Have a language expert improve your writing
Run a free plagiarism check in 10 minutes, generate accurate citations for free.
- Knowledge Base
Methodology
- Survey Research | Definition, Examples & Methods

Survey Research | Definition, Examples & Methods
Published on August 20, 2019 by Shona McCombes . Revised on June 22, 2023.
Survey research means collecting information about a group of people by asking them questions and analyzing the results. To conduct an effective survey, follow these six steps:
- Determine who will participate in the survey
- Decide the type of survey (mail, online, or in-person)
- Design the survey questions and layout
- Distribute the survey
- Analyze the responses
- Write up the results
Surveys are a flexible method of data collection that can be used in many different types of research .
Table of contents
What are surveys used for, step 1: define the population and sample, step 2: decide on the type of survey, step 3: design the survey questions, step 4: distribute the survey and collect responses, step 5: analyze the survey results, step 6: write up the survey results, other interesting articles, frequently asked questions about surveys.
Surveys are used as a method of gathering data in many different fields. They are a good choice when you want to find out about the characteristics, preferences, opinions, or beliefs of a group of people.
Common uses of survey research include:
- Social research : investigating the experiences and characteristics of different social groups
- Market research : finding out what customers think about products, services, and companies
- Health research : collecting data from patients about symptoms and treatments
- Politics : measuring public opinion about parties and policies
- Psychology : researching personality traits, preferences and behaviours
Surveys can be used in both cross-sectional studies , where you collect data just once, and in longitudinal studies , where you survey the same sample several times over an extended period.
Receive feedback on language, structure, and formatting
Professional editors proofread and edit your paper by focusing on:
- Academic style
- Vague sentences
- Style consistency
See an example
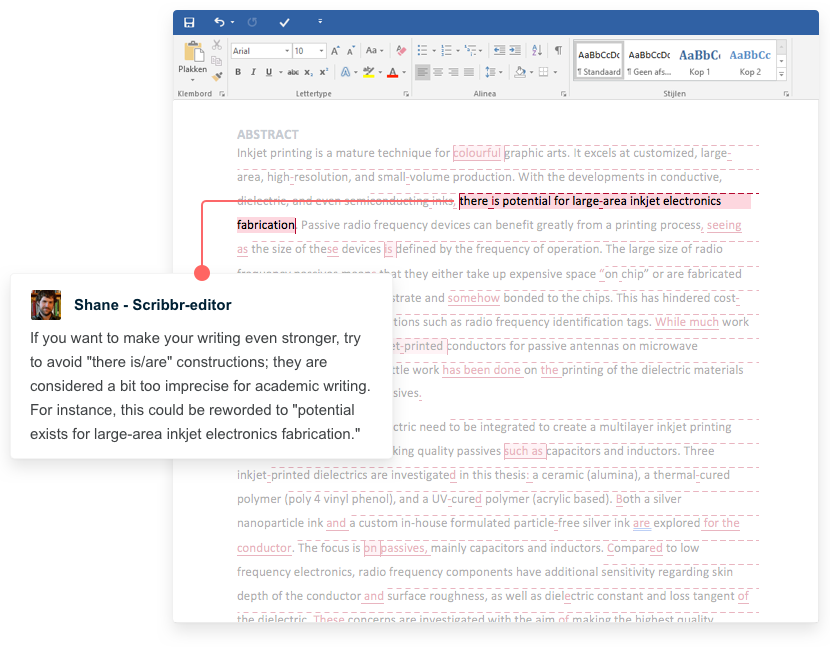
Before you start conducting survey research, you should already have a clear research question that defines what you want to find out. Based on this question, you need to determine exactly who you will target to participate in the survey.
Populations
The target population is the specific group of people that you want to find out about. This group can be very broad or relatively narrow. For example:
- The population of Brazil
- US college students
- Second-generation immigrants in the Netherlands
- Customers of a specific company aged 18-24
- British transgender women over the age of 50
Your survey should aim to produce results that can be generalized to the whole population. That means you need to carefully define exactly who you want to draw conclusions about.
Several common research biases can arise if your survey is not generalizable, particularly sampling bias and selection bias . The presence of these biases have serious repercussions for the validity of your results.
It’s rarely possible to survey the entire population of your research – it would be very difficult to get a response from every person in Brazil or every college student in the US. Instead, you will usually survey a sample from the population.
The sample size depends on how big the population is. You can use an online sample calculator to work out how many responses you need.
There are many sampling methods that allow you to generalize to broad populations. In general, though, the sample should aim to be representative of the population as a whole. The larger and more representative your sample, the more valid your conclusions. Again, beware of various types of sampling bias as you design your sample, particularly self-selection bias , nonresponse bias , undercoverage bias , and survivorship bias .
There are two main types of survey:
- A questionnaire , where a list of questions is distributed by mail, online or in person, and respondents fill it out themselves.
- An interview , where the researcher asks a set of questions by phone or in person and records the responses.
Which type you choose depends on the sample size and location, as well as the focus of the research.
Questionnaires
Sending out a paper survey by mail is a common method of gathering demographic information (for example, in a government census of the population).
- You can easily access a large sample.
- You have some control over who is included in the sample (e.g. residents of a specific region).
- The response rate is often low, and at risk for biases like self-selection bias .
Online surveys are a popular choice for students doing dissertation research , due to the low cost and flexibility of this method. There are many online tools available for constructing surveys, such as SurveyMonkey and Google Forms .
- You can quickly access a large sample without constraints on time or location.
- The data is easy to process and analyze.
- The anonymity and accessibility of online surveys mean you have less control over who responds, which can lead to biases like self-selection bias .
If your research focuses on a specific location, you can distribute a written questionnaire to be completed by respondents on the spot. For example, you could approach the customers of a shopping mall or ask all students to complete a questionnaire at the end of a class.
- You can screen respondents to make sure only people in the target population are included in the sample.
- You can collect time- and location-specific data (e.g. the opinions of a store’s weekday customers).
- The sample size will be smaller, so this method is less suitable for collecting data on broad populations and is at risk for sampling bias .
Oral interviews are a useful method for smaller sample sizes. They allow you to gather more in-depth information on people’s opinions and preferences. You can conduct interviews by phone or in person.
- You have personal contact with respondents, so you know exactly who will be included in the sample in advance.
- You can clarify questions and ask for follow-up information when necessary.
- The lack of anonymity may cause respondents to answer less honestly, and there is more risk of researcher bias.
Like questionnaires, interviews can be used to collect quantitative data: the researcher records each response as a category or rating and statistically analyzes the results. But they are more commonly used to collect qualitative data : the interviewees’ full responses are transcribed and analyzed individually to gain a richer understanding of their opinions and feelings.
Next, you need to decide which questions you will ask and how you will ask them. It’s important to consider:
- The type of questions
- The content of the questions
- The phrasing of the questions
- The ordering and layout of the survey
Open-ended vs closed-ended questions
There are two main forms of survey questions: open-ended and closed-ended. Many surveys use a combination of both.
Closed-ended questions give the respondent a predetermined set of answers to choose from. A closed-ended question can include:
- A binary answer (e.g. yes/no or agree/disagree )
- A scale (e.g. a Likert scale with five points ranging from strongly agree to strongly disagree )
- A list of options with a single answer possible (e.g. age categories)
- A list of options with multiple answers possible (e.g. leisure interests)
Closed-ended questions are best for quantitative research . They provide you with numerical data that can be statistically analyzed to find patterns, trends, and correlations .
Open-ended questions are best for qualitative research. This type of question has no predetermined answers to choose from. Instead, the respondent answers in their own words.
Open questions are most common in interviews, but you can also use them in questionnaires. They are often useful as follow-up questions to ask for more detailed explanations of responses to the closed questions.
The content of the survey questions
To ensure the validity and reliability of your results, you need to carefully consider each question in the survey. All questions should be narrowly focused with enough context for the respondent to answer accurately. Avoid questions that are not directly relevant to the survey’s purpose.
When constructing closed-ended questions, ensure that the options cover all possibilities. If you include a list of options that isn’t exhaustive, you can add an “other” field.
Phrasing the survey questions
In terms of language, the survey questions should be as clear and precise as possible. Tailor the questions to your target population, keeping in mind their level of knowledge of the topic. Avoid jargon or industry-specific terminology.
Survey questions are at risk for biases like social desirability bias , the Hawthorne effect , or demand characteristics . It’s critical to use language that respondents will easily understand, and avoid words with vague or ambiguous meanings. Make sure your questions are phrased neutrally, with no indication that you’d prefer a particular answer or emotion.
Ordering the survey questions
The questions should be arranged in a logical order. Start with easy, non-sensitive, closed-ended questions that will encourage the respondent to continue.
If the survey covers several different topics or themes, group together related questions. You can divide a questionnaire into sections to help respondents understand what is being asked in each part.
If a question refers back to or depends on the answer to a previous question, they should be placed directly next to one another.
Prevent plagiarism. Run a free check.
Before you start, create a clear plan for where, when, how, and with whom you will conduct the survey. Determine in advance how many responses you require and how you will gain access to the sample.
When you are satisfied that you have created a strong research design suitable for answering your research questions, you can conduct the survey through your method of choice – by mail, online, or in person.
There are many methods of analyzing the results of your survey. First you have to process the data, usually with the help of a computer program to sort all the responses. You should also clean the data by removing incomplete or incorrectly completed responses.
If you asked open-ended questions, you will have to code the responses by assigning labels to each response and organizing them into categories or themes. You can also use more qualitative methods, such as thematic analysis , which is especially suitable for analyzing interviews.
Statistical analysis is usually conducted using programs like SPSS or Stata. The same set of survey data can be subject to many analyses.
Finally, when you have collected and analyzed all the necessary data, you will write it up as part of your thesis, dissertation , or research paper .
In the methodology section, you describe exactly how you conducted the survey. You should explain the types of questions you used, the sampling method, when and where the survey took place, and the response rate. You can include the full questionnaire as an appendix and refer to it in the text if relevant.
Then introduce the analysis by describing how you prepared the data and the statistical methods you used to analyze it. In the results section, you summarize the key results from your analysis.
In the discussion and conclusion , you give your explanations and interpretations of these results, answer your research question, and reflect on the implications and limitations of the research.
If you want to know more about statistics , methodology , or research bias , make sure to check out some of our other articles with explanations and examples.
- Student’s t -distribution
- Normal distribution
- Null and Alternative Hypotheses
- Chi square tests
- Confidence interval
- Quartiles & Quantiles
- Cluster sampling
- Stratified sampling
- Data cleansing
- Reproducibility vs Replicability
- Peer review
- Prospective cohort study
Research bias
- Implicit bias
- Cognitive bias
- Placebo effect
- Hawthorne effect
- Hindsight bias
- Affect heuristic
- Social desirability bias
A questionnaire is a data collection tool or instrument, while a survey is an overarching research method that involves collecting and analyzing data from people using questionnaires.
A Likert scale is a rating scale that quantitatively assesses opinions, attitudes, or behaviors. It is made up of 4 or more questions that measure a single attitude or trait when response scores are combined.
To use a Likert scale in a survey , you present participants with Likert-type questions or statements, and a continuum of items, usually with 5 or 7 possible responses, to capture their degree of agreement.
Individual Likert-type questions are generally considered ordinal data , because the items have clear rank order, but don’t have an even distribution.
Overall Likert scale scores are sometimes treated as interval data. These scores are considered to have directionality and even spacing between them.
The type of data determines what statistical tests you should use to analyze your data.
The priorities of a research design can vary depending on the field, but you usually have to specify:
- Your research questions and/or hypotheses
- Your overall approach (e.g., qualitative or quantitative )
- The type of design you’re using (e.g., a survey , experiment , or case study )
- Your sampling methods or criteria for selecting subjects
- Your data collection methods (e.g., questionnaires , observations)
- Your data collection procedures (e.g., operationalization , timing and data management)
- Your data analysis methods (e.g., statistical tests or thematic analysis )
Cite this Scribbr article
If you want to cite this source, you can copy and paste the citation or click the “Cite this Scribbr article” button to automatically add the citation to our free Citation Generator.
McCombes, S. (2023, June 22). Survey Research | Definition, Examples & Methods. Scribbr. Retrieved July 30, 2024, from https://www.scribbr.com/methodology/survey-research/
Is this article helpful?
Shona McCombes
Other students also liked, qualitative vs. quantitative research | differences, examples & methods, questionnaire design | methods, question types & examples, what is a likert scale | guide & examples, get unlimited documents corrected.
✔ Free APA citation check included ✔ Unlimited document corrections ✔ Specialized in correcting academic texts
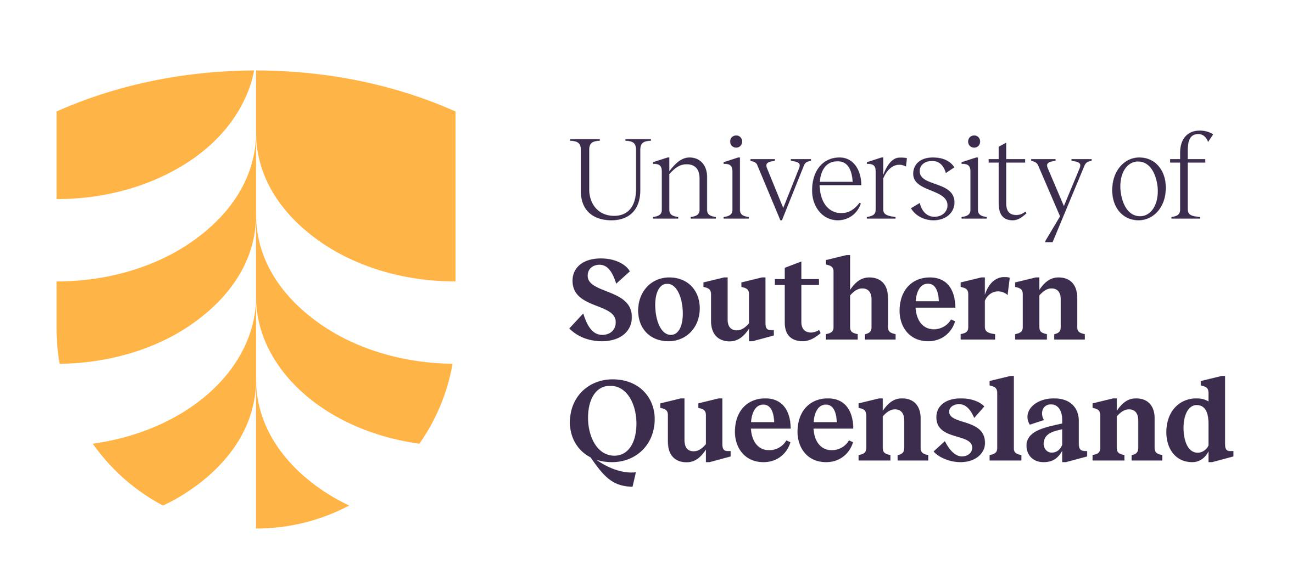
Want to create or adapt books like this? Learn more about how Pressbooks supports open publishing practices.
9 Survey research
Survey research is a research method involving the use of standardised questionnaires or interviews to collect data about people and their preferences, thoughts, and behaviours in a systematic manner. Although census surveys were conducted as early as Ancient Egypt, survey as a formal research method was pioneered in the 1930–40s by sociologist Paul Lazarsfeld to examine the effects of the radio on political opinion formation of the United States. This method has since become a very popular method for quantitative research in the social sciences.
The survey method can be used for descriptive, exploratory, or explanatory research. This method is best suited for studies that have individual people as the unit of analysis. Although other units of analysis, such as groups, organisations or dyads—pairs of organisations, such as buyers and sellers—are also studied using surveys, such studies often use a specific person from each unit as a ‘key informant’ or a ‘proxy’ for that unit. Consequently, such surveys may be subject to respondent bias if the chosen informant does not have adequate knowledge or has a biased opinion about the phenomenon of interest. For instance, Chief Executive Officers may not adequately know employees’ perceptions or teamwork in their own companies, and may therefore be the wrong informant for studies of team dynamics or employee self-esteem.
Survey research has several inherent strengths compared to other research methods. First, surveys are an excellent vehicle for measuring a wide variety of unobservable data, such as people’s preferences (e.g., political orientation), traits (e.g., self-esteem), attitudes (e.g., toward immigrants), beliefs (e.g., about a new law), behaviours (e.g., smoking or drinking habits), or factual information (e.g., income). Second, survey research is also ideally suited for remotely collecting data about a population that is too large to observe directly. A large area—such as an entire country—can be covered by postal, email, or telephone surveys using meticulous sampling to ensure that the population is adequately represented in a small sample. Third, due to their unobtrusive nature and the ability to respond at one’s convenience, questionnaire surveys are preferred by some respondents. Fourth, interviews may be the only way of reaching certain population groups such as the homeless or illegal immigrants for which there is no sampling frame available. Fifth, large sample surveys may allow detection of small effects even while analysing multiple variables, and depending on the survey design, may also allow comparative analysis of population subgroups (i.e., within-group and between-group analysis). Sixth, survey research is more economical in terms of researcher time, effort and cost than other methods such as experimental research and case research. At the same time, survey research also has some unique disadvantages. It is subject to a large number of biases such as non-response bias, sampling bias, social desirability bias, and recall bias, as discussed at the end of this chapter.
Depending on how the data is collected, survey research can be divided into two broad categories: questionnaire surveys (which may be postal, group-administered, or online surveys), and interview surveys (which may be personal, telephone, or focus group interviews). Questionnaires are instruments that are completed in writing by respondents, while interviews are completed by the interviewer based on verbal responses provided by respondents. As discussed below, each type has its own strengths and weaknesses in terms of their costs, coverage of the target population, and researcher’s flexibility in asking questions.
Questionnaire surveys
Invented by Sir Francis Galton, a questionnaire is a research instrument consisting of a set of questions (items) intended to capture responses from respondents in a standardised manner. Questions may be unstructured or structured. Unstructured questions ask respondents to provide a response in their own words, while structured questions ask respondents to select an answer from a given set of choices. Subjects’ responses to individual questions (items) on a structured questionnaire may be aggregated into a composite scale or index for statistical analysis. Questions should be designed in such a way that respondents are able to read, understand, and respond to them in a meaningful way, and hence the survey method may not be appropriate or practical for certain demographic groups such as children or the illiterate.
Most questionnaire surveys tend to be self-administered postal surveys , where the same questionnaire is posted to a large number of people, and willing respondents can complete the survey at their convenience and return it in prepaid envelopes. Postal surveys are advantageous in that they are unobtrusive and inexpensive to administer, since bulk postage is cheap in most countries. However, response rates from postal surveys tend to be quite low since most people ignore survey requests. There may also be long delays (several months) in respondents’ completing and returning the survey, or they may even simply lose it. Hence, the researcher must continuously monitor responses as they are being returned, track and send non-respondents repeated reminders (two or three reminders at intervals of one to one and a half months is ideal). Questionnaire surveys are also not well-suited for issues that require clarification on the part of the respondent or those that require detailed written responses. Longitudinal designs can be used to survey the same set of respondents at different times, but response rates tend to fall precipitously from one survey to the next.
A second type of survey is a group-administered questionnaire . A sample of respondents is brought together at a common place and time, and each respondent is asked to complete the survey questionnaire while in that room. Respondents enter their responses independently without interacting with one another. This format is convenient for the researcher, and a high response rate is assured. If respondents do not understand any specific question, they can ask for clarification. In many organisations, it is relatively easy to assemble a group of employees in a conference room or lunch room, especially if the survey is approved by corporate executives.
A more recent type of questionnaire survey is an online or web survey. These surveys are administered over the Internet using interactive forms. Respondents may receive an email request for participation in the survey with a link to a website where the survey may be completed. Alternatively, the survey may be embedded into an email, and can be completed and returned via email. These surveys are very inexpensive to administer, results are instantly recorded in an online database, and the survey can be easily modified if needed. However, if the survey website is not password-protected or designed to prevent multiple submissions, the responses can be easily compromised. Furthermore, sampling bias may be a significant issue since the survey cannot reach people who do not have computer or Internet access, such as many of the poor, senior, and minority groups, and the respondent sample is skewed toward a younger demographic who are online much of the time and have the time and ability to complete such surveys. Computing the response rate may be problematic if the survey link is posted on LISTSERVs or bulletin boards instead of being emailed directly to targeted respondents. For these reasons, many researchers prefer dual-media surveys (e.g., postal survey and online survey), allowing respondents to select their preferred method of response.
Constructing a survey questionnaire is an art. Numerous decisions must be made about the content of questions, their wording, format, and sequencing, all of which can have important consequences for the survey responses.
Response formats. Survey questions may be structured or unstructured. Responses to structured questions are captured using one of the following response formats:
Dichotomous response , where respondents are asked to select one of two possible choices, such as true/false, yes/no, or agree/disagree. An example of such a question is: Do you think that the death penalty is justified under some circumstances? (circle one): yes / no.
Nominal response , where respondents are presented with more than two unordered options, such as: What is your industry of employment?: manufacturing / consumer services / retail / education / healthcare / tourism and hospitality / other.
Ordinal response , where respondents have more than two ordered options, such as: What is your highest level of education?: high school / bachelor’s degree / postgraduate degree.
Interval-level response , where respondents are presented with a 5-point or 7-point Likert scale, semantic differential scale, or Guttman scale. Each of these scale types were discussed in a previous chapter.
Continuous response , where respondents enter a continuous (ratio-scaled) value with a meaningful zero point, such as their age or tenure in a firm. These responses generally tend to be of the fill-in-the blanks type.
Question content and wording. Responses obtained in survey research are very sensitive to the types of questions asked. Poorly framed or ambiguous questions will likely result in meaningless responses with very little value. Dillman (1978) [1] recommends several rules for creating good survey questions. Every single question in a survey should be carefully scrutinised for the following issues:
Is the question clear and understandable ?: Survey questions should be stated in very simple language, preferably in active voice, and without complicated words or jargon that may not be understood by a typical respondent. All questions in the questionnaire should be worded in a similar manner to make it easy for respondents to read and understand them. The only exception is if your survey is targeted at a specialised group of respondents, such as doctors, lawyers and researchers, who use such jargon in their everyday environment. Is the question worded in a negative manner ?: Negatively worded questions such as ‘Should your local government not raise taxes?’ tend to confuse many respondents and lead to inaccurate responses. Double-negatives should be avoided when designing survey questions.
Is the question ambiguous ?: Survey questions should not use words or expressions that may be interpreted differently by different respondents (e.g., words like ‘any’ or ‘just’). For instance, if you ask a respondent, ‘What is your annual income?’, it is unclear whether you are referring to salary/wages, or also dividend, rental, and other income, whether you are referring to personal income, family income (including spouse’s wages), or personal and business income. Different interpretation by different respondents will lead to incomparable responses that cannot be interpreted correctly.
Does the question have biased or value-laden words ?: Bias refers to any property of a question that encourages subjects to answer in a certain way. Kenneth Rasinky (1989) [2] examined several studies on people’s attitude toward government spending, and observed that respondents tend to indicate stronger support for ‘assistance to the poor’ and less for ‘welfare’, even though both terms had the same meaning. In this study, more support was also observed for ‘halting rising crime rate’ and less for ‘law enforcement’, more for ‘solving problems of big cities’ and less for ‘assistance to big cities’, and more for ‘dealing with drug addiction’ and less for ‘drug rehabilitation’. A biased language or tone tends to skew observed responses. It is often difficult to anticipate in advance the biasing wording, but to the greatest extent possible, survey questions should be carefully scrutinised to avoid biased language.
Is the question double-barrelled ?: Double-barrelled questions are those that can have multiple answers. For example, ‘Are you satisfied with the hardware and software provided for your work?’. In this example, how should a respondent answer if they are satisfied with the hardware, but not with the software, or vice versa? It is always advisable to separate double-barrelled questions into separate questions: ‘Are you satisfied with the hardware provided for your work?’, and ’Are you satisfied with the software provided for your work?’. Another example: ‘Does your family favour public television?’. Some people may favour public TV for themselves, but favour certain cable TV programs such as Sesame Street for their children.
Is the question too general ?: Sometimes, questions that are too general may not accurately convey respondents’ perceptions. If you asked someone how they liked a certain book and provided a response scale ranging from ‘not at all’ to ‘extremely well’, if that person selected ‘extremely well’, what do they mean? Instead, ask more specific behavioural questions, such as, ‘Will you recommend this book to others, or do you plan to read other books by the same author?’. Likewise, instead of asking, ‘How big is your firm?’ (which may be interpreted differently by respondents), ask, ‘How many people work for your firm?’, and/or ‘What is the annual revenue of your firm?’, which are both measures of firm size.
Is the question too detailed ?: Avoid unnecessarily detailed questions that serve no specific research purpose. For instance, do you need the age of each child in a household, or is just the number of children in the household acceptable? However, if unsure, it is better to err on the side of details than generality.
Is the question presumptuous ?: If you ask, ‘What do you see as the benefits of a tax cut?’, you are presuming that the respondent sees the tax cut as beneficial. Many people may not view tax cuts as being beneficial, because tax cuts generally lead to lesser funding for public schools, larger class sizes, and fewer public services such as police, ambulance, and fire services. Avoid questions with built-in presumptions.
Is the question imaginary ?: A popular question in many television game shows is, ‘If you win a million dollars on this show, how will you spend it?’. Most respondents have never been faced with such an amount of money before and have never thought about it—they may not even know that after taxes, they will get only about $640,000 or so in the United States, and in many cases, that amount is spread over a 20-year period—and so their answers tend to be quite random, such as take a tour around the world, buy a restaurant or bar, spend on education, save for retirement, help parents or children, or have a lavish wedding. Imaginary questions have imaginary answers, which cannot be used for making scientific inferences.
Do respondents have the information needed to correctly answer the question ?: Oftentimes, we assume that subjects have the necessary information to answer a question, when in reality, they do not. Even if a response is obtained, these responses tend to be inaccurate given the subjects’ lack of knowledge about the question being asked. For instance, we should not ask the CEO of a company about day-to-day operational details that they may not be aware of, or ask teachers about how much their students are learning, or ask high-schoolers, ‘Do you think the US Government acted appropriately in the Bay of Pigs crisis?’.
Question sequencing. In general, questions should flow logically from one to the next. To achieve the best response rates, questions should flow from the least sensitive to the most sensitive, from the factual and behavioural to the attitudinal, and from the more general to the more specific. Some general rules for question sequencing:
Start with easy non-threatening questions that can be easily recalled. Good options are demographics (age, gender, education level) for individual-level surveys and firmographics (employee count, annual revenues, industry) for firm-level surveys.
Never start with an open ended question.
If following a historical sequence of events, follow a chronological order from earliest to latest.
Ask about one topic at a time. When switching topics, use a transition, such as, ‘The next section examines your opinions about…’
Use filter or contingency questions as needed, such as, ‘If you answered “yes” to question 5, please proceed to Section 2. If you answered “no” go to Section 3′.
Other golden rules . Do unto your respondents what you would have them do unto you. Be attentive and appreciative of respondents’ time, attention, trust, and confidentiality of personal information. Always practice the following strategies for all survey research:
People’s time is valuable. Be respectful of their time. Keep your survey as short as possible and limit it to what is absolutely necessary. Respondents do not like spending more than 10-15 minutes on any survey, no matter how important it is. Longer surveys tend to dramatically lower response rates.
Always assure respondents about the confidentiality of their responses, and how you will use their data (e.g., for academic research) and how the results will be reported (usually, in the aggregate).
For organisational surveys, assure respondents that you will send them a copy of the final results, and make sure that you follow up with your promise.
Thank your respondents for their participation in your study.
Finally, always pretest your questionnaire, at least using a convenience sample, before administering it to respondents in a field setting. Such pretesting may uncover ambiguity, lack of clarity, or biases in question wording, which should be eliminated before administering to the intended sample.
Interview survey
Interviews are a more personalised data collection method than questionnaires, and are conducted by trained interviewers using the same research protocol as questionnaire surveys (i.e., a standardised set of questions). However, unlike a questionnaire, the interview script may contain special instructions for the interviewer that are not seen by respondents, and may include space for the interviewer to record personal observations and comments. In addition, unlike postal surveys, the interviewer has the opportunity to clarify any issues raised by the respondent or ask probing or follow-up questions. However, interviews are time-consuming and resource-intensive. Interviewers need special interviewing skills as they are considered to be part of the measurement instrument, and must proactively strive not to artificially bias the observed responses.
The most typical form of interview is a personal or face-to-face interview , where the interviewer works directly with the respondent to ask questions and record their responses. Personal interviews may be conducted at the respondent’s home or office location. This approach may even be favoured by some respondents, while others may feel uncomfortable allowing a stranger into their homes. However, skilled interviewers can persuade respondents to co-operate, dramatically improving response rates.
A variation of the personal interview is a group interview, also called a focus group . In this technique, a small group of respondents (usually 6–10 respondents) are interviewed together in a common location. The interviewer is essentially a facilitator whose job is to lead the discussion, and ensure that every person has an opportunity to respond. Focus groups allow deeper examination of complex issues than other forms of survey research, because when people hear others talk, it often triggers responses or ideas that they did not think about before. However, focus group discussion may be dominated by a dominant personality, and some individuals may be reluctant to voice their opinions in front of their peers or superiors, especially while dealing with a sensitive issue such as employee underperformance or office politics. Because of their small sample size, focus groups are usually used for exploratory research rather than descriptive or explanatory research.
A third type of interview survey is a telephone interview . In this technique, interviewers contact potential respondents over the phone, typically based on a random selection of people from a telephone directory, to ask a standard set of survey questions. A more recent and technologically advanced approach is computer-assisted telephone interviewing (CATI). This is increasing being used by academic, government, and commercial survey researchers. Here the interviewer is a telephone operator who is guided through the interview process by a computer program displaying instructions and questions to be asked. The system also selects respondents randomly using a random digit dialling technique, and records responses using voice capture technology. Once respondents are on the phone, higher response rates can be obtained. This technique is not ideal for rural areas where telephone density is low, and also cannot be used for communicating non-audio information such as graphics or product demonstrations.
Role of interviewer. The interviewer has a complex and multi-faceted role in the interview process, which includes the following tasks:
Prepare for the interview: Since the interviewer is in the forefront of the data collection effort, the quality of data collected depends heavily on how well the interviewer is trained to do the job. The interviewer must be trained in the interview process and the survey method, and also be familiar with the purpose of the study, how responses will be stored and used, and sources of interviewer bias. They should also rehearse and time the interview prior to the formal study.
Locate and enlist the co-operation of respondents: Particularly in personal, in-home surveys, the interviewer must locate specific addresses, and work around respondents’ schedules at sometimes undesirable times such as during weekends. They should also be like a salesperson, selling the idea of participating in the study.
Motivate respondents: Respondents often feed off the motivation of the interviewer. If the interviewer is disinterested or inattentive, respondents will not be motivated to provide useful or informative responses either. The interviewer must demonstrate enthusiasm about the study, communicate the importance of the research to respondents, and be attentive to respondents’ needs throughout the interview.
Clarify any confusion or concerns: Interviewers must be able to think on their feet and address unanticipated concerns or objections raised by respondents to the respondents’ satisfaction. Additionally, they should ask probing questions as necessary even if such questions are not in the script.
Observe quality of response: The interviewer is in the best position to judge the quality of information collected, and may supplement responses obtained using personal observations of gestures or body language as appropriate.
Conducting the interview. Before the interview, the interviewer should prepare a kit to carry to the interview session, consisting of a cover letter from the principal investigator or sponsor, adequate copies of the survey instrument, photo identification, and a telephone number for respondents to call to verify the interviewer’s authenticity. The interviewer should also try to call respondents ahead of time to set up an appointment if possible. To start the interview, they should speak in an imperative and confident tone, such as, ‘I’d like to take a few minutes of your time to interview you for a very important study’, instead of, ‘May I come in to do an interview?’. They should introduce themself, present personal credentials, explain the purpose of the study in one to two sentences, and assure respondents that their participation is voluntary, and their comments are confidential, all in less than a minute. No big words or jargon should be used, and no details should be provided unless specifically requested. If the interviewer wishes to record the interview, they should ask for respondents’ explicit permission before doing so. Even if the interview is recorded, the interviewer must take notes on key issues, probes, or verbatim phrases
During the interview, the interviewer should follow the questionnaire script and ask questions exactly as written, and not change the words to make the question sound friendlier. They should also not change the order of questions or skip any question that may have been answered earlier. Any issues with the questions should be discussed during rehearsal prior to the actual interview sessions. The interviewer should not finish the respondent’s sentences. If the respondent gives a brief cursory answer, the interviewer should probe the respondent to elicit a more thoughtful, thorough response. Some useful probing techniques are:
The silent probe: Just pausing and waiting without going into the next question may suggest to respondents that the interviewer is waiting for more detailed response.
Overt encouragement: An occasional ‘uh-huh’ or ‘okay’ may encourage the respondent to go into greater details. However, the interviewer must not express approval or disapproval of what the respondent says.
Ask for elaboration: Such as, ‘Can you elaborate on that?’ or ‘A minute ago, you were talking about an experience you had in high school. Can you tell me more about that?’.
Reflection: The interviewer can try the psychotherapist’s trick of repeating what the respondent said. For instance, ‘What I’m hearing is that you found that experience very traumatic’ and then pause and wait for the respondent to elaborate.
After the interview is completed, the interviewer should thank respondents for their time, tell them when to expect the results, and not leave hastily. Immediately after leaving, they should write down any notes or key observations that may help interpret the respondent’s comments better.
Biases in survey research
Despite all of its strengths and advantages, survey research is often tainted with systematic biases that may invalidate some of the inferences derived from such surveys. Five such biases are the non-response bias, sampling bias, social desirability bias, recall bias, and common method bias.
Non-response bias. Survey research is generally notorious for its low response rates. A response rate of 15-20 per cent is typical in a postal survey, even after two or three reminders. If the majority of the targeted respondents fail to respond to a survey, this may indicate a systematic reason for the low response rate, which may in turn raise questions about the validity of the study’s results. For instance, dissatisfied customers tend to be more vocal about their experience than satisfied customers, and are therefore more likely to respond to questionnaire surveys or interview requests than satisfied customers. Hence, any respondent sample is likely to have a higher proportion of dissatisfied customers than the underlying population from which it is drawn. In this instance, not only will the results lack generalisability, but the observed outcomes may also be an artefact of the biased sample. Several strategies may be employed to improve response rates:
Advance notification: Sending a short letter to the targeted respondents soliciting their participation in an upcoming survey can prepare them in advance and improve their propensity to respond. The letter should state the purpose and importance of the study, mode of data collection (e.g., via a phone call, a survey form in the mail, etc.), and appreciation for their co-operation. A variation of this technique may be to ask the respondent to return a prepaid postcard indicating whether or not they are willing to participate in the study.
Relevance of content: People are more likely to respond to surveys examining issues of relevance or importance to them.
Respondent-friendly questionnaire: Shorter survey questionnaires tend to elicit higher response rates than longer questionnaires. Furthermore, questions that are clear, non-offensive, and easy to respond tend to attract higher response rates.
Endorsement: For organisational surveys, it helps to gain endorsement from a senior executive attesting to the importance of the study to the organisation. Such endorsement can be in the form of a cover letter or a letter of introduction, which can improve the researcher’s credibility in the eyes of the respondents.
Follow-up requests: Multiple follow-up requests may coax some non-respondents to respond, even if their responses are late.
Interviewer training: Response rates for interviews can be improved with skilled interviewers trained in how to request interviews, use computerised dialling techniques to identify potential respondents, and schedule call-backs for respondents who could not be reached.
Incentives : Incentives in the form of cash or gift cards, giveaways such as pens or stress balls, entry into a lottery, draw or contest, discount coupons, promise of contribution to charity, and so forth may increase response rates.
Non-monetary incentives: Businesses, in particular, are more prone to respond to non-monetary incentives than financial incentives. An example of such a non-monetary incentive is a benchmarking report comparing the business’s individual response against the aggregate of all responses to a survey.
Confidentiality and privacy: Finally, assurances that respondents’ private data or responses will not fall into the hands of any third party may help improve response rates
Sampling bias. Telephone surveys conducted by calling a random sample of publicly available telephone numbers will systematically exclude people with unlisted telephone numbers, mobile phone numbers, and people who are unable to answer the phone when the survey is being conducted—for instance, if they are at work—and will include a disproportionate number of respondents who have landline telephone services with listed phone numbers and people who are home during the day, such as the unemployed, the disabled, and the elderly. Likewise, online surveys tend to include a disproportionate number of students and younger people who are constantly on the Internet, and systematically exclude people with limited or no access to computers or the Internet, such as the poor and the elderly. Similarly, questionnaire surveys tend to exclude children and the illiterate, who are unable to read, understand, or meaningfully respond to the questionnaire. A different kind of sampling bias relates to sampling the wrong population, such as asking teachers (or parents) about their students’ (or children’s) academic learning, or asking CEOs about operational details in their company. Such biases make the respondent sample unrepresentative of the intended population and hurt generalisability claims about inferences drawn from the biased sample.
Social desirability bias . Many respondents tend to avoid negative opinions or embarrassing comments about themselves, their employers, family, or friends. With negative questions such as, ‘Do you think that your project team is dysfunctional?’, ‘Is there a lot of office politics in your workplace?’, ‘Or have you ever illegally downloaded music files from the Internet?’, the researcher may not get truthful responses. This tendency among respondents to ‘spin the truth’ in order to portray themselves in a socially desirable manner is called the ‘social desirability bias’, which hurts the validity of responses obtained from survey research. There is practically no way of overcoming the social desirability bias in a questionnaire survey, but in an interview setting, an astute interviewer may be able to spot inconsistent answers and ask probing questions or use personal observations to supplement respondents’ comments.
Recall bias. Responses to survey questions often depend on subjects’ motivation, memory, and ability to respond. Particularly when dealing with events that happened in the distant past, respondents may not adequately remember their own motivations or behaviours, or perhaps their memory of such events may have evolved with time and no longer be retrievable. For instance, if a respondent is asked to describe his/her utilisation of computer technology one year ago, or even memorable childhood events like birthdays, their response may not be accurate due to difficulties with recall. One possible way of overcoming the recall bias is by anchoring the respondent’s memory in specific events as they happened, rather than asking them to recall their perceptions and motivations from memory.
Common method bias. Common method bias refers to the amount of spurious covariance shared between independent and dependent variables that are measured at the same point in time, such as in a cross-sectional survey, using the same instrument, such as a questionnaire. In such cases, the phenomenon under investigation may not be adequately separated from measurement artefacts. Standard statistical tests are available to test for common method bias, such as Harmon’s single-factor test (Podsakoff, MacKenzie, Lee & Podsakoff, 2003), [3] Lindell and Whitney’s (2001) [4] market variable technique, and so forth. This bias can potentially be avoided if the independent and dependent variables are measured at different points in time using a longitudinal survey design, or if these variables are measured using different methods, such as computerised recording of dependent variable versus questionnaire-based self-rating of independent variables.
- Dillman, D. (1978). Mail and telephone surveys: The total design method . New York: Wiley. ↵
- Rasikski, K. (1989). The effect of question wording on public support for government spending. Public Opinion Quarterly , 53(3), 388–394. ↵
- Podsakoff, P. M., MacKenzie, S. B., Lee, J.-Y., & Podsakoff, N. P. (2003). Common method biases in behavioral research: A critical review of the literature and recommended remedies. Journal of Applied Psychology , 88(5), 879–903. http://dx.doi.org/10.1037/0021-9010.88.5.879. ↵
- Lindell, M. K., & Whitney, D. J. (2001). Accounting for common method variance in cross-sectional research designs. Journal of Applied Psychology , 86(1), 114–121. ↵
Social Science Research: Principles, Methods and Practices (Revised edition) Copyright © 2019 by Anol Bhattacherjee is licensed under a Creative Commons Attribution-NonCommercial-ShareAlike 4.0 International License , except where otherwise noted.
Share This Book

Want to create or adapt books like this? Learn more about how Pressbooks supports open publishing practices.
Chapter 9: Survey Research
9.1 Overview of Survey Research 9.2 Constructing Survey Questionnaires 9.3 Conducting Surveys
Research Methods in Psychology Copyright © 2016 by University of Minnesota is licensed under a Creative Commons Attribution-NonCommercial-ShareAlike 4.0 International License , except where otherwise noted.

Want to create or adapt books like this? Learn more about how Pressbooks supports open publishing practices.
5 Approaching Survey Research
What is survey research.
Survey research is a quantitative and qualitative method with two important characteristics. First, the variables of interest are measured using self-reports (using questionnaires or interviews). In essence, survey researchers ask their participants (who are often called respondents in survey research) to report directly on their own thoughts, feelings, and behaviors. Second, considerable attention is paid to the issue of sampling. In particular, survey researchers have a strong preference for large random samples because they provide the most accurate estimates of what is true in the population. Beyond these two characteristics, almost anything goes in survey research. Surveys can be long or short. They can be conducted in person, by telephone, through the mail, or over the Internet. They can be about voting intentions, consumer preferences, social attitudes, health, or anything else that it is possible to ask people about and receive meaningful answers. Although survey data are often analyzed using statistics, there are many questions that lend themselves to more qualitative analysis.
Most survey research is non-experimental. It is used to describe single variables (e.g., the percentage of voters who prefer one presidential candidate or another, the prevalence of schizophrenia in the general population, etc.) and also to assess statistical relationships between variables (e.g., the relationship between income and health). But surveys can also be used within experimental research; as long as there is manipulation of an independent variable (e.g. anger vs. fear) to assess an effect on a dependent variable (e.g. risk judgments).
Chapter 5: Learning Objectives
If your research question(s) center on the experience or perception of a particular phenomenon, process, or practice, utilizing a survey method may help glean useful data. After reading this chapter, you will
- Identify the purpose of survey research
- Describe the cognitive processes involved in responding to questions
- Discuss the importance of context in drafting survey items
- Contrast the utility of open and closed ended questions
- Describe the BRUSO method of drafting survey questions
- Describe the format for survey questionnaires
The heart of any survey research project is the survey itself. Although it is easy to think of interesting questions to ask people, constructing a good survey is not easy at all. The problem is that the answers people give can be influenced in unintended ways by the wording of the items, the order of the items, the response options provided, and many other factors. At best, these influences add noise to the data. At worst, they result in systematic biases and misleading results. In this section, therefore, we consider some principles for constructing surveys to minimize these unintended effects and thereby maximize the reliability and validity of respondents’ answers.
Cognitive Processes of Responses
To best understand how to write a ‘good’ survey question, it is important to frame the act of responding to a survey question as a cognitive process. That is, there are are involuntary mechanisms that take place when someone is asked a question. Sudman, Bradburn, & Schwarz (1996, as cited in Jhangiani et. al, 2012) illustrate this cognitive process here.
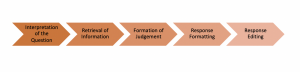
Framing the formulation of survey questions in this way is extremely helpful to ensure that the questions posed on your survey glean accurate information.
Example of a Poorly Worded Survey Question
How many alcoholic drinks do you consume in a typical day?
- A lot more of average
- Somewhat more than average
- Average number
- Somewhat fewer than average
- A lot fewer than average
Although this item at first seems straightforward, it poses several difficulties for respondents. First, they must interpret the question. For example, they must decide whether “alcoholic drinks” include beer and wine (as opposed to just hard liquor) and whether a “typical day” is a typical weekday, typical weekend day, or both. Even though Chang and Krosnick (2003, as cited in Jhangiani et al. 2012) found that asking about “typical” behavior has been shown to be more valid than asking about “past” behavior, their study compared “typical week” to “past week” and may be different when considering typical weekdays or weekend days). Once respondents have interpreted the question, they must retrieve relevant information from memory to answer it. But what information should they retrieve, and how should they go about retrieving it? They might think vaguely about some recent occasions on which they drank alcohol, they might carefully try to recall and count the number of alcoholic drinks they consumed last week, or they might retrieve some existing beliefs that they have about themselves (e.g., “I am not much of a drinker”). Then they must use this information to arrive at a tentative judgment about how many alcoholic drinks they consume in a typical day. For example, this mental calculation might mean dividing the number of alcoholic drinks they consumed last week by seven to come up with an average number per day. Then they must format this tentative answer in terms of the response options actually provided. In this case, the options pose additional problems of interpretation. For example, what does “average” mean, and what would count as “somewhat more” than average? Finally, they must decide whether they want to report the response they have come up with or whether they want to edit it in some way. For example, if they believe that they drink a lot more than average, they might not want to report that for fear of looking bad in the eyes of the researcher, so instead, they may opt to select the “somewhat more than average” response option.
From this perspective, what at first appears to be a simple matter of asking people how much they drink (and receiving a straightforward answer from them) turns out to be much more complex.
Context Effects on Survey Responses
Again, this complexity can lead to unintended influences on respondents’ answers. These are often referred to as context effects because they are not related to the content of the item but to the context in which the item appears (Schwarz & Strack, 1990, as cited in Jhangiani et al. 2012). For example, there is an item-order effect when the order in which the items are presented affects people’s responses. One item can change how participants interpret a later item or change the information that they retrieve to respond to later items. For example, researcher Fritz Strack and his colleagues asked college students about both their general life satisfaction and their dating frequency (Strack, Martin, & Schwarz, 1988, as cited in Jhangiani et al. 2012) . When the life satisfaction item came first, the correlation between the two was only −.12, suggesting that the two variables are only weakly related. But when the dating frequency item came first, the correlation between the two was +.66, suggesting that those who date more have a strong tendency to be more satisfied with their lives. Reporting the dating frequency first made that information more accessible in memory so that they were more likely to base their life satisfaction rating on it.
The response options provided can also have unintended effects on people’s responses (Schwarz, 1999, as cited in Jhangiani et al. 2012) . For example, when people are asked how often they are “really irritated” and given response options ranging from “less than once a year” to “more than once a month,” they tend to think of major irritations and report being irritated infrequently. But when they are given response options ranging from “less than once a day” to “several times a month,” they tend to think of minor irritations and report being irritated frequently. People also tend to assume that middle response options represent what is normal or typical. So if they think of themselves as normal or typical, they tend to choose middle response options. For example, people are likely to report watching more television when the response options are centered on a middle option of 4 hours than when centered on a middle option of 2 hours. To mitigate against order effects, rotate questions and response items when there is no natural order. Counterbalancing or randomizing the order of presentation of the questions in online surveys are good practices for survey questions and can reduce response order effects that show that among undecided voters, the first candidate listed in a ballot receives a 2.5% boost simply by virtue of being listed first!
Writing Survey Items
Types of Items
Questionnaire items can be either open-ended or closed-ended. Open-ended items simply ask a question and allow participants to answer in whatever way they choose. The following are examples of open-ended questionnaire items.
- “What is the most important thing to teach children to prepare them for life?”
- “Please describe a time when you were discriminated against because of your age.”
- “Is there anything else you would like to tell us about?”
Open-ended items are useful when researchers do not know how participants might respond or when they want to avoid influencing their responses. Open-ended items are more qualitative in nature, so they tend to be used when researchers have more vaguely defined research questions—often in the early stages of a research project. Open-ended items are relatively easy to write because there are no response options to worry about. However, they take more time and effort on the part of participants, and they are more difficult for the researcher to analyze because the answers must be transcribed, coded, and submitted to some form of qualitative analysis, such as content analysis. Another disadvantage is that respondents are more likely to skip open-ended items because they take longer to answer. It is best to use open-ended questions when the answer is unsure or for quantities which can easily be converted to categories later in the analysis.
Closed-ended items ask a question and provide a set of response options for participants to choose from.
Examples of Closed-Ended Questions
How old are you?
On a scale of 0 (no pain at all) to 10 (the worst pain ever experienced), how much pain are you in right now?
Closed-ended items are used when researchers have a good idea of the different responses that participants might make. They are more quantitative in nature, so they are also used when researchers are interested in a well-defined variable or construct such as participants’ level of agreement with some statement, perceptions of risk, or frequency of a particular behavior. Closed-ended items are more difficult to write because they must include an appropriate set of response options. However, they are relatively quick and easy for participants to complete. They are also much easier for researchers to analyze because the responses can be easily converted to numbers and entered into a spreadsheet. For these reasons, closed- ended items are much more common.
All closed-ended items include a set of response options from which a participant must choose. For categorical variables like sex, race, or political party preference, the categories are usually listed and participants choose the one (or ones) to which they belong. For quantitative variables, a rating scale is typically provided. A rating scale is an ordered set of responses that participants must choose from.
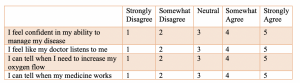
The number of response options on a typical rating scale ranges from three to 11—although five and seven are probably most common. Five-point scales are best for unipolar scales where only one construct is tested, such as frequency (Never, Rarely, Sometimes, Often, Always). Seven- point scales are best for bipolar scales where there is a dichotomous spectrum, such as liking (Like very much, Like somewhat, Like slightly, Neither like nor dislike, Dislike slightly, Dislike somewhat, Dislike very much). For bipolar questions, it is useful to offer an earlier question that branches them into an area of the scale; if asking about liking ice cream, first ask “Do you generally like or dislike ice cream?” Once the respondent chooses like or dislike, refine it by offering them relevant choices from the seven-point scale. Branching improves both reliability and validity (Krosnick & Berent, 1993, as cited in Jhangiani et al. 2012 ) . Although you often see scales with numerical labels, it is best to only present verbal labels to the respondents but convert them to numerical values in the analyses. Avoid partial labels or length or overly specific labels. In some cases, the verbal labels can be supplemented with (or even replaced by) meaningful graphics.
Writing Effective Items
We can now consider some principles of writing questionnaire items that minimize unintended context effects and maximize the reliability and validity of participants’ responses. A rough guideline for writing 9 questionnaire items is provided by the BRUSO model (Peterson, 2000, as cited in Jhangiani et al. 2012 ) . An acronym, BRUSO stands for “brief,” “relevant,” “unambiguous,” “specific,” and “objective.” Effective questionnaire items are brief and to the point. They avoid long, overly technical, or unnecessary words. This brevity makes them easier for respondents to understand and faster for them to complete. Effective questionnaire items are also relevant to the research question. If a respondent’s sexual orientation, marital status, or income is not relevant, then items on them should probably not be included. Again, this makes the questionnaire faster to complete, but it also avoids annoying respondents with what they will rightly perceive as irrelevant or even “nosy” questions. Effective questionnaire items are also unambiguous; they can be interpreted in only one way. Part of the problem with the alcohol item presented earlier in this section is that different respondents might have different ideas about what constitutes “an alcoholic drink” or “a typical day.” Effective questionnaire items are also specific so that it is clear to respondents what their response should be about and clear to researchers what it is about. A common problem here is closed- ended items that are “double barreled .” They ask about two conceptually separate issues but allow only one response.
Example of a “Double Barreled” question
Please rate the extent to which you have been feeling anxious and depressed
Note: The issue in the question itself is that anxiety and depression are two separate items and should likely be separated
Finally, effective questionnaire items are objective in the sense that they do not reveal the researcher’s own opinions or lead participants to answer in a particular way. The best way to know how people interpret the wording of the question is to conduct a pilot test and ask a few people to explain how they interpreted the question.
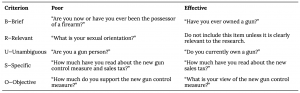
For closed-ended items, it is also important to create an appropriate response scale. For categorical variables, the categories presented should generally be mutually exclusive and exhaustive. Mutually exclusive categories do not overlap. For a religion item, for example, the categories of Christian and Catholic are not mutually exclusive but Protestant and Catholic are mutually exclusive. Exhaustive categories cover all possible responses. Although Protestant and Catholic are mutually exclusive, they are not exhaustive because there are many other religious categories that a respondent might select: Jewish, Hindu, Buddhist, and so on. In many cases, it is not feasible to include every possible category, in which case an ‘Other’ category, with a space for the respondent to fill in a more specific response, is a good solution. If respondents could belong to more than one category (e.g., race), they should be instructed to choose all categories that apply.
For rating scales, five or seven response options generally allow about as much precision as respondents are capable of. However, numerical scales with more options can sometimes be appropriate. For dimensions such as attractiveness, pain, and likelihood, a 0-to-10 scale will be familiar to many respondents and easy for them to use. Regardless of the number of response options, the most extreme ones should generally be “balanced” around a neutral or modal midpoint.
Example of an unbalanced versus balanced rating scale
Unbalanced rating scale measuring perceived likelihood
Unlikely | Somewhat Likely | Likely | Very Likely | Extremely Likely
Balanced rating scale measuring perceived likelihood
Extremely Unlikely | Somewhat Unlikely | As Likely as Not | Somewhat Likely |Extremely Likely
Note, however, that a middle or neutral response option does not have to be included. Researchers sometimes choose to leave it out because they want to encourage respondents to think more deeply about their response and not simply choose the middle option by default. However, including middle alternatives on bipolar dimensions can be used to allow people to choose an option that is neither.
Formatting the Survey
Writing effective items is only one part of constructing a survey. For one thing, every survey should have a written or spoken introduction that serves two basic functions (Peterson, 2000, as cited by Jhangiani et al. 2012 ). One is to encourage respondents to participate in the survey. In many types of research, such encouragement is not necessary either because participants do not know they are in a study (as in naturalistic observation) or because they are part of a subject pool and have already shown their willingness to participate by signing up and showing up for the study. Survey research usually catches respondents by surprise when they answer their phone, go to their mailbox, or check their e-mail—and the researcher must make a good case for why they should agree to participate. This means that the researcher has only a moment to capture the attention of the respondent and must make it as easy as possible for the respondent to participate . Thus the introduction should briefly explain the purpose of the survey and its importance, provide information about the sponsor of the survey (university-based surveys tend to generate higher response rates), acknowledge the importance of the respondent’s participation, and describe any incentives for participating.
The second function of the introduction is to establish informed consent. Remember that this involves describing to respondents everything that might affect their decision to participate. This includes the topics covered by the survey, the amount of time it is likely to take, the respondent’s option to withdraw at any time, confidentiality issues, and so on. Written consent forms are not always used in survey research (when the research is of minimal risk and completion of the survey instrument is often accepted by the IRB as evidence of consent to participate), so it is important that this part of the introduction be well documented and presented clearly and in its entirety to every respondent.
The introduction should be followed by the substantive questionnaire items. But first, it is important to present clear instructions for completing the questionnaire, including examples of how to use any unusual response scales. Remember that the introduction is the point at which respondents are usually most interested and least fatigued, so it is good practice to start with the most important items for purposes of the research and proceed to less important items. Items should also be grouped by topic or by type. For example, items using the same rating scale (e.g., a 5-point agreement scale) should be grouped together if possible to make things faster and easier for respondents. Demographic items are often presented last because they are least interesting to participants but also easy to answer in the event respondents have become tired or bored. Of course, any survey should end with an expression of appreciation to the respondent.
Coding your survey responses
Once you’ve closed your survey, you’ll need to identify how to quantify the data you’ve collected. Much of this can be done in ways similar to methods described in the previous two chapters. Although there are several ways by which to do this, here are some general tips:
- Transfer data : Transfer your data to a program which will allow you to organize and ‘clean’ the data. If you’ve used an online tool to gather data, you should be able to download the survey results into a format appropriate for working the data. If you’ve collected responses by hand, you’ll need to input the data manually.
- Save: ALWAYS save a copy of your original data. Save changes you make to the data under a different name or version in case you need to refer back to the original data.
- De-identify: This step will depend on the overall approach that you’ve taken to answer your research question and may not be appropriate for your project.
- Name the variables: Again, there is no ‘right’ way to do this; however, as you move forward, you will want to be sure you can easily identify what data you are extracting. Many times, when you transfer your data the program will automatically associate data collected with the question asked. It is a good idea to name the variable something associated with the data, rather than the question
- Code the attributes : Each variable will likely have several different attributes, or layers. You’ll need to come up with a coding method to distinguish the different responses. As discussed in previous chapters, each attribute should have a numeric code associated so that you can quantify the data and use descriptive and/or inferential statistical methods to either describe or explore relationships within the dataset.
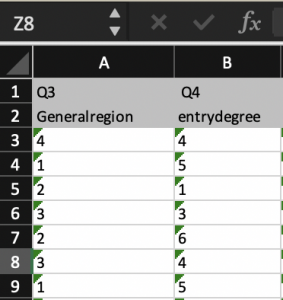
This is relatively simple to accomplish with closed-ended questions. Because you’ve ‘forced’ the respondent to pick a concrete answer, you can create a code that is associated with each answer. In the picture above, respondents were asked to identify their region and given a list of geographical regions and in structed to pick one. The researcher then created a code for the regions. In this case, 1= West; 2= Midwest; 3= Northeast; 4= Southeast; and 5= Southwest. If you’re working to quantify data that is somewhat qualitative in nature (i.e. open ended questions) the process is a little more complicated. You’ll need to either create themes or categories, classify types or similar responses, and then assign codes to those themes or categories.
6. Create a codebook : This.is.essential. Once you begin to code the data, you will have somewhat disconnected yourself from the data by translating the data from a language that we understand to a language which a computer understands. Af ter you run your statistical methods, you’ll translate it back to the native language and share findings. To stay organized and accurate, it is important that you keep a record of how the data has been translated.
7. Analyze: Once you have the data inputted, cleaned, and coded, you should be ready to analyze your data using either descriptive or inferential methods, depend. ing on your approach and overarching goal.
Key Takeaways
- Surveys are a great method to identify information about perceptions and experiences
- Question items must be carefully crafted to elicit an appropriate response
- Surveys are often a mixed-methods approach to research
- Both descriptive and inferential statistical approaches can be applied to the data gleaned through survey responses
- Surveys utilize both open and closed ended questions; identifying which types of questions will yield specific data will be helpful as you plan your approach to analysis
- Most surveys will need to include a method of informed consent, and an introduction. The introduction should clearly delineate the purpose of the survey and how the results will be utilized
- Pilot tests of your survey can save you a lot of time and heartache. Pilot testing helps to catch issues in the development of item, accessibility, and type of information derived prior to initiating the survey on a larger scale
- Survey data can be analyzed much like other types of data; following a systematic approach to coding will help ensure you get the answers you’re looking for
- This section is attributed to Research Methods in Psychology by Rajiv S. Jhangiani, I-Chant A. Chiang, Carrie Cuttler, & Dana C. Leighton is licensed under a Creative Commons Attribution-NonCommercial-ShareAlike 4.0 International License, except where otherwise noted. ↵
- The majority of content in these sections can be attributed to Research Methods in Psychology by Rajiv S. Jhangiani, I-Chant A. Chiang, Carrie Cuttler, & Dana C. Leighton is licensed under a Creative Commons Attribution-NonCommercial-ShareAlike 4.0 International License, except where otherwise noted. ↵
A mixed methods approach using self-reports of respondents who are sampled using stringent methods
A type of survey question that allows the respondent to insert their own response; typically qualitative in nature
A type of survey question which forces a respondent to select a response; no subjectivity.
Practical Research: A Basic Guide to Planning, Doing, and Writing Copyright © by megankoster. All Rights Reserved.
Share This Book
7.1 Overview of Survey Research
Learning objectives.
- Define what survey research is, including its two important characteristics.
- Describe several different ways that survey research can be used and give some examples.
What Is Survey Research?
Survey research is a quantitative and qualitative method with two important characteristics. First, the variables of interest are measured using self-reports (using questionnaires or interviews). In essence, survey researchers ask their participants (who are often called respondents in survey research) to report directly on their own thoughts, feelings, and behaviors. Second, considerable attention is paid to the issue of sampling. In particular, survey researchers have a strong preference for large random samples because they provide the most accurate estimates of what is true in the population. In fact, survey research may be the only approach in psychology in which random sampling is routinely used. Beyond these two characteristics, almost anything goes in survey research. Surveys can be long or short. They can be conducted in person, by telephone, through the mail, or over the Internet. They can be about voting intentions, consumer preferences, social attitudes, health, or anything else that it is possible to ask people about and receive meaningful answers. Although survey data are often analyzed using statistics, there are many questions that lend themselves to more qualitative analysis.
Most survey research is non-experimental. It is used to describe single variables (e.g., the percentage of voters who prefer one presidential candidate or another, the prevalence of schizophrenia in the general population) and also to assess statistical relationships between variables (e.g., the relationship between income and health). But surveys can also be experimental. The study by Lerner and her colleagues is a good example. Their use of self-report measures and a large national sample identifies their work as survey research. But their manipulation of an independent variable (anger vs. fear) to assess its effect on a dependent variable (risk judgments) also identifies their work as experimental.
History and Uses of Survey Research
Survey research may have its roots in English and American “social surveys” conducted around the turn of the 20th century by researchers and reformers who wanted to document the extent of social problems such as poverty (Converse, 1987) [1] . By the 1930s, the US government was conducting surveys to document economic and social conditions in the country. The need to draw conclusions about the entire population helped spur advances in sampling procedures. At about the same time, several researchers who had already made a name for themselves in market research, studying consumer preferences for American businesses, turned their attention to election polling. A watershed event was the presidential election of 1936 between Alf Landon and Franklin Roosevelt. A magazine called Literary Digest conducted a survey by sending ballots (which were also subscription requests) to millions of Americans. Based on this “straw poll,” the editors predicted that Landon would win in a landslide. At the same time, the new pollsters were using scientific methods with much smaller samples to predict just the opposite—that Roosevelt would win in a landslide. In fact, one of them, George Gallup, publicly criticized the methods of Literary Digest before the election and all but guaranteed that his prediction would be correct. And of course, it was. (We will consider the reasons that Gallup was right later in this chapter.) Interest in surveying around election times has led to several long-term projects, notably the Canadian Election Studies which has measured opinions of Canadian voters around federal elections since 1965. Anyone can access the data and read about the results of the experiments in these studies (see http://ces-eec.arts.ubc.ca/ )
From market research and election polling, survey research made its way into several academic fields, including political science, sociology, and public health—where it continues to be one of the primary approaches to collecting new data. Beginning in the 1930s, psychologists made important advances in questionnaire design, including techniques that are still used today, such as the Likert scale. (See “What Is a Likert Scale?” in Section 7.2 “Constructing Survey Questionnaires” .) Survey research has a strong historical association with the social psychological study of attitudes, stereotypes, and prejudice. Early attitude researchers were also among the first psychologists to seek larger and more diverse samples than the convenience samples of university students that were routinely used in psychology (and still are).
Survey research continues to be important in psychology today. For example, survey data have been instrumental in estimating the prevalence of various mental disorders and identifying statistical relationships among those disorders and with various other factors. The National Comorbidity Survey is a large-scale mental health survey conducted in the United States (see http://www.hcp.med.harvard.edu/ncs ). In just one part of this survey, nearly 10,000 adults were given a structured mental health interview in their homes in 2002 and 2003. Table 7.1 presents results on the lifetime prevalence of some anxiety, mood, and substance use disorders. (Lifetime prevalence is the percentage of the population that develops the problem sometime in their lifetime.) Obviously, this kind of information can be of great use both to basic researchers seeking to understand the causes and correlates of mental disorders as well as to clinicians and policymakers who need to understand exactly how common these disorders are.
Generalized anxiety disorder | 5.7 | 7.1 | 4.2 |
Obsessive-compulsive disorder | 2.3 | 3.1 | 1.6 |
Major depressive disorder | 16.9 | 20.2 | 13.2 |
Bipolar disorder | 4.4 | 4.5 | 4.3 |
Alcohol abuse | 13.2 | 7.5 | 19.6 |
Drug abuse | 8.0 | 4.8 | 11.6 |
And as the opening example makes clear, survey research can even be used to conduct experiments to test specific hypotheses about causal relationships between variables. Such studies, when conducted on large and diverse samples, can be a useful supplement to laboratory studies conducted on university students. Although this approach is not a typical use of survey research, it certainly illustrates the flexibility of this method.
Key Takeaways
- Survey research features the use of self-report measures on carefully selected samples. It is a flexible approach that can be used to study a wide variety of basic and applied research questions.
- Survey research has its roots in applied social research, market research, and election polling. It has since become an important approach in many academic disciplines, including political science, sociology, public health, and, of course, psychology.
- a social psychologist
- an educational researcher
- a market researcher who works for a supermarket chain
- the mayor of a large city
- the head of a university police force
- Converse, J. M. (1987). Survey research in the United States: Roots and emergence, 1890–1960 . Berkeley, CA: University of California Press. ↵

Share This Book
- Increase Font Size
Last updated 10th July 2024: Online ordering is currently unavailable due to technical issues. We apologise for any delays responding to customers while we resolve this. For further updates please visit our website https://www.cambridge.org/news-and-insights/technical-incident
We use cookies to distinguish you from other users and to provide you with a better experience on our websites. Close this message to accept cookies or find out how to manage your cookie settings .
Login Alert

- > Research Methods in Information
- > Surveys
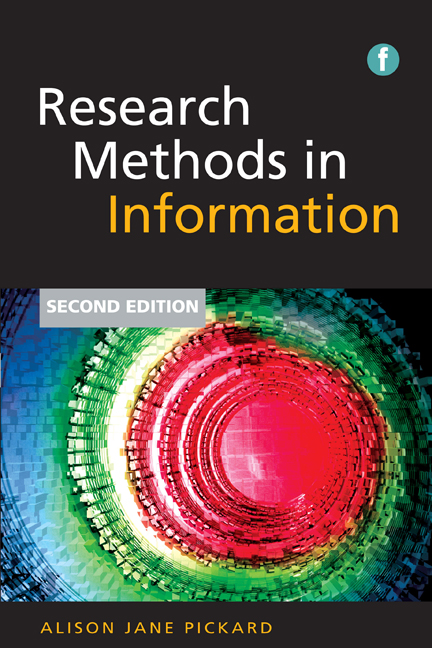
Book contents
- Frontmatter
- Preface to the second edition
- Acknowledgements
Introduction
- Part 1 Starting the research process
- Part 2 Research methods
- 8 Case studies
- 10 Experimental research
- 11 Usability testing
- 12 Ethnography
- 13 Delphi study
- 14 Action research
- 15 Historical research
- 16 Grounded theory: method or analysis?
- Part 3 Data collection techniques
- Part 4 Data analysis and research presentation
- Part 5 Glossary and references
9 - Surveys
from Part 2 - Research methods
Published online by Cambridge University Press: 08 June 2018
The aim of a survey is to obtain information which can be analysed and patterns extracted and comparisons made.
The purpose of survey research is to gather and analyse information by questioning individuals who are either representative of the research population or are the entire research population. The term ‘survey’ usually refers to a study that has used a representative sample; if the entire population is involved in the study it is a ‘census’ . Questions must be asked using a standardized questioning procedure applied equally and consistently to all research participants.
The aim of survey research is to study relationships between specific variables, which are identified at the outset of the research and stated as either a hypothesis or a research question, or to describe certain characteristics of the population. The findings from the survey can then be generalized to the wider population. Survey research can include qualitative and quantitative research, but is usually quantitative with a limited qualitative element, which is more likely to be anecdotal than truly qualitative.
The term ‘survey’ is often used interchangeably with ‘questionnaire’; the two are not the same thing and it can lead to confusion if the distinction between the two is not made very obvious. A survey is a research method, the purpose and aims of which have already been stated; although data collection must be standardized, there are options for data collection within a survey. A questionnaire is a very specific data collection technique, which can be used within a variety of research methods. A survey, then, is the research method used to structure the collection and analysis of standardized information from a defined population using a representative sample of that population. Probability sampling is vital in order to make valid general izations about the wider population. When non-probability sampling is used you must take care with any statements you make that attempt to generalize to the wider population.
There are two types of survey: descriptive surveys and explanatory surveys. It is possible to apply both methods in the same study, as will become apparent when examining the nature of the two approaches.
Access options
Save book to kindle.
To save this book to your Kindle, first ensure [email protected] is added to your Approved Personal Document E-mail List under your Personal Document Settings on the Manage Your Content and Devices page of your Amazon account. Then enter the ‘name’ part of your Kindle email address below. Find out more about saving to your Kindle .
Note you can select to save to either the @free.kindle.com or @kindle.com variations. ‘@free.kindle.com’ emails are free but can only be saved to your device when it is connected to wi-fi. ‘@kindle.com’ emails can be delivered even when you are not connected to wi-fi, but note that service fees apply.
Find out more about the Kindle Personal Document Service .
- Alison Jane Pickard
- Book: Research Methods in Information
- Online publication: 08 June 2018
- Chapter DOI: https://doi.org/10.29085/9781783300235.013
Save book to Dropbox
To save content items to your account, please confirm that you agree to abide by our usage policies. If this is the first time you use this feature, you will be asked to authorise Cambridge Core to connect with your account. Find out more about saving content to Dropbox .
Save book to Google Drive
To save content items to your account, please confirm that you agree to abide by our usage policies. If this is the first time you use this feature, you will be asked to authorise Cambridge Core to connect with your account. Find out more about saving content to Google Drive .
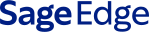
- Request new password
- Create a new account
The Practice of Research in Criminology and Criminal Justice
Student resources, chapter 8: survey research.
1. Identify the circumstances that make survey research an appropriate methodology. 2. List the different methods for improving survey questions, along with the mistakes you do not want to make when writing questions. 3. Discuss the advantages and disadvantages of including don’t know and neutral responses among response choices and of using open-ended questions. 4. Describe the important issues to consider when designing a questionnaire. 5. List the strengths and weaknesses of each mode of survey design, giving particular attention to response rates. 6. Highlight the most common errors related to survey research. 7. Discuss the key ethical issues in survey research.
Root out friction in every digital experience, super-charge conversion rates, and optimize digital self-service
Uncover insights from any interaction, deliver AI-powered agent coaching, and reduce cost to serve
Increase revenue and loyalty with real-time insights and recommendations delivered to teams on the ground
Know how your people feel and empower managers to improve employee engagement, productivity, and retention
Take action in the moments that matter most along the employee journey and drive bottom line growth
Whatever they’re are saying, wherever they’re saying it, know exactly what’s going on with your people
Get faster, richer insights with qual and quant tools that make powerful market research available to everyone
Run concept tests, pricing studies, prototyping + more with fast, powerful studies designed by UX research experts
Track your brand performance 24/7 and act quickly to respond to opportunities and challenges in your market
Explore the platform powering Experience Management
- Free Account
- Product Demos
- For Digital
- For Customer Care
- For Human Resources
- For Researchers
- Financial Services
- All Industries
Popular Use Cases
- Customer Experience
- Employee Experience
- Net Promoter Score
- Voice of Customer
- Customer Success Hub
- Product Documentation
- Training & Certification
- XM Institute
- Popular Resources
- Customer Stories
- Artificial Intelligence
- Market Research
- Partnerships
- Marketplace
The annual gathering of the experience leaders at the world’s iconic brands building breakthrough business results, live in Salt Lake City.
- English/AU & NZ
- Español/Europa
- Español/América Latina
- Português Brasileiro
- REQUEST DEMO
- Experience Management
- What is a survey?
- Survey Research
Try Qualtrics for free
What is survey research.
15 min read Find out everything you need to know about survey research, from what it is and how it works to the different methods and tools you can use to ensure you’re successful.
Survey research is the process of collecting data from a predefined group (e.g. customers or potential customers) with the ultimate goal of uncovering insights about your products, services, or brand overall .
As a quantitative data collection method, survey research can provide you with a goldmine of information that can inform crucial business and product decisions. But survey research needs careful planning and execution to get the results you want.
So if you’re thinking about using surveys to carry out research, read on.
Get started with our free survey maker tool
Types of survey research
Calling these methods ‘survey research’ slightly underplays the complexity of this type of information gathering. From the expertise required to carry out each activity to the analysis of the data and its eventual application, a considerable amount of effort is required.
As for how you can carry out your research, there are several options to choose from — face-to-face interviews, telephone surveys, focus groups (though more interviews than surveys), online surveys , and panel surveys.
Typically, the survey method you choose will largely be guided by who you want to survey, the size of your sample , your budget, and the type of information you’re hoping to gather.
Here are a few of the most-used survey types:
Face-to-face interviews
Before technology made it possible to conduct research using online surveys, telephone, and mail were the most popular methods for survey research. However face-to-face interviews were considered the gold standard — the only reason they weren’t as popular was due to their highly prohibitive costs.
When it came to face-to-face interviews, organizations would use highly trained researchers who knew when to probe or follow up on vague or problematic answers. They also knew when to offer assistance to respondents when they seemed to be struggling. The result was that these interviewers could get sample members to participate and engage in surveys in the most effective way possible, leading to higher response rates and better quality data.
Telephone surveys
While phone surveys have been popular in the past, particularly for measuring general consumer behavior or beliefs, response rates have been declining since the 1990s .
Phone surveys are usually conducted using a random dialing system and software that a researcher can use to record responses.
This method is beneficial when you want to survey a large population but don’t have the resources to conduct face-to-face research surveys or run focus groups, or want to ask multiple-choice and open-ended questions .
The downsides are they can: take a long time to complete depending on the response rate, and you may have to do a lot of cold-calling to get the information you need.
You also run the risk of respondents not being completely honest . Instead, they’ll answer your survey questions quickly just to get off the phone.
Focus groups (interviews — not surveys)
Focus groups are a separate qualitative methodology rather than surveys — even though they’re often bunched together. They’re normally used for survey pretesting and designing , but they’re also a great way to generate opinions and data from a diverse range of people.
Focus groups involve putting a cohort of demographically or socially diverse people in a room with a moderator and engaging them in a discussion on a particular topic, such as your product, brand, or service.
They remain a highly popular method for market research , but they’re expensive and require a lot of administration to conduct and analyze the data properly.
You also run the risk of more dominant members of the group taking over the discussion and swaying the opinions of other people — potentially providing you with unreliable data.
Online surveys
Online surveys have become one of the most popular survey methods due to being cost-effective, enabling researchers to accurately survey a large population quickly.
Online surveys can essentially be used by anyone for any research purpose – we’ve all seen the increasing popularity of polls on social media (although these are not scientific).
Using an online survey allows you to ask a series of different question types and collect data instantly that’s easy to analyze with the right software.
There are also several methods for running and distributing online surveys that allow you to get your questionnaire in front of a large population at a fraction of the cost of face-to-face interviews or focus groups.
This is particularly true when it comes to mobile surveys as most people with a smartphone can access them online.
However, you have to be aware of the potential dangers of using online surveys, particularly when it comes to the survey respondents. The biggest risk is because online surveys require access to a computer or mobile device to complete, they could exclude elderly members of the population who don’t have access to the technology — or don’t know how to use it.
It could also exclude those from poorer socio-economic backgrounds who can’t afford a computer or consistent internet access. This could mean the data collected is more biased towards a certain group and can lead to less accurate data when you’re looking for a representative population sample.
When it comes to surveys, every voice matters.
Find out how to create more inclusive and representative surveys for your research.
Panel surveys
A panel survey involves recruiting respondents who have specifically signed up to answer questionnaires and who are put on a list by a research company. This could be a workforce of a small company or a major subset of a national population. Usually, these groups are carefully selected so that they represent a sample of your target population — giving you balance across criteria such as age, gender, background, and so on.
Panel surveys give you access to the respondents you need and are usually provided by the research company in question. As a result, it’s much easier to get access to the right audiences as you just need to tell the research company your criteria. They’ll then determine the right panels to use to answer your questionnaire.
However, there are downsides. The main one being that if the research company offers its panels incentives, e.g. discounts, coupons, money — respondents may answer a lot of questionnaires just for the benefits.
This might mean they rush through your survey without providing considered and truthful answers. As a consequence, this can damage the credibility of your data and potentially ruin your analyses.
What are the benefits of using survey research?
Depending on the research method you use, there are lots of benefits to conducting survey research for data collection. Here, we cover a few:
1. They’re relatively easy to do
Most research surveys are easy to set up, administer and analyze. As long as the planning and survey design is thorough and you target the right audience , the data collection is usually straightforward regardless of which survey type you use.
2. They can be cost effective
Survey research can be relatively cheap depending on the type of survey you use.
Generally, qualitative research methods that require access to people in person or over the phone are more expensive and require more administration.
Online surveys or mobile surveys are often more cost-effective for market research and can give you access to the global population for a fraction of the cost.
3. You can collect data from a large sample
Again, depending on the type of survey, you can obtain survey results from an entire population at a relatively low price. You can also administer a large variety of survey types to fit the project you’re running.
4. You can use survey software to analyze results immediately
Using survey software, you can use advanced statistical analysis techniques to gain insights into your responses immediately.
Analysis can be conducted using a variety of parameters to determine the validity and reliability of your survey data at scale.
5. Surveys can collect any type of data
While most people view surveys as a quantitative research method, they can just as easily be adapted to gain qualitative information by simply including open-ended questions or conducting interviews face to face.
How to measure concepts with survey questions
While surveys are a great way to obtain data, that data on its own is useless unless it can be analyzed and developed into actionable insights.
The easiest, and most effective way to measure survey results, is to use a dedicated research tool that puts all of your survey results into one place.
When it comes to survey measurement, there are four measurement types to be aware of that will determine how you treat your different survey results:
Nominal scale
With a nominal scale , you can only keep track of how many respondents chose each option from a question, and which response generated the most selections.
An example of this would be simply asking a responder to choose a product or brand from a list.
You could find out which brand was chosen the most but have no insight as to why.
Ordinal scale
Ordinal scales are used to judge an order of preference. They do provide some level of quantitative value because you’re asking responders to choose a preference of one option over another.
Ratio scale
Ratio scales can be used to judge the order and difference between responses. For example, asking respondents how much they spend on their weekly shopping on average.
Interval scale
In an interval scale, values are lined up in order with a meaningful difference between the two values — for example, measuring temperature or measuring a credit score between one value and another.
Step by step: How to conduct surveys and collect data
Conducting a survey and collecting data is relatively straightforward, but it does require some careful planning and design to ensure it results in reliable data.
Step 1 – Define your objectives
What do you want to learn from the survey? How is the data going to help you? Having a hypothesis or series of assumptions about survey responses will allow you to create the right questions to test them.
Step 2 – Create your survey questions
Once you’ve got your hypotheses or assumptions, write out the questions you need answering to test your theories or beliefs. Be wary about framing questions that could lead respondents or inadvertently create biased responses .
Step 3 – Choose your question types
Your survey should include a variety of question types and should aim to obtain quantitative data with some qualitative responses from open-ended questions. Using a mix of questions (simple Yes/ No, multiple-choice, rank in order, etc) not only increases the reliability of your data but also reduces survey fatigue and respondents simply answering questions quickly without thinking.
Find out how to create a survey that’s easy to engage with
Step 4 – Test your questions
Before sending your questionnaire out, you should test it (e.g. have a random internal group do the survey) and carry out A/B tests to ensure you’ll gain accurate responses.
Step 5 – Choose your target and send out the survey
Depending on your objectives, you might want to target the general population with your survey or a specific segment of the population. Once you’ve narrowed down who you want to target, it’s time to send out the survey.
After you’ve deployed the survey, keep an eye on the response rate to ensure you’re getting the number you expected. If your response rate is low, you might need to send the survey out to a second group to obtain a large enough sample — or do some troubleshooting to work out why your response rates are so low. This could be down to your questions, delivery method, selected sample, or otherwise.

Step 6 – Analyze results and draw conclusions
Once you’ve got your results back, it’s time for the fun part.
Break down your survey responses using the parameters you’ve set in your objectives and analyze the data to compare to your original assumptions. At this stage, a research tool or software can make the analysis a lot easier — and that’s somewhere Qualtrics can help.
Get reliable insights with survey software from Qualtrics
Gaining feedback from customers and leads is critical for any business, data gathered from surveys can prove invaluable for understanding your products and your market position, and with survey software from Qualtrics, it couldn’t be easier.
Used by more than 13,000 brands and supporting more than 1 billion surveys a year, Qualtrics empowers everyone in your organization to gather insights and take action. No coding required — and your data is housed in one system.
Get feedback from more than 125 sources on a single platform and view and measure your data in one place to create actionable insights and gain a deeper understanding of your target customers .
Automatically run complex text and statistical analysis to uncover exactly what your survey data is telling you, so you can react in real-time and make smarter decisions.
We can help you with survey management, too. From designing your survey and finding your target respondents to getting your survey in the field and reporting back on the results, we can help you every step of the way.
And for expert market researchers and survey designers, Qualtrics features custom programming to give you total flexibility over question types, survey design, embedded data, and other variables.
No matter what type of survey you want to run, what target audience you want to reach, or what assumptions you want to test or answers you want to uncover, we’ll help you design, deploy and analyze your survey with our team of experts.
Ready to find out more about Qualtrics CoreXM?
Get started with our free survey maker tool today
Related resources
Survey bias types 24 min read, post event survey questions 10 min read, best survey software 16 min read, close-ended questions 7 min read, survey vs questionnaire 12 min read, response bias 13 min read, double barreled question 11 min read, request demo.
Ready to learn more about Qualtrics?

Chapter 3 -- Survey Research Design and Quantitative Methods of Analysis for Cross-sectional Data
Almost everyone has had experience with surveys. Market surveys ask respondents whether they recognize products and their feelings about them. Political polls ask questions about candidates for political office or opinions related to political and social issues. Needs assessments use surveys that identify the needs of groups. Evaluations often use surveys to assess the extent to which programs achieve their goals. Survey research is a method of collecting information by asking questions. Sometimes interviews are done face-to-face with people at home, in school, or at work. Other times questions are sent in the mail for people to answer and mail back. Increasingly, surveys are conducted by telephone. SAMPLE SURVEYS Although we want to have information on all people, it is usually too expensive and time consuming to question everyone. So we select only some of these individuals and question them. It is important to select these people in ways that make it likely that they represent the larger group. The population is all the individuals in whom we are interested. (A population does not always consist of individuals. Sometimes, it may be geographical areas such as all cities with populations of 100,000 or more. Or we may be interested in all households in a particular area. In the data used in the exercises of this module the population consists of individuals who are California residents.) A sample is the subset of the population involved in a study. In other words, a sample is part of the population. The process of selecting the sample is called sampling . The idea of sampling is to select part of the population to represent the entire population. The United States Census is a good example of sampling. The census tries to enumerate all residents every ten years with a short questionnaire. Approximately every fifth household is given a longer questionnaire. Information from this sample (i.e., every fifth household) is used to make inferences about the population. Political polls also use samples. To find out how potential voters feel about a particular race, pollsters select a sample of potential voters. This module uses opinions from three samples of California residents age 18 and over. The data were collected during July, 1985, September, 1991, and February, 1995, by the Field Research Corporation (The Field Institute 1985, 1991, 1995). The Field Research Corporation is a widely-respected survey research firm and is used extensively by the media, politicians, and academic researchers. Since a survey can be no better than the quality of the sample, it is essential to understand the basic principles of sampling. There are two types of sampling-probability and nonprobability. A probability sample is one in which each individual in the population has a known, nonzero chance of being selected in the sample. The most basic type is the simple random sample . In a simple random sample, every individual (and every combination of individuals) has the same chance of being selected in the sample. This is the equivalent of writing each person's name on a piece of paper, putting them in plastic balls, putting all the balls in a big bowl, mixing the balls thoroughly, and selecting some predetermined number of balls from the bowl. This would produce a simple random sample. The simple random sample assumes that we can list all the individuals in the population, but often this is impossible. If our population were all the households or residents of California, there would be no list of the households or residents available, and it would be very expensive and time consuming to construct one. In this type of situation, a multistage cluster sample would be used. The idea is very simple. If we wanted to draw a sample of all residents of California, we might start by dividing California into large geographical areas such as counties and selecting a sample of these counties. Our sample of counties could then be divided into smaller geographical areas such as blocks and a sample of blocks would be selected. We could then construct a list of all households for only those blocks in the sample. Finally, we would go to these households and randomly select one member of each household for our sample. Once the household and the member of that household have been selected, substitution would not be allowed. This often means that we must call back several times, but this is the price we must pay for a good sample. The Field Poll used in this module is a telephone survey. It is a probability sample using a technique called random-digit dialing . With random-digit dialing, phone numbers are dialed randomly within working exchanges (i.e., the first three digits of the telephone number). Numbers are selected in such a way that all areas have the proper proportional chance of being selected in the sample. Random-digit dialing makes it possible to include numbers that are not listed in the telephone directory and households that have moved into an area so recently that they are not included in the current telephone directory. A nonprobability sample is one in which each individual in the population does not have a known chance of selection in the sample. There are several types of nonprobability samples. For example, magazines often include questionnaires for readers to fill out and return. This is a volunteer sample since respondents self-select themselves into the sample (i.e., they volunteer to be in the sample). Another type of nonprobability sample is a quota sample . Survey researchers may assign quotas to interviewers. For example, interviewers might be told that half of their respondents must be female and the other half male. This is a quota on sex. We could also have quotas on several variables (e.g., sex and race) simultaneously. Probability samples are preferable to nonprobability samples. First, they avoid the dangers of what survey researchers call "systematic selection biases" which are inherent in nonprobability samples. For example, in a volunteer sample, particular types of persons might be more likely to volunteer. Perhaps highly-educated individuals are more likely to volunteer to be in the sample and this would produce a systematic selection bias in favor of the highly educated. In a probability sample, the selection of the actual cases in the sample is left to chance. Second, in a probability sample we are able to estimate the amount of sampling error (our next concept to discuss). We would like our sample to give us a perfectly accurate picture of the population. However, this is unrealistic. Assume that the population is all employees of a large corporation, and we want to estimate the percent of employees in the population that is satisfied with their jobs. We select a simple random sample of 500 employees and ask the individuals in the sample how satisfied they are with their jobs. We discover that 75 percent of the employees in our sample are satisfied. Can we assume that 75 percent of the population is satisfied? That would be asking too much. Why would we expect one sample of 500 to give us a perfect representation of the population? We could take several different samples of 500 employees and the percent satisfied from each sample would vary from sample to sample. There will be a certain amount of error as a result of selecting a sample from the population. We refer to this as sampling error . Sampling error can be estimated in a probability sample, but not in a nonprobability sample. It would be wrong to assume that the only reason our sample estimate is different from the true population value is because of sampling error. There are many other sources of error called nonsampling error . Nonsampling error would include such things as the effects of biased questions, the tendency of respondents to systematically underestimate such things as age, the exclusion of certain types of people from the sample (e.g., those without phones, those without permanent addresses), or the tendency of some respondents to systematically agree to statements regardless of the content of the statements. In some studies, the amount of nonsampling error might be far greater than the amount of sampling error. Notice that sampling error is random in nature, while nonsampling error may be nonrandom producing systematic biases. We can estimate the amount of sampling error (assuming probability sampling), but it is much more difficult to estimate nonsampling error. We can never eliminate sampling error entirely, and it is unrealistic to expect that we could ever eliminate nonsampling error. It is good research practice to be diligent in seeking out sources of nonsampling error and trying to minimize them. DATA ANALYSIS Examining Variables One at a Time (Univariate Analysis) The rest of this chapter will deal with the analysis of survey data . Data analysis involves looking at variables or "things" that vary or change. A variable is a characteristic of the individual (assuming we are studying individuals). The answer to each question on the survey forms a variable. For example, sex is a variable-some individuals in the sample are male and some are female. Age is a variable; individuals vary in their ages. Looking at variables one at a time is called univariate analysis . This is the usual starting point in analyzing survey data. There are several reasons to look at variables one at a time. First, we want to describe the data. How many of our sample are men and how many are women? How many are black and how many are white? What is the distribution by age? How many say they are going to vote for Candidate A and how many for Candidate B? How many respondents agree and how many disagree with a statement describing a particular opinion? Another reason we might want to look at variables one at a time involves recoding. Recoding is the process of combining categories within a variable. Consider age, for example. In the data set used in this module, age varies from 18 to 89, but we would want to use fewer categories in our analysis, so we might combine age into age 18 to 29, 30 to 49, and 50 and over. We might want to combine African Americans with the other races to classify race into only two categories-white and nonwhite. Recoding is used to reduce the number of categories in the variable (e.g., age) or to combine categories so that you can make particular types of comparisons (e.g., white versus nonwhite). The frequency distribution is one of the basic tools for looking at variables one at a time. A frequency distribution is the set of categories and the number of cases in each category. Percent distributions show the percentage in each category. Table 3.1 shows frequency and percent distributions for two hypothetical variables-one for sex and one for willingness to vote for a woman candidate. Begin by looking at the frequency distribution for sex. There are three columns in this table. The first column specifies the categories-male and female. The second column tells us how many cases there are in each category, and the third column converts these frequencies into percents. Table 3.1 -- Frequency and Percent Distributions for Sex and Willingness to Vote for a Woman Candidate (Hypothetical Data) Sex Voting Preference Category Freq. Percent Category Freq. Percent Valid Percent Male 380 40.0 Willing to Vote for a Woman 460 48.4 51.1 Female 570 60.0 Not Willing to Vote for a Woman 440 46.3 48.9 Total 950 100.0 Refused 50 5.3 Missing Total 950 100.0 100.0 In this hypothetical example, there are 380 males and 570 females or 40 percent male and 60 percent female. There are a total of 950 cases. Since we know the sex for each case, there are no missing data (i.e., no cases where we do not know the proper category). Look at the frequency distribution for voting preference in Table 3.1. How many say they are willing to vote for a woman candidate and how many are unwilling? (Answer: 460 willing and 440 not willing) How many refused to answer the question? (Answer: 50) What percent say they are willing to vote for a woman, what percent are not, and what percent refused to answer? (Answer: 48.4 percent willing to vote for a woman, 46.3 percent not willing, and 5.3 percent refused to tell us.) The 50 respondents who didn't want to answer the question are called missing data because we don't know which category into which to place them, so we create a new category (i.e., refused) for them. Since we don't know where they should go, we might want a percentage distribution considering only the 900 respondents who answered the question. We can determine this easily by taking the 50 cases with missing information out of the base (i.e., the denominator of the fraction) and recomputing the percentages. The fourth column in the frequency distribution (labeled "valid percent") gives us this information. Approximately 51 percent of those who answered the question were willing to vote for a woman and approximately 49 percent were not. With these data we will use frequency distributions to describe variables one at a time. There are other ways to describe single variables. The mean, median, and mode are averages that may be used to describe the central tendency of a distribution. The range and standard deviation are measures of the amount of variability or dispersion of a distribution. (We will not be using measures of central tendency or variability in this module.) Exploring the Relationship Between Two Variables (Bivariate Analysis) Usually we want to do more than simply describe variables one at a time. We may want to analyze the relationship between variables. Morris Rosenberg (1968:2) suggests that there are three types of relationships: "(1) neither variable may influence one another .... (2) both variables may influence one another ... (3) one of the variables may influence the other." We will focus on the third of these types which Rosenberg calls "asymmetrical relationships." In this type of relationship, one of the variables (the independent variable ) is assumed to be the cause and the other variable (the dependent variable ) is assumed to be the effect. In other words, the independent variable is the factor that influences the dependent variable. For example, researchers think that smoking causes lung cancer. The statement that specifies the relationship between two variables is called a hypothesis (see Hoover 1992, for a more extended discussion of hypotheses). In this hypothesis, the independent variable is smoking (or more precisely, the amount one smokes) and the dependent variable is lung cancer. Consider another example. Political analysts think that income influences voting decisions, that rich people vote differently from poor people. In this hypothesis, income would be the independent variable and voting would be the dependent variable. In order to demonstrate that a causal relationship exists between two variables, we must meet three criteria: (1) there must be a statistical relationship between the two variables, (2) we must be able to demonstrate which one of the variables influences the other, and (3) we must be able to show that there is no other alternative explanation for the relationship. As you can imagine, it is impossible to show that there is no other alternative explanation for a relationship. For this reason, we can show that one variable does not influence another variable, but we cannot prove that it does. We can only show that it is more plausible or credible to believe that a causal relationship exists. In this section, we will focus on the first two criteria and leave this third criterion to the next section. In the previous section we looked at the frequency distributions for sex and voting preference. All we can say from these two distributions is that the sample is 40 percent men and 60 percent women and that slightly more than half of the respondents said they would be willing to vote for a woman, and slightly less than half are not willing to. We cannot say anything about the relationship between sex and voting preference. In order to determine if men or women are more likely to be willing to vote for a woman candidate, we must move from univariate to bivariate analysis. A crosstabulation (or contingency table ) is the basic tool used to explore the relationship between two variables. Table 3.2 is the crosstabulation of sex and voting preference. In the lower right-hand corner is the total number of cases in this table (900). Notice that this is not the number of cases in the sample. There were originally 950 cases in this sample, but any case that had missing information on either or both of the two variables in the table has been excluded from the table. Be sure to check how many cases have been excluded from your table and to indicate this figure in your report. Also be sure that you understand why these cases have been excluded. The figures in the lower margin and right-hand margin of the table are called the marginal distributions. They are simply the frequency distributions for the two variables in the whole table. Here, there are 360 males and 540 females (the marginal distribution for the column variable-sex) and 460 people who are willing to vote for a woman candidate and 440 who are not (the marginal distribution for the row variable-voting preference). The other figures in the table are the cell frequencies. Since there are two columns and two rows in this table (sometimes called a 2 x 2 table), there are four cells. The numbers in these cells tell us how many cases fall into each combination of categories of the two variables. This sounds complicated, but it isn't. For example, 158 males are willing to vote for a woman and 302 females are willing to vote for a woman. Table 3.2 -- Crosstabulation of Sex and Voting Preference (Frequencies) Sex Voting Preference Male Female Total Willing to Vote for a Woman 158 302 460 Not Willing to Vote for a Woman 202 238 440 Total 360 540 900 We could make comparisons rather easily if we had an equal number of women and men. Since these numbers are not equal, we must use percentages to help us make the comparisons. Since percentages convert everything to a common base of 100, the percent distribution shows us what the table would look like if there were an equal number of men and women. Before we percentage Table 3.2, we must decide which of these two variables is the independent and which is the dependent variable. Remember that the independent variable is the variable we think might be the influencing factor. The independent variable is hypothesized to be the cause, and the dependent variable is the effect. Another way to express this is to say that the dependent variable is the one we want to explain. Since we think that sex influences willingness to vote for a woman candidate, sex would be the independent variable. Once we have decided which is the independent variable, we are ready to percentage the table. Notice that percentages can be computed in different ways. In Table 3.3, the percentages have been computed so that they sum down to 100. These are called column percents . If they sum across to 100, they are called row percents . If the independent variable is the column variable, then we want the percents to sum down to 100 (i.e., we want the column percents). If the independent variable is the row variable, we want the percents to sum across to 100 (i.e., we want the row percents). This is a simple, but very important, rule to remember. We'll call this our rule for computing percents . Although we often see the independent variable as the column variable so the table sums down to 100 percent, it really doesn't matter whether the independent variable is the column or the row variable. In this module, we will put the independent variable as the column variable. Many others (but not everyone) use this convention. It would be helpful if you did this when you write your report. Table 3.3 -- Voting Preference by Sex (Percents) Voting Preference Male Female Total Willing to Vote for a Woman 43.9 55.9 51.1 Not Willing to Vote for a Woman 56.1 44.1 100.0 Total Percent 100.0 100.0 100.0 (Total Frequency) (360) (540) (900) Now we are ready to interpret this table. Interpreting a table means to explain what the table is saying about the relationship between the two variables. First, we can look at each category of the independent variable separately to describe the data and then we compare them to each other. Since the percents sum down to 100 percent, we describe down and compare across. The rule for interpreting percents is to compare in the direction opposite to the way the percents sum to 100. So, if the percents sum down to 100, we compare across, and if the percents sum across to 100, compare down. If the independent variable is the column variable, the percents will always sum down to 100. We can look at each category of the independent variable separately to describe the data and then compare them to each other-describe down and then compare across. In Table 3.3, row one shows the percent of males and the percent of females who are willing to vote for a woman candidate--43.9 percent of males are willing to vote for a woman, while 55.9 percent of the females are. This is a difference of 12 percentage points. Somewhat more females than males are willing to vote for a woman. The second row shows the percent of males and females who are not willing to vote for a woman. Since there are only two rows, the second row will be the complement (or the reverse) of the first row. It shows that males are somewhat more likely to be unwilling to vote for a woman candidate (a difference of 12 percentage points in the opposite direction). When we observe a difference, we must also decide whether it is significant. There are two different meanings for significance-statistical significance and substantive significance. Statistical significance considers whether the difference is great enough that it is probably not due to chance factors. Substantive significance considers whether a difference is large enough to be important. With a very large sample, a very small difference is often statistically significant, but that difference may be so small that we decide it isn't substantively significant (i.e., it's so small that we decide it doesn't mean very much). We're going to focus on statistical significance, but remember that even if a difference is statistically significant, you must also decide if it is substantively significant. Let's discuss this idea of statistical significance. If our population is all men and women of voting age in California, we want to know if there is a relationship between sex and voting preference in the population of all individuals of voting age in California. All we have is information about a sample from the population. We use the sample information to make an inference about the population. This is called statistical inference . We know that our sample is not a perfect representation of our population because of sampling error . Therefore, we would not expect the relationship we see in our sample to be exactly the same as the relationship in the population. Suppose we want to know whether there is a relationship between sex and voting preference in the population. It is impossible to prove this directly, so we have to demonstrate it indirectly. We set up a hypothesis (called the null hypothesis ) that says that sex and voting preference are not related to each other in the population. This basically says that any difference we see is likely to be the result of random variation. If the difference is large enough that it is not likely to be due to chance, we can reject this null hypothesis of only random differences. Then the hypothesis that they are related (called the alternative or research hypothesis ) will be more credible.
- f ) | - f ) | - f ) /f | ||
12.52 = chi square |
In the first column of Table 3.4, we have listed the four cell frequencies from the crosstabulation of sex and voting preference. We'll call these the observed frequencies (f o ) because they are what we observe from our table. In the second column, we have listed the frequencies we would expect if, in fact, there is no relationship between sex and voting preference in the population. These are called the expected frequencies (f e ). We'll briefly explain how these expected frequencies are obtained. Notice from Table 3.1 that 51.1 percent of the sample were willing to vote for a woman candidate, while 48.9 percent were not. If sex and voting preference are independent (i.e., not related), we should find the same percentages for males and females. In other words, 48.9 percent (or 176) of the males and 48.9 percent (or 264) of the females would be unwilling to vote for a woman candidate. (This explanation is adapted from Norusis 1997.) Now, we want to compare these two sets of frequencies to see if the observed frequencies are really like the expected frequencies. All we do is to subtract the expected from the observed frequencies (column three). We are interested in the sum of these differences for all cells in the table. Since they always sum to zero, we square the differences (column four) to get positive numbers. Finally, we divide this squared difference by the expected frequency (column five). (Don't worry about why we do this. The reasons are technical and don't add to your understanding.) The sum of column five (12.52) is called the chi square statistic . If the observed and the expected frequencies are identical (no difference), chi square will be zero. The greater the difference between the observed and expected frequencies, the larger the chi square. If we get a large chi square, we are willing to reject the null hypothesis. How large does the chi square have to be? We reject the null hypothesis of no relationship between the two variables when the probability of getting a chi square this large or larger by chance is so small that the null hypothesis is very unlikely to be true. That is, if a chi square this large would rarely occur by chance (usually less than once in a hundred or less than five times in a hundred). In this example, the probability of getting a chi square as large as 12.52 or larger by chance is less than one in a thousand. This is so unlikely that we reject the null hypothesis, and we conclude that the alternative hypothesis (i.e., there is a relationship between sex and voting preference) is credible (not that it is necessarily true, but that it is credible). There is always a small chance that the null hypothesis is true even when we decide to reject it. In other words, we can never be sure that it is false. We can only conclude that there is little chance that it is true. Just because we have concluded that there is a relationship between sex and voting preference does not mean that it is a strong relationship. It might be a moderate or even a weak relationship. There are many statistics that measure the strength of the relationship between two variables. Chi square is not a measure of the strength of the relationship. It just helps us decide if there is a basis for saying a relationship exists regardless of its strength. Measures of association estimate the strength of the relationship and are often used with chi square. (See Appendix D for a discussion of how to compute the two measures of association discussed below.) Cramer's V is a measure of association appropriate when one or both of the variables consists of unordered categories. For example, race (white, African American, other) or religion (Protestant, Catholic, Jewish, other, none) are variables with unordered categories. Cramer's V is a measure based on chi square. It ranges from zero to one. The closer to zero, the weaker the relationship; the closer to one, the stronger the relationship. Gamma (sometimes referred to as Goodman and Kruskal's Gamma) is a measure of association appropriate when both of the variables consist of ordered categories. For example, if respondents answer that they strongly agree, agree, disagree, or strongly disagree with a statement, their responses are ordered. Similarly, if we group age into categories such as under 30, 30 to 49, and 50 and over, these categories would be ordered. Ordered categories can logically be arranged in only two ways-low to high or high to low. Gamma ranges from zero to one, but can be positive or negative. For this module, the sign of Gamma would have no meaning, so ignore the sign and focus on the numerical value. Like V, the closer to zero, the weaker the relationship and the closer to one, the stronger the relationship. Choosing whether to use Cramer's V or Gamma depends on whether the categories of the variable are ordered or unordered. However, dichotomies (variables consisting of only two categories) may be treated as if they are ordered even if they are not. For example, sex is a dichotomy consisting of the categories male and female. There are only two possible ways to order sex-male, female and female, male. Or, race may be classified into two categories-white and nonwhite. We can treat dichotomies as if they consisted of ordered categories because they can be ordered in only two ways. In other words, when one of the variables is a dichotomy, treat this variable as if it were ordinal and use gamma. This is important when choosing an appropriate measure of association. In this chapter we have described how surveys are done and how we analyze the relationship between two variables. In the next chapter we will explore how to introduce additional variables into the analysis. REFERENCES AND SUGGESTED READING Methods of Social Research Riley, Matilda White. 1963. Sociological Research I: A Case Approach . New York: Harcourt, Brace and World. Hoover, Kenneth R. 1992. The Elements of Social Scientific Thinking (5 th Ed.). New York: St. Martin's. Interviewing Gorden, Raymond L. 1987. Interviewing: Strategy, Techniques and Tactics . Chicago: Dorsey. Survey Research and Sampling Babbie, Earl R. 1990. Survey Research Methods (2 nd Ed.). Belmont, CA: Wadsworth. Babbie, Earl R. 1997. The Practice of Social Research (8 th Ed). Belmont, CA: Wadsworth. Statistical Analysis Knoke, David, and George W. Bohrnstedt. 1991. Basic Social Statistics . Itesche, IL: Peacock. Riley, Matilda White. 1963. Sociological Research II Exercises and Manual . New York: Harcourt, Brace & World. Norusis, Marija J. 1997. SPSS 7.5 Guide to Data Analysis . Upper Saddle River, New Jersey: Prentice Hall. Data Sources The Field Institute. 1985. California Field Poll Study, July, 1985 . Machine-readable codebook. The Field Institute. 1991. California Field Poll Study, September, 1991 . Machine-readable codebook. The Field Institute. 1995. California Field Poll Study, February, 1995 . Machine-readable codebook.
Document Viewers
- Free PDF Viewer
- Free Word Viewer
- Free Excel Viewer
- Free PowerPoint Viewer

Principles of Sociological Inquiry
Chapter 8: survey research: a quantitative technique, chapter 8 survey research: a quantitative technique, why survey research.
In 2008, the voters of the United States elected our first African American president, Barack Obama. It may not surprise you to learn that when President Obama was coming of age in the 1970s, one-quarter of Americans reported that they would not vote for a qualified African American presidential nominee. Three decades later, when President Obama ran for the presidency, fewer than 8% of Americans still held that position, and President Obama won the election (Smith, 2009). Smith, T. W. (2009). Trends in willingness to vote for a black and woman for president, 1972–2008. GSS Social Change Report No. 55 . Chicago, IL: National Opinion Research Center. We know about these trends in voter opinion because the General Social Survey ( http://www.norc.uchicago.edu/GSS+Website ), a nationally representative survey of American adults, included questions about race and voting over the years described here. Without survey research, we may not know how Americans’ perspectives on race and the presidency shifted over these years.
8.1 Survey Research: What Is It and When Should It Be Used?
Learning objectives.
- Define survey research.
- Identify when it is appropriate to employ survey research as a data-collection strategy.
Most of you have probably taken a survey at one time or another, so you probably have a pretty good idea of what a survey is. Sometimes students in my research methods classes feel that understanding what a survey is and how to write one is so obvious, there’s no need to dedicate any class time to learning about it. This feeling is understandable—surveys are very much a part of our everyday lives—we’ve probably all taken one, we hear about their results in the news, and perhaps we’ve even administered one ourselves. What students quickly learn is that there is more to constructing a good survey than meets the eye. Survey design takes a great deal of thoughtful planning and often a great many rounds of revision. But it is worth the effort. As we’ll learn in this chapter, there are many benefits to choosing survey research as one’s method of data collection. We’ll take a look at what a survey is exactly, what some of the benefits and drawbacks of this method are, how to construct a survey, and what to do with survey data once one has it in hand.
Survey research A quantitative method for which a researcher poses the same set of questions, typically in a written format, to a sample of individuals. is a quantitative method whereby a researcher poses some set of predetermined questions to an entire group, or sample, of individuals. Survey research is an especially useful approach when a researcher aims to describe or explain features of a very large group or groups. This method may also be used as a way of quickly gaining some general details about one’s population of interest to help prepare for a more focused, in-depth study using time-intensive methods such as in-depth interviews or field research. In this case, a survey may help a researcher identify specific individuals or locations from which to collect additional data.
As is true of all methods of data collection, survey research is better suited to answering some kinds of research question more than others. In addition, as you’ll recall from Chapter 6 “Defining and Measuring Concepts” , operationalization works differently with different research methods. If your interest is in political activism, for example, you likely operationalize that concept differently in a survey than you would for a field research study of the same topic.
Key Takeaway
- Survey research is often used by researchers who wish to explain trends or features of large groups. It may also be used to assist those planning some more focused, in-depth study.
- Recall some of the possible research questions you came up with while reading previous chapters of this text. How might you frame those questions so that they could be answered using survey research?
8.2 Pros and Cons of Survey Research
- Identify and explain the strengths of survey research.
- Identify and explain the weaknesses of survey research.
Survey research, as with all methods of data collection, comes with both strengths and weaknesses. We’ll examine both in this section.
Strengths of Survey Method
Researchers employing survey methods to collect data enjoy a number of benefits. First, surveys are an excellent way to gather lots of information from many people. In my own study of older people’s experiences in the workplace, I was able to mail a written questionnaire to around 500 people who lived throughout the state of Maine at a cost of just over $1,000. This cost included printing copies of my seven-page survey, printing a cover letter, addressing and stuffing envelopes, mailing the survey, and buying return postage for the survey. I realize that $1,000 is nothing to sneeze at. But just imagine what it might have cost to visit each of those people individually to interview them in person. Consider the cost of gas to drive around the state, other travel costs, such as meals and lodging while on the road, and the cost of time to drive to and talk with each person individually. We could double, triple, or even quadruple our costs pretty quickly by opting for an in-person method of data collection over a mailed survey. Thus surveys are relatively cost effective .
Related to the benefit of cost effectiveness is a survey’s potential for generalizability . Because surveys allow researchers to collect data from very large samples for a relatively low cost, survey methods lend themselves to probability sampling techniques, which we discussed in Chapter 7 “Sampling” . Of all the data-collection methods described in this text, survey research is probably the best method to use when one hopes to gain a representative picture of the attitudes and characteristics of a large group.
Survey research also tends to be a reliable method of inquiry. This is because surveys are standardized The same questions, phrased in the same way, are posed to all participants, consistent. in that the same questions, phrased in exactly the same way, are posed to participants. Other methods, such as qualitative interviewing, which we’ll learn about in Chapter 9 “Interviews: Qualitative and Quantitative Approaches” , do not offer the same consistency that a quantitative survey offers. This is not to say that all surveys are always reliable. A poorly phrased question can cause respondents to interpret its meaning differently, which can reduce that question’s reliability. Assuming well-constructed question and questionnaire design, one strength of survey methodology is its potential to produce reliable results.
The versatility A feature of survey research meaning that many different people use surveys for a variety of purposes and in a variety of settings. of survey research is also an asset. Surveys are used by all kinds of people in all kinds of professions. I repeat, surveys are used by all kinds of people in all kinds of professions. Is there a light bulb switching on in your head? I hope so. The versatility offered by survey research means that understanding how to construct and administer surveys is a useful skill to have for all kinds of jobs. Lawyers might use surveys in their efforts to select juries, social service and other organizations (e.g., churches, clubs, fundraising groups, activist groups) use them to evaluate the effectiveness of their efforts, businesses use them to learn how to market their products, governments use them to understand community opinions and needs, and politicians and media outlets use surveys to understand their constituencies.
In sum, the following are benefits of survey research:
- Cost-effective
- Generalizable
Weaknesses of Survey Method
As with all methods of data collection, survey research also comes with a few drawbacks. First, while one might argue that surveys are flexible in the sense that we can ask any number of questions on any number of topics in them, the fact that the survey researcher is generally stuck with a single instrument for collecting data (the questionnaire), surveys are in many ways rather inflexible . Let’s say you mail a survey out to 1,000 people and then discover, as responses start coming in, that your phrasing on a particular question seems to be confusing a number of respondents. At this stage, it’s too late for a do-over or to change the question for the respondents who haven’t yet returned their surveys. When conducting in-depth interviews, on the other hand, a researcher can provide respondents further explanation if they’re confused by a question and can tweak their questions as they learn more about how respondents seem to understand them.
Validity can also be a problem with surveys. Survey questions are standardized; thus it can be difficult to ask anything other than very general questions that a broad range of people will understand. Because of this, survey results may not be as valid as results obtained using methods of data collection that allow a researcher to more comprehensively examine whatever topic is being studied. Let’s say, for example, that you want to learn something about voters’ willingness to elect an African American president, as in our opening example in this chapter. General Social Survey respondents were asked, “If your party nominated an African American for president, would you vote for him if he were qualified for the job?” Respondents were then asked to respond either yes or no to the question. But what if someone’s opinion was more complex than could be answered with a simple yes or no? What if, for example, a person was willing to vote for an African American woman but not an African American man? I am not at all suggesting that such a perspective makes any sense, but it is conceivable that an individual might hold such a perspective.
In sum, potential drawbacks to survey research include the following:
- Inflexibility
Key Takeaways
- Strengths of survey research include its cost effectiveness, generalizability, reliability, and versatility.
- Weaknesses of survey research include inflexibility and issues with validity.
- What are some ways that survey researchers might overcome the weaknesses of this method?
- Find an article reporting results from survey research (remember how to use Sociological Abstracts?). How do the authors describe the strengths and weaknesses of their study? Are any of the strengths or weaknesses described here mentioned in the article?
8.3 Types of Surveys
- Define cross-sectional surveys, provide an example of a cross-sectional survey, and outline some of the drawbacks of cross-sectional research.
- Describe the various types of longitudinal surveys.
- Define retrospective surveys, and identify their strengths and weaknesses.
- Discuss some of the benefits and drawbacks of the various methods of delivering self-administered questionnaires.
There is much variety when it comes to surveys. This variety comes both in terms of time —when or with what frequency a survey is administered—and in terms of administration —how a survey is delivered to respondents. In this section we’ll take a look at what types of surveys exist when it comes to both time and administration.
In terms of time, there are two main types of surveys: cross-sectional and longitudinal. Cross-sectional surveys Surveys that are administered at one point in time. are those that are administered at just one point in time. These surveys offer researchers a sort of snapshot in time and give us an idea about how things are for our respondents at the particular point in time that the survey is administered. My own study of older workers mentioned previously is an example of a cross-sectional survey. I administered the survey at just one time.
Another example of a cross-sectional survey comes from Aniko Kezdy and colleagues’ study (Kezdy, Martos, Boland, & Horvath-Szabo, 2011) Kezdy, A., Martos, T., Boland, V., & Horvath-Szabo, K. (2011). Religious doubts and mental health in adolescence and young adulthood: The association with religious attitudes. Journal of Adolescence, 34 , 39–47. of the association between religious attitudes, religious beliefs, and mental health among students in Hungary. These researchers administered a single, one-time-only, cross-sectional survey to a convenience sample of 403 high school and college students. The survey focused on how religious attitudes impact various aspects of one’s life and health. The researchers found from analysis of their cross-sectional data that anxiety and depression were highest among those who had both strong religious beliefs and also some doubts about religion. Yet another recent example of cross-sectional survey research can be seen in Bateman and colleagues’ study (Bateman, Pike, & Butler, 2011) of how the perceived publicness of social networking sites influences users’ self-disclosures. Bateman, P. J., Pike, J. C., & Butler, B. S. (2011). To disclose or not: Publicness in social networking sites. Information Technology & People, 24 , 78–100. These researchers administered an online survey to undergraduate and graduate business students. They found that even though revealing information about oneself is viewed as key to realizing many of the benefits of social networking sites, respondents were less willing to disclose information about themselves as their perceptions of a social networking site’s publicness rose. That is, there was a negative relationship between perceived publicness of a social networking site and plans to self-disclose on the site.
One problem with cross-sectional surveys is that the events, opinions, behaviors, and other phenomena that such surveys are designed to assess don’t generally remain stagnant. Thus generalizing from a cross-sectional survey about the way things are can be tricky; perhaps you can say something about the way things were in the moment that you administered your survey, but it is difficult to know whether things remained that way for long after you administered your survey. Think, for example, about how Americans might have responded if administered a survey asking for their opinions on terrorism on September 10, 2001. Now imagine how responses to the same set of questions might differ were they administered on September 12, 2001. The point is not that cross-sectional surveys are useless; they have many important uses. But researchers must remember what they have captured by administering a cross-sectional survey; that is, as previously noted, a snapshot of life as it was at the time that the survey was administered.
One way to overcome this sometimes problematic aspect of cross-sectional surveys is to administer a longitudinal survey. Longitudinal surveys Surveys that enable a researcher to make observations over some extended period of time. are those that enable a researcher to make observations over some extended period of time. There are several types of longitudinal surveys, including trend, panel, and cohort surveys. We’ll discuss all three types here, along with another type of survey called retrospective. Retrospective surveys fall somewhere in between cross-sectional and longitudinal surveys.
The first type of longitudinal survey is called a trend survey A type of longitudinal survey where a researcher examines changes in trends over time; the same people do not necessarily participate in the survey more than once. . The main focus of a trend survey is, perhaps not surprisingly, trends. Researchers conducting trend surveys are interested in how people’s inclinations change over time. The Gallup opinion polls are an excellent example of trend surveys. You can read more about Gallup on their website: http://www.gallup.com/Home.aspx . To learn about how public opinion changes over time, Gallup administers the same questions to people at different points in time. For example, for several years Gallup has polled Americans to find out what they think about gas prices (something many of us happen to have opinions about). One thing we’ve learned from Gallup’s polling is that price increases in gasoline caused financial hardship for 67% of respondents in 2011, up from 40% in the year 2000. Gallup’s findings about trends in opinions about gas prices have also taught us that whereas just 34% of people in early 2000 thought the current rise in gas prices was permanent, 54% of people in 2011 believed the rise to be permanent. Thus through Gallup’s use of trend survey methodology, we’ve learned that Americans seem to feel generally less optimistic about the price of gas these days than they did 10 or so years ago. You can read about these and other findings on Gallup’s gasoline questions at http://www.gallup.com/poll/147632/Gas-Prices.aspx#1 . It should be noted that in a trend survey, the same people are probably not answering the researcher’s questions each year. Because the interest here is in trends, not specific people, as long as the researcher’s sample is representative of whatever population he or she wishes to describe trends for, it isn’t important that the same people participate each time.
Next are panel surveys A type of longitudinal survey in which a researcher surveys the exact same sample several times over a period of time. . Unlike in a trend survey, in a panel survey the same people do participate in the survey each time it is administered. As you might imagine, panel studies can be difficult and costly. Imagine trying to administer a survey to the same 100 people every year for, say, 5 years in a row. Keeping track of where people live, when they move, and when they die takes resources that researchers often don’t have. When they do, however, the results can be quite powerful. The Youth Development Study (YDS), administered from the University of Minnesota, offers an excellent example of a panel study. You can read more about the Youth Development Study at its website: http://www.soc.umn.edu/research/yds . Since 1988, YDS researchers have administered an annual survey to the same 1,000 people. Study participants were in ninth grade when the study began, and they are now in their thirties. Several hundred papers, articles, and books have been written using data from the YDS. One of the major lessons learned from this panel study is that work has a largely positive impact on young people (Mortimer, 2003). Mortimer, J. T. (2003). Working and growing up in America . Cambridge, MA: Harvard University Press. Contrary to popular beliefs about the impact of work on adolescents’ performance in school and transition to adulthood, work in fact increases confidence, enhances academic success, and prepares students for success in their future careers. Without this panel study, we may not be aware of the positive impact that working can have on young people.
Another type of longitudinal survey is a cohort survey A type of longitudinal survey where a researcher’s interest is in a particular group of people who share some common experience or characteristic. . In a cohort survey, a researcher identifies some category of people that are of interest and then regularly surveys people who fall into that category. The same people don’t necessarily participate from year to year, but all participants must meet whatever categorical criteria fulfill the researcher’s primary interest. Common cohorts that may be of interest to researchers include people of particular generations or those who were born around the same time period, graduating classes, people who began work in a given industry at the same time, or perhaps people who have some specific life experience in common. An example of this sort of research can be seen in Christine Percheski’s work (2008) Percheski, C. (2008). Opting out? Cohort differences in professional women’s employment rates from 1960 to 2005. American Sociological Review, 73 , 497–517. on cohort differences in women’s employment. Percheski compared women’s employment rates across seven different generational cohorts, from Progressives born between 1906 and 1915 to Generation Xers born between 1966 and 1975. She found, among other patterns, that professional women’s labor force participation had increased across all cohorts. She also found that professional women with young children from Generation X had higher labor force participation rates than similar women from previous generations, concluding that mothers do not appear to be opting out of the workforce as some journalists have speculated (Belkin, 2003). Belkin, L. (2003, October 26). The opt-out revolution. New York Times , pp. 42–47, 58, 85–86.
All three types of longitudinal surveys share the strength that they permit a researcher to make observations over time. This means that if whatever behavior or other phenomenon the researcher is interested in changes, either because of some world event or because people age, the researcher will be able to capture those changes. Table 8.1 “Types of Longitudinal Surveys” summarizes each of the three types of longitudinal surveys.
Table 8.1 Types of Longitudinal Surveys
Sample type | Description |
---|---|
Trend | Researcher examines changes in trends over time; the same people do not necessarily participate in the survey more than once. |
Panel | Researcher surveys the exact same sample several times over a period of time. |
Cohort | Researcher identifies some category of people that are of interest and then regularly surveys people who fall into that category. |
Finally, retrospective surveys A type of survey in which participants are asked to report events from the past. are similar to other longitudinal studies in that they deal with changes over time, but like a cross-sectional study, they are administered only once. In a retrospective survey, participants are asked to report events from the past. By having respondents report past behaviors, beliefs, or experiences, researchers are able to gather longitudinal-like data without actually incurring the time or expense of a longitudinal survey. Of course, this benefit must be weighed against the possibility that people’s recollections of their pasts may be faulty. Imagine, for example, that you’re asked in a survey to respond to questions about where, how, and with whom you spent last Valentine’s Day. As last Valentine’s Day can’t have been more than 12 months ago, chances are good that you might be able to respond accurately to any survey questions about it. But now let’s say the research wants to know how last Valentine’s Day compares to previous Valentine’s Days, so he asks you to report on where, how, and with whom you spent the preceding six Valentine’s Days. How likely is it that you will remember? Will your responses be as accurate as they might have been had you been asked the question each year over the past 6 years rather than asked to report on all years today?
In sum, when or with what frequency a survey is administered will determine whether your survey is cross-sectional or longitudinal. While longitudinal surveys are certainly preferable in terms of their ability to track changes over time, the time and cost required to administer a longitudinal survey can be prohibitive. As you may have guessed, the issues of time described here are not necessarily unique to survey research. Other methods of data collection can be cross-sectional or longitudinal—these are really matters of research design. But we’ve placed our discussion of these terms here because they are most commonly used by survey researchers to describe the type of survey administered. Another aspect of survey administration deals with how surveys are administered. We’ll examine that next.
Administration
Surveys vary not just in terms of when they are administered but also in terms of how they are administered. One common way to administer surveys is in the form of self-administered questionnaires A set of written questions that a research participant responds to by filling in answers on her or his own without the assistance of a researcher. . This means that a research participant is given a set of questions, in writing, to which he or she is asked to respond. Self-administered questionnaires can be delivered in hard copy format, typically via mail, or increasingly more commonly, online. We’ll consider both modes of delivery here.
Hard copy self-administered questionnaires may be delivered to participants in person or via snail mail. Perhaps you’ve take a survey that was given to you in person; on many college campuses it is not uncommon for researchers to administer surveys in large social science classes (as you might recall from the discussion in our chapter on sampling). In my own introduction to sociology courses, I’ve welcomed graduate students and professors doing research in areas that are relevant to my students, such as studies of campus life, to administer their surveys to the class. If you are ever asked to complete a survey in a similar setting, it might be interesting to note how your perspective on the survey and its questions could be shaped by the new knowledge you’re gaining about survey research in this chapter.
Researchers may also deliver surveys in person by going door-to-door and either asking people to fill them out right away or making arrangements for the researcher to return to pick up completed surveys. Though the advent of online survey tools has made door-to-door delivery of surveys less common, I still see an occasional survey researcher at my door, especially around election time. This mode of gathering data is apparently still used by political campaign workers, at least in some areas of the country.
If you are not able to visit each member of your sample personally to deliver a survey, you might consider sending your survey through the mail. While this mode of delivery may not be ideal (imagine how much less likely you’d probably be to return a survey that didn’t come with the researcher standing on your doorstep waiting to take it from you), sometimes it is the only available or the most practical option. As I’ve said, this may not be the most ideal way of administering a survey because it can be difficult to convince people to take the time to complete and return your survey.
Often survey researchers who deliver their surveys via snail mail may provide some advance notice to respondents about the survey to get people thinking about and preparing to complete it. They may also follow up with their sample a few weeks after their survey has been sent out. This can be done not only to remind those who have not yet completed the survey to please do so but also to thank those who have already returned the survey. Most survey researchers agree that this sort of follow-up is essential for improving mailed surveys’ return rates (Babbie, 2010). Babbie, E. (2010). The practice of social research (12th ed.). Belmont, CA: Wadsworth.
In my own study of older workers’ harassment experiences, people in the sample were notified in advance of the survey mailing via an article describing the research in a newsletter they received from the agency with whom I had partnered to conduct the survey. When I mailed the survey, a $1 bill was included with each in order to provide some incentive and an advance token of thanks to participants for returning the surveys. Two months after the initial mailing went out, those who were sent a survey were contacted by phone. While returned surveys did not contain any identifying information about respondents, my research assistants contacted individuals to whom a survey had been mailed to remind them that it was not too late to return their survey and to say thank to those who may have already done so. Four months after the initial mailing went out, everyone on the original mailing list received a letter thanking those who had returned the survey and once again reminding those who had not that it was not too late to do so. The letter included a return postcard for respondents to complete should they wish to receive another copy of the survey. Respondents were also provided a telephone number to call and were provided the option of completing the survey by phone. As you can see, administering a survey by mail typically involves much more than simply arranging a single mailing; participants may be notified in advance of the mailing, they then receive the mailing, and then several follow-up contacts will likely be made after the survey has been mailed.
Earlier I mentioned online delivery as another way to administer a survey. This delivery mechanism is becoming increasingly common, no doubt because it is easy to use, relatively cheap, and may be quicker than knocking on doors or waiting for mailed surveys to be returned. To deliver a survey online, a researcher may subscribe to a service that offers online delivery or use some delivery mechanism that is available for free. SurveyMonkey offers both free and paid online survey services ( http://www.surveymonkey.com ). One advantage to using a service like SurveyMonkey, aside from the advantages of online delivery already mentioned, is that results can be provided to you in formats that are readable by data analysis programs such as SPSS, Systat, and Excel. This saves you, the researcher, the step of having to manually enter data into your analysis program, as you would if you administered your survey in hard copy format.
Many of the suggestions provided for improving the response rate on a hard copy questionnaire apply to online questionnaires as well. One difference of course is that the sort of incentives one can provide in an online format differ from those that can be given in person or sent through the mail. But this doesn’t mean that online survey researchers cannot offer completion incentives to their respondents. I’ve taken a number of online surveys; many of these did not come with an incentive other than the joy of knowing that I’d helped a fellow social scientist do his or her job, but on one I was given a printable $5 coupon to my university’s campus dining services on completion, and another time I was given a coupon code to use for $10 off any order on Amazon.com. I’ve taken other online surveys where on completion I could provide my name and contact information if I wished to be entered into a drawing together with other study participants to win a larger gift, such as a $50 gift card or an iPad.
Sometimes surveys are administered by having a researcher actually pose questions directly to respondents rather than having respondents read the questions on their own. These types of surveys are a form of interviews. We discuss interviews in Chapter 9 “Interviews: Qualitative and Quantitative Approaches” , where we’ll examine interviews of the survey (or quantitative) type and qualitative interviews as well. Interview methodology differs from survey research in that data are collected via a personal interaction. Because asking people questions in person comes with a set of guidelines and concerns that differ from those associated with asking questions on paper or online, we’ll reserve our discussion of those guidelines and concerns for Chapter 9 “Interviews: Qualitative and Quantitative Approaches” .
Whatever delivery mechanism you choose, keep in mind that there are pros and cons to each of the options described here. While online surveys may be faster and cheaper than mailed surveys, can you be certain that every person in your sample will have the necessary computer hardware, software, and Internet access in order to complete your online survey? On the other hand, perhaps mailed surveys are more likely to reach your entire sample but also more likely to be lost and not returned. The choice of which delivery mechanism is best depends on a number of factors including your resources, the resources of your study participants, and the time you have available to distribute surveys and wait for responses. In my own survey of older workers, I would have much preferred to administer my survey online, but because so few people in my sample were likely to have computers, and even fewer would have Internet access, I chose instead to mail paper copies of the survey to respondents’ homes. Understanding the characteristics of your study’s population is key to identifying the appropriate mechanism for delivering your survey.
- Time is a factor in determining what type of survey researcher administers; cross-sectional surveys are administered at one time, and longitudinal surveys are administered over time.
- Retrospective surveys offer some of the benefits of longitudinal research but also come with their own drawbacks.
- Self-administered questionnaires may be delivered in hard copy form to participants in person or via snail mail or online.
- If the idea of a panel study piqued your interest, check out the Up series of documentary films. While not a survey, the films offer one example of a panel study. Filmmakers began filming the lives of 14 British children in 1964, when the children were 7 years old. They have since caught up with the children every 7 years. In 2012, the eighth installment of the documentary, 56 Up , will come out. Many clips from the series are available on YouTube.
- For more information about online delivery of surveys, check out SurveyMonkey’s website: http://www.surveymonkey.com .
8.4 Designing Effective Questions and Questionnaires
- Identify the steps one should take in order to write effective survey questions.
- Describe some of the ways that survey questions might confuse respondents and how to overcome that possibility.
- Recite the two response option guidelines when writing closed-ended questions.
- Define fence-sitting and floating.
- Describe the steps involved in constructing a well-designed questionnaire.
- Discuss why pretesting is important.
To this point we’ve considered several general points about surveys including when to use them, some of their pros and cons, and how often and in what ways to administer surveys. In this section we’ll get more specific and take a look at how to pose understandable questions that will yield useable data and how to present those questions on your questionnaire.
Asking Effective Questions
The first thing you need to do in order to write effective survey questions is identify what exactly it is that you wish to know. As silly as it sounds to state what seems so completely obvious, I can’t stress enough how easy it is to forget to include important questions when designing a survey. Let’s say you want to understand how students at your school made the transition from high school to college. Perhaps you wish to identify which students were comparatively more or less successful in this transition and which factors contributed to students’ success or lack thereof. To understand which factors shaped successful students’ transitions to college, you’ll need to include questions in your survey about all the possible factors that could contribute. Consulting the literature on the topic will certainly help, but you should also take the time to do some brainstorming on your own and to talk with others about what they think may be important in the transition to college. Perhaps time or space limitations won’t allow you to include every single item you’ve come up with, so you’ll also need to think about ranking your questions so that you can be sure to include those that you view as most important.
Although I have stressed the importance of including questions on all topics you view as important to your overall research question, you don’t want to take an everything-but-the-kitchen-sink approach by uncritically including every possible question that occurs to you. Doing so puts an unnecessary burden on your survey respondents. Remember that you have asked your respondents to give you their time and attention and to take care in responding to your questions; show them your respect by only asking questions that you view as important.
Once you’ve identified all the topics about which you’d like to ask questions, you’ll need to actually write those questions. Questions should be as clear and to the point as possible. This is not the time to show off your creative writing skills; a survey is a technical instrument and should be written in a way that is as direct and succinct as possible. As I’ve said, your survey respondents have agreed to give their time and attention to your survey. The best way to show your appreciation for their time is to not waste it. Ensuring that your questions are clear and not overly wordy will go a long way toward showing your respondents the gratitude they deserve.
Related to the point about not wasting respondents’ time, make sure that every question you pose will be relevant to every person you ask to complete it. This means two things: first, that respondents have knowledge about whatever topic you are asking them about, and second, that respondents have experience with whatever events, behaviors, or feelings you are asking them to report. You probably wouldn’t want to ask a sample of 18-year-old respondents, for example, how they would have advised President Reagan to proceed when news of the United States’ sale of weapons to Iran broke in the mid-1980s. For one thing, few 18-year-olds are likely to have any clue about how to advise a president (nor does this 30-something-year-old). Furthermore, the 18-year-olds of today were not even alive during Reagan’s presidency, so they have had no experience with the event about which they are being questioned. In our example of the transition to college, heeding the criterion of relevance would mean that respondents must understand what exactly you mean by “transition to college” if you are going to use that phrase in your survey and that respondents must have actually experienced the transition to college themselves.
If you decide that you do wish to pose some questions about matters with which only a portion of respondents will have had experience, it may be appropriate to introduce a filter question A question designed to identify some subset of survey respondents who are then asked additional questions that are not relevant to the entire sample. into your survey. A filter question is designed to identify some subset of survey respondents who are asked additional questions that are not relevant to the entire sample. Perhaps in your survey on the transition to college you want to know whether substance use plays any role in students’ transitions. You may ask students how often they drank during their first semester of college. But this assumes that all students drank. Certainly some may have abstained, and it wouldn’t make any sense to ask the nondrinkers how often they drank. Nevertheless, it seems reasonable that drinking frequency may have an impact on someone’s transition to college, so it is probably worth asking this question even if doing so violates the rule of relevance for some respondents. This is just the sort of instance when a filter question would be appropriate. You may pose the question as it is presented in Figure 8.8 “Filter Question” .
Figure 8.8 Filter Question
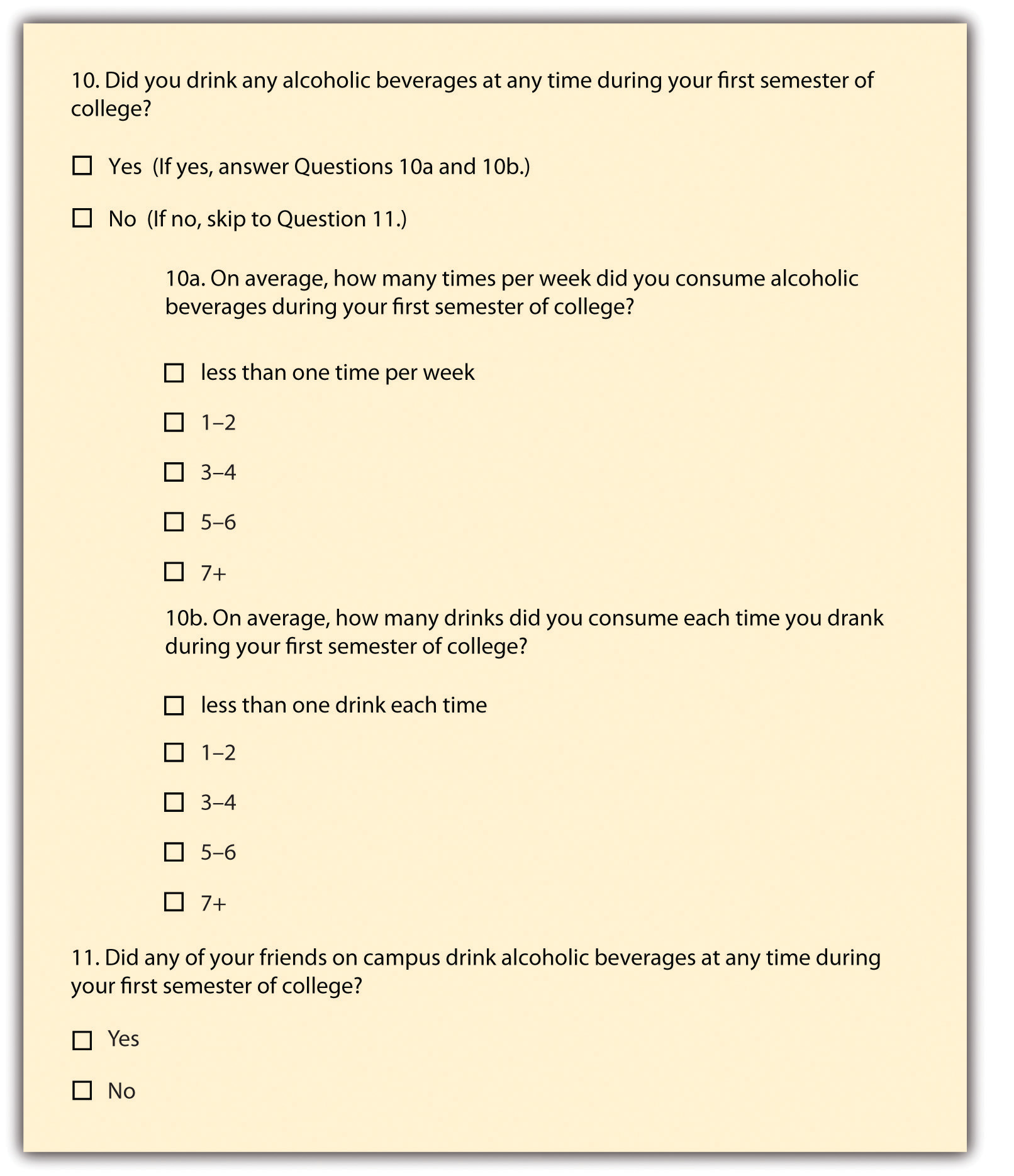
There are some ways of asking questions that are bound to confuse a good many survey respondents. Survey researchers should take great care to avoid these kinds of questions. These include questions that pose double negatives, those that use confusing or culturally specific terms, and those that ask more than one question but are posed as a single question. Any time respondents are forced to decipher questions that utilize two forms of negation, confusion is bound to ensue. Taking the previous question about drinking as our example, what if we had instead asked, “Did you not drink during your first semester of college?” A response of no would mean that the respondent did actually drink—he or she did not not drink. This example is obvious, but hopefully it drives home the point to be careful about question wording so that respondents are not asked to decipher double negatives. In general, avoiding negative terms in your question wording will help to increase respondent understanding. Though this is generally true, some researchers argue that negatively worded questions should be integrated with positively worded questions in order to ensure that respondents have actually carefully read each question. See, for example, the following: Vaterlaus, M., & Higgenbotham, B. (2011). Writing survey questions for local program evaluations. Retrieved from http://extension.usu.edu/files/publications/publication/FC_Evaluation_2011-02pr.pdf
You should also avoid using terms or phrases that may be regionally or culturally specific (unless you are absolutely certain all your respondents come from the region or culture whose terms you are using). When I first moved to Maine from Minnesota, I was totally confused every time I heard someone use the word wicked . This term has totally different meanings across different regions of the country. I’d come from an area that understood the term wicked to be associated with evil. In my new home, however, wicked is used simply to put emphasis on whatever it is that you’re talking about. So if this chapter is extremely interesting to you, if you live in Maine you might say that it is “wicked interesting.” If you hate this chapter and you live in Minnesota, perhaps you’d describe the chapter simply as wicked. I once overheard one student tell another that his new girlfriend was “wicked athletic.” At the time I thought this meant he’d found a woman who used her athleticism for evil purposes. I’ve come to understand, however, that this woman is probably just exceptionally athletic. While wicked may not be a term you’re likely to use in a survey, the point is to be thoughtful and cautious about whatever terminology you do use.
Asking multiple questions as though they are a single question can also be terribly confusing for survey respondents. There’s a specific term for this sort of question; it is called a double-barreled question A question that is posed as a single question but in fact asks more than one question. . Using our example of the transition to college, Figure 8.9 “Double-Barreled Question” shows a double-barreled question.
Figure 8.9 Double-Barreled Question
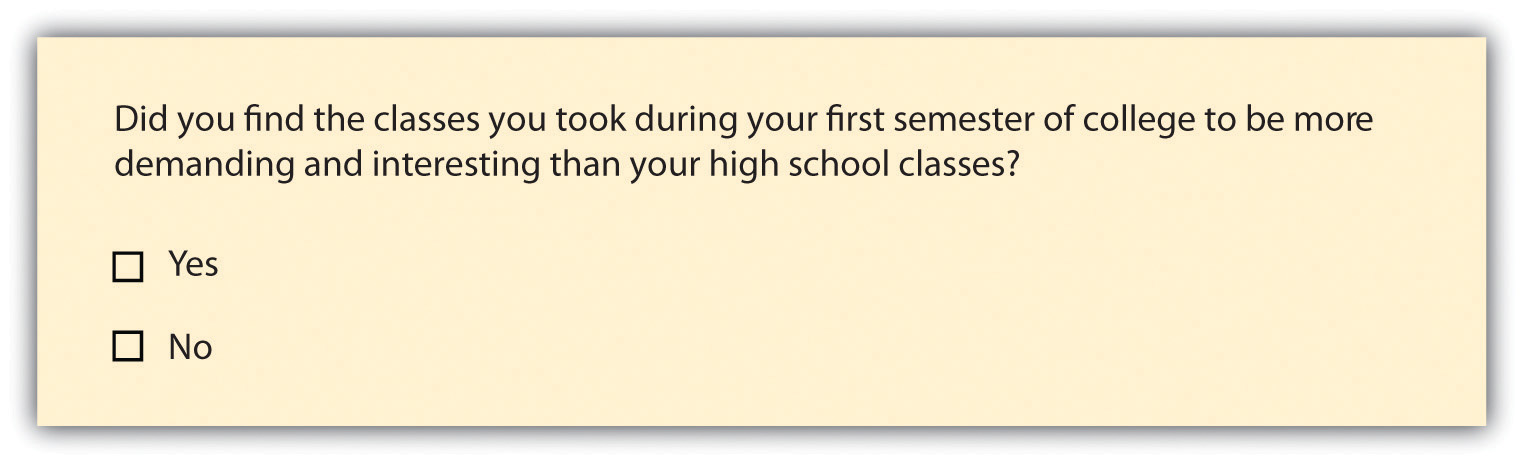
Do you see what makes the question double-barreled? How would someone respond if they felt their college classes were more demanding but also more boring than their high school classes? Or less demanding but more interesting? Because the question combines “demanding” and “interesting,” there is no way to respond yes to one criterion but no to the other.
Another thing to avoid when constructing survey questions is the problem of social desirability The idea that respondents will try to answer questions in a way that will present them in a favorable light. . We all want to look good, right? And we all probably know the politically correct response to a variety of questions whether we agree with the politically correct response or not. In survey research, social desirability refers to the idea that respondents will try to answer questions in a way that will present them in a favorable light. Perhaps we decide that to understand the transition to college, we need to know whether respondents ever cheated on an exam in high school or college. We all know that cheating on exams is generally frowned upon (at least I hope we all know this). So it may be difficult to get people to admit to cheating on a survey. But if you can guarantee respondents’ confidentiality, or even better, their anonymity, chances are much better that they will be honest about having engaged in this socially undesirable behavior. Another way to avoid problems of social desirability is to try to phrase difficult questions in the most benign way possible. Earl Babbie (2010) Babbie, E. (2010). The practice of social research (12th ed.). Belmont, CA: Wadsworth. offers a useful suggestion for helping you do this—simply imagine how you would feel responding to your survey questions. If you would be uncomfortable, chances are others would as well.
Finally, it is important to get feedback on your survey questions from as many people as possible, especially people who are like those in your sample. Now is not the time to be shy. Ask your friends for help, ask your mentors for feedback, ask your family to take a look at your survey as well. The more feedback you can get on your survey questions, the better the chances that you will come up with a set of questions that are understandable to a wide variety of people and, most importantly, to those in your sample.
In sum, in order to pose effective survey questions, researchers should do the following:
- Identify what it is they wish to know.
- Keep questions clear and succinct.
- Make questions relevant to respondents.
- Use filter questions when necessary.
- Avoid questions that are likely to confuse respondents such as those that use double negatives, use culturally specific terms, or pose more than one question in the form of a single question.
- Imagine how they would feel responding to questions.
- Get feedback, especially from people who resemble those in the researcher’s sample.
Response Options
While posing clear and understandable questions in your survey is certainly important, so, too, is providing respondents with unambiguous response options The answers that are provided to for each question in a survey. . Response options are the answers that you provide to the people taking your survey. Generally respondents will be asked to choose a single (or best) response to each question you pose, though certainly it makes sense in some cases to instruct respondents to choose multiple response options. One caution to keep in mind when accepting multiple responses to a single question, however, is that doing so may add complexity when it comes to tallying and analyzing your survey results.
Offering response options assumes that your questions will be closed-ended questions A survey question for which the researcher provides respondents with a limited set of clear response options. . In a quantitative written survey, which is the type of survey we’ve been discussing here, chances are good that most if not all your questions will be closed ended. This means that you, the researcher, will provide respondents with a limited set of options for their responses. To write an effective closed-ended question, there are a couple of guidelines worth following. First, be sure that your response options are mutually exclusive . Look back at Figure 8.8 “Filter Question” , which contains questions about how often and how many drinks respondents consumed. Do you notice that there are no overlapping categories in the response options for these questions? This is another one of those points about question construction that seems fairly obvious but that can be easily overlooked. Response options should also be exhaustive . In other words, every possible response should be covered in the set of response options that you provide. For example, note that in question 10a in Figure 8.8 “Filter Question” we have covered all possibilities—those who drank, say, an average of once per month can choose the first response option (“less than one time per week”) while those who drank multiple times a day each day of the week can choose the last response option (“7+”). All the possibilities in between these two extremes are covered by the middle three response options.
Surveys need not be limited to closed-ended questions. Sometimes survey researchers include open-ended questions A survey question for which the researcher does not provide respondents with response options; instead, respondents answer in their own words. in their survey instruments as a way to gather additional details from respondents. An open-ended question does not include response options; instead, respondents are asked to reply to the question in their own way, using their own words. These questions are generally used to find out more about a survey participant’s experiences or feelings about whatever they are being asked to report in the survey. If, for example, a survey includes closed-ended questions asking respondents to report on their involvement in extracurricular activities during college, an open-ended question could ask respondents why they participated in those activities or what they gained from their participation. While responses to such questions may also be captured using a closed-ended format, allowing participants to share some of their responses in their own words can make the experience of completing the survey more satisfying to respondents and can also reveal new motivations or explanations that had not occurred to the researcher.
In Section 8.4.1 “Asking Effective Questions” we discussed double-barreled questions, but response options can also be double barreled, and this should be avoided. Figure 8.10 “Double-Barreled Response Options” is an example of a question that uses double-barreled response options.
Figure 8.10 Double-Barreled Response Options
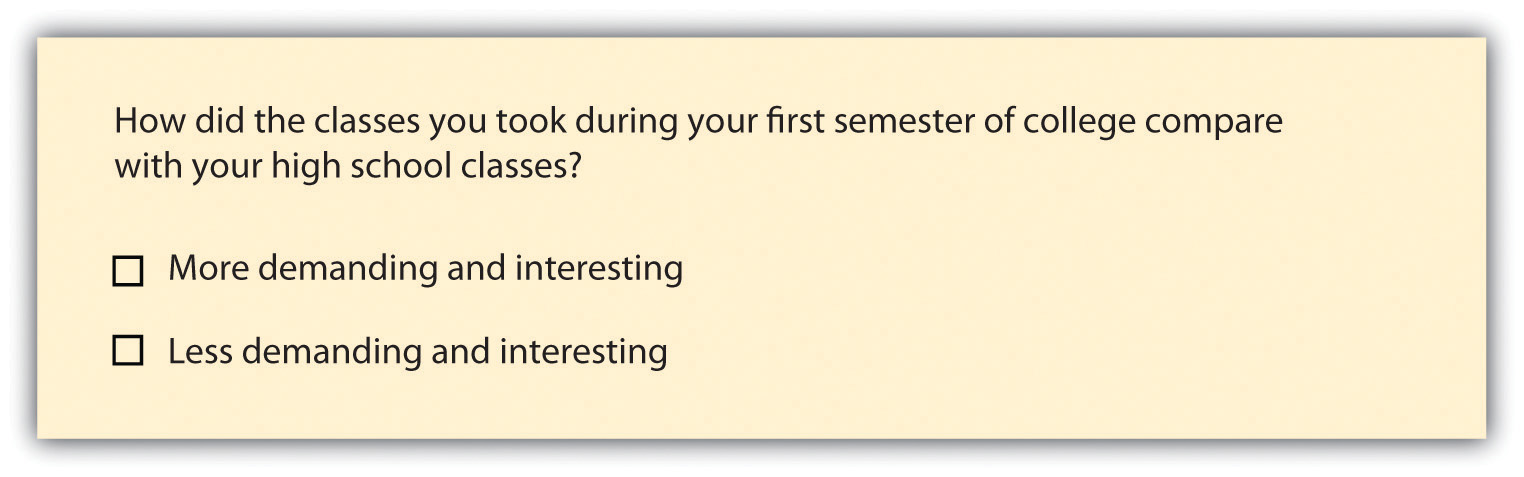
Other things to avoid when it comes to response options include fence-sitting and floating. Fence-sitters Respondents who present themselves as neutral when in truth they have an opinion. are respondents who choose neutral response options, even if they have an opinion. This can occur if respondents are given, say, five rank-ordered response options, such as strongly agree, agree, no opinion, disagree, and strongly disagree. Some people will be drawn to respond “no opinion” even if they have an opinion, particularly if their true opinion is the nonsocially desirable opinion. Floaters Respondents who choose a substantive answer to a question when in truth they don’t understand the question or the response options. , on the other hand, are those that choose a substantive answer to a question when really they don’t understand the question or don’t have an opinion. If a respondent is only given four rank-ordered response options, such as strongly agree, agree, disagree, and strongly disagree, those who have no opinion have no choice but to select a response that suggests they have an opinion.
As you can see, floating is the flip side of fence-sitting. Thus the solution to one problem is often the cause of the other. How you decide which approach to take depends on the goals of your research. Sometimes researchers actually want to learn something about people who claim to have no opinion. In this case, allowing for fence-sitting would be necessary. Other times researchers feel confident their respondents will all be familiar with every topic in their survey. In this case, perhaps it is OK to force respondents to choose an opinion. There is no always-correct solution to either problem.
Finally, using a matrix is a nice way of streamlining response options. A matrix Question type that that lists a set of questions for which the answer categories are all the same. is a question type that that lists a set of questions for which the answer categories are all the same. If you have a set of questions for which the response options are the same, it may make sense to create a matrix rather than posing each question and its response options individually. Not only will this save you some space in your survey but it will also help respondents progress through your survey more easily. A sample matrix can be seen in Figure 8.11 “Survey Questions Utilizing Matrix Format” .
Figure 8.11 Survey Questions Utilizing Matrix Format
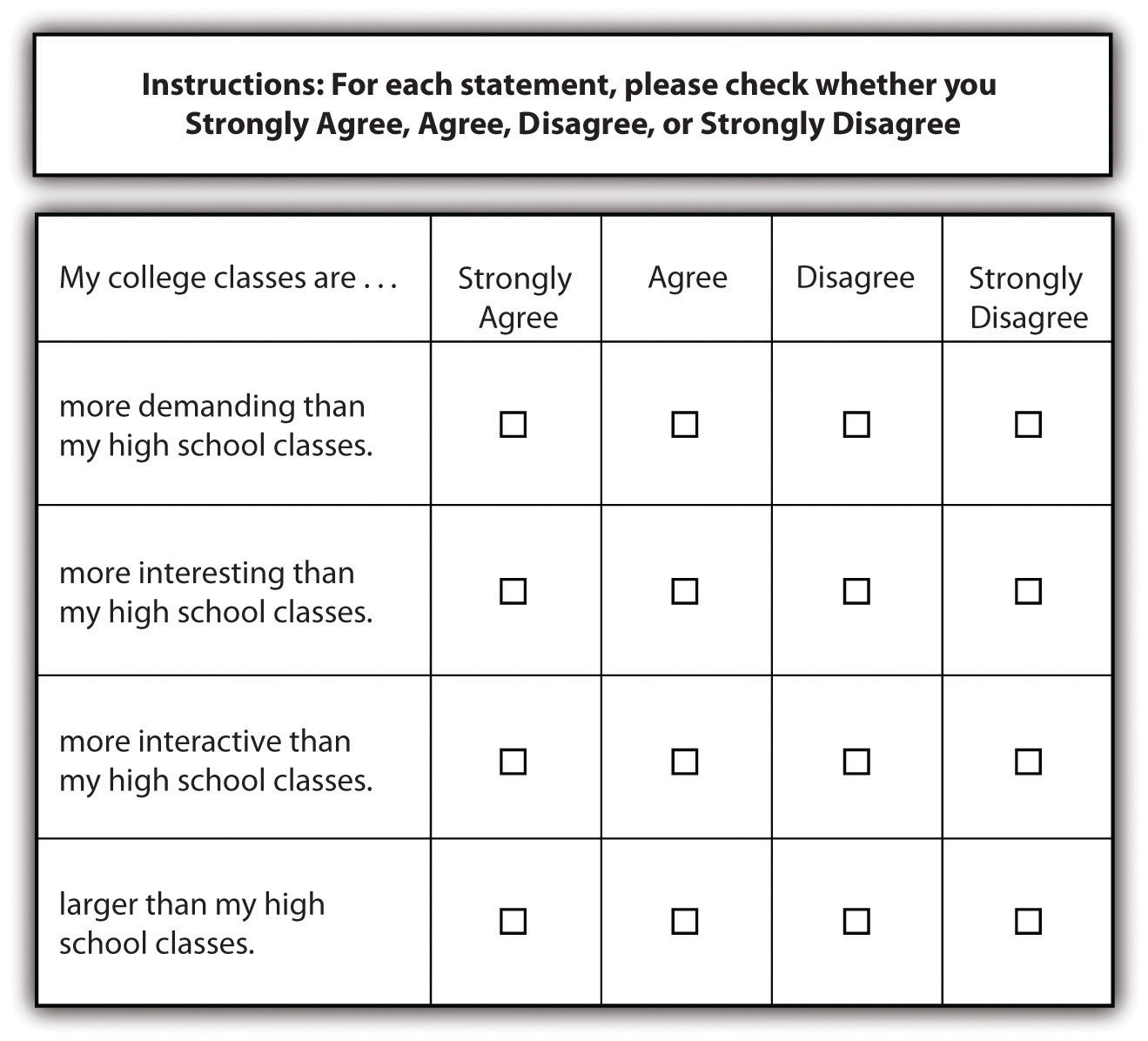
Designing Questionnaires
In addition to constructing quality questions and posing clear response options, you’ll also need to think about how to present your written questions and response options to survey respondents. Questions are presented on a questionnaire The document (either hard copy or online) that contains survey questions on which respondents read and mark their responses. , the document (either hard copy or online) that contains all your survey questions that respondents read and mark their responses on. Designing questionnaires takes some thought, and in this section we’ll discuss the sorts of things you should think about as you prepare to present your well-constructed survey questions on a questionnaire.
One of the first things to do once you’ve come up with a set of survey questions you feel confident about is to group those questions thematically. In our example of the transition to college, perhaps we’d have a few questions asking about study habits, others focused on friendships, and still others on exercise and eating habits. Those may be the themes around which we organize our questions. Or perhaps it would make more sense to present any questions we had about precollege life and habits and then present a series of questions about life after beginning college. The point here is to be deliberate about how you present your questions to respondents.
Once you have grouped similar questions together, you’ll need to think about the order in which to present those question groups. Most survey researchers agree that it is best to begin a survey with questions that will want to make respondents continue (Babbie, 2010; Dillman, 2000; Neuman, 2003). Babbie, E. (2010). The practice of social research (12th ed.). Belmont, CA: Wadsworth; Dillman, D. A. (2000). Mail and Internet surveys: The tailored design method (2nd ed.). New York, NY: Wiley; Neuman, W. L. (2003). Social research methods: Qualitative and quantitative approaches (5th ed.). Boston, MA: Pearson. In other words, don’t bore respondents, but don’t scare them away either. There’s some disagreement over where on a survey to place demographic questions such as those about a person’s age, gender, and race. On the one hand, placing them at the beginning of the questionnaire may lead respondents to think the survey is boring, unimportant, and not something they want to bother completing. On the other hand, if your survey deals with some very sensitive or difficult topic, such as child sexual abuse or other criminal activity, you don’t want to scare respondents away or shock them by beginning with your most intrusive questions.
In truth, the order in which you present questions on a survey is best determined by the unique characteristics of your research—only you, the researcher, hopefully in consultation with people who are willing to provide you with feedback, can determine how best to order your questions. To do so, think about the unique characteristics of your topic, your questions, and most importantly, your sample. Keeping in mind the characteristics and needs of the people you will ask to complete your survey should help guide you as you determine the most appropriate order in which to present your questions.
You’ll also need to consider the time it will take respondents to complete your questionnaire. Surveys vary in length, from just a page or two to a dozen or more pages, which means they also vary in the time it takes to complete them. How long to make your survey depends on several factors. First, what is it that you wish to know? Wanting to understand how grades vary by gender and year in school certainly requires fewer questions than wanting to know how people’s experiences in college are shaped by demographic characteristics, college attended, housing situation, family background, college major, friendship networks, and extracurricular activities. Keep in mind that even if your research question requires a good number of questions be included in your questionnaire, do your best to keep the questionnaire as brief as possible. Any hint that you’ve thrown in a bunch of useless questions just for the sake of throwing them in will turn off respondents and may make them not want to complete your survey.
Second, and perhaps more important, how long are respondents likely to be willing to spend completing your questionnaire? If you are studying college students, asking them to use their precious fun time away from studying to complete your survey may mean they won’t want to spend more than a few minutes on it. But if you have the endorsement of a professor who is willing to allow you to administer your survey in class, students may be willing to give you a little more time (though perhaps the professor will not). The time that survey researchers ask respondents to spend on questionnaires varies greatly. Some advise that surveys should not take longer than about 15 minutes to complete (cited in Babbie 2010), This can be found at http://www.worldopinion.com/the_frame/frame4.html , cited in Babbie, E. (2010). The practice of social research (12th ed.). Belmont, CA: Wadsworth. others suggest that up to 20 minutes is acceptable (Hopper, 2010). Hopper, J. (2010). How long should a survey be? Retrieved from http://www.verstaresearch.com/blog/how-long-should-a-survey-be As with question order, there is no clear-cut, always-correct answer about questionnaire length. The unique characteristics of your study and your sample should be considered in order to determine how long to make your questionnaire.
A good way to estimate the time it will take respondents to complete your questionnaire is through pretesting Getting feedback on a questionnaire so that it can be improved before it is administered. . Pretesting allows you to get feedback on your questionnaire so you can improve it before you actually administer it. Pretesting can be quite expensive and time consuming if you wish to test your questionnaire on a large sample of people who very much resemble the sample to whom you will eventually administer the finalized version of your questionnaire. But you can learn a lot and make great improvements to your questionnaire simply by pretesting with a small number of people to whom you have easy access (perhaps you have a few friends who owe you a favor). By pretesting your questionnaire you can find out how understandable your questions are, get feedback on question wording and order, find out whether any of your questions are exceptionally boring or offensive, and learn whether there are places where you should have included filter questions, to name just a few of the benefits of pretesting. You can also time pretesters as they take your survey. Ask them to complete the survey as though they were actually members of your sample. This will give you a good idea about what sort of time estimate to provide respondents when it comes time to actually administer your survey, and about whether you have some wiggle room to add additional items or need to cut a few items.
Perhaps this goes without saying, but your questionnaire should also be attractive. A messy presentation style can confuse respondents or, at the very least, annoy them. Be brief, to the point, and as clear as possible. Avoid cramming too much into a single page, make your font size readable (at least 12 point), leave a reasonable amount of space between items, and make sure all instructions are exceptionally clear. Think about books, documents, articles, or web pages that you have read yourself—which were relatively easy to read and easy on the eyes and why? Try to mimic those features in the presentation of your survey questions.
- Brainstorming and consulting the literature are two important early steps to take when preparing to write effective survey questions.
- Make sure that your survey questions will be relevant to all respondents and that you use filter questions when necessary.
- Getting feedback on your survey questions is a crucial step in the process of designing a survey.
- When it comes to creating response options, the solution to the problem of fence-sitting might cause floating, whereas the solution to the problem of floating might cause fence sitting.
- Pretesting is an important step for improving one’s survey before actually administering it.
- Do a little Internet research to find out what a Likert scale is and when you may use one.
- Write a closed-ended question that follows the guidelines for good survey question construction. Have a peer in the class check your work (you can do the same for him or her!).
8.5 Analysis of Survey Data
- Define response rate, and discuss some of the current thinking about response rates.
- Describe what a codebook is and what purpose it serves.
- Define univariate, bivariate, and multivariate analysis.
- Describe each of the measures of central tendency.
- Describe what a contingency table displays.
This text is primarily focused on designing research, collecting data, and becoming a knowledgeable and responsible consumer of research. We won’t spend as much time on data analysis, or what to do with our data once we’ve designed a study and collected it, but I will spend some time in each of our data-collection chapters describing some important basics of data analysis that are unique to each method. Entire textbooks could be (and have been) written entirely on data analysis. In fact, if you’ve ever taken a statistics class, you already know much about how to analyze quantitative survey data. Here we’ll go over a few basics that can get you started as you begin to think about turning all those completed questionnaires into findings that you can share.
From Completed Questionnaires to Analyzable Data
It can be very exciting to receive those first few completed surveys back from respondents. Hopefully you’ll even get more than a few back, and once you have a handful of completed questionnaires, your feelings may go from initial euphoria to dread. Data are fun and can also be overwhelming. The goal with data analysis is to be able to condense large amounts of information into usable and understandable chunks. Here we’ll describe just how that process works for survey researchers.
As mentioned, the hope is that you will receive a good portion of the questionnaires you distributed back in a completed and readable format. The number of completed questionnaires you receive divided by the number of questionnaires you distributed is your response rate The percentage of completed questionnaires returned; determined by dividing the number of completed questionnaires by the number originally distributed. . Let’s say your sample included 100 people and you sent questionnaires to each of those people. It would be wonderful if all 100 returned completed questionnaires, but the chances of that happening are about zero. If you’re lucky, perhaps 75 or so will return completed questionnaires. In this case, your response rate would be 75% (75 divided by 100). That’s pretty darn good. Though response rates vary, and researchers don’t always agree about what makes a good response rate, having three-quarters of your surveys returned would be considered good, even excellent, by most survey researchers. There has been lots of research done on how to improve a survey’s response rate. We covered some of these previously, but suggestions include personalizing questionnaires by, for example, addressing them to specific respondents rather than to some generic recipient such as “madam” or “sir”; enhancing the questionnaire’s credibility by providing details about the study, contact information for the researcher, and perhaps partnering with agencies likely to be respected by respondents such as universities, hospitals, or other relevant organizations; sending out prequestionnaire notices and postquestionnaire reminders; and including some token of appreciation with mailed questionnaires even if small, such as a $1 bill.
The major concern with response rates is that a low rate of response may introduce nonresponse bias The possible result of having too few sample members return completed questionnaires; occurs when respondents differ in important ways from nonrespondents. into a study’s findings. What if only those who have strong opinions about your study topic return their questionnaires? If that is the case, we may well find that our findings don’t at all represent how things really are or, at the very least, we are limited in the claims we can make about patterns found in our data. While high return rates are certainly ideal, a recent body of research shows that concern over response rates may be overblown (Langer, 2003). Langer, G. (2003). About response rates: Some unresolved questions. Public Perspective , May/June, 16–18. Retrieved from http://www.aapor.org/Content/aapor/Resources/PollampSurveyFAQ1/DoResponseRatesMatter/Response_Rates_-_Langer.pdf Several studies have shown that low response rates did not make much difference in findings or in sample representativeness (Curtin, Presser, & Singer, 2000; Keeter, Kennedy, Dimock, Best, & Craighill, 2006; Merkle & Edelman, 2002). Curtin, R., Presser, S., & Singer, E. (2000). The effects of response rate changes on the index of consumer sentiment. Public Opinion Quarterly, 64 , 413–428; Keeter, S., Kennedy, C., Dimock, M., Best, J., & Craighill, P. (2006). Gauging the impact of growing nonresponse on estimates from a national RDD telephone survey. Public Opinion Quarterly, 70 , 759–779; Merkle, D. M., & Edelman, M. (2002). Nonresponse in exit polls: A comprehensive analysis. In M. Groves, D. A. Dillman, J. L. Eltinge, & R. J. A. Little (Eds.), Survey nonresponse (pp. 243–258). New York, NY: John Wiley and Sons. For now, the jury may still be out on what makes an ideal response rate and on whether, or to what extent, researchers should be concerned about response rates. Nevertheless, certainly no harm can come from aiming for as high a response rate as possible.
Whatever your survey’s response rate, the major concern of survey researchers once they have their nice, big stack of completed questionnaires is condensing their data into manageable, and analyzable, bits. One major advantage of quantitative methods such as survey research, as you may recall from Chapter 1 “Introduction” , is that they enable researchers to describe large amounts of data because they can be represented by and condensed into numbers. In order to condense your completed surveys into analyzable numbers, you’ll first need to create a codebook A document that outlines how a survey researcher has translated her or his data from words into numbers. . A codebook is a document that outlines how a survey researcher has translated her or his data from words into numbers. An excerpt from the codebook I developed from my survey of older workers can be seen in Table 8.2 “Codebook Excerpt From Survey of Older Workers” . The coded responses you see can be seen in their original survey format in Chapter 6 “Defining and Measuring Concepts” , Figure 6.12 “Example of an Index Measuring Financial Security” . As you’ll see in the table, in addition to converting response options into numerical values, a short variable name is given to each question. This shortened name comes in handy when entering data into a computer program for analysis.
Table 8.2 Codebook Excerpt From Survey of Older Workers
Variable # | Variable name | Question | Options |
---|---|---|---|
11 | FINSEC | In general, how financially secure would you say you are? | 1 = Not at all secure |
2 = Between not at all and moderately secure | |||
3 = Moderately secure | |||
4 = Between moderately and very secure | |||
5 = Very secure | |||
12 | FINFAM | Since age 62, have you ever received money from family members or friends to help make ends meet? | 0 = No |
1 = Yes | |||
13 | FINFAMT | If yes, how many times? | 1 = 1 or 2 times |
2 = 3 or 4 times | |||
3 = 5 times or more | |||
14 | FINCHUR | Since age 62, have you ever received money from a church or other organization to help make ends meet? | 0 = No |
1 = Yes | |||
15 | FINCHURT | If yes, how many times? | 1 = 1 or 2 times |
2 = 3 or 4 times | |||
3 = 5 times or more | |||
16 | FINGVCH | Since age 62, have you ever donated money to a church or other organization? | 0 = No |
1 = Yes | |||
17 | FINGVFAM | Since age 62, have you ever given money to a family member or friend to help them make ends meet? | 0 = No |
1 = Yes |
If you’ve administered your questionnaire the old fashioned way, via snail mail, the next task after creating your codebook is data entry. If you’ve utilized an online tool such as SurveyMonkey to administer your survey, here’s some good news—most online survey tools come with the capability of importing survey results directly into a data analysis program. Trust me—this is indeed most excellent news. (If you don’t believe me, I highly recommend administering hard copies of your questionnaire next time around. You’ll surely then appreciate the wonders of online survey administration.)
For those who will be conducting manual data entry, there probably isn’t much I can say about this task that will make you want to perform it other than pointing out the reward of having a database of your very own analyzable data. We won’t get into too many of the details of data entry, but I will mention a few programs that survey researchers may use to analyze data once it has been entered. The first is SPSS, or the Statistical Package for the Social Sciences ( http://www.spss.com ). SPSS is a statistical analysis computer program designed to analyze just the sort of data quantitative survey researchers collect. It can perform everything from very basic descriptive statistical analysis to more complex inferential statistical analysis. SPSS is touted by many for being highly accessible and relatively easy to navigate (with practice). Other programs that are known for their accessibility include MicroCase ( http://www.microcase.com/index.html ), which includes many of the same features as SPSS, and Excel ( http://office.microsoft.com/en-us/excel-help/about-statistical-analysis-tools-HP005203873.aspx ), which is far less sophisticated in its statistical capabilities but is relatively easy to use and suits some researchers’ purposes just fine. Check out the web pages for each, which I’ve provided links to in the chapter’s endnotes, for more information about what each package can do.
Identifying Patterns
Data analysis is about identifying, describing, and explaining patterns. Univariate analysis Analysis of a single variable. is the most basic form of analysis that quantitative researchers conduct. In this form, researchers describe patterns across just one variable. Univariate analysis includes frequency distributions and measures of central tendency. A frequency distribution is a way of summarizing the distribution of responses on a single survey question. Let’s look at the frequency distribution for just one variable from my older worker survey. We’ll analyze the item mentioned first in the codebook excerpt given earlier, on respondents’ self-reported financial security.
Table 8.3 Frequency Distribution of Older Workers’ Financial Security
In general, how financially secure would you say you are? | Value | Frequency | Percentage |
---|---|---|---|
Not at all secure | 1 | 46 | 25.6 |
Between not at all and moderately secure | 2 | 43 | 23.9 |
Moderately secure | 3 | 76 | 42.2 |
Between moderately and very secure | 4 | 11 | 6.1 |
Very secure | 5 | 4 | 2.2 |
Total valid cases = 180; no response = 3 |
As you can see in the frequency distribution on self-reported financial security, more respondents reported feeling “moderately secure” than any other response category. We also learn from this single frequency distribution that fewer than 10% of respondents reported being in one of the two most secure categories.
Another form of univariate analysis that survey researchers can conduct on single variables is measures of central tendency. Measures of central tendency tell us what the most common, or average, response is on a question. Measures of central tendency can be taken for any level variable of those we learned about in Chapter 6 “Defining and Measuring Concepts” , from nominal to ratio. There are three kinds of measures of central tendency: modes, medians, and means. Mode A measure of central tendency that identifies the most common response given to a question. refers to the most common response given to a question. Modes are most appropriate for nominal-level variables. A median A measure of central tendency that identifies the middle point in a distribution of responses. is the middle point in a distribution of responses. Median is the appropriate measure of central tendency for ordinal-level variables. Finally, the measure of central tendency used for interval- and ratio-level variables is the mean. To obtain a mean A measure of central tendency that identifies the average response to an interval- or ratio-level question; found by adding the value of all responses on a single variable and dividing by the total number of responses to that question. , one must add the value of all responses on a given variable and then divide that number of the total number of responses.
In the previous example of older workers’ self-reported levels of financial security, the appropriate measure of central tendency would be the median, as this is an ordinal-level variable. If we were to list all responses to the financial security question in order and then choose the middle point in that list, we’d have our median. In Figure 8.12 “Distribution of Responses and Median Value on Workers’ Financial Security” , the value of each response to the financial security question is noted, and the middle point within that range of responses is highlighted. To find the middle point, we simply divide the number of valid cases by two. The number of valid cases, 180, divided by 2 is 90, so we’re looking for the 90th value on our distribution to discover the median. As you’ll see in Figure 8.12 “Distribution of Responses and Median Value on Workers’ Financial Security” , that value is 3, thus the median on our financial security question is 3, or “moderately secure.”
Figure 8.12 Distribution of Responses and Median Value on Workers’ Financial Security
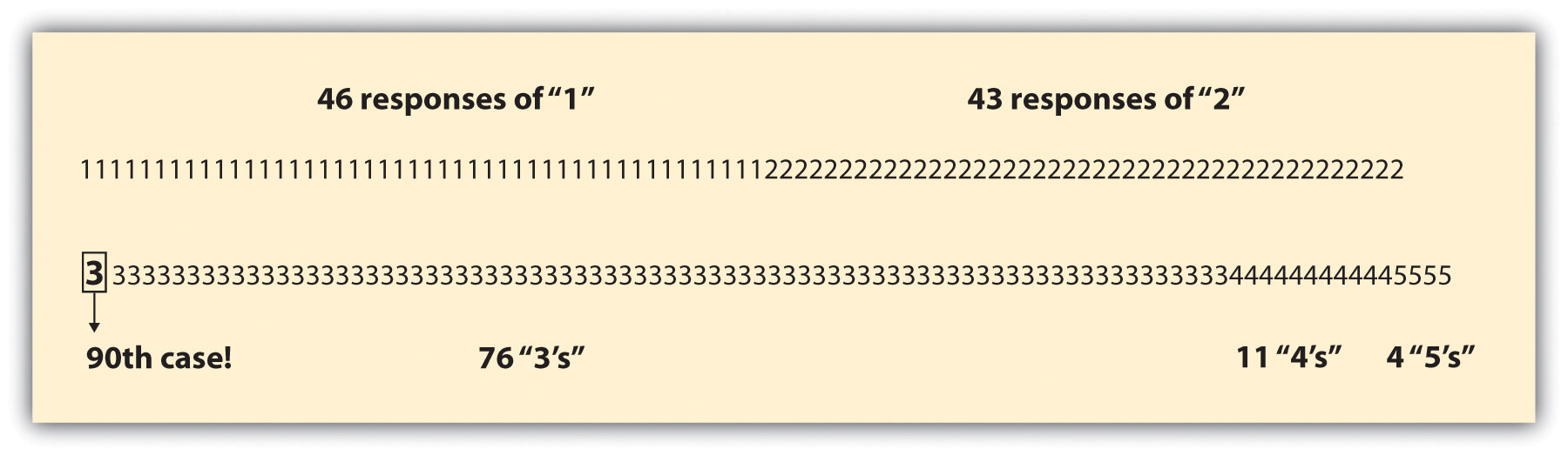
As you can see, we can learn a lot about our respondents simply by conducting univariate analysis of measures on our survey. We can learn even more, of course, when we begin to examine relationships among variables. Either we can analyze the relationships between two variables, called bivariate analysis Analysis of the relationships between two variables. , or we can examine relationships among more than two variables. This latter type of analysis is known as multivariate analysis Analysis of the relationships among multiple variables. .
Bivariate analysis allows us to assess covariation Occurs when changes in one variable happen together with changes in another. among two variables. This means we can find out whether changes in one variable occur together with changes in another. If two variables do not covary, they are said to have independence Occurs when there is no relationship between the variables in question. . This means simply that there is no relationship between the two variables in question. To learn whether a relationship exists between two variables, a researcher may cross-tabulate The process for creating a contingency table. the two variables and present their relationship in a contingency table. A contingency table Displays how variation on one variable may be contingent on variation on another. shows how variation on one variable may be contingent on variation on the other. Let’s take a look at a contingency table. In Table 8.4 “Financial Security Among Men and Women Workers Age 62 and Up” , I have cross-tabulated two questions from my older worker survey: respondents’ reported gender and their self-rated financial security.
Table 8.4 Financial Security Among Men and Women Workers Age 62 and Up
Men | Women | |
---|---|---|
Not financially secure (%) | 44.1 | 51.8 |
Moderately financially secure (%) | 48.9 | 39.2 |
Financially secure (%) | 7.0 | 9.0 |
Total | N = 43 | N = 135 |
You’ll see in Table 8.4 “Financial Security Among Men and Women Workers Age 62 and Up” that I collapsed a couple of the financial security response categories (recall that there were five categories presented in Table 8.3 “Frequency Distribution of Older Workers’ Financial Security” ; here there are just three). Researchers sometimes collapse response categories on items such as this in order to make it easier to read results in a table. You’ll also see that I placed the variable “gender” in the table’s columns and “financial security” in its rows. Typically, values that are contingent on other values are placed in rows (a.k.a. dependent variables), while independent variables are placed in columns. This makes comparing across categories of our independent variable pretty simple. Reading across the top row of our table, we can see that around 44% of men in the sample reported that they are not financially secure while almost 52% of women reported the same. In other words, more women than men reported that they are not financially secure. You’ll also see in the table that I reported the total number of respondents for each category of the independent variable in the table’s bottom row. This is also standard practice in a bivariate table, as is including a table heading describing what is presented in the table.
Researchers interested in simultaneously analyzing relationships among more than two variables conduct multivariate analysis. If I hypothesized that financial security declines for women as they age but increases for men as they age, I might consider adding age to the preceding analysis. To do so would require multivariate, rather than bivariate, analysis. We won’t go into detail here about how to conduct multivariate analysis of quantitative survey items here, but we will return to multivariate analysis in Chapter 14 “Reading and Understanding Social Research” , where we’ll discuss strategies for reading and understanding tables that present multivariate statistics. If you are interested in learning more about the analysis of quantitative survey data, I recommend checking out your campus’s offerings in statistics classes. The quantitative data analysis skills you will gain in a statistics class could serve you quite well should you find yourself seeking employment one day.
- While survey researchers should always aim to obtain the highest response rate possible, some recent research argues that high return rates on surveys may be less important than we once thought.
- There are several computer programs designed to assist survey researchers with analyzing their data include SPSS, MicroCase, and Excel.
- Data analysis is about identifying, describing, and explaining patterns.
- Contingency tables show how, or whether, one variable covaries with another.
- Codebooks can range from relatively simple to quite complex. For an excellent example of a more complex codebook, check out the coding for the General Social Survey (GSS): http://publicdata.norc.org:41000/gss/documents//BOOK/GSS_Codebook.pdf .
- The GSS allows researchers to cross-tabulate GSS variables directly from its website. Interested? Check out http://www.norc.uchicago.edu/GSS+Website/Data+Analysis .
- Principles of Sociological Inquiry: Qualitative and Quantitative Methods. Provided by : Saylor Academy. Located at : https://saylordotorg.github.io/text_principles-of-sociological-inquiry-qualitative-and-quantitative-methods/ . License : CC BY-NC-SA: Attribution-NonCommercial-ShareAlike
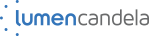
Privacy Policy
Breadcrumbs Section. Click here to navigate to respective pages.

Survey Research
DOI link for Survey Research
Click here to navigate to parent product.
This chapter provides an overview of several key issues in survey research. It first describes the processes of writing questions and some pitfalls to avoid when writing questions. The chapter next discusses issues in choosing question response formats, including levels of measurement and types of response formats. It then considers the advantage of using multi-item response scales, especially for measuring hypothetical constructs. The chapter next discusses the problem of question-related response such as scale ambiguity, category anchoring, estimation biases, respondent interpretation of numerical scales, and primacy and recency effects. It then considers person-related response biases such as social desirability, acquiescence, and extremity. Cultural response sets are also discussed. The chapter concludes with an overview of the principles of questionnaire design, including question order, instructions, and the use of existing measures, and compares the various methods of questionnaire administration, including group administration, online surveys, telephone interviews, and in-person interviews.
- Privacy Policy
- Terms & Conditions
- Cookie Policy
- Taylor & Francis Online
- Taylor & Francis Group
- Students/Researchers
- Librarians/Institutions
Connect with us
Registered in England & Wales No. 3099067 5 Howick Place | London | SW1P 1WG © 2024 Informa UK Limited
A Short Introduction to Survey Research
- First Online: 20 November 2018
Cite this chapter
- Daniel Stockemer 2
158k Accesses
1 Citations
This chapter offers a brief introduction into survey research. In the first part of the chapter, students learn about the importance of survey research in the social and behavioral sciences, substantive research areas where survey research is frequently used, and important cross-national survey such as the World Values Survey and the European Social Survey. In the second, I introduce different types of surveys.
This is a preview of subscription content, log in via an institution to check access.
Access this chapter
Subscribe and save.
- Get 10 units per month
- Download Article/Chapter or eBook
- 1 Unit = 1 Article or 1 Chapter
- Cancel anytime
- Available as PDF
- Read on any device
- Instant download
- Own it forever
- Available as EPUB and PDF
Tax calculation will be finalised at checkout
Purchases are for personal use only
Institutional subscriptions
In the literature, such reversed causation is often referred to as an endogeneity problem.
Almond, G., & Verba, S. (1963) [1989]. The civic culture: Political attitudes and democracy in five nations. Newbury Park, CA: Sage.
Google Scholar
Archer, K., & Berdahl, L. (2011). Explorations: Conducting empirical research in canadian political science . Toronto: Oxford University Press.
Behnke, J., Baur, N., & Behnke, N. (2006). Empirische Methoden der Politikwissenschaft . Paderborn: Schöningh.
Brady, H. E., Verba, S., & Schlozman, K. L. (1995). Beyond SES: A resource model of political participation. American Political Science Review, 89 (2), 271–294.
Article Google Scholar
Burnham, P., Lutz, G. L., Grant, W., & Layton-Henry, Z. (2008). Research methods in politics (2nd ed.). Basingstoke, Hampshire: Palgrave Macmillan.
Book Google Scholar
Converse, J. M. (2011). Survey research in the United States: Roots and emergence 1890–1960 . Picataway: Transaction.
De Leeuw, E. D., Hox, J. J., & Dillman, D. A. (2008). The cornerstones of survey research. In E. D. De Leeuw, J. J. Hox, & D. A. Dillman (Eds.), International handbook of survey methodology . New York: Lawrence Erlbaum Associates.
De Vaus, D. (2001). Research design in social research . London: Sage.
ESS (European Social Survey). (2017). Source questionnaire . Retrieved August 7, 2017, from http://www.europeansocialsurvey.org/methodology/ess_methodology/source_questionnaire/
Fowler, F. J. (2009). Survey research methods (4th ed.). Thousand Oaks, CA: Sage.
Frees, E. W. (2004). Longitudinal and panel data: Analysis and applications in the social sciences . Cambridge: Cambridge University Press.
Hooper, K. (2006). Using William the conqueror’s accounting record to assess manorial efficiency: A critical appraisal. Accounting History, 11 (1), 63–72.
Hurtienne, T., & Kaufmann, G. (2015). Methodological biases: Inglehart’s world value survey and Q methodology . Berlin: Folhas do NAEA.
Loosveldt, G. (2008). Face-to-face interviews . In E. D. De Leeuw, J. J. Hox, & D. A. Dillman (Eds.), International handbook of survey methodology . New York: Lawrence Erlbaum Associates.
Petty, W., & Graunt, J. (1899). The economic writings of Sir William Petty (Vol. 1). London: University Press.
Putnam, R. D. (2001). Bowling alone: The collapse and revival of American community . New York: Simon and Schuster.
Schnell, R., Hill, P. B., & Esser, E. (2011). Methoden der empirischen Sozialforschung (9th ed.). München: Oldenbourg.
Schumann, S. (2012). Repräsentative Umfrage: Praxisorientierte Einführung in empirische Methoden und statistische Analyseverfahren (6th ed.). München: Oldenbourg.
Willcox, W. F. (1934). Note on the chronology of statistical societies. Journal of the American Statistical Association, 29 (188), 418–420.
Wood, E. J. (2003). Insurgent collective action and civil war in El Salvador . Cambridge: Cambridge University Press.
Further Reading
Why do we need survey research.
Converse, J. M. (2017). Survey research in the United States: Roots and emergence 1890–1960. New York: Routledge. This book has more of an historical ankle. It tackles the history of survey research in the United States.
Davidov, E., Schmidt, P., & Schwartz, S. H. (2008). Bringing values back in: The adequacy of the European Social Survey to measure values in 20 countries. Public Opinion Quarterly, 72 (3), 420–445. This rather short article highlights the importance of conducting a large pan-European survey to measure European’s social and political beliefs.
Schmitt, H., Hobolt, S. B., Popa, S. A., & Teperoglou, E. (2015). European parliament election study 2014, voter study. GESIS Data Archive, Cologne. ZA5160 Data file Version , 2 (0). The European Voter Study is another important election study that researchers and students can access freely. It provides a comprehensive battery of variables about voting, political preferences, vote choice, demographics, and political and social opinions of the electorate.
Applied Survey Research
Almond, G. A., & Verba, S. (1963). The civic culture: Political attitudes and democracy in five nations. Princeton: Princeton University Press. Almond’s and Verba’s masterpiece is a seminal work in survey research measuring citizens’ political and civic attitudes in key Western democracies. The book is also one of the first books that systematically uses survey research to measure political traits.
Inglehart, R., & Welzel, C. (2005). Modernization, cultural change, and democracy: The human development sequence . Cambridge: Cambridge University Press. This is an influential book, which uses data from the World Values Survey to explain modernization as a process that changes individual’s values away from traditional and patriarchal values and toward post-materialist values including environmental protection, minority rights, and gender equality.
Download references
Author information
Authors and affiliations.
School of Political Studies, University of Ottawa, Ottawa, Ontario, Canada
Daniel Stockemer
You can also search for this author in PubMed Google Scholar
Rights and permissions
Reprints and permissions
Copyright information
© 2019 Springer International Publishing AG
About this chapter
Stockemer, D. (2019). A Short Introduction to Survey Research. In: Quantitative Methods for the Social Sciences. Springer, Cham. https://doi.org/10.1007/978-3-319-99118-4_3
Download citation
DOI : https://doi.org/10.1007/978-3-319-99118-4_3
Published : 20 November 2018
Publisher Name : Springer, Cham
Print ISBN : 978-3-319-99117-7
Online ISBN : 978-3-319-99118-4
eBook Packages : Social Sciences Social Sciences (R0)
Share this chapter
Anyone you share the following link with will be able to read this content:
Sorry, a shareable link is not currently available for this article.
Provided by the Springer Nature SharedIt content-sharing initiative
- Publish with us
Policies and ethics
- Find a journal
- Track your research
- Latest Latest
- The West The West
- Sports Sports
- Opinion Opinion
- Magazine Magazine
Is it common to invite people to church? Here’s what new research shows
A new survey finds that two-thirds of protestant churchgoers invite their friends to go to church with them.

By Sydney Jezik
Even if you’re a regular churchgoer, it can seem arduous or even frightening to invite someone to join you. After all, faith is a deeply personal part of one’s life.
But a new survey from Lifeway Research found that nerves don’t get the best of most Protestant churchgoers: 3 in 5 have issued at least one invitation to someone to come to church with them in the last six months.
The survey found that 21% of churchgoers have extended at least two invitations and 20% have extended three or more.
Why some churchgoers do or don’t invite guests
Some key differences exist between the churchgoers who are most likely and least likely to invite someone to church, per Lifeway Research .
For example, people age 50 or older were less likely to invite someone than people younger than 50. And Black Americans were more likely to extend invitations than any other racial demographic, while white churchgoers were the least likely.
More than one-third of white churchgoers (36%) said they have not invited anyone to join them at church in the past six months, LifeWay Research reported.
Evangelical Christians were more likely than members of other faith groups to invite someone to join them at church. Lutherans, meanwhile, are some of the least likely to have invited anyone in the last six months, the survey found.
When asked to explain why they don’t invite guests more often, respondents offered a variety of reasons, according to Lifeway Research.
“Around a quarter say they don’t know anyone to invite (27%) or those they invite refuse their invitations (26%). Another 13% say they’re just not comfortable asking people to church, while 7% say they don’t think it’s up to them to bring people to church,” the survey report said.
“It can be easy for churchgoers to have their own relationship needs met at church and not know anyone else to invite,” said Scott McConnell, executive director of Lifeway Research. “It takes intentionality to be meeting new people in your community to have opportunities to invite them.”

Are people likely to accept invitations to church?
An older survey from Lifeway Research found that about one-third of surveyed non-churchgoers were willing to accept invitations to church, according to the Christian Standard .
Willingness seems to depend on the relationship between the inviter and person they invited. Someone is more willing to attend church for the first time with a close friend or family member.
In addition, if the invited person accepts, they usually tell others about what they experienced at church. Statistics from Auxano say that “guests will talk about their initial experiences 8-15 times with other people,” per the Christian Standard .
These surveys come in the context of a shifting religious landscape in America. As the Deseret News reported in January, the growth of religiously unaffiliated Americans is plateauing — and may even reverse course in the future.
Numbers, Facts and Trends Shaping Your World
Read our research on:
Full Topic List
Regions & Countries
- Publications
- Our Methods
- Short Reads
- Tools & Resources
Read Our Research On:
The Experiences of U.S. Adults Who Don’t Have Children
57% of adults under 50 who say they’re unlikely to ever have kids say a major reason is they just don’t want to; 31% of those ages 50 and older without kids cite this as a reason they never had them, table of contents.
- Reasons for not having children
- The impact of not having children
- How the survey findings do – or don’t – differ by gender
- Views on wanting children
- Reasons adults ages 50 and older didn’t have children
- Reasons adults under 50 are unlikely to have children
- General impact of not having children
- Personal impact of not having children
- Experiences in the workplace
- Worries about the future
- Pros and cons of not having children, according to younger adults who say they’re unlikely to have kids
- The impact of not having children on relationships
- Pressure to have children
- Relationships with nieces and nephews
- Providing care for aging parents
- How often younger adults talk about having children
- Friends and children
- The impact of not having children on dating
- Educational attainment
- Marital status and living arrangements
- Employment, wages and wealth
- Acknowledgments
- The American Trends Panel survey methodology
- Secondary data methodology
Pew Research Center conducted this study to better understand the experiences of two groups of U.S. adults who don’t have children: those ages 50 and older, and those younger than 50 who say they are unlikely to ever have children. It explores their reasons for not having children or being unlikely to do so, the perceived pros and cons of not having children, and the impact of not having children on their relationships.
Most of the analysis in this report is based on a survey of 2,542 adults ages 50 and older who have never had children and 770 adults ages 18 to 49 who don’t have children and say they are not too or not at all likely to have them. The survey was conducted April 29 to May 19, 2024. Most of the respondents who took part are members of the Center’s American Trends Panel (ATP), an online survey panel that is recruited through national, random sampling of residential addresses. This survey also included an oversample of adults ages 50 and older who have never had children from Ipsos’ KnowledgePanel, another probability-based online survey web panel recruited primarily through national, random sampling of residential addresses.
Address-based sampling ensures that nearly all U.S. adults have a chance of selection. The survey is weighted to be representative of the U.S. adult population by gender, race, ethnicity, partisan affiliation, education and other categories. Read more about the ATP’s methodology .
The report also includes an analysis comparing the demographic characteristics and economic outcomes of adults ages 50 and older who do not have children with those of parents in the same age group. The data for this analysis comes from the U.S. Census Bureau’s 2021 and 2022 Surveys of Income and Program Participation (SIPP).
Here are the questions we asked adults ages 50 and older who don’t have children and adults younger than 50 who don’t have children and say they’re unlikely to have them, along with responses, and the survey’s methodology .
In this report, we do not use the terms “childless” or “child-free” to refer to adults who don’t have children. The Associated Press Stylebook , a resource we use often, recommends against using these terms.
In the survey findings featured in Chapters 1-3, references to adults who do not have children include those who indicated they have never been a parent or guardian to any children, living or deceased, including biological or adopted children.
In the analysis of government data in Chapter 4, references to those who do and do not have children include those who have or have not had biological children.
References to college graduates or people with a college degree comprise those with a bachelor’s degree or more education. “Some college” includes those with an associate degree and those who attended college but did not obtain a degree.
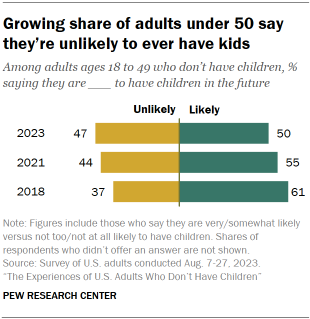
The U.S. fertility rate reached a historic low in 2023 , with a growing share of women ages 25 to 44 having never given birth .
And the share of U.S. adults younger than 50 without children who say they are unlikely to ever have kids rose 10 percentage points between 2018 and 2023 (from 37% to 47%), according to a Pew Research Center survey .
In this report, we explore the experiences of two groups of U.S. adults :
- Those ages 50 and older who don’t have children
- Those younger than 50 who don’t have children and say they are unlikely to in the future
About four-in-ten of those in the older group (38%) say there was a time when they wanted to have children. A smaller but sizable share (32%) say they never wanted children, and 25% say they weren’t sure one way or the other. Few say they frequently felt pressure to have children from family, friends or society in general.
Reasons for not having children – or being unlikely to ever have them – differ between the older and younger groups. The top response for those ages 50 and older is that it just didn’t happen. Meanwhile, those in the younger group are most likely to say they just don’t want to have kids. Women younger than 50 are especially likely to say they just don’t want to have children (64% vs. 50% of men in this group).
Majorities in both groups say not having kids has made it easier for them to afford the things they want, have time for hobbies and interests, and save for the future. In the younger group, about six-in-ten also say not having kids has made it easier for them to be successful in their job or career and to have an active social life.
Still, majorities in both groups say parents have it easier when it comes to having someone to care for them as they age. Large shares in both groups say having a fulfilling life doesn’t have much to do with whether someone does or doesn’t have children.
These are among the key findings from a new Pew Research Center survey of 2,542 adults ages 50 and older who don’t have children and 770 adults ages 18 to 49 who don’t have children and say they are not too or not at all likely to have them. The survey was conducted April 29 to May 19, 2024.
Jump to read more about:
- Reasons adults give for not having children
- Perceived pros and cons of not having children
- Relationships and caregiving among adults without children
- Demographic and economic characteristics of adults 50 and older without children
The study explores reasons U.S. adults give for not having children, among those ages 50 and older who haven’t had kids and those under 50 who say they’re unlikely to ever become parents.
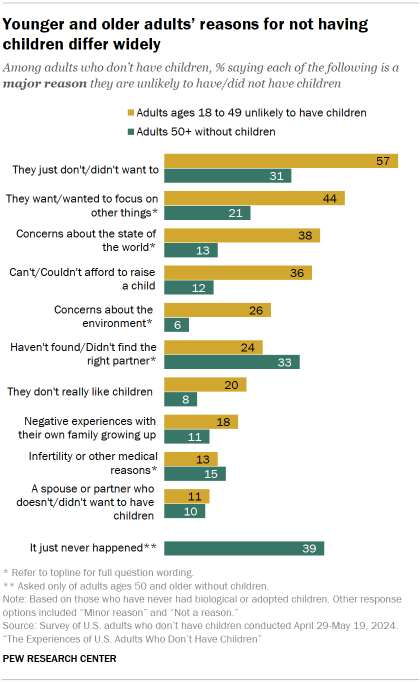
By margins of at least 10 points, those in the younger group are more likely than those ages 50 and older to say each of the following is a major reason:
- They just don’t want to have children (57% in the younger group vs. 31% in the older group)
- They want to focus on other things, such as their career or interests (44% vs. 21%)
- Concerns about the state of the world, other than the environment (38% vs. 13%)
- They can’t afford to raise a child (36% vs. 12%)
- Concerns about the environment, including climate change (26% vs. 6%)
- They don’t really like children (20% vs. 8%)
In turn, a larger share of those in the older group say a major reason they didn’t have kids is that they didn’t find the right partner (33% vs. 24% of those in the younger group).
There are no significant differences between the two groups in the shares pointing to infertility or other medical reasons (their own or their spouse’s or partner’s) or to a spouse or partner who didn’t want to have children as major reasons.
Among those in their 40s, 22% say infertility or other medical reasons are a major factor in why they’re unlikely to ever have children. About one-in-ten of those ages 18 to 39 (9%) say the same.
Majorities of adults ages 50 and older who don’t have kids and those under 50 who say they’re unlikely to do so see some benefits to not having children.
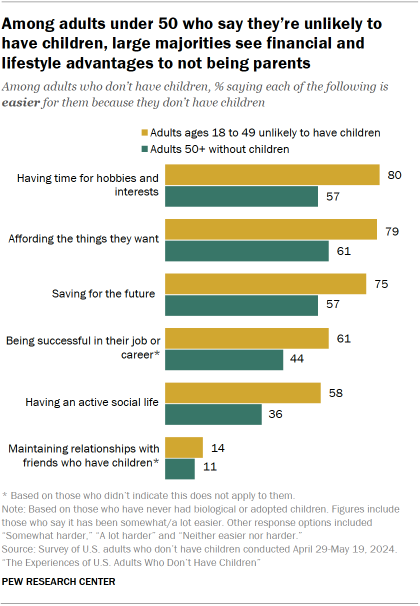
But by margins ranging from 17 to 23 points, those in the younger group are more likely than those ages 50 and older to say each of the following has been easier for them because they don’t have children:
- Having time for hobbies and interests (80% in the younger group vs. 57% in the older group)
- Affording the things they want (79% vs. 61%)
- Saving for the future (75% vs. 57%)
- Being successful in their job or career (61% vs. 44%, among those who don’t indicate this doesn’t apply to them)
- Having an active social life (58 vs. 36%)
The impact at work
We also asked those who are employed about the impact not having children has had on their work lives.
Experiences are mixed. For example, 45% of those in the younger group and 35% of those in the older group say they’ve had more opportunities to network outside of work hours because they don’t have kids. At the same time, about a third in each group say they’ve been expected to take on extra work or responsibilities, and many also say they’ve been given less flexibility than those who have children.
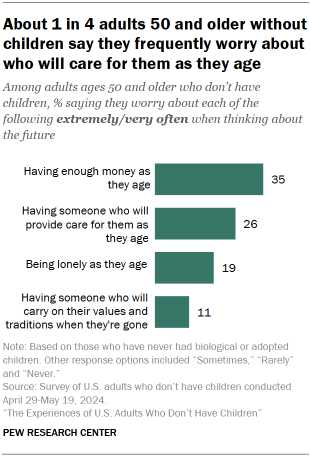
The survey also asked adults ages 50 and older without children about certain concerns they may have as they age .
About one-in-five or more say they worry extremely or very often about:
- Having enough money (35%)
- Having someone who will provide care for them (26%)
- Being lonely (19%)
A smaller share (11%) say they frequently worry about having someone who will carry on their values and traditions when they’re gone.
In a separate survey , 46% of parents ages 50 and older said they frequently worry about having enough money as they age. Smaller shares said the same about having someone who will provide care for them as they age (20%), having someone who will carry on their values and traditions (17%) and being lonely as they age (15%).
For the most part, the experiences of adults without children and the reasons they give for not having them don’t vary much by gender. This is the case across both age groups.
Still, there are some questions on which men and women without kids differ considerably.
Among those ages 50 and older, women are more likely than men to say:
- Being successful in their job or career has been easier because they don’t have children (50% among women vs. 39% among men).
- They felt pressure to have children from society in general at least sometimes when they were younger (42% vs. 27%).
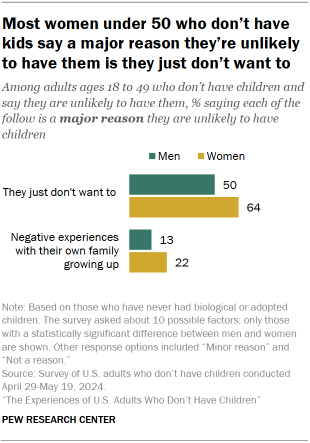
Among those ages 18 to 49, women are more likely than men to say each of the following is a major reason they’re unlikely to have children:
- They just don’t want to (64% vs. 50%)
- Negative experiences with their own families growing up (22% vs. 13%)
Women in the younger group are also more likely than their male counterparts to say the topic of whether they’ll have children comes up in conversation with their friends at least sometimes (41% vs. 26%).
Demographic and economic differences between adults 50 and older with and without children
In addition to the survey findings, this report includes an analysis of government data to show how the demographic characteristics and economic outcomes of adults ages 50 and older who don’t have children differ from those ages 50 and older who are parents.
Among adults in this age group, those who don’t have children are less likely to have ever been married. They are more likely to have a bachelor’s degree or more education. This difference in educational attainment is especially pronounced among women.
Older women who don’t have children have higher median monthly wages than mothers. The opposite is true among older men; those without children tend to earn less than fathers.
Sign up for our weekly newsletter
Fresh data delivery Saturday mornings
Sign up for The Briefing
Weekly updates on the world of news & information
Cultural Issues and the 2024 Election
Americans overwhelmingly say access to ivf is a good thing, few east asian adults believe women have an obligation to society to have children, among parents with young adult children, some dads feel less connected to their kids than moms do, how teens and parents approach screen time, most popular, report materials.
- Topline ages 18-49
- Topline ages 50+
901 E St. NW, Suite 300 Washington, DC 20004 USA (+1) 202-419-4300 | Main (+1) 202-857-8562 | Fax (+1) 202-419-4372 | Media Inquiries
Research Topics
- Email Newsletters
ABOUT PEW RESEARCH CENTER Pew Research Center is a nonpartisan fact tank that informs the public about the issues, attitudes and trends shaping the world. It conducts public opinion polling, demographic research, media content analysis and other empirical social science research. Pew Research Center does not take policy positions. It is a subsidiary of The Pew Charitable Trusts .
© 2024 Pew Research Center
Research Team Reports Latest Leadership Survey Findings

Is social media scaring away potential leaders?
by Jim Hanchett | July 29, 2024
Above: Dr. Lynn Shollen, center, speaks at the Latin Honors Ceremony for graduates.
Is violence as “activism” now considered leadership?
The way social media functions may have the unintended consequence of scaring people away from taking on leadership roles, according to the results of an annual national survey about leadership.
The scientific online survey of 2,050 people as a nationally representative sample was conducted by Leadership Studies researchers Dr. Lynn Shollen, Dr. Elizabeth Gagnon and Dr. Kat Callahan. The survey, entitled “Attitudes About Leadership in the United States,” is the fourth annual version of a longitudinal research project based at Christopher Newport. The project’s primary goal is to bring awareness to the data so that it can be used by other researchers. A glimpse of the CNU team’s findings are as follows:
There is indication that the social climate within the United States and reactions on social media make people hesitant to lead.
- In 2022, only 34% agreed that the social climate during the previous year made people willing to lead, while the same proportion agreed that it was too risky to be a leader considering the social climate during the previous year.
- In 2022, 45% believed that social media makes it more difficult to be a leader.
- Across the past three years, almost half of respondents indicated that social media lowers people’s expectations of leaders, whereas one-third indicated that social media raises people’s expectations of leaders.
- Almost half disagree with the statement that social media makes it easier to accurately evaluate public leaders.
A large majority of respondents considered activism as leadership if it involved armed or unarmed, violent/destructive protesting. Unarmed, non-violent/peaceful protesting was much less likely to be seen as leadership.
- When asked about this rather alarming result, Shollen explained that “the research team was also surprised by this result, so we triple-checked the data. The only factor we could think of that may have biased the results is that we defined activism for participants as the principle or practice of vigorous action or involvement as a means of achieving political or social change, so perhaps respondents thought of the vigorous action component as violence and destruction. However, two-thirds of participants indicated that armed, non-violent/peaceful protesting would also be considered leadership, so the results may truly be showing that being armed and being violent were considered by many as leadership in 2021 and 2022.”
About 65% of respondents felt it is important or very important for leaders at the national and local levels to care for the natural environment, with another 15 to 20% indicating it is somewhat important.
The trend continues that about 60% of respondents believe that people ages 25 and younger are not being equipped to lead. Further research could explore why the perception exists and if it is indeed true.
The percentage who felt that it is important or very important for national leaders to consider perspectives of diverse people when making decisions dropped notably from 2019-2020 to 2021-2022.
There was consensus that the best leaders should understand the experiences of ordinary, everyday people, but the respondents said most leaders do not, and are far removed from understanding how average people live.
The trend continues that over 75% believe that within their lifetime leaders in the United States have become less effective.
“The survey isn’t intended to examine perceptions of how specific leaders are performing, rather how people view the effectiveness of leaders and leadership generally within the U.S., as well as the impact of various factors on their perceptions of leadership and willingness to follow” Shollen said. “Although certainly a challenge and never fully controllable, the survey was designed to be as apolitical and ideologically unbiased as possible.”
More comprehensive findings and a fuller explanation of the project is available here . The research team is currently working on making the 2023 data available. For researchers who are interested, results can also be analyzed by demographics.

An official website of the United States government
Here's how you know
Official websites use .gov A .gov website belongs to an official government organization in the United States.
Secure .gov websites use HTTPS A lock ( ) or https:// means you’ve safely connected to the .gov website. Share sensitive information only on official, secure websites.
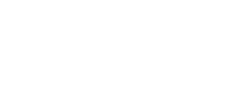
- Digg
Latest Earthquakes | Chat Share Social Media
Relieving the Sting: Spatial Prioritization for Pollinator Conservation Under a Changing Climate
Project Overview The Rusty Patched Bumble Bee, and other native bees and pollinators, are declining due to climate change, habitat loss, and other stressors like pathogens and pesticide-use. Researchers supported by this Midwest CASC project will study how certain stressors interact to affect the geographic distribution of Rusty Patched Bumble Bees, using mapping techniques and future climate data to identify vulnerable populations and future strongholds. A resulting model and web application will enable resource managers and conservation practitioners to improve pollinator recovery efforts by identifying and prioritizing future locations for conservation action, including potential species reintroductions.
- Source: USGS Sciencebase (id: 6674658bd34e68d163086bab )
Clint Otto, PhD
Research ecologist, ralph grundel, phd, branch chief, research ecologist, audrey lothspeich, kristen ellis, phd.
Texas Manufacturing Outlook Survey
- Current report
- Results table
- Historical data
Texas manufacturing activity remains flat in July amid weakening demand
For this month’s survey, Texas business executives were asked supplemental questions on labor market and financial conditions. Results for these questions from the Texas Manufacturing Outlook Survey, Texas Service Sector Outlook Survey and Texas Retail Outlook Survey have been released together. Read the special questions results.
This month’s data release also includes annual seasonal factor revisions. Once per year, the Federal Reserve Bank of Dallas revises the historical data for the Texas Manufacturing Outlook Survey after calculating new seasonal adjustment factors. Annual seasonal revisions result in slight changes in the seasonally adjusted series. Read more information on seasonal adjustment .
Texas factory activity was flat again in July, according to business executives responding to the Texas Manufacturing Outlook Survey. The production index, a key measure of state manufacturing conditions, held fairly steady at -1.3, with the near-zero reading signaling little change in output from June.
Other measures of manufacturing activity weakened this month. The new orders index dropped 12 points to -12.8 in July, signaling a pullback in demand. The capacity utilization and shipments indexes also slipped, falling to -10.0 and -16.3, respectively.
Perceptions of broader business conditions continued to worsen in July. The general business activity index inched down to -17.5 and the company outlook index stumbled 12 points to -18.4. The outlook uncertainty index shot up to 30.7, its highest reading since fall 2022.
Labor market measures suggested employment increases but shorter workweeks this month. The employment index posted a 10-point gain, rising to 7.1 and its highest level in 10 months. Nineteen percent of firms noted net hiring, while 12 percent noted net layoffs. The hours worked index remained negative and fell to -13.8 from -5.0.
Upward pressure on prices and wages continued in July. The wages and benefits index edged down to 21.2, a reading in line with the historical average. The raw materials prices index was mostly unchanged at 23.1, while the finished goods prices index slid down 11 points to 3.4.
Expectations regarding future manufacturing activity mostly pushed up this month. The future production index rose five points to 32.0, and the future general business activity index rose nine points to 21.6, its highest reading since fall 2021. Most other indexes of future manufacturing activity also rose in July.
Next release: Monday, August 26
Data were collected July 16–24, and 80 of the 125 Texas manufacturers surveyed submitted a response. The Dallas Fed conducts the Texas Manufacturing Outlook Survey monthly to obtain a timely assessment of the state’s factory activity. Firms are asked whether output, employment, orders, prices and other indicators increased, decreased or remained unchanged over the previous month.
Survey responses are used to calculate an index for each indicator. Each index is calculated by subtracting the percentage of respondents reporting a decrease from the percentage reporting an increase. When the share of firms reporting an increase exceeds the share reporting a decrease, the index will be greater than zero, suggesting the indicator has increased over the prior month. If the share of firms reporting a decrease exceeds the share reporting an increase, the index will be below zero, suggesting the indicator has decreased over the prior month. An index will be zero when the number of firms reporting an increase is equal to the number of firms reporting a decrease. Data have been seasonally adjusted as necessary.
Results summary
Historical data are available from June 2004 to the most current release month.
Business Indicators Relating to Facilities and Products in Texas Current (versus previous month) | ||||||||
Indicator | Jul Index | Jun Index | Change | Series Average | Trend* | % Reporting Increase | % Reporting No Change | % Reporting Decrease |
Production | –1.3 | 0.7 | –2.0 | 9.8 | 1(–) | 27.5 | 43.6 | 28.8 |
Capacity Utilization | –10.0 | –4.8 | –5.2 | 7.8 | 3(–) | 18.8 | 52.4 | 28.8 |
New Orders | –12.8 | –1.3 | –11.5 | 5.1 | 5(–) | 23.8 | 39.6 | 36.6 |
Growth Rate of Orders | –16.6 | –4.3 | –12.3 | –0.7 | 3(–) | 16.9 | 49.6 | 33.5 |
Unfilled Orders | –26.6 | –4.7 | –21.9 | –2.2 | 10(–) | 5.7 | 62.0 | 32.3 |
Shipments | –16.3 | 2.8 | –19.1 | 8.1 | 1(–) | 20.8 | 42.2 | 37.1 |
Delivery Time | –5.3 | –3.2 | –2.1 | 0.9 | 16(–) | 8.9 | 76.9 | 14.2 |
Finished Goods Inventories | –5.0 | –2.5 | –2.5 | –3.2 | 4(–) | 16.3 | 62.5 | 21.3 |
Prices Paid for Raw Materials | 23.1 | 21.5 | +1.6 | 27.2 | 51(+) | 32.4 | 58.3 | 9.3 |
Prices Received for Finished Goods | 3.4 | 14.4 | –11.0 | 8.6 | 8(+) | 12.4 | 78.6 | 9.0 |
Wages and Benefits | 21.2 | 24.3 | –3.1 | 21.2 | 51(+) | 23.1 | 75.0 | 1.9 |
Employment | 7.1 | –2.9 | +10.0 | 7.5 | 1(+) | 19.3 | 68.5 | 12.2 |
Hours Worked | –13.8 | –5.0 | –8.8 | 3.2 | 10(–) | 8.6 | 69.1 | 22.4 |
Capital Expenditures | 0.8 | 0.9 | –0.1 | 6.5 | 10(+) | 18.4 | 64.0 | 17.6 |
General Business Conditions Current (versus previous month) | ||||||||
Indicator | Jul Index | Jun Index | Change | Series Average | Trend** | % Reporting Improved | % Reporting No Change | % Reporting Worsened |
Company Outlook | –18.4 | –6.9 | –11.5 | 4.5 | 29(–) | 12.4 | 56.8 | 30.8 |
General Business Activity | –17.5 | –15.1 | –2.4 | 0.8 | 27(–) | 13.6 | 55.3 | 31.1 |
Indicator | Jul Index | Jun Index | Change | Series Average | Trend* | % Reporting Increase | % Reporting No Change | % Reporting Decrease |
Outlook Uncertainty | 30.7 | 9.8 | +20.9 | 17.2 | 39(+) | 33.3 | 64.1 | 2.6 |
Business Indicators Relating to Facilities and Products in Texas Future (six months ahead) | ||||||||
Indicator | Jul Index | Jun Index | Change | Series Average | Trend* | % Reporting Increase | % Reporting No Change | % Reporting Decrease |
Production | 32.0 | 27.1 | +4.9 | 36.3 | 51(+) | 42.3 | 47.4 | 10.3 |
Capacity Utilization | 21.4 | 21.3 | +0.1 | 33.1 | 51(+) | 32.0 | 57.4 | 10.6 |
New Orders | 30.3 | 29.8 | +0.5 | 33.6 | 21(+) | 40.6 | 49.0 | 10.3 |
Growth Rate of Orders | 19.3 | 24.5 | –5.2 | 24.8 | 14(+) | 28.6 | 62.1 | 9.3 |
Unfilled Orders | –2.6 | –2.9 | +0.3 | 2.8 | 5(–) | 7.7 | 82.0 | 10.3 |
Shipments | 29.1 | 28.1 | +1.0 | 34.7 | 51(+) | 39.7 | 49.7 | 10.6 |
Delivery Time | 4.6 | –0.4 | +5.0 | –1.5 | 1(+) | 8.9 | 86.8 | 4.3 |
Finished Goods Inventories | –6.9 | –8.0 | +1.1 | –0.1 | 3(–) | 12.3 | 68.5 | 19.2 |
Prices Paid for Raw Materials | 28.5 | 21.2 | +7.3 | 33.4 | 52(+) | 38.4 | 51.7 | 9.9 |
Prices Received for Finished Goods | 23.0 | 19.5 | +3.5 | 20.8 | 51(+) | 31.1 | 60.8 | 8.1 |
Wages and Benefits | 39.2 | 33.2 | +6.0 | 39.3 | 242(+) | 40.2 | 58.8 | 1.0 |
Employment | 18.7 | 11.7 | +7.0 | 22.8 | 50(+) | 24.5 | 69.7 | 5.8 |
Hours Worked | 8.8 | 3.0 | +5.8 | 8.8 | 4(+) | 18.1 | 72.6 | 9.3 |
Capital Expenditures | 22.4 | 12.6 | +9.8 | 19.4 | 50(+) | 32.3 | 57.8 | 9.9 |
General Business Conditions Future (six months ahead) | ||||||||
Indicator | Jul Index | Jun Index | Change | Series Average | Trend** | % Reporting Increase | % Reporting No Change | % Reporting Worsened |
Company Outlook | 22.0 | 22.9 | –0.9 | 18.3 | 8(+) | 29.1 | 63.8 | 7.1 |
General Business Activity | 21.6 | 12.9 | +8.7 | 12.2 | 2(+) | 28.7 | 64.2 | 7.1 |
*Shown is the number of consecutive months of expansion or contraction in the underlying indicator. Expansion is indicated by a positive index reading and denoted by a (+) in the table. Contraction is indicated by a negative index reading and denoted by a (–) in the table.
**Shown is the number of consecutive months of improvement or worsening in the underlying indicator. Improvement is indicated by a positive index reading and denoted by a (+) in the table. Worsening is indicated by a negative index reading and denoted by a (–) in the table.
Data have been seasonally adjusted as necessary.
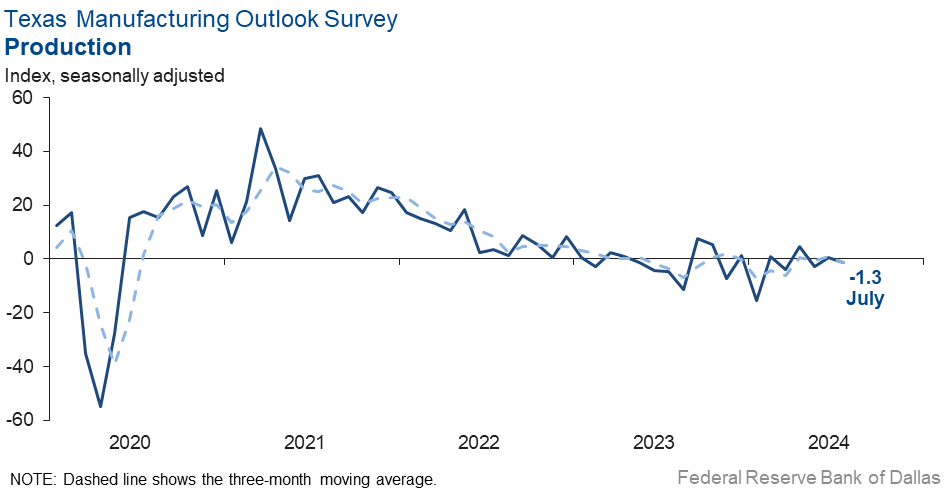
Downloadable chart
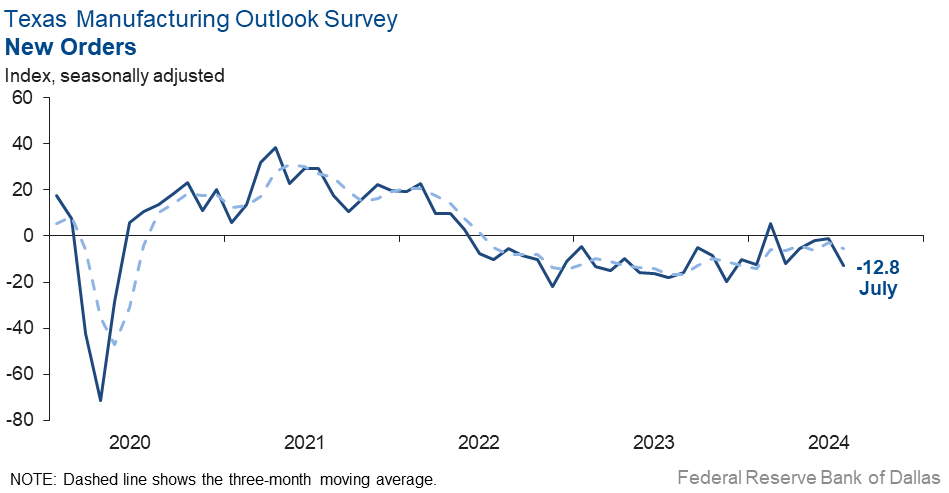
Comments from survey respondents
These comments are from respondents’ completed surveys and have been edited for publication.
- We have seen a more stable market over the last six months for our products (which is primarily dinner sausage). Nielsen data for our category show small-to-moderate growth over the last 52 weeks, and our market share is growing in our core markets. This is leading to a more predictable environment for our company. Wages increased this month due to merit increases that we gave our team at the beginning of our fiscal year, which is July.
- The business environment feels stable, but beef prices continue to increase beyond typical seasonality due to supply constraints.
- The destabilization of our country and the politicization of things continue to impact our business.
- Activity has slowed down, but we anticipate an uptick soon.
- We have slowed down some from our very hectic pace of activity in late spring and through June. We hear about lots of slowness in our industry, but we continue to be pretty busy, mainly with larger jobs but then also with other smaller ones. We have a very large capital purchase machine arriving in early October. We are hopeful that the Federal Reserve will have a rate cut and that general business activity will pick up. We are actually seeing some reduction in prices and are hopeful we will not need to raise prices next year.
- Hurricane damage and power outages have decreased production.
- Customer demand is the overriding concern. Decreased credit availability and affordability for the markets we sell into have all but stopped demand. I estimate we are operating at 30–40 percent capacity.
- Order volume continues to see small declines. Inefficiencies in the short term will require staffing adjustments over the next few months to align with the new lower baseline. There is no major change to our long-term outlook; we are still viewing the current pullback as temporary, and we maintain a positive outlook beyond the next 12–24 months.
- Elections [are an issue affecting our business].
- Business activity is horrible, and we are seeing no signs of improvement.
- Inquiries and orders activity has seemingly halted. The brakes are on. This is pretty common in presidential election years, but this comes at a time when things were already volatile due to price pressures and massive inflationary pressures.
- At this point, we're just hoping for a favorable election outcome and looking forward to 2025. The summer doldrums have hit hard and would appear to be unrelenting with respect to what we see in our crystal ball. We have some small jobs to complete, and we're searching wider and further for new work, but we have yet to strike gold.
- Many customers are holding off on expenditures as well as allowing for cost-of-living adjustments to prices. The market continues to be soft, and with uncertainty in the election year, even our federal business is a bit stagnant.
- We need lower interest rates, so end customers resume buying capital equipment again.
- We typically see weakness in presidential election years as companies sort through uncertainty or slow down during those periods. With the recent events involving both presumed candidates, that uncertainty has increased, which makes it very difficult to guess what might happen. Our business is likely to be impacted by tariff policies in a negative way because some projects potentially will go away entirely. For businesses we serve that ship to other countries, we have already lost sales because it is more affordable to build those products outside the U.S. for consumption outside the U.S. That was not the case before tariffs entered the equation. We were able to build products for shipment to other parts of the globe. Both [political] parties have shown a willingness to maintain and increase tariffs, which could negatively impact our sales.
- We are expecting to see stronger signs of a cyclical recovery in industrial and automotive markets, which have not materialized, yet. This is leading to higher uncertainty that the recovery may be delayed or muted.
- While we still have a large order backlog, new orders are significantly below where we forecasted them to be this year. This will shorten lead times and should allow us to pick up additional orders next year.
- Customer demand is softening. We are also experiencing increasing unfair competition directly from China.
- Due to the hurricane and not having power for over a week, we had significant lost production hours.
Historical Data
Historical data can be downloaded dating back to June 2004.
Download indexes for all indicators. For the definitions of all variables, see Data Definitions .
Download indexes and components of the indexes (percentage of respondents reporting increase, decrease, or no change). For the definitions of all variables, see Data Definitions .
Questions regarding the Texas Manufacturing Outlook Survey can be addressed to Laila Assanie at [email protected] .
Questions regarding the Texas Business Outlook Surveys can be addressed to Jesus Cañas at [email protected] .-->
Sign up for our email alert to be automatically notified as soon as the latest Texas Manufacturing Outlook Survey is released on the web.
Cookies on the Gambling Commission website
The Gambling Commission website uses cookies to make the site work better for you. Some of these cookies are essential to how the site functions and others are optional. Optional cookies help us remember your settings, measure your use of the site and personalise how we communicate with you. Any data collected is anonymised and we do not set optional cookies unless you consent.
You've accepted all cookies. You can change your cookie settings at any time.
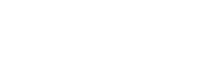
Statistics and research release
Statistics on gambling participation – Annual report Year 1 (2023): Official statistics
Findings from the Gambling Survey for Great Britain: Statistics on gambling participation, experiences of and reasons for gambling, and consequences from gambling.
Statistics Statistics and research hub
Collection Consumer gambling behaviour
Series Gambling Survey for Great Britain
Published on 25 July 2024
Find out more about the Gambling Survey for Great Britain
Find out more about Official statistics
Also published recently
- Statistics on gambling participation – Year 1 (2023), wave 2: Official statistics
- Statistics on gambling participation – Year 1 (2023), wave 1: Official statistics
Additional data sets in this series
The data being released today contains findings from the first year of the Gambling Survey for Great Britain (GSGB). The survey aims to collect data to enable us to further understand:
- who participates in gambling
- what type of gambling activities they participate in
- experiences of and reasons for gambling
- the consequences that gambling can have on individuals and others close to them.
This survey was conducted using a push-to-web approach, with data collected from 9,804 adults aged 18 years and older living in Great Britain. Fieldwork was carried out between July 2023 and February 2024, consisting of two waves. The survey is commissioned by the Gambling Commission and carried out by the National Centre for Social Research in collaboration with the University of Glasgow.
The new push-to-web methodology of this survey means that estimates presented in this report are not directly comparable with results from prior gambling or health surveys and such comparisons should not be used to assess trends over time. The GSGB data outlined in this report represents the first year of a new baseline, against which future changes can be compared.
The GSGB, like most other surveys, collects information from a sample of the population. Statistics based on surveys are estimates, rather than precise figures, and are subject to a margin of error (a 95 percent confidence interval). Generally, the larger the sample the smaller the margin of error.
Further details on the GSGB methodology can be found in the GSGB technical report .
All surveys have strengths and limitations and we have outlined the strengths and limitations of our approach in the data analysis and reporting section of the technical report . We have also published guidance on how to use the statistics from the GSGB.
Participation
Nearly half (48 percent) of participants aged 18 and over participated in any form of gambling in the past four weeks. Gambling participation was 27 percent when those who only participated in lottery draws were excluded.
Participants were more likely to gamble online than gamble in person (37 percent and 29 percent respectively), however, much of this difference was accounted for by people purchasing lottery tickets online. When lottery draws are removed, 18 percent of participants had gambled in person, compared with 15 percent online.
The mean number of activities for those who had participated in gambling in the past 4 weeks was 2.2 activities. The most commonly reported activities were the National Lottery (31 percent), buying tickets for other charity lotteries (16 percent), and buying scratchcards (13 percent).
Experiences of and reasons for gambling
When asked to rate their feelings towards gambling out of 10, where 10 represented that they loved it, and 0 represented that they hated it. 41 percent of adults who gambled in the past 12 months rated the last time they gambled with a positive score of between 6 and 10, 37 percent gave a score of 5, expressing that they neither loved or hated it, and 21 percent gave a negative score of between 0 and 4. When participation in lottery draws was excluded, the pattern was similar with a higher proportion giving a positive score (50 percent between 6 and 10, 31 percent a neutral score of 5, and 19 percent a negative score between 0 and 4).
The most common reasons for adults to participate in gambling were for the chance of winning big money (86 percent), because gambling is fun (70 percent), to make money (58 percent) and because it was exciting (55 percent). Those aged 18 to 24 were the only age group where gambling because it was fun (83 percent) was more common than gambling to win big money (79 percent).
Consequences from gambling
Problem Gambling Severity Index (PGSI) scores for specific activities are shown as relative differences which can be higher or lower than the average for all people who had gambled in the past 12 months. Our data shows that those who had bet on non-sports events in person, were over 9 times more likely than the average for all past 12-month gamblers to have a PGSI score of 8 or more. Those who had gambled on online slots were more than six times more likely than average to have a PGSI score of 8 or more.
Of all adults who had gambled in the past 12 months, the most reported severe consequence was relationship breakdown due to own gambling (1.6 percent), whilst the most frequently reported potential adverse consequences (happening at least occasionally) were reducing spending on everyday items (6.6 percent), lying to family (6.4 percent) and feeling isolated (5.5 percent).
The survey allows us to look at the relationship between PGSI and consequences from own gambling, something that has not been previously possible. The data shows that 41.3 percent of those with a PSGI score of 8 or more reported experiencing at least one of the severe consequences asked about. Equivalent estimates were 7.9 percent for those with a PGSI score of 3 to 7, 1.4 percent for those with a PGSI score of 1 to 2, and 0.6 percent for those with a PGSI score of 0, demonstrating how experience of severe consequences can be experienced by individuals with a range of PGSI scores.
For the first time, the Commission has collected data on the consequences of someone else gambling. In the GSGB survey, nearly half (47.9 percent) of adults reported someone close to them gambled. The most reported severe consequence being relationship breakdown (3.5 percent). The most frequently experienced consequences were experiencing embarrassment, guilt or shame, experiencing of conflict or arguments and experiencing health problems, including stress and anxiety.
These statistics comprise our official statistics on gambling participation, experiences of and reasons for gambling and consequences from gambling.
Please note that the data presented from the GSGB is not comparable to previous gambling survey publications due to changes in the methodology.
The next quarterly publication in this series (wave 1, 2024) will be released on 12 September 2024.
The next GSGB annual release (2024 Annual publication) will be released in summer 2025.
Full publication and key information
View the GSGB Annual report (2023)
Publication produced by: National Centre for Social Research and the University of Glasgow.
Publication authors: Wardle, H., Ridout, K., Tipping, S., Wilson, H., Maxineanu, I., & Hill, S.
Responsible Statistician: Helen Bryce (Head of Statistics).
This publication is primarily for anyone who has an involvement or interest in the gambling industry including government, licensed operators, trade bodies, international regulators, journalists, academic researchers, financial institutions, statisticians, consumers and local authorities.
About the status of official statistics .
Data and downloads
GSGB Annual report (2023) data tables XLSX 176.1 kB
GSGB Annual report (2023) supplementary data tables - country and region XLSX 113.2 kB
We are always keen to hear how these statistics are used and would welcome your views on this publication.
Give us feedback about these statistics (opens in a new tab) .
We are not able to respond to comments but your feedback will help us improve our website.
Complaints and queries
Do not use this form for complaints as we will not reply. If you want to complain about a gambling business or need further help please contact us .
User research
If you want to provide feedback about new services and features, join our user research programme .
This form is protected by reCAPTCHA and the Google Privacy Policy (opens in new tab) and Terms of Service (opens in new tab) apply.

COMMENTS
Chapter 9 Survey Research. Survey research a research method involving the use of standardized questionnaires or interviews to collect data about people and their preferences, thoughts, and behaviors in a systematic manner. Although census surveys were conducted as early as Ancient Egypt, survey as a formal research method was pioneered in the ...
Survey research means collecting information about a group of people by asking them questions and analyzing the results. To conduct an effective survey, follow these six steps: Determine who will participate in the survey. Decide the type of survey (mail, online, or in-person) Design the survey questions and layout.
Survey research is a research method involving the use of standardised questionnaires or interviews to collect data about people and their preferences, thoughts, and behaviours in a systematic manner. Although census surveys were conducted as early as Ancient Egypt, survey as a formal research method was pioneered in the 1930-40s by sociologist Paul Lazarsfeld to examine the effects of the ...
Survey research is a quantitative and qualitative method with two important characteristics. First, the variables of interest are measured using self-reports. In essence, survey researchers ask their participants (who are often called respondents in survey research) to report directly on their own thoughts, feelings, and behaviours.
What Is Survey Research? Survey research is a quantitative approach that has two important characteristics. First, the variables of interest are measured using self-reports. In essence, survey researchers ask their participants (who are often called respondents in survey research) to report directly on their own thoughts, feelings, and behaviors.
Chapter 9: Survey Research. 9.1 Overview of Survey Research. 9.2 Constructing Survey Questionnaires. 9.3 Conducting Surveys. Previous: 8.3 Complex Correlational Designs.
Chapter 3SURVEY RESEARCHA survey is a method of collecting. data in a consistent way. Survey research is useful for doc-umenting existing community conditions, characteristics of a populati. n, and community opinion. In this chapter, you will find an outline of the steps needed to conduct surveys using both the questionna.
What Is Survey Research? Survey research is a quantitative and qualitative method with two important characteristics. First, the variables of interest are measured using self-reports (using questionnaires or interviews). In essence, survey researchers ask their participants (who are often called respondents in survey research) to report directly on their own thoughts, feelings, and behaviors.
Abstract. The purpose of this chapter is to provide an easy-to-understand overview of several important concepts for selecting and creating survey instruments for dissertations and other types of ...
Chapter 9: Survey Research. Shortly after the terrorist attacks in New York City and Washington, DC, in September of 2001, researcher Jennifer Lerner and her colleagues conducted an Internet-based survey of nearly 2,000 American teens and adults ranging in age from 13 to 88 (Lerner, Gonzalez, Small, & Fischhoff, 2003)[1]. They asked ...
The third chapter offers a brief introduction into survey research. In the first part of the chapter, students learn about the importance of survey research in the social and behavioral sciences, substantive research areas where survey research is frequently used and important cross-national surveys such as the World Value Survey and the European Social Survey.
Survey research is a quantitative and qualitative method with two important characteristics. First, the variables of interest are measured using self-reports (using questionnaires or interviews). In essence, survey researchers ask their participants (who are often called respondents in survey research) to report directly on their own thoughts ...
A survey is a research method, the purpose and aims of which have already been stated; although data collection must be standardized, there are options for data collection within a survey. A questionnaire is a very specific data collection technique, which can be used within a variety of research methods. A survey, then, is the research method ...
6. Highlight the most common errors related to survey research. 7. Discuss the key ethical issues in survey research. 1. Identify the circumstances that make survey research an appropriate methodology.2. List the different methods for improving survey questions, along with the mistakes you do not want to make when writing questions.3.
Survey research is the process of collecting data from a predefined group (e.g. customers or potential customers) with the ultimate goal of uncovering insights about your products, services, or brand overall.. As a quantitative data collection method, survey research can provide you with a goldmine of information that can inform crucial business and product decisions.
This chapter describes a research methodology that we believe has much to offer social psychologists in- terested in a multimethod approach: survey research. Survey research is a specific type of field study that in- volves the collection of data from a sample of ele- ments (e.g., adult women) drawn from a well-defined
Rarely taught topics, such as how to enter and clean data, offer students information missed in both research methods and statistics courses. Shows how to write up survey results for academic, business and nonprofit reports to alleviate the confusion students feel about how to write up findings.
Survey research is a method of gathering information from a sample of individuals through interviews and systematic sampling. It aims to identify distributions of societal characteristics and make inferences about larger groups or populations. ... In addition to the references cited throughout the chapter the reader is referred to Alreck and ...
Surveys involve asking a series of questions to participants. They can be administered online, in person, or remotely (e.g. by post/mail). The data collected can be analysed quantitatively or qualitatively (or both). Researchers might carry out statistical surveys to make statistical inferences about the population being studied.
The Field Research Corporation is a widely-respected survey research firm and is used extensively by the media, politicians, and academic researchers. Since a survey can be no better than the quality of the sample, it is essential to understand the basic principles of sampling.
Survey research is a quantitative method whereby a researcher poses some set of predetermined questions to an entire group, or sample, of individuals. Survey research is an especially useful approach when a researcher aims to describe or explain features of a very large group or groups.
The chapter concludes with an overview of the principles of questionnaire design, including question order, instructions, and the use of existing measures, and compares the various methods of questionnaire administration, including group administration, online surveys, telephone interviews, and in-person interviews. This chapter provides an ...
The Pew Research Center survey conducted June 5-11, 2023, on the Center's American Trends Panel, used two separate questions to measure personal and household ownership. About a third of adults (32%) say they own a gun, while another 10% say they do not personally own a gun but someone else in their household does.
This chapter offers a brief introduction into survey research. In the first part of the chapter, students learn about the importance of survey research in the social and behavioral sciences, substantive research areas where survey research is frequently used, and important cross-national survey such as the World Values Survey and the European ...
An older survey from Lifeway Research found that about one-third of surveyed non-churchgoers were willing to accept invitations to church, according to the Christian Standard. Willingness seems to depend on the relationship between the inviter and person they invited. Someone is more willing to attend church for the first time with a close ...
In the analysis of government data in Chapter 4, references to those who do and do not have children include those who have or have not had biological children. ... These are among the key findings from a new Pew Research Center survey of 2,542 adults ages 50 and older who don't have children and 770 adults ages 18 to 49 who don't have ...
The scientific online survey of 2,050 people as a nationally representative sample was conducted by Leadership Studies researchers Dr. Lynn Shollen, Dr. Elizabeth Gagnon and Dr. Kat Callahan. The survey, entitled "Attitudes About Leadership in the United States," is the fourth annual version of a longitudinal research project based at ...
Project Overview The Rusty Patched Bumble Bee, and other native bees and pollinators, are declining due to climate change, habitat loss, and other stressors like pathogens and pesticide-use. Researchers supported by this Midwest CASC project will study how certain stressors interact to affect the geographic distribution of Rusty Patched Bumble Bees, using mapping techniques and future climate data
For this month's survey, Texas business executives were asked supplemental questions on labor market and financial conditions. Results for these questions from the Texas Manufacturing Outlook Survey, Texas Service Sector Outlook Survey and Texas Retail Outlook Survey have been released together. Read the special questions results.
In the GSGB survey, nearly half (47.9 percent) of adults reported someone close to them gambled. The most reported severe consequence being relationship breakdown (3.5 percent). The most frequently experienced consequences were experiencing embarrassment, guilt or shame, experiencing of conflict or arguments and experiencing health problems ...