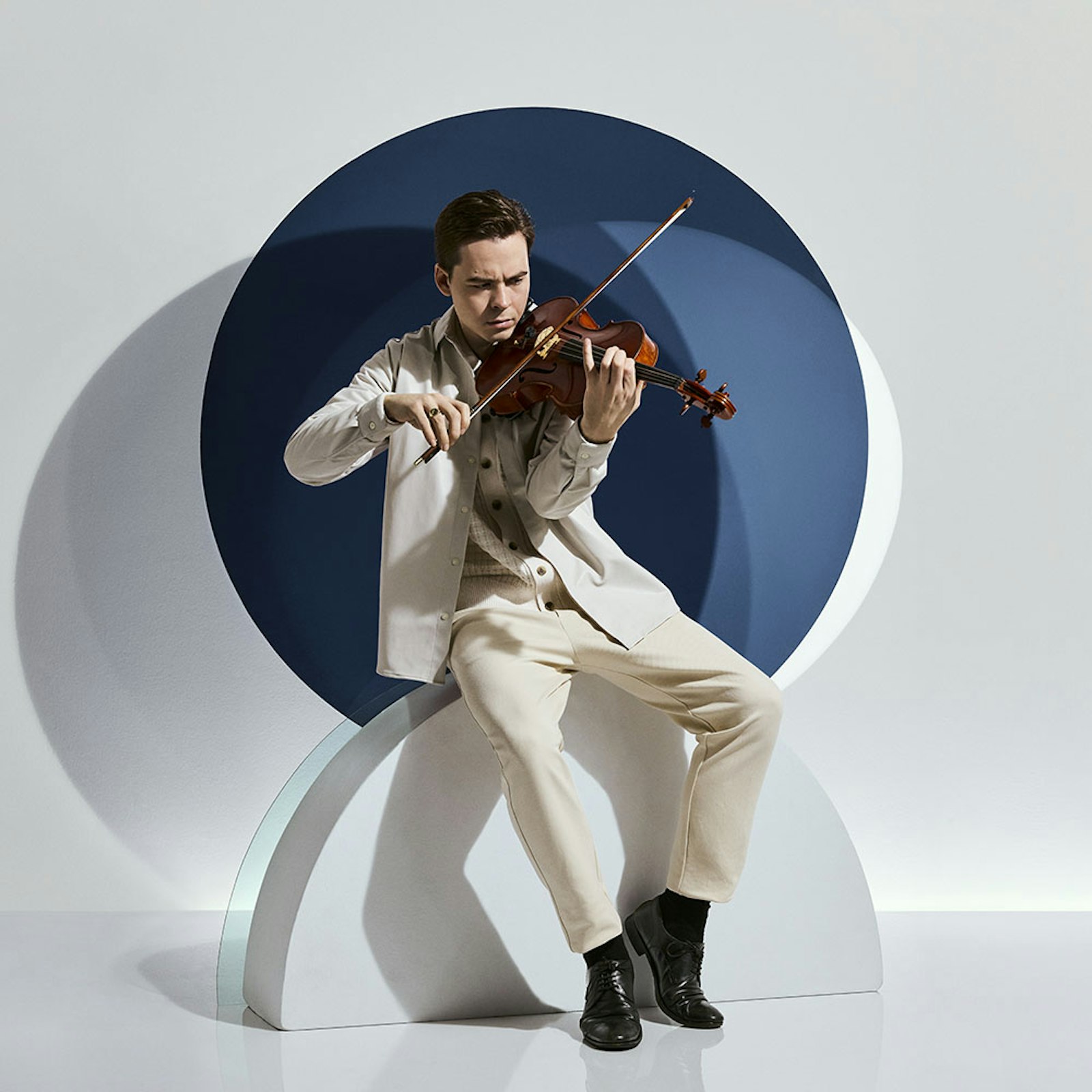
THURSDAY AND MATINEE SERIES
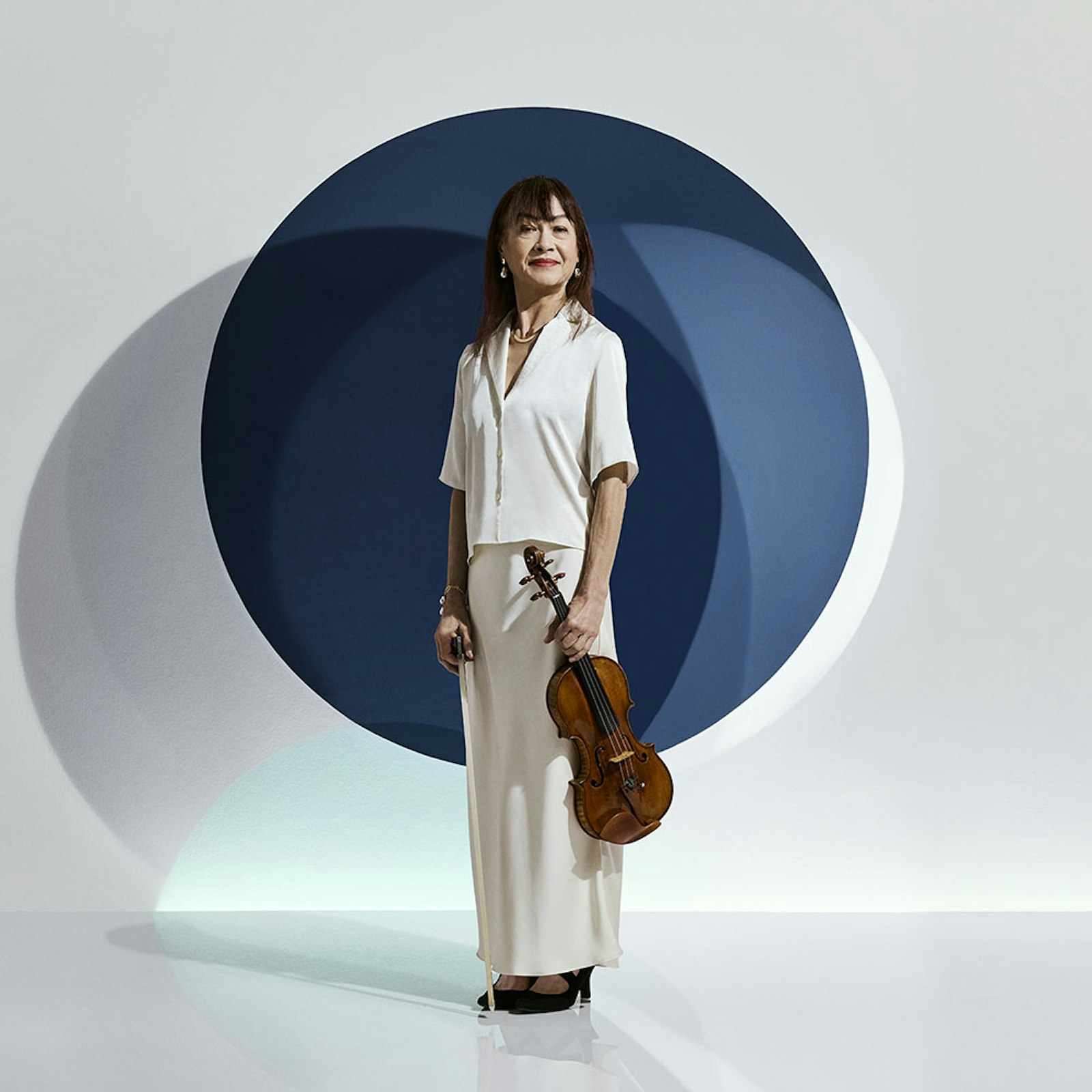
FRIDAY SERIES
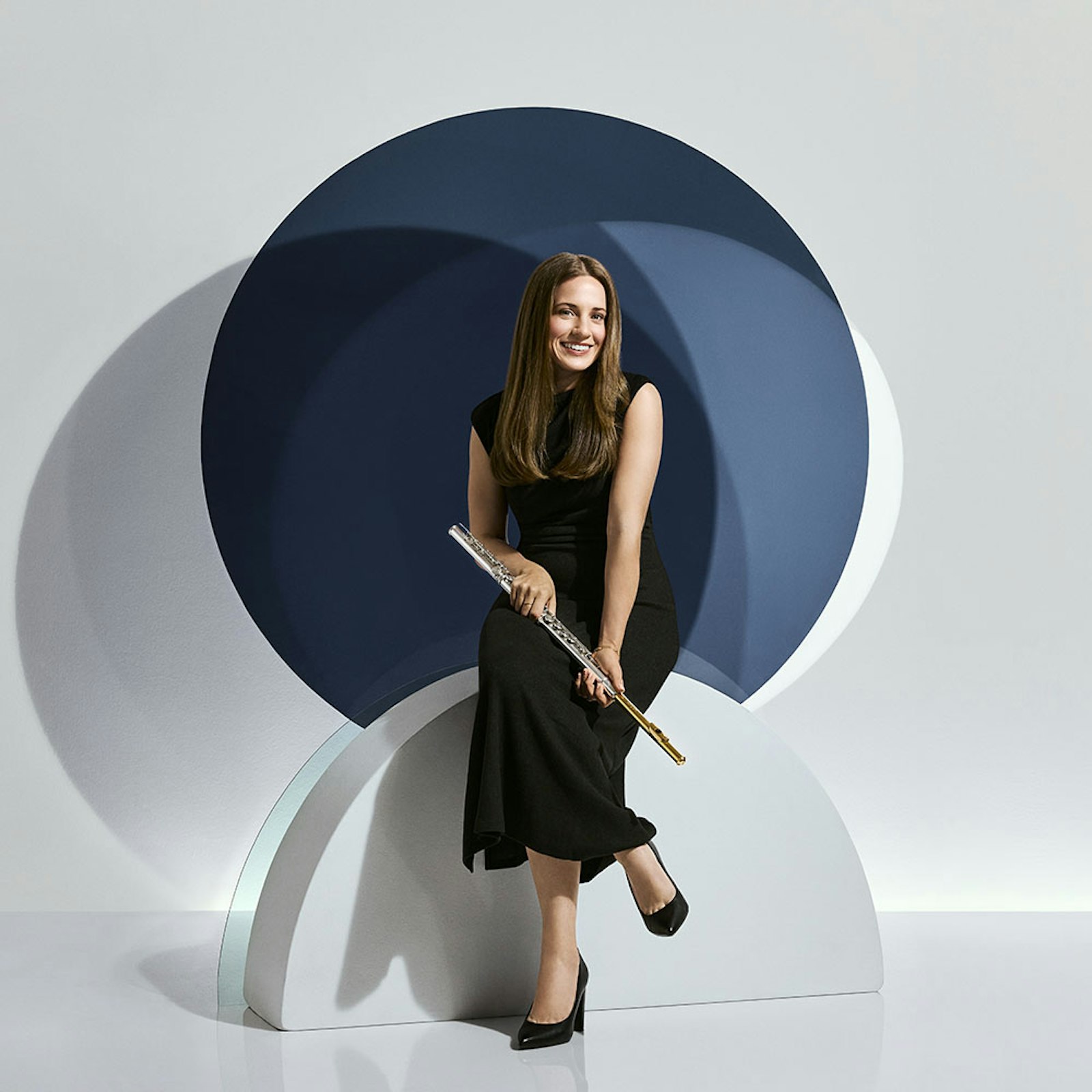
SATURDAY SERIES
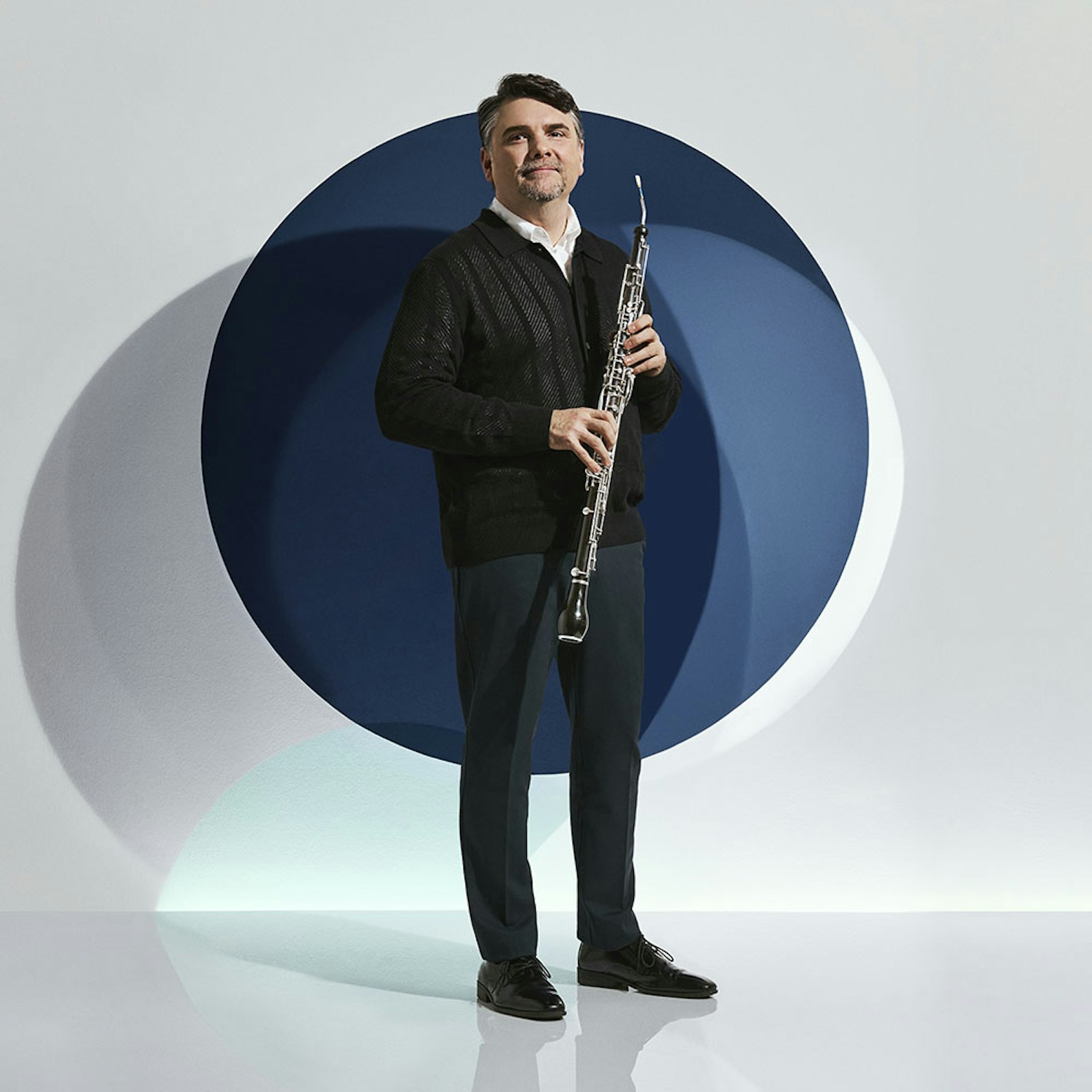
SUNDAY MATINEE SERIES
BUY ORCHESTRAL SERIES
ADDITIONAL FIXED SERIES
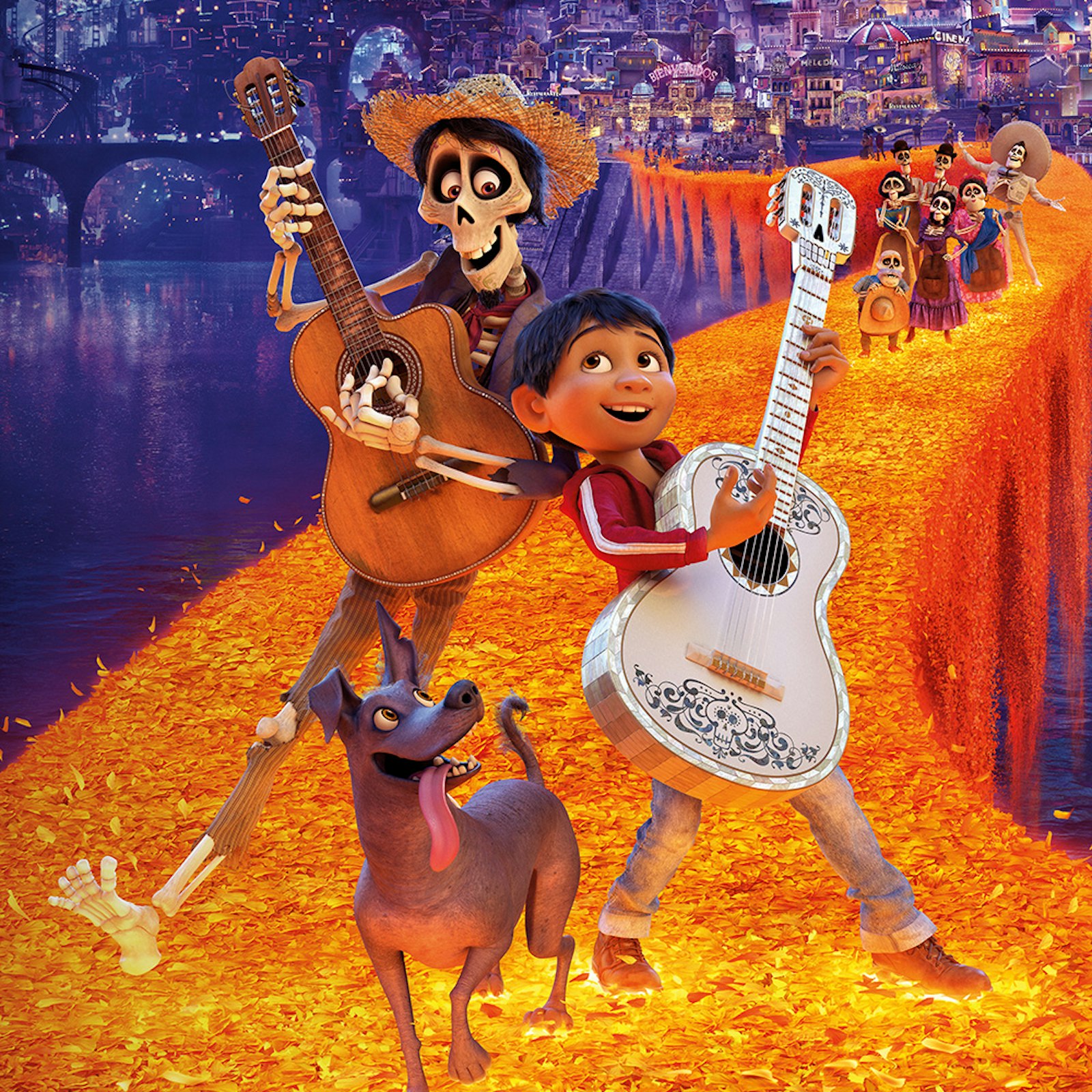
FILM SERIES
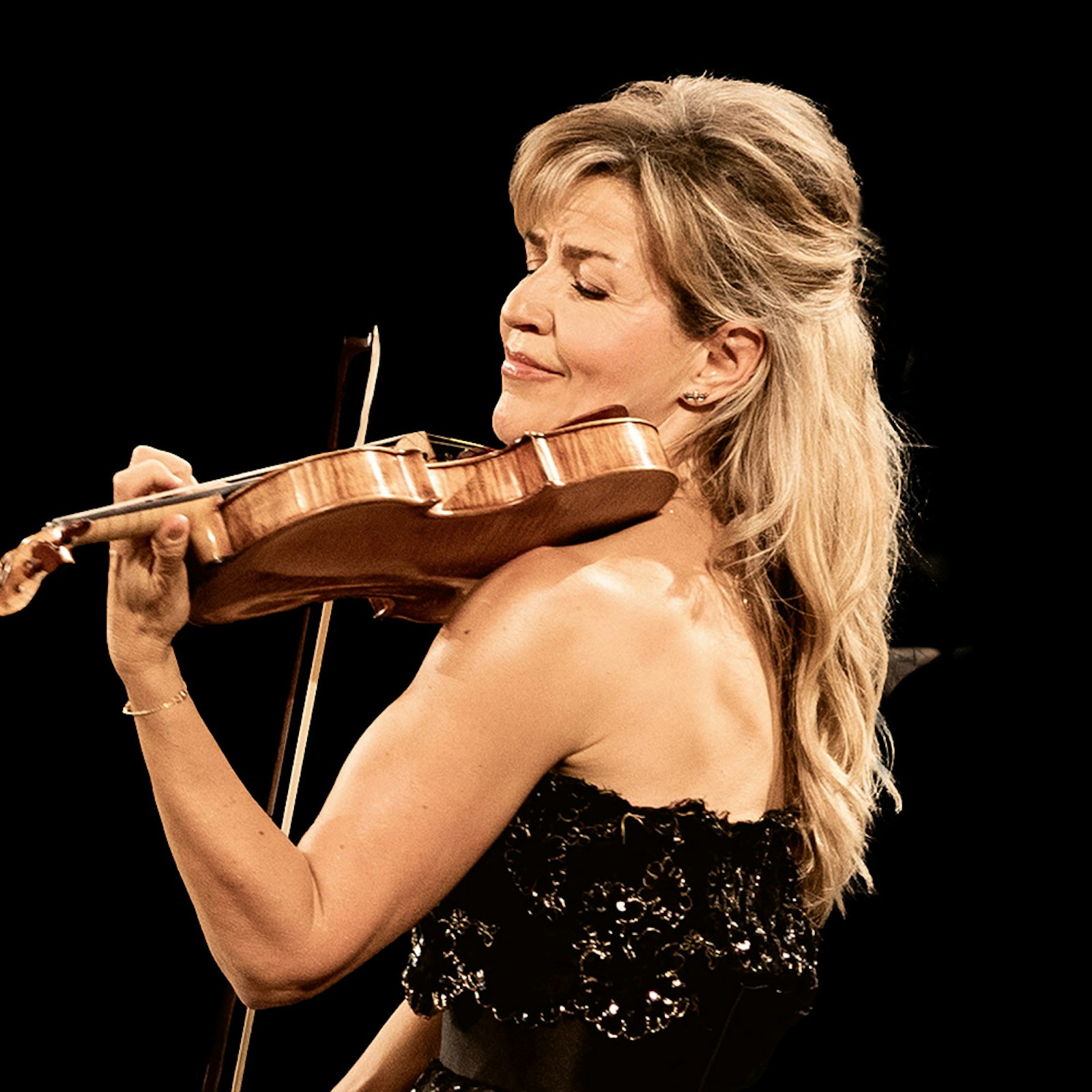
GREAT PERFORMERS SERIES
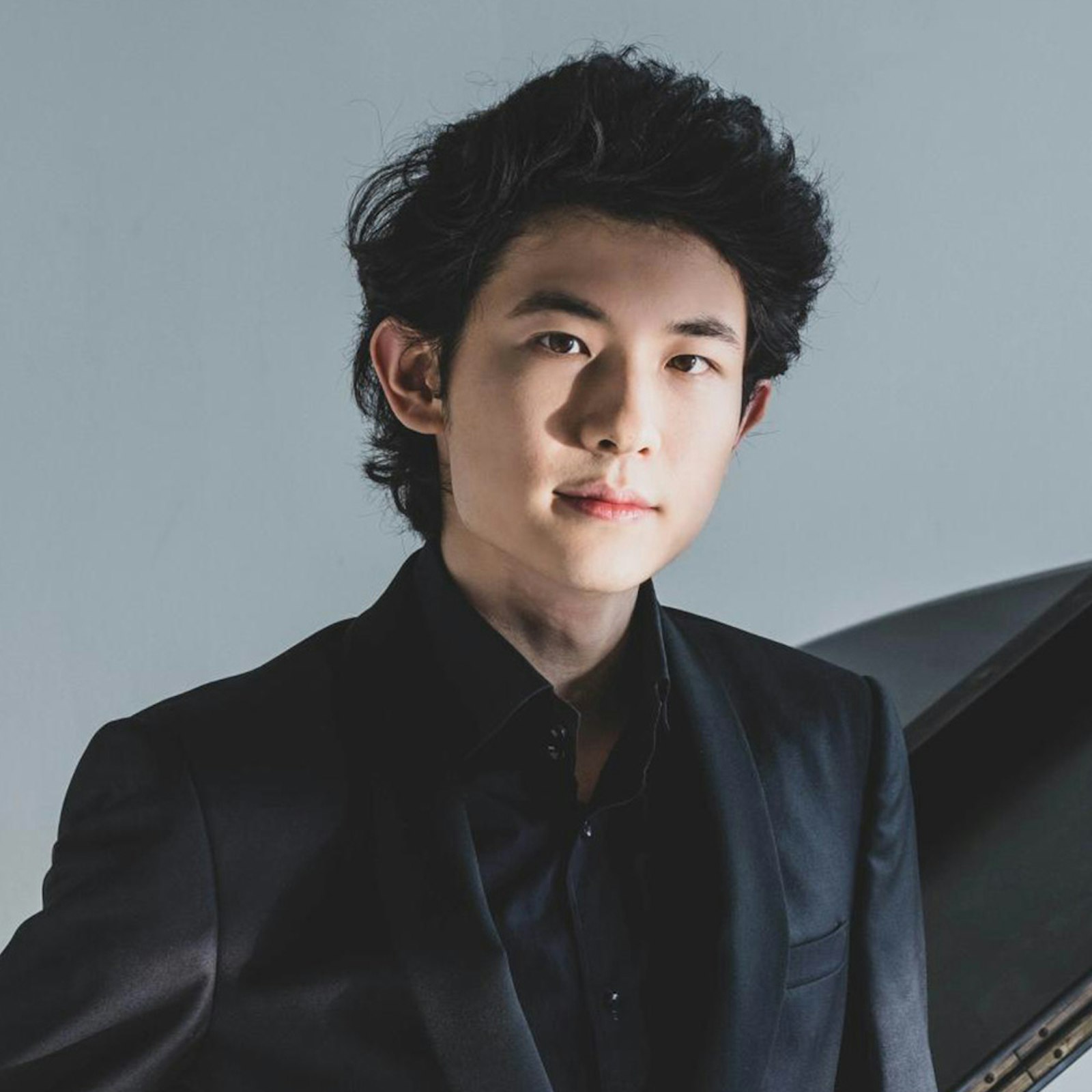
SHENSON SPOTLIGHT SERIES
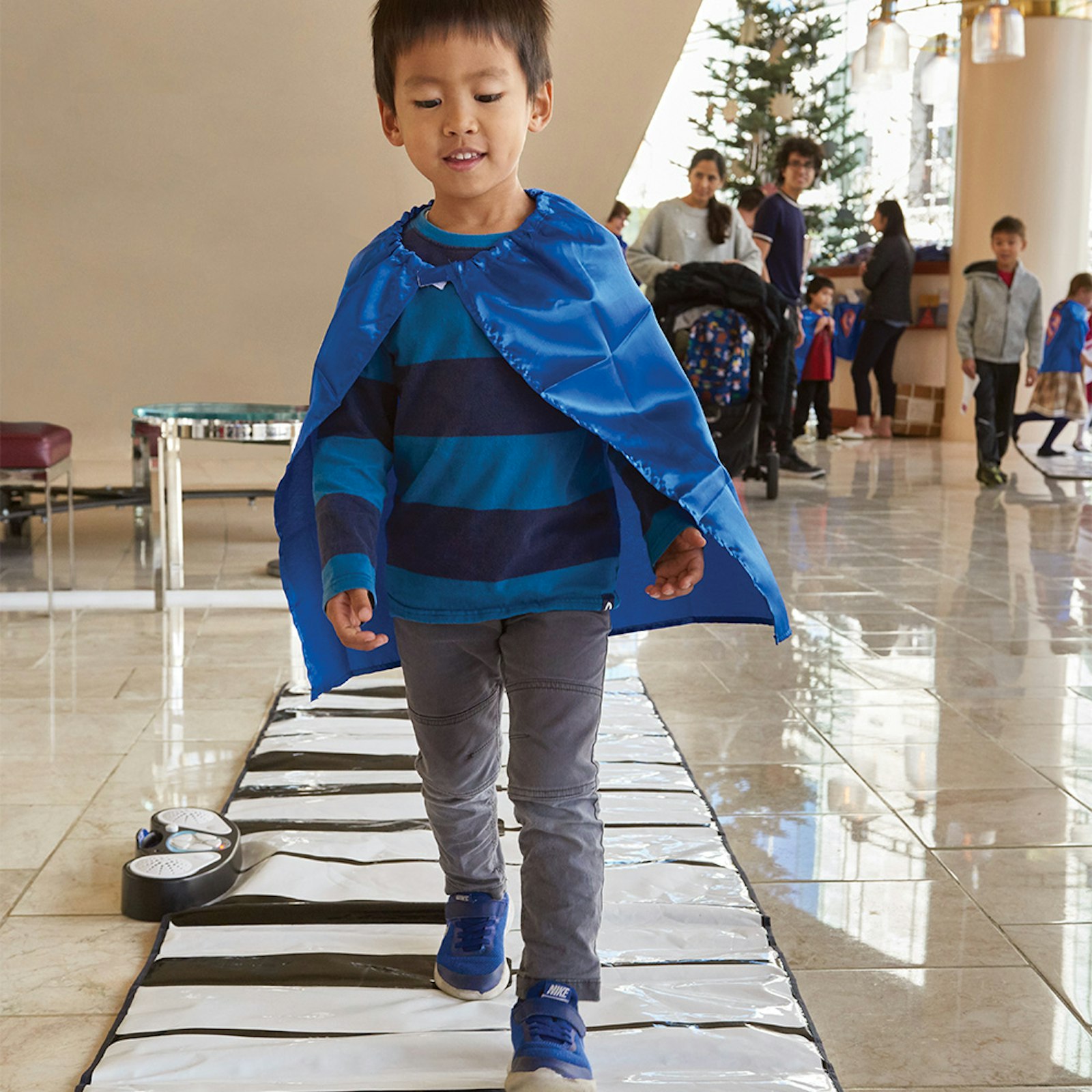
MUSIC FOR FAMILIES
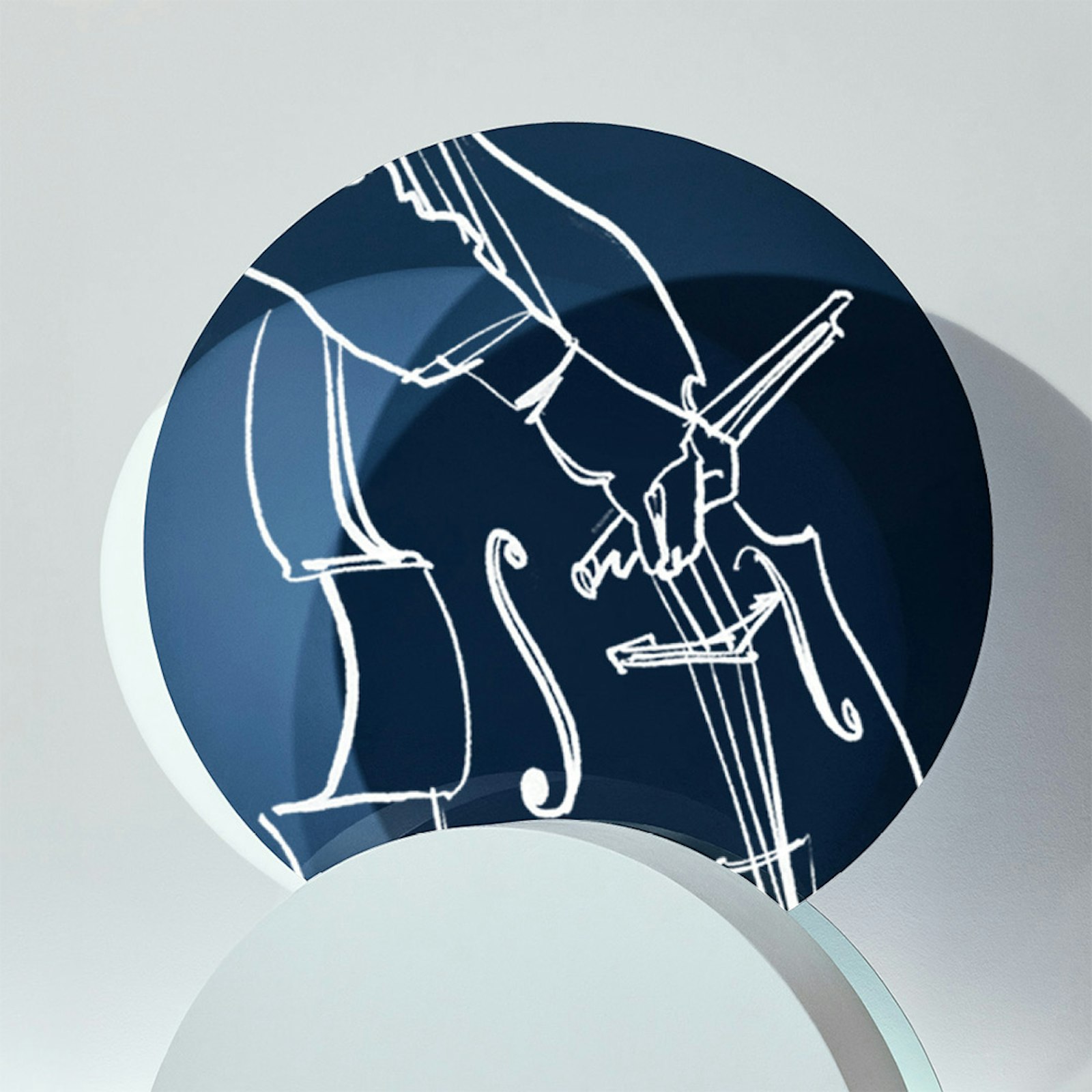
CHAMBER SERIES
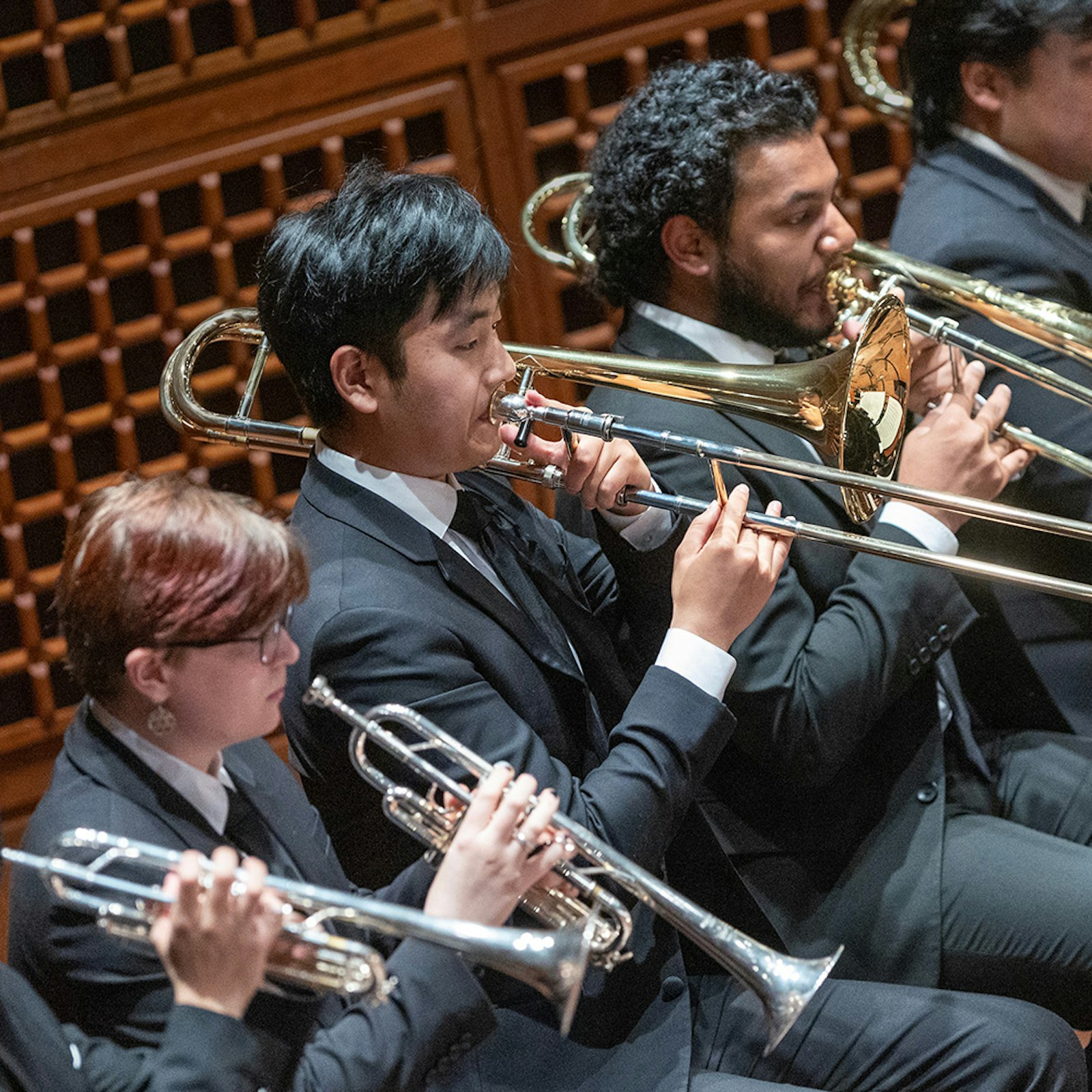
YOUTH ORCHESTRA SERIES
SUBSCRIBE TODAY
*Promotional offer applies to all subscribers who purchase a minimum of four full-price subscription concerts. Add-ons and special concerts are not applicable towards minimum purchase. Offer applies to full-price subscriptions and cannot be combined with any other discounts. The following SF Symphony Orchestral Series concerts are excluded from this offer: 2/13–16, 5/29–30 & 6/1. Subject to availability. Promotion expires 4/19/2024. After your subscription has been purchased, the San Francisco Symphony will email you to select your free concert tickets.
Never miss a beat!
Please wait...

IMAGES
VIDEO
COMMENTS
Hilary Mason is an American entrepreneur and data scientist. She is the co-founder of the startup Fast Forward Labs. Early life and education. Mason was born in New York City, and graduated from Grinnell College with a B.A. in computer science in 2000, and attended Brown University. Career. In 2010 ...
I <3 data and cheeseburgers. · Experience: Hidden Door · Location: New York, New York, United States · 500+ connections on LinkedIn. View Hilary Mason's profile on LinkedIn, a professional ...
About. Hi, I'm Hilary. Simply: I make beautiful things with data. I'm the Founder of Fast Forward Labs, a machine intelligence research company, and the Data Scientist in Residence at Accel. Previously, I was the Chief Scientist at bitly. I also co-founded of HackNY, co-host DataGotham, and am a member of NYCResistor. More: I believe ...
Hilary Mason. Hilary Mason is Founder and CEO of Fast Forward Labs, a machine intelligence research company, where she leads a team of applied researchers advising Fortune 500 executives, governments, and startups. She's also the Data Scientist in Residence at Accel Partners, a silicon valley venture capital firm. Previously, Hilary was Chief ...
Early life and education. Mason was born in New York City, and graduated from Grinnell College with a B.A. in computer science in 2000, and attended Brown University.. Career. Mason was the chief data scientist at bitly for four years. She is the founder and CEO of Fast Forward Labs and is a consultant data scientist at Accel, evaluating and advising on data strategies regarding technologies ...
I like to tinker. I'm the Founder & CEO of Fast Forward Labs, a machine intelligence research company, and the Data Scientist in Residence at Accel Partners. I co-founded HackNY, and I'm a member of NYC Resistor. More about Hilary….
Mason divides data science into two halves. The one half is analytics, which she simplifies as "counting things.". The other half is the invention of new techniques that can draw insights from ...
Data gives people superpowers. Behind her Clark Kentian glasses, Hilary Mason is something of a superhero by that logic. A former chief scientist at Bitly and now data scientist-in-residence at Accel Partners and founder of Fast Forward Labs, she has long been interested in data science and is perhaps more aptly described as a data hipster: She was into the big data movement before it was so ...
Hilary Mason: I've had a series of those moments at different times in my career but the set of problems and places where the technology can actually be impactful has radically expanded over the past 10 years. ML techniques are not new; people have been using them in financial services and for really high-value problems for a very long time ...
Hilary Mason, chief scientist at Bitly, makes meaning out of massive data sets. Or, as she describes it, she "pushes potential forward."
Hilary Mason, CEO of Hidden Door and data scientist in residence at Accel Partners, talks with World of DaaS host Auren Hoffman. Hilary previously co-founded...
Hilary Mason is an American entrepreneur and data scientist. She is the co-founder of the startup Fast Forward Labs. This biographical article is written like a résumé. (January 2023) Quick Facts Born, Nationality ... Hilary Mason. Hilary Mason, 2015. Born. New York City.
Hilary Mason, Co-Founder and CEO of Hidden Door, joins Jon Krohn for a live discussion that explores narrative A.I., emerging ML techniques and how her OSEMN data science process developed. ... but still many people and maybe people who work in specific industries like in education or in public sectors, still think machine learning is a scary ...
Hilary Mason, co-founder of Hidden Door and data scientist in residence at Accel Partners, talks with World of DaaS host Auren Hoffman. Hilary previously co-founded Fast Forward Labs, which was acquired by Cloudera, and served as the Chief Scientist at bit.ly. Auren and Hilary explore how data science has progressed in the past decade, the role of data science in an organization, and data ethics.
Number of Past Jobs 7. Hilary Mason has 2 current jobs as Co-Founder, Advisor at hackNY and Co-Founder at Hidden Door. Additionally, Hilary Mason has had 7 past jobs including General Manager, Machine Learning at Cloudera. hackNY Co-Founder, Advisor Feb 23, 2010. Hidden Door Co-Founder.
WIRED has challenged computer scientist and Hidden Door cofounder and CEO Hilary Mason to explain machine learning to 5 different people; a child, teen, a co...
About. WIRED has challenged computer scientist and Hidden Door cofounder and CEO Hilary Mason to explain machine learning to 5 different people; a child, teen, a college student, a grad student ...
Hilary Mason's career is defined by pushing the limits of what big data and machine learning can achieve. She is the co-founder and CEO of Hidden Door, a new gameplay platform that uses generative AI and LLMs to allow users to play in the world of their favorite book, movie, or TV show. She is also the Founder of Fast Forward Labs, a machine ...
Established in 2020, Hidden Door is building a new kind of story game experience, powered by a proprietary generative AI platform, that can adapt any existing work of fiction into an online social roleplaying game. We're excited to collaborate with storytellers from around the world, to create immersive fan-focused story experiences for everyone.
Hilary Mason is the Founder of Fast Forward Labs, a machine intelligence research company, and the Data Scientist in Residence at Accel. Previously, Hilary was the Chief Scientist at bitly. She also co-founded of HackNY, co-host DataGotham, and is a member of NYCResistor. Data & Society advances public understanding of the social implications ...
Hilary Mason is a Data Scientist who is now the Founder of Fast Forward Labs, a machine intelligence research company, and the Data Scientist in Residence at Accel. Previously, she was the Chief Scientist at bitly. She is famous for proving that social-media users like to share breaking news and current events, but are more likely to read inane gossip, watch sneezing pandas or play online games.
Hilary Mason is the author of Data Driven (3.81 avg rating, 410 ratings, 36 reviews, published 2015), Ethics and Data Science (4.20 avg rating, 147 ratin...
1 of 5 stars 2 of 5 stars 3 of 5 stars 4 of 5 stars 5 of 5 stars. In a Moment: Mini Topics for Classroom, Substitute and Supply Teachers With Children from Five to Eight (Belair Series) by. Stephanie Mudd, Hilary Mason. 3.50 avg rating — 2 ratings — published 1994 — 2 editions. Want to Read. saving….
Education & Community ... SHEKU & ISATA KANNEH-MASON DUO RECITAL. BUCKET-LIST BRILLIANCE. BIG SCREEN, BIG SCORES. Films and music with live orchestra. September 5-8. MUSIC FROM THE STUDIO GHIBLI FILMS OF HAYAO MIYAZAKI. ... ESA-PEKKA SALONEN & HILARY HAHN. FAVORITES IN FOCUS. FIXED PACKAGES: ORCHESTRAL SERIES ...
The Jimmy and Rosalynn Carter School at George Mason University, the top-ranked national public institution for peace and conflict resolution, has partnered with Rotary International, one of the largest global membership service organizations, to provide education and develop modalities to assist Rotary clubs in promoting local, regional, and global peace.