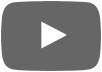

Sign up for the newsletter
Digital editions.
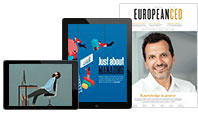
Total quality management: three case studies from around the world
With organisations to run and big orders to fill, it’s easy to see how some ceos inadvertently sacrifice quality for quantity. by integrating a system of total quality management it’s possible to have both.
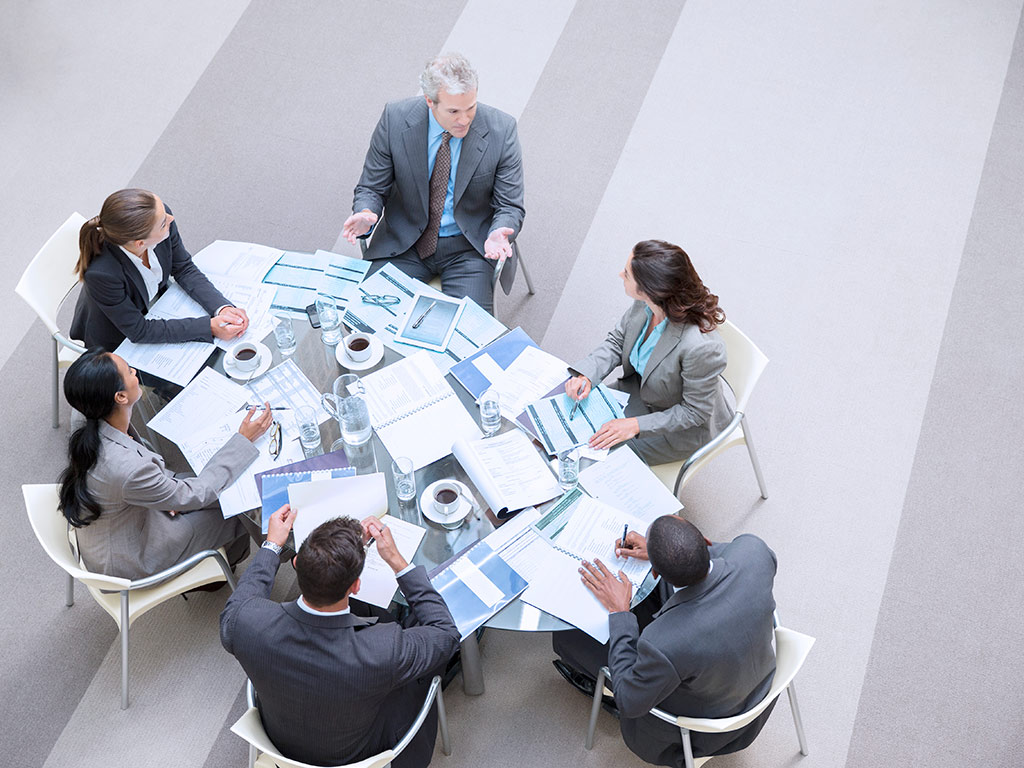
Top 5 ways to manage the board during turbulent times Top 5 ways to create a family-friendly work culture Top 5 tips for a successful joint venture Top 5 ways managers can support ethnic minority workers Top 5 ways to encourage gender diversity in the workplace Top 5 ways CEOs can create an ethical company culture Top 5 tips for going into business with your spouse Top 5 ways to promote a healthy workforce Top 5 ways to survive a recession Top 5 tips for avoiding the ‘conference vortex’ Top 5 ways to maximise new parents’ work-life balance with technology Top 5 ways to build psychological safety in the workplace Top 5 ways to prepare your workforce for the AI revolution Top 5 ways to tackle innovation stress in the workplace Top 5 tips for recruiting Millennials
There are few boardrooms in the world whose inhabitants don’t salivate at the thought of engaging in a little aggressive expansion. After all, there’s little room in a contemporary, fast-paced business environment for any firm whose leaders don’t subscribe to ambitions of bigger factories, healthier accounts and stronger turnarounds. Yet too often such tales of excess go hand-in-hand with complaints of a severe drop in quality.
Food and entertainment markets are riddled with cautionary tales, but service sectors such as health and education aren’t immune to the disappointing by-products of unsustainable growth either. As always, the first steps in avoiding a catastrophic forsaking of quality begins with good management.
There are plenty of methods and models geared at managing the quality of a particular company’s goods or services. Yet very few of those models take into consideration the widely held belief that any company is only as strong as its weakest link. With that in mind, management consultant William Deming developed an entirely new set of methods with which to address quality.
Deming, whose managerial work revolutionised the titanic Japanese manufacturing industry, perceived quality management to be more of a philosophy than anything else. Top-to-bottom improvement, he reckoned, required uninterrupted participation of all key employees and stakeholders. Thus, the total quality management (TQM) approach was born.
All in Similar to the Six Sigma improvement process, TQM ensures long-term success by enforcing all-encompassing internal guidelines and process standards to reduce errors. By way of serious, in-depth auditing – as well as some well-orchestrated soul-searching – TQM ensures firms meet stakeholder needs and expectations efficiently and effectively, without forsaking ethical values.
By opting to reframe the way employees think about the company’s goals and processes, TQM allows CEOs to make sure certain things are done right from day one. According to Teresa Whitacre, of international consulting firm ASQ , proper quality management also boosts a company’s profitability.
“Total quality management allows the company to look at their management system as a whole entity — not just an output of the quality department,” she says. “Total quality means the organisation looks at all inputs, human resources, engineering, production, service, distribution, sales, finance, all functions, and their impact on the quality of all products or services of the organisation. TQM can improve a company’s processes and bottom line.”
Embracing the entire process sees companies strive to improve in several core areas, including: customer focus, total employee involvement, process-centred thinking, systematic approaches, good communication and leadership and integrated systems. Yet Whitacre is quick to point out that companies stand to gain very little from TQM unless they’re willing to go all-in.
“Companies need to consider the inputs of each department and determine which inputs relate to its governance system. Then, the company needs to look at the same inputs and determine if those inputs are yielding the desired results,” she says. “For example, ISO 9001 requires management reviews occur at least annually. Aside from minimum standard requirements, the company is free to review what they feel is best for them. While implementing TQM, they can add to their management review the most critical metrics for their business, such as customer complaints, returns, cost of products, and more.”
The customer knows best: AtlantiCare TQM isn’t an easy management strategy to introduce into a business; in fact, many attempts tend to fall flat. More often than not, it’s because firms maintain natural barriers to full involvement. Middle managers, for example, tend to complain their authority is being challenged when boots on the ground are encouraged to speak up in the early stages of TQM. Yet in a culture of constant quality enhancement, the views of any given workforce are invaluable.
AtlantiCare in numbers
5,000 Employees
$280m Profits before quality improvement strategy was implemented
$650m Profits after quality improvement strategy
One firm that’s proven the merit of TQM is New Jersey-based healthcare provider AtlantiCare . Managing 5,000 employees at 25 locations, AtlantiCare is a serious business that’s boasted a respectable turnaround for nearly two decades. Yet in order to increase that margin further still, managers wanted to implement improvements across the board. Because patient satisfaction is the single-most important aspect of the healthcare industry, engaging in a renewed campaign of TQM proved a natural fit. The firm chose to adopt a ‘plan-do-check-act’ cycle, revealing gaps in staff communication – which subsequently meant longer patient waiting times and more complaints. To tackle this, managers explored a sideways method of internal communications. Instead of information trickling down from top-to-bottom, all of the company’s employees were given freedom to provide vital feedback at each and every level.
AtlantiCare decided to ensure all new employees understood this quality culture from the onset. At orientation, staff now receive a crash course in the company’s performance excellence framework – a management system that organises the firm’s processes into five key areas: quality, customer service, people and workplace, growth and financial performance. As employees rise through the ranks, this emphasis on improvement follows, so managers can operate within the company’s tight-loose-tight process management style.
After creating benchmark goals for employees to achieve at all levels – including better engagement at the point of delivery, increasing clinical communication and identifying and prioritising service opportunities – AtlantiCare was able to thrive. The number of repeat customers at the firm tripled, and its market share hit a six-year high. Profits unsurprisingly followed. The firm’s revenues shot up from $280m to $650m after implementing the quality improvement strategies, and the number of patients being serviced dwarfed state numbers.
Hitting the right notes: Santa Cruz Guitar Co For companies further removed from the long-term satisfaction of customers, it’s easier to let quality control slide. Yet there are plenty of ways in which growing manufacturers can pursue both quality and sales volumes simultaneously. Artisan instrument makers the Santa Cruz Guitar Co (SCGC) prove a salient example. Although the California-based company is still a small-scale manufacturing operation, SCGC has grown in recent years from a basement operation to a serious business.
SCGC in numbers
14 Craftsmen employed by SCGC
800 Custom guitars produced each year
Owner Dan Roberts now employs 14 expert craftsmen, who create over 800 custom guitars each year. In order to ensure the continued quality of his instruments, Roberts has created an environment that improves with each sale. To keep things efficient (as TQM must), the shop floor is divided into six workstations in which guitars are partially assembled and then moved to the next station. Each bench is manned by a senior craftsman, and no guitar leaves that builder’s station until he is 100 percent happy with its quality. This product quality is akin to a traditional assembly line; however, unlike a traditional, top-to-bottom factory, Roberts is intimately involved in all phases of instrument construction.
Utilising this doting method of quality management, it’s difficult to see how customers wouldn’t be satisfied with the artists’ work. Yet even if there were issues, Roberts and other senior management also spend much of their days personally answering web queries about the instruments. According to the managers, customers tend to be pleasantly surprised to find the company’s senior leaders are the ones answering their technical questions and concerns. While Roberts has no intentions of taking his manufacturing company to industrial heights, the quality of his instruments and high levels of customer satisfaction speak for themselves; the company currently boasts one lengthy backlog of orders.
A quality education: Ramaiah Institute of Management Studies Although it may appear easier to find success with TQM at a boutique-sized endeavour, the philosophy’s principles hold true in virtually every sector. Educational institutions, for example, have utilised quality management in much the same way – albeit to tackle decidedly different problems.
The global financial crisis hit higher education harder than many might have expected, and nowhere have the odds stacked higher than in India. The nation plays home to one of the world’s fastest-growing markets for business education. Yet over recent years, the relevance of business education in India has come into question. A report by one recruiter recently asserted just one in four Indian MBAs were adequately prepared for the business world.
RIMS in numbers
9% Increase in test scores post total quality management strategy
22% Increase in number of recruiters hiring from the school
20,000 Increase in the salary offered to graduates
50,000 Rise in placement revenue
At the Ramaiah Institute of Management Studies (RIMS) in Bangalore, recruiters and accreditation bodies specifically called into question the quality of students’ educations. Although the relatively small school has always struggled to compete with India’s renowned Xavier Labour Research Institute, the faculty finally began to notice clear hindrances in the success of graduates. The RIMS board decided it was time for a serious reassessment of quality management.
The school nominated Chief Academic Advisor Dr Krishnamurthy to head a volunteer team that would audit, analyse and implement process changes that would improve quality throughout (all in a particularly academic fashion). The team was tasked with looking at three key dimensions: assurance of learning, research and productivity, and quality of placements. Each member underwent extensive training to learn about action plans, quality auditing skills and continuous improvement tools – such as the ‘plan-do-study-act’ cycle.
Once faculty members were trained, the team’s first task was to identify the school’s key stakeholders, processes and their importance at the institute. Unsurprisingly, the most vital processes were identified as student intake, research, knowledge dissemination, outcomes evaluation and recruiter acceptance. From there, Krishnamurthy’s team used a fishbone diagram to help identify potential root causes of the issues plaguing these vital processes. To illustrate just how bad things were at the school, the team selected control groups and administered domain-based knowledge tests.
The deficits were disappointing. A RIMS students’ knowledge base was rated at just 36 percent, while students at Harvard rated 95 percent. Likewise, students’ critical thinking abilities rated nine percent, versus 93 percent at MIT. Worse yet, the mean salaries of graduating students averaged $36,000, versus $150,000 for students from Kellogg. Krishnamurthy’s team had their work cut out.
To tackle these issues, Krishnamurthy created an employability team, developed strategic architecture and designed pilot studies to improve the school’s curriculum and make it more competitive. In order to do so, he needed absolutely every employee and student on board – and there was some resistance at the onset. Yet the educator asserted it didn’t actually take long to convince the school’s stakeholders the changes were extremely beneficial.
“Once students started seeing the results, buy-in became complete and unconditional,” he says. Acceptance was also achieved by maintaining clearer levels of communication with stakeholders. The school actually started to provide shareholders with detailed plans and projections. Then, it proceeded with a variety of new methods, such as incorporating case studies into the curriculum, which increased general test scores by almost 10 percent. Administrators also introduced a mandate saying students must be certified in English by the British Council – increasing scores from 42 percent to 51 percent.
By improving those test scores, the perceived quality of RIMS skyrocketed. The number of top 100 businesses recruiting from the school shot up by 22 percent, while the average salary offers graduates were receiving increased by $20,000. Placement revenue rose by an impressive $50,000, and RIMS has since skyrocketed up domestic and international education tables.
No matter the business, total quality management can and will work. Yet this philosophical take on quality control will only impact firms that are in it for the long haul. Every employee must be in tune with the company’s ideologies and desires to improve, and customer satisfaction must reign supreme.
Contributors
- Industry Outlook
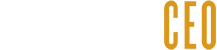
Digitization, automation, and online testing: Embracing smart quality control
In the next five to ten years, new technologies that characterize Industry 4.0—from connectivity to advanced analytics, robotics, and automation—have the potential to revolutionize every element of pharmaceutical quality control labs. The smart quality approach allows pharma companies to deploy these technologies and to integrate their quality controls in development and manufacturing. 1 A previous version of this article was originally published on January 4, 2019 as, “ Digitization, automation, and online testing: The future of pharma quality control .” The current updated version introduces more holistically the smart quality approach and outlines key success factors for its implementation. (see sidebar, “Smart quality at a glance”). Well-performing manufacturing facilities have started to create paperless labs, optimize testing, automate processes, and shift testing to the shop floor. These moves have enabled well-performing labs to substantially improve speed. These technologies typically boost productivity by between 50 to 100 percent. 2 Estimates in this article are based on McKinsey models leveraging proprietary POBOS pharmaceutical manufacturing and quality benchmarks, industry use cases, and diagnostic observations in quality control labs. Average-performing labs could achieve an even larger productivity improvement of 150 to 200 percent of their current rates.
About the authors
Beyond these effects on efficiency, digitization and automation specifically can also ensure better quality and compliance by reducing manual errors and variability. They enable faster and more effective problem resolution and a risk-based approach to optimizing testing volume, tools, and methods. In some cases digitization and automation have resulted in a more than 65 percent reduction in overall deviations and over 90 percent faster closure times. They can also prevent major compliance issues, which can in themselves be worth millions in cost savings. Furthermore, improved agility and shorter testing time can reduce lead times for quality control labs by 60 to 70 percent and eventually lead to real-time product releases.
Smart quality at a glance
“Smart quality” is a framework that pharma and medtech companies can apply to redesign key quality control processes and create value for the organization.
Smart quality has explicit objectives:
- to perceive and deliver on multifaceted and ever-changing customer needs
- to deploy user-friendly processes built organically into business workflows, reimagined with leading-edge technologies
- to leapfrog existing quality management systems with breakthrough innovation, naturally fulfilling the spirit—not just the letter—of the regulations
The new ways in which smart quality achieves its objectives can be categorized in five building blocks (exhibit).
To learn more about smart quality and how leading companies are reimagining the quality function, please see “ Smart quality: Reimagining the way quality works .”
However, few pharmaceutical companies have seen such significant benefits yet. This is usually due to the sometimes-significant upfront investments required, and the fact that some labs are simply not large enough to justify such investments. Many lack the granular performance and costing data necessary to build adequately sized digitization and automation business cases, and their efforts do not meet expectations for creating business value. Organizational silos and misaligned objectives between analytical method development and quality control labs often slow down innovation in both the mid- and long-term. In addition, since pharma product portfolios—and therefore pharma companies’ technological needs—evolve over time, it is sometimes hard to ascertain the right, clear, long-term lab-evolution strategy and blueprint needed for a clear long-term business case.
Before implementing and capturing benefits from new technologies, companies must first set clear goals, define robust business cases for any level of investment, and create rapid pilots of emerging technological solutions. Then, they must quickly scale-up the pilots that deliver promising results. To succeed, pharma companies need both the foresight to make long-term strategic investments, including those in R&D for developing and filing new test methods, and the agility to adapt those plans as technologies rapidly evolve.
Three horizons of lab evolution
Multiple digital and automation technologies have created opportunities for change in pharmaceutical laboratories, and this transformation typically evolves over three horizons (Exhibit 1). Most pharma labs have not yet achieved full technological transformation, but labs can start by aiming for one of the three future horizons of technological evolution. However, these horizons are not mutually exclusive and may not follow a linear path. In fact, pharma companies can create a compounding effect when they implement an element from another horizon at a different stage: for example, testing automation can be implemented in paper-based labs.
At the same time, some elements of one horizon may be a prerequisite for elements of another. For example, without having digitally enabled labs in place, a company would not be able to fully capture the benefits of automated labs.
Horizon 1: Digitally enabled labs
This horizon is comprised of the transition from manual data transcription and second-person verification to automatic data transcription between equipment and the laboratory information-management system (LIMS). Integrating quality control systems and sharing data with internal and external suppliers by automating data transcription creates better visibility and helps reduce risk. This integration also allow for targeted investments that improve the quality of inputs upstream, minimizing the need for often redundant raw-material testing, and accelerating the release of incoming materials.
Digitally enabled labs use advanced real-time data analytics for ongoing process verification to track trends and prevent deviations or out-of-specifications, and for optimizing scheduling and capacity management. These labs employ digital tools such as smart glasses to explain standard operating procedures with step-by-step visual guidance on how to execute a process. A digital twin can help predict impact before making physical changes to a lab. All these technologies have already been available for at least a few years, and the time to impact for each case can be as short as three months.
By becoming digitally enabled, an average chemical quality control lab could reduce costs by 25 to 45 percent, and an average microbiology quality control lab 15 to 35 percent. Productivity improvements come from two main sources:
- the elimination of up to 80 percent of manual documentation work and the requirement that two people must review everything (the four-eye principle);
- the automation, and especially optimization, of planning and scheduling to improve personnel, equipment, and materials utilization.
After the lighthouse plant of one large global pharma company transitioned to a digitally enabled lab, for example, their lab productivity jumped by more than 30 percent. 3 According to the World Economic Forum, lighthouses are “the world’s most advanced factories, which are leading the way in the adoption of Fourth Industrial Revolution technologies.” This increase was largely due to dynamic schedule optimization achieved with a modular and scalable digital-twin platform. The site also used advanced analytics to reduce deviations by 80 percent, eliminating reoccurring deviations altogether and accelerating deviation closure by 90 percent.
Horizon 2: Automated labs
In this horizon, pharma companies use robots, such as collaborative robots or other advanced automation technologies, to perform all repeatable tasks such as sample delivery and preparation and other lab-specific automation techniques. At the automated-lab stage, some high-volume testing (for example, instantaneous microbial detection in water for injection and air) can be performed online instead of physically in labs. Automated labs can also use predictive-maintenance technologies to plan for infrequent tasks such as large-equipment maintenance, which can be performed by lab analysts with remote expert support.
Automated labs can build upon a horizon 1 level of digitization to deliver greater value and higher cost savings. Automated microbiology labs can reduce costs beyond that achieved by digital enablement—10 to 25 percent savings inside the lab, while also capturing a similar amount of savings outside the lab. Likewise, in chemical labs automation can produce 10 to 20 percent incremental savings inside the lab.
The productivity improvements come from automating up to 80 percent of sample-taking and sample-delivery tasks and up to 50 percent of sample-preparation tasks. Improvements also come from reducing equipment-maintenance costs through remote monitoring and failure prevention. Outside the lab, automation reduces the number of sampling and related logistics tasks performed by operations, which saves the equivalent of up to 25 percent of lab costs for microbiology labs and up to 8 percent for chemical labs.
There are additional benefits: remote-monitoring and predictive-maintenance capabilities built into the equipment will decrease downtime and ultimately enable companies to reduce their use of expensive devices, such as chromatographs, near-infrared spectrometers, and isolators. By shifting to instantaneous microbial detection for environmental monitoring, companies may also reduce their overall lab lead time by 40 to 75 percent.
Technologies already exist—in healthcare and research labs and in manufacturing operations—that can be adapted to pharma quality control labs in a relatively straightforward way to reach the automated-lab horizon. Vendors offer solutions such as sample-distribution systems, online and instantaneous microbial-testing systems, automated sample-preparation stations, workflow optimization with visual guidance, and remote equipment monitoring and assisted maintenance. Next-generation analytical testing technology such as Ultra Performance Liquid Chromatography (UPLC) and Raman spectroscopy, often offer a more industrialized design with additional automation features or design for more and faster throughput.
Since 2018, additional technologies have emerged, such as remote equipment monitoring to reduce downtime and failures, advanced sterility testing techniques, and advanced analytics for faster and more effective lab investigations. Moreover, parallel to the evolution in the general robotics and collaborative robot space, lab-automation technology has become more cost effective.
Horizon 3: Distributed quality control
The third horizon represents a true disruption to traditional quality control, where nearly all routine product testing takes place on the production line, enabling real-time release testing (RTRT). To date, pharmaceutical manufacturing facilities have been slow to adopt process analytical technology and RTRT—both essential to Horizon 3—due to complex regulatory requirements. To be able to make a smooth shift to online testing in the future, operations need to start collaborating with R&D in earlier development stages to define an optimal quality control and filing strategy, especially for new products and manufacturing sites. At distributed quality control facilities, equipment and robots have artificial-intelligence capabilities, and labs continue to perform specialty and stability testing. This testing can take place on- or off-site, such as in a centralized and highly automated location.
Distributed quality control facilities add value by significantly reducing the physical footprint and costs of a traditional lab and accelerating product release. Because of the significant R&D-investment requirements, as well as the need for equipment and operational changes, existing sites with stable or declining volumes are unlikely to make a compelling business case for distributed quality control in the short and even medium term. However, sites that have been rapidly growing or under construction may be able to capture significant value from reducing capital-expenditure investment for building or expanding traditional quality control labs if they can move a significant share of routine testing online. Distributed quality control and real-time release, as well as supplier-produced certificates of analysis as a form of distributed quality control, would also enable true continuous-manufacturing processes.
As pharma companies start exploring ways to build distributed quality control facilities, they may be able to pull in relevant technologies from adjacent spaces. For example, platforms that can provide the advanced process control necessary to enable parametric release are now available. Meanwhile, artificial-intelligence systems could allow pharma companies to automate tasks that historically have been performed by highly trained expert employees.
The COVID-19 pandemic has levied new constraints on quality control operations—such as social-distancing requirements in often-crowded laboratories, restrictions on having analysts present on-site, and requirements to document all close, interpersonal contact for contact-tracing purposes. A “smart” approach to quality controls offers practical solutions to manage testing with fewer analysts present in the labs, as more work can be completed remotely (Exhibit 2).
Key success factors in implementing a smart quality control approach
While pharma companies continue deploying traditional operational-excellence levers, they have an opportunity to do so in tandem with their journey toward smart quality. As they progress in their digital quality control transformations, most pharma companies face five challenges: clearly articulating a vision, defining a business case, scaling up with an agile approach, formulating a technology plan, and ensuring they have the change-management capabilities they’ll need to transform.
Developing a cross-functional vision
Quality control leaders often struggle to envision a comprehensive future state, such as what we describe in our horizon model, that combines a number of technologies and use cases to create the most impact. There are a few possible explanations for this phenomenon. First, in many organizations, analytical method development and operational quality control are separated so they often have different objectives, and even report into different functions. Second, quality control innovation projects are chronically deprioritized in favor of individual innovation projects in other areas, such as in production, or in favor of lab-digitization programs triggered by regulatory requirements that usually focus on compliance and data integrity. Third, most innovation focuses on the narrow parts within quality control (such as reducing incoming inspection and automating in-line testing) and therefore misses an opportunity to create end-to-end impact by redesigning the entire quality control approach.
To break existing silos and define a shared, cross-functional vision for delivering quality controls in a new way and that span the entire value chain, quality control leaders can start by sharing perspectives on possibilities offered by new technologies with their peers outside as well as within the quality control organization. Setting aspirational business-performance targets helps send the signal to the organization that change is imminent. Visiting other lighthouse quality control labs can showcase the potential benefits of amalgamating these innovative technologies and create further excitement within the organization.
Defining a compelling business case for the transformation
To build a compelling business case, companies must define the right set of use cases for each lab—and the cases work best when they are integrated. Note that the baseline cost and the impact of improvements may differ significantly for chemical labs versus microbiology labs, and therefore different sets of levers may need to be deployed. Also, organizations often define their use-case scope too narrowly. For example, scheduling automation can deliver 2 to 3 percent of the quality control cost savings, but automation plus dynamic scheduling optimization can yield a more than 20 percent efficiency improvement.
While most labs can make a solid business case for working toward the digitally enabled horizon 1, not all labs have sufficient volumes and operational setups to justify reaching horizons 2 (automation) and 3 (distributed quality control). For example, it could be hard to justify an investment in automating a smaller lab where the potential cost savings might be less than $200,000 a year, whereas the same investment at a large sterile-manufacturing facility with significant environmental-monitoring volumes would quickly generate positive ROI.
In our experience, a good early place to start is establishing both a clear, holistic performance baseline and a clear target state with the tailored use cases for each lab, and then launching use cases in waves. By deploying use cases in waves, companies can track how much value is captured along the way, and reinvest the savings toward the next set of use cases. This approach helps capture value faster and with lower initial investment.
Using an agile approach to design and scale up pilots
One common misstep is targeting a fully tested, potentially overly complex end-to-end future state that takes a long time to design and even longer to test and implement. An alternative approach that has been proven successful is based on the lighthouses established by the World Economic Forum to advance progress in scaling Fourth Industrial Revolution technologies: rapidly testing possible solutions, identifying high-value ones, then rapidly scaling them up to capture benefits faster. When companies take this approach, they can, for example, implement schedule automation and optimization quickly and start generating significant value even if a lab is not fully paperless nor fully digitized.
Companies who are most successful in their smart quality control journey focus on identifying the innovative tools that can have the greatest immediate impact, and then rolling them out quickly across multiple sites.
Creating well-considered plans and structures for rolling out new systems and technologies
While some use cases can be built using existing IT systems, many require additional investment into IT infrastructure. In extreme cases, it can take pharma companies several years and more than $100 million to implement a LIMS. Given this lengthy time frame and the fast pace of technological change, some of the LIMS capabilities are liable to become obsolete before they’re even rolled out across an entire network. A poor rollout can cost five to ten times more and take three to five times longer than a properly planned and executed investment. To avoid these issues, pharma companies need skilled resources, in the form of a project management office (PMO) and formal change-management programs for helping workers acquire new skills. A PMO will help accelerate technology rollout by eliminating the temptation to excessively customize technology at each site.
Investing in robust change-management capabilities
Digital transformation requires radical changes in mindset. This has major implications for the organization and for individual employees who must develop new skills and competencies. To succeed, companies must invest up-front effort in creating buy-in across the organization, defining and launching up-skilling and re-skilling programs, and forging strong links between business and IT functions.
For example, a typical pharma lab does not have the advanced analytical capabilities needed to get the maximum value from its data sources. As a result, the labs collect a lot of useful data, but fail to generate the insights that could prevent problems, improve test methods, or optimize testing volumes. It is critical to define the right operating model for impact. For example, when labs build internal skills for defining advanced analytics use cases, they can work with specialized advanced-analytics specialists, often outside of labs, who can execute the complex analyses and help gain deep insight into specific cases. Clearly understanding future capability needs, investing in training high-potential employees, and hiring employees with the new required skill sets (for instance, advanced data analytics) during early stages will enable faster scale-up. And where labs are unable to develop the right skills in house, they will need to effectively recruit talent from outside their organizations.
The latest technologies and digital solutions can make quality control faster, more agile, more reliable, more compliant, and more efficient. By setting appropriate goals, choosing the right technologies, and developing the right capabilities, pharma companies can transform the way they do quality control to deliver safer and more efficacious products in a cost-efficient way.
Explore a career with us
Related articles.

Making quality assurance smart

Smart quality: Reimagining the way quality works
- Browse All Articles
- Newsletter Sign-Up
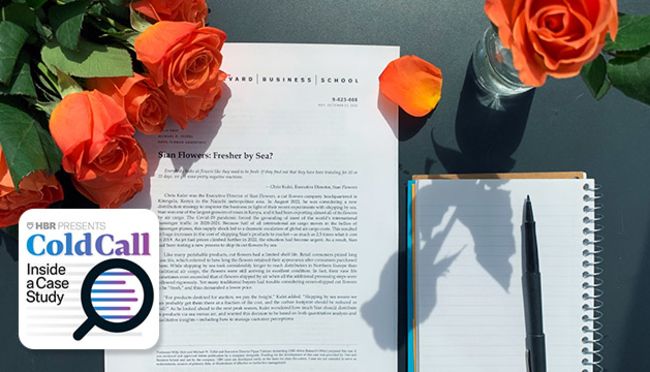
- 11 Apr 2023
- Cold Call Podcast
A Rose by Any Other Name: Supply Chains and Carbon Emissions in the Flower Industry
Headquartered in Kitengela, Kenya, Sian Flowers exports roses to Europe. Because cut flowers have a limited shelf life and consumers want them to retain their appearance for as long as possible, Sian and its distributors used international air cargo to transport them to Amsterdam, where they were sold at auction and trucked to markets across Europe. But when the Covid-19 pandemic caused huge increases in shipping costs, Sian launched experiments to ship roses by ocean using refrigerated containers. The company reduced its costs and cut its carbon emissions, but is a flower that travels halfway around the world truly a “low-carbon rose”? Harvard Business School professors Willy Shih and Mike Toffel debate these questions and more in their case, “Sian Flowers: Fresher by Sea?”
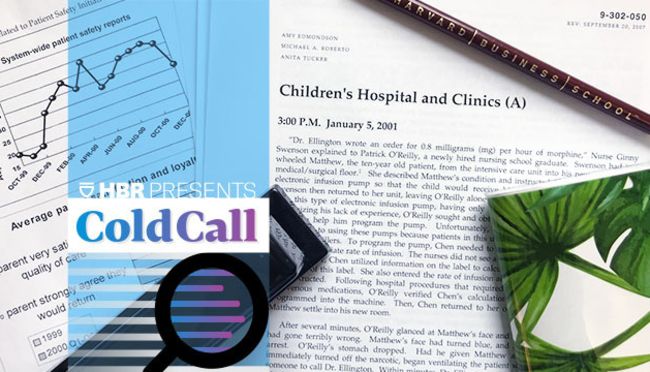
- 17 Sep 2019
How a New Leader Broke Through a Culture of Accuse, Blame, and Criticize
Children’s Hospital & Clinics COO Julie Morath sets out to change the culture by instituting a policy of blameless reporting, which encourages employees to report anything that goes wrong or seems substandard, without fear of reprisal. Professor Amy Edmondson discusses getting an organization into the “High Performance Zone.” Open for comment; 0 Comments.
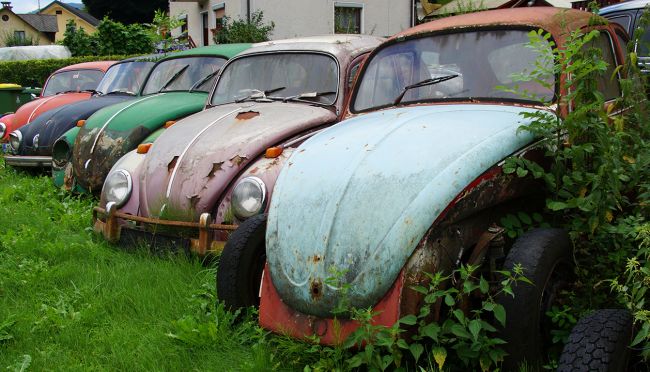
- 27 Feb 2019
- Research & Ideas
The Hidden Cost of a Product Recall
Product failures create managerial challenges for companies but market opportunities for competitors, says Ariel Dora Stern. The stakes have only grown higher. Open for comment; 0 Comments.
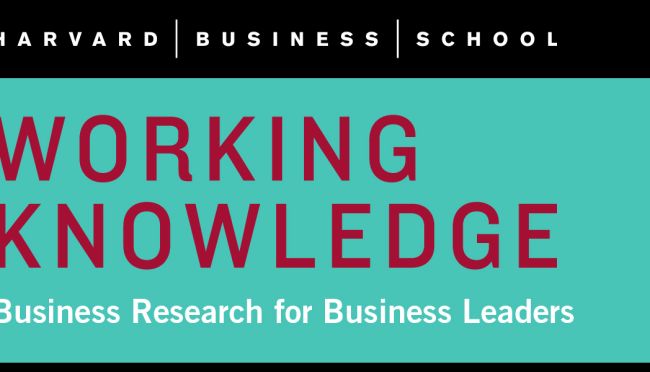
- 31 Mar 2018
- Working Paper Summaries
Expected Stock Returns Worldwide: A Log-Linear Present-Value Approach
Over the last 20 years, shortcomings of classical asset-pricing models have motivated research in developing alternative methods for measuring ex ante expected stock returns. This study evaluates the main paradigms for deriving firm-level expected return proxies (ERPs) and proposes a new framework for estimating them.
- 26 Apr 2017
Assessing the Quality of Quality Assessment: The Role of Scheduling
Accurate inspections enable companies to assess the quality, safety, and environmental practices of their business partners, and enable regulators to protect consumers, workers, and the environment. This study finds that inspectors are less stringent later in their workday and after visiting workplaces with fewer problems. Managers and regulators can improve inspection accuracy by mitigating these biases and their consequences.
- 23 Sep 2013
Status: When and Why It Matters
Status plays a key role in everything from the things we buy to the partnerships we make. Professor Daniel Malter explores when status matters most. Closed for comment; 0 Comments.
- 16 May 2011
What Loyalty? High-End Customers are First to Flee
Companies offering top-drawer customer service might have a nasty surprise awaiting them when a new competitor comes to town. Their best customers might be the first to defect. Research by Harvard Business School's Ryan W. Buell, Dennis Campbell, and Frances X. Frei. Key concepts include: Companies that offer high levels of customer service can't expect too much loyalty if a new competitor offers even better service. High-end businesses must avoid complacency and continue to proactively increase relative service levels when they're faced with even the potential threat of increased service competition. Even though high-end customers can be fickle, a company that sustains a superior service position in its local market can attract and retain customers who are more valuable over time. Firms rated lower in service quality are more or less immune from the high-end challenger. Closed for comment; 0 Comments.
- 08 Dec 2008
Thinking Twice About Supply-Chain Layoffs
Cutting the wrong employees can be counterproductive for retailers, according to research from Zeynep Ton. One suggestion: Pay special attention to staff who handle mundane tasks such as stocking and labeling. Your customers do. Closed for comment; 0 Comments.
- 01 Dec 2006
- What Do You Think?
How Important Is Quality of Labor? And How Is It Achieved?
A new book by Gregory Clark identifies "labor quality" as the major enticement for capital flows that lead to economic prosperity. By defining labor quality in terms of discipline and attitudes toward work, this argument minimizes the long-term threat of outsourcing to developed economies. By understanding labor quality, can we better confront anxieties about outsourcing and immigration? Closed for comment; 0 Comments.
- 20 Sep 2004
How Consumers Value Global Brands
What do consumers expect of global brands? Does it hurt to be an American brand? This Harvard Business Review excerpt co-written by HBS professor John A. Quelch identifies the three characteristics consumers look for to make purchase decisions. Closed for comment; 0 Comments.
Using machine learning prediction models for quality control: a case study from the automotive industry
- Original Paper
- Open access
- Published: 16 March 2023
- Volume 20 , article number 14 , ( 2023 )
Cite this article
You have full access to this open access article
- Mohamed Kais Msakni ORCID: orcid.org/0000-0003-3060-6970 1 ,
- Anders Risan 1 na1 &
- Peter Schütz 1 na1
3233 Accesses
Explore all metrics
This paper studies a prediction problem using time series data and machine learning algorithms. The case study is related to the quality control of bumper beams in the automotive industry. These parts are milled during the production process, and the locations of the milled holes are subject to strict tolerance limits. Machine learning models are used to predict the location of milled holes in the next beam. By doing so, tolerance violations are detected at an early stage, and the production flow can be improved. A standard neural network, a long short term memory network (LSTM), and random forest algorithms are implemented and trained with historical data, including a time series of previous product measurements. Experiments indicate that all models have similar predictive capabilities with a slight dominance for the LSTM and random forest. The results show that some holes can be predicted with good quality, and the predictions can be used to improve the quality control process. However, other holes show poor results and support the claim that real data problems are challenged by inappropriate information or a lack of relevant information.
Similar content being viewed by others
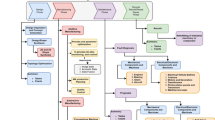
A comprehensive literature review of the applications of AI techniques through the lifecycle of industrial equipment
Mahboob Elahi, Samuel Olaiya Afolaranmi, … Jose Antonio Perez Garcia
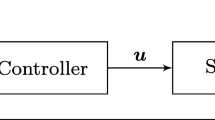
Review on model predictive control: an engineering perspective
Max Schwenzer, Muzaffer Ay, … Dirk Abel
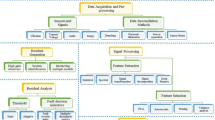
A review on fault detection and diagnosis techniques: basics and beyond
Anam Abid, Muhammad Tahir Khan & Javaid Iqbal
Avoid common mistakes on your manuscript.
1 Introduction
The emergence of the fourth industrial revolution, Industry 4.0, is primarily driven by advancements in information, communication, and intelligence technologies that can improve production flexibility, efficiency, and productivity in industry (Ibarra et al. 2018 ). While the definition of Industry 4.0 is broad, there are several key concepts associated with it, such as smart factories, the Internet of Things (IoT), cloud computing, cyber-physical systems, and Big Data Manufacturing (Santos et al. 2017 ). IoT technology enables to connect manufacturing resources, like sensors, machines, and other equipment, enabling interconnection between components and reducing human intervention. This also allows real-time, high-accuracy monitoring of product quality, equipment, and production processes. Real-time data flow can help identify problems early on and provide better visibility into the flow of materials and products. In addition, cloud computing makes data available to other systems with powerful resources, such as servers, storage, and software (Lee and Lee 2015 ). As many manufacturers have large amounts of data that go unused, cloud computing is seen as a way to transform the traditional manufacturing business model into an effective collaboration, helping manufacturers align business strategies and product innovation and create smart networks (Xu 2012 ). The amount of data collected from various systems and objects is growing at an exponential rate and is commonly referred to as Big Data. This concept is characterized by high dimensionality and high complexity due to the variety of formats, semantics, and quality of sensors and processes generating the data (Wuest et al. 2016 ). As a key concept in smart factories, Big Data can impact Industry 4.0 in three ways: enabling self-diagnosis, forecasting, and control (Tao et al. 2017 ). Conventional data processing software and technologies cannot fully leverage the potential of these large and complex datasets, and advanced methods such as machine learning algorithms are needed to organize and derive value from the data.
In the context of Industry 4.0, machine learning has been applied to different levels of the industrial process, such as anomaly detection, process optimization, predictive maintenance, quality control, diagnosis, and resource management (Roblek et al. 2016 ). Machine learning is seen as a promising improvement in manufacturing as it allows for decentralized, autonomous, and real-time decision-making without human interaction. It has the advantages of addressing large and complex processes and enabling continuous quality improvement (Dogan and Birant 2021 ). Unlike conventional algorithms, machine learning algorithms can dynamically learn from the system and automatically adapt to changes in the environment. It can also detect patterns and implicit knowledge from the data, improving existing processes and methods in manufacturing (Wuest et al. 2016 ). However, the application of machine learning is not straightforward. The performance of these algorithms can be hindered by the acquisition of relevant data in terms of volume and quality. On the one hand, the training data must be sufficiently numerous to reach the level of generalization, for which the learning model also performs well on new (unseen) data. On the other hand, the data may either contain inappropriate and redundant information or lack relevant information, as not all data is captured during the manufacturing process, and some attributes may not be available. Data preprocessing, which includes selecting relevant inputs and normalizing the data (Wuest et al. 2016 ), is also an important step before learning. The challenges of machine learning are not only limited to data but also include the algorithm itself. Some machine learning algorithms are more appropriate for specific applications, and the performance of some of them depends on selecting suitable hyperparameter settings. Despite these challenges, machine learning algorithms have the capacity to extract new information and provide better results than conventional algorithms.
One of the advances offered by Industry 4.0 is the opportunity to improve quality control in manufacturing. Traditionally, manufacturers have used Statistical Process Control (SPC) to ensure that product features are defect-free and meet specifications. SPC is based on the statistical assumption that random factors, such as humidity, temperature changes, and variations in raw material, tend to form a normal distribution centered on the quality characteristics of the product (e.g., length, weight, and hardness). Thus, the process is under statistical control, which allows for analyzing the outputs and the capability of the process. SPC provides tools and techniques for monitoring and exploring the process behavior and identifying anomalies (Tao et al. 2017 ; Oakland and Oakland 2018 ). With the technological capabilities of Industry 4.0, SPC can be supplemented to improve quality control further. Big data and cloud computing can use real-time data to detect quality defects and process instability at an early stage. For example, Gokalp et al. ( 2017 ) describe real-time data analysis to self-calibrate a process when a deviation in the trajectory of an ongoing machining process. In addition, machine learning can use time-series data of process and product variables to identify patterns and detect early process deviations so that preventive measures can be taken and the production process is stabilized.
This paper investigates the use of machine learning algorithms to predict product quality in manufacturing in order to support quality control. The focus is on bumper beams, which are an essential component of automotive crash management systems and are subject to strict quality control. The goal is to improve the quality control process in production by predicting the quality of future products, allowing for early adjustments, and reducing scrap production and downtime in the production system. The machine learning algorithms used in this study are based on neural networks and random forests. They are trained on historical data consisting of previously produced and measured parts provided by the manufacturer. The effectiveness of the neural network and random forest models is compared and evaluated for their ability to predict key product characteristics important for quality control. This work differs from previous research in that it develops machine learning models that use previously measured products to predict the quality of the next product rather than using the real-time state of the system to predict the quality of the current part.
The outline of the remainder of this paper is as follows. Section 2 discusses machine learning for quality control in manufacturing systems and presents related works in the literature. Section 3 introduces the concept of time series and relates it to process control and machine learning prediction models. The case study of this paper is discussed in Sect. 4 . Section 5 shows the implementation and the obtained performance of the learning models. Finally, Sect. 6 gives a conclusion to this paper.
2 Related works
Maintaining high-quality products and processes is essential for success in a competitive environment. In manufacturing, product quality is related to the functional aspects of the product that must be out of defects and out-of-tolerance conditions. The process of ensuring that any manufactured product meets the requirements is called quality control. If a product does not satisfy the requirements, it is considered a poor-quality product and will be removed from the production line. Many factors can cause quality to vary in the production process, such as humidity, temperature, and variations in raw materials and tools.
As technology advances and data becomes more available, new ways to perform more accurate, real-time quality control are emerging. Machine learning has already been successfully applied to tasks involving quality control and quality assessments and is expected to further improve the field of quality control in the future (Wuest et al. 2016 ). Collected data can be analyzed by learning algorithms in two ways (Tao et al. 2018 ). The first is to monitor the process in real-time to ensure product quality; for example, a deviation in tool trajectory can be detected using real-time analysis, and the process can be adjusted according to the requirements. The second is to identify emerging problems. Using historical data, the learning algorithms can identify patterns or predict the output characteristics of a process, enabling early detection of faulty products.
The neural network is one of the most widely used machine learning algorithms for process and quality control in manufacturing environments. Most of the applications are related to real-time analysis for process control or detection of defective products by image recognition. Karayel ( 2009 ) uses a feedforward neural network as an observer for a control system by predicting surface roughness in a computer numerical control (CNC) lathe. The prediction model uses process parameters as input data, i.e., cutting depth, cutting speed, and feed rate, to predict surface roughness. This prediction is later sent to the controller to determine the best cutting parameters for the CNC turning system. A similar network structure is used by Tsai et al. ( 1999 ) to predict real-time surface roughness in milling cutting operations. The model uses process parameters that consist of vibration measures, depth of cut, speed of the axis, and rotation and feed speed. Martin et al. ( 2007 ) propose a supervised feedforward neural network to replace a human expert in the quality control process of resistance spot welding. The machine learning model uses ultrasonic oscillograms to classify the quality spot welds into one of the six predefined levels. Zhao et al. ( 2020 ) use power signals to predict the nugget diameter from spot-welded joints in a real-time prediction system. The paper compares the performance of a regression model and feedforward network in monitoring weld quality and shows that the latter model provides better performance. To detect defects in parts, Wang et al. ( 2018 ) propose a deep convolutional neural network that uses raw images of flat surfaces to automatically extract product features for defect detection and improve production efficiency. For geometrically complex products (such as turbo blades), Wang et al. ( 2020 ) develop a similar model architecture in a cloud-based platform to meet the high-speed performance required by complex product images. Risan et al. ( 2021 ) develop a feedforward neural network to predict the location of a milled hole in a bumper beam.
Other works in the literature focus on prediction models to support quality control using random forest algorithms. With respect to the machining process, most works use process parameters as input variables for prediction models such as feed rates, tool wear, and drive power. Bustillo et al. ( 2021 ) investigate the prediction of surface flatness deviations in a face milling process using different machine learning algorithms. The input data consists of tool life and wear and drive power. The problem is first designed and evaluated as a regression problem and then as a classification problem using discretized flatness levels. For the regression problem, the random forest is outperformed by the two artificial neural networks, a multilayer perceptrons network and a radial basis functions network. However, when the classification problem is considered, the random forest gives the most accurate predictions. Wu et al. ( 2018 ) develop a random forest model to predict the surface roughness in fused deposition modeling. The input data is mainly based on the temperature and vibration of the table and extruder. The model can predict the surface roughness of a printed part with very high accuracy. Bustillo et al. ( 2018 ) propose different machine learning algorithms for surface roughness and loss-of-mass predictions in machining processes. The models studied include regression trees, multilayer perceptrons, radio basis networks, and random forest. The experiments show that multilayer perceptrons achieve the best surface roughness prediction. However, the random forest has the advantage of being more suitable for industrial use in the absence of experts in machine learning as this model has a non-parametric property. Agrawal et al. ( 2015 ) develop a multiple regression model and a random forest model for the prediction of surface roughness during hard turning of a hardened steel piece. Both models use the same cutting parameters as input variables. The results show better surface predictions for the random forest model. Li et al. ( 2019 ) use an ensemble of machine learning models to predict the surface roughness for an extrusion-based additive manufacturing process. A random forest is used to reduce the input variable size to improve the computational efficiency and avoid overfitting. Then, an ensemble of different machine learning models is used for surface roughness prediction.
Quality control can also be enhanced using time series data and prediction models. Ma et al. ( 2022 ) proposed a soft sensor model for quality prediction of industrial products using time series data and process features. The model framework is based on a neighborhood dimension reduction and a bidirectional gated recurrent unit. Shohan et al. ( 2022 ) use time series modeling to help improve the prediction quality of biofabrication process. Standard autoregressive time series models and machine learning models were tested. Experiments showed that the Long-Short Term Memory model provides the best performance in terms of mean square errors. In another application, Freeman et al. ( 2018 ) implement deep learning techniques in the prediction of air quality. The data consists of time series events of hourly air quality and meteorological events. Kim et al. ( 2022 ) use a descriptive time series analysis to predict downtime of a linear medical accelerator by using long-term maintenance data. Meng et al. ( 2022 ) deploy a deep learning model using time series data of historical images and image recognition of plants. The objective is to improve the quality by helping have healthy plants with high yields. Prediction using time series data is not limited to quality improvement but covers a wide range of applications. Many works have recently emerged to predict COVID-19 transmission using time series and deep learning models (Long Short Term Memory networks and Gated Recurrent Units), e.g., Rauf et al. ( 2021 ); Ayoobi et al. ( 2021 ).
3 Methodology
Time series data, which consists of observations recorded at specific times, is often available in manufacturing processes and equipment. It is then important to exploit these data to extract valuable information for the manufacturers. This task corresponds to finding a model that describes a time series. This model estimates the relationship between the variable of interest Y and the input variables X using a function f . While various approaches can be applied, i.e., physical, statistical, and machine learning models, the nonlinear and high-dimensional aspects of manufacturing systems make it very difficult to develop a satisfactory model for estimating f . Despite this challenge, developing a time series model has several advantages, such as a compact description of the time series, hypothesis testing, separation and filtering of noise from data, and time series prediction (Brockwell and Davis 2016 ).
3.1 Times series and statistical process control
In the context of quality control, SPC is a widely used method that involves process capability and statistical analysis of process results. These methods rely on monitoring and analyzing the product features relevant to product quality. By using samples of a specific size from the process, causes for variation can be identified, and adjustments can be made (Groover 2019 ).
One of the primary techniques is the control chart, which offers a visualization way to study the evolution of a process over time. A time-series data is represented in a chart with a central line for the average, an upper line for the upper control limit, and a lower line for the lower control limit. These control limits are then compared to the actual data to see if the process variation is under control. When the process is under statistical control, the control limits are defined based on the process capability ( PC ), which provides information about the accuracy of a process’s performance over time and measures the ability of a process to meet its specifications (Oakland and Oakland 2018 ). It can be defined as:
where \(\mu \) is the mean of the process, and \(\sigma \) is the standard deviation. Thus, 99.73% of outputs of a controlled process are within \(3\sigma \) limits.
3.2 Machine learning prediction models
The main challenge of machine learning models is to establish a valid representation of the input data (the input variables X of the time series) by performing some transformation (the model or function f ) that can approximate the expected outcomes (the variable of interest Y ). The provided data set is commonly referred to as the “training set" and is used by machine learning algorithms to apply a predefined set of operations to build a model. Thus, machine learning models are not explicitly programmed to make decisions or predictions but are created during the learning stage. Machine learning has been successfully applied to a variety of problems across different domains, such as image recognition, anomaly detection, and quality control. In this work, two classes of machine learning algorithms are developed, namely neural networks and random forests, to predict the location of holes in future products. Since the locations are continuous values and the training set is composed of input and output variables, the problem is referred to as a regression problem with supervised learning. A general description of neural networks and random forests is given in the following subsections.
3.2.1 Neural networks
Neural networks are one of the most commonly known machine learning algorithms, and they have been successfully applied to a wide range of fields. This learning algorithm is inspired by a biological network of neurons, in which neurons are chemically connected to form an extensive network. In artificial neural networks, neurons are modeled as nodes and connections as weights. The role of weights is to computationally activate or deactivate a connection between two nodes: A positive weight indicates an active connection, whereas a negative weight prohibits the link between the nodes. A node receives many connections (weights) that are transformed into a single output. Typically, the neurons in a neural network are organized in layers. The first (input) layer passes the input data to the network without any transformation, and the last (output) layer consists of output variables. The hidden layers connect the input layer to the output layer and perform the data transformation using activation functions. The role of an activation function in the hidden layers is to transform the weighted sum of the input into an output that will be used in the following layers. A general structure of the feedforward network is illustrated in Fig. 1 .
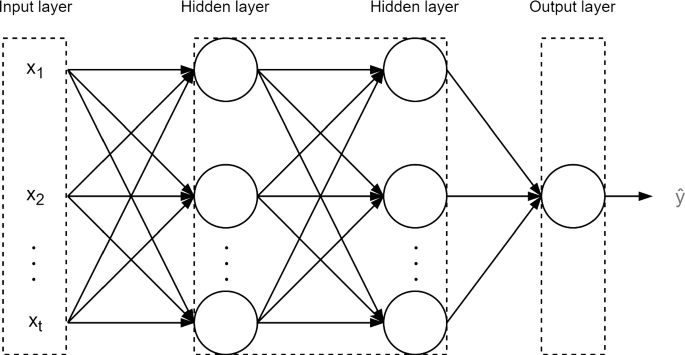
An example of a feedforward neural network with an input layer, two hidden layers, and one output layer with one target variable. Adapted from (Ketkar and Moolayil 2021 )
The layered representation of neurons can represent complex relationships between input and output data and extract complex patterns. Indeed, neural networks can model non-linear-statistical data and handle high-dimensional and multivariate data. However, they require more data than other machine learning models, and the best performance requires extensive customization as neural networks depend on several hyper-parameters. Also, neural networks do not provide any information about how the outputs are computed, a problem commonly referred to as the black box in machine learning (Ian et al. 2016 ).
Although neural networks are well suited for a large variety of problems, such as image recognition and text recognition, they suffer from a major issue known as the vanishing gradient problem, which prevents learning long-term dependencies (Rehmer and Kroll 2020 ). This makes it difficult to train standard neural networks on long data series (Kinyua and Jouandeau 2021 ). The vanishing gradient problem can be addressed by including gated units, such as the Long Short-Term Memory and the Gated Recurrent Unit (Rehmer and Kroll 2020 ).
3.2.2 Random forests
The random forest algorithm has become a widely used machine learning algorithm because of its simplicity and accuracy (Biau and Scornet 2016 ) and its ability to perform both supervised and unsupervised learning, as well as classification and regression problems (Genuer and Poggi 2020 ). This algorithm is a statistical learning method proposed by (Breiman 2001 ), based on the principles of ensemble learning. In machine learning, ensemble learning refers to the techniques of combining the predictions of a group of trained models (an ensemble). The idea is that by aggregating the outcomes of several models, the prediction of the ensemble is more likely to perform better than any individual model in the ensemble. For the random forest, the algorithm is trained on different and independent training subsets (bootstraps) to obtain several models, referred to as trees. Figure 2 illustrates a general structure of the random forest.
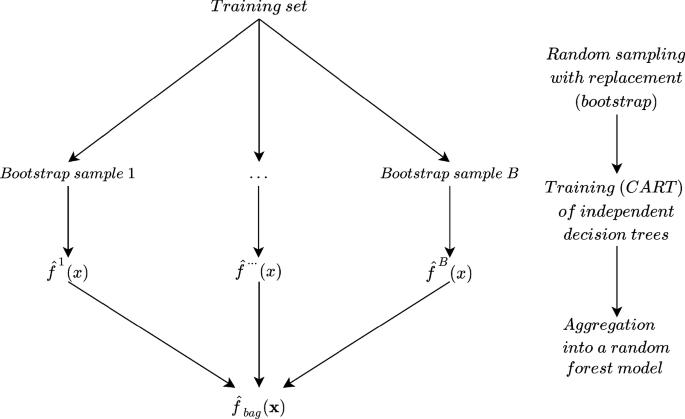
Flowchart of training a random forest tree and aggregation of results. Adapted from (Genuer and Poggi 2020 )
A decision tree is a predictive model with a tree-like structure where the decision progresses from the root node through internal nodes until it reaches a leaf. A node corresponds to a binary split of the predictor space to continue the decision flow in one of the two sub-trees of the node. A leaf in the decision tree represents a predicted value or class label, and the path to the leaf represents the classification rules. Such a decision representation makes decision trees readable and simple to interpret. Although there are different algorithms for building a decision tree, the classification and Regression Tree (CART) algorithm is widely used for random forests (James et al. 2013 ).
For a classification problem, the decision tree uses the input values to reach one of its leaves and, here, to find the predicted class. Similarly, a regression problem uses the same decision tree structure, with the difference that the leaves correspond to continuous target values. To make the decision trees independent of each other, they are trained on B randomly drawn and independent subsets of equal size. Each subset is used to train B decision trees that will finally be aggregated into a forest.
One advantage of the random forest algorithm is that it does not require heavy computation for training. It is easy to tune as it depends only on a few hyper-parameters. Another advantage is that it is suitable for high-dimensional problems with multivariate data where the number of variables far exceeds the number of observations, and vice versa (Géron 2019 ). However, the prediction quality of the random forest is highly dependent on the quality of the training set, e.g., it cannot predict values outside the minimum and maximum of the values in the training set.
4 A case study
The product studied in this paper is the bumper beam, a component of a crash management system in cars. The beam is formed from an extruded aluminum profile and is machined and cut before being assembled to the bumper with screws.
The beam is placed using clamps at predefined locations during the machining step. Then, the CNC machining starts with the milling of reference holes, which are of particular interest because they are used to locate and mill other holes by CNC machining. In total, there are 20 milled holes, each with narrow tolerance ranges regarding their location in the beam. Any displacement of the reference holes results in deviation of the connected holes. Quality control of the milled holes is performed after the machining process, when a new product is released, or at predefined intervals. The interval length between two quality controls is typically two hours and is considered by the manufacturer as satisfactory to guarantee high-quality standards while ensuring smooth production. During quality control, the geometric characteristics of all milled holes and the beam curvature are automatically measured in an XYZ grid system, resulting in a total of 144 different features. When the measurement report shows any deviation, the entire batch of products is rejected, and the production line is disrupted. The production batch since the last control is scrapped. An experienced operator makes the necessary changes to the machine settings. Then a new beam is machined, and another quality control is performed. The goal of the manufacturer is to reduce the downtime of the production as much as possible to minimize direct economic loss.
Many factors can cause variations in the CNC machining process, including both random variations such as clamping force, temperature, and variations in upstream activity, as well as assignable variations such as replacement of CNC parts and change in the beam type being processed. Unfortunately, not all of these variations are available to be considered as part of the input to the learning models.
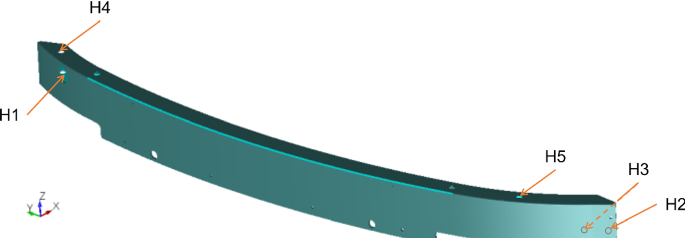
Illustration of the bumper beam shape and the locations of the five reference holes
Figure 3 illustrates the shape of the bumper beam and the locations of the reference holes. Two reference holes (H1 and H4) are located on the left side of the beam, and three other holes (H2, H3, and H5) are located on the right side of the beam. H1, H2, and H3 are located using the YZ coordinate system, whereas H4 and H5 are located using the XZ coordinate system. This work aims to improve the quality control of the milled holes by predicting the reference hole locations of the next product to be manufactured. Machine learning models are implemented to predict future hole positions, which can be used as a preventive measure to avoid out-of-tolerance products. Since this information is available, early adjustments can be made, and the production flow is smooth. The proposed learning models do not depend on real-time data, as is the case in many literature works, but consider a time series analysis that uses previous measures to predict the hole locations in the upcoming product. Historical data from all available measurements is used as input to train the models. The target variables are the coordinates of all reference holes.
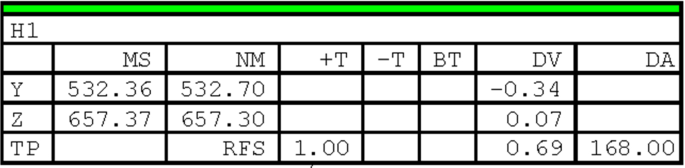
A table from a control report of the studied beam showing the measurements of the reference hole H1
Figure 4 shows an example of the measurements and data collected for the reference hole H1. This hole is located using a measured value (MS) and a nominal value (NM). The deviation (DV) of H1 is the difference between MS and NM. Based on the deviations of Y and Z , denoted here by dy and dz , the true position (TP) of the measured hole can be computed. The TP defines a circular tolerance area for the hole position and is defined by Eq. 2 .
For the example of Fig. 4 , the TP measure is within the predefined tolerances limits ( \(-T\) and \(+T\) ). The angular deviation ( DA ) complements the TP measure and provides information about what direction the hole has moved. The actual location of H1 related to this example is represented by a dotted circle in Fig. 5 .
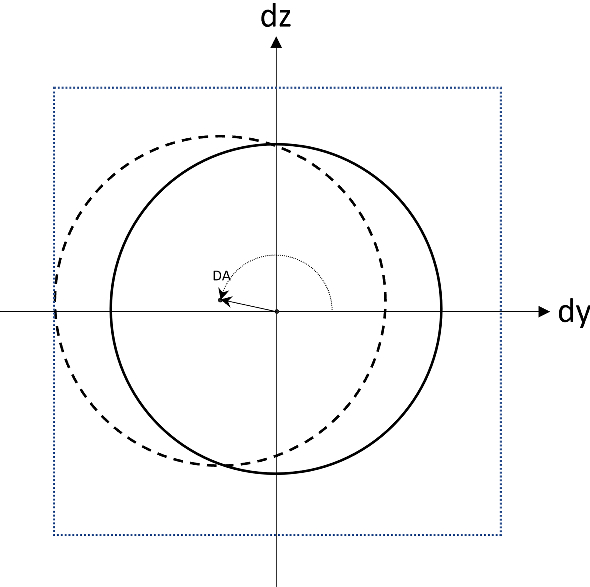
An illustration of the displacement of H1 of Fig. 4 . The related milled hole is represented by a dotted circle. The solid circle corresponds to the nominal position, and the dotted square represents the area where the hole meets the specifications. DA shows the angular deviation of the milled hole
The TP values of the other holes are calculated similarly using the deviations of the two coordinates locating a hole. The decision as to whether a hole location is within specification or not depends on the TP values, which must be within the lower and upper limits defined by the manufacturer.
5 Experiments and results
In this section, the data, implementation, and performance of each machine learning model are discussed.
5.1 Training, validation and test set
The dataset used for the quality control prediction consists of 1255 measurement reports, covering three years. Each report includes a timestamp of the measurement operation, the locations of the 20 milled holes, and the curvature of the beam, resulting in 144 different point measurements. It should be mentioned that the interval between two quality control measurements is not always two hours and can vary greatly depending on the production schedule, holidays, priorities, etc. As shown in Fig. 6 , which depicts the measurements of two hole-coordinate pairs using a time-stamped axis, the production for the bumper beam under study was partially interrupted during November and December 2019. This kind of interruption can be found several times (about 15 times) throughout the dataset, with most of them lasting for one or two weeks. Despite these interruptions, we assume that the dataset is continuous as the number of interruptions is small, and the machine learning models used in this study depend only on lag features for prediction.
For a given learning algorithm, the variable to be predicted (the output) is a single hole-coordinate pair, e.g., H1-Y, that is trained and tested separately. The prediction of measure t uses all points of the three lagged measurements, i.e., \(t-3\) , \(t-2\) , and \(t-1\) , as input, resulting in \(3\times 144\) independent variables for every variable to predict. Indeed, preliminary testing showed that the prediction mainly depends on the last observation \(t-1\) , and that it can be slightly improved by integrating three-lagged input. Furthermore, it should be stated that all measures are related to relative deviation. These values are available with the raw data used in this study.
The dataset is divided into two subsets. The first 70% of the dataset is used to train and validate the machine learning algorithms, and the remaining 30% is used to test the prediction performance of the models. Since the default hyperparameters of all models cannot guarantee optimal results for the prediction problem, we performed a hyperparameter tuning step that was done on the first subset of data. This subset was, in turn, divided into two other subsets, where the 50% of the dataset is used for training, and the next 20% is used for validation. The hyperparameters test was done on a randomly chosen hole, namely H5-X (the results of subsection 5.4 show that this hole has an average performance). The best parameters found were then used for the other holes. It should be noted that other holes were selected for the hyperparameter tests, and similar results were obtained.
5.2 Implementation
In addition to the random forest, two neural network models are considered for prediction purposes. The first model is based on a standard neural network, hereafter referred to simply as a ‘neural network’, and the second is a Long-Short Term Memory (LSTM). All machine learning models were implemented in Python 3.8.6 using the Scikit-Learn library (for the neural network and the random forest) and the Keras library (for the LSTM). The input data is processed using Pandas 1.1.3 and Numpy 1.19.2, and the visualization tools are based on Matplotlib 3.3.2. The working environment is Jupyter Notebook on a Windows machine with a Core i7 CPU and 32 GB of RAM.
The parameters of the neural network are one hidden layer of 100 neurons, and the LBFGS solver is used as the identity activation function. The hyperparameter function of the random forest is used to find the best parameters, with the best results obtained with the default settings. A greedy hyperparameter tuning was performed for LSTM, where the number of epochs ranged between 1 and 1000, the batch size was set to 1, 2, and 4, and the number of neurons was set to 1, 2, 4, and 10. The best parameters were found for 100 as the number of epochs, two as the number of batches, and one as the number of neurons.
Finally, it should be mentioned that the neural network and LSTM models are more sensitive to data scaling than the random forest. The input data is pre-processed by standardizing, and the same scaling is then applied to the input of the test set.
5.3 Data analysis
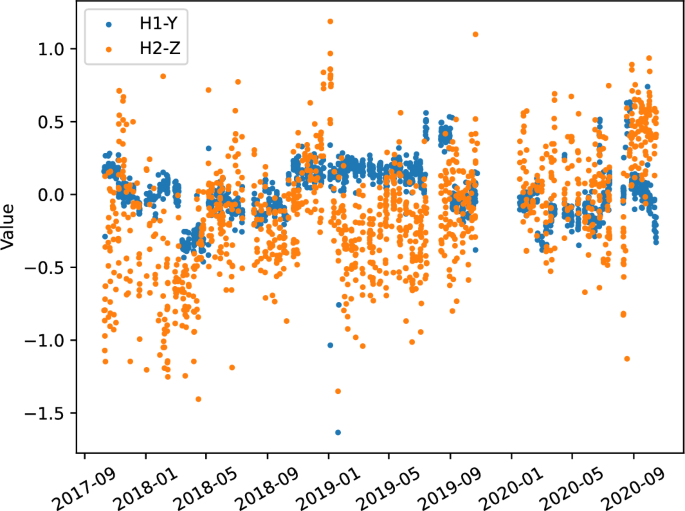
Scatter plot of the measurement values of H1-Y and H2-Z over the data collection period
The first step in analyzing the collected data is to understand the problem and verify its quality. This step involves visualizing and evaluating the relevance of the data, identifying outliers, and removing bad entries. Figure 6 presents the different measured values for the hole-coordinate pairs H1-Y and H2-Z over the data collection period. It can be observed that the H2-Z measurements are more spread out than H1-Y measurements. In contrast, H1-Y measurements fall within a narrow range that varies over time without a distinctive trend (e.g., degradation over time). These variations could potentially be explained by changes in the production process, but without additional data, it cannot be confirmed. Furthermore, the dispersion of the values in Fig. 6 (especially for H1-Y) supports the idea of using lagged measurements for prediction purposes.
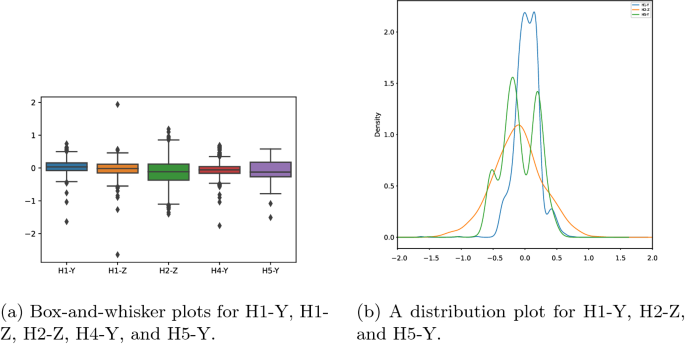
Analysis of measurement data for a subset of reference holes and coordinates
Figure 7 a illustrates the distribution of the different measurements of hole-coordinate pairs. Since showing all pairs in the same figure makes it difficult to read, we restrict the representation to only five variables that are selected randomly and have data properties compared to other variables. Figure 7 a indicates that all variables share similar data distribution properties. The median is almost equal to zero, and the interquartile ranges (IQRs) presented by boxes are very narrow, revealing that the measurements are concentrated within this area, especially for H1-Y, H1-Z, and H4-Y. In addition, Fig. 7 a shows for each pair the outliers, defined as the points outside of the whiskers, at 1.5 of IQR from the first and third quartiles. We can see that the majority of outliers are condensed at the head and tail of whiskers, and very few measurements are far from the rest of the data. Thus, the data available for this study is considered to be of good quality and does not require preprocessing.
Furthermore, Fig. 7 b illustrates the distribution plots of pairs of hole-coordinate. For the sake of readability, we select only three representative pairs as they have similar distributions to the other holes. The H2-Z plot shows a normal distribution that is symmetric and bell-shaped but slightly deviated from the zero mean. That is, this particular pair of hole-coordinate can be represented by a standard normal distribution. As for the H1-Y measurements, the distribution has a slight multimodal shape that can be smoothed to a normal distribution with high kurtosis, meaning that most observations have zero deviation. However, H5-Y has different peaks with different densities, which means that most measurements are slightly deviated from zero.
5.4 Performance of the models
In this subsection, the performance of the neural network, LSTM and random forest are assessed from both a quantitative and qualitative perspective. In addition, the models are compared to a standard autoregressive time series model.
5.4.1 A quantitative comparison
Three common performance metrics for regression problems are used to evaluate the predictive quality of models. The first is the Mean Absolute Error (MAE) which shows the magnitude of the overall error between the observed and predicted values. It neither eliminates the effect of positive and negative errors nor penalizes extreme forecast errors. The second is the Mean Squared Error (MSE), which penalizes extreme values. A high value of MSE shows a significant deviation between observed and predicted values, whereas a low value indicates that the predicted values are very close to the observations. Finally, the root mean square error (RMSE) is commonly used for regression problems and measures the square root of the second sample moment of residuals. RMSE is used to compare prediction errors of different models for the same data set and a particular variable, as it is scale-dependent. The definition of these three metrics are given in Eqs. ( 3 ), ( 4 ), and ( 5 ).
\(y_i\) is the observed target value,
\({\hat{y}}_i\) represents the predicted target value, and
n is the number of observations.
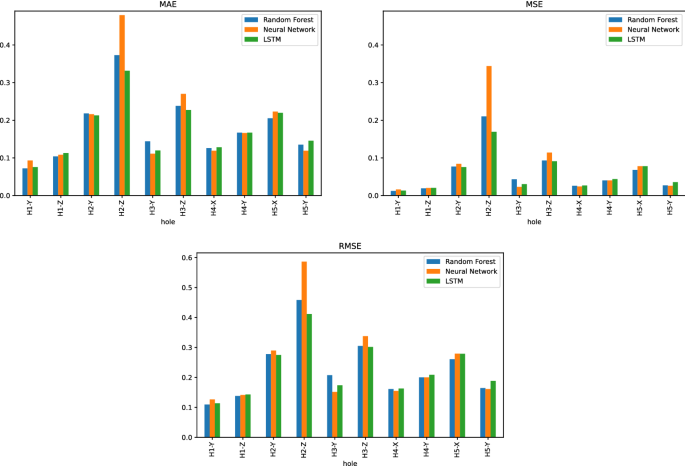
Comparison between the Random Forest, Neural Network and LSTM models using the MAE, MSE and RMSE metrics for the locations of all reference holes
Figure 8 shows the MAE, MSE, and RMSE metrics for the Random Forest and the two versions of Neural Network. Except for the reference hole H2, all models can provide reasonable predictions relative to the actual observations, i.e., the MAE metric ranges from 0.11 to 0.28 mm for the other holes. In particular, the predictions for hole H1 provide the best metrics, and H2 has the worst prediction metrics for the Y and Z coordinates. When learning models are considered, LSTM provides the best performance for H2-Z, where the metric MAE is improved by 31% over the neural network. For the remaining hole-coordinate pairs, the average MAE is the same for all models, i.e., 0.16. However, Random Forest and LSTM perform slightly better than Neural Network for MSE, i.e., 0.045 against 0.047.
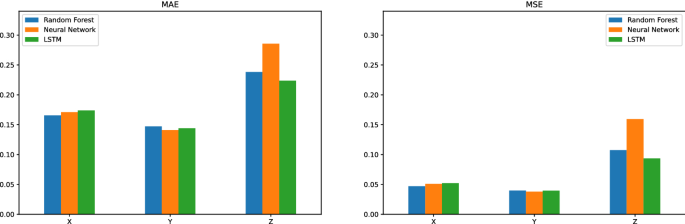
Comparison between the prediction models by grouping the holes located in the same coordinate (X, Y or Z). The metrics are MAE and MSE
Figure 9 groups the hole-coordinate pairs that are located in the same direction and provides the MAE and MSE metrics of each direction by learning model. Together with the results shown in Fig. 8 , we observe that for holes in the YZ coordinates (H1, H2, and H3), all prediction errors in the Z coordinate are higher than the corresponding Y coordinate. The same pattern appears for holes in the XY coordinates (H4 and H5) where the prediction errors in the X direction are slightly higher than in the Y direction. Since the comparison by coordinate is consistent for all learning models, it can be concluded that the models are better suited to one direction than another. Furthermore, when considering the locations of the holes in the beam, it can be observed that the holes on the left side of the beam, i.e., H1 and H4, are better predicted than the holes on the right side of the beam, i.e., H2, H3, and H5.
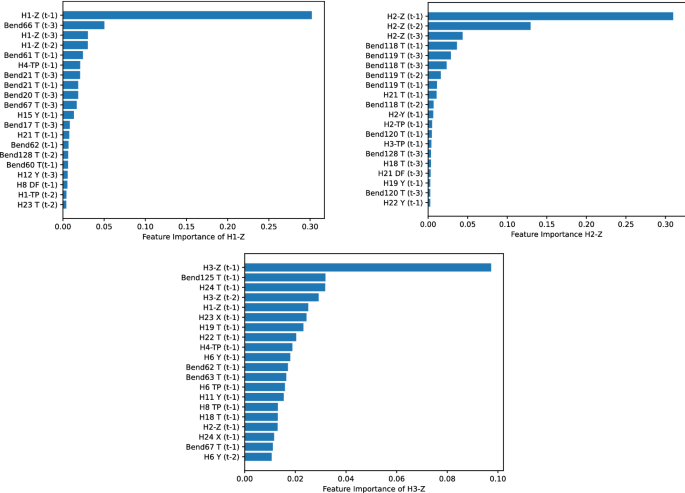
Top 20 most important features of the random forest model for the hole-coordinate pairs in the Y direction (H1-Z, H2-Z and H3-Z)
The previous analysis is further extended to include the feature importance of the predicted hole-coordinate pairs in the Y direction. The metrics of H2-Z and H3-Z show poor predictions compared to H1-Z, despite all of them being located in the same direction. Therefore, it is worth exploring which variables are the most significant and which factors have the greatest impact on the prediction. This can be done by analyzing the average feature importance of the decision trees in the random forest model. Figure 10 shows the top 20 most important features for H1-Z, H2-Z, and H3-Z. It can be observed that there is a variety of variables involved in the importance feature, including the previous measurements of the hole to be predicted, with the lagged time in parenthesis, as well as bend and other (non-reference) hole measurements. In the label in front of a variable name, the letter T refers to the twist tolerance for a bend measurement. The same letter is also used for small holes and indicates the distance to a reference hole (a specific metric set by the manufacturer is used). Lastly, the DF denotes the diameter of a milled hole.
Figure 10 shows that three factors affect the prediction quality. First, all predicted pairs depend strongly on their immediate previous measurement \(t-1\) and, to a lesser extent, on \(t-2\) and \(t-3\) . However, basing the predictions solely on previous measurements leads to poor prediction metrics, as can be seen in H2-Z. Second, a diverse range of information leads to better predictions. The feature importance of H1-Z shows that different sources of information are used, i.e., Bend 66 and Bend 67 are bend measurements near the location of H1, Bend 61 is in the middle of the beam, and Bend 20 and Bend 21 are on the other side of the beam. Third, having only low-importance values does not help to have good predictions (i.e., H3-Z). This indicates that the learning model cannot identify the relevant features for a good prediction of the target variable. Overall, this analysis confirms the importance of considering all available information and three-lagged measurements for prediction purposes.
5.4.2 A qualitative comparison
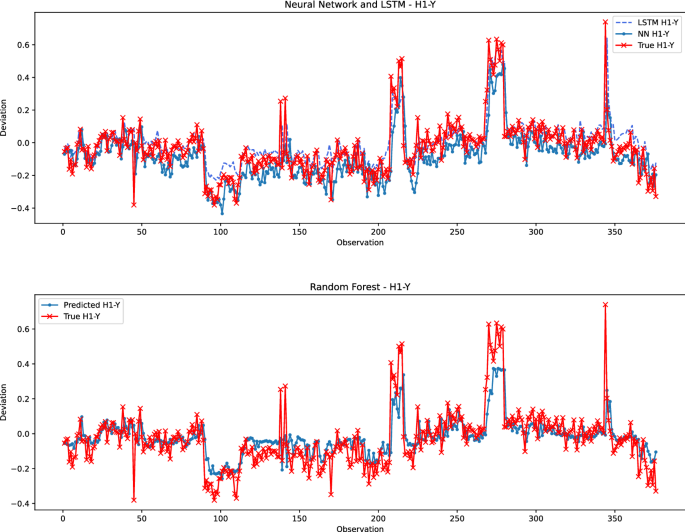
Prediction performance of the neural network (top) and random forest (bottom) models for the best-predicted coordinate – H1-Y
Figure 11 gives a qualitative comparison of the prediction models for the best-performing hole-coordinate pair, namely H1-Y. The bottom part of Fig. 11 plots the actual values and those predicted by the random forest, while the top part draws the predictions of Neural Network and LSTM (since they belong to the same family of learning models) together with the actual values. It can be observed that, in general, the random forest provides restricted and smooth predicted values. This observation is notable for the first and last segments of observations, where the values predicted by the random forest are always within the fluctuations of the actual measurements. However, LSTM and neural network models can track the spikes better to generate predictions as high as the actual values. Except for the measurements around Observation 100, LSTM performs marginally better than the neural network. Overall, all H1-Y predictions can be considered of high quality with good performance for both the random forest and LSTM.
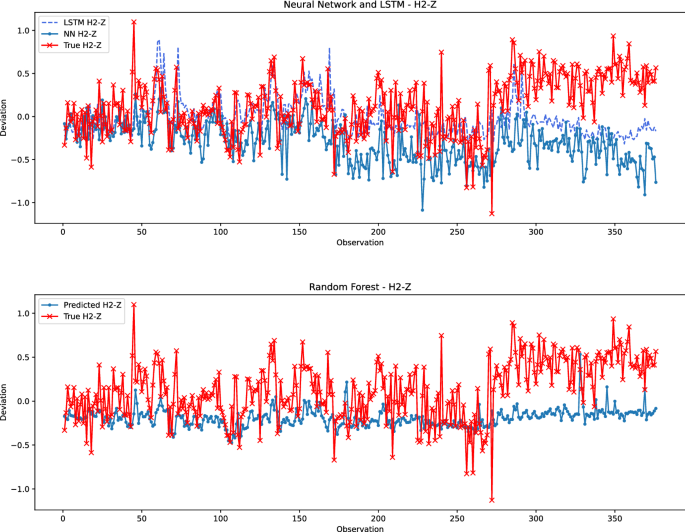
Prediction performance of the neural network (top) and random forest (bottom) models for the worst predicted coordinate – H2-Z
In the second qualitative comparison, Fig. 12 illustrates the performance of the prediction models for the worst-performing hole-coordinate pair, namely H2-Z. It can be seen that there is a significant gap between the actual observations and the predicted values for all models. Except for the first 40 observations, the random forest and neural network models generate poor predictions compared to the actual values. For the interval between observations 40 and 270, the neural network attempts to follow the trend of the actual values without providing good predictions, while the random forest predicts deviations close to zero. However, the LSTM shows much better performance as the actual values are better tracked. Indeed, when this segment of observations is considered, the MAE of LSTM is 0.24 against 0.36 and 0.30 for the neural network and random forest, respectively. This explains the better metrics obtained by LSTM for H2-Z. From about observation 270, the deviation of the predicted values from the actual observations becomes increasingly significant for all models. In particular, the random forest generates a prediction close to zero. We can conclude that this last segment is very peculiar; unknown changes have been made to the production process preventing the learning models from making good predictions. The limited performance is not due to limited learning capacity but rather to missing information not provided to the models. Indeed, as previously discussed and shown in Fig. 8 and Fig. 9 , the prediction is better in one direction than in another. Similarly, the prediction of the holes located on the left side of the beam is better than the holes located on the other side of the beam. This may be due to the clamping forces applied to the beam during the machining process, which is not available for this study. Another reason may be a variation in the upstream activity, for example, when the aluminum profiles are bent.
5.4.3 Comparison with an autoregressive time series model
The performance of the machine learning models is compared to an autoregressive integrated moving average (ARIMA) model. The model was fitted to the time series data to predict future points in the series. We recall that ARIMA is a univariate model, which means that previous data of a specific hole-coordinate pair is used to predict the next observations. The ‘statsmodels’ library was used to implement the ARIMA model, and the pre-built ‘auto_arima’ function was called to identify the most optimal values for trend elements ( p , d , q ), where p , d , and q are the trends in autoregression order, difference order, and moving average order, respectively. The best parameters differ from one hole to another, for example, the ARIMA (3,1,3) model is used for H1-Y, and the ARIMA (2,0,4) is used for H2-Z.
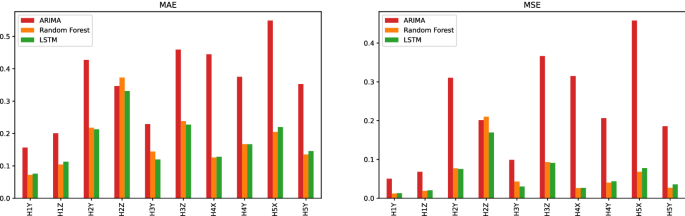
A comparison between ARIMA, random forest, and LSTM using MAE and MSE metrics
The metrics of the ARIMA models are shown in Fig. 13 and compared to the random forest and LSTM models. To compute the MAE and MSE, a static prediction for ARIMA for all pairs is used, meaning that the fitted models employ the actual value of the lagged dependent variable for prediction. It is clear that the learning models perform much better than the ARIMA models for all holes. This indicates that integrating other information such as bend and other holes’ measures help to achieve a better prediction. The exception is for H2-Z where ARIMA performs better than the random forest and is comparable to LSTM. This can be explained by the fact that ARIMA provides a very restrictive prediction that is always within the bounds of the actual values, which helps to reduce the error, especially for the last observation segment for H2-Z. However, as shown in Fig. 12 , the random forest and LSTM perform poorly for this last segment, starting at observation 270. Despite this poor performance, the learning models provide important information for this particular segment. They indicate that unknown changes have been made to the production process. This information cannot be derived from the ARIMA results.
5.5 Residual evaluation
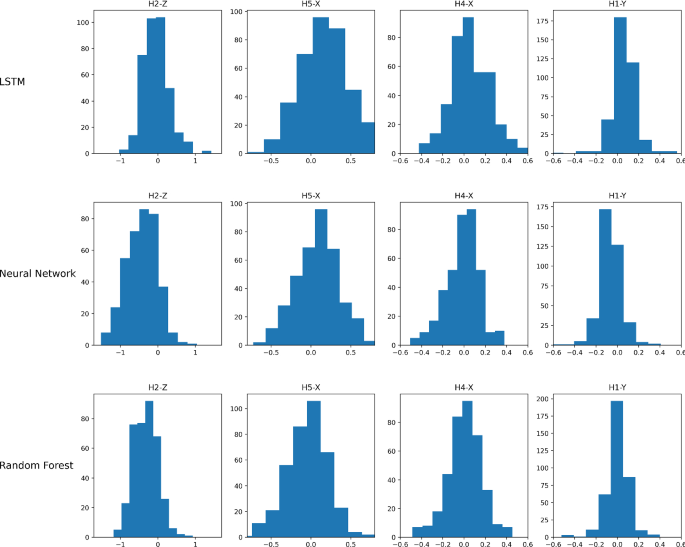
Residual normality test for the predictions of LSTM, Neural Network, and Random Forest for the worst and best MAE metric, H2-Z and H1-Y, respectively. The comparison also includes H5-X and H4-X ranked 7th and 3rd on the same MAE metric
The prediction quality of the proposed models can be further assessed by analyzing the residuals, which are the difference between the actual and predicted values. These residuals should be uncorrelated and normally distributed with zero mean (Kuhn and Johnson 2013 ). Figure 14 shows the histograms of residuals for the hole-coordinate pairs studied above, namely H2-Z and H1-Y, for all models. In addition to the worst- and best-predicted coordinates, the analysis includes H5-X and H4-X, which are ranked around 7th and 3rd positions in terms of MAE for all models considered.
The histograms in Fig. 14 provide insight into the distribution of residual errors and the prediction quality. In particular, the distribution of residuals for H2-Z (the worst-predicted coordinate) is non-Gaussian and positively skewed with high kurtosis (large tails) for the neural network and random forest. This confirms the poor prediction quality for these two prediction models. However, LSTM shows a better distribution of residuals for H2-Z, which confirms the better performance obtained for this coordinate compared to the other two prediction models. For the H1-Y coordinate, the distribution of residuals shows a mean close to zero and a low kurtosis. The histograms confirm the strong performance obtained with the H1-Y coordinate. As for H5-X and H4-X, the visualization of the residuals is close to the Gaussian distribution, especially for H5-X with the neural network. With the mean of the distribution for H5-X and H4-X being almost equal to zero for all models, it can be concluded that the predicted and actual values are not correlated. The prediction performance of the learning models is generally good for some hole-coordinate pairs.
5.6 Confidence bounds and TP-outliers detection
Figure 15 shows the predicted TP values for the hole H1 (discussed in Sect. 4 ), along with the corresponding \(3\sigma \) level (shown with orange dashed line) and the TP limits set by the manufacturer (between 0.0 and 1.0). The predicted TP value is calculated according to Eq. 2 and is based on the coordinates H1-Y and H1-Z predicted by the random forest model. This figure aims to assess whether the predicted TP values are within statistical control and whether they can be used for quality control of the bumper beam. The validation test is used for the \(3\sigma \) control. As can be seen, the TP limits are stricter than the \(3\sigma \) level. Only two outliers out of four are above the confidence bound. When the remaining holes are considered, a similar observation is obtained, i.e., the TP limits and \(3\sigma \) are almost the same. The only exception is for the hole H4, for which the \(3\sigma \) level is stricter than the TP limit; however, this can be considered acceptable as only one observation of H4 deviates from both TP and \(3\sigma \) limits. In conclusion, the process variation is under control and subject to random factors.
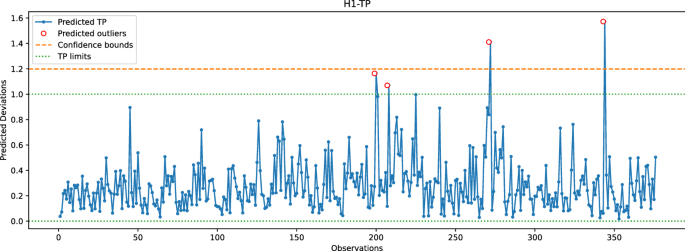
Predicted TP values for the hole H1 using the random forest model with a \(3\sigma \) level. The TP limits for H1 are between 0.0 and 1.0 as set by the manufacturer
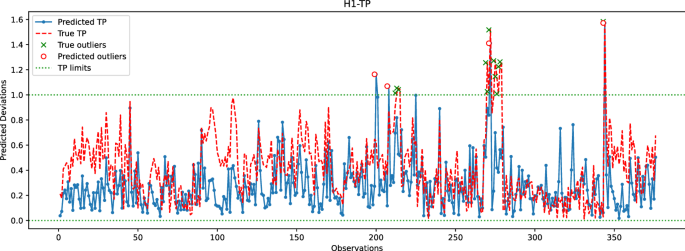
Comparison between the predicted and true TP values for the hole H1. The random forest model is used to predict the coordinates of H1
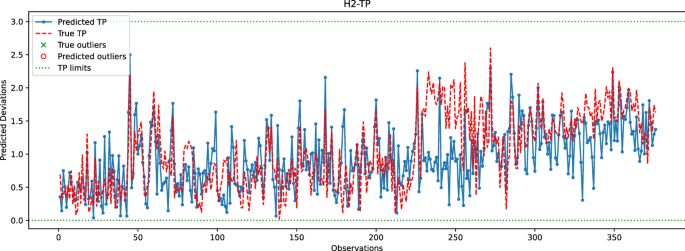
Comparison between the predicted and true TP values for the hole H2. The random forest model is used to predict the coordinates of H2
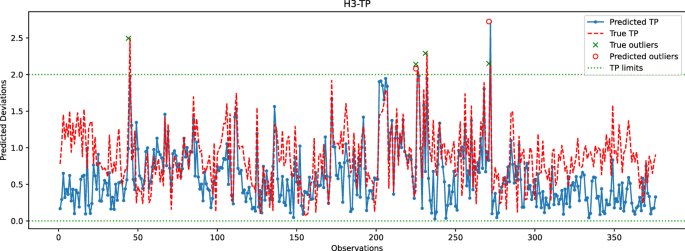
Comparison between the predicted and true TP values for the hole H3. The random forest model is used to predict the coordinates of H3
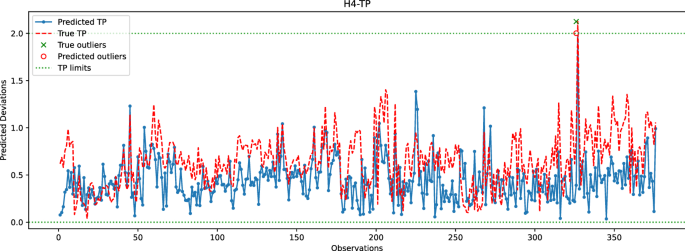
Comparison between the predicted and true TP values for the hole H4. The random forest model is used to predict the coordinates of H4
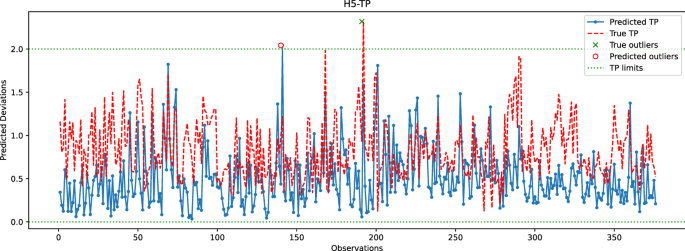
Comparison between the predicted and true TP values for the hole H5. The random forest model is used to predict the coordinates of H5
In the last experiment, Figs. 16 , 17 , 18 , 19 and 20 compare the real and predicted TP values for holes H1–H5. The TP limits of each hole, as set by the manufacturer, are highlighted with a dashed green line. These figures show a difference between the actual and predicted TP values. The results of the prediction model cannot follow the fluctuations of the actual TP values. However, when it comes to outliers, the model can give some insights into when the deviations might occur. For the hole with the best-predicted coordinates (H1, shown in Fig. 16 ), the predicted TP values are located in the same observation area where actual TP deviations are observed. The first area, located around observations 210-213, is detected in advance by the prediction model before observation 210. The second area records consecutive actual TP measures that exceed the upper limit. Although the prediction model cannot detect all of these outliers, it has a prediction located in this area. The last area includes only observation 344, which is perfectly detected by the prediction model with a similar actual TP value.
The same statement is also valid for H3, with the deviations of observations 226 and 272 being correctly reported by the model. Although the deviation of observation 45 is not detected, the predicted value of TP is very close to the upper limit, which may indicate that an early adjustment of the CNC machining settings should be made. As for H4, only one deviation is reported that is perfectly predicted by the model. While the results are satisfactory for the holes discussed above, the prediction of TP outliers for H5 is poor. The predictions of the X and Y coordinates of H5 are among the worst, which may explain the poor quality of the prediction of TP values for this hole. Finally, no conclusions can be drawn from the analysis of the H2 results because there are no outliers for this set of observations. However, it is unlikely that the prediction model would be able to identify outliers for H2, as the H2-Y and H2-Z coordinates are poorly predicted.
Furthermore, the accuracy of the learning model is evaluated using the True Positive Rate (TPR or Sensitivity) and the True Negative Rate (TNR or Specificity) measures. The accuracy is defined as the ability of the learning model to predict actual TP-outliers. In this case, measuring the specificity is not relevant as the number of actual TP values within limits is significantly higher than actual outliers. Therefore, the focus is on the ability of the model to correctly predict actual TP outliers. As the model can detect outliers in nearby areas, the definition of a True Positive is expanded to include predicted outliers that will actually occur within the next 24 h. A False Positive is defined as when no actual TP outlier occurs during the next 24 h, and a False Negative is defined when there is an actual TP-outlier that is not predicted within the previous 24 h. Equations ( 6 ), ( 7 ), and ( 8 ) define TPR, False Negative Rate (FNR), and Threat Score (TS), respectively.
Table 1 reports the number of outliers, TPR , FNR , and TS rates for each hole. The performance of holes H4 and H5, while noteworthy, is not significant since they are only related to a single outlier that the model either predicts well or badly. For hole H1, Table 1 indicates low TPR and TS rates, which is a result of some predictions and actual TP outliers being separated by more than 24 h. For instance, the predicted TP outlier at observation 200 is separated by more than 24 h from the actual TP outliers at observations 213-215, and similarly with the predicted TP outlier at observation 272 and the actual outliers at subsequent observations. In the case of hole H3, the predicted TP outlier at observation 226 is correctly reported by the learning model; however, the next actual outlier at observation 232 occurs after 24 h, explaining the relatively low TS score obtained for H3. Overall, Table 1 confirms that the learning model cannot accurately predict TP outliers. Nonetheless, the predicted information can still be used by the manufacturer to make early adjustments.
6 Conclusion
This paper deals with a prediction problem for quality control. The underlying problem is related to the automotive industry, and the product under study is the bumper beam, subject to stringent quality criteria. To support the quality control process of this product, we proposed machine learning models to predict the location of the reference holes of the next produced beam. The models are based on a time series that consisting of the historical data set of previous measurements that includes the beam characteristics. The learning models developed are a neural network, a long short-term memory network, and a random forest, and all are trained under similar conditions. The experimental study showed that the performance of all models is generally quite similar, with a slight dominance of the long short-term memory network and the random forest models. The results also indicate that the prediction can be good for some hole-coordinate pairs. However, there are considerable discrepancies for some other coordinates, and the predictions deviate significantly from the actual values. Since both models showed similar behavior, it can be concluded that the available information is not sufficient for prediction and that other resources should be included, such as process parameters or data from an upstream activity.
This work shows that applying machine learning models to real-life problems is not as easy as it sounds and is hampered by several factors. Not all data is captured or made available to be used for other purposes. For example, in the context of this work, information about changes in CNC settings is volatile and cannot be retrieved later, limiting its use for learning purposes. This example also shows that the transition to Industry 4.0 is not a straightforward process and could be challenging in several areas.
Agrawal A, Goel S, Rashid WB et al (2015) Prediction of surface roughness during hard turning of AISI 4340 steel (69 HRC). Appl Soft Comput 30:279–286
Article Google Scholar
Ayoobi N, Sharifrazi D, Alizadehsani R et al (2021) Time series forecasting of new cases and new deaths rate for covid-19 using deep learning methods. Results Phys 27(104):495
Google Scholar
Biau G, Scornet E (2016) A random forest guided tour. Test 25(2):197–227
Breiman L (2001) Random forests. Mach Learn 45(1):5–32
Brockwell PJ, Davis RA (2016) Introduction to time series and forecasting. Springer texts in statistics. Springer, Cham, pp 73–96
Book Google Scholar
Bustillo A, Pimenov DY, Matuszewski M et al (2018) Using artificial intelligence models for the prediction of surface wear based on surface isotropy levels. Robot Comput Integr Manufact 53:215–227
Bustillo A, Pimenov DY, Mia M et al (2021) Machine-learning for automatic prediction of flatness deviation considering the wear of the face mill teeth. J Intell Manufact 32(3):895–912
Dogan A, Birant D (2021) Machine learning and data mining in manufacturing. Expert Syst Appl 166(114):060
Freeman BS, Taylor G, Gharabaghi B et al (2018) Forecasting air quality time series using deep learning. J Air & Waste Manag Assoc 68(8):866–886
Genuer R, Poggi JM (2020) Random forests. Springer, London
Géron A (2019) Hands-on machine learning with scikit-learn, keras and tensorflow: concepts, tools, and techniques to build intelligent systems. O’Reilly Media
Gokalp MO, Kayabay K, Akyol MA, et al (2017) Big data for industry 4.0: a conceptual framework. In: proceedings - 2016 international conference on computational science and computational intelligence, CSCI 2016 pp 431–434
Groover MP (2019) Fundamentals of modern manufacturing: materials, processes, and systems, vol 7. Wiley
Ian G, Yoshua B, Aaron C (2016) Deep learning. The MIT Press, Adaptive Computation and Machine Learning
Ibarra D, Ganzarain J, Igartua JI (2018) Business model innovation through Industry 4.0: a review. Procedia Manufact 22:4–10
James G, Witten D, Hastie T et al (2013) An introduction to statistical learning, vol 112. Springer
Karayel D (2009) Prediction and control of surface roughness in CNC lathe using artificial neural network. J Mater Process Technol 209(7):3125–3137
Ketkar N, Moolayil J (2021) Feed-forward neural networks. Deep learning with python pp 93–131
Kim KH, Sohn MJ, Lee S et al (2022) Descriptive time series analysis for downtime prediction using the maintenance data of a medical linear accelerator. Appl Sci 12(11):5431
Kinyua P, Jouandeau N (2021) Sample-label view transfer active learning for time series classification. In: international conference on artificial neural networks, Springer, London pp 600–611
Kuhn M, Johnson K (2013) Applied predictive modeling, vol 26. Springer, New York
Lee I, Lee K (2015) The internet of things (IoT): applications, investments, and challenges for enterprises. Bus Horiz 58(4):431–440
Li Z, Zhang Z, Shi J et al (2019) Prediction of surface roughness in extrusion-based additive manufacturing with machine learning. Robot Comput Integr Manufact 57:488–495
Ma L, Wang M, Peng K (2022) A novel bidirectional gated recurrent unit-based soft sensor modeling framework for quality prediction in manufacturing processes. IEEE Sens J 22(19):18,610-18,619
Martin O, Lopez M, Martin F (2007) Artificial neural networks for quality control by ultrasonic testing in resistance spot welding. J Mater Process Technol 183(2–3):226–233
Meng Y, Xu M, Yoon S et al (2022) Flexible and high quality plant growth prediction with limited data. Front Plant Sci 13:304–989
Oakland RJ, Oakland JS (2018) Statistical process control. Routledge
Rauf HT, Lali M, Khan MA et al (2021) Time series forecasting of covid-19 transmission in asia pacific countries using deep neural networks. Pers Ubiquitous Comput 20:1–18
Rehmer A, Kroll A (2020) On the vanishing and exploding gradient problem in gated recurrent units. IFAC-PapersOnLine 53(2):1243–1248
Risan A, Msakni MK, Schütz P (2021) A Neural Network Model for Quality Prediction in the Automotive Industry. In: IFIP advances in information and communication technology. Springer: Berlin pp 567–575
Roblek V, Meško M, Krapež A (2016) A complex view of industry 4.0. SAGE Open 6(2):1–11
Santos C, Mehrsai A, Barros AC et al (2017) Towards industry 4.0: an overview of European strategic roadmaps. Procedia Manufact 13:972–979
Shohan S, Hasan M, Starly B et al (2022) Investigating autoregressive and machine learning-based time series modeling with dielectric spectroscopy for predicting quality of biofabricated constructs. Manufact Lett 33:902–908
Tao F, Cheng J, Qi Q et al (2017) (2017) Digital twin-driven product design, manufacturing and service with big data. Int J Adv Manufact Technol 94(9):3563–3576
Tao F, Qi Q, Liu A et al (2018) Data-driven smart manufacturing. J Manufact Syst 48(C):157–169
Tsai YH, Chen JC, Lou SJ (1999) An in-process surface recognition system based on neural networks in end milling cutting operations. Int J Mach Tools Manufact 39(4):583–605
Wang T, Chen Y, Qiao M et al (2018) A fast and robust convolutional neural network-based defect detection model in product quality control. Int J Adv Manufact Technol 94(9–12):3465–3471
Wang Y, Liu M, Zheng P et al (2020) A smart surface inspection system using faster R-CNN in cloud-edge computing environment. Adv Eng Informat 43(101):037
Wu D, Wei Y, Terpenny J, (2018) Surface roughness prediction in additive manufacturing using machine learning. ASME, (2018) 13th international manufacturing science and engineering conference. MSEC 2018:3
Wuest T, Weimer D, Irgens C et al (2016) Machine learning in manufacturing: advantages, challenges, and applications. Prod Manufact Res 4(1):23–45
Xu X (2012) From cloud computing to cloud manufacturing. Robot Comput Integr Manufact 28(1):75–86
Zhao D, Wang Y, Liang D et al (2020) Performances of regression model and artificial neural network in monitoring welding quality based on power signal. J Mater Res Technol 9(2):1231–1240
Download references
Open access funding provided by NTNU Norwegian University of Science and Technology (incl St. Olavs Hospital - Trondheim University Hospital). This work was supported by the Research Council of Norway as part of the LeanDigital research project, number 295145.
Author information
Anders Risan and Peter Schütz contributed equally to this work.
Authors and Affiliations
Department of Industrial Economics and Technology Management, Norwegian University of Science and Technology, Torgarden, 7491, Trondheim, Norway
Mohamed Kais Msakni, Anders Risan & Peter Schütz
You can also search for this author in PubMed Google Scholar
Corresponding author
Correspondence to Mohamed Kais Msakni .
Ethics declarations
Conflict of interest.
One of the co-authors of this manuscript is a member of the editorial board of Computational Management Science. The authors declare no-conflict of interest regarding the publication of this paper.
Additional information
Publisher's note.
Springer Nature remains neutral with regard to jurisdictional claims in published maps and institutional affiliations.
Rights and permissions
Open Access This article is licensed under a Creative Commons Attribution 4.0 International License, which permits use, sharing, adaptation, distribution and reproduction in any medium or format, as long as you give appropriate credit to the original author(s) and the source, provide a link to the Creative Commons licence, and indicate if changes were made. The images or other third party material in this article are included in the article's Creative Commons licence, unless indicated otherwise in a credit line to the material. If material is not included in the article's Creative Commons licence and your intended use is not permitted by statutory regulation or exceeds the permitted use, you will need to obtain permission directly from the copyright holder. To view a copy of this licence, visit http://creativecommons.org/licenses/by/4.0/ .
Reprints and permissions
About this article
Msakni, M.K., Risan, A. & Schütz, P. Using machine learning prediction models for quality control: a case study from the automotive industry. Comput Manag Sci 20 , 14 (2023). https://doi.org/10.1007/s10287-023-00448-0
Download citation
Received : 03 June 2022
Accepted : 01 March 2023
Published : 16 March 2023
DOI : https://doi.org/10.1007/s10287-023-00448-0
Share this article
Anyone you share the following link with will be able to read this content:
Sorry, a shareable link is not currently available for this article.
Provided by the Springer Nature SharedIt content-sharing initiative
- Neural network
- Random forest
- Quality control
- Manufacturing
Mathematics Subject Classification
Advertisement
- Find a journal
- Publish with us
- Track your research

- SUGGESTED TOPICS
- The Magazine
- Newsletters
- Managing Yourself
- Managing Teams
- Work-life Balance
- The Big Idea
- Data & Visuals
- Reading Lists
- Case Selections
- HBR Learning
- Topic Feeds
- Account Settings
- Email Preferences
Quality management
- Business management
- Process management
- Project management
Time-and-Motion Regained
- Paul S. Adler
- From the January–February 1993 Issue
Creating a Culture of Quality
- Ashwin Srinivasan
- Bryan Kurey
- From the April 2014 Issue

U.S. Health Care Reform Can't Wait for Quality Measures to Be Perfect
- Brian J Marcotte
- Annette Guarisco Fildes
- Michael Thompson
- Leah Binder
- October 04, 2017
Reign of Zero Tolerance (HBR Case Study)
- Janet Parker
- Eugene Volokh
- Jean Halloran
- Michael G. Cherkasky
- November 01, 2006
Framing the Big Picture
- Scott D. Anthony
- March 31, 2011

Teaching Smart People How to Learn
- Chris Argyris
- From the May–June 1991 Issue
Fixing Health Care from the Inside, Today
- Steven J. Spear
- September 01, 2005
Growth as a Process: The HBR Interview
- Jeffrey R. Immelt
- Thomas A. Stewart
- From the June 2006 Issue

Health Care Needs Real Competition
- Leemore S Dafny
- Thomas H. Lee M.D.
- From the December 2016 Issue
Why (and How) to Take a Plant Tour
- David M. Upton
- Stephen E. MacAdam
- From the May–June 1997 Issue

The CEO of Canada Goose on Creating a Homegrown Luxury Brand
- From the September–October 2019 Issue
Listening at Scale
- Charlene Li
- April 13, 2020
Beyond Toyota: How to Root Out Waste and Pursue Perfection
- James P. Womack
- Daniel T. Jones
- From the September–October 1996 Issue

The Case for Capitation
- Brent C. James
- Gregory P. Poulsen
- From the July–August 2016 Issue
The Contradictions That Drive Toyota's Success
- Hirotaka Takeuchi
- Norihiko Shimizu
- From the June 2008 Issue
Tailored, Not Benchmarked: A Fresh Look at Corporate Planning
- Andrew Campbell
- From the March–April 1999 Issue

A Better Way to Onboard AI
- Boris Babic
- Daniel L. Chen
- Theodoros Evgeniou
- Anne-Laure Fayard
- From the July–August 2020 Issue

Organizational Grit
- Thomas H. Lee
- Angela L. Duckworth
- From the September–October 2018 Issue
Reign of Zero Tolerance (HBR Case Study and Commentary)
- From the November 2006 Issue

The Employer-Led Health Care Revolution
- Patricia A. McDonald
- Robert S. Mecklenburg
- Lindsay A. Martin
- From the July–August 2015 Issue

Pete & Gerry's
- Jose B. Alvarez
- Natalie Kindred
- November 30, 2016
John Smithers
- Todd D. Jick
- December 17, 2001
Pleasant Bluffs: Launching a Home-Based Hospital Program
- Laura Erskine
- April 07, 2016
Danaher Corporation, 2007-2017
- John R. Wells
- Gabriel Ellsworth
- January 18, 2017
Continuous Software Development: Agile's Successor
- Jeffrey J. Bussgang
- Samuel Clemens
- Olivia Hull
- January 07, 2018
Apollo Hospitals: Differentiation through Hospitality
- Suhruta Kulkarni
- Kripa Makhija
- Unnikrishnan Dinesh Kumar
- June 01, 2013
ISSC Solution Center--Dallas: Transforming Software Development
- Sirkka Jarvenpaa
- September 08, 1995
Ritz-Carlton Hotel Co.
- Sandra J. Sucher
- Stacy McManus
- March 20, 2001
Note on Quality Function Deployment
- Jitendra R. Sharma
- November 18, 2009
A Maestro without Borders: How André Rieu Created the Classical Music Market for the Masses
- W. Chan Kim
- Renee Mauborgne
- August 28, 2017
Romeo Engine Plant
- Robert S. Kaplan
- Amy P. Hutton
- November 19, 1993
John Smithers at Sigtek
- October 05, 1990
Year Up: A Social Entrepreneur Builds High Performance
- Allen S. Grossman
- Naomi Greckol-Herlich
- June 11, 2008
Sterling Chemicals, Inc.: Quality and Productivity Improvement Program
- Karen H. Wruck
- A. Scott Keating
- October 27, 1992
Molino Cañuelas: Serving Customers from Seed Development to the Kitchen Table
- Mariana Cal
- Fernanda Miguel
- December 10, 2017
Freqon--Buyer-Supplier Evolution?
- Thomas E. Vollmann
- Carlos Cordon
- Chris Ellegaard
- January 01, 2001
Strategy Execution Module 12: Aligning Performance Goals and Incentives
- Robert Simons
- November 21, 2016
Happy Family: Investing for the Future
- Fanyin Zheng
- April 06, 2020
Cristo Rey St. Martin College Preparatory School: Promoting a Culture of Continuous Improvement, Epilogue
- Gail Berger Darlow
- Liz Livingston Howard
- March 08, 2019
Institute for Healthcare Improvement: The 5 Million Lives Campaign
- Hayagreeva Rao
- David W. Hoyt
- March 05, 2010

On Weldon's Watch: Recalls at Johnson & Johnson from 2009 to 2010 Teaching Note
- Clayton Rose
- November 09, 2012
Pleasant Bluffs: Launching a Home-Based Hospital Program, Teaching Note
Popular topics, partner center.
Academia.edu no longer supports Internet Explorer.
To browse Academia.edu and the wider internet faster and more securely, please take a few seconds to upgrade your browser .
Enter the email address you signed up with and we'll email you a reset link.
- We're Hiring!
- Help Center
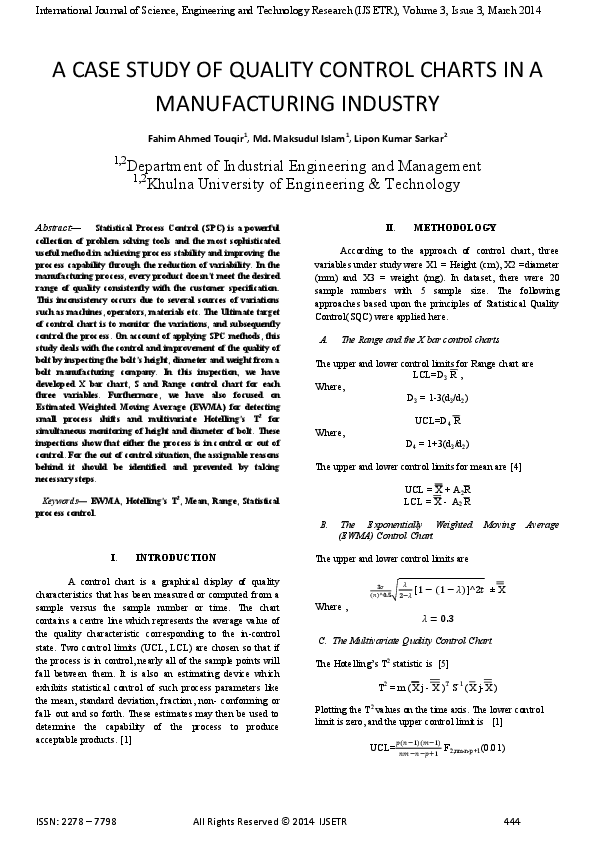
A CASE STUDY OF QUALITY CONTROL CHARTS IN A MANUFACTURING INDUSTRY

Related Papers
Eng Mohamed Hamdy
– Most of the modern industrial processes are naturally multivariate. Multivariate control charts are supplanted univariate control charts, as it takes into account the relationship between variables and identifies the real process changes, which are undetectable by univariate control charts. In practice, the basic assumption that the measurements are independently and identically distributed about a target value is not always valid. Violation of this assumption increases the False Alarm Rate (FAR) and deteriorates the separation of assignable causes from common causes. This paper presents the application of Multivariate Statistical Process Control (MSPC) charts (e. g., Hotelling , MEWMA) to monitor the flare making process in a straight fluorescent light bulb industry. Furthermore, it develops the appropriate procedure for monitoring a multivariate autocorrelated data variable (i. e., dynamic behavior) by using Autoregressive Integrated Moving Average (ARIMA) models. Univariate SPC charts and decomposition approach are used to identify the out-of-control signals that are generated from multivariate SPC charts. Software packages such as Minitab 17 and Statgraphics Centurion XVI are used to construct the control charts.
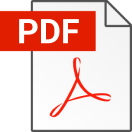
Jonathan Quiroz
cristian palacio
control estadistico de la calidad
Abidemi Adeniyi
This project is aimed at providing a stock price prediction system which can be used to forecast the future stock price of the Nigerian Stock Exchange using the artificial neural network. This study will attempt to reduce the stress people have in analyzing large amount of data in order to predict stock. This study will have to look into the problem areas of the stock market prediction and devise a method of proffering solutions to all these problems.
Seyed Taghi Akhavan Niaki
In this paper, two control charts based on the generalized linear test (GLT) and contingency table are proposed for Phase-II monitoring of multivariate categorical processes. The performances of the proposed methods are compared with the exponentially weighted moving average-generalized likelihood ratio test (EWMA-GLRT) control chart proposed in the literature. The results show the better performance of the proposed control charts under moderate and large shifts. Moreover, a new scheme is proposed to identify the parameter responsible for an out-of-control signal. The performance of the proposed diagnosing procedure is evaluated through some simulation experiments.
OLUMA URGESSA
Statistical Quality Control, 6th Edition
Regiz Faria
Anwar Shaker
sanika kamtekar
RELATED PAPERS
shayne cabrera
Journal of Materials in Civil Engineering
Barzin Mobasher
Shawn Dmello
Everardo Emmanuel Tovar
Jayesh Hirani
Russian Geology and Geophysics
Alexey Ariskin , Georgy Nikolaev
Loévanofski Hiribarnovitch
Alexey Ariskin
The Hymenoptera of Costa Rica
James M Carpenter
transstellar
TJPRC Publication
Mohammed Alami
Eli Bressert
Nature chemistry
Frank Neese
Ulrich Wienand , Michele Scagliarini , Napoli Nicola
Communications in Statistics - Theory and Methods
Nasir A R Syed , Raja Fawad Zafar
Ridwan Sanusi
Astronomy and Astrophysics
Quentin A Parker
Earth and Planetary Science Letters
Roberta Rudnick
Noorlisa Harun
Science Park Research Organization & Counselling
Amirhossein Amiri
Astronomical Journal
Ricardo Covarrubias
E. Nikogossian
Communications in Statistics - Simulation and Computation
Subha Chakraborti
T. Movsessian
Ridwan Sanusi , Nurudeen Adegoke
Environmental Science and Pollution Research
Pranvera Lazo
Economic Quality Control
Saddam Akber Abbasi
Dhaka University Institutional Repository
Md. Anwar Hossain
Arnoldo Sandoval
Hungarian Journal Of Industry And Chemistry Veszprém, Vol. 41(1) pp. 77-82 (2013)
Janos Abonyi
Héctor O Portillo Reyes, Fausto Elvir y Marcio Martínez
Héctor O R L A N D O Portillo Reyes
Michelle Cluver
Journal of Cellular Plastics
Nelson Oliveira
Jeh-Nan Pan
RELATED TOPICS
- We're Hiring!
- Help Center
- Find new research papers in:
- Health Sciences
- Earth Sciences
- Cognitive Science
- Mathematics
- Computer Science
- Academia ©2024

Case Study: Connecting Cloud-based Quality Control to the Edge
- By Josh Eastburn
- May 06, 2022
Intrinsics Imaging’s AI-powered vision system uses edge I/O to integrate cloud analytics into process controls. This case study originally appeared in Automation 2022: Cybersecurity & Connectivity Volume 2.

Machine vision systems can recognize superficial aberrations in manufactured goods—like lines, spots, holes, color discrepancies and more—that would typically require time-consuming manual inspection. However, these systems require specialized programming and maintenance, which can make them difficult to implement. California-based Intrinsics Imaging solves this problem with its Heijunka Vision analytics-as-a-service software. Instead of programming specialized cameras, Heijunka provides a library of image processing and machine learning algorithms running in the cloud that allow any IP camera to perform intelligent defect detection. Intrinsics works with customers to tailor analytics to their specific objectives, then provides a concierge level of service so that customers no longer need to train, adapt, or maintain their vision system. Typically, Heijunka integrates with SCADA systems to create analytics dashboards, alarms, and quality control actions. But when Intrinsics was approached by a customer hoping to integrate Heijunka directly into process controls, it looked to Opto 22’s groov RIO edge I/O for a way to connect the cloud to the edge.
Securing a path to the edge
For applications like manufacturing asphalt roofing and other kinds of building products, Heijunka not only finds defects in coatings, but also detects problems in fiberglass underlayment, color consistency, product wrapping, and even pallet counts. It can also continuously inspect in-process materials to ensure that specifications, such as dimensions, smoothness, straightness, and color, are met. For this application, Heijunka would be looking at two production lines moving discrete boards at high speed.
- The primary line cuts large sheets of raw material to size. Cut sheets would need to be inspected for excess moisture as well as dents, debris, and scratches as small as a grain of rice. The customer runs hundreds of different product types through this conveyor, each being cut to a different size and configuration.
- The second line would be responsible for monitoring the quality of the milling process, specifically looking for chipping along the edges.
Machine vision analytics where they need to be
Intrinsic Imaging’s Heijunka Vision analytics-as-a-service software continuously inspects in-process materials to ensure that specifications such as dimensions, smoothness, straightness, and color are met. A manufacturer wanted to integrate this cloud-based machine vision solution directly into its process control system, rather than hook it to a SCADA system for quality control. Intrinsic engineers found Opto 22’s groov RIO edge I/O, which let the Heijunka software be hosted on AWS and publish MQTT messages to a hosted broker that were then bridged to an on-premises broker. The groov RIO edge I/O solution let Intrinsic support more of the last mile between cloud software and physical action, while letting the manufacturer speak the language they were most comfortable with. However, unlike most Heijunka applications, the customer also wanted a PASS/FAIL I/O signal that it could integrate directly into the PLCs that would handle material rejection. By bypassing the SCADA and providing a direct path to PLC action, the customer hoped to simplify integration and reduce latency. However, Intrinsics hadn’t integrated its cloud-based software directly with a hardware system before. “This was surprisingly difficult to figure out,” said Eric Cheng, CTO at Intrinsics. “I wasn’t sure what kind of device could provide a physical interface to our cloud-based system and also satisfy our technical and operating requirements.” Besides needing a device that could tolerate an industrial environment and integrate with Heijunka’s existing software stack, Eric needed something that would operate with minimal latency. From the time a given video capture was sent to Heijunka, the customer would have a roughly five-second window in which to detect and reject a problematic part. Heijunka would need to return a pass or fail indication that consistently fell within that window of opportunity.
Finding the missing piece
According to Eric, Heijunka’s communication infrastructure is usually built on MQTT. “Generally, we set up an MQTT broker and publish to an agreed topic,” Eric explained. “It’s usually new for customers, but it’s typically the best way to meet their performance and security requirements. [MQTT is] lightweight and low-latency, there’s plenty of open-source support for it, and it doesn’t require us to reach into their network from the outside. Amazon Web Services (AWS) has MQTT managed services to host a broker, and in cases where we are hosting the system ourselves, it’s all [deployable] out of the box and in the cloud.” In this case, Heijunka would be hosted on AWS and publish MQTT messages to a hosted broker, which would be bridged to an on-premises broker in the customer’s facility. (Bridging allows brokers to exchange data behind the scenes so that it’s available to clients on both systems.) This architecture proved to be the key factor in choosing groov RIO for the final piece of Intrinsics’ solution. “The customer found [a similar edge I/O device, and it] made me realize that an MQTT device could work for what we were doing,” said Eric. “I started searching around . . . Groov RIO was exactly what we needed, and it was eye-opening to see everything we could do with it.” groov RIO had the industrial build Eric needed, including a wide operating temperature range and UL Hazardous Locations and ATEX approvals. It was also compatible with his software stack. He said, “Groov RIO was on the same wavelength as us: built-in MQTT, Linux-based, web interface, and it just seemed more modern than some [other devices] that still require Windows 7 executables for configuration. I didn’t want to have those kinds of dependencies." Given the nature of the request, Eric’s customer was also interested in the cybersecurity of the proposed architecture and appreciated that the groov RIO modules included a network firewall.
Putting the cloud in control

Fast, automated quality control

About The Author
Josh Eastburn is director of technical marketing for Opto 22. After 12 years as an automation engineer working in the semiconductor, petrochemical, food and beverage, and life sciences industries, Eastburn works with the engineers at Opto 22 to understand the needs of tomorrow’s customers. He is a contributing writer here.
Did you enjoy this great article?
Check out our free e-newsletters to read more great articles..
What are you looking for?
Suggestions.
- Journalists
Pharma Quality Control Case Studies
BIOCAD’s Quest for a Reliable Microbiological Quantitative Reference Material
®</sup> 3D", "link" : "/content/biomerieux/corp/en/resource-hub/knowledge/case-studies/pharmaceutical-qc-case-studies/how-a-top-5-pharma-company-protects-production-an-increases-productivity-using-bact-alert-3d-media-statement.html", "type" : "Link"}}' href="/corp/en/resource-hub/knowledge/case-studies/pharmaceutical-qc-case-studies/how-a-top-5-pharma-company-protects-production-an-increases-productivity-using-bact-alert-3d-media-statement.html"> How a Top 5 Pharma Company Protects Production and Increases Productivity Using BACT/ALERT ® 3D
®</sup>", "link" : "/content/biomerieux/corp/en/resource-hub/knowledge/case-studies/pharmaceutical-qc-case-studies/how-thalgo-increased-productivity-with-chemunex-media-statement.html", "type" : "Link"}}' href="/corp/en/resource-hub/knowledge/case-studies/pharmaceutical-qc-case-studies/how-thalgo-increased-productivity-with-chemunex-media-statement.html"> How Thalgo Increased Productivity With CHEMUNEX ®
®</sup>", "link" : "/content/biomerieux/corp/en/resource-hub/knowledge/case-studies/pharmaceutical-qc-case-studies/how-shiseido-increased-the-efficiency-of-microbiological-controls-with-chemunex-case-study.html", "type" : "Link"}}' href="/corp/en/resource-hub/knowledge/case-studies/pharmaceutical-qc-case-studies/how-shiseido-increased-the-efficiency-of-microbiological-controls-with-chemunex-case-study.html"> How Shiseido Increased the Efficiency of Microbiological Controls With CHEMUNEX ®
®</sup> System?", "link" : "/content/biomerieux/corp/en/resource-hub/knowledge/case-studies/pharmaceutical-qc-case-studies/how-did-loreal-optimize-microbial-testing-with-the-chemunex-system-case-study.html", "type" : "Link"}}' href="/corp/en/resource-hub/knowledge/case-studies/pharmaceutical-qc-case-studies/how-did-loreal-optimize-microbial-testing-with-the-chemunex-system-case-study.html"> How did L’Oréal Optimize Microbial Testing With the CHEMUNEX ® System?
®</sup>?", "link" : "/content/biomerieux/corp/en/resource-hub/knowledge/case-studies/pharmaceutical-qc-case-studies/how-did-cosmebac-improve-microbiological-testing-process-with-chemunex-case-study.html", "type" : "Link"}}' href="/corp/en/resource-hub/knowledge/case-studies/pharmaceutical-qc-case-studies/how-did-cosmebac-improve-microbiological-testing-process-with-chemunex-case-study.html"> How did Cosmebac Improve Microbiological Testing Process With CHEMUNEX ® ?

An official website of the United States government
Here’s how you know
Official websites use .gov A .gov website belongs to an official government organization in the United States.
Secure .gov websites use HTTPS A lock ( A locked padlock ) or https:// means you’ve safely connected to the .gov website. Share sensitive information only on official, secure websites.
- Heart-Healthy Living
- High Blood Pressure
- Sickle Cell Disease
- Sleep Apnea
- Information & Resources on COVID-19
- The Heart Truth®
- Learn More Breathe Better®
- Blood Diseases and Disorders Education Program
- Publications and Resources
- Blood Disorders and Blood Safety
- Sleep Science and Sleep Disorders
- Lung Diseases
- Health Disparities and Inequities
- Heart and Vascular Diseases
- Precision Medicine Activities
- Obesity, Nutrition, and Physical Activity
- Population and Epidemiology Studies
- Women’s Health
- Research Topics
- Clinical Trials
- All Science A-Z
- Grants and Training Home
- Policies and Guidelines
- Funding Opportunities and Contacts
- Training and Career Development
- Email Alerts
- NHLBI in the Press
- Research Features
- Past Events
- Upcoming Events
- Mission and Strategic Vision
- Divisions, Offices and Centers
- Advisory Committees
- Budget and Legislative Information
- Jobs and Working at the NHLBI
- Contact and FAQs
- NIH Sleep Research Plan
- < Back To Health Topics
Study Quality Assessment Tools
In 2013, NHLBI developed a set of tailored quality assessment tools to assist reviewers in focusing on concepts that are key to a study’s internal validity. The tools were specific to certain study designs and tested for potential flaws in study methods or implementation. Experts used the tools during the systematic evidence review process to update existing clinical guidelines, such as those on cholesterol, blood pressure, and obesity. Their findings are outlined in the following reports:
- Assessing Cardiovascular Risk: Systematic Evidence Review from the Risk Assessment Work Group
- Management of Blood Cholesterol in Adults: Systematic Evidence Review from the Cholesterol Expert Panel
- Management of Blood Pressure in Adults: Systematic Evidence Review from the Blood Pressure Expert Panel
- Managing Overweight and Obesity in Adults: Systematic Evidence Review from the Obesity Expert Panel
While these tools have not been independently published and would not be considered standardized, they may be useful to the research community. These reports describe how experts used the tools for the project. Researchers may want to use the tools for their own projects; however, they would need to determine their own parameters for making judgements. Details about the design and application of the tools are included in Appendix A of the reports.
Quality Assessment of Controlled Intervention Studies - Study Quality Assessment Tools
*CD, cannot determine; NA, not applicable; NR, not reported
Guidance for Assessing the Quality of Controlled Intervention Studies
The guidance document below is organized by question number from the tool for quality assessment of controlled intervention studies.
Question 1. Described as randomized
Was the study described as randomized? A study does not satisfy quality criteria as randomized simply because the authors call it randomized; however, it is a first step in determining if a study is randomized
Questions 2 and 3. Treatment allocation–two interrelated pieces
Adequate randomization: Randomization is adequate if it occurred according to the play of chance (e.g., computer generated sequence in more recent studies, or random number table in older studies). Inadequate randomization: Randomization is inadequate if there is a preset plan (e.g., alternation where every other subject is assigned to treatment arm or another method of allocation is used, such as time or day of hospital admission or clinic visit, ZIP Code, phone number, etc.). In fact, this is not randomization at all–it is another method of assignment to groups. If assignment is not by the play of chance, then the answer to this question is no. There may be some tricky scenarios that will need to be read carefully and considered for the role of chance in assignment. For example, randomization may occur at the site level, where all individuals at a particular site are assigned to receive treatment or no treatment. This scenario is used for group-randomized trials, which can be truly randomized, but often are "quasi-experimental" studies with comparison groups rather than true control groups. (Few, if any, group-randomized trials are anticipated for this evidence review.)
Allocation concealment: This means that one does not know in advance, or cannot guess accurately, to what group the next person eligible for randomization will be assigned. Methods include sequentially numbered opaque sealed envelopes, numbered or coded containers, central randomization by a coordinating center, computer-generated randomization that is not revealed ahead of time, etc. Questions 4 and 5. Blinding
Blinding means that one does not know to which group–intervention or control–the participant is assigned. It is also sometimes called "masking." The reviewer assessed whether each of the following was blinded to knowledge of treatment assignment: (1) the person assessing the primary outcome(s) for the study (e.g., taking the measurements such as blood pressure, examining health records for events such as myocardial infarction, reviewing and interpreting test results such as x ray or cardiac catheterization findings); (2) the person receiving the intervention (e.g., the patient or other study participant); and (3) the person providing the intervention (e.g., the physician, nurse, pharmacist, dietitian, or behavioral interventionist).
Generally placebo-controlled medication studies are blinded to patient, provider, and outcome assessors; behavioral, lifestyle, and surgical studies are examples of studies that are frequently blinded only to the outcome assessors because blinding of the persons providing and receiving the interventions is difficult in these situations. Sometimes the individual providing the intervention is the same person performing the outcome assessment. This was noted when it occurred.
Question 6. Similarity of groups at baseline
This question relates to whether the intervention and control groups have similar baseline characteristics on average especially those characteristics that may affect the intervention or outcomes. The point of randomized trials is to create groups that are as similar as possible except for the intervention(s) being studied in order to compare the effects of the interventions between groups. When reviewers abstracted baseline characteristics, they noted when there was a significant difference between groups. Baseline characteristics for intervention groups are usually presented in a table in the article (often Table 1).
Groups can differ at baseline without raising red flags if: (1) the differences would not be expected to have any bearing on the interventions and outcomes; or (2) the differences are not statistically significant. When concerned about baseline difference in groups, reviewers recorded them in the comments section and considered them in their overall determination of the study quality.
Questions 7 and 8. Dropout
"Dropouts" in a clinical trial are individuals for whom there are no end point measurements, often because they dropped out of the study and were lost to followup.
Generally, an acceptable overall dropout rate is considered 20 percent or less of participants who were randomized or allocated into each group. An acceptable differential dropout rate is an absolute difference between groups of 15 percentage points at most (calculated by subtracting the dropout rate of one group minus the dropout rate of the other group). However, these are general rates. Lower overall dropout rates are expected in shorter studies, whereas higher overall dropout rates may be acceptable for studies of longer duration. For example, a 6-month study of weight loss interventions should be expected to have nearly 100 percent followup (almost no dropouts–nearly everybody gets their weight measured regardless of whether or not they actually received the intervention), whereas a 10-year study testing the effects of intensive blood pressure lowering on heart attacks may be acceptable if there is a 20-25 percent dropout rate, especially if the dropout rate between groups was similar. The panels for the NHLBI systematic reviews may set different levels of dropout caps.
Conversely, differential dropout rates are not flexible; there should be a 15 percent cap. If there is a differential dropout rate of 15 percent or higher between arms, then there is a serious potential for bias. This constitutes a fatal flaw, resulting in a poor quality rating for the study.
Question 9. Adherence
Did participants in each treatment group adhere to the protocols for assigned interventions? For example, if Group 1 was assigned to 10 mg/day of Drug A, did most of them take 10 mg/day of Drug A? Another example is a study evaluating the difference between a 30-pound weight loss and a 10-pound weight loss on specific clinical outcomes (e.g., heart attacks), but the 30-pound weight loss group did not achieve its intended weight loss target (e.g., the group only lost 14 pounds on average). A third example is whether a large percentage of participants assigned to one group "crossed over" and got the intervention provided to the other group. A final example is when one group that was assigned to receive a particular drug at a particular dose had a large percentage of participants who did not end up taking the drug or the dose as designed in the protocol.
Question 10. Avoid other interventions
Changes that occur in the study outcomes being assessed should be attributable to the interventions being compared in the study. If study participants receive interventions that are not part of the study protocol and could affect the outcomes being assessed, and they receive these interventions differentially, then there is cause for concern because these interventions could bias results. The following scenario is another example of how bias can occur. In a study comparing two different dietary interventions on serum cholesterol, one group had a significantly higher percentage of participants taking statin drugs than the other group. In this situation, it would be impossible to know if a difference in outcome was due to the dietary intervention or the drugs.
Question 11. Outcome measures assessment
What tools or methods were used to measure the outcomes in the study? Were the tools and methods accurate and reliable–for example, have they been validated, or are they objective? This is important as it indicates the confidence you can have in the reported outcomes. Perhaps even more important is ascertaining that outcomes were assessed in the same manner within and between groups. One example of differing methods is self-report of dietary salt intake versus urine testing for sodium content (a more reliable and valid assessment method). Another example is using BP measurements taken by practitioners who use their usual methods versus using BP measurements done by individuals trained in a standard approach. Such an approach may include using the same instrument each time and taking an individual's BP multiple times. In each of these cases, the answer to this assessment question would be "no" for the former scenario and "yes" for the latter. In addition, a study in which an intervention group was seen more frequently than the control group, enabling more opportunities to report clinical events, would not be considered reliable and valid.
Question 12. Power calculation
Generally, a study's methods section will address the sample size needed to detect differences in primary outcomes. The current standard is at least 80 percent power to detect a clinically relevant difference in an outcome using a two-sided alpha of 0.05. Often, however, older studies will not report on power.
Question 13. Prespecified outcomes
Investigators should prespecify outcomes reported in a study for hypothesis testing–which is the reason for conducting an RCT. Without prespecified outcomes, the study may be reporting ad hoc analyses, simply looking for differences supporting desired findings. Investigators also should prespecify subgroups being examined. Most RCTs conduct numerous post hoc analyses as a way of exploring findings and generating additional hypotheses. The intent of this question is to give more weight to reports that are not simply exploratory in nature.
Question 14. Intention-to-treat analysis
Intention-to-treat (ITT) means everybody who was randomized is analyzed according to the original group to which they are assigned. This is an extremely important concept because conducting an ITT analysis preserves the whole reason for doing a randomized trial; that is, to compare groups that differ only in the intervention being tested. When the ITT philosophy is not followed, groups being compared may no longer be the same. In this situation, the study would likely be rated poor. However, if an investigator used another type of analysis that could be viewed as valid, this would be explained in the "other" box on the quality assessment form. Some researchers use a completers analysis (an analysis of only the participants who completed the intervention and the study), which introduces significant potential for bias. Characteristics of participants who do not complete the study are unlikely to be the same as those who do. The likely impact of participants withdrawing from a study treatment must be considered carefully. ITT analysis provides a more conservative (potentially less biased) estimate of effectiveness.
General Guidance for Determining the Overall Quality Rating of Controlled Intervention Studies
The questions on the assessment tool were designed to help reviewers focus on the key concepts for evaluating a study's internal validity. They are not intended to create a list that is simply tallied up to arrive at a summary judgment of quality.
Internal validity is the extent to which the results (effects) reported in a study can truly be attributed to the intervention being evaluated and not to flaws in the design or conduct of the study–in other words, the ability for the study to make causal conclusions about the effects of the intervention being tested. Such flaws can increase the risk of bias. Critical appraisal involves considering the risk of potential for allocation bias, measurement bias, or confounding (the mixture of exposures that one cannot tease out from each other). Examples of confounding include co-interventions, differences at baseline in patient characteristics, and other issues addressed in the questions above. High risk of bias translates to a rating of poor quality. Low risk of bias translates to a rating of good quality.
Fatal flaws: If a study has a "fatal flaw," then risk of bias is significant, and the study is of poor quality. Examples of fatal flaws in RCTs include high dropout rates, high differential dropout rates, no ITT analysis or other unsuitable statistical analysis (e.g., completers-only analysis).
Generally, when evaluating a study, one will not see a "fatal flaw;" however, one will find some risk of bias. During training, reviewers were instructed to look for the potential for bias in studies by focusing on the concepts underlying the questions in the tool. For any box checked "no," reviewers were told to ask: "What is the potential risk of bias that may be introduced by this flaw?" That is, does this factor cause one to doubt the results that were reported in the study?
NHLBI staff provided reviewers with background reading on critical appraisal, while emphasizing that the best approach to use is to think about the questions in the tool in determining the potential for bias in a study. The staff also emphasized that each study has specific nuances; therefore, reviewers should familiarize themselves with the key concepts.
Quality Assessment of Systematic Reviews and Meta-Analyses - Study Quality Assessment Tools
Guidance for Quality Assessment Tool for Systematic Reviews and Meta-Analyses
A systematic review is a study that attempts to answer a question by synthesizing the results of primary studies while using strategies to limit bias and random error.424 These strategies include a comprehensive search of all potentially relevant articles and the use of explicit, reproducible criteria in the selection of articles included in the review. Research designs and study characteristics are appraised, data are synthesized, and results are interpreted using a predefined systematic approach that adheres to evidence-based methodological principles.
Systematic reviews can be qualitative or quantitative. A qualitative systematic review summarizes the results of the primary studies but does not combine the results statistically. A quantitative systematic review, or meta-analysis, is a type of systematic review that employs statistical techniques to combine the results of the different studies into a single pooled estimate of effect, often given as an odds ratio. The guidance document below is organized by question number from the tool for quality assessment of systematic reviews and meta-analyses.
Question 1. Focused question
The review should be based on a question that is clearly stated and well-formulated. An example would be a question that uses the PICO (population, intervention, comparator, outcome) format, with all components clearly described.
Question 2. Eligibility criteria
The eligibility criteria used to determine whether studies were included or excluded should be clearly specified and predefined. It should be clear to the reader why studies were included or excluded.
Question 3. Literature search
The search strategy should employ a comprehensive, systematic approach in order to capture all of the evidence possible that pertains to the question of interest. At a minimum, a comprehensive review has the following attributes:
- Electronic searches were conducted using multiple scientific literature databases, such as MEDLINE, EMBASE, Cochrane Central Register of Controlled Trials, PsychLit, and others as appropriate for the subject matter.
- Manual searches of references found in articles and textbooks should supplement the electronic searches.
Additional search strategies that may be used to improve the yield include the following:
- Studies published in other countries
- Studies published in languages other than English
- Identification by experts in the field of studies and articles that may have been missed
- Search of grey literature, including technical reports and other papers from government agencies or scientific groups or committees; presentations and posters from scientific meetings, conference proceedings, unpublished manuscripts; and others. Searching the grey literature is important (whenever feasible) because sometimes only positive studies with significant findings are published in the peer-reviewed literature, which can bias the results of a review.
In their reviews, researchers described the literature search strategy clearly, and ascertained it could be reproducible by others with similar results.
Question 4. Dual review for determining which studies to include and exclude
Titles, abstracts, and full-text articles (when indicated) should be reviewed by two independent reviewers to determine which studies to include and exclude in the review. Reviewers resolved disagreements through discussion and consensus or with third parties. They clearly stated the review process, including methods for settling disagreements.
Question 5. Quality appraisal for internal validity
Each included study should be appraised for internal validity (study quality assessment) using a standardized approach for rating the quality of the individual studies. Ideally, this should be done by at least two independent reviewers appraised each study for internal validity. However, there is not one commonly accepted, standardized tool for rating the quality of studies. So, in the research papers, reviewers looked for an assessment of the quality of each study and a clear description of the process used.
Question 6. List and describe included studies
All included studies were listed in the review, along with descriptions of their key characteristics. This was presented either in narrative or table format.
Question 7. Publication bias
Publication bias is a term used when studies with positive results have a higher likelihood of being published, being published rapidly, being published in higher impact journals, being published in English, being published more than once, or being cited by others.425,426 Publication bias can be linked to favorable or unfavorable treatment of research findings due to investigators, editors, industry, commercial interests, or peer reviewers. To minimize the potential for publication bias, researchers can conduct a comprehensive literature search that includes the strategies discussed in Question 3.
A funnel plot–a scatter plot of component studies in a meta-analysis–is a commonly used graphical method for detecting publication bias. If there is no significant publication bias, the graph looks like a symmetrical inverted funnel.
Reviewers assessed and clearly described the likelihood of publication bias.
Question 8. Heterogeneity
Heterogeneity is used to describe important differences in studies included in a meta-analysis that may make it inappropriate to combine the studies.427 Heterogeneity can be clinical (e.g., important differences between study participants, baseline disease severity, and interventions); methodological (e.g., important differences in the design and conduct of the study); or statistical (e.g., important differences in the quantitative results or reported effects).
Researchers usually assess clinical or methodological heterogeneity qualitatively by determining whether it makes sense to combine studies. For example:
- Should a study evaluating the effects of an intervention on CVD risk that involves elderly male smokers with hypertension be combined with a study that involves healthy adults ages 18 to 40? (Clinical Heterogeneity)
- Should a study that uses a randomized controlled trial (RCT) design be combined with a study that uses a case-control study design? (Methodological Heterogeneity)
Statistical heterogeneity describes the degree of variation in the effect estimates from a set of studies; it is assessed quantitatively. The two most common methods used to assess statistical heterogeneity are the Q test (also known as the X2 or chi-square test) or I2 test.
Reviewers examined studies to determine if an assessment for heterogeneity was conducted and clearly described. If the studies are found to be heterogeneous, the investigators should explore and explain the causes of the heterogeneity, and determine what influence, if any, the study differences had on overall study results.
Quality Assessment Tool for Observational Cohort and Cross-Sectional Studies - Study Quality Assessment Tools
Guidance for Assessing the Quality of Observational Cohort and Cross-Sectional Studies
The guidance document below is organized by question number from the tool for quality assessment of observational cohort and cross-sectional studies.
Question 1. Research question
Did the authors describe their goal in conducting this research? Is it easy to understand what they were looking to find? This issue is important for any scientific paper of any type. Higher quality scientific research explicitly defines a research question.
Questions 2 and 3. Study population
Did the authors describe the group of people from which the study participants were selected or recruited, using demographics, location, and time period? If you were to conduct this study again, would you know who to recruit, from where, and from what time period? Is the cohort population free of the outcomes of interest at the time they were recruited?
An example would be men over 40 years old with type 2 diabetes who began seeking medical care at Phoenix Good Samaritan Hospital between January 1, 1990 and December 31, 1994. In this example, the population is clearly described as: (1) who (men over 40 years old with type 2 diabetes); (2) where (Phoenix Good Samaritan Hospital); and (3) when (between January 1, 1990 and December 31, 1994). Another example is women ages 34 to 59 years of age in 1980 who were in the nursing profession and had no known coronary disease, stroke, cancer, hypercholesterolemia, or diabetes, and were recruited from the 11 most populous States, with contact information obtained from State nursing boards.
In cohort studies, it is crucial that the population at baseline is free of the outcome of interest. For example, the nurses' population above would be an appropriate group in which to study incident coronary disease. This information is usually found either in descriptions of population recruitment, definitions of variables, or inclusion/exclusion criteria.
You may need to look at prior papers on methods in order to make the assessment for this question. Those papers are usually in the reference list.
If fewer than 50% of eligible persons participated in the study, then there is concern that the study population does not adequately represent the target population. This increases the risk of bias.
Question 4. Groups recruited from the same population and uniform eligibility criteria
Were the inclusion and exclusion criteria developed prior to recruitment or selection of the study population? Were the same underlying criteria used for all of the subjects involved? This issue is related to the description of the study population, above, and you may find the information for both of these questions in the same section of the paper.
Most cohort studies begin with the selection of the cohort; participants in this cohort are then measured or evaluated to determine their exposure status. However, some cohort studies may recruit or select exposed participants in a different time or place than unexposed participants, especially retrospective cohort studies–which is when data are obtained from the past (retrospectively), but the analysis examines exposures prior to outcomes. For example, one research question could be whether diabetic men with clinical depression are at higher risk for cardiovascular disease than those without clinical depression. So, diabetic men with depression might be selected from a mental health clinic, while diabetic men without depression might be selected from an internal medicine or endocrinology clinic. This study recruits groups from different clinic populations, so this example would get a "no."
However, the women nurses described in the question above were selected based on the same inclusion/exclusion criteria, so that example would get a "yes."
Question 5. Sample size justification
Did the authors present their reasons for selecting or recruiting the number of people included or analyzed? Do they note or discuss the statistical power of the study? This question is about whether or not the study had enough participants to detect an association if one truly existed.
A paragraph in the methods section of the article may explain the sample size needed to detect a hypothesized difference in outcomes. You may also find a discussion of power in the discussion section (such as the study had 85 percent power to detect a 20 percent increase in the rate of an outcome of interest, with a 2-sided alpha of 0.05). Sometimes estimates of variance and/or estimates of effect size are given, instead of sample size calculations. In any of these cases, the answer would be "yes."
However, observational cohort studies often do not report anything about power or sample sizes because the analyses are exploratory in nature. In this case, the answer would be "no." This is not a "fatal flaw." It just may indicate that attention was not paid to whether the study was sufficiently sized to answer a prespecified question–i.e., it may have been an exploratory, hypothesis-generating study.
Question 6. Exposure assessed prior to outcome measurement
This question is important because, in order to determine whether an exposure causes an outcome, the exposure must come before the outcome.
For some prospective cohort studies, the investigator enrolls the cohort and then determines the exposure status of various members of the cohort (large epidemiological studies like Framingham used this approach). However, for other cohort studies, the cohort is selected based on its exposure status, as in the example above of depressed diabetic men (the exposure being depression). Other examples include a cohort identified by its exposure to fluoridated drinking water and then compared to a cohort living in an area without fluoridated water, or a cohort of military personnel exposed to combat in the Gulf War compared to a cohort of military personnel not deployed in a combat zone.
With either of these types of cohort studies, the cohort is followed forward in time (i.e., prospectively) to assess the outcomes that occurred in the exposed members compared to nonexposed members of the cohort. Therefore, you begin the study in the present by looking at groups that were exposed (or not) to some biological or behavioral factor, intervention, etc., and then you follow them forward in time to examine outcomes. If a cohort study is conducted properly, the answer to this question should be "yes," since the exposure status of members of the cohort was determined at the beginning of the study before the outcomes occurred.
For retrospective cohort studies, the same principal applies. The difference is that, rather than identifying a cohort in the present and following them forward in time, the investigators go back in time (i.e., retrospectively) and select a cohort based on their exposure status in the past and then follow them forward to assess the outcomes that occurred in the exposed and nonexposed cohort members. Because in retrospective cohort studies the exposure and outcomes may have already occurred (it depends on how long they follow the cohort), it is important to make sure that the exposure preceded the outcome.
Sometimes cross-sectional studies are conducted (or cross-sectional analyses of cohort-study data), where the exposures and outcomes are measured during the same timeframe. As a result, cross-sectional analyses provide weaker evidence than regular cohort studies regarding a potential causal relationship between exposures and outcomes. For cross-sectional analyses, the answer to Question 6 should be "no."
Question 7. Sufficient timeframe to see an effect
Did the study allow enough time for a sufficient number of outcomes to occur or be observed, or enough time for an exposure to have a biological effect on an outcome? In the examples given above, if clinical depression has a biological effect on increasing risk for CVD, such an effect may take years. In the other example, if higher dietary sodium increases BP, a short timeframe may be sufficient to assess its association with BP, but a longer timeframe would be needed to examine its association with heart attacks.
The issue of timeframe is important to enable meaningful analysis of the relationships between exposures and outcomes to be conducted. This often requires at least several years, especially when looking at health outcomes, but it depends on the research question and outcomes being examined.
Cross-sectional analyses allow no time to see an effect, since the exposures and outcomes are assessed at the same time, so those would get a "no" response.
Question 8. Different levels of the exposure of interest
If the exposure can be defined as a range (examples: drug dosage, amount of physical activity, amount of sodium consumed), were multiple categories of that exposure assessed? (for example, for drugs: not on the medication, on a low dose, medium dose, high dose; for dietary sodium, higher than average U.S. consumption, lower than recommended consumption, between the two). Sometimes discrete categories of exposure are not used, but instead exposures are measured as continuous variables (for example, mg/day of dietary sodium or BP values).
In any case, studying different levels of exposure (where possible) enables investigators to assess trends or dose-response relationships between exposures and outcomes–e.g., the higher the exposure, the greater the rate of the health outcome. The presence of trends or dose-response relationships lends credibility to the hypothesis of causality between exposure and outcome.
For some exposures, however, this question may not be applicable (e.g., the exposure may be a dichotomous variable like living in a rural setting versus an urban setting, or vaccinated/not vaccinated with a one-time vaccine). If there are only two possible exposures (yes/no), then this question should be given an "NA," and it should not count negatively towards the quality rating.
Question 9. Exposure measures and assessment
Were the exposure measures defined in detail? Were the tools or methods used to measure exposure accurate and reliable–for example, have they been validated or are they objective? This issue is important as it influences confidence in the reported exposures. When exposures are measured with less accuracy or validity, it is harder to see an association between exposure and outcome even if one exists. Also as important is whether the exposures were assessed in the same manner within groups and between groups; if not, bias may result.
For example, retrospective self-report of dietary salt intake is not as valid and reliable as prospectively using a standardized dietary log plus testing participants' urine for sodium content. Another example is measurement of BP, where there may be quite a difference between usual care, where clinicians measure BP however it is done in their practice setting (which can vary considerably), and use of trained BP assessors using standardized equipment (e.g., the same BP device which has been tested and calibrated) and a standardized protocol (e.g., patient is seated for 5 minutes with feet flat on the floor, BP is taken twice in each arm, and all four measurements are averaged). In each of these cases, the former would get a "no" and the latter a "yes."
Here is a final example that illustrates the point about why it is important to assess exposures consistently across all groups: If people with higher BP (exposed cohort) are seen by their providers more frequently than those without elevated BP (nonexposed group), it also increases the chances of detecting and documenting changes in health outcomes, including CVD-related events. Therefore, it may lead to the conclusion that higher BP leads to more CVD events. This may be true, but it could also be due to the fact that the subjects with higher BP were seen more often; thus, more CVD-related events were detected and documented simply because they had more encounters with the health care system. Thus, it could bias the results and lead to an erroneous conclusion.
Question 10. Repeated exposure assessment
Was the exposure for each person measured more than once during the course of the study period? Multiple measurements with the same result increase our confidence that the exposure status was correctly classified. Also, multiple measurements enable investigators to look at changes in exposure over time, for example, people who ate high dietary sodium throughout the followup period, compared to those who started out high then reduced their intake, compared to those who ate low sodium throughout. Once again, this may not be applicable in all cases. In many older studies, exposure was measured only at baseline. However, multiple exposure measurements do result in a stronger study design.
Question 11. Outcome measures
Were the outcomes defined in detail? Were the tools or methods for measuring outcomes accurate and reliable–for example, have they been validated or are they objective? This issue is important because it influences confidence in the validity of study results. Also important is whether the outcomes were assessed in the same manner within groups and between groups.
An example of an outcome measure that is objective, accurate, and reliable is death–the outcome measured with more accuracy than any other. But even with a measure as objective as death, there can be differences in the accuracy and reliability of how death was assessed by the investigators. Did they base it on an autopsy report, death certificate, death registry, or report from a family member? Another example is a study of whether dietary fat intake is related to blood cholesterol level (cholesterol level being the outcome), and the cholesterol level is measured from fasting blood samples that are all sent to the same laboratory. These examples would get a "yes." An example of a "no" would be self-report by subjects that they had a heart attack, or self-report of how much they weigh (if body weight is the outcome of interest).
Similar to the example in Question 9, results may be biased if one group (e.g., people with high BP) is seen more frequently than another group (people with normal BP) because more frequent encounters with the health care system increases the chances of outcomes being detected and documented.
Question 12. Blinding of outcome assessors
Blinding means that outcome assessors did not know whether the participant was exposed or unexposed. It is also sometimes called "masking." The objective is to look for evidence in the article that the person(s) assessing the outcome(s) for the study (for example, examining medical records to determine the outcomes that occurred in the exposed and comparison groups) is masked to the exposure status of the participant. Sometimes the person measuring the exposure is the same person conducting the outcome assessment. In this case, the outcome assessor would most likely not be blinded to exposure status because they also took measurements of exposures. If so, make a note of that in the comments section.
As you assess this criterion, think about whether it is likely that the person(s) doing the outcome assessment would know (or be able to figure out) the exposure status of the study participants. If the answer is no, then blinding is adequate. An example of adequate blinding of the outcome assessors is to create a separate committee, whose members were not involved in the care of the patient and had no information about the study participants' exposure status. The committee would then be provided with copies of participants' medical records, which had been stripped of any potential exposure information or personally identifiable information. The committee would then review the records for prespecified outcomes according to the study protocol. If blinding was not possible, which is sometimes the case, mark "NA" and explain the potential for bias.
Question 13. Followup rate
Higher overall followup rates are always better than lower followup rates, even though higher rates are expected in shorter studies, whereas lower overall followup rates are often seen in studies of longer duration. Usually, an acceptable overall followup rate is considered 80 percent or more of participants whose exposures were measured at baseline. However, this is just a general guideline. For example, a 6-month cohort study examining the relationship between dietary sodium intake and BP level may have over 90 percent followup, but a 20-year cohort study examining effects of sodium intake on stroke may have only a 65 percent followup rate.
Question 14. Statistical analyses
Were key potential confounding variables measured and adjusted for, such as by statistical adjustment for baseline differences? Logistic regression or other regression methods are often used to account for the influence of variables not of interest.
This is a key issue in cohort studies, because statistical analyses need to control for potential confounders, in contrast to an RCT, where the randomization process controls for potential confounders. All key factors that may be associated both with the exposure of interest and the outcome–that are not of interest to the research question–should be controlled for in the analyses.
For example, in a study of the relationship between cardiorespiratory fitness and CVD events (heart attacks and strokes), the study should control for age, BP, blood cholesterol, and body weight, because all of these factors are associated both with low fitness and with CVD events. Well-done cohort studies control for multiple potential confounders.
Some general guidance for determining the overall quality rating of observational cohort and cross-sectional studies
The questions on the form are designed to help you focus on the key concepts for evaluating the internal validity of a study. They are not intended to create a list that you simply tally up to arrive at a summary judgment of quality.
Internal validity for cohort studies is the extent to which the results reported in the study can truly be attributed to the exposure being evaluated and not to flaws in the design or conduct of the study–in other words, the ability of the study to draw associative conclusions about the effects of the exposures being studied on outcomes. Any such flaws can increase the risk of bias.
Critical appraisal involves considering the risk of potential for selection bias, information bias, measurement bias, or confounding (the mixture of exposures that one cannot tease out from each other). Examples of confounding include co-interventions, differences at baseline in patient characteristics, and other issues throughout the questions above. High risk of bias translates to a rating of poor quality. Low risk of bias translates to a rating of good quality. (Thus, the greater the risk of bias, the lower the quality rating of the study.)
In addition, the more attention in the study design to issues that can help determine whether there is a causal relationship between the exposure and outcome, the higher quality the study. These include exposures occurring prior to outcomes, evaluation of a dose-response gradient, accuracy of measurement of both exposure and outcome, sufficient timeframe to see an effect, and appropriate control for confounding–all concepts reflected in the tool.
Generally, when you evaluate a study, you will not see a "fatal flaw," but you will find some risk of bias. By focusing on the concepts underlying the questions in the quality assessment tool, you should ask yourself about the potential for bias in the study you are critically appraising. For any box where you check "no" you should ask, "What is the potential risk of bias resulting from this flaw in study design or execution?" That is, does this factor cause you to doubt the results that are reported in the study or doubt the ability of the study to accurately assess an association between exposure and outcome?
The best approach is to think about the questions in the tool and how each one tells you something about the potential for bias in a study. The more you familiarize yourself with the key concepts, the more comfortable you will be with critical appraisal. Examples of studies rated good, fair, and poor are useful, but each study must be assessed on its own based on the details that are reported and consideration of the concepts for minimizing bias.
Quality Assessment of Case-Control Studies - Study Quality Assessment Tools
Guidance for Assessing the Quality of Case-Control Studies
The guidance document below is organized by question number from the tool for quality assessment of case-control studies.
Did the authors describe their goal in conducting this research? Is it easy to understand what they were looking to find? This issue is important for any scientific paper of any type. High quality scientific research explicitly defines a research question.
Question 2. Study population
Did the authors describe the group of individuals from which the cases and controls were selected or recruited, while using demographics, location, and time period? If the investigators conducted this study again, would they know exactly who to recruit, from where, and from what time period?
Investigators identify case-control study populations by location, time period, and inclusion criteria for cases (individuals with the disease, condition, or problem) and controls (individuals without the disease, condition, or problem). For example, the population for a study of lung cancer and chemical exposure would be all incident cases of lung cancer diagnosed in patients ages 35 to 79, from January 1, 2003 to December 31, 2008, living in Texas during that entire time period, as well as controls without lung cancer recruited from the same population during the same time period. The population is clearly described as: (1) who (men and women ages 35 to 79 with (cases) and without (controls) incident lung cancer); (2) where (living in Texas); and (3) when (between January 1, 2003 and December 31, 2008).
Other studies may use disease registries or data from cohort studies to identify cases. In these cases, the populations are individuals who live in the area covered by the disease registry or included in a cohort study (i.e., nested case-control or case-cohort). For example, a study of the relationship between vitamin D intake and myocardial infarction might use patients identified via the GRACE registry, a database of heart attack patients.
NHLBI staff encouraged reviewers to examine prior papers on methods (listed in the reference list) to make this assessment, if necessary.
Question 3. Target population and case representation
In order for a study to truly address the research question, the target population–the population from which the study population is drawn and to which study results are believed to apply–should be carefully defined. Some authors may compare characteristics of the study cases to characteristics of cases in the target population, either in text or in a table. When study cases are shown to be representative of cases in the appropriate target population, it increases the likelihood that the study was well-designed per the research question.
However, because these statistics are frequently difficult or impossible to measure, publications should not be penalized if case representation is not shown. For most papers, the response to question 3 will be "NR." Those subquestions are combined because the answer to the second subquestion–case representation–determines the response to this item. However, it cannot be determined without considering the response to the first subquestion. For example, if the answer to the first subquestion is "yes," and the second, "CD," then the response for item 3 is "CD."
Question 4. Sample size justification
Did the authors discuss their reasons for selecting or recruiting the number of individuals included? Did they discuss the statistical power of the study and provide a sample size calculation to ensure that the study is adequately powered to detect an association (if one exists)? This question does not refer to a description of the manner in which different groups were included or excluded using the inclusion/exclusion criteria (e.g., "Final study size was 1,378 participants after exclusion of 461 patients with missing data" is not considered a sample size justification for the purposes of this question).
An article's methods section usually contains information on sample size and the size needed to detect differences in exposures and on statistical power.
Question 5. Groups recruited from the same population
To determine whether cases and controls were recruited from the same population, one can ask hypothetically, "If a control was to develop the outcome of interest (the condition that was used to select cases), would that person have been eligible to become a case?" Case-control studies begin with the selection of the cases (those with the outcome of interest, e.g., lung cancer) and controls (those in whom the outcome is absent). Cases and controls are then evaluated and categorized by their exposure status. For the lung cancer example, cases and controls were recruited from hospitals in a given region. One may reasonably assume that controls in the catchment area for the hospitals, or those already in the hospitals for a different reason, would attend those hospitals if they became a case; therefore, the controls are drawn from the same population as the cases. If the controls were recruited or selected from a different region (e.g., a State other than Texas) or time period (e.g., 1991-2000), then the cases and controls were recruited from different populations, and the answer to this question would be "no."
The following example further explores selection of controls. In a study, eligible cases were men and women, ages 18 to 39, who were diagnosed with atherosclerosis at hospitals in Perth, Australia, between July 1, 2000 and December 31, 2007. Appropriate controls for these cases might be sampled using voter registration information for men and women ages 18 to 39, living in Perth (population-based controls); they also could be sampled from patients without atherosclerosis at the same hospitals (hospital-based controls). As long as the controls are individuals who would have been eligible to be included in the study as cases (if they had been diagnosed with atherosclerosis), then the controls were selected appropriately from the same source population as cases.
In a prospective case-control study, investigators may enroll individuals as cases at the time they are found to have the outcome of interest; the number of cases usually increases as time progresses. At this same time, they may recruit or select controls from the population without the outcome of interest. One way to identify or recruit cases is through a surveillance system. In turn, investigators can select controls from the population covered by that system. This is an example of population-based controls. Investigators also may identify and select cases from a cohort study population and identify controls from outcome-free individuals in the same cohort study. This is known as a nested case-control study.
Question 6. Inclusion and exclusion criteria prespecified and applied uniformly
Were the inclusion and exclusion criteria developed prior to recruitment or selection of the study population? Were the same underlying criteria used for all of the groups involved? To answer this question, reviewers determined if the investigators developed I/E criteria prior to recruitment or selection of the study population and if they used the same underlying criteria for all groups. The investigators should have used the same selection criteria, except for study participants who had the disease or condition, which would be different for cases and controls by definition. Therefore, the investigators use the same age (or age range), gender, race, and other characteristics to select cases and controls. Information on this topic is usually found in a paper's section on the description of the study population.
Question 7. Case and control definitions
For this question, reviewers looked for descriptions of the validity of case and control definitions and processes or tools used to identify study participants as such. Was a specific description of "case" and "control" provided? Is there a discussion of the validity of the case and control definitions and the processes or tools used to identify study participants as such? They determined if the tools or methods were accurate, reliable, and objective. For example, cases might be identified as "adult patients admitted to a VA hospital from January 1, 2000 to December 31, 2009, with an ICD-9 discharge diagnosis code of acute myocardial infarction and at least one of the two confirmatory findings in their medical records: at least 2mm of ST elevation changes in two or more ECG leads and an elevated troponin level. Investigators might also use ICD-9 or CPT codes to identify patients. All cases should be identified using the same methods. Unless the distinction between cases and controls is accurate and reliable, investigators cannot use study results to draw valid conclusions.
Question 8. Random selection of study participants
If a case-control study did not use 100 percent of eligible cases and/or controls (e.g., not all disease-free participants were included as controls), did the authors indicate that random sampling was used to select controls? When it is possible to identify the source population fairly explicitly (e.g., in a nested case-control study, or in a registry-based study), then random sampling of controls is preferred. When investigators used consecutive sampling, which is frequently done for cases in prospective studies, then study participants are not considered randomly selected. In this case, the reviewers would answer "no" to Question 8. However, this would not be considered a fatal flaw.
If investigators included all eligible cases and controls as study participants, then reviewers marked "NA" in the tool. If 100 percent of cases were included (e.g., NA for cases) but only 50 percent of eligible controls, then the response would be "yes" if the controls were randomly selected, and "no" if they were not. If this cannot be determined, the appropriate response is "CD."
Question 9. Concurrent controls
A concurrent control is a control selected at the time another person became a case, usually on the same day. This means that one or more controls are recruited or selected from the population without the outcome of interest at the time a case is diagnosed. Investigators can use this method in both prospective case-control studies and retrospective case-control studies. For example, in a retrospective study of adenocarcinoma of the colon using data from hospital records, if hospital records indicate that Person A was diagnosed with adenocarcinoma of the colon on June 22, 2002, then investigators would select one or more controls from the population of patients without adenocarcinoma of the colon on that same day. This assumes they conducted the study retrospectively, using data from hospital records. The investigators could have also conducted this study using patient records from a cohort study, in which case it would be a nested case-control study.
Investigators can use concurrent controls in the presence or absence of matching and vice versa. A study that uses matching does not necessarily mean that concurrent controls were used.
Question 10. Exposure assessed prior to outcome measurement
Investigators first determine case or control status (based on presence or absence of outcome of interest), and then assess exposure history of the case or control; therefore, reviewers ascertained that the exposure preceded the outcome. For example, if the investigators used tissue samples to determine exposure, did they collect them from patients prior to their diagnosis? If hospital records were used, did investigators verify that the date a patient was exposed (e.g., received medication for atherosclerosis) occurred prior to the date they became a case (e.g., was diagnosed with type 2 diabetes)? For an association between an exposure and an outcome to be considered causal, the exposure must have occurred prior to the outcome.
Question 11. Exposure measures and assessment
Were the exposure measures defined in detail? Were the tools or methods used to measure exposure accurate and reliable–for example, have they been validated or are they objective? This is important, as it influences confidence in the reported exposures. Equally important is whether the exposures were assessed in the same manner within groups and between groups. This question pertains to bias resulting from exposure misclassification (i.e., exposure ascertainment).
For example, a retrospective self-report of dietary salt intake is not as valid and reliable as prospectively using a standardized dietary log plus testing participants' urine for sodium content because participants' retrospective recall of dietary salt intake may be inaccurate and result in misclassification of exposure status. Similarly, BP results from practices that use an established protocol for measuring BP would be considered more valid and reliable than results from practices that did not use standard protocols. A protocol may include using trained BP assessors, standardized equipment (e.g., the same BP device which has been tested and calibrated), and a standardized procedure (e.g., patient is seated for 5 minutes with feet flat on the floor, BP is taken twice in each arm, and all four measurements are averaged).
Question 12. Blinding of exposure assessors
Blinding or masking means that outcome assessors did not know whether participants were exposed or unexposed. To answer this question, reviewers examined articles for evidence that the outcome assessor(s) was masked to the exposure status of the research participants. An outcome assessor, for example, may examine medical records to determine the outcomes that occurred in the exposed and comparison groups. Sometimes the person measuring the exposure is the same person conducting the outcome assessment. In this case, the outcome assessor would most likely not be blinded to exposure status. A reviewer would note such a finding in the comments section of the assessment tool.
One way to ensure good blinding of exposure assessment is to have a separate committee, whose members have no information about the study participants' status as cases or controls, review research participants' records. To help answer the question above, reviewers determined if it was likely that the outcome assessor knew whether the study participant was a case or control. If it was unlikely, then the reviewers marked "no" to Question 12. Outcome assessors who used medical records to assess exposure should not have been directly involved in the study participants' care, since they probably would have known about their patients' conditions. If the medical records contained information on the patient's condition that identified him/her as a case (which is likely), that information would have had to be removed before the exposure assessors reviewed the records.
If blinding was not possible, which sometimes happens, the reviewers marked "NA" in the assessment tool and explained the potential for bias.
Question 13. Statistical analysis
Were key potential confounding variables measured and adjusted for, such as by statistical adjustment for baseline differences? Investigators often use logistic regression or other regression methods to account for the influence of variables not of interest.
This is a key issue in case-controlled studies; statistical analyses need to control for potential confounders, in contrast to RCTs in which the randomization process controls for potential confounders. In the analysis, investigators need to control for all key factors that may be associated with both the exposure of interest and the outcome and are not of interest to the research question.
A study of the relationship between smoking and CVD events illustrates this point. Such a study needs to control for age, gender, and body weight; all are associated with smoking and CVD events. Well-done case-control studies control for multiple potential confounders.
Matching is a technique used to improve study efficiency and control for known confounders. For example, in the study of smoking and CVD events, an investigator might identify cases that have had a heart attack or stroke and then select controls of similar age, gender, and body weight to the cases. For case-control studies, it is important that if matching was performed during the selection or recruitment process, the variables used as matching criteria (e.g., age, gender, race) should be controlled for in the analysis.
General Guidance for Determining the Overall Quality Rating of Case-Controlled Studies
NHLBI designed the questions in the assessment tool to help reviewers focus on the key concepts for evaluating a study's internal validity, not to use as a list from which to add up items to judge a study's quality.
Internal validity for case-control studies is the extent to which the associations between disease and exposure reported in the study can truly be attributed to the exposure being evaluated rather than to flaws in the design or conduct of the study. In other words, what is ability of the study to draw associative conclusions about the effects of the exposures on outcomes? Any such flaws can increase the risk of bias.
In critical appraising a study, the following factors need to be considered: risk of potential for selection bias, information bias, measurement bias, or confounding (the mixture of exposures that one cannot tease out from each other). Examples of confounding include co-interventions, differences at baseline in patient characteristics, and other issues addressed in the questions above. High risk of bias translates to a poor quality rating; low risk of bias translates to a good quality rating. Again, the greater the risk of bias, the lower the quality rating of the study.
In addition, the more attention in the study design to issues that can help determine whether there is a causal relationship between the outcome and the exposure, the higher the quality of the study. These include exposures occurring prior to outcomes, evaluation of a dose-response gradient, accuracy of measurement of both exposure and outcome, sufficient timeframe to see an effect, and appropriate control for confounding–all concepts reflected in the tool.
If a study has a "fatal flaw," then risk of bias is significant; therefore, the study is deemed to be of poor quality. An example of a fatal flaw in case-control studies is a lack of a consistent standard process used to identify cases and controls.
Generally, when reviewers evaluated a study, they did not see a "fatal flaw," but instead found some risk of bias. By focusing on the concepts underlying the questions in the quality assessment tool, reviewers examined the potential for bias in the study. For any box checked "no," reviewers asked, "What is the potential risk of bias resulting from this flaw in study design or execution?" That is, did this factor lead to doubt about the results reported in the study or the ability of the study to accurately assess an association between exposure and outcome?
By examining questions in the assessment tool, reviewers were best able to assess the potential for bias in a study. Specific rules were not useful, as each study had specific nuances. In addition, being familiar with the key concepts helped reviewers assess the studies. Examples of studies rated good, fair, and poor were useful, yet each study had to be assessed on its own.
Quality Assessment Tool for Before-After (Pre-Post) Studies With No Control Group - Study Quality Assessment Tools
Guidance for Assessing the Quality of Before-After (Pre-Post) Studies With No Control Group
Question 1. Study question
Question 2. Eligibility criteria and study population
Did the authors describe the eligibility criteria applied to the individuals from whom the study participants were selected or recruited? In other words, if the investigators were to conduct this study again, would they know whom to recruit, from where, and from what time period?
Here is a sample description of a study population: men over age 40 with type 2 diabetes, who began seeking medical care at Phoenix Good Samaritan Hospital, between January 1, 2005 and December 31, 2007. The population is clearly described as: (1) who (men over age 40 with type 2 diabetes); (2) where (Phoenix Good Samaritan Hospital); and (3) when (between January 1, 2005 and December 31, 2007). Another sample description is women who were in the nursing profession, who were ages 34 to 59 in 1995, had no known CHD, stroke, cancer, hypercholesterolemia, or diabetes, and were recruited from the 11 most populous States, with contact information obtained from State nursing boards.
To assess this question, reviewers examined prior papers on study methods (listed in reference list) when necessary.
Question 3. Study participants representative of clinical populations of interest
The participants in the study should be generally representative of the population in which the intervention will be broadly applied. Studies on small demographic subgroups may raise concerns about how the intervention will affect broader populations of interest. For example, interventions that focus on very young or very old individuals may affect middle-aged adults differently. Similarly, researchers may not be able to extrapolate study results from patients with severe chronic diseases to healthy populations.
Question 4. All eligible participants enrolled
To further explore this question, reviewers may need to ask: Did the investigators develop the I/E criteria prior to recruiting or selecting study participants? Were the same underlying I/E criteria used for all research participants? Were all subjects who met the I/E criteria enrolled in the study?
Question 5. Sample size
Did the authors present their reasons for selecting or recruiting the number of individuals included or analyzed? Did they note or discuss the statistical power of the study? This question addresses whether there was a sufficient sample size to detect an association, if one did exist.
An article's methods section may provide information on the sample size needed to detect a hypothesized difference in outcomes and a discussion on statistical power (such as, the study had 85 percent power to detect a 20 percent increase in the rate of an outcome of interest, with a 2-sided alpha of 0.05). Sometimes estimates of variance and/or estimates of effect size are given, instead of sample size calculations. In any case, if the reviewers determined that the power was sufficient to detect the effects of interest, then they would answer "yes" to Question 5.
Question 6. Intervention clearly described
Another pertinent question regarding interventions is: Was the intervention clearly defined in detail in the study? Did the authors indicate that the intervention was consistently applied to the subjects? Did the research participants have a high level of adherence to the requirements of the intervention? For example, if the investigators assigned a group to 10 mg/day of Drug A, did most participants in this group take the specific dosage of Drug A? Or did a large percentage of participants end up not taking the specific dose of Drug A indicated in the study protocol?
Reviewers ascertained that changes in study outcomes could be attributed to study interventions. If participants received interventions that were not part of the study protocol and could affect the outcomes being assessed, the results could be biased.
Question 7. Outcome measures clearly described, valid, and reliable
Were the outcomes defined in detail? Were the tools or methods for measuring outcomes accurate and reliable–for example, have they been validated or are they objective? This question is important because the answer influences confidence in the validity of study results.
An example of an outcome measure that is objective, accurate, and reliable is death–the outcome measured with more accuracy than any other. But even with a measure as objective as death, differences can exist in the accuracy and reliability of how investigators assessed death. For example, did they base it on an autopsy report, death certificate, death registry, or report from a family member? Another example of a valid study is one whose objective is to determine if dietary fat intake affects blood cholesterol level (cholesterol level being the outcome) and in which the cholesterol level is measured from fasting blood samples that are all sent to the same laboratory. These examples would get a "yes."
An example of a "no" would be self-report by subjects that they had a heart attack, or self-report of how much they weight (if body weight is the outcome of interest).
Question 8. Blinding of outcome assessors
Blinding or masking means that the outcome assessors did not know whether the participants received the intervention or were exposed to the factor under study. To answer the question above, the reviewers examined articles for evidence that the person(s) assessing the outcome(s) was masked to the participants' intervention or exposure status. An outcome assessor, for example, may examine medical records to determine the outcomes that occurred in the exposed and comparison groups. Sometimes the person applying the intervention or measuring the exposure is the same person conducting the outcome assessment. In this case, the outcome assessor would not likely be blinded to the intervention or exposure status. A reviewer would note such a finding in the comments section of the assessment tool.
In assessing this criterion, the reviewers determined whether it was likely that the person(s) conducting the outcome assessment knew the exposure status of the study participants. If not, then blinding was adequate. An example of adequate blinding of the outcome assessors is to create a separate committee whose members were not involved in the care of the patient and had no information about the study participants' exposure status. Using a study protocol, committee members would review copies of participants' medical records, which would be stripped of any potential exposure information or personally identifiable information, for prespecified outcomes.
Question 9. Followup rate
Higher overall followup rates are always desirable to lower followup rates, although higher rates are expected in shorter studies, and lower overall followup rates are often seen in longer studies. Usually an acceptable overall followup rate is considered 80 percent or more of participants whose interventions or exposures were measured at baseline. However, this is a general guideline.
In accounting for those lost to followup, in the analysis, investigators may have imputed values of the outcome for those lost to followup or used other methods. For example, they may carry forward the baseline value or the last observed value of the outcome measure and use these as imputed values for the final outcome measure for research participants lost to followup.
Question 10. Statistical analysis
Were formal statistical tests used to assess the significance of the changes in the outcome measures between the before and after time periods? The reported study results should present values for statistical tests, such as p values, to document the statistical significance (or lack thereof) for the changes in the outcome measures found in the study.
Question 11. Multiple outcome measures
Were the outcome measures for each person measured more than once during the course of the before and after study periods? Multiple measurements with the same result increase confidence that the outcomes were accurately measured.
Question 12. Group-level interventions and individual-level outcome efforts
Group-level interventions are usually not relevant for clinical interventions such as bariatric surgery, in which the interventions are applied at the individual patient level. In those cases, the questions were coded as "NA" in the assessment tool.
General Guidance for Determining the Overall Quality Rating of Before-After Studies
The questions in the quality assessment tool were designed to help reviewers focus on the key concepts for evaluating the internal validity of a study. They are not intended to create a list from which to add up items to judge a study's quality.
Internal validity is the extent to which the outcome results reported in the study can truly be attributed to the intervention or exposure being evaluated, and not to biases, measurement errors, or other confounding factors that may result from flaws in the design or conduct of the study. In other words, what is the ability of the study to draw associative conclusions about the effects of the interventions or exposures on outcomes?
Critical appraisal of a study involves considering the risk of potential for selection bias, information bias, measurement bias, or confounding (the mixture of exposures that one cannot tease out from each other). Examples of confounding include co-interventions, differences at baseline in patient characteristics, and other issues throughout the questions above. High risk of bias translates to a rating of poor quality; low risk of bias translates to a rating of good quality. Again, the greater the risk of bias, the lower the quality rating of the study.
In addition, the more attention in the study design to issues that can help determine if there is a causal relationship between the exposure and outcome, the higher quality the study. These issues include exposures occurring prior to outcomes, evaluation of a dose-response gradient, accuracy of measurement of both exposure and outcome, and sufficient timeframe to see an effect.
Generally, when reviewers evaluate a study, they will not see a "fatal flaw," but instead will find some risk of bias. By focusing on the concepts underlying the questions in the quality assessment tool, reviewers should ask themselves about the potential for bias in the study they are critically appraising. For any box checked "no" reviewers should ask, "What is the potential risk of bias resulting from this flaw in study design or execution?" That is, does this factor lead to doubt about the results reported in the study or doubt about the ability of the study to accurately assess an association between the intervention or exposure and the outcome?
The best approach is to think about the questions in the assessment tool and how each one reveals something about the potential for bias in a study. Specific rules are not useful, as each study has specific nuances. In addition, being familiar with the key concepts will help reviewers be more comfortable with critical appraisal. Examples of studies rated good, fair, and poor are useful, but each study must be assessed on its own.
Quality Assessment Tool for Case Series Studies - Study Quality Assessment Tools
Background: development and use - study quality assessment tools.
Learn more about the development and use of Study Quality Assessment Tools.
Last updated: July, 2021
Statistical quality control: A case study research
Ieee account.
- Change Username/Password
- Update Address
Purchase Details
- Payment Options
- Order History
- View Purchased Documents
Profile Information
- Communications Preferences
- Profession and Education
- Technical Interests
- US & Canada: +1 800 678 4333
- Worldwide: +1 732 981 0060
- Contact & Support
- About IEEE Xplore
- Accessibility
- Terms of Use
- Nondiscrimination Policy
- Privacy & Opting Out of Cookies
A not-for-profit organization, IEEE is the world's largest technical professional organization dedicated to advancing technology for the benefit of humanity. © Copyright 2024 IEEE - All rights reserved. Use of this web site signifies your agreement to the terms and conditions.
Analytical Instruments & Supplies
- Chromatography
- Mass Spectrometry
- Certified Pre-Owned Instruments
- Spectroscopy
- Capillary Electrophoresis
- Chromatography & Spectroscopy Lab Supplies
- Instrument Repair
- Sample Preparation
- Chemical Standards
Life Science
- Cell Analysis
- Automated Electrophoresis
- Microarray Solutions
- Mutagenesis & Cloning
- Next Generation Sequencing
- Research Flow Cytometry
- PCR/Real-Time PCR (qPCR)
- CRISPR/Cas9
- Microscopes and Microplate Instrumentation
- Oligo Pools & Oligo GMP Manufacturing
Clinical & Diagnostic Testing
- Immunohistochemistry
- Companion Diagnostics
- Hematoxylin & Eosin
- Special Stains
- In Situ Hybridization
- Clinical Flow Cytometry
- Specific Proteins
- Clinical Microplate Instrumentation
Lab Management & Consulting
- Lab Management
- Lab Consulting
Lab Software
- Software & Informatics
- Genomics Informatics
Lab Supplies
- Microplates
Dissolution Testing
- Dissolution
Lab Automation
- Automated Liquid Handling
Vacuum & Leak Detection
- Vacuum Technologies
- Leak Detection
- Applications & Industries
- Biopharma/Pharma
- Cancer Research
- Cannabis & Hemp Testing
- Clinical Diagnostics
- Clinical Research
- Infectious Disease
- Energy & Chemicals
- Environmental
- Food & Beverage Testing
- Materials Testing & Research
- Security, Defense & First Response
- Vacuum Solutions
- Training & Events
Mass spectrometry, chromatography, spectroscopy, software, dissolution, sample handling and vacuum technologies courses
On-demand continuing education
Instrument training and workshops
Live or on-demand webinars on product introductions, applications and software enhancements
Worldwide trade shows, conferences, local seminars and user group meetings

Lab Management Services
Service Plans, On Demand Repair, Preventive Maintenance, and Service Center Repair
Software to manage instrument access, sample processing, inventories, and more
Instrument/software qualifications, consulting, and data integrity validations
Learn essential lab skills and enhance your workflows
Instrument & equipment deinstallation, transportation, and reinstallation
Other Services Header1
CrossLab Connect services use laboratory data to improve control and decision-making
Advance lab operations with lab-wide services, asset management, relocation
Shorten the time it takes to start seeing the full value of your instrument investment
Other Services Header2
- Agilent Community
- Financial Solutions
- Agilent University
- Instrument Trade-In & BuyBack
Pathology Services
- Lab Solution Deployment Services
- Instrument & Solution Services
- Training & Application Services
- Workflow & Connectivity Services
Nucleic Acid Therapeutics
- Oligonucleotide GMP Manufacturing
Vacuum Product & Leak Detector Services
- Advance Exchange Service
- Repair Support Services & Spare Parts
- Support Services, Agreements & Training
- Technology Refresh & Upgrade
- Leak Detector Services
- Support & Resources
Technical Support
- Instrument Support Resources
- Columns, Supplies, & Standards
- Contact Support
- See All Technical Support
Purchase & Order Support
- Instrument Subscriptions
- Flexible Spend Plan
- eProcurement
- eCommerce Guides
Literature & Videos
- Application Notes
- Technical Overviews
- User Manuals
- Life Sciences Publication Database
- Electronic Instructions for Use (eIFU)
- Safety Data Sheets
- Technical Data Sheets
- Site Prep Checklist
E-Newsletters
- Solution Insights
- ICP-MS Journal
Certificates
- Certificate of Analysis
- Certificate of Conformance
- Certificate of Performance
- ISO Certificates
More Resources
- iOS & Android Apps
- QuikChange Primer Design Tools
- GC Calculators & Method Translation Software
- BioCalculators / Nucleic Acid Calculators
- Order Center
- Quick Order
- Request a Quote
- My Favorites
- Where to Buy
- Flex Spend Portal
- Genomics Applications and Solutions
- Sample Quality Control Solutions
- Sample Quality Control Case Studies

2-way Texting
A better way to communicate with vehicle owners by text

Digital Vehicle Inspection
A better way to DVI with a best in class digital vehicle inspection
Streamline and standardize your process to automate your profits
Track technician efficiency in your bays with our digital work order.

Quality Control
Avoid costly mistakes with a digital quality control process
The easiest to boost your authentic online review score
CRM Marketing Module
Automate your customer follow-up and vehicle service reminders
Rewards & Referrals
Easy-to-use rewards program with a built-in innovative referral tool
Remote Payments
Text invoices to customers allowing them to pay from anywhere

Online scheduling for your website with calendar integration
Quality Control Case Study
Golden Rule Auto Care

- Is there grease on the hood, door, steering wheel, console, etc.?
- Are check engine or warning lights on?
- If an oil change was performed, are lights reset and sticker placed in windshield?
- Are tools left in the vehicle?
- Is the work completed and the vehicle fully reassembled?
- Are all caps put back on?
- Are all belts and hoses tightened?
- Are all fluids filled up?
- Are the tires properly inflated?
Chris found that 80% of the vehicles he inspected had one or more of these issues .. He presented the data to his team, and it was decided something had to change; a quality control process was to be implemented.

Quality control is a process followed by nearly all Fortune 500 companies to ensure that top quality work and performance are met on every single product and service. With his software engineering background, Chris applied the practice of quality control from the software industry to the auto repair industry. Software is not released until a separate team or person reviews and tests it for bugs.
Click here for a free quality control checklist 1
Click here for a free quality control checklist 2
Following is the plan that was implemented at Golden Rule Auto Care:
- Every vehicle was to be inspected and wiped down by someone at the front counter.
- If a vehicle had driveability work performed, a counter person would perform another test drive and complete the quality control inspection.
- The results were to be captured in a monthly report for employees to see the effectiveness of their new quality process.
- Golden Rule Auto Care wanted to celebrate the fact that they were finding the issues vs. the customer but also wanted to make sure the technicians were not using the quality control process as a crutch to not properly complete their jobs.
- The customer would be informed about the process as an added advantage to the shop and invited to observe or even participate in the quality control inspection.
Since initiating the quality control process, Chris has created a quality control checklist in Autoflow .
Note: There was push back from the counter people and technicians.
- Counter people said they didn’t have time but agreed change was needed with an 80% quality issue. It turned out, one average “QC” or quality control inspection took 5 to 10 minutes.
- Technicians felt they were being disrespected by someone reviewing their work. They were assured that mistakes are common and needed to be caught by the shop and not by customers.
- Customer Retention – Customers will keep coming back, knowing that they received top quality service the first time and every time they visit your shop. Loyal customers are worth 10 times their initial visit . It is 6-7 times more expensive to acquire a new customer than it is to keep an existing one.
- Positive Reputation – It takes 12 positive consumer experiences to make up for one bad consumer experience . Consumers are likely to tell 10-15 people about their bad experience.
- Add-on Sales – A service writer/counter person may notice something that was originally not written up, such as a past due oil change sticker that a technician missed, which can lead to add-on sales.
- Quality control time without drive time: 5 to 10 minutes
- Quality control time with drive time: 15 to 20 minutes
- Golden Rule Auto Care’s average: 12 minutes
- KPI or goal for Golden Rule Auto Care’s QC issues per month: 10% or less
- This year, they have run as high as 25% and as low as 9% per month.
- Most common issue found at Golden Rule Auto Care: grease on handle, door panel, seat, console, floor, kick plate, etc.
- Second most common issue found: fluids not full
- Intake boot under upper radiator hose was disconnected and pressed against exhaust manifold…melting & smoking.
- Vehicle started leaking a large amount of coolant due to incorrect installation.
- Positive battery terminal not fully tightened.
- Scanner still plugged in (these can get expensive to give away!)
Following is a snapshot of Golden Rule Auto Care’s monthly report:

Thank you for visiting nature.com. You are using a browser version with limited support for CSS. To obtain the best experience, we recommend you use a more up to date browser (or turn off compatibility mode in Internet Explorer). In the meantime, to ensure continued support, we are displaying the site without styles and JavaScript.
- View all journals
- My Account Login
- Explore content
- About the journal
- Publish with us
- Sign up for alerts
- Open access
- Published: 18 April 2024
Memory type Bayesian adaptive max-EWMA control chart for weibull processes
- Abdullah A. Zaagan 1 ,
- Imad Khan 2 ,
- Amel Ayari-Akkari 3 ,
- Aamir Raza 4 &
- Bakhtiyar Ahmad 5
Scientific Reports volume 14 , Article number: 8923 ( 2024 ) Cite this article
Metrics details
- Engineering
- Mathematics and computing
The simultaneous monitoring of both the process mean and dispersion has gained considerable attention in statistical process control, especially when the process follows the normal distribution. This paper introduces a novel Bayesian adaptive maximum exponentially weighted moving average (Max-EWMA) control chart, designed to jointly monitor the mean and dispersion of a non-normal process. This is achieved through the utilization of the inverse response function, particularly suitable for processes conforming to a Weibull distribution. To assess the effectiveness of the proposed control chart, we employed the average run length (ARL) and the standard deviation of run length (SDRL). Subsequently, we compared the performance of our proposed control chart with that of an existing Max-EWMA control chart. Our findings suggest that the proposed control chart demonstrates a higher level of sensitivity in detecting out-of-control signals. Finally, to illustrate the effectiveness of our Bayesian Max-EWMA control chart under various Loss Functions (LFs) for a Weibull process, we present a practical case study focusing on the hard-bake process in the semiconductor manufacturing industry. This case study highlights the adaptability of the chart to different scenarios. Our results provide compelling evidence of the exceptional performance of the suggested control chart in rapidly detecting out-of-control signals during the hard-bake process, thereby significantly contributing to the improvement of process monitoring and quality control.
Introduction
Statistical process control (SPC) is an essential quality management approach ensuring efficient operations and consistent high-quality products and services. It employs statistical methods such as control charts and process capability analysis to detect and rectify production variations, fostering data-driven decision-making, process stability, and waste reduction. SPC implementation promotes proactive quality management, resulting in enhanced customer satisfaction, cost reduction, and improved operational efficiency. SPC serves as a systematic approach for managing and enhancing the quality of production processes. It primarily utilizes control charts (CCs), graphically depicting process performance by tracking data points over time. SPC aids in recognizing patterns and fluctuations, distinguishing between natural variability (common causes) and irregularities caused by specific events (special causes). By enabling early identification of process deviations, CCs empower organizations to implement timely corrective measures, ensuring consistent adherence to predefined quality standards. As a fundamental quality management tool, SPC fosters continuous improvement and operational efficiency across various industries. In the genesis of control charts (CCs), Shewhart 1 laid the foundation, employing current sample data to identify significant changes in production processes. In contrast, memory-type CCs, exemplified by cumulative sum (CUSUM) and exponentially weighted moving average (EWMA) CCs, introduced by Page 2 and Roberts 3 , respectively, incorporate both current and historical sample data. Notably, CUSUM and EWMA CCs exhibit heightened sensitivity in detecting subtle to moderate shifts in process parameters, surpassing the capabilities of traditional Shewhart CCs. These memory-type CCs, especially CUSUM and EWMA, find extensive application across diverse domains, prominently in chemical and industrial production processes. You et al. 4 evaluate the performance of the EWMA median chart using EARL as a metric and show consistent performance with both EARL and ARL metrics when the deterministic shift size matches the process layer size range. Chatterjeea et al. 5 introduces the TEWMA-Max CC, demonstrating its higher sensitivity compared to Max-EWMA and Max-DEWMA in detecting shifts in mean and variability, with a satisfactory overall performance for various shift combinations. Jalilibal et al. 6 examines the need for continuous process monitoring emphasizing the importance of detecting changes in dispersion and location parameters of process. Yung 7 highlights green manufacturing policies to minimize waste, emphasizing optimal product quality through the Qpm MQCAC for joint monitoring of mean µ and standard deviation σ, demonstrating its efficacy in quality improvement and aligning with environmental goals. Arif et al. 8 proposed a new control chart integrating EWMA with the generalized likelihood ratio test statistic for joint monitoring of process mean and dispersion under double ranked set sampling. Noor-ul-Amin et al. 9 introduced a Max-EWMA control chart for joint monitoring of mean and dispersion under the Weibull distribution, showcasing improved sensitivity compared to the existing Max-EWMA control chart. Evaluation is based on ARL and SDRL, with practical application demonstrated through two examples. Lee and Lin 10 integrate adaptive techniques with Max charts for enhanced process control, utilizing a Markov chain approach to develop statistical indicators and design models. Comparing the adaptive Max charts with EWMA, CUSUM, and double-sampling charts, it demonstrates their superior ability to detect small shifts in mean and variance. Nazir et al. 11 studied four robust adaptive EWMA schemes for monitoring process location parameters, examining their performance under normal and contaminated environments through comparisons of ARL, SDRL, and run length distribution percentiles, with practical examples included for implementation. Sarwar and Noor-ul-Amin 12 propose an adaptive EWMA chart for monitoring the mean of a normal process, showcasing its efficacy in detecting shifts through comprehensive Monte Carlo simulations. Noor-ul-Amin et al. 9 introduces a CC for jointly identifying the variations in the process mean and dispersion, with the help of inverse response function for Weibull distribution. Previous studies indicate a prevalent dependence on traditional methods centered solely on sample data, often overlooking prior information. Conversely, the Bayesian methodology incorporates both sample data and prior knowledge to revise and construct a posterior distribution (P), thereby improving the estimation process. Menzefricke 13 presents combined EWMA charts for mean and variance using Bayesian approach. Simulations compare charts for different specifications, including weighing constant and calibration sample size, and evaluate the variance-focused EWMA chart's performance. Aunali and Venkatesan 14 suggested a Bayesian estimator for quick detection of small shifts in a CC assuming a normal process. It compares this estimator to classical Moving Average, CUSUM, and EWMA control charts in detecting small shifts in the process mean, utilizing a simulation study based on individual observations. Alia 15 Bayesian predictive monitoring of time-between-events using CUSUM and EWMA CCs with predictive control limits, demonstrating feasibility for online process monitoring and comparison with frequentist sequential charts. Asalam and Anwar 16 introduce a Bayesian Modified-EWMA chart, indicating higher performance in monitoring slight to large changes and surpassing current alternatives. Bourazasa et al. 17 propose a versatile Bayesian method for online monitoring using power priors for outlier detection and accommodating diverse data distributions, showcasing superiority over frequentist approaches in an extended simulation study, with practical applications in short production runs and online Phase I monitoring. Khan et al. 18 proposed a Bayesian HEWMA control chart utilizing RSS schemes, demonstrating improved sensitivity for detecting out-of-control signals compared to existing Bayesian control charts, as evidenced by extensive Monte Carlo simulations and a numerical example from semiconductor manufacturing. Noor-ul-Amin et al. 19 introduce a novel Bayesian Max-EWMA control chart for simultaneous tracking of non-normal process mean and dispersion, demonstrating superior sensitivity and adaptability under different LFs for Weibull processes, particularly in semiconductor manufacturing. Iqbal et al. 20 introduce a novel Bayesian Max-EWMA control chart for concurrent monitoring of mean and variance under various loss functions, demonstrating superior sensitivity and performance compared to existing methods. This is validated through Monte Carlo simulations and a practical case study in semiconductor manufacturing. Differing from prior studies exploring classical and Bayesian methods, as well as SRS and RSS schemes, for monitoring mean, variance, and their joint behavior, this article focuses uniquely on the use of Bayesian approaches for monitoring both mean and variance by adapting the value of smoothing constant that helps to adjust the value of smoothing constant according to the estimated shift size. This adaptation ensures that the monitoring process remains effective across a wide range of shift sizes, a capability not addressed in prior studies. The performance evaluation of this Bayesian Max-EWMA CC is carried out through the use of Monte Carlo simulations. The article is structured with specific segments, including a part dedicated to Bayesian theory and various LFs in Section " Bayesian approach ", the proposed Bayesian adaptive Max-EWMA CC method in Section " Suggested Bayesian adaptive max-EWMA CC for Weibull process ", Section " Simulation study " contains simulation study and extensive discussions and significant findings in Section " Results discussion and main findings ", practical applications using real-life data in Section " Real Life application ", and concluding remarks in Section " Conclusion ".
- Bayesian approach
The Bayesian methodology in statistics sights probability as an indication of the plausibility or confidence in an event. It employs Bayes' theorem to revise the probability associated with a hypothesis in response to additional evidence. In contrast to frequentist statistics, which regard probability as a frequency-based limit, the Bayesian approach integrates prior knowledge or assumptions about relevant parameters during analysis. By merging prior information with fresh data, it facilitates the determination of unknown parameters and the measurement of uncertainty in a more adaptable and intuitive manner. This approach is widely applicable across a range of fields, including machine learning, decision-making, and experimental design. Let V be the random variable representing lifetimes following a Weibull distribution with both the shape parameter (λ) and scale parameter (α) being greater than zero (α > 0, λ > 0). In this scenario, the mathematical expressions for the probability density function (pdf) and cumulative distribution function (cdf) are as follows:
Squared error loss function
The SELF is a critical tool in the Bayesian framework, used to gauge the gap between predicted and actual parameter values. It computes the average squared difference between estimated and true parameter values, assessing the model's suitability. In Bayesian decision theory, it aids in choosing an optimal estimator that minimizes the expected value of the squared error loss. Additionally, this function plays a key role in Bayesian inference, helping to derive posterior distributions and evaluate model accuracy. The SELF, originally presented by Gauss 21 , consider the interest as X and estimator \(\hat{\theta }\) employed for the estimation of population parameter, referred to as θ. Its expression for SELF is mathematized as:
Applying SELF, Bayes estimator is mathematized as
Linex loss function
In Bayesian methodology, the linex loss function (LLF) is instrumental in gauging the difference between the estimated and true values of a parameter. It measures the absolute difference between these values, allowing for an evaluation of the model's accuracy and reliability. This function aids in the selection of an appropriate estimator to minimize the expected value of the LLF within Bayesian decision theory. Furthermore, it contributes to Bayesian inference by helping derive posterior distributions and improving the assessment of model performance and precision. Varian 22 introduced the LLF to minimize risks in Bayesian estimation. The mathematical representation of the LLF is as follows:
Bayesian estimator \(\hat{\theta }\) under LLF is mathematizied as
Suggested Bayesian adaptive max-EWMA CC for Weibull process
In the context of statistical analysis, let's examine a sequence of random samples represented as V i1 , V i2 , …, V in , derived from a Weibull distribution represented at various time instances, with i representing distinct sequences ( \(i = \, 1, \, 2, \, 3\) , and so forth). It is common for Weibull process that the parameters ( \(\alpha\) and \(\lambda\) ) to be initially unknown, prompting the need for estimation using historical data. The process of parameter estimation typically assumes the process is under control. Let \(\alpha_{0}\) and \(\lambda_{0}\) represent the estimated scale and shape parameters, respectively. The estimation of these parameters involves establishing a connection between standard normal and Weibull distribution, as elucidated Faraz et al. 23 in Eq. ( 7 ), expressed as:
The properties of the above function is given as
Useful information about the effects of changing the parameters of a Weibull distribution on the mean and variance of a random variable with a standard normal distribution can be obtained from Eqs. ( 8 ) and ( 9 ). Essentially, these formulas express the precise influence that changes in the Weibull distribution parameters have on the features and attributes of the normal distribution.
Suppose we have a series of random samples, denoted as \(z_{i1} = W\left( {V_{i1} ,\alpha_{0} ,\lambda_{0} } \right)\) , \(z_{i2} = W_{N} \left( {V_{i2} ,\alpha_{0} ,\lambda_{0} } \right)\) , …, \(z_{in} = W_{N} \left( {V_{in} ,\alpha_{0} ,\lambda_{0} } \right)\) , originating from a Weibull distribution and transformed to a normal distribution. In this context, the Max-EWMA Control Chart, integrating the principles of Bayesian theory, allows for the concurrent monitoring of both the mean and variance of the process that follows the normal distribution. This approach facilitates effective and simultaneous monitoring of the underlying process parameters, ensuring improved control and management of the system's performance.
In a Bayesian framework, assuming normal distributions for both the likelihood function and prior distribution, the resulting posterior distribution also adopts a normal distribution form. This distribution is characterized by its mean (θ) and variance (σ) as defining parameters. The probability density function (pdf) of the posterior distribution is articulated as:
where \(\theta_{n} = \frac{{n\overline{z} \delta_{0}^{2} + \delta^{2} \theta_{0} }}{{\delta^{2} + n\delta_{0}^{2} }}\) and \(\delta_{n}^{2} = \frac{{\delta^{2} \delta_{0}^{2} }}{{\delta^{2} + n\delta_{0}^{2} }}\) respectively.
Selecting a sample of “ n ” values that represent a specific quality attribute “ Z ” from the production process is the first step in creating a Max-EWMA chart using Bayesian methodology. Then, using the SELF method, we calculate the transformed statistics for the mean and variance as shown below:
where \(\hat{\theta }_{(SELF)} = \frac{{n\overline{x} \delta_{0}^{2} + \delta^{2} \theta_{0} }}{{\delta^{2} + n\delta_{0}^{2} }}\) and \(\hat{\delta }_{(SELF)}^{2} = \frac{{\delta^{2} \delta_{0}^{2} }}{{\delta^{2} + n\delta_{0}^{2} }}\) are the Bayes estimators applying SELF, while using LLF, the Bayes estimators are given as \(\hat{\theta }_{{\left( {_{LLF} } \right)}} = \frac{{n\overline{z} \delta_{0}^{2} + \delta^{2} \theta_{0} }}{{\delta^{2} + n\delta_{0}^{2} }} - \frac{{C{\prime} }}{2}\delta_{n}^{2}\) and \(\hat{\delta }_{{\left( {LLF} \right)}}^{2} = \frac{{\delta^{2} \delta_{0}^{2} }}{{\delta^{2} + n\delta_{0}^{2} }}\) , The mathematical description of the transformed statistic for both the process mean and variance under LLF is as follows:
In this context, \(H\left( {n,\nu } \right)\) represents a chi-square distribution with \(\nu\) degrees of freedom, and \(\phi^{ - 1}\) signifies the inverse function. The following is the breakdown of the calculations for the EWMA statistics related to the process mean and dispersion.
In this scenario, for the EWMA statistic Pt and Qt, the initially values are denoted by \(P_{0}\) and \(Q_{0}\) respectively. Furthermore, both Pt and Qt are independent and normally distributed with a mean of zero and variances of σ 2 respectively. This is expressed as follows:
Integration of adaptive approach
Subsequently, we introduce the adaptive technique. Consider \({X}_{t}\) a normally distributed random variable at time t with a sample size of n , characterized by a mean \({\upmu }_{{\text{X}}}\) and a variance \({\upsigma }_{{\text{X}}}^{2}.\mathrm{ i}.{\text{e}}.,{{\text{X}}}_{{\text{t}}}\sim {\text{N}}\left({\upmu }_{{\text{X}}}, {\upsigma }_{{\text{X}}}^{2}\right)\) . Jiang et al. 24 introduced an estimator for estimating the magnitude of the shift using the following expression:
The symbol ψ represents the smoothing constant, typically constrained to the range (0,1]. In real-world applications, having prior knowledge about the precise magnitude of a shift is uncommon. Consequently, we initially estimate its value. Haq et al. 25 suggested an unbiased estimator, denoted as \(\hat{\delta }_{t}^{{**}}\) , which can be expressed as:
where \({\text{E}}\left( {\hat{\delta }_{t}^{{**}} } \right) = {\updelta } = 0\) . The process remains stable for a certain period of time, denoted as \({\text{t}}\le {{\text{t}}}_{0}\) . In practical scenarios, the true magnitude is frequently unknown. Instead, δ is estimated by considering \(\tilde{\delta }_{t}^{{**}} = \left| {\hat{\delta }_{t}^{{**}} } \right|\) .
The detection is accomplished through the recursive calculation of the subsequent EWMA statistic, known as the suggested chart statistic, as follows:
Setting the initializing value as \({{\text{A}}}_{0}\) = 0, we avoid using a fixed value for the smoothing constant. Instead, we adopt a function that can adjust to different values depending on the changing conditions of the process. To achieve this, we propose the following function for the self-adjusting weighting factor η( \({\tilde{\upomega }}_{{\text{t}}}^{*}\) ).
The function is segmented into three parts based on the estimated shift. In the initial part, the function is constructed when the estimated shift is less than 1, resulting in a small smoothing constant to accommodate minor shifts. In the second segment, the smoothing constant takes on a larger value compared to the first part, aligning with moderate estimated shifts. For shifts categorized as large, the third part of the function assigns a smoothing constant equal to 1. Here, η( \({\tilde{\omega }}_{t}^{*}\) ) represents a random variable shaped by a continuous function, optimizing the performance of our chart for shift detection. When \({\tilde{\delta }}_{t}\) ≤ 2.7, η() is specifically designed to be sensitive to small to moderate shifts. Our adaptive chart outperforms other methods by excelling in detecting shifts of any size. If the shift surpasses \({\tilde{\delta }}_{t}\) ≥ 2.7, our chart functions similarly to a Shewhart chart, effectively identifying larger shifts with \({\tilde{\delta }}_{t}\) = 2.7 serving as the pivotal point. It provides flexibility in model adjustments and the inclusion of additional factors. Instead of using a fixed value in Eqs. ( 10 ) and ( 11 ), assuming its constancy throughout the process, we utilize the self-adjusting function from Eq. ( 16 ) and iteratively update Eqs. ( 10 ) and ( 11 ) to calculate the individual EWMA under the adaptive approach. Thus, the transformed equations are mathematically described as:
Finally, these values are inserted into the Max-EWMA provided by Chen and Change 26 to jointly monitor the process mean and variance in a single chart. Thus, the plotting can be expressed as:
where Max is function to get the maximum value of the given inputs.
The suggested statistic being a positive value simplifies the monitoring process. In this context, we only need to plot the UCL for the joint monitoring of the process mean and variance. The plotting statistic is then compared with the UCL threshold. If the value is above the UCL, the process is considered out of control, indicating deviations in the mean, variance, or both. On the other hand, if the value is below the UCL, the process is considered to be under control.
Simulation study
The study employs the Monte Carlo simulation method to intricately examine the effectiveness of the Bayesian adaptive Max-EWMA CC in identifying shifts in process parameters through the computation of ARLs and SDRLs, with a particular focus on the in-control process ARL set at 370. This approach is pivotal for understanding the impact of varying the smoothing constant, such as \(\gamma\) = 0.10 and 0.25, the study aims to shed light on how these adjustments can enhance the CCs responsiveness to detecting quality shifts in manufacturing processes. The Monte Carlo simulation method's comprehensive steps are crafted to provide a deep dive into the performance of the Bayesian adaptive Max-EWMA CC, evaluating its potential in improving process monitoring and control. This rigorous analysis not only highlights the adaptability and efficiency of the proposed control chart in signaling out-of-control conditions but also contributes valuable insights into optimizing process quality management strategies, thereby offering a significant advancement in the field of SPC. The following are main steps.
Step 1. setting up control limits
We take Weibull distribution as a sampling distribution and transform to standard normal distribution. The prior distribution is also a standard normal for calculating the mean and variance of posterior distribution under different LF.
Chose the initial value of h with a specific value of \(\gamma\) at fixed \({ARL}_{0}\) = 370.
Random samples of size n is generated for the in-control process from the normal distribution such that \(X\sim N\left( {E(\hat{\theta }),E\left( {\hat{\delta }} \right)} \right)\) .
Compute the plotting statistic of the offered Bayesian adaptive Max-EWMA CC and evaluate the process according to the suggested design.
If the process declared in-control, then repeat steps (iii-iv) until the process is showed to be out-of-control. When the process is declared as out-of-control then record the in-control number as run length.
Repeat the steps (iii-v) 50,000 times and calculate the in-control ARL.
If \({ARL}_{0}=370\) , then go to Step 2 with the same value of \(\gamma\) , and h . Otherwise, repeat steps (ii-vi) with the change value of L .
Step 2. Evaluate the out-of-control ARL
Select random sample for the shifted process from the normal distribution such that \(X\sim N\left( {E(\hat{\theta }) + \sigma \frac{{E\left( {\hat{\delta }} \right)}}{\sqrt n },E\left( {\hat{\delta }} \right)} \right),\) where \(\sigma\) is the amount of shift in the process parameter.
Calculate plotting statistic and appraise the process according to the design of the recommended CC.
If the plotted statistic lies within the UCL, repeat steps (i–ii). Otherwise, record the number of generated points, indicating a single out-of-control run length.
Repeat the aforementioned process (steps i–iii) 50,000 times to determine the ARL 1 and SDRL 1 for out-of-control scenarios.
Results discussion and main findings
In this examination, Tables 1 , 2 , 3 and 4 play a pivotal role in presenting the extensive findings resulting from the implementation suggested CC in the context of the Weibull process. The study conducts a detailed exploration of the implications of two different LFs, highlighting the significance of the posterior distribution. In the context of informative priors, these assessments are conducted by incorporating pre-existing knowledge and beliefs into the analytical procedures. This integration aims to augment the overall understanding of the results, recognizing and leveraging prior information to inform the Bayesian analysis. The use of 50,000 replicates ensures robust statistical findings, enhancing the reliability of the ARL and SDRL calculations. Careful selection of smoothing constants demonstrates a commitment to precision, refining the analysis and enabling a thorough evaluation of the suggested Bayesian CC performance in diverse scenarios. Additionally, the study comprehensively explores a wide range of combinations, spanning from 0.00 to 3.00 of shift values (a) and from 0.25 to 3.00 for variance shift values (b). In this comprehensive study, we thoroughly examine the efficacy of the offered CC designed for jointly identifying both the process mean and dispersion. The study's findings unequivocally highlight the exceptional sensitivity of the method in detecting deviations from established norms within production processes. This underscores the significant potential of the proposed CC as a reliable tool for continuous monitoring and quality control across various industrial sectors. It is crucial to continuously confirm during the analysis that the plotting statistic was computed stays less than the UCLi. The outcome of each trial is determined by the plotting statistic exceeding the UCLi, which indicates a substantial change in the process mean and standard deviation. These observed changes are associated with variations in the parameters of the Weibull distribution, indicating potential deviations from the expected norms in the production process. In our examination, we specifically investigate shifts in shape parameters within the range of 0.25 to 4.00 and shifts in scale parameters within the range of 0.0 to 5.00. For W(1, 1.5), the process initially adheres to control limits, following a N(0,1) normal distribution. The ARL under in-control conditions was determined as 370 for two distinct values of λ. The findings presented in Tables 1 and 2 robustly affirm the performance of offered CC, particularly when employed in conjunction with SELF applying posterior distribution. This combined approach showcases an impressive capability to detect shifts in both process mean and variance concurrently, ensuring process stability and product quality. Notably, a trend that consistently shows up in the data indicates that ARLs decrease as mean shift magnitude increases. ARLs exhibit a decline in response to variance shifts as well. These consistent patterns strongly emphasize the recommended CC's ability to promptly recognize and indicate process shifts, enabling timely intervention and process control. This characteristic positions it as an invaluable instrument for comprehensive production process monitoring, ensuring the swift identification and resolution of deviations from established norms. The ultimate goal of implementing these control charts is to enhance product quality and improve process efficiency, rendering them valuable assets across diverse industries. For instance, when scrutinizing the ARL results for the suggested Bayesian Max-EWMA CC, emphasis is placed on the application of the SELF. In this context, a smoothing parameter of λ = 0.10 and a sample size of n = 5 are employed. In particular, our exploration involves examining different Weibull distribution shape parameter shift values, spanning from 1.50 to 5.00, while keeping the scale parameter shift constant at 1.0. The corresponding run length outcomes for mentioned shifts are enumerated as follows: 369.15, 80.19, 24.05, 13.71, 10.14, 8.16, 7.12, 4.88, and 4.13. Notably, as shift magnitude increases, a distinct reduction in the corresponding ARL values is observed. This finding underscores the heightened efficiency of the proposed Bayesian Max-EWMA CC in promptly identifying shifts in the shape parameter. Practically, this increased sensitivity implies that the control chart can swiftly identifying minor variations in the process mean, facilitating a rapid response—a critical aspect in upholding process quality and consistency. The analysis extends to evaluating the influence of varying scale parameter values, ranging from 1.0 to 4.00, while keeping the shape parameter constant at a = 1.50. The resultant run length are: 369.51, 24.90, 16.82, 7.16, 5.08, 3.60, 2.60, 2.11, and 1.50. Notably, these results exhibit a distinct trend—as the shape parameter diverges from its baseline value of 1, there is a noticeable decrease in ARL values. This pattern demonstrates how the recommended Control Chart can effectively identify changes in process dispersion quickly. It is crucial to underscore a key observation from Table 2 . In assessing the performance of the recommended CC, it becomes apparent that the effectiveness of the CC diminishes with higher values of the smoothing constant. According to this realization, there are some situations where choosing a smaller smoothing constant might be more beneficial for getting the best results. ARL results for the proposed CC using the LLF with a consistent λ = 0.25 and n = 5 are similarly presented in Tables 3 and 4 . 370.09, 20.34, 6.34, 2.81, 1.99, 1.57, and 1.17 were The ARL values that resulted from several trials that involved shifting the shape parameter from 1.50 to 5.00 and corresponding shifts in the scale parameter kept at 1.0. The key findings indicate a noticeable pattern: as process shifts become more significant, ARL values show a steep decrease, underlining the remarkable accuracy of the provided control chart in quickly detecting deviations in both process mean and dispersion. It is also important to recognize that the effectiveness of the suggested CC in concurrently identifying process mean and dispersion is impacted by the sample size. The results consistently show that as the sample size grows, ARL values decrease, demonstrating the improved precision of the recommended control chart in promptly identifying deviations from the anticipated process parameters:
The analysis of the ARL and SDRL across Tables 1 , 2 , 3 and 4 associated with proposed CC using Weibull process highlights its effectiveness in identifying both process mean and variance, particularly in the detection of minor to moderate shifts. These profiles demonstrate the CC's performance across various scenarios, with a consistent trend indicating its proficiency in swiftly identifying variations in process mean and dispersion, thus establishing its significance and consistency.
Simulation outcomes clearly show reducing the smoothing constant enhances effectiveness of the recommended Bayesian CC for simultaneous process monitoring. Essentially, decreasing the \(\lambda\) makes CC more responsive and adept at swiftly identifying changes in both the process mean and variance. This insight suggests that, in particular situations or applications, opting for a lower \(\lambda\) can result in more streamlined monitoring, leading to faster identification of deviations from expected process parameters and ultimately improving the quality and reliability of the process.
In our investigation, a critical aspect that we have thoroughly examined is the variation in sample size. Our analysis reveals a crucial and compelling insight. Clearly, the efficacy and performance of the proposed CC improve noticeably and significantly with increasing sample size. In practical terms, this suggests that when utilizing larger sample sizes, the CC becomes more skilled at accurately and swiftly detecting changes in both the process mean and variance. This development holds particular significance as it has the potential to improve process monitoring's dependability and resilience, thereby leading to an overall improvement in process consistency and quality.
Real Life application
The article provides a practical illustration of the proposed CC by applying it to data obtained from Montgomery's research 27 , which focuses on the hard-bake process in semiconductor manufacturing. The dataset used in this application comprises 45 samples, with each sample containing 5 wafers, resulting in a total of 225 data points measured in microns. The measurements were taken at regular one-hour intervals during the data collection. Within the dataset, the initial 30 samples, totaling 150 observations, represent a well-controlled process referred to as the phase I dataset. This phase serves as a baseline for the controlled state of the manufacturing process. On the other hand, the subsequent 15 samples, comprising 75 observations, depict an out-of-control process and are identified as the phase II dataset. This phase is characterized by variations or deviations from the expected or controlled behavior in the semiconductor manufacturing process. The differentiation between phase I and phase II datasets allows for the assessment of the proposed CC's effectiveness in identifying and responding to variations, providing insights into its practical utility in monitoring and maintaining the quality of the manufacturing process (Figs. 1 , 2 ).
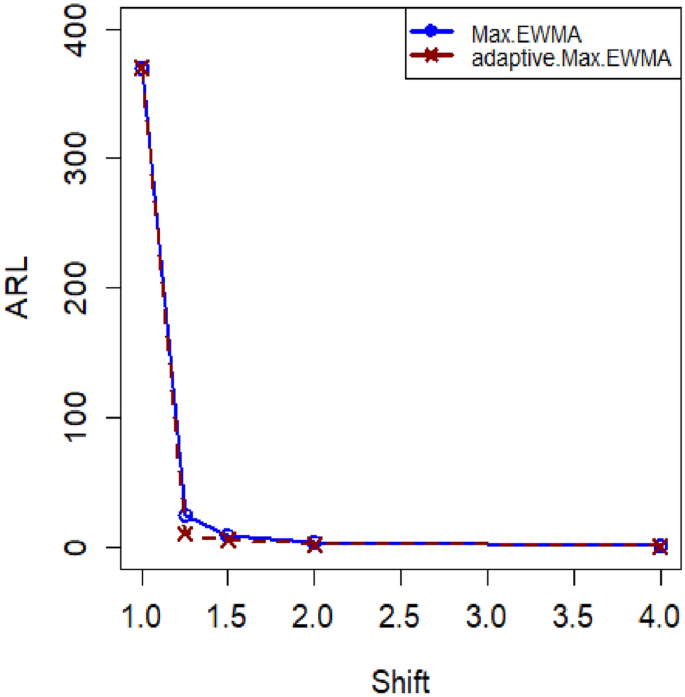
ARL plot for the offered CC under SELF.
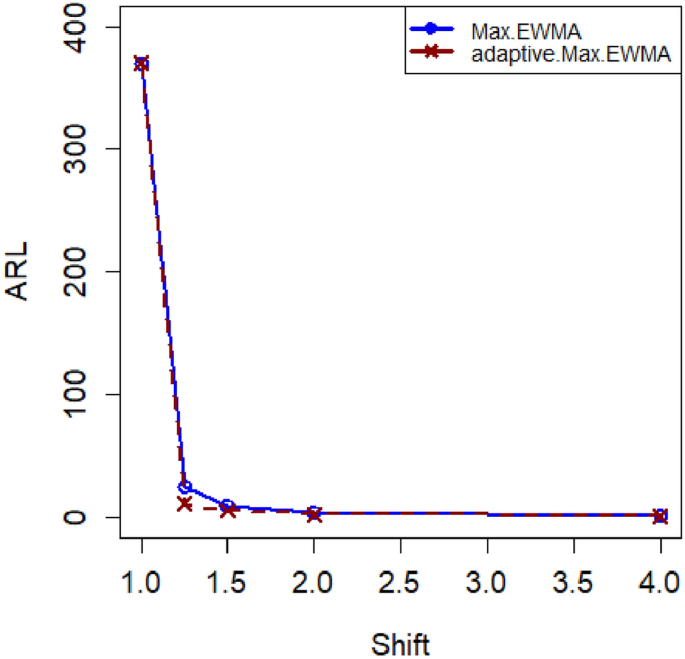
ARL plot for suggested CC utilizing LLF.
Figures 3 and 4 visually demonstrate the application of the existing Bayesian Max-EWMA CC, which is specifically designed to monitor both process mean and dispersion simultaneously, utilizing the SELF and LLF methods. A detailed examination of these charts clearly indicates instances where the process has deviated from the expected control state, notably in the 38th and 39th samples, particularly when considering a smoothing constant of 0.25, while Figs. 5 and 6 identifying the out-of-control signals for both the process mean and dispersion on the 35th and 36th samples for the proposed CC. These changes in process behavior are closely related to changes in process mean and dispersion of the Weibull distribution. In reliability and survival analysis, the Weibull distribution is a statistical model often used to describe the distribution of data. Changes in the shape and scale parameters directly influence the distribution's characteristics, resulting in the observed discrepancies in the control charts. In essence, the identified deviations in the 36th and 35th samples can be traced back to shifts in the fundamental parameters of the underlying statistical distribution, impacting the overall behavior of the manufacturing process and triggering the out-of-control conditions reflected in the CCs.
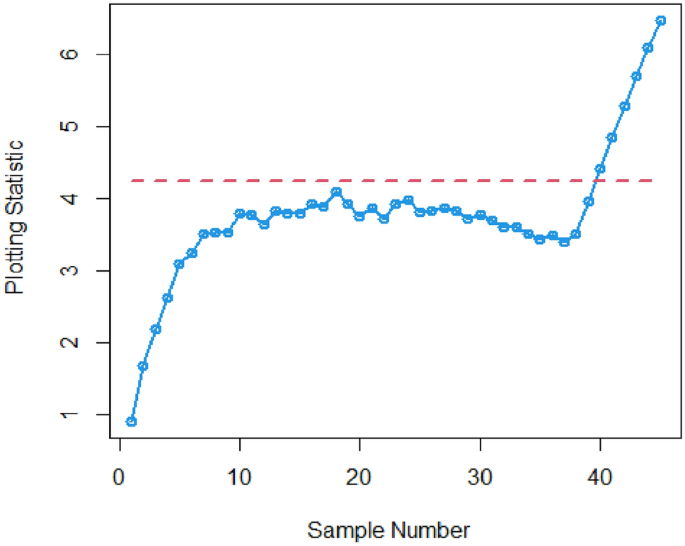
Existing Max-EWMA CC under Weibull process for jointly monitoring with \(\lambda = 0.25\) .
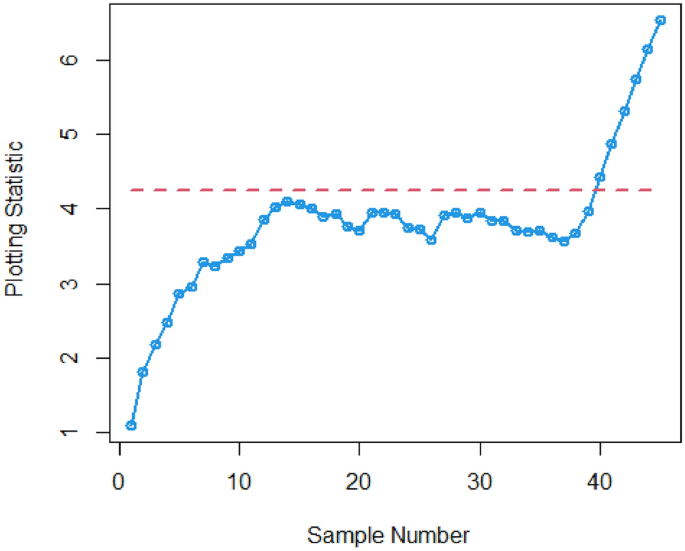
Utilizing LLF, Max-EWMA CC applying Weibull process for jointly monitoring at \(\lambda = 0.25\) .
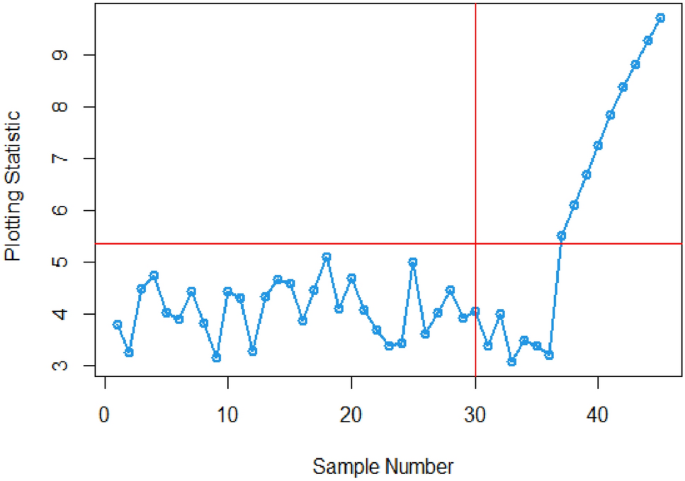
plot for the suggested CC Using SELF, for jointly monitoring with \(\lambda = 0.25\) .
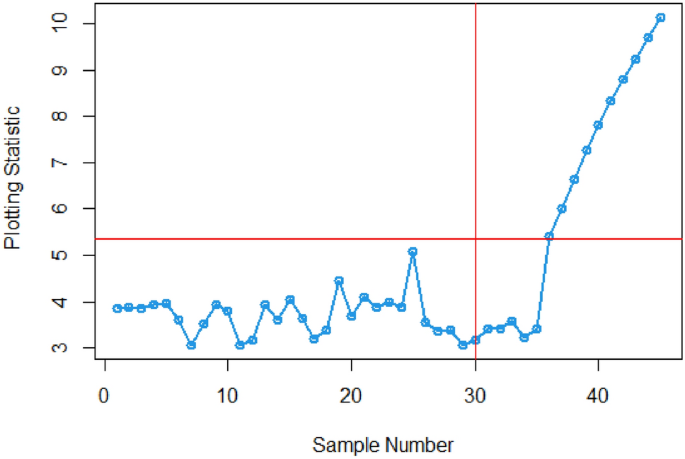
Plot using LLF, for recommended CC for jointly monitoring with \(\lambda = 0.25\) .
In this research, we present an innovative Bayesian CC specifically designed for monitoring Weibull processes, enabling simultaneous tracking of process mean and variance. This CC includes informative prior distributions and integrates two different LFs within the framework of the posterior distribution. To thoroughly assess the performance of this novel approach, we conducted an extensive analysis, presenting detailed results in Tables 1 , 2 , 3 and 4 . ARL and SDRL were used as evaluation metrics These evaluations utilized key metrics such as ARL and SDRL and ARL plots i.e., Figs. 1 and 2 also show efficient performance of the suggested CC. A useful case study was also carried out, with an emphasis on the hard bake procedure used in the production of semiconductors. Importantly, when applied to posterior distributions, the proposed CC demonstrated significant proficiency in detecting out-of-control signals within the process. The insights gained from this study hold promise for extending these principles to the development of other memory-type Control Charts, enhancing their effectiveness across diverse industrial applications. Expanding the application of this innovative technique beyond non-normal distributions to various types of Control Charts contributes to a more comprehensive understanding of underlying data patterns. This increased effort reduces the likelihood of costly errors and defects by facilitating early detection of potential quality issues in multiple areas and enabling timely remedial action. This method is essential to quickly detect irregularities in patient data, enable rapid interventions, and improve patient care outcomes in real-world settings, particularly healthcare settings. Applying this methodology to non-normal distributions and various types of control charts in the manufacturing industry helps detect process variations, which in turn leads to improved product quality and waste reduction.
Data availability
If there is a reasonable request, interested individuals can directly obtain the datasets that were utilized and/or analyzed in the present study from the corresponding author.
Shewhart, W. A. The application of statistics as an aid in maintaining quality of a manufactured product. J. Am. Stat. Assoc. 20 (152), 546–548 (1925).
Article Google Scholar
Page, E. S. Continuous inspection schemes. Biometrika 41 (1/2), 100–115 (1954).
Article MathSciNet Google Scholar
Roberts, S. W. Control chart tests based on geometric moving averages. Technometrics 42 (1), 97–101 (2000).
You, H., Shahrin, M., & Mustafa, Z. (2021). Design of EWMA-type control chart. Paper presented at the IOP Conference Series: Materials Science and Engineering.
Chatterjee, K., Koukouvinos, C. & Lappa, A. A joint monitoring of the process mean and variance with a TEWMA-Max control chart. Commun. Stat.-Theory Methods 52 (22), 8069–8095 (2023).
Jalilibal, Z., Amiri, A. & Khoo, M. B. A literature review on joint control schemes in statistical process monitoring. Qual. Reliab. Eng. Int. 38 (6), 3270–3289 (2022).
Yang, C.-M. An improved multiple quality characteristic analysis chart for simultaneous monitoring of process mean and variance of steering knuckle pin for green manufacturing. Qual. Eng. 33 (3), 383–394 (2021).
Arif, F., Noor-ul-Amin, M. & Hanif, M. Joint monitoring of mean and variance under double ranked set sampling using likelihood ratio test statistic. Commun. Stat.-theory methods 51 (17), 6032–6048 (2022).
Noor-ul-Amin, M., Aslam, I. & Feroze, N. Joint monitoring of mean and variance using Max-EWMA for Weibull process. Commun. Stat.-Simulat. Comput. 52 (7), 3257–3272 (2023).
Lee, P. H. & Lin, C. S. Adaptive Max charts for monitoring process mean and variability. J. Chin. Inst. Ind. Eng. 29 (3), 193–205 (2012).
Google Scholar
Nazir, H. Z., Hussain, T., Akhtar, N., Abid, M. & Riaz, M. Robust adaptive exponentially weighted moving average control charts with applications of manufacturing processes. Int. J. Adv. Manuf. Technol. 105 , 733–748 (2019).
Sarwar, M. A. & Noor-ul-Amin, M. Design of a new adaptive EWMA control chart. Qual. Reliab. Eng. Int. 38 (7), 3422–3436 (2022).
Menzefricke, U. Combined exponentially weighted moving average charts for the mean and variance based on the predictive distribution. Commun. Stat.-Theory Methods 42 (22), 4003–4016 (2013).
Aunali, A. S. & Venkatesan, D. Comparison of bayesian method and classical charts in detection of small shifts in the control charts. Int. J. 6 (2), 101–114 (2017).
Ali, S. A predictive Bayesian approach to EWMA and CUSUM charts for time-between-events monitoring. J. Stat. Comput. Simul. 90 (16), 3025–3050 (2020).
Aslam, M. & Anwar, S. M. An improved Bayesian Modified-EWMA location chart and its applications in mechanical and sport industry. PLoS ONE 15 (2), e0229422 (2020).
Article CAS PubMed PubMed Central Google Scholar
Bourazas, K., Kiagias, D. & Tsiamyrtzis, P. Predictive control charts (PCC): a Bayesian approach in online monitoring of short runs. J. Quality Technol. 54 (4), 367–391 (2022).
Khan, I. et al. Hybrid EWMA control chart under bayesian approach using ranked set sampling schemes with applications to hard-bake process. Appl. Sci. 13 (5), 2837 (2023).
Article CAS Google Scholar
Noor-ul-Amin, M. et al. Memory type Max-EWMA control chart for the Weibull process under the Bayesian theory. Sci. Rep. 14 (1), 3111 (2024).
Article CAS PubMed Google Scholar
Iqbal, J. et al. A novel Bayesian Max-EWMA control chart for jointly monitoring the process mean and variance: an application to hard bake process. Sci. Rep. 13 (1), 21224 (2023).
Article ADS CAS PubMed PubMed Central Google Scholar
Gauss, C. (1955). Methods Moindres Carres Memoire sur la Combination des Observations, 1810 Translated by J. In: Bertrand.
Varian, H. R. Bayesian approach to real estate assessment. Stud. Bayesian Econ. Stat. Honor Leonard J. Savage 35 (2), 115–135 (1975).
MathSciNet Google Scholar
Faraz, A., Saniga, E. M. & Heuchenne, C. Shewhart control charts for monitoring reliability with Weibull lifetimes. Quality Reliability Eng. Int. 31 (8), 1565–1574 (2015).
Jiang, W., Shu, L. & Apley, D. W. Adaptive CUSUM procedures with EWMA-based shift estimators. Iie Transact. 40 (10), 992–1003 (2008).
Haq, A., Gulzar, R. & Khoo, M. B. An efficient adaptive EWMA control chart for monitoring the process mean. Qual. Reliability Eng. Int. 34 (4), 563–571 (2018).
Chen, G., Cheng, S. W. & Xie, H. Monitoring process mean and variability with one EWMA chart. Journal of Quality Technology 33 (2), 223–233 (2001).
Montgomery, D. C. (2009). Introduction to statistical quality control : John Wiley & Sons.
Download references
Acknowledgements
The authors extend their appreciation to the Deanship of Scientific Research at King Khalid University, Saudi Arabia for funding this work through Large Groups Project under grant number R.G.P2/186/44.
Author information
Authors and affiliations.
Department of Mathematics, Faculty of Science, Jazan University, P.O. Box 2097, 45142, Jazan, Saudi Arabia
Abdullah A. Zaagan
Department of Statistics, Abdul Wali Khan University Mardan, Mardan, Pakistan
Biology Department, College of Sciences in Abha, King Khalid University, P.O. Box 960, Abha, Saudi Arabia
Amel Ayari-Akkari
Government College Women University, Sialkot, Pakistan
Higher Education Department, Kabul, Afghanistan
Bakhtiyar Ahmad
You can also search for this author in PubMed Google Scholar
Contributions
I.K. and A.A.Z. contributed to the manuscript through mathematical analyses and numerical simulations. A.R. and A.A.A. conceptualized the primary idea, analyzed data, and assisted in restructuring the manuscript. B.A. and A.A.Z. rigorously validated the findings, revised the manuscript, and secured funding. Additionally, A.A.Z. and A.A.A. enhanced the manuscript's language and performed additional numerical simulations. The final version of the manuscript, ready for submission, represents a consensus reached by all authors.
Corresponding author
Correspondence to Bakhtiyar Ahmad .
Ethics declarations
Competing interests.
The authors declare no competing interests.
Additional information
Publisher's note.
Springer Nature remains neutral with regard to jurisdictional claims in published maps and institutional affiliations.
Supplementary Information
Supplementary information., rights and permissions.
Open Access This article is licensed under a Creative Commons Attribution 4.0 International License, which permits use, sharing, adaptation, distribution and reproduction in any medium or format, as long as you give appropriate credit to the original author(s) and the source, provide a link to the Creative Commons licence, and indicate if changes were made. The images or other third party material in this article are included in the article's Creative Commons licence, unless indicated otherwise in a credit line to the material. If material is not included in the article's Creative Commons licence and your intended use is not permitted by statutory regulation or exceeds the permitted use, you will need to obtain permission directly from the copyright holder. To view a copy of this licence, visit http://creativecommons.org/licenses/by/4.0/ .
Reprints and permissions
About this article
Cite this article.
A. Zaagan, A., Khan, I., Ayari-Akkari, A. et al. Memory type Bayesian adaptive max-EWMA control chart for weibull processes. Sci Rep 14 , 8923 (2024). https://doi.org/10.1038/s41598-024-59680-6
Download citation
Received : 31 October 2023
Accepted : 13 April 2024
Published : 18 April 2024
DOI : https://doi.org/10.1038/s41598-024-59680-6
Share this article
Anyone you share the following link with will be able to read this content:
Sorry, a shareable link is not currently available for this article.
Provided by the Springer Nature SharedIt content-sharing initiative
- Average run length
- Control chart
- Inverse response function
- The Weibull process
By submitting a comment you agree to abide by our Terms and Community Guidelines . If you find something abusive or that does not comply with our terms or guidelines please flag it as inappropriate.
Quick links
- Explore articles by subject
- Guide to authors
- Editorial policies
Sign up for the Nature Briefing: AI and Robotics newsletter — what matters in AI and robotics research, free to your inbox weekly.

Asking the better questions that unlock new answers to the working world's most complex issues.
Trending topics
AI insights
EY podcasts
EY webcasts
Operations leaders
Technology leaders
Marketing and growth leaders
Cybersecurity and privacy leaders
Risk leaders
EY Center for Board Matters
EY helps clients create long-term value for all stakeholders. Enabled by data and technology, our services and solutions provide trust through assurance and help clients transform, grow and operate.
Artificial Intelligence (AI)
Strategy, transaction and transformation consulting
Technology transformation
Tax function operations
Climate change and sustainability services
EY Ecosystems
Supply chain and operations
EY Partner Ecosystem
Explore Services
We bring together extraordinary people, like you, to build a better working world.
Experienced professionals
MBA and advanced-degree students
Student and entry level programs
Contract workers
EY-Parthenon careers
Discover how EY insights and services are helping to reframe the future of your industry.
Case studies
Energy and resources
How data analytics can strengthen supply chain performance
13-Jul-2023 Ben Williams
How Takeda harnessed the power of the metaverse for positive human impact
26-Jun-2023 Edwina Fitzmaurice
Banking and Capital Markets
How cutting back infused higher quality in transaction monitoring
11-Jul-2023 Ron V. Giammarco
At EY, our purpose is building a better working world. The insights and services we provide help to create long-term value for clients, people and society, and to build trust in the capital markets.
EY is now carbon negative
19-Sep-2022 Carmine Di Sibio
Our commitment to audit quality
13-Nov-2023 Julie A. Boland
No results have been found
Recent Searches
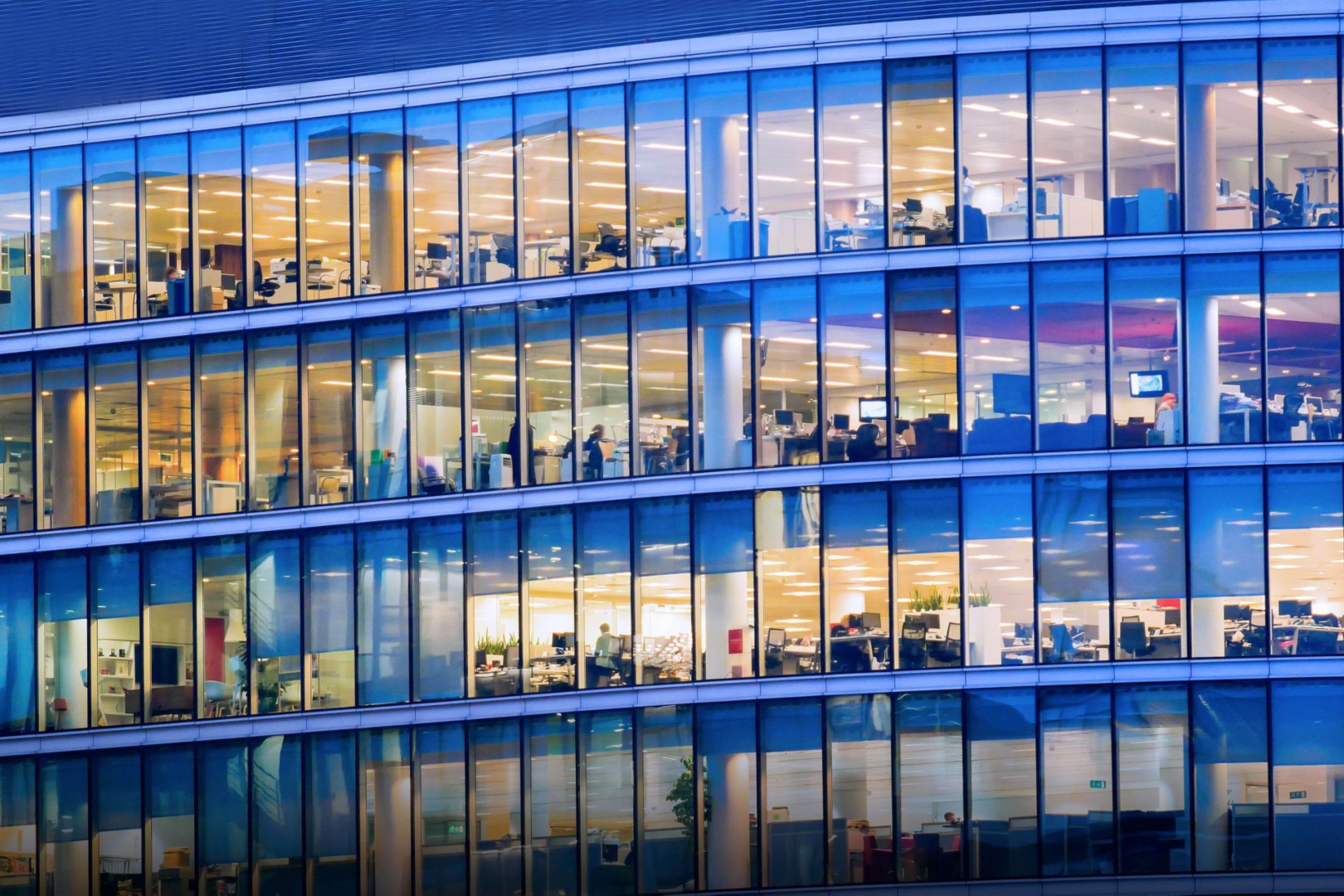
BEPS 2.0: as policies evolve, engagement is key
It remains to be seen whether the US will align its tax law with the OECD/G20’s global BEPS 2.0 rules. MNEs will feel the impact in 2024. Learn more.
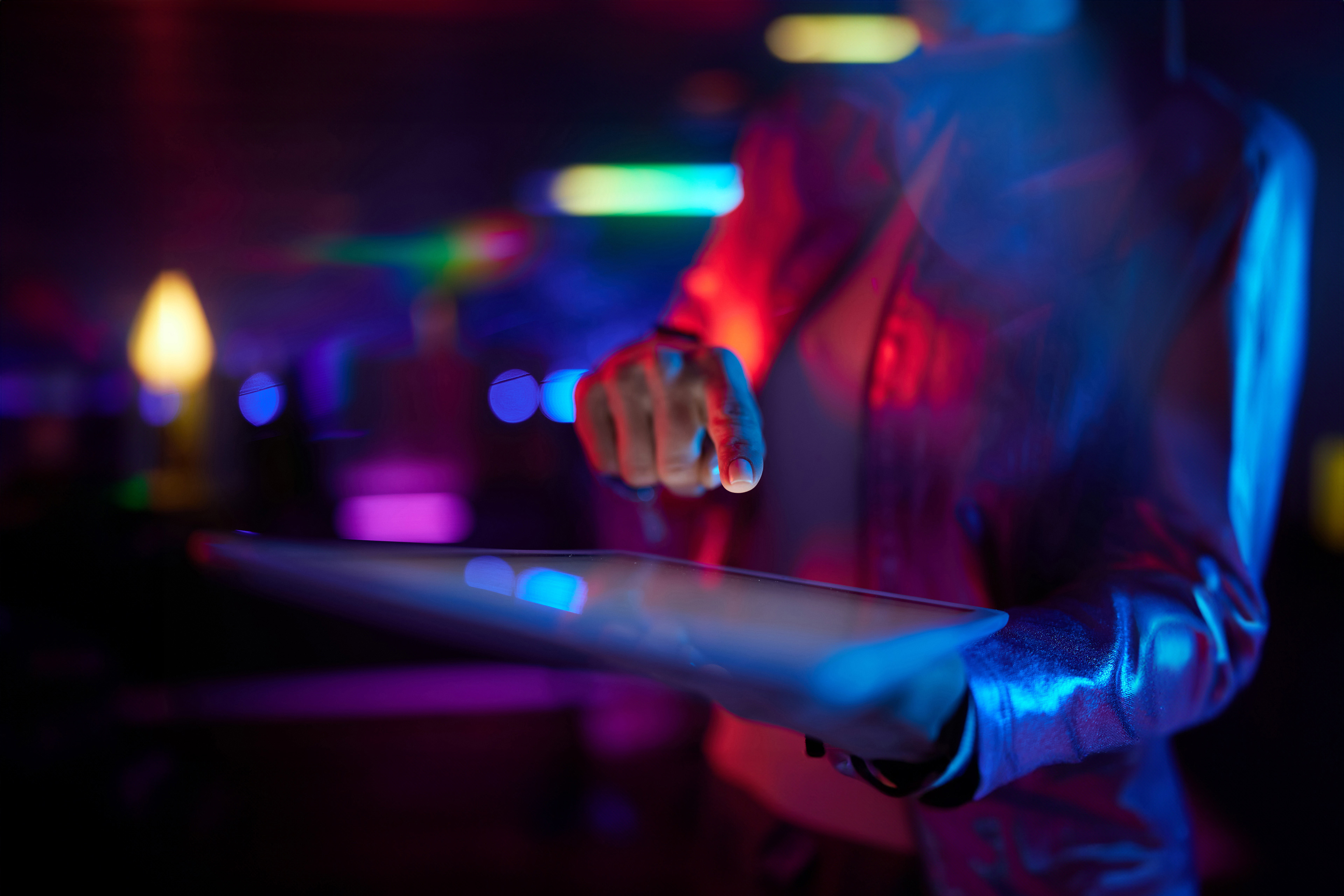
How GenAI strategy can transform innovation
Companies considering or investing in a transformative GenAI strategy should tie generative artificial intelligence use cases to revenue, cost and expense. Learn more
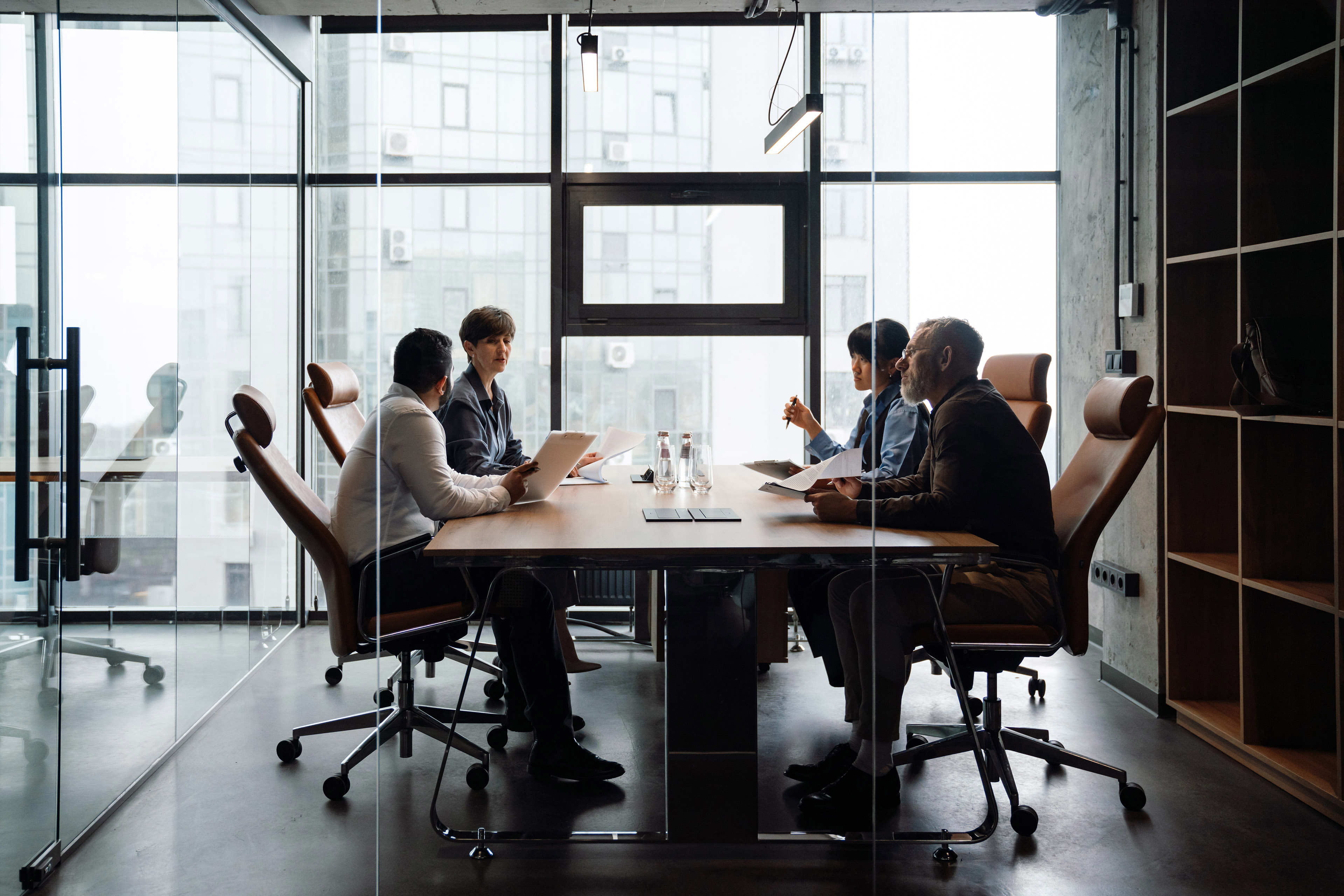
Top five private equity trends for 2024
Read about the five key trends private equity firms will emphasize in 2024 as they create value
Select your location
close expand_more
Consulting at EY is building a better working world by realizing business transformation through the power of people, technology and innovation.
The nature of work is evolving fast, we’re all being asked to adopt new behaviors — to be more innovative, more agile, more collaborative, more everything.
Business today is anything but usual. There is an urgency demanding we look ahead through a new lens, reframe the future and ask new and different questions:
- How do you create customer intimacy without proximity?
- Where does employee centricity meet the future of work?
- How can technology at speed create competitive advantage?
- Where does innovation at scale meet the new "S-curve" of growth?
By placing humans at the center, leveraging technology at speed and enabling innovation at scale, our clients are transforming to realize long-term value for people, business and society as a whole.
How organizations can stop skyrocketing AI use from fueling anxiety</p> "> How organizations can stop skyrocketing AI use from fueling anxiety
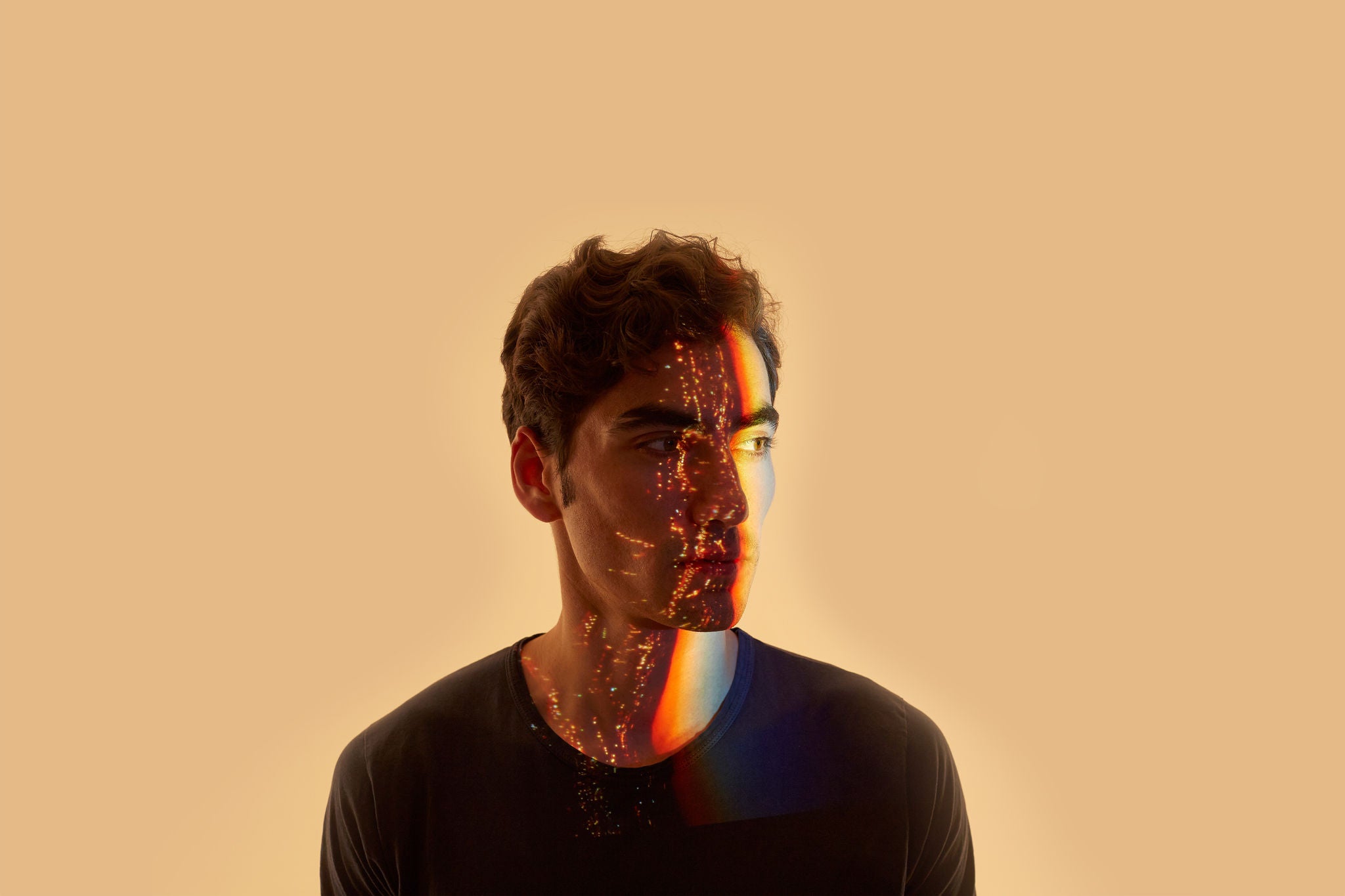
Discover c-suite executive agendas
- Technology leaders' agenda
- Operation leaders' agenda
- Marketing leaders' agenda
- Cyber and privacy leaders' agenda
- Risk leaders' agenda
- Finance leaders’ agenda
Explore our case study library
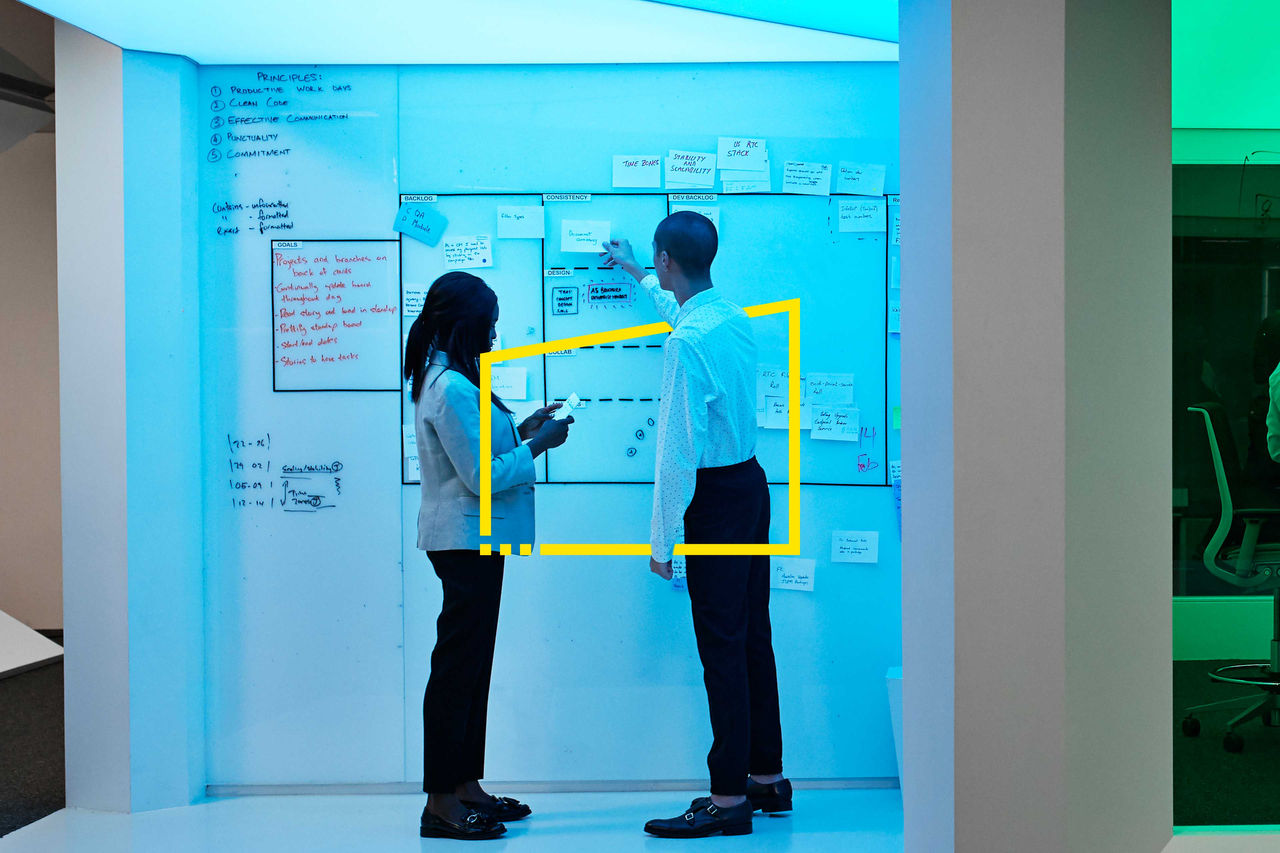
- Our case studies
- Our latest thinking
Explore our case studies
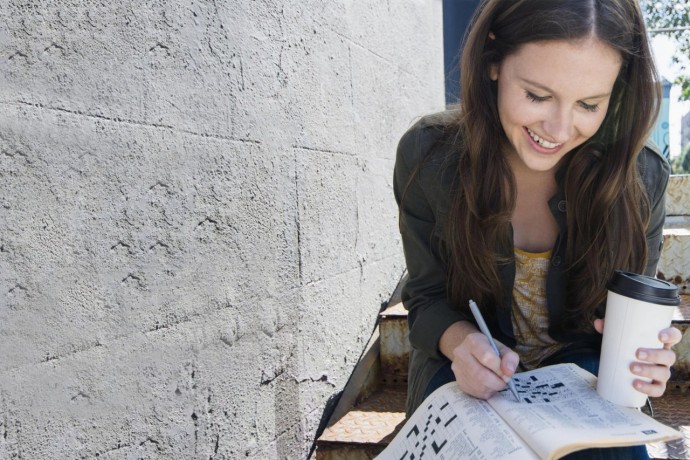
Natural language processing turns search speed into light speed
A managed care company saves hundreds of hours in compliance verification with a new, AI-enabled database. Learn more in this case study.
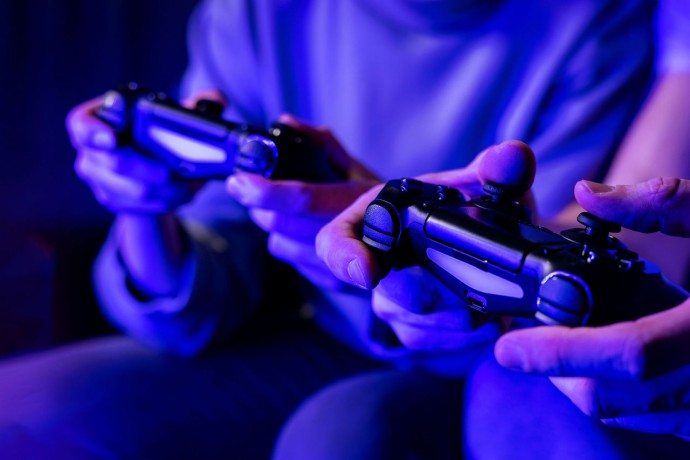
How strong data management becomes a real game-changer
For one video game company, a data-driven culture meant gigs of innovation. Learn more.
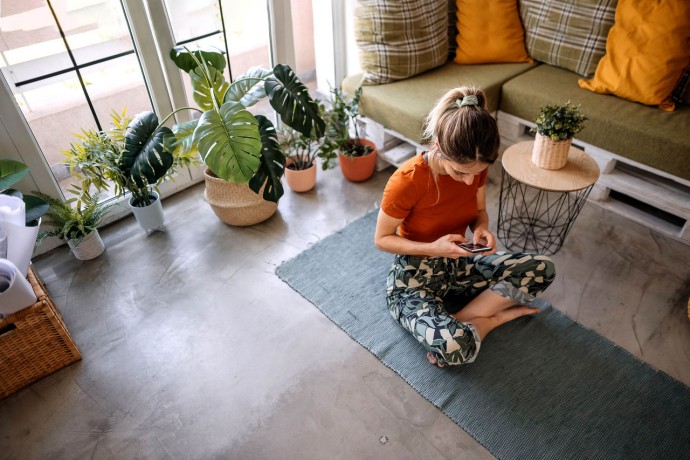
How a major health provider is putting care into patients’ hands
Learn how a leading health care system used digital transformation to enhance its telehealth application and better serve both patients and providers.
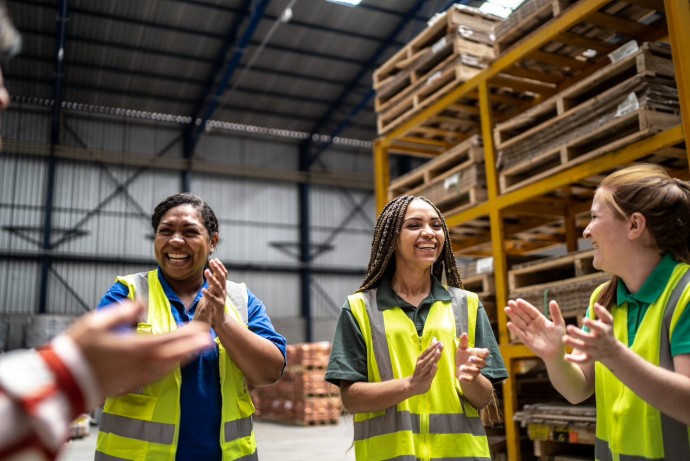
How Ownership Works used a digital springboard to launch a movement
EY teams helped create an online presence — providing employees with the opportunity to build wealth at work.
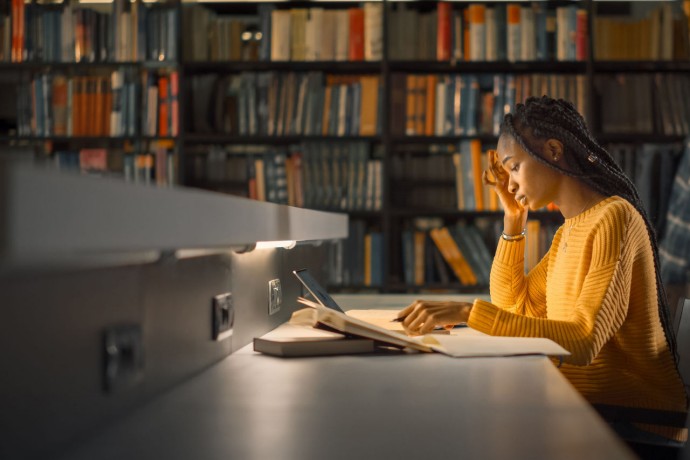
Why cybersecurity should be required reading for higher education
Following a full cybersecurity assessment, a university lands on a solution that can identify, triage and manage data risks.
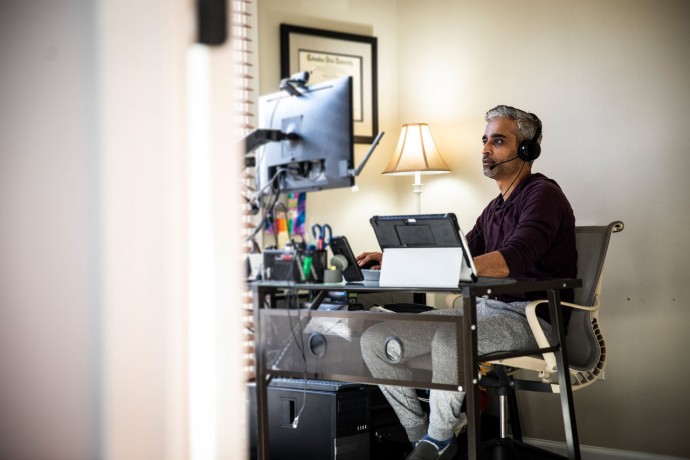
How EY is delivering an exceptional experience for its workforce
Learn how EY People Advisory Services has helped the global EY organization realize one of the largest end-to-end HR transformations on record.
Explore our latest thinking
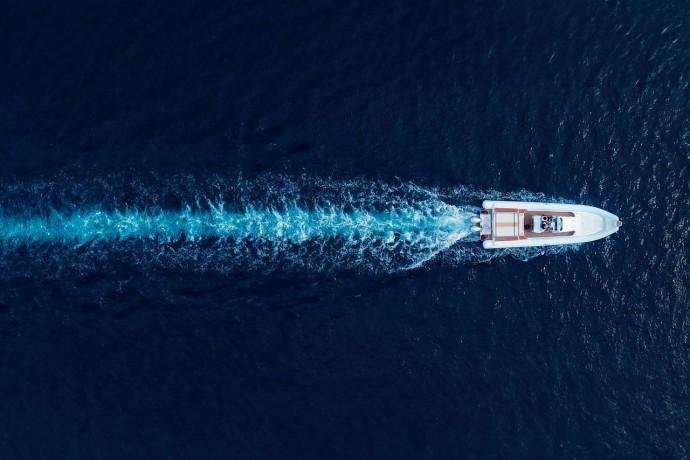
Can core platform modernization position a bank for future success?
Case study: how one regional bank used core platform modernization to build a strong foundation for future profitability.
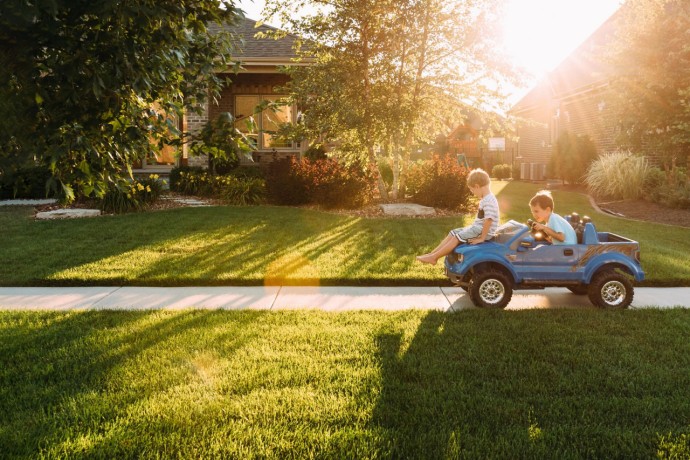
Case study: Intelligent automation shifts a state agency into higher gear
In this case study, learn how a state agency used robotic process automation to serve its customers with speed and convenience.
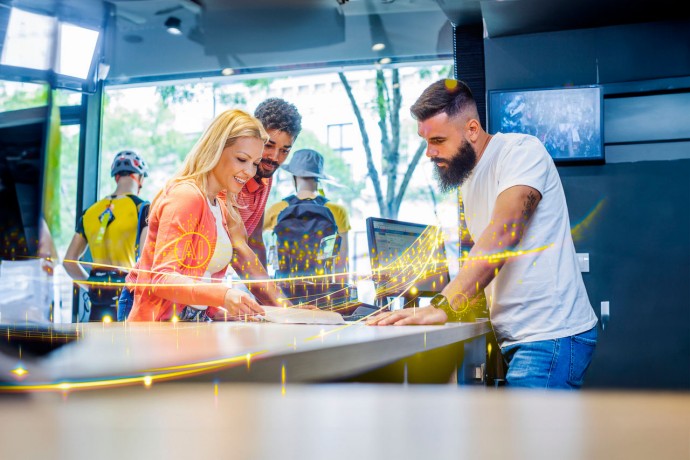
What strategic ops mean for your business
Many executives think of operations as a cost center. A 180-degree shift in thinking could lead to competitive advantage. Learn more.
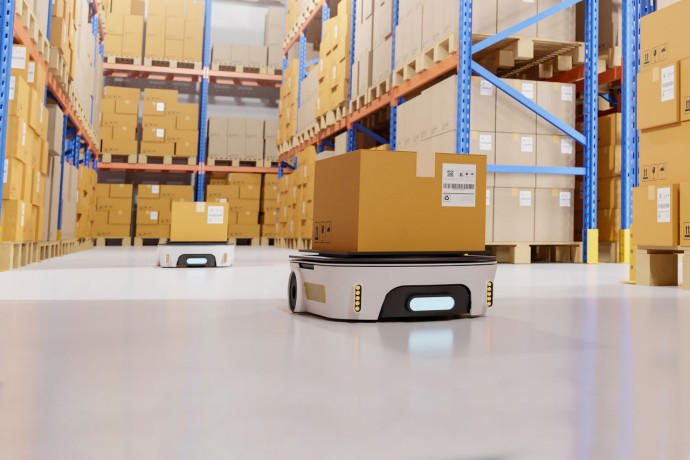
How companies are achieving distribution transformation at speed and scale
Read how organizations can improve global supply chain efficiency through an integrated network of distribution centers.
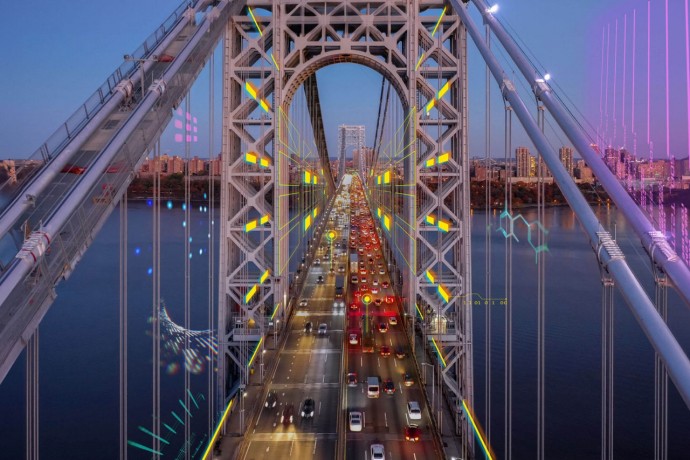
AI Summit NYC: How leaders are tapping the potential of AI
Read session summaries and watch videos from AI Summit NYC where leaders demonstrate how AI in business boosts efficiency and productivity and drives growth.
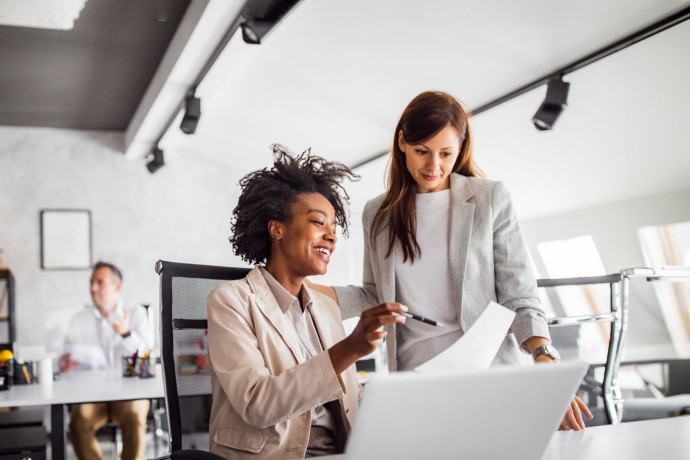
Six ways CFOs and CSCOs can collectively drive value
Despite the challenges of frequently diverging objectives, the value that these executives can collaboratively unlock is transformative. Learn more.
EY Consulting case studies are a window into how we work alongside our clients to deliver strategic, sustainable growth and success.
How EY can help
Transformation EQ™ services
EY Transformation EQ™ services help your business implement leading practices and harness the power of your people to drive transformation success. Learn more.
Service Leader

EY Global People Advisory Services Tax Deputy Leader
Business reinvention consulting services
EY Business reinvention consulting services can help your business deliver long-term value for customers, employees, businesses and society. Learn more.
Evolving technology and data through cloud services
EY’s Business transformation through cloud services can help your business unlock the agility, efficiency and innovation of composable business services. Learn more.
Commercial excellence services
EY Commercial excellence helps organizations unlock, create and sustain new organic value in a fast-changing world.
Customer experience services
The EY Customer Experience solution can help your business integrate CX programs that help deliver sustainable long-term value. Discover more.
Cybersecurity Transformation
Discover how EY's Cybersecurity Transformation solution can help your organization design, deliver, and maintain cybersecurity programs.
Data and Insight-driven Transformation solution
EY Data and Insight-Driven Transformation teams can help you use technology and data to modernize and innovate your business and prepare for future disruption. Learn more.
</b>Enterprise Resilience" href="https://www.ey.com/en_us/services/consulting/enterprise-resilience"> Enterprise Resilience
Discover how EY can help transform your business to navigate disruption with agility, stay competitive in the market and help generate long-term value.
Internal Audit Transformation Services
EY IA services can help your business define the IA vision to create value from thought leadership, digital insights, and risk management. Learn more.
Organization and Workforce Transformation
Our Organization and Workforce Transformation solution can help build workforce capabilities needed to realize organizational strategy. Read more.
People Experience solution
EY People Experience can help you build insight, develop interventions and scale people experience capabilities. Learn more.
Product and Service Innovation consulting services
EY Product and Service Innovation teams can help your business identify, define and design products and services that deliver long-term value. Discover more.
EY Supply Chain Transformation solution
Discover how EY's Supply Chain Transformation solution can help your business move towards fully autonomous, connected supply chains that drive business growth.
Sustainability governance, risk and compliance solution
EY Sustainability GRC can help you implement a digitally enabled ESG framework that is embedded in your operating model and meets regulatory requirements.
Transformation delivery solution
EY Transformation Delivery solution improves your confidence over time in achieving your transformation outcomes. Find out how.
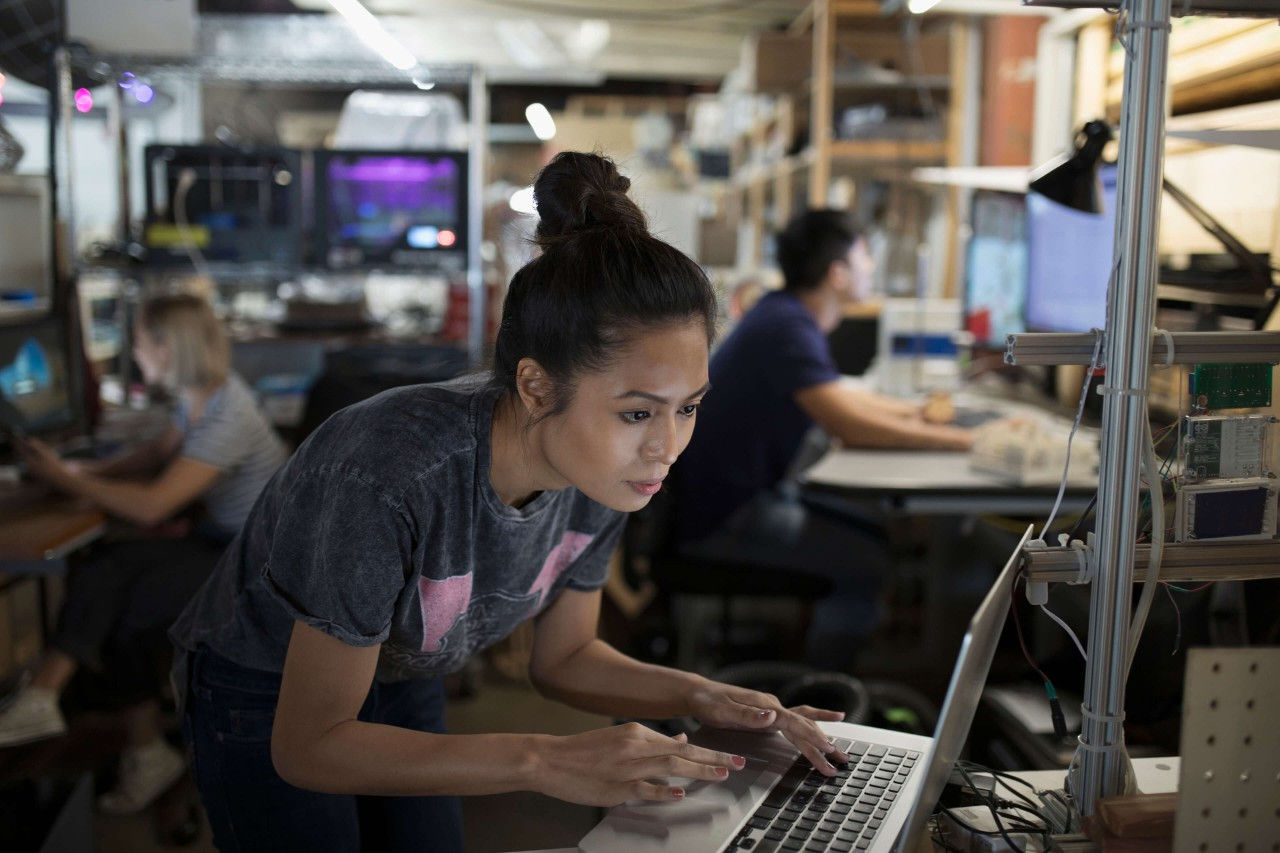
Transformational leader. Innovator. Technologist. Complex problem solver. Strategist. Husband. Father. Mentor. Volunteer. Auto enthusiast.
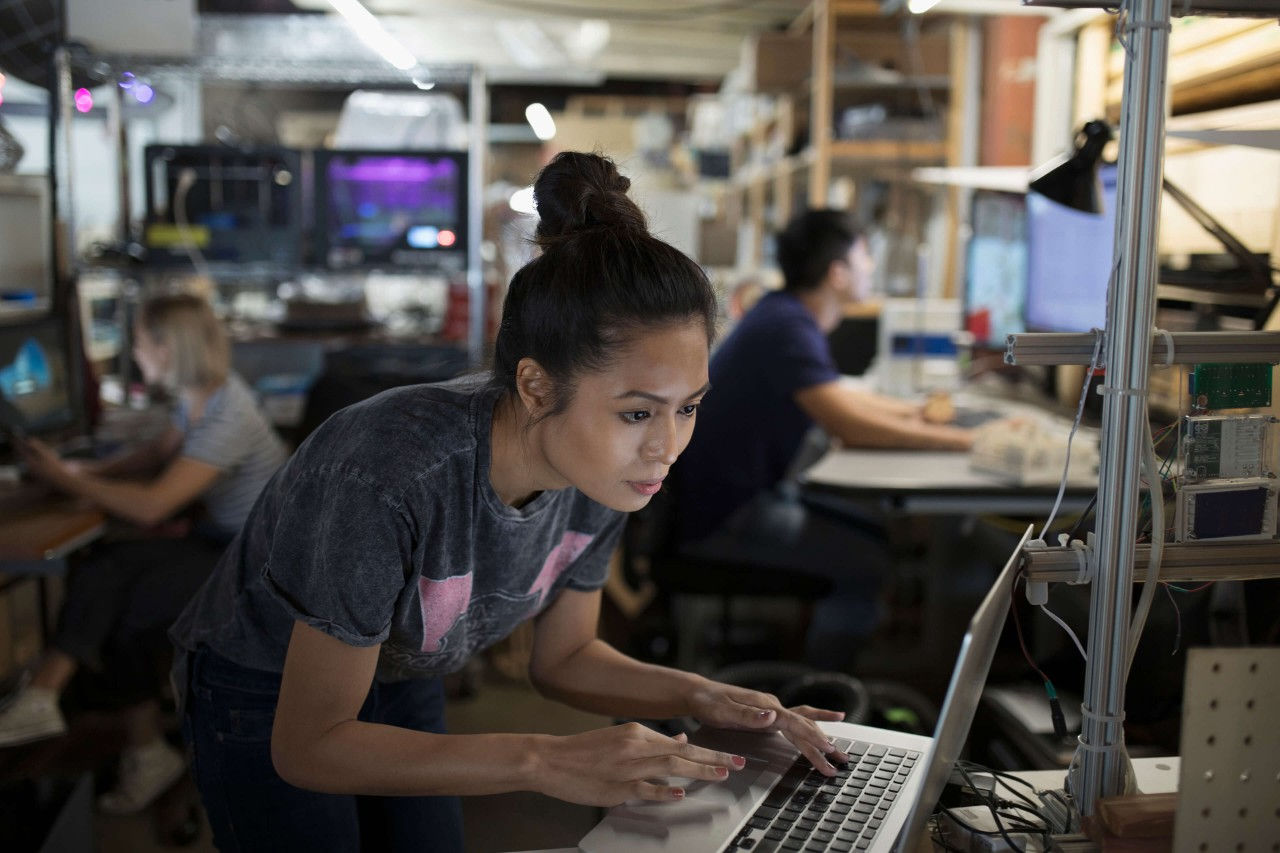
Errol Gardner
Helping clients embrace disruption to drive change. Passionate about inclusion and mentoring. Avid Manchester United fan.
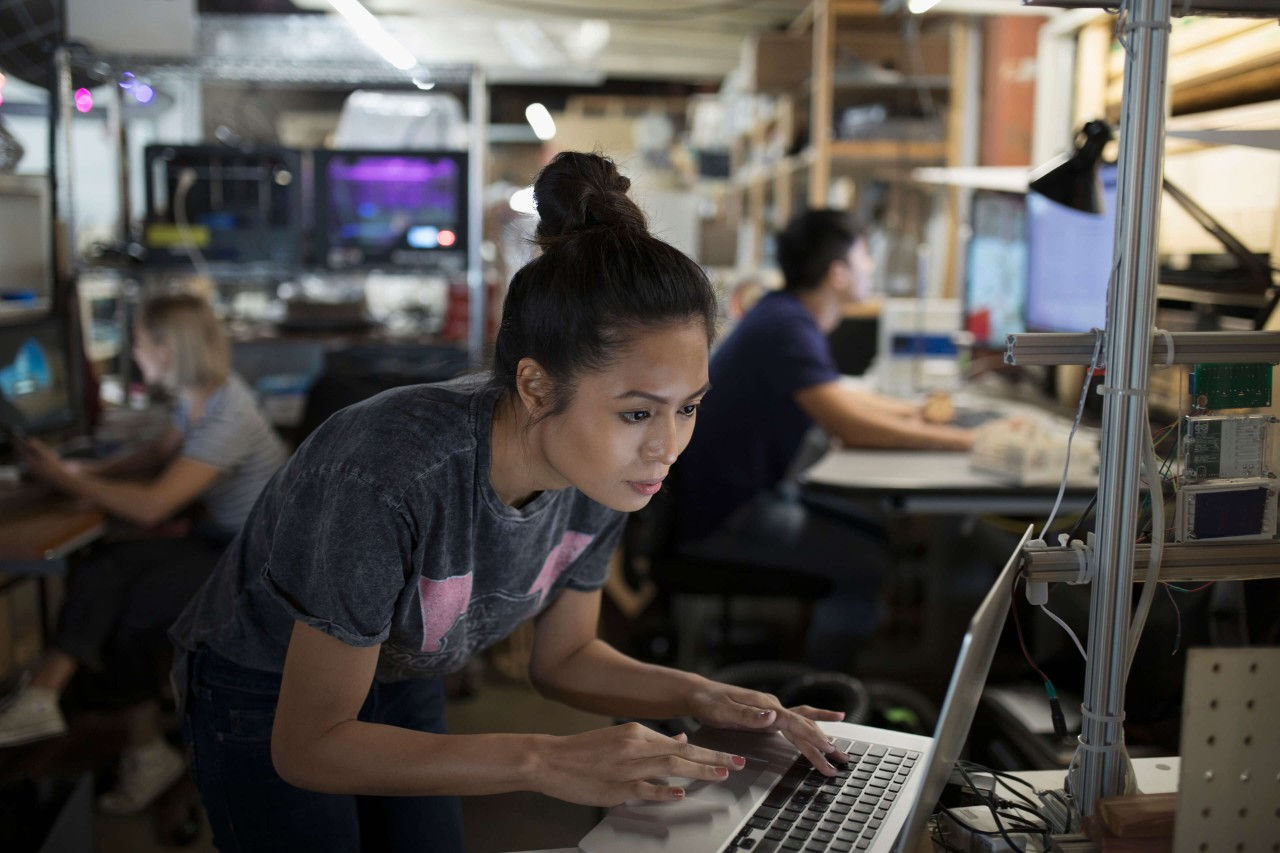
Lisa Caldwell
Recognized for building business models, systems and processes that drive profitable growth in highly competitive markets while championing the next generation of leaders.
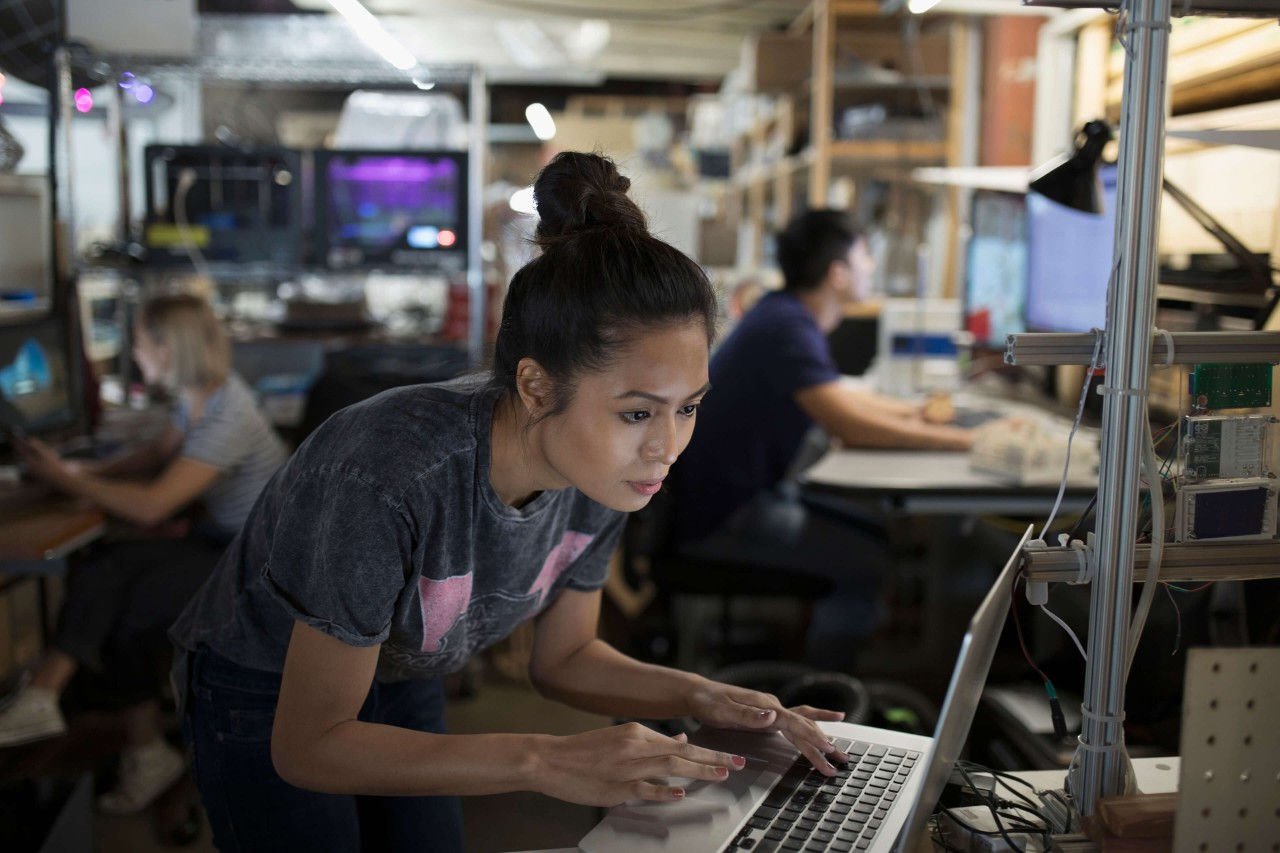
Focused on driving technology transformation, innovation and strategy. Husband. Father. Volunteer. Golfer. Hockey fan. Supports diversity and inclusion.
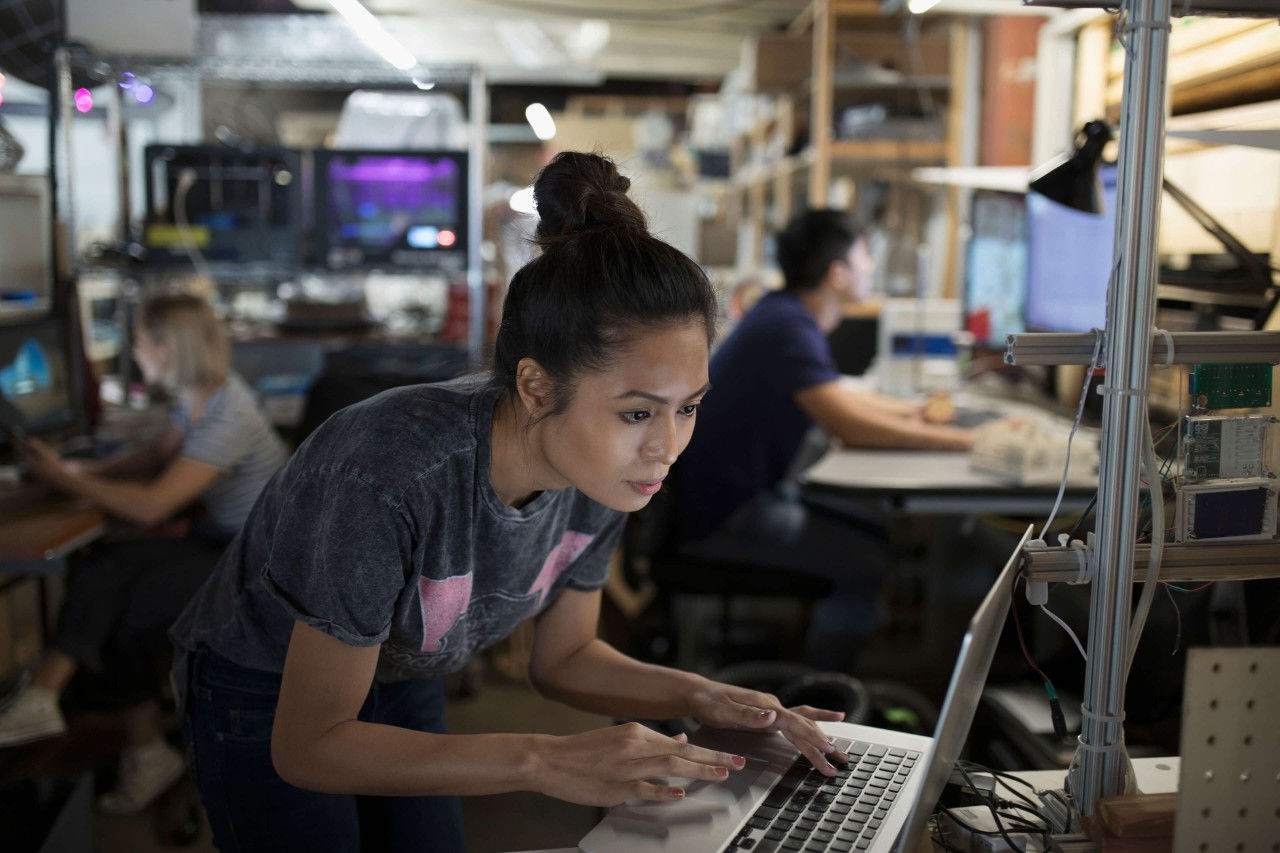
Nalika Nanayakkara
Wealth and Asset management industry leader. Passionate about innovative market solutions as well as helping underserved demographic groups get the financial advice they need.
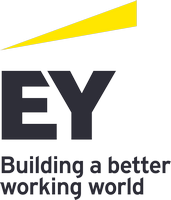
- Connect with us
- Our locations
- Do Not Sell or Share My Personal Information
- Legal and privacy
- Accessibility
- Open Facebook profile
- Open X profile
- Open LinkedIn profile
- Open Youtube profile
EY refers to the global organization, and may refer to one or more, of the member firms of Ernst & Young Global Limited, each of which is a separate legal entity. Ernst & Young Global Limited, a UK company limited by guarantee, does not provide services to clients.
- Open access
- Published: 18 April 2024
The predictive power of data: machine learning analysis for Covid-19 mortality based on personal, clinical, preclinical, and laboratory variables in a case–control study
- Maryam Seyedtabib ORCID: orcid.org/0000-0003-1599-9374 1 ,
- Roya Najafi-Vosough ORCID: orcid.org/0000-0003-2871-5748 2 &
- Naser Kamyari ORCID: orcid.org/0000-0001-6245-5447 3
BMC Infectious Diseases volume 24 , Article number: 411 ( 2024 ) Cite this article
Metrics details
Background and purpose
The COVID-19 pandemic has presented unprecedented public health challenges worldwide. Understanding the factors contributing to COVID-19 mortality is critical for effective management and intervention strategies. This study aims to unlock the predictive power of data collected from personal, clinical, preclinical, and laboratory variables through machine learning (ML) analyses.
A retrospective study was conducted in 2022 in a large hospital in Abadan, Iran. Data were collected and categorized into demographic, clinical, comorbid, treatment, initial vital signs, symptoms, and laboratory test groups. The collected data were subjected to ML analysis to identify predictive factors associated with COVID-19 mortality. Five algorithms were used to analyze the data set and derive the latent predictive power of the variables by the shapely additive explanation values.
Results highlight key factors associated with COVID-19 mortality, including age, comorbidities (hypertension, diabetes), specific treatments (antibiotics, remdesivir, favipiravir, vitamin zinc), and clinical indicators (heart rate, respiratory rate, temperature). Notably, specific symptoms (productive cough, dyspnea, delirium) and laboratory values (D-dimer, ESR) also play a critical role in predicting outcomes. This study highlights the importance of feature selection and the impact of data quantity and quality on model performance.
This study highlights the potential of ML analysis to improve the accuracy of COVID-19 mortality prediction and emphasizes the need for a comprehensive approach that considers multiple feature categories. It highlights the critical role of data quality and quantity in improving model performance and contributes to our understanding of the multifaceted factors that influence COVID-19 outcomes.
Peer Review reports
Introduction
The World Health Organization (WHO) has declared COVID-19 a global pandemic in March 2020 [ 1 ]. The first cases of SARSCoV-2, a new severe acute respiratory syndrome coronavirus, were detected in Wuhan, China, and rapidly spread to become a global public health problem [ 2 ]. The clinical presentation and symptoms of COVID-19 may be similar to those of Middle East Respiratory Syndrome (MERS) and Severe Acute Respiratory Syndrome (SARS), however the rate of spread is higher [ 3 ]. By December 31, 2022, the pandemic had caused more than 729 million cases and nearly 6.7 million deaths (0.92%) were confirmed in 219 countries worldwide [ 4 ]. For many countries, figuring out what measures to take to prevent death or serious illness is a major challenge. Due to the complexity of transmission and the lack of proven treatments, COVID-19 is a major challenge worldwide [ 5 , 6 ]. In middle- and low-income countries, the situation is even more catastrophic due to high illiteracy rates, a very poor health care system, and lack of intensive care units [ 5 ]. In addition, understanding the factors contributing to COVID-19 mortality is critical for effective management and intervention strategies [ 6 ].
Numerous studies have shown several factors associated with COVID-19 outcomes, including socioeconomic, environmental, individual demographic, and health factors [ 7 , 8 , 9 ]. Risk factors for COVID -19 mortality vary by study and population studied [ 10 ]. Age [ 11 , 12 ], comorbidities such as hypertension, cardiovascular disease, diabetes, and COPD [ 13 , 14 , 15 ], sex [ 13 ], race/ethnicity [ 11 ], dementia, and neurologic disease [ 16 , 17 ], are some of the factors associated with COVID-19 mortality. Laboratory factors such as elevated levels of inflammatory markers, lymphopenia, elevated creatinine levels, and ALT are also associated with COVID-19 mortality [ 5 , 18 ]. Understanding these multiple risk factors is critical to accurately diagnose and treat COVID-19 patients.
Accurate diagnosis and treatment of the disease requires a comprehensive assessment that considers a variety of factors. These factors include personal factors such as medical history, lifestyle, and genetics; clinical factors such as observations on physical examinations and physician reports; preclinical factors such as early detection through screening or surveillance; laboratory factors such as results of diagnostic tests and medical imaging; and patient-reported signs and symptoms. However, the variety of characteristics associated with COVID-19 makes it difficult for physicians to accurately classify COVID-19 patients during the pandemic.
In today's digital transformation era, machine learning plays a vital role in various industries, including healthcare, where substantial data is generated daily [ 19 , 20 , 21 ]. Numerous studies have explored machine learning (ML) and explainable artificial intelligence (AI) in predicting COVID-19 prognosis and diagnosis [ 22 , 23 , 24 , 25 ]. Chadaga et al. have developed decision support systems and triage prediction systems using clinical markers and biomarkers [ 22 , 23 ]. Similarly, Khanna et al. have developed a ML and explainable AI system for COVID-19 triage prediction [ 24 ]. Zoabi has also made contributions in this field, developing ML models that predict COVID-19 test results with high accuracy based on a small number of features such as gender, age, contact with an infected person and initial clinical symptoms [ 25 ]. These studies emphasize the potential of ML and explainable AI to improve COVID-19 prediction and diagnosis. Nonetheless, the efficacy of ML algorithms heavily relies on the quality and quantity of data utilized for training. Recent research has indicated that deep learning algorithms' performance can be significantly enhanced compared to traditional ML methods by increasing the volume of data used [ 26 ]. However, it is crucial to acknowledge that the impact of data volume on model performance can vary based on data characteristics and experimental setup, highlighting the need for careful consideration and analysis when selecting data for model training. While the studies emphasize the importance of features in training ML algorithms for COVID-19 prediction and diagnosis, additional research is required on methods to enhance the interpretability of features.
Therefore, the primary aim of this study is to identify the key factors associated with mortality in COVID -19 patients admitted to hospitals in Abadan, Iran. For this purpose, seven categories of factors were selected, including demographic, clinical and conditions, comorbidities, treatments, initial vital signs, symptoms, and laboratory tests, and machine learning algorithms were employed. The predictive power of the data was assessed using 139 predictor variables across seven feature sets. Our next goal is to improve the interpretability of the extracted important features. To achieve this goal, we will utilize the innovative SHAP analysis, which illustrates the impact of features through a diagram.
Materials and methods
Study population and data collection.
Using data from the COVID-19 hospital-based registry database, a retrospective study was conducted from April 2020 to December 2022 at Ayatollah Talleghani Hospital (a COVID‑19 referral center) in Abadan City, Iran.
A total of 14,938 patients were initially screened for eligibility for the study. Of these, 9509 patients were excluded because their transcriptase polymerase chain reaction (RT-PCR) test results were negative or unspecified. The exclusion of patients due to incomplete or missing data is a common issue in medical research, particularly in the use of electronic medical records (EMRs) [ 27 ]. In addition, 1623 patients were excluded because their medical records contained more than 70% incomplete or missing data. In addition, patients younger than 18 years were not included in the study. The criterion for excluding 1623 patients due to "70% incomplete or missing data" means that the medical records of these patients did not contain at least 30% of the data required for a meaningful analysis. This threshold was set to ensure that the dataset used for the study contained a sufficient amount of complete and reliable information to draw accurate conclusions. Incomplete or missing data in a medical record may relate to key variables such as patient demographics, symptoms, lab results, treatment information, outcomes, or other data points important to the research. Insufficient data can affect the validity and reliability of study results and lead to potential bias or inaccuracies in the findings. It is important to exclude such incomplete records to maintain the quality and integrity of the research findings and to ensure that the conclusions drawn are based on robust and reliable data. After these exclusions, 3806 patients remained. Of these patients, 474 died due to COVID -19, while the remaining 3332 patients recovered and were included in the control group. To obtain a balanced sample, the control group was selected with a propensity score matching (PSM). The PSM refers to a statistical technique used to create a balanced comparison group by matching individuals in the control group (in this case, the survived group) with individuals in the case group (in this case, the deceased group) based on their propensity scores. In this study, the propensity scores for each person represented the probability of death (coded as a binary outcome; survived = 0, deceased = 1) calculated from a set of covariates (demographic factors) using the matchit function from the MatchIt library. Two individuals, one from the deceased group and one from the survived group, are considered matched if the difference between their propensity scores is small. Non-matching participants are discarded. The matching aims to reduce bias by making the distribution of observed characteristics similar between groups, which ultimately improves the comparability of groups in observational studies [ 28 ]. In total, the study included 1063 COVID-19 patients who belonged to either the deceased group (case = 474) or the survived group (control = 589) (Fig. 1 ).
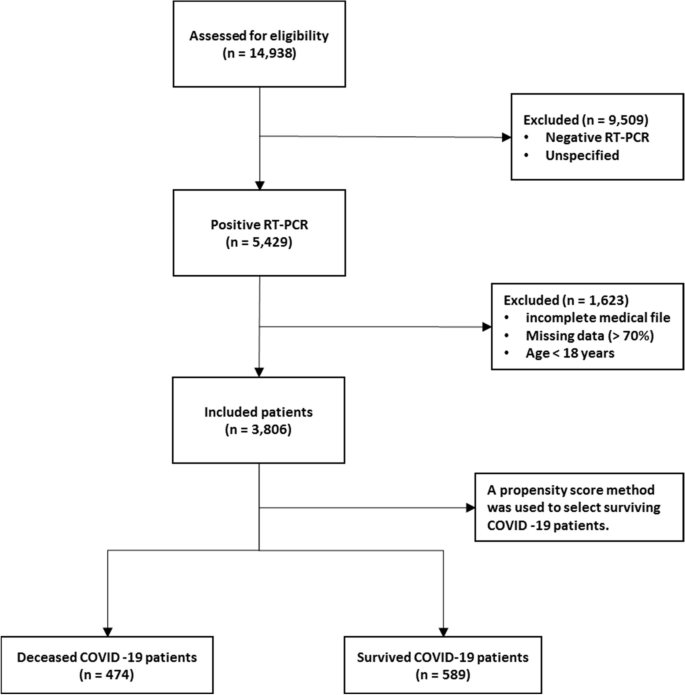
Flowchart describing the process of patient selection
In the COVID‑19 hospital‑based registry database, one hundred forty primary features in eight main classes including patient’s demographics (eight features), clinical and conditions features (16 features), comorbidities (18 features), treatment (17 features), initial vital sign (14 features), symptoms during hospitalization (31 features), laboratory results (35 features), and an output (0 for survived and 1 for deceased) was recorded for COVID-19 patients. The main features included in the hospital-based COVID-19 registry database are provided in Appendix Table 1 .
To ensure the accuracy of the recorded information, discharged patients or their relatives were called and asked to review some of the recorded information (demographic information, symptoms, and medical history). Clinical symptoms and vital signs were referenced to the first day of hospitalization (at admission). Laboratory test results were also referenced to the patient’s first blood sample at the time of hospitalization.
The study analyzed 140 variables in patients' records, normalizing continuous variables and creating a binary feature to categorize patients based on outcomes. To address the issue of an imbalanced dataset, the Synthetic Minority Over-sampling Technique (SMOTE) was utilized. Some classes were combined to simplify variables. For missing data, an imputation technique was applied, assuming a random distribution [ 29 ]. Little's MCAR test was performed with the naniar package to assess whether missing data in a dataset is missing completely at random (MCAR) [ 30 ]. The null hypothesis in this test is that the data are MCAR, and the test statistic is a chi-square value.
The Ethics Committee of Abadan University of Medical Science approved the research protocol (No. IR.ABADANUMS.REC.1401.095).
Predictor variables
All data were collected in eight categories, including demographic, clinical and conditions, comorbidities, treatment, initial vital signs, symptoms, and laboratory tests in medical records, for a total of 140 variables.
The "Demographics" category encompasses eight features, three of which are binary variables and five of which are categorical. The "Clinical Conditions" category includes 16 features, comprising one quantitative variable, 12 binary variables, and five categorical features. " Comorbidities ", " Treatment ", and " Symptoms " each have 18, 17, and 30 binary features, respectively. Also, there is one quantitative variable in symptoms category. The "Initial Vital Signs" category features 11 quantitative variables, two binary variables, and one categorical variable. Finally, the "Laboratory Tests" category comprises 35 features, with 33 being quantitative, one categorical, and one binary (Appendix Table 1 ).
Outcome variable
The primary outcome variable was mortality, with December 31, 2022, as the last date of follow‐up. The feature shows the class variable, which is binary. For any patient in the survivor group, the outcome is 0; otherwise, it is 1. In this study, 44.59% ( n = 474) of the samples were in the deceased group and were labeled 1.
Data balancing
In case–control studies, it is common to have unequal size groups since cases are typically fewer than controls [ 31 ]. However, in case–control studies with equal sizes, data balancing may not be necessary for ML algorithms [ 32 ]. When using ML algorithms, data balancing is generally important when there is an imbalance between classes, i.e., when one class has significantly fewer observations than the other [ 33 ]. In such cases, balancing can improve the performance of the algorithm by reducing the bias in favor of the majority class [ 34 ]. For case–control studies of the same size, the balance of the classes has already been reached and balancing may not be necessary. However, it is always recommended to evaluate the performance of the ML algorithm with the given data set to determine the need for data balancing. This is because unbalanced case–control ratios can cause inflated type I error rates and deflated type I error rates in balanced studies [ 35 ].
Feature selection
Feature selection is about selecting important variables from a large dataset to be used in a ML model to achieve better performance and efficiency. Another goal of feature selection is to reduce computational effort by eliminating irrelevant or redundant features [ 36 , 37 ]. Before generating predictions, it is important to perform feature selection to improve the accuracy of clinical decisions and reduce errors [ 37 ]. To identify the best predictors, researchers often compare the effectiveness of different feature selection methods. In this study, we used five common methods, including Decision Tree (DT), eXtreme Gradient Boosting (XGBoost), Support Vector Machine (SVM), Naïve Bayes (NB), and Random Forest (RF), to select relevant features for predicting mortality of COVID -19 patients. To avoid overfitting, we performed ten-fold cross-validation when training our dataset. This approach may help ensure that our model is optimized for accurate predictions of health status in COVID -19 patients.
Model development, evaluation, and clarity
In this study, the predictive models were developed with five ML algorithms, including DT, XGBoost, SVM, NB, and RF, using the R programming language (v4.3.1) and its packages [ 38 ]. We used cross-validation (CV) to tune the hyperparameters of our models based on the training subset of the dataset. For training and evaluating our ML models, we used a common technique called tenfold cross validation [ 39 ]. The primary training dataset was divided into ten folding, each containing 10% of the total data, using a technique called stratified random sampling. For each of the 30% of the data, a ML model was built and trained on the remaining 70% of the data. The performance of the model was then evaluated on the 30%-fold sample. This process was repeated 100 times with different training and test combinations, and the average performance was reported.
Performance measures include sensitivity (recall), specificity, accuracy, F1-score, and the area under the receiver operating characteristics curve (AUC ROC). Sensitivity is defined as TP / (TP + FN), whereas specificity is TN / (TN + FP). F1-score is defined as the harmonic mean of Precision and Recall with equal weight, where Precision equals TP + TN / total. Also, AUC refers to the area under the ROC curve. In the evaluation of ML techniques, values were classified as poor if below 50%, ok if between 50 and 80%, good if between 80 and 90%, and very good if greater than 90%. These criteria are commonly used in reporting model evaluations [ 40 , 41 ].
Finally, the shapely additive explanation (SHAP) method was used to provide clarity and understanding of the models. SHAP uses cooperative game theory to determine how each feature contributes to the prediction of ML models. This approach allows the computation of the contribution of each feature to model performance [ 42 , 43 ]. For this purpose, the package shapr was used, which includes a modified iteration of the kernel SHAP approach that takes into account the interdependence of the features when computing the Shapley values [ 44 ].
Patient characteristics
Table 1 shows the baseline characteristics of patients infected with COVID-19, including demographic data such as age and sex and other factors such as occupation, place of residence, marital status, education level, BMI, and season of admission. A total of 1063 adult patients (≥ 18 years) were enrolled in the study, of whom 589 (55.41%) survived and 474 (44.59%) died. Analysis showed that age was significantly different between the two groups, with a mean age of 54.70 ± 15.60 in the survivor group versus 65.53 ± 15.18 in the deceased group ( P < 0.001). There was also a significant association between age and survival, with a higher proportion of patients aged < 40 years in the survivor group (77.0%) than in the deceased group (23.0%) ( P < 0.001). No significant differences were found between the two groups in terms of sex, occupation, place of residence, marital status, and time of admission. However, there was a significant association between educational level and survival, with a lower proportion of patients with a college degree in the deceased group (37.2%) than in the survivor group (62.8%) ( P = 0.017). BMI also differed significantly between the two groups, with the proportion of patients with a BMI > 30 (kg/cm 2 ) being higher in the deceased group (56.5%) than in the survivor group (43.5%) ( P < 0.001).
Clinical and conditions
Important insights into the various clinical and condition characteristics associated with COVID-19 infection outcomes provides in Table 2 . The results show that patients who survived the infection had a significantly shorter hospitalization time (2.20 ± 1.63 days) compared to those who died (4.05 ± 3.10 days) ( P < 0.001). Patients who were admitted as elective cases had a higher survival rate (84.6%) compared to those who were admitted as urgent (61.3%) or emergency (47.4%) cases. There were no significant differences with regard to the number of infections or family infection history. However, patients who had a history of travel had a lower decease rate (40.1%).
A significantly higher proportion of deceased patients had cases requiring CPR (54.7% vs. 45.3%). Patients who had underlying medical conditions had a significantly lower survival rate (38.3%), with hyperlipidemia being the most prevalent condition (18.7%). Patients who had a history of alcohol consumption (12.5%), transplantation (30.0%), chemotropic (21.4%) or special drug use (0.0%), and immunosuppressive drug use (30.0%) also had a lower survival rate. Pregnant patients (44.4%) had similar survival outcomes compared to non-pregnant patients (55.6%). Patients who were recent or current smokers (36.4%) also had a significantly lower survival rate.
Comorbidities
Table 3 summarizes the comorbidity characteristics of COVID-19 infected patients. Out of 1063 patients, 54.84% had comorbidities. Chi-Square tests for individual comorbidities showed that most of them had a significant association with COVID-19 outcomes, with P -values less than 0.05. Among the various comorbidities, hypertension (HTN) and diabetes mellitus (DM) were the most prevalent, with 12% and 11.5% of patients having these conditions, respectively. The highest fatality rates were observed among patients with cardiovascular disease (95.5%), chronic kidney disease (62.5%), gastrointestinal (GI) (93.3%), and liver diseases (73.3%). Conversely, patients with neurology comorbidities had the lowest fatality rate (0%). These results highlight the significant role of comorbidities in COVID-19 outcomes and emphasize the need for special attention to be paid to patients with pre-existing health conditions.
The treatment characteristics of the COVID-19 patients and the resulting outcomes are shown in Table 4 . The table shows the frequency of patients who received different types of medications or therapies during their treatment. According to the results, the use of antibiotics (35.1%), remdesivir (29.6%), favipiravir (36.0%), and Vitamin zinc (33.5%) was significantly associated with a lower mortality rate ( P < 0.001), suggesting that these medications may have a positive impact on patient outcomes. On the other hand, the use of Heparin (66.1%), Insulin (82.6%), Antifungal (89.6%), ACE inhibitors (78.1%), and Angiotensin II Receptor Blockers (ARB) (83.8%) was significantly associated with increased mortality ( P < 0.001), suggesting that these medications may have a negative effect on the patient's outcome. Also, It seems that taking hydroxychloroquine (51.0%) is associated with a worse outcome at lower significance ( P = 0.022). The use of Atrovent, Corticosteroids and Non-Steroidal Anti-Inflammatory Drugs (NSAIDs) did not show a significant association with survival or mortality rates. Similarly, the use of Intravenous Immunoglobulin (IVIg), Vitamin C, Vitamin D, and Diuretic did not show a significant association with the patient’s outcome.
Initial vital signs
Table 5 provides initial vital sign characteristics of COVID-19 patients, including heart rate, respiratory rate, temperature, blood pressure, oxygen therapy, and radiography test result. The findings shows that deceased patients had higher HR (83.03 bpm vs. 76.14 bpm, P < 0.001), lower RR (11.40 bpm vs. 16.25 bpm, P < 0.001), higher temperature (37.43 °C vs. 36.91 °C, P < 0.001), higher SBP (128.16 mmHg vs. 123.33 mmHg, P < 0.001), and higher O 2 requirements (invasive: 75.0% vs. 25.0%, P < 0.001) compared to the survived patients. Additionally, deceased patients had higher MAP (99.35 mmHg vs. 96.08 mmHg, P = 0.005), and lower SPO 2 percentage (81.29% vs. 91.95%, P < 0.001) compared to the survived patients. Furthermore, deceased patients had higher PEEP levels (5.83 cmH2O vs. 0.69 cmH2O, P < 0.001), higher FiO2 levels (51.43% vs. 8.97%, P < 0.001), and more frequent bilateral pneumonia (63.0% vs. 37.0%, P < 0.001) compared to the survived patients. There appears to be no relationship between diastolic blood pressure and treatment outcome (83.44 mmHg vs. 85.61 mmHg).
Table 6 provides information on the symptoms of patients infected with COVID-19 by survival outcome. The table also shows the frequency of symptoms among patients. The most common symptom reported by patients was fever, which occurred in 67.0% of surviving and deceased patients. Dyspnea and nonproductive cough were the second and third most common symptoms, reported by 40.4% and 29.3% of the total sample, respectively. Other common symptoms listed in the Table were malodor (28.7%), dyspepsia (28.4%), and myalgia (25.6%).
The P -values reported in the table show that some symptoms are significantly associated with death, including productive cough, dyspnea, sore throat, headache, delirium, olfactory symptoms, dyspepsia, nausea, vomiting, sepsis, respiratory failure, heart failure, MODS, coagulopathy, secondary infection, stroke, acidosis, and admission to the intensive care unit. Surviving and deceased patients also differed significantly in the average number of days spent in the ICU. There was no significant association between patient outcomes and symptoms such as nonproductive cough, chills, diarrhea, chest pain, and hyperglycemia.
Laboratory tests
Table 7 shows the laboratory values of COVID-19 patients with the average values of the different laboratory results. The results show that the deceased patients had significantly lower levels of red blood cells (3.78 × 106/µL vs. 5.01 × 106/µL), hemoglobin (11.22 g/dL vs. 14.10 g/dL), and hematocrit (34.10% vs. 42.46%), whereas basophils and white blood cells did not differ significantly between the two groups. The percentage of neutrophils (65.59% vs. 62.58%) and monocytes (4.34% vs. 3.93%) was significantly higher in deceased patients, while the percentage of lymphocytes and eosinophils did not differ significantly between the two groups. In addition, deceased patients had higher levels of certain biomarkers, including D-dimer (1.347 mgFEU/L vs. 0.155 mgFEU/L), lactate dehydrogenase (174.61 U/L vs. 128.48 U/L), aspartate aminotransferase (93.09 U/L vs. 39.63 U/L), alanine aminotransferase (74.48 U/L vs. 28.70 U/L), alkaline phosphatase (119.51 IU/L vs. 81.34 IU/L), creatine phosphokinase-MB (4.65 IU/L vs. 3.33 IU/L), and positive troponin I (56.5% vs. 43.5%). The proportion of patients with positive C-reactive protein was also higher in the deceased group.
Other laboratory values with statistically significant differences between the two groups ( P < 0.001) were INR, ESR, BUN, Cr, Na, K, P, PLT, TSH, T3, and T4. The surviving patients generally had lower values in these laboratory characteristics than the deceased patients.
Model performance and evaluation
Five ML algorithms, namely DT, XGBoost, SVM, NB, and RF, were used in this study to build mortality prediction models COVID -19. The models were based on the optimal feature set selected in a previous step and were trained on the same data set. The effectiveness of the models was evaluated by calculating sensitivity, specificity, accuracy, F1 score, and AUC metrics. Table 8 shows the results of this performance evaluation. The average values are expressed from the test set as the mean (standard deviation).
The results show that the performance of the models varies widely in the different feature categories. The Laboratory Tests category achieved the highest performance, with all models scoring 100% in all metrics. The Symptoms and initial Vital Signs categories also show high performance, with XGBoost achieving the highest accuracy of 98.03% and DT achieving the highest sensitivity of 92.79%.
The Clinical and Conditions category also showed high performance, with all models showing accuracy above 91%. XGBoost achieved the highest sensitivity and specificity of 92.74% and 92.96%, respectively. In contrast, the Demographics category showed the lowest performance, with all models achieving less than 66.5% accuracy.
In summary, the results suggest that certain feature categories may be more useful than others in predicting mortality from COVID-19 and that some ML models may perform better than others depending on the feature category used.
Feature importance
SHapley Additive exPlanations (SHAP) values indicate the importance or contribution of each feature in predicting model output. These values help to understand the influence and importance of each feature on the model's decision-making process.
In Fig. 2 , the mean absolute SHAP values are shown to depict global feature importance. Figure 2 shows the contribution of each feature within its respective group as calculated by the XGBoost prediction model using SHAP. According to the SHAP method, the features that had the greatest impact on predicting COVID-19 mortality were, in descending order: D-dimer, CPR, PEEP, underlying disease, ESR, antifungal treatment, PaO2, age, dyspnea, and nausea.
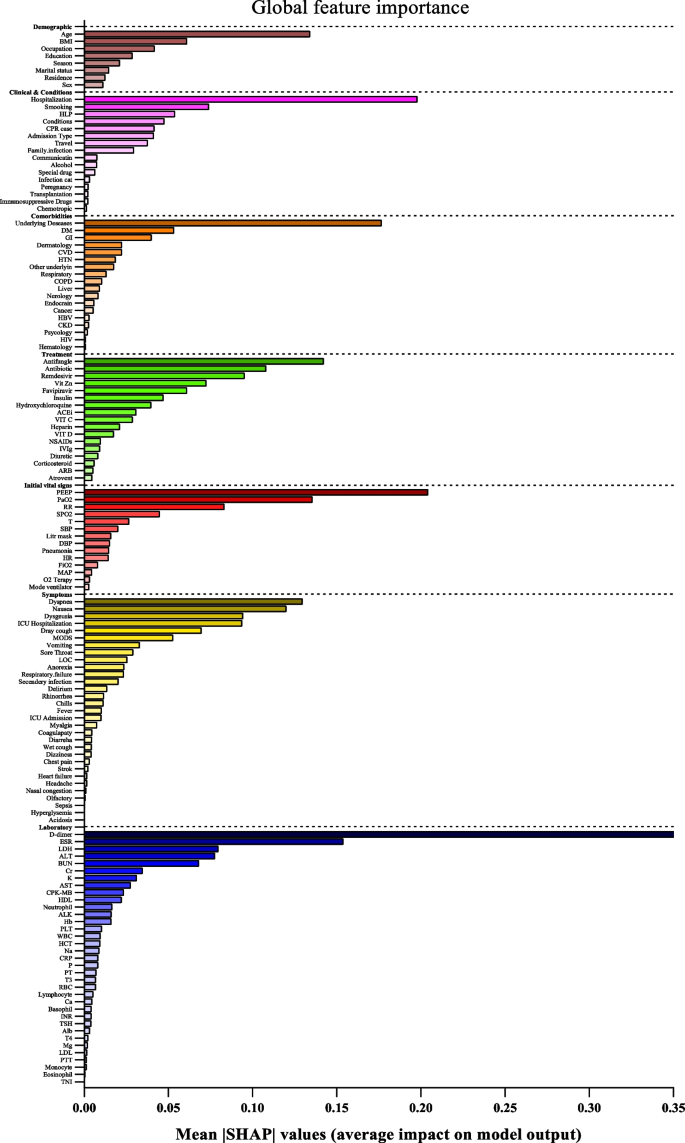
Feature importance based on SHAP-values. The mean absolute SHAP values are depicted, to illustrate global feature importance. The SHAP values change in the spectrum from dark (higher) to light (lower) color
On the other hand, Fig. 3 presents the local explanation summary that indicates the direction of the relationship between a variable and COVID-19 outcome. As shown in Fig. 3 (I to VII), older age and very low BMI were the two demographic factors with the greatest impact on model outcome, followed by clinical factors such as higher CPR, hospitalization, and hyperlipidemia. Higher mortality rates were associated with patients who smoked and had traveled in the past 14 days. Patients with underlying diseases, especially HTN, died more frequently. In contrast, the use of remdesivir, Vit Zn, and favipiravir is associated with lower mortality. Initial vital signs such as high PEEP, low PaO2 and RR had the greatest impact, as did symptoms such as dyspnea, MODS, sore throat and LOC. A higher risk of mortality is observed in patients with higher D-dimer levels and ESR as the most consequential laboratory tests, followed by K, AST and CPK-MB.
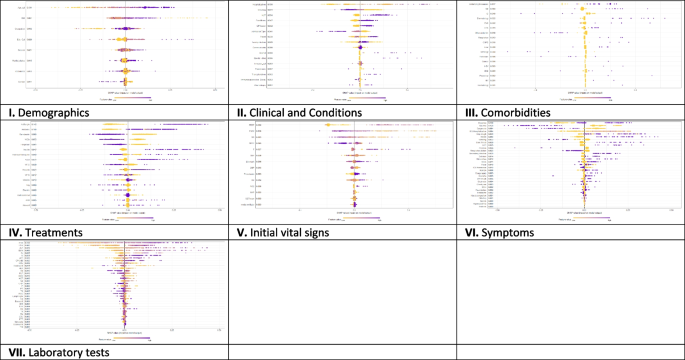
The SHAP-based feature importance of all categories (I to VII) for COVID‑19 mortality prediction, calculated with the XGBoost model. The local explanatory summary shows the direction of the relationship between a feature and patient outcome. Positive SHAP values indicate death, whereas negative SHAP values indicate survival. As the color scale shows, higher values are blue while lower values are orenge
Using the feature types listed in Appendix Table 1 , Fig. 4 shows that the performance of ML algorithms can be improved by increasing the number of features used in training, especially in distinguishing between symptoms, comorbidities, and treatments. In addition, the amount and quality of data used for training can significantly affect algorithm performance, with laboratory tests being more informative than initial vital signs. Regarding the influence of features, quantitative features tend to have a more positive effect on performance than qualitative features; clinical conditions tend to be more informative than demographic data. Thus, both the amount of data and the type of features used have a significant impact on the performance of ML algorithms.
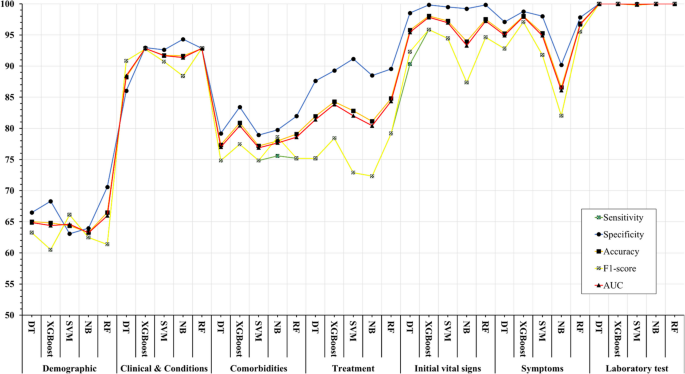
Association between feature sets and performance of machine learning algorithms in predicting COVID-19’s mortality
The COVID-19 pandemic has presented unprecedented public health challenges worldwide and requires a deep understanding of the factors contributing to COVID-19 mortality to enable effective management and intervention. This study used machine learning analysis to uncover the predictive power of an extensive dataset that includes wide range of personal, clinical, preclinical, and laboratory variables associated with COVID-19 mortality.
This study confirms previous research on COVID-19 outcomes that highlighted age as a significant predictor of mortality [ 45 , 46 , 47 ], along with comorbidities such as hypertension and diabetes [ 48 , 49 ]. Underlying conditions such as cardiovascular and renal disease also contribute to mortality risk [ 50 , 51 ].
Regarding treatment, antibiotics, remdesivir, favipiravir, and vitamin zinc are associated with lower mortality [ 52 , 53 ], whereas heparin, insulin, antifungals, ACE, and ARBs are associated with higher mortality [ 54 ]. This underscores the importance of drug choice in COVID -19 treatment.
Initial vital signs such as heart rate, respiratory rate, temperature, and oxygen therapy differ between surviving and deceased patients [ 55 ]. Deceased patients often have increased heart rate, lower respiratory rate, higher temperature, and increased oxygen requirements, which can serve as early indicators of disease severity.
Symptoms such as productive cough, dyspnea, and delirium are significantly associated with COVID-19 mortality, emphasizing the need for immediate monitoring and intervention [ 56 ]. Laboratory tests show altered hematologic and biochemical markers in deceased patients, underscoring the importance of routine laboratory monitoring in COVID-19 patients [ 57 , 58 ].
The ML algorithms were used in the study to predict mortality COVID-19 based on these multilayered variables. XGBoost and Random Forest performed better than other algorithms and had high recall, specificity, accuracy, F1 score, and AUC. This highlights the potential of ML, particularly the XGBoost algorithm, in improving prediction accuracy for COVID-19 mortality [ 59 ]. The study also highlighted the importance of drug choice in treatment and the potential of ML algorithms, particularly XGBoost, in improving prediction accuracy. However, the study's findings differ from those of Moulaei [ 60 ], Nopour [ 61 ], and Mehraeen [ 62 ] in terms of the best-performing ML algorithm and the most influential variables. While Moulaei [ 60 ] found that the random forest algorithm had the best performance, Nopour [ 61 ] and Ikemura [ 63 ] identified the artificial neural network and stacked ensemble models, respectively, as the most effective. Additionally, the most influential variables in predicting mortality varied across the studies, with Moulaei [ 60 ] highlighting dyspnea, ICU admission, and oxygen therapy, and Ikemura [ 63 ] identifying systolic and diastolic blood pressure, age, and other biomarkers. These differences may be attributed to variations in the datasets, feature selection, and model training.
However, it is important to note that the choice of algorithm should be tailored to the specific dataset and research question. In addition, the results suggest that a comprehensive approach that incorporates different feature categories may lead to more accurate prediction of COVID-19 mortality. In general, the results suggest that the performance of ML models is influenced by the number and type of features in each category. While some models consistently perform well across different categories (e.g., XGBoost), others perform better for specific types of features (e.g., SVM for Demographics).
Analysis of the importance of characteristics using SHAP values revealed critical factors affecting model results. D-dimer values, CPR, PEEP, underlying diseases, and ESR emerged as the most important features, highlighting the importance of these variables in predicting COVID-19 mortality. These results provide valuable insights into the underlying mechanisms and risk factors associated with severe COVID-19 outcomes.
The types of features used in ML models fall into two broad categories: quantitative (numerical) and qualitative (binary or categorical). The performance of ML methods can vary depending on the type of features used. Some algorithms work better with quantitative features, while others work better with qualitative features. For example, decision trees and random forests work well with both types of features [ 64 ], while neural networks often work better with quantitative features [ 65 , 66 ]. Accordingly, we consider these levels for the features under study to better assess the impact of the data.
The success of ML algorithms depends largely on the quality and quantity of the data on which they are trained [ 67 , 68 , 69 ]. Recent research, including the 2021 study by Sarker IH. [ 26 ], has shown that a larger amount of data can significantly improve the performance of deep learning algorithms compared to traditional machine learning techniques. However, it should be noted that the effect of data size on model performance depends on several factors, such as data characteristics and experimental design. This underscores the importance of carefully and judiciously selecting data for training.
Limitations
One of the limitations of this study is that it relies on data collected from a single hospital in Abadan, Iran. The data may not be representative of the diversity of COVID -19 cases in different regions, and there may be differences in data quality and completeness. In addition, retrospectively collected data may have biases and inaccuracies. Although the study included a substantial number of COVID -19 patients, the sample size may still limit the generalizability of the results, especially for less common subgroups or certain demographic characteristics.
Future works
Future studies could adopt a multi-center approach to improve the scope and depth of research on COVID-19 outcomes. This could include working with multiple hospitals in different regions of Iran to ensure a more diverse and representative sample. By conducting prospective studies, researchers can collect data in real time, which reduces the biases associated with retrospective data collection and increases the reliability of the results. Increasing sample size, conducting longitudinal studies to track patient progression, and implementing quality assurance measures are critical to improving generalizability, understanding long-term effects, and ensuring data accuracy in future research efforts. Collectively, these strategies aim to address the limitations of individual studies and make an important contribution to a more comprehensive understanding of COVID-19 outcomes in different populations and settings.
Conclusions
In summary, this study demonstrates the potential of ML algorithms in predicting COVID-19 mortality based on a comprehensive set of features. In addition, the interpretability of the models using SHAP-based feature importance, which revealed the variables strongly correlated with mortality. This study highlights the power of data-driven approaches in addressing critical public health challenges such as the COVID-19 pandemic. The results suggest that the performance of ML models is influenced by the number and type of features in each feature set. These findings may be a valuable resource for health professionals to identify high-risk patients COVID-19 and allocate resources effectively.
Availability of data and materials
The datasets used and/or analyzed during the current study are available from the corresponding author on reasonable request.
Abbreviations
World Health Organization
Middle east respiratory syndrome
Severe acute respiratory syndrome
Reverse transcription polymerase chain reaction
Propensity score matching
Synthetic minority over-sampling technique
Missing completely at random
Decision tree
EXtreme gradient boosting
Support vector machine
Naïve bayes
Random forest
Cross-validation
True positive
True negative
False positive
False negative
- Machine learning
Artificial Intelligence
Shapely additive explanation
Cardiopulmonary Resuscitation
Hypertension
Diabetes mellitus
Cardiovascular disease
Chronic Kidney disease
Chronic obstructive pulmonary disease
Human immunodeficiency virus
Hepatitis B virus
Such as influenza, pneumonia, asthma, bronchitis, and chronic obstructive airways disease
Gastrointestinal
Such as epilepsy, learning disabilities, neuromuscular disorders, autism, ADD, brain tumors, and cerebral palsy
Such as fatty liver disease and cirrhosis
Blood disease
Skin diseases
Mental disorders
Intravenous immunoglobulin
Non-steroidal anti-Inflammatory drugs
Angiotensin converting enzyme inhibitors
Angiotensin II receptor blockers
Beats per minute
Respiratory rate
Temperatures
Systolic blood pressure
Diastolic blood pressure
Mean arterial pressure
Oxygen saturation
Partial pressure of oxygen in the alveoli
Positive end-expiratory pressure
Fraction of inspired oxygen
Radiography (X-ray) test result
Smell disorders
Indigestion
Level of consciousness
Multiple organ dysfunction syndrome
Coughing up blood; Coagulopathy: bleeding disorder
High blood glucose
Intensive care unit
Red blood cell
White blood cell
Low-density lipoprotein
High-density lipoprotein
Prothrombin time
Partial thromboplastin time
International normalized ratio
Erythrocyte sedimentation rate
C-reactive-protein
Lactate dehydrogenase
Aspartate aminotransferase
Alanine aminotransferase
Alkaline phosphatase
Creatine phosphokinase-MB
Blood urea nitrogen
Thyroid stimulating hormone
Triiodothyronine
Coronavirus disease (COVID-19) pandemic. Available from: https://www.who.int/europe/emergencies/situations/covid-19 . [cited 2023 Sep 5].
Moolla I, Hiilamo H. Health system characteristics and COVID-19 performance in high-income countries. BMC Health Serv Res. 2023;23(1):1–14. https://doi.org/10.1186/s12913-023-09206-z . [cited 2023 Sep 5].
Article Google Scholar
Peeri NC, Shrestha N, Rahman MS, Zaki R, Tan Z, Bibi S, et al. The SARS, MERS and novel coronavirus (COVID-19) epidemics, the newest and biggest global health threats: what lessons have we learned? Int J Epidemiol. 2020;49(3):717–26.
Article PubMed Google Scholar
WHO Coronavirus (COVID-19) Dashboard | WHO Coronavirus (COVID-19) Dashboard With Vaccination Data. Available from: https://covid19.who.int/ . [cited 2023 Sep 5].
Dessie ZG, Zewotir T. Mortality-related risk factors of COVID-19: a systematic review and meta-analysis of 42 studies and 423,117 patients. BMC Infect Dis. 2021;21(1):1–28. https://doi.org/10.1186/s12879-021-06536-3 . [cited 2023 Sep 5].
Article CAS Google Scholar
Wong ELY, Ho KF, Wong SYS, Cheung AWL, Yau PSY, Dong D, et al. Views on Workplace Policies and its Impact on Health-Related Quality of Life During Coronavirus Disease (COVID-19) Pandemic: Cross-Sectional Survey of Employees. Int J Heal Policy Manag. 2022;11(3):344–53. Available from: https://www.ijhpm.com/article_3879.html .
Google Scholar
Drefahl S, Wallace M, Mussino E, Aradhya S, Kolk M, Brandén M, et al. A population-based cohort study of socio-demographic risk factors for COVID-19 deaths in Sweden. Nat Commun. 2020;11(1):5097.
Article CAS PubMed PubMed Central Google Scholar
Islam N, Khunti K, Dambha-Miller H, Kawachi I, Marmot M. COVID-19 mortality: a complex interplay of sex, gender and ethnicity. Eur J Public Health. 2020;30(5):847–8.
Sarmadi M, Marufi N, Moghaddam VK. Association of COVID-19 global distribution and environmental and demographic factors: An updated three-month study. Environ Res. 2020;188:109748.
Aghazadeh-Attari J, Mohebbi I, Mansorian B, Ahmadzadeh J, Mirza-Aghazadeh-Attari M, Mobaraki K, et al. Epidemiological factors and worldwide pattern of Middle East respiratory syndrome coronavirus from 2013 to 2016. Int J Gen Med. 2018;11:121–5.
Risk of COVID-19-Related Mortality. Available from: https://www.cdc.gov/coronavirus/2019-ncov/science/data-review/risk.html . [cited 2023 Aug 26].
Bhaskaran K, Bacon S, Evans SJW, Bates CJ, Rentsch CT, MacKenna B, et al. Factors associated with deaths due to COVID-19 versus other causes: population-based cohort analysis of UK primary care data and linked national death registrations within the OpenSAFELY platform. Lancet Reg Heal. 2021;6:100-9.
Dessie ZG, Zewotir T. Mortality-related risk factors of COVID-19: a systematic review and meta-analysis of 42 studies and 423,117 patients. BMC Infect Dis. 2021;21(1):855. https://doi.org/10.1186/s12879-021-06536-3 .
Talebi SS, Hosseinzadeh A, Zare F, Daliri S, JamaliAtergeleh H, Khosravi A, et al. Risk Factors Associated with Mortality in COVID-19 Patient’s: Survival Analysis. Iran J Public Health. 2022;51(3):652–8.
PubMed PubMed Central Google Scholar
Singh J, Alam A, Samal J, Maeurer M, Ehtesham NZ, Chakaya J, et al. Role of multiple factors likely contributing to severity-mortality of COVID-19. Infect Genet Evol J Mol Epidemiol Evol Genet Infect Dis. 2021;96:105101.
CAS Google Scholar
Bhaskaran K, Bacon S, Evans SJ, Bates CJ, Rentsch CT, MacKenna B, et al. Factors associated with deaths due to COVID-19 versus other causes: population-based cohort analysis of UK primary care data and linked national death registrations within the OpenSAFELY platform. Lancet Reg Heal - Eur. 2021;6:100109. Available from: https://www.pmc/articles/PMC8106239/ . [cited 2023 Aug 26].
Ge E, Li Y, Wu S, Candido E, Wei X. Association of pre-existing comorbidities with mortality and disease severity among 167,500 individuals with COVID-19 in Canada: A population-based cohort study. PLoS One. 2021;16(10):e0258154. https://journals.plos.org/plosone/article?id=10.1371/journal.pone.0258154 . [cited 2023 Aug 26].
Tian S, Liu H, Liao M, Wu Y, Yang C, Cai Y, et al. Analysis of mortality in patients with COVID-19: clinical and laboratory parameters. Open Forum Infect Dis. 2020;7(5). Available from: https://dx.doi.org/10.1093/ofid/ofaa152 . [cited 2023 Aug 26].
Rashidi HH, Tran N, Albahra S, Dang LT. Machine learning in health care and laboratory medicine: General overview of supervised learning and Auto-ML. Int J Lab Hematol. 2021;43:15–22.
Najafi-Vosough R, Faradmal J, Hosseini SK, Moghimbeigi A, Mahjub H. Predicting hospital readmission in heart failure patients in Iran: a comparison of various machine learning methods. Healthc Inform Res. 2021;27(4):307–14.
Article PubMed PubMed Central Google Scholar
Alanazi A. Using machine learning for healthcare challenges and opportunities. Informatics Med Unlocked. 2022;100924:1–5.
Chadaga K, Prabhu S, Sampathila N, Chadaga R, Umakanth S, Bhat D, et al. Explainable artificial intelligence approaches for COVID-19 prognosis prediction using clinical markers. Sci Rep. 2024;14(1):1783.
Chadaga K, Prabhu S, Bhat V, Sampathila N, Umakanth S, Chadaga R, et al. An explainable multi-class decision support framework to predict COVID-19 prognosis utilizing biomarkers. Cogent Eng. 2023;10(2):2272361.
Khanna VV, Chadaga K, Sampathila N, Prabhu S, Chadaga R. A machine learning and explainable artificial intelligence triage-prediction system for COVID-19. Decis Anal J. 2023;100246:1–14.
Zoabi Y, Deri-Rozov S, Shomron N. Machine learning-based prediction of COVID-19 diagnosis based on symptoms. npj Digit Med. 2021;4(1):1–5.
IH Sarker 2021 Machine Learning: Algorithms, Real-World Applications and Research Directions SN Comput Sci. 2 3 160 Available from: https://doi.org/10.1007/s42979-021-00592-x .
Jones JA, Farnell B. Missing and Incomplete Data Reduces the Value of General Practice Electronic Medical Records as Data Sources in Research. Aust J Prim Health. 2007;13(1):74–80. Available from: https://www.publish.csiro.au/py/py07010 . [cited 2023 Dec 16].
Austin PC. An Introduction to Propensity Score Methods for Reducing the Effects of Confounding in Observational Studies. Multivariate Behav Res. 2011;46(3):399–424.
Torjusen H, Lieblein G, Næs T, Haugen M, Meltzer HM, Brantsæter AL. Food patterns and dietary quality associated with organic food consumption during pregnancy; Data from a large cohort of pregnant women in Norway. BMC Public Health. 2012;12(1):1–11.
Little RJA. A test of missing completely at random for multivariate data with missing values. J Am Stat Assoc. 1988;83(404):1198–202.
Tenny S, Kerndt CC, Hoffman MR. Case Control Studies. Encycl Pharm Pract Clin Pharm Vol 1-3 [Internet]. 2023;1–3:V2-356-V2-366. [cited 2024 Apr 14] Available from: https://www.ncbi.nlm.nih.gov/books/NBK448143/ .
Stanfill B, Reehl S, Bramer L, Nakayasu ES, Rich SS, Metz TO, et al. Extending Classification Algorithms to Case-Control Studies. Biomed Eng Comput Biol. 2019;10:117959721985895. Available from: https://www.pmc/articles/PMC6630079/ .[cited 2023 Sep 3].
Mulugeta G, Zewotir T, Tegegne AS, Juhar LH, Muleta MB. Classification of imbalanced data using machine learning algorithms to predict the risk of renal graft failures in Ethiopia. BMC Med Inform Decis Mak. 2023;23(1):1–17. https://bmcmedinformdecismak.biomedcentral.com/articles/10.1186/s12911-023-02185-5 . [cited 2023 Sep 3].
Sadeghi S, Khalili D, Ramezankhani A, Mansournia MA, Parsaeian M. Diabetes mellitus risk prediction in the presence of class imbalance using flexible machine learning methods. BMC Med Inform Decis Mak. 2022;22(1):36. https://doi.org/10.1186/s12911-022-01775-z .
Zhou W, Nielsen JB, Fritsche LG, Dey R, Gabrielsen ME, Wolford BN, et al. Efficiently controlling for case-control imbalance and sample relatedness in large-scale genetic association studies. Nat Genet. 2018;50(9):1335. Available from: https://www.pmc/articles/PMC6119127/ . [cited 2023 Sep 3].
Miao J, Niu L. A Survey on Feature Selection. Procedia Comput Sci. 2016;91(1):919–26.
Remeseiro B, Bolon-Canedo V. A review of feature selection methods in medical applications. Comput Biol Med. 2019;112:103375.
Article CAS PubMed Google Scholar
R Studio Team. A language and environment for statistical computing. R Found Stat Comput. 2021;1.
Training Sets, Test Sets, and 10-fold Cross-validation - KDnuggets. Available from: https://www.kdnuggets.com/2018/01/training-test-sets-cross-validation.html . [cited 2023 Sep 4].
Hossin M, Sulaiman MN. A review on evaluation metrics for data classification evaluations. Int J data Min Knowl Manag Process. 2015;5(2):1.
Seyedtabib M, Kamyari N. Predicting polypharmacy in half a million adults in the Iranian population: comparison of machine learning algorithms. BMC Med Inform Decis Mak. 2023;23(1):84. https://doi.org/10.1186/s12911-023-02177-5 .
Lundberg SM, Lee S-I. A unified approach to interpreting model predictions. Adv Neural Inf Process Syst. 2017;30:4765–74.
Greenwell B. Fastshap: Fast approximate shapley values. Man R Packag v0 05. 2020;9–12. https://www.CRANR-projectorg/package=fastshap . Last accessed.
Aas K, Jullum M, Løland A. Explaining individual predictions when features are dependent: More accurate approximations to Shapley values. Artif Intell. 2021;298:103502.
Mesas AE, Cavero-Redondo I, Álvarez-Bueno C, Sarriá Cabrera MA, de Maffei Andrade S, Sequí-Dominguez I, et al. Predictors of in-hospital COVID-19 mortality: A comprehensive systematic review and meta-analysis exploring differences by age, sex and health conditions. PLoS One. 2020;15(11):e0241742.
Yanez ND, Weiss NS, Romand J-A, Treggiari MM. COVID-19 mortality risk for older men and women. BMC Public Health. 2020;20(1):1–7.
Sasson I. Age and COVID-19 mortality. Demogr Res. 2021;44:379–96.
Huang I, Lim MA, Pranata R. Diabetes mellitus is associated with increased mortality and severity of disease in COVID-19 pneumonia–a systematic review, meta-analysis, and meta-regression. Diabetes Metab Syndr Clin Res Rev. 2020;14(4):395–403.
Albitar O, Ballouze R, Ooi JP, Ghadzi SMS. Risk factors for mortality among COVID-19 patients. Diabetes Res Clin Pract. 2020;166:108293.
Di Castelnuovo A, Bonaccio M, Costanzo S, Gialluisi A, Antinori A, Berselli N, et al. Common cardiovascular risk factors and in-hospital mortality in 3,894 patients with COVID-19: survival analysis and machine learning-based findings from the multicentre Italian CORIST Study. Nutr Metab Cardiovasc Dis. 2020;30(11):1899–913.
Ssentongo P, Ssentongo AE, Heilbrunn ES, Ba DM, Chinchilli VM. Association of cardiovascular disease and 10 other pre-existing comorbidities with COVID-19 mortality: A systematic review and meta-analysis. PLoS ONE. 2020;15(8):e0238215.
Beran A, Mhanna M, Srour O, Ayesh H, Stewart JM, Hjouj M, et al. Clinical significance of micronutrient supplements in patients with coronavirus disease 2019: A comprehensive systematic review and meta-analysis. Clin Nutr ESPEN. 2022;48:167–77.
Perveen RA, Nasir M, Murshed M, Nazneen R, Ahmad SN. Remdesivir and favipiravir changes hepato-renal profile in COVID-19 patients: a cross sectional observation in Bangladesh. Int J Med Sci Clin Inven. 2021;8(1):5196–201.
El-Arif G, Khazaal S, Farhat A, Harb J, Annweiler C, Wu Y, et al. Angiotensin II Type I Receptor (AT1R): the gate towards COVID-19-associated diseases. Molecules. 2022;27(7):2048.
Ikram AS, Pillay S. Admission vital signs as predictors of COVID-19 mortality: a retrospective cross-sectional study. BMC Emerg Med. 2022;22(1):1–10.
Martí-Pastor A, Moreno-Perez O, Lobato-Martínez E, Valero-Sempere F, Amo-Lozano A, Martínez-García M-Á, et al. Association between Clinical Frailty Scale (CFS) and clinical presentation and outcomes in older inpatients with COVID-19. BMC Geriatr. 2023;23(1):1.
Lippi G, Plebani M. Laboratory abnormalities in patients with COVID-2019 infection. Clin Chem Lab Med. 2020;58(7):1131–4.
Naghashpour M, Ghiassian H, Mobarak S, Adelipour M, Piri M, Seyedtabib M, et al. Profiling serum levels of glutathione reductase and interleukin-10 in positive and negative-PCR COVID-19 outpatients: A comparative study from southwestern Iran. J Med Virol. 2022;94(4):1457–64.
Sharifi-Kia A, Nahvijou A, Sheikhtaheri A. Machine learning-based mortality prediction models for smoker COVID-19 patients. BMC Med Inform Decis Mak. 2023;23(1):1–15.
Moulaei K, Shanbehzadeh M, Mohammadi-Taghiabad Z, Kazemi-Arpanahi H. Comparing machine learning algorithms for predicting COVID-19 mortality. BMC Med Inform Decis Mak. 2022;22(1):2. https://doi.org/10.1186/s12911-021-01742-0 .
Nopour R, Erfannia L, Mehrabi N, Mashoufi M, Mahdavi A, Shanbehzadeh M. Comparison of Two Statistical Models for Predicting Mortality in COVID-19 Patients in Iran. Shiraz E-Medical J 2022 236 [Internet]. 2022;23(6):119172. [cited 2024 Apr 14] Available from: https://brieflands.com/articles/semj-119172 .
Mehraeen E, Karimi A, Barzegary A, Vahedi F, Afsahi AM, Dadras O, et al. Predictors of mortality in patients with COVID-19–a systematic review. Eur J Integr Med. 2020;40:101226.
Ikemura K, Bellin E, Yagi Y, Billett H, Saada M, Simone K, et al. Using Automated Machine Learning to Predict the Mortality of Patients With COVID-19: Prediction Model Development Study. J Med Internet Res [Internet]. 2021;23(2):e23458. Available from: https://www.jmir.org/2021/2/e23458 .
Breiman L. Random forests. Mach Learn. 2001;45:5–32.
Hinton G, Srivastava N, Swersky K. Neural networks for machine learning lecture 6a overview of mini-batch gradient descent. Cited on. 2012;14(8):2.
Zheng A, Casari A. Feature Engineering for Machine Learning: Principles and Techniques for Data Scientists. O’Reilly [Internet]. 2018;218. [cited 2024 Apr 14] Available from: https://www.amazon.com/Feature-Engineering-Machine-Learning-Principles/dp/1491953241 .
Adamson AS, Smith A. Machine Learning and Health Care Disparities in Dermatology. JAMA Dermatology. 2018;154(11):1247–8. Available from: https://jamanetwork.com/journals/jamadermatology/fullarticle/2688587 . [cited 2023 Sep 15].
Kavakiotis I, Tsave O, Salifoglou A, Maglaveras N, Vlahavas I, Chouvarda I. Machine Learning and Data Mining Methods in Diabetes Research. Comput Struct Biotechnol J. 2017;1(15):104–16.
Schmidt J, Marques MRG, Botti S, Marques MAL. Recent advances and applications of machine learning in solid-state materials science. Comput Mater. 2019;5(1):83. https://doi.org/10.1038/s41524-019-0221-0 .
Download references
Acknowledgements
We thank the Research Deputy of the Abadan University of Medical Sciences for financially supporting this project.
Summary points
∙ How can datasets improve mortality prediction using ML models for COVID-19 patients?
∙ In order, quantity and quality variables have more effect on the model performances.
∙ Intelligent techniques such as SHAP analysis can be used to improve the interpretability of features in ML algorithms.
∙ Well-structured data are critical to help health professionals identify at-risk patients and improve pandemic outcomes.
This research was supported by grant No. 1456 from the Abadan University of Medical Sciences. However, the funding source did not influence the study design, data collection, analysis and interpretation, report writing, or decision to publish the article.
Author information
Authors and affiliations.
Department of Biostatistics and Epidemiology, School of Health, Ahvaz Jundishapur University of Medical Sciences, Ahvaz, Iran
Maryam Seyedtabib
Research Center for Health Sciences, Hamadan University of Medical Sciences, Hamadan, Iran
Roya Najafi-Vosough
Department of Biostatistics and Epidemiology, School of Health, Abadan University of Medical Sciences, Abadan, Iran
Naser Kamyari
You can also search for this author in PubMed Google Scholar
Contributions
MS: Conceptualization, Methodology, Validation, Formal analysis, Investigation, Resources, Data curation, Writing–original draft, writing—review & editing, Visualization, Project administration. RNV: Conceptualization, Data curation, Formal analysis, Investigation, Writing–original draft, writing—review & editing. NK: Conceptualization, Methodology, Software, Validation, Formal analysis, Investigation, Resources, Data curation, Writing–original draft, writing—review & editing, Visualization, Supervision.
Corresponding author
Correspondence to Naser Kamyari .
Ethics declarations
Ethics approval and consent to participate.
This study was approved by the Research Ethics Committee (REC) of Abadan University of Medical Sciences under the ID number IR.ABADANUMS.REC.1401.095. Methods used complied with all relevant ethical guidelines and regulations. The Ethics Committee of Abadan University of Medical Sciences waived the requirement for written informed consent from study participants.
Competing interests
The authors declare no competing interests.
Additional information
Publisher’s note.
Springer Nature remains neutral with regard to jurisdictional claims in published maps and institutional affiliations.
Supplementary Information
Supplementary material 1., rights and permissions.
Open Access This article is licensed under a Creative Commons Attribution 4.0 International License, which permits use, sharing, adaptation, distribution and reproduction in any medium or format, as long as you give appropriate credit to the original author(s) and the source, provide a link to the Creative Commons licence, and indicate if changes were made. The images or other third party material in this article are included in the article's Creative Commons licence, unless indicated otherwise in a credit line to the material. If material is not included in the article's Creative Commons licence and your intended use is not permitted by statutory regulation or exceeds the permitted use, you will need to obtain permission directly from the copyright holder. To view a copy of this licence, visit http://creativecommons.org/licenses/by/4.0/ . The Creative Commons Public Domain Dedication waiver ( http://creativecommons.org/publicdomain/zero/1.0/ ) applies to the data made available in this article, unless otherwise stated in a credit line to the data.
Reprints and permissions
About this article
Cite this article.
Seyedtabib, M., Najafi-Vosough, R. & Kamyari, N. The predictive power of data: machine learning analysis for Covid-19 mortality based on personal, clinical, preclinical, and laboratory variables in a case–control study. BMC Infect Dis 24 , 411 (2024). https://doi.org/10.1186/s12879-024-09298-w
Download citation
Received : 22 December 2023
Accepted : 05 April 2024
Published : 18 April 2024
DOI : https://doi.org/10.1186/s12879-024-09298-w
Share this article
Anyone you share the following link with will be able to read this content:
Sorry, a shareable link is not currently available for this article.
Provided by the Springer Nature SharedIt content-sharing initiative
- Predictive model
- Coronavirus disease
- Data quality
- Performance
BMC Infectious Diseases
ISSN: 1471-2334
- Submission enquiries: [email protected]
- General enquiries: [email protected]
- Open access
- Published: 16 April 2024
Implementation of an antimicrobial stewardship program for urinary tract infections in long-term care facilities: a cluster-controlled intervention study
- Elisabeth König 1 na1 ,
- Lisa Kriegl 1 na1 ,
- Christian Pux 2 ,
- Michael Uhlmann 2 ,
- Walter Schippinger 2 ,
- Alexander Avian 3 ,
- Robert Krause 1 &
- Ines Zollner-Schwetz 1
Antimicrobial Resistance & Infection Control volume 13 , Article number: 43 ( 2024 ) Cite this article
11 Accesses
1 Altmetric
Metrics details
Widespread inappropriate use of antimicrobial substances drives resistance development worldwide. In long-term care facilities (LTCF), antibiotics are among the most frequently prescribed medications. More than one third of antimicrobial agents prescribed in LTCFs are for urinary tract infections (UTI). We aimed to increase the number of appropriate antimicrobial treatments for UTIs in LTCFs using a multi-faceted antimicrobial stewardship intervention.
We performed a non-randomized cluster-controlled intervention study. Four LTCFs of the Geriatric Health Centers Graz were the intervention group, four LTCFs served as control group. The main components of the intervention were: voluntary continuing medical education for primary care physicians, distribution of a written guideline, implementation of the project homepage to distribute guidelines and videos and onsite training for nursing staff. Local nursing staff recorded data on UTI episodes in an online case report platform. Two blinded reviewers assessed whether treatments were adequate.
326 UTI episodes were recorded, 161 in the intervention group and 165 in the control group. During the intervention period, risk ratio for inadequate indication for treatment was 0.41 (95% CI 0.19–0.90), p = 0.025. In theintervention group, the proportion of adequate antibiotic choices increased from 42.1% in the pre-intervention period, to 45.9% during the intervention and to 51% in the post-intervention period (absolute increase of 8.9%). In the control group, the proportion was 36.4%, 33.3% and 33.3%, respectively. The numerical difference between intervention group and control group in the post-intervention period was 17.7% (difference did not reach statistical significance). There were no significant differences between the control group and intervention group in the safety outcomes (proportion of clinical failure, number of hospital admissions due to UTI and adverse events due to antimicrobial treatment).
Conclusions
An antimicrobial stewardship program consisting of practice guidelines, local and web-based education for nursing staff and general practitioners resulted in a significant increase in adequate treatments (in terms of decision to treat the UTI) during the intervention period. However, this difference was not maintained in the post-intervention phase. Continued efforts to improve the quality of prescriptions further are necessary.
Trial registration
The trial was registered at ClinicalTrials.gov NCT04798365.
Introduction
Antimicrobial resistance is a major threat to human health. The widespread inappropriate use of antimicrobial substances drives resistance development at the individual and population level [ 1 , 2 ]. Infections due to resistant pathogens are responsible for a high healthcare burden and are estimated to cause over 700.000 deaths annually worldwide– a rate that is projected to rise to 10 million by 2050 [ 3 ].
Approximately 2–5% of the population of high-income countries resides in some type of long-term care facilities (LTCFs) [ 4 ]. These residents are at increased risk for nosocomial and healthcare-associated infections mostly due to age-related factors such as immunosenescene, decline in functional status, chronic comorbidities and the use of invasive medical devices [ 5 , 6 ]. Infections at LTCFs are a common cause for residents’ mortality and morbidity associated with a significant socio-economic burden [ 7 ].
Antibiotics are one of the most frequently prescribed medications in LTCFs [ 8 , 9 ]. In a multinational European point-prevalence study, 4.9% of residents received at least one antimicrobial substance on the study day [ 10 ]. Over 30% of antibiotics prescribed in LTCFs are for urinary tract infections (UTIs) [ 11 ]. Studies have shown that 30–43% of antibiotic courses prescribed in LTCFs were unnecessary [ 12 , 13 , 14 ]. In addition, up to 72% of patients with UTIs in LTCFs were shown to be treated with inappropriate antimicrobial drugs based on society guidelines [ 13 ]. Antimicrobial stewardship programs targeting prescriptions for residents of LTCFs can therefore be a valuable contribution in the strive to curb antimicrobial resistance [ 15 ].
The aim of our study was to increase the number of appropriate antimicrobial treatment courses prescribed for UTIs in LTCFs using a multi-faceted antimicrobial stewardship intervention.
Materials and methods
Non-randomized cluster-controlled intervention study.
The Geriatric Health Centers Graz are a local institution comprising among others four LTCFs (total of 400 beds). General practitioners who are located off-site are in charge of medical treatments (approximately ten general practitioners per LTCF). These LTCFs were the intervention group. Four LTCFs located in the surroundings of Graz served as control group. Randomisation of LTCFs was not feasible because several general practitioners care for patients in more than one LTCF in Graz. We therefore chose LTCFs in another region as control group to avoid spillover of the intervention. The study was conducted from January 2021 to June 2022.
Interventions
A multifaceted educational intervention targeting nursing staff as well as physicians was initiated (“Urinary tract infection program”) targeting the following key-points:
obtain urine specimen in symptomatic residents when criteria for urine culture have been met (Supplement Table 1 ).
obtain urine specimen using proper techniques to avoid contamination.
change indwelling urinary catheters before obtaining urine specimen.
prescribe antibiotics only when clinical criteria have been met (Supplement Table 2 ).
prescribe antibiotics according to guideline (Supplement 3).
Main components of the intervention:
two online sessions of voluntary continuing medical education on the UTI program for treating general practitioners.
two online meetings with team leaders of nursing staff of all LTCFs discussing the UTI program.
interactive educational session with all nursing staff onsite at each of the LTCFs led by the principal investigator during the intervention period including information on the aims of the project, on how to recognize UTIs in the elderly and indications for urinary cultures according to the UTI guideline (see supplement).
onsite trainings on infection control for all nursing staff led by ICP team including information on UTI prevention strategies, on how to recognize UTIs in the elderly and correct techniques for collection of urine specimens.
educational materials: handouts during educational sessions, written guideline on antibiotic prescribing (supplement 3), videos about different aspects of the UTI program available on the project homepage.
project homepage used as platform to distribute the guideline (supplement 3) and educational videos.
Data collection
Baseline data on participating LTCFs were obtained: number of beds and ownership (municipal, private). For data on prescriptions, the nurse responsible was requested to fill in an online case report form (CRF) for all patients with infectious symptoms suggestive of UTI requiring a physician’s opinion. Recorded information included age, sex, indwelling urinary catheter, signs and symptoms suggestive of UTI, duration of symptoms, performance of urinary culture, type of antibiotic treatment, treatment length and dosing, referral to hospital, history of hypersensitivity to antimicrobial substances, pre-existing diagnosis of renal impairment, pre-existing urologic diagnosis, method of communication with the treating physician. The study was divided into a pre-intervention, an intervention and a post-intervention phase (for specific dates see supplement Table 3 ).
To assess the appropriateness of prescriptions in terms of antimicrobial choice and in terms of decision to treat, two independent blinded infectious disease specialists (LK, EK) reviewed each prescription at the end of the data collection period. Data on residents and UTI episodes were provided anonymously. Each physician reviewed cases separately based on clinical criteria for UTI, prescription guidelines provided (supplement Table 2 , supplement 3) and on published criteria for initiating antibiotics. Discrepancies were resolved in discussion.
The primary outcome was the proportion of adequate prescriptions (adequate in terms of antimicrobial choice and dosage; termed “adequate antibiotic choice”).
Secondary outcomes were:
proportion of adequate prescriptions (adequate in terms of decision to treat; termed “adequate indication for treatment”).
proportion of quinolones used for UTI without indwelling urinary catheter.
proportion of urinary cultures performed.
proportion of cases with clinical failure (defined as need for additional antimicrobial treatment for UTI within 7 days of previous episode).
proportion of admissions to hospital due to UTI.
proportion of adverse events attributed to antimicrobial treatment for UTI.
The last three outcomes were intended as safety outcomes.
The proportion of nursing and medical staff who underwent training was recorded.
Statistical analysis
To investigate the primary hypothesis whether the frequency of adequate prescriptions are different in both groups generalized linear models (probability distribution: binomial; link function: log) were used to estimate adjusted risk ratios (RR) with 95%CI. The unit of analysis was the patient, which were nested within centres. Two reviewers evaluated the adequacy of prescription. Inter-reviewer consistency was evaluated comparing the evaluation of both reviewers. Therefore Cohen’s ĸ and 95% confidence intervals of Cohen’s ĸ were calculated.
Three study periods (pre-intervention period, intervention-period, post-intervention period) were analysed separately.
Secondary outcomes (adequate indication for treatment, quinolone use for UTI without catheter, urinary culture performed, clinical failure, hospital admission due to UTI) were analysed in the same way. Similar to the primary outcome adequate decision to treat was evaluated by two reviewers.
Data including demographic and baseline characteristics were compared using χ²-test or Fisher’s-exact-test for categorical variables and t-test or Mann-Whitney-U-test for continuous variables depending on if the data were normally distributed or skewed. A P-value < 0.05 was considered statistically significant. Statistical analyses were performed using SAS 9.4 (2002–2012 by SAS Institute Inc., Cary, NC, USA).
The characteristics of LTCFs of both groups are summarized in Table 1 .
During the study, 326 UTI episodes were recorded, 161 in the intervention group and 165 in the control group. In the pre-intervention phase, we recorded 71 UTI episodes. During the intervention and post-intervention period, 167 and 88 episodes were documented, respectively. In the control group, 55/165 (33%) of UTIs were recorded in patients with an indwelling urinary catheter compared to 22/161 (14%) in the intervention group ( p < 0.004). There was a statistically significant difference in the method of communication between LTCF and physicians. In the intervention group, the physician was present at the LTCF in 26/161 (16%) of cases of UTI compared to 72/165 (44%) in the control group. In contrast, physicians were contacted by phone or fax 135/165 (84%) in the intervention group and in 93/165 (56%) in the control group ( p < 0.001).
Patient characteristics
UTIs were diagnosed in 194 patients. There were no significant differences between the study populations, i.e. patients with UTIs, in terms of age, sex, history of allergies to antimicrobial substances, history of renal impairment or urologic diseases (Table 2 ). There was a statistically significant difference in reported weight (control group median 66 kg (range 38–135 kg) vs. intervention group median 59 kg (range 38–143 kg), p = 0.014).
Intervention reach
During the intervention period (April 12, 2021– November 3, 2021), 30 onsite trainings were conducted by ICP team. Overall, 209 nursing staff members took part in these trainings. On October 31, 2021, the number of nursing staff employed by the four LTCFs of the intervention group was 205. The discrepancy between team members who took part in the trainings and the number of employees as of October 31, 2021 can be explained by staff turnover. All physicians received written information about the project during their visits at the LTCFs including the guideline and information on the website at five time points throughout the study. They were invited to the online educational sessions on the UTI program. Half of the physicians (15/ 30) attended at least one of the online sessions.
Primary outcome
Inter-reviewer consistency was high for the primary outcome (ĸ = 0.98; 95%CI 0.96-1.00). Out of 326 UTI episodes 323 (99.1%) were evaluated the same. Only in three UTI episodes discordant evaluations were observed. Due to the high level of agreement between the two reviewers, the two evaluations were combined for analysis. Therefore, adequate prescription was defined as follows: both reviewers evaluated the prescription adequate.
In the intervention group, the proportion of adequate antibiotic choices increased from 42.1% in the pre-intervention period, to 45.9% during the intervention and to 51% in the post-intervention period (absolute increase of 8.9%). In the control group, the proportion was 36.4%, 33.3% and 33.3%, respectively (Fig. 1 ). Therefore, the numerical difference between intervention group and control group in the post-intervention period was 17.7%. However, the differences between intervention group and control group did not reach statistical significance (Tables 3 and 4 ).
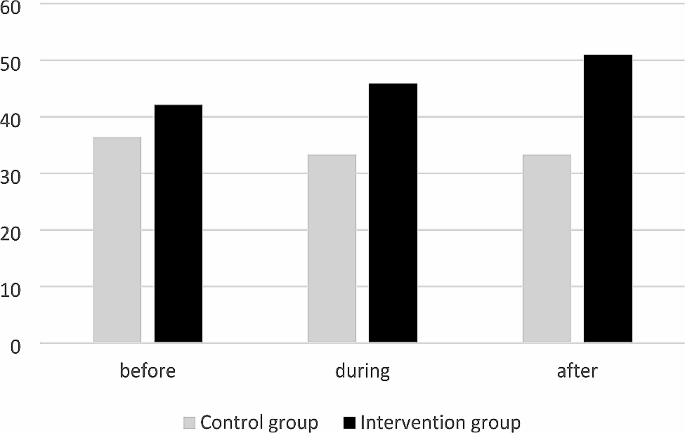
Proportion of adequate antimicrobial treatments (adequate in terms of choice) before, during and after interventions
Secondary outcomes
Because agreement in secondary outcomes was also high (ĸ = 0.97; 95%CI 0.93-1.00; concordant evaluations: 99.1%) the two evaluations were combined for analysis in the same way as for the primary outcome. During the intervention period, the risk ratio (RR) for inadequate indication for treatment was 0.41 (95% CI 0.19–0.90), p = 0.025 (Fig. 2 ; Table 3 ). In the post-intervention period, there was no difference between intervention group and control group, due to an increase in adequate indications for treatment in the control group (from 72.0% in the intervention period to 89.7% in the post-intervention period).
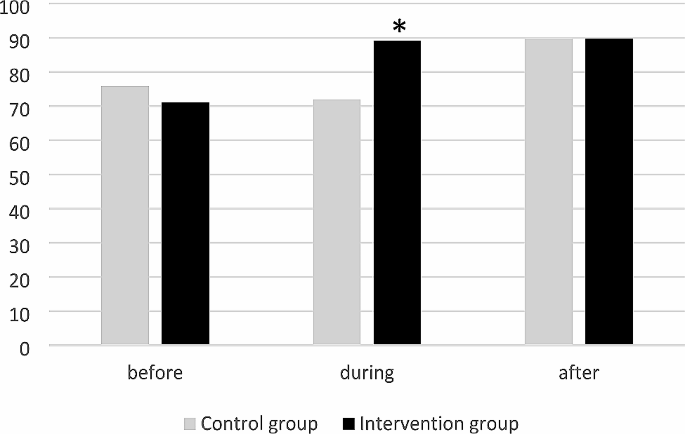
Proportion of adequate antimicrobial treatments (adequate decision to treat) before, during and after the interventions. * p < 0.05 comparing intervention and control group during the intervention period
During the study, 227 (69.6%) UTI episodes without an indwelling urinary catheter were recorded, 133 in the intervention group and 94 in the control group. Before and after the intervention period, the risk ratio (RR) for use of quinolones for UTI without indwelling urinary catheters were significantly reduced (RR: 0.17, 95%CI: 0.04–0.72; and 0.18; 95%CI: 0.04–0.89, respectively. Tables 3 and 4 ).
During the study period, 28 (8.6%) urinary cultures were performed (326 UTI episodes recorded). Throughout the study, more urinary cultures were ordered in the IG compared to control group. This difference was statistically significant during the intervention period (RR 6.89, 95%CI 1.59–29.83, p = 0.010, Table 3 ). In the post-intervention period, a statistical analysis was not possible as no cultures were performed in the control group (compared to 7 in the intervention group).
Our safety outcomes were: proportion of clinical failure (defined as need for additional antimicrobial treatment for UTI within 7 days of previous episode), number of hospital admissions due to UTI and adverse events due to antimicrobial treatment. There were no significant differences between the control group and intervention group in the safety outcomes (Tables 3 and 4 ). Only one adverse event due to antimicrobial treatment was documented during the entire study period (in the intervention group in the pre-intervention period).
In this non-randomized cluster-controlled intervention study in LTCFs in Austria, a multifaceted bundle of antimicrobial stewardship interventions consisting of clinical practice guidelines, local as well as web-based education targeting nursing staff and primary care physicians resulted in a significant increase in adequate indications for treatment during the intervention period. We found a higher number of adequate antibiotic choices for UTIs with a numerical difference between the intervention group and control group of 17.7% in the post-intervention phase. However, this difference did not reach statistical significance. The interventions did not lead to an increase in proportion of clinical failures, hospital admissions due to UTI or adverse events due to antimicrobial treatment.
During the intervention period, the risk ratio for inadequate treatment (in terms of decision to treat the UTI) was 0.41 ( p = 0.025), indicating that the interventions led to a change in prescription culture. The proportion of adequate indications for treatment remained high (89%) in the intervention group in the post-intervention phase, suggesting an effect also during this phase. However, there was no significant difference between intervention group and control group in the post-intervention period, due to an increase in adequate indications for treatment in the control group (from 72.0% in the intervention period to 89.7% in the post-intervention period). The reasons for this increase in the control group remain unclear. Although the intervention was restricted to LTCFs in Graz and controls were located outside of the city, we cannot completely rule out a partial spill over of the intervention within the trans-regional physician community.
Quinolone use for treatment of UTIs without indwelling urinary catheter was significantly lower in the intervention group. However, this difference existed already before our interventions and persisted thereafter. This difference could reflect a different prescription culture between physicians serving at LTCFs in the intervention group and control group. In 2018, systematic surveillance of health-care associated infections was initiated in the LTCFs of the Geriatric Health Centers Graz (intervention group ) [ 16 ]. Antimicrobial prescriptions were also recorded. Implementation of this surveillance system could have contributed to physicians’ awareness on negative aspects of quinolone use in elderly patients. Several other aspects shed light on differences in daily practice between the LTCFs in the two groups. In the control group, significantly more UTIs were recorded in patients with an indwelling urinary catheter (33% compared to 14% in the IG, p < 0.004). This could indicate a higher use of urinary catheters in the control group overall. In addition, physicians prescribing antibiotics for UTIs were physically present in 16% of cases in intervention group compared to 44% in the control group. In the intervention group, physicians were contacted mainly by phone or fax. Our findings are in contrast to a study from Northern Ireland reporting that between 58% and 70% of systemic antimicrobial prescriptions were initiated following a physician’s visits at the LTCF [ 17 ]. The fact that physicians were mainly contacted by phone or fax before prescribing antibiotics for a UTI underlines the importance of the role of nursing staff in the prescription process as has been described before [ 18 ]. Knowledge on UTIs and communication skills of nurses as well as stable staffing and continuity of care play an important role in adequate reporting of signs and symptoms suggestive of UTIs in residents [ 19 ].
Even though urinary cultures are recommended as part of the diagnostic procedures for complicated UTIs [ 20 ], only 3 urinary cultures were ordered during the entire study period in the control group. Logistic considerations such as limited access to microbiological tests and the length of time needed to obtain culture results could explain this finding in the control group as has been described previously [ 18 ]. Our finding of very low utilization of urinary cultures is contrasted by a study by Brown et al. which successfully aimed at reducing unnecessary urinary cultures in Canadian LTCFs starting from a baseline of 3.2 urinary cultures per 1000 resident days [ 21 ].
The reach of our interventions among nursing staff was high, as every member of nursing staff attended one onsite training. Every physician received written information on the project several times during the study period during their visits at the LTCFs. This included the guideline that was written in co-operation with a general practitioner active at one of the LTCFs. Unfortunately, only half of the physicians attended at least one of the online sessions. The differences in reach of the intervention between nursing staff and physicians can probably be explained by the fact that nursing staff is employed by the LTCFs whereas the general practitioners are not. In Austria, general practitioners typically work as freelancers. The outcomes could probably have improved if the reach within the physician community had been better.
Several studies describe successful antibiotic stewardship interventions for the prescription of antimicrobial substances for UTIs in long-term care facilities in different settings and countries [ 22 , 23 , 24 , 25 , 26 , 27 ]. Different intervention strategies were used: audit and feedback [ 22 ], guidelines and implementation of interdisciplinary quality circles [ 23 ], educational sessions [ 24 ] and implementation of local ASB teams [ 26 ]. The primary outcomes were mainly number of antimicrobial prescriptions for UTI/ time period [ 24 , 25 ] or overall use of antimicrobials [ 23 , 26 ]. Our study focused a qualitative improvement of antimicrobial prescriptions for UTIs rather than an overall reduction of actual prescriptions. Therefore, a direct comparison of results is difficult. We selected our outcomes based on a previous study indicating a relatively low number of health-care associated infections in our intervention group compared to data from the literature [ 16 ]. Therefore, it appeared less promising to attempt a decrease in the number of antimicrobial prescriptions compared to improving the quality of prescriptions.
Our study has several limitations. We used a non-randomized cluster-controlled study design because randomisation of LTCFs was not feasible as several general practitioners cared for patients in more than one LTCF in Graz. We therefore chose LTCFs in another region to avoid spillover of the intervention. Voluntary participation of LTCFs might mean generalisability of our findings is limited to LTCFs interested in antibiotic stewardship. Our study was conducted during the COVID-19 pandemic. As this was a demanding period for nursing staff and general practitioners (e.g. handling COVID-19 cases at the LTCFs, shortage of staff due to illness, etc.) the impact of the interventions may have been reduced.
Antimicrobial stewardship interventions consisting of clinical practice guidelines, local as well as web-based education for nursing staff and primary care physicians resulted in a significant increase in adequate indications for treatment ) during the intervention period. However, this difference was not maintained in the post-intervention phase. The interventions did not lead to an increase in proportion of clinical failures, hospital admissions due to UTI or adverse events due to antimicrobial treatment. Continued efforts to improve the quality of prescriptions further are necessary.
Data availability
The datasets analysed during the current study are available from the corresponding author on reasonable request.
Abbreviations
long-term care facilities
urinary tract infections
intervention group
control group
institutional review board
Costelloe C, Metcalfe C, Lovering A, Mant D, Hay AD. Effect of antibiotic prescribing in primary care on antimicrobial resistance in individual patients: systematic review and meta-analysis. BMJ. 2010;340:c2096.
Article PubMed Google Scholar
Goossens H, Ferech M, Vander Stichele R, Elseviers M, Group EP. Outpatient antibiotic use in Europe and association with resistance: a cross-national database study. Lancet. 2005;365(9459):579–87.
Antimicrobial Resistance. Tackling a crisis for the health and wealth of nations. 2014.
Ribbe MW, Ljunggren G, Steel K, Topinkova E, Hawes C, Ikegami N, et al. Nursing homes in 10 nations: a comparison between countries and settings. Age Ageing. 1997;26(Suppl 2):3–12.
Strausbaugh LJ. Emerging health care-associated infections in the geriatric population. Emerg Infect Dis. 2001;7(2):268–71.
Article CAS PubMed PubMed Central Google Scholar
Hepper HJ, Sieber C, Walger P, Bahrmann P, Singler K. Infections in the elderly. Crit Care Clin. 2013;29(3):757–74.
Moro ML, Jans B, Cookson B, Fabry J. The burden of healthcare-associated infections in European long-term care facilities. Infect Control Hosp Epidemiol. 2010;31(Suppl 1):S59–62.
Mody L, Crnich C. Effects of Excessive Antibiotic use in nursing homes. JAMA Intern Med. 2015;175(8):1339–41.
Article PubMed PubMed Central Google Scholar
Nicolle LE, Bentley DW, Garibaldi R, Neuhaus EG, Smith PW. Antimicrobial use in long-term-care facilities. SHEA Long-Term-Care Committee. Infect Control Hosp Epidemiol. 2000;21(8):537–45.
Article CAS PubMed Google Scholar
Ricchizzi E, Latour K, Karki T, Buttazzi R, Jans B, Moro ML et al. Antimicrobial use in European long-term care facilities: results from the third point prevalence survey of healthcare-associated infections and antimicrobial use, 2016 to 2017. Euro Surveill. 2018;23(46).
Benoit SR, Nsa W, Richards CL, Bratzler DW, Shefer AM, Steele LM, et al. Factors associated with antimicrobial use in nursing homes: a multilevel model. J Am Geriatr Soc. 2008;56(11):2039–44.
Loeb M, Simor AE, Landry L, Walter S, McArthur M, Duffy J, et al. Antibiotic use in Ontario facilities that provide chronic care. J Gen Intern Med. 2001;16(6):376–83.
Rotjanapan P, Dosa D, Thomas KS. Potentially inappropriate treatment of urinary tract infections in two Rhode Island nursing homes. Arch Intern Med. 2011;171(5):438–43.
Peron EP, Hirsch AA, Jury LA, Jump RL, Donskey CJ. Another setting for stewardship: high rate of unnecessary antimicrobial use in a veterans affairs long-term care facility. J Am Geriatr Soc. 2013;61(2):289–90.
Moro ML, Gagliotti C. Antimicrobial resistance and stewardship in long-term care settings. Future Microbiol. 2013;8(8):1011–25.
Konig E, Medwed M, Pux C, Uhlmann M, Schippinger W, Krause R et al. Prospective Surveillance of Healthcare-Associated Infections in residents in Four Long-Term Care facilities in Graz, Austria. Antibiot (Basel). 2021;10(5).
McClean P, Tunney M, Gilpin D, Parsons C, Hughes C. Antimicrobial prescribing in residential homes. J Antimicrob Chemother. 2012;67(7):1781–90.
van Buul LW, van der Steen JT, Doncker SM, Achterberg WP, Schellevis FG, Veenhuizen RB, et al. Factors influencing antibiotic prescribing in long-term care facilities: a qualitative in-depth study. BMC Geriatr. 2014;14:136.
Kirsebom M, Hedstrom M, Poder U, Wadensten B. General practitioners’ experiences as nursing home medical consultants. Scand J Caring Sci. 2017;31(1):37–44.
Bonkat G, EAU Guidelines on Urological Infections.: European Association of Urology 2023 https://d56bochluxqnz.cloudfront.net/documents/full-guideline/EAU-Guidelines-on-Urological-infections-2023.pdf .
Brown KA, Chambers A, MacFarlane S, Langford B, Leung V, Quirk J, et al. Reducing unnecessary urine culturing and antibiotic overprescribing in long-term care: a before-and-after analysis. CMAJ Open. 2019;7(1):E174–81.
Doernberg SB, Dudas V, Trivedi KK. Implementation of an antimicrobial stewardship program targeting residents with urinary tract infections in three community long-term care facilities: a quasi-experimental study using time-series analysis. Antimicrob Resist Infect Control. 2015;4:54.
Pluss-Suard C, Niquille A, Hequet D, Krahenbuhl S, Pichon R, Zanetti G, et al. Decrease in Antibacterial Use and Facility-Level Variability after the introduction of guidelines and implementation of physician-pharmacist-nurse quality circles in Swiss Long-Term Care facilities. J Am Med Dir Assoc. 2020;21(1):78–83.
Arnold SH, Nygaard Jensen J, Bjerrum L, Siersma V, Winther Bang C, Brostrom Kousgaard M, et al. Effectiveness of a tailored intervention to reduce antibiotics for urinary tract infections in nursing home residents: a cluster, randomised controlled trial. Lancet Infect Dis. 2021;21(11):1549–56.
Hartman EAR, van de Pol AC, Heltveit-Olsen SR, Lindbaek M, Hoye S, Lithen SS, et al. Effect of a multifaceted antibiotic stewardship intervention to improve antibiotic prescribing for suspected urinary tract infections in frail older adults (ImpresU): pragmatic cluster randomised controlled trial in four European countries. BMJ. 2023;380:e072319.
Penalva G, Crespo-Rivas JC, Guisado-Gil AB, Rodriguez-Villodres A, Pachon-Ibanez ME, Cachero-Alba B, et al. Clinical and ecological impact of an Educational Program to optimize antibiotic treatments in nursing homes (PROA-SENIOR): a cluster, Randomized, controlled trial and interrupted time-series analysis. Clin Infect Dis. 2023;76(5):824–32.
Pettersson E, Vernby A, Molstad S, Lundborg CS. Can a multifaceted educational intervention targeting both nurses and physicians change the prescribing of antibiotics to nursing home residents? A cluster randomized controlled trial. J Antimicrob Chemother. 2011;66(11):2659–66.
Download references
Acknowledgements
Not applicable.
This study was supported by Pfizer Global Medical Grants 62587955. The sponsor had no role in study design, data collection and analyses, decision to publish, or preparation of the manuscript.
Author information
Elisabeth König and Lisa Kriegl contributed equally to this work.
Authors and Affiliations
Division of Infectious Diseases, Department of Internal Medicine, Medical University of Graz, Auenbruggerplatz 15, A-8036, Graz, Austria
Elisabeth König, Lisa Kriegl, Robert Krause & Ines Zollner-Schwetz
Geriatric Health Centers of the City of Graz, Graz, Austria
Christian Pux, Michael Uhlmann & Walter Schippinger
Institute of Medical Informatics, Statistics and Documentation, Medical University of Graz, Graz, Austria
Alexander Avian
You can also search for this author in PubMed Google Scholar
Contributions
EK: data analysis, writing original draft, review and editing; LK: data analysis, writing original draft, review and editing; CP: conceptualization, investigation, supervision, project administration; MU: conductig study interventions, writing review and editing; WS: writing review and editing; AA: statistical analysis, writing review and editing; RK: writing review and editing; IZS: conceptualization, supervision, writing original draft, review and editing, project administration.
Corresponding author
Correspondence to Ines Zollner-Schwetz .
Ethics declarations
Ethics approval and consent to participate.
The protocol was approved by the local ethics committee at the Medical University of Graz (IRB approval number 33–035 ex 20/21). Informed consent was waived by the IRB.
Consent for publication
Competing interests.
The authors declare that they have no competing interests.
Additional information
Publisher’s note.
Springer Nature remains neutral with regard to jurisdictional claims in published maps and institutional affiliations.
Electronic supplementary material
Below is the link to the electronic supplementary material.
Supplementary Material 1
Rights and permissions.
Open Access This article is licensed under a Creative Commons Attribution 4.0 International License, which permits use, sharing, adaptation, distribution and reproduction in any medium or format, as long as you give appropriate credit to the original author(s) and the source, provide a link to the Creative Commons licence, and indicate if changes were made. The images or other third party material in this article are included in the article’s Creative Commons licence, unless indicated otherwise in a credit line to the material. If material is not included in the article’s Creative Commons licence and your intended use is not permitted by statutory regulation or exceeds the permitted use, you will need to obtain permission directly from the copyright holder. To view a copy of this licence, visit http://creativecommons.org/licenses/by/4.0/ . The Creative Commons Public Domain Dedication waiver ( http://creativecommons.org/publicdomain/zero/1.0/ ) applies to the data made available in this article, unless otherwise stated in a credit line to the data.
Reprints and permissions
About this article
Cite this article.
König, E., Kriegl, L., Pux, C. et al. Implementation of an antimicrobial stewardship program for urinary tract infections in long-term care facilities: a cluster-controlled intervention study. Antimicrob Resist Infect Control 13 , 43 (2024). https://doi.org/10.1186/s13756-024-01397-2
Download citation
Received : 20 December 2023
Accepted : 04 April 2024
Published : 16 April 2024
DOI : https://doi.org/10.1186/s13756-024-01397-2
Share this article
Anyone you share the following link with will be able to read this content:
Sorry, a shareable link is not currently available for this article.
Provided by the Springer Nature SharedIt content-sharing initiative
- Nursing home
- Healthcare associated infection
- Antibiotic stewardship
- Urinary tract infections
Antimicrobial Resistance & Infection Control
ISSN: 2047-2994
- General enquiries: [email protected]
- Open access
- Published: 15 April 2024
A pathway study of factors influencing quality of fertility life
- Abulizi Maierhaba 2 na1 ,
- Ming Jiang 2 na1 ,
- Lihuan Zhi 1 ,
- Xueyu Wei 1 ,
- Lijuan He 1 &
- Li Wang 2
BMC Public Health volume 24 , Article number: 1045 ( 2024 ) Cite this article
44 Accesses
Metrics details
To investigate the factors influencing fertility quality of life in infertile men, constructing a structural equation model of the factors influencing fertility quality of life in infertile men, and to provide suggested measures for improving fertility quality of life in infertile men.
It is a Observational study. Infertile men (n = 250) attending a fertility centre in a hospital in Xinjiang, matched 1:2 men with no obvious male factor in the control group (n = 500).The Quality of Fertility Life Scale, the Social Support Scale, the Fertility Stress Scale and the Positive Attention Awareness Scale were used to conduct the survey. The model was constructed by applying the maximum likelihood estimation method in Mplus 8.3 software, to explore the factors influencing the quality of reproductive life of infertile men through path analyses. Differences between the case and control groups were statistically significant ( P < 0.05) in terms of total fertility quality of life scores, core entry dimensions, affective responses, physical and mental relationships, selective treatment dimensions, and treatment tolerance.
Past medical history, history of exposure to hazardous environments, health insurance reimbursement, social support, fertility stress, and mindfulness are important factors affecting the quality of fertility life of infertile men.
The quality of fertility life of infertile men is not optimistic. By improving the level of mindfulness, fertility stress, and social support, we propose appropriate measures to improve the quality of fertility life of infertile men. These measures can improve their confidence in clinical diagnosis and infertility treatment, enabling them to cope positively with these challenges.
Peer Review reports
Introduction
According to the World Health Organisation, failure to conceive in a man and a woman who have lived together for more than one year, have a normal sexual life, and are not using contraception is called infertility [ 1 ]. People who meet this description are described as infertile. Rapid socio-economic development, rising living standards, and increasing social pressure, along with the increase of environmental pollution, drug abuse, poor dietary and lifestyle habits, and other influencing factors, have resulted in an increase in the number of infertility patients [ 2 , 3 ]. The reproductive health of the infertile population is also regarded as a public health problem that needs worldwide attention [ 4 ]. In September 2020, the Expert Consensus on Male Reproduction-Related Genetic Tests issued by the Chinese Medical Association Men’s Branch showed that the incidence rate of male infertility in China is 10% ∼ 15% [ 5 ].
Quality of life (QoL) is the most important topic to be addressed in infertility counselling [ 6 ], and its assessment and the identification of its influencing factors have become as important for treatment [ 7 ]. The results of a study on the QoL of Japanese men with infertility [ 8 ] showed that men with primary infertility had significantly lower scores in the emotional, physical, mental, and social domains, indicating a poorer QoL. Compared to them, Turkish men had significantly lower QoL, which may be related to the improvement of the treatment system for infertility and social subsidies in Japan [ 9 ]. In addition, the quality of fertility life of men with infertility in Middle Eastern countries such as Turkey and Iran is significantly lower, which is closely related to the traditional culture of each country [ 10 ]. Infertility brings a series of physical and psychological traumas to patients and families, which not only affects family happiness and harmony but also gradually affects social harmony [ 11 ].
At present, more studies exist on the quality of fertility life of infertile women than men. Additionally, the majority of these studies focus on examining the mechanism of the impact on the quality of life, with a dearth of literature addressing the pathways of the impact and positive thoughts. Many factors influence the quality of fertility life, and these factors are not mutually exclusive; instead, they interact with each other. They can have a direct effect on the quality of fertility life or an indirect effect through other factors. The purpose of this paper is to explore the influencing factors on the quality of reproductive life of male infertility patients, to construct a path model with the quality of reproductive life as the outcome variable, to explore the direct or indirect effects of exogenous potential variables on the outcome variable, and to clarify the path relationship between the variables, and one of the goals of this study is to determine whether the model proposed by the researchers is supported by the data in real conditions. To provide theoretical ideas for improving the quality of reproductive life of infertile men.
Materials and methods
Study population.
This study was conducted using the case-control studies. Men attending the outpatient clinic of the Reproductive Fertility Centre of the First Affiliated Hospital of Xinjiang Medical University were recruited during the period of December 2020 to August 2023, with infertile men in the case group (n = 250) and men with no obvious male factor in the control group matched on a 1:2 basis (n = 500).
Inclusion criteria were men of reproductive age with normal sex life and not using contraception, regular menstruation of the female partner, no obvious abnormalities in gynaecological reproductive-related examinations [ 12 ]; abnormalities detected in routine semen examination (fifth edition of the World Health Organisation standards for semen analysis [ 13 ]); and providing informed consent and voluntary participation in the study.
Exclusion criteria were having a major disease [ 14 ]; having a proven psychological disorder, substance abuse or dependence history (including alcohol) [ 15 ]; and difficulty in understanding or an inability to complete the questionnaire.
Survey instruments
General information questionnaire.
Primary contents were age, ethnicity, place of residence, education level, weight, height, reproductive history, history of previous illnesses, monthly household income, occupation, history of smoking, history of alcohol consumption, exercise, sleep time, health insurance reimbursement, and history of exposure to hazardous environments.
Social support rating scale (SSRS)
The Social Support Rating Scale (SSRS) scale consists of 10 entries to calculate the total score of social support. The higher the score, the better the level of social support [ 16 ]. The Cronbach’s a coefficients of support rating scale was greater than 0.7, indicating good internal consistency, and the KMO value was greater than 0.7.
Massive attention and awareness scale(MAAS)
The MAAS is a scale developed by Ryan and Brown in 2003 [ 17 ]. It consists of 15 questions on a six-point Likert scale, with ‘1’ almost always and ‘6’ almost never, where the minimum score is 15 and the maximum score is 90. The higher the score, the higher the level of positive thinking.The Cronbach’s a coefficients of massive attention and awareness scale was greater than 0.7, indicating good internal consistency, and the KMO value was greater than 0.7.
Fertility pressure inventory (FPI)
The Fertility Problem Inventory (FPI) scale was used to measure the fertility-related stress of infertility patients, which was specially compiled to assess the fertility-related stress of infertility patients, with high reliability and validity of the total score and the subscales, which consisted of five dimensions with a total of 46 entries. The scale consists of 46 entries in five dimensions, including social pressure, sexual pressure, couple relationship, parental role demand, and rejection of childless lifestyle, with total scores ranging from 46 to 276, from ‘1 (totally disagree)’ to ‘6 (totally agree)’. The higher the score, the higher the level of fertility stress.The Cronbach’s a coefficient and KMO value of the Fertility Stress Scale are greater than 0.7, indicating that the questionnaire has good reliability and validity.
Fertility quality of life scale
The Fertility Quality of Life Scale consists of two modules (the core module and the selective treatment module) totalling 36 items [ 18 ]. The core module contains 24 items and four dimensions, with six items in each dimension; the selective treatment module contains 10 items, including the dimensions of therapeutic environment (six items) and tolerance (four items). Each entry is scored from 0 to 4, and the total score ranges from 0 to 100 by converting the scores of the total scale and subscales, and the standardised score is calculated by dividing the original score of the total scale × 25/number of entries in the total scale, with a higher score indicating a better QoL.The Cronbach’s a coefficient and KMO value of the Fertility Quality of Life Scale are greater than 0.7, indicating that the questionnaire has good reliability and validity.
Statistical methods
Statistical analyses were performed in SPSS 25.0, and quantitative data were expressed as mean ± standard deviation. The t-test and one-way ANOVA were used for those conforming to normal distribution, and the rank-sum test was used for those parts not conforming to normal distribution to establish the multiple linear regression model. The maximum likelihood estimation method was applied to construct the model in Mplus 8.3 software to explore the fit of the actual data to the theoretical model as well as the direct and indirect effect paths and effect sizes. The Bootstrap procedure (5000 repeated extractions) was applied to test the existence of chained mediation effects, with 95% confidence intervals being the most important criterion for judgement, and the absence of 0 in the intervals means that the mediation effect is significant. The significance level was set at 0.05.
Status of quality of reproductive life in the study population
The current status of the quality of reproductive life of the case group and the control group and the analysis of differences are shown in Table 1 . The total score of the quality of reproductive life of the case group was significantly lower than that of the control group, of which the scores of the case group in the core entry dimension in terms of emotional response and physical and mental relationship were significantly lower than those of the control group, and the scores of the case group in terms of the selective treatment dimension were significantly lower than those of the control group. The case group scored significantly lower than the control group in treatment tolerance. (See Table 1 )
Univariate analysis of quality of reproductive life
The results of univariate linear regression showed that the score of quality of reproductive life in patients with a history of fertility was 6.139 units higher than that in patients without a history of fertility; the quality of reproductive life score for patients with a mean daily sleep time > 6 h was 6.743 units higher than that for patients with a mean sleep time ≤ 6 h. For every 1-unit increase in social support of patients in the case group, the corresponding quality of reproductive life increased on average by 0.621; for every 1-unit increase in the level of positive thinking of patients in the case group, their corresponding quality of reproductive life increased by 0.270; and for every 1-unit increase in the level of stress of fertility, the corresponding quality of reproductive life increased by 0.270. (See Table 2 )
Unitary linear regression was used to analyse the factors influencing the emotional response dimension, physical and mental relationship dimension, and treatment tolerance dimension of the quality of reproductive life of the men in the case group, and the results showed the following:
In the emotional response dimension, no history of disease and having a history of childbearing were the factors influencing the emotional response; in the physical and mental relationship dimension, no history of disease and having a history of childbearing were the factors influencing the physical and mental relationship; and in the treatment tolerance dimension, treatment tolerance was higher in men with reproductive history.
In the affective response dimension, the mean increase in affective response was 0.284 units for every 1-unit increase in positive thoughts; the mean decrease in affective response was 0.095 units for every 1-unit increase in fertility stress; and in the mind-body relationship dimension, the mean increase in mind-body relationship was 0.343 units for every 1-unit increase in positive thoughts; and the mean decrease in mind-body relationship was 0.151 units for every 1-unit increase in fertility stress.
The mind-body relationship was 6.677 units higher in patients with an average sleep duration of > 6 h/day than in patients with an average sleep duration of ≤ 6 h/day; in the dimension of treatment tolerance, for each increase of 1-unit in positive thoughts, the mean increase in treatment tolerance was 0.370 units. For every 1-unit increase in social support, emotional response, physical and mental relationships, and treatment tolerance increased by a mean of 0.450, 0.565, and 0.534 units, respectively.
In the dimension of emotional response, monthly family income of 10,000 yuan and above positively affected emotional response; in the dimension of physical and mental relationship, monthly family income of 10,000 yuan and above, and no history of exposure to harmful environments were factors affecting physical and mental relationship, and students’ physical and mental relationship was 20.685 units lower than the physical and mental relationship of patients with other occupations.
In the dimension of treatment tolerability, the self-employed, those engaged in agriculture, fishery, and animal husbandry, monthly household income of 7000 ∼ 10,000 yuan, and no history of hazardous environmental exposure were the influencing factors of treatment tolerance. Patients without health insurance reimbursement scored lower than patients with health insurance reimbursement in the dimensions of emotional response, physical and mental relationship, and treatment tolerance.
Multifactorial analysis of quality of fertility life
Based on unitary linear regression, statistically significant variables were included in the multiple linear regression model, with quality of reproductive life as the dependent variable, which showed that the adjusted R 2 was 0.249, the independent variable explained 24.9% of the dependent variable. No history of illness, positive thoughts, social support, and no history of harmful environmental exposure, fertility stress, and no health insurance reimbursement were the influencing factors of QoL in childbearing. (See Table 3 )
Statistically significant variables were included in the multiple linear regression model with affective response as the dependent variable, and the results showed that the adjusted R 2 was 0.117, which means that the independent variable explained 11.7% of the dependent variable. History of childbearing, no history of illness, positive thoughts, and no health insurance reimbursement were the influencing factors of affective response.
Statistically significant variables were included in the multiple linear regression model, with the mind-body relationship as the dependent variable, and the results showed that the adjusted R 2 was 0.141, which means that the independent variable explained 14.1% of the dependent variable. No history of illness, positive thoughts, and no health insurance reimbursement were the factors affecting the mind-body relationship.
Statistically significant variables were included in the multiple linear regression model, with treatment tolerance as the dependent variable, and the results showed that the adjusted R 2 was 0.119, which means that the independent variable explained 11.9% of the dependent variable. Positive thoughts, not working in agriculture, fishery, or pastoral labour, no history of harmful environmental exposure, and no health insurance reimbursement were the factors affecting treatment tolerance.
Path analysis of reproductive quality of life
Path analysis is an extension of multivariate linear regression analysis, which does not require the variables to be independent of each other, and it can accommodate the causal structure of multiple links. Consequently, it can represent these causal relationships very clearly through path diagrams, according to which deeper analyses can be conducted, and it is suitable for analysing multivariate dependence problems that contain indirect influence relationships [ 19 ].
The main reference fit indicators in structural equation modelling were CFI > 0.9; TLI > 0.9; RMSEA < 0.06; SRMR < 0.08; 𝟀 2/df is the composite fit indicator, 𝟀 2/df < 3 is the best fit, and 𝟀 2/df < 5 is a fit that is moderate ( N ≥ 250). The established hypothetical model was tested for the goodness-of-fit of the model and model correction several times, and the fitting results of the final impact path theoretical model and the actual data showed that they all met the required fit criteria. The specific indexes were: 𝟀 2/df = 1.56, CFI = 0.968, TLI = 0.920, RMSEA = 0.047, SRMR = 0.045, and the model fit is good. The path model is shown in Fig. 1 .
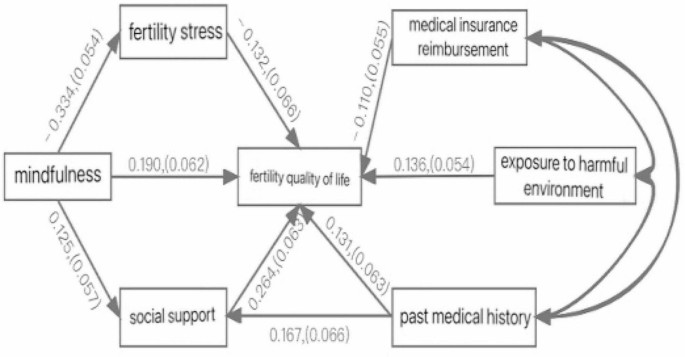
The test results of mediating effect of path analysis of infertile men’s reproductive QoL are shown in Table 4 . The standardised path coefficient from mindfulness to fertility stress is -0.334, from mindfulness to social support is 0.125, from mindfulness to fertility quality of life is 0.190. Similarly, all other standardised path coefficients can be seen in Fig. 1 .
Path 1: Disease history can directly affect the reproductive QoL and also indirectly affect the reproductive QoL through social support, the value of direct path effect is 0.131, which accounted for 74.86% of the total effect size. The 95% confidence interval for the simple mediation effect of disease history→social support→quality of reproductive life was (0.011, 0.095), and the interval did not contain 0, indicating that the mediation effect of this indirect pathway was significant, and that disease history positively predicted quality of reproductive life through social support. The total indirect effect value was 0.044, accounting for 25.14% of the total effect size.
Path 2: mindfulness can directly affect the quality of reproductive life, but also indirectly affect the quality of reproductive life through the two paths of reproductive stress and social support, the direct path effect value is 0.190, accounting for 71.16% of the total effect size. The 95% confidence interval for the simple mediation effect of Positive Thoughts→Fertility Stress→Quality of Reproductive Life is (0.001, 0.087), and the interval does not contain 0, indicating that the mediation effect of this indirect path is significant. The value of the indirect path effect is 0.044, indicating that its effect on the quality of reproductive life through the mediation of fertility stress increases by 0.044, accounting for 16.48% of the total effect; the 95% confidence interval for the simple mediation effect of Positive Thoughts→Social Support→Quality of Reproductive Life is (0.005, 0.075), with an interval that does not contain 0, indicating that the mediation effect of this indirect path is significant. The indirect path effect value is 0.033, indicating that its effect on the quality of reproductive life through the mediation of social support increased by 0.033, accounting for 12.36% of the total effect; positive thoughts positively predicted the quality of reproductive life through fertility stress and social support.
Discussion and conclusion
Under the special cultural background of China, it is believed that the transmission of a family is a major event in life, and infertility brings great mental trauma to the patients themselves as well as great pain to both families [ 14 ]. Infertility is a reproductive disease that involves the privacy of the patient, and the treatment process is often a combination of hope and disappointment, during which the patient’s psychological fluctuations are large, resulting in psychological stress and thus reducing the quality of reproductive life [ 20 ].
We explored the factors and pathway analysis affecting the quality of reproductive life of infertile men based on the health ecology model. The core entry dimensions between our case groups and control group differed significantly in emotional response and physical and mental relationships. The emotional response score of men in the case group was lower than the study of 304 infertile men by Cai and Dong [ 21 ]. The score of physical and mental relations in the case group was lower than the control group, and likewise lower than the results of the survey conducted by Cusatis et al. [ 22 ] in 2018 in the US.
Infertility itself and its treatment process will cause great damage to the body and mind, affecting their QoL [ 23 ]. The score of treatment environment in the selective treatment dimension was lower than 60. Healthcare professionals should try to create a harmonious and warm medical environment for male infertility patients [ 24 ]. A good environment for sperm retrieval examination and treatment is especially important for male infertility patients to face the disease more positively. Infertile men face long-term infertility treatment and suffer from great social and psychological pressure, and the length of treatment and repeated treatments also significantly affect the QoL of the patients’ fertility.
Long-term repeated treatments may lead to mental health problems and even cause a decrease in the conception rate [ 25 , 26 ]. The difference in quality of reproductive life between the case and control groups was statistically significant. Under the traditional Chinese concept, not having children is not considered as a complete family, and the disease itself brings multiple pressures on the patients such as a long duration of the disease and a large financial burden, which greatly reduce the quality of reproductive life of male infertility patients [ 23 ].
By developing structural equation modelling, we showed that infertile men’s past disease history not only directly affects their reproductive QoL, but disease history also affects reproductive QoL by influencing social support, which in this paper indicates that men with no history of disease receive more support in their social network relationships and thus improve their reproductive QoL. Positive thoughts directly affect the quality of reproductive life and can also indirectly affect the quality of reproductive life by affecting fertility stress. The higher scores of parental role demands in fertility stress among the study participants may be related to the traditional concept in China that men, as the breadwinners of the family, reproducing offspring is a major role expectation, while infertile men experience fertility-related stress due to fertility problems [ 21 ].
Although some infertility can be improved and cured through later treatment, due to social prejudice, infertile men avoid their condition due to inferiority complex, rarely discuss their condition with doctors and family members, and actively accept appropriate treatment, resulting in physical and mental pain [ 27 ]. Positive thoughts → fertility stress → quality of reproductive life, indicating that the indirect effect of positive thoughts was weakened after fertility stress mediation. Consistent with the results of a previous study [ 28 ], patients with high levels of positive thoughts can better regulate their emotions and alleviate the stress of childbirth, which helps to eliminate intense stress and improve QoL. Positive thoughts can indirectly affect the quality of reproductive life through social support, and studies have shown [ 29 ] that improving the quality of reproductive life of infertile men requires a high level of support from spouses and family members. Therefore, the attention to the patient can be improved by establishing certain social support networks so that the patient can increase his self-confidence to cope with the disease treatment process. Medical personnel can conduct interventions from the perspective of enhancing social support, which can improve the QoL of patients and enhance the recognition of self [ 30 ].
This study has the following limitations: (1) This study only focused on infertile men who came to the hospital to seek treatment for infertility making the findings somewhat biased; (2) it was limited to infertile men in the Reproductive Centre of the First Affiliated Hospital of Xinjiang Medical University; (3) it is a cross-sectional study; and (4) it only examined the quality of reproductive life of infertile men but did not investigate their spouses at the same time, ignoring the mutual influence and differences between spouses and infertile men in the quality of reproductive life.
Future research could include the following: (1) Building a WeChat platform to incorporate more cases, (2) promoting the use of samples at all levels of hospitals and in all communities, (3) including a longitudinal intervention study, and (4) including infertile men’s spouses.
Data availability
The datasets generated and/or analysed during the current study are not publicly available due considere private to the patients. but are available from the corresponding author on reasonable request.
Feijing Z. Study on the correlation between fertility-related stress and quality of life in infertile couples [D]. Zhengzhou: Zhengzhou University; 2016.
Google Scholar
Ying L, Loke AY. An analysis of the concept of partnership in the couples undergoing infertility treatment. J Sex Marital Ther. 2016;42:243–56.
Article PubMed Google Scholar
Na LU, Hongyi FU, Yan W. Mediating effects of second-child fertility stress and individual coping styles in elderly infertile women. China Med News. 2018;15:62–6.
Wenxi SUN, Lingjuan HU. Discussion on the current status of infertility at home and abroad and intervention strategies in China. Popul Health. 2019;46:19–23.
Xiangping L, Chunhua D. Important advances in men’s science in 2020. China Med News. 2020;35:10.
Hai C, Cristiana C. Gender differences in quality of life, intensity of dysfunctional attitudes, unconditional self-acceptance, emotional distress and dyadic adjustment of infertile couples. Procedia Soc Behav Sci. 2013;78:506–10.
Article Google Scholar
Karabulut A, Özkan S, Oğuz N. Predictors of fertility quality of life (FertiQoL) in infertile women: analysis of confounding factors. Eur J Obstet Gynecol Reprod Biol. 2013;170:193–7.
Asazawa K, Jitsuzaki M, Mori A, et al. Quality-of-life predictors for men undergoing infertility treatment in Japan [J]. Japan J Nurs Science: JJNS. 2019;16(3):329–41.
Goker A, Yanikkerem E, Birge O, Kuscu NK. Quality of life in Turkish infertile couples and related factors. Hum Fertil (Camb). 2018;21(3):195–203.
Ahmadi H, Montaser-Kouhsari L, Nowroozi MR, Bazargan-Hejazi S. Male infertility and depression: a neglected problem in the Middle East. J Sex Med. 2011;8:824–30.
Yanping G. Analysis of cases and related factors of infertility patients in a hospital in Daqing [D]. Changchun: Jilin University; 2010.
Huimei S. Pathway analysis of factors influencing health-related quality of life [D]. Beijing: Beijing University of Chinese Medicine; 2015.
Jianzhong Z, Haisong LI, Yong Z, Bin W, Zhonglin C. Analysis of the prevalence of erectile dysfunction and related factors in male infertility patients. Zhonghua Nan Ke Xue (National J Andrology) 2020;26(11).
Zhenwen C, Longjie GU. Standardization of semen analysis and assessment of semen quality - publication of the WHO Laboratory Manual for the examination and Processing of Human Semen. [J] Chin J Family Plann. 2012;20:58–62. 5th ed.
Li X, Ye L, Lifeng T, Yafen Huo, MingJie Z. Infertility-related stress and life satisfaction among Chinese infertile women: A moderated mediation model of marital satisfaction and resilience. [J]. Sex Roles: A Journal of Research. 2020;82(5).
Hosseini MS, Mousavi P, Hekmat K, Haghighyzadeh MH, Johari Fard R, Mohammad Jafari R. Effects of a short-term mindfulness-based stress reduction program on the quality of life of women with infertility: a randomized controlled clinical trial. Complement Ther Med. 2020;50:102403.
Brown KW, Ryan RM. The benefits of being present: mindfulness and its role in psychological well-being. J Pers Soc Psychol. 2003;84(4):822–48.
Jie C, Haixin BO, Yanping D. Research on the quality of reproductive life and influencing factors of female infertility patients. J Nurs. 2018;25:1–5.
Xiaoping Y. Application and reliability and validity analysis of fertility quality of life specific scale [D], 2016.
Abliz B, Lijuan HE, Wanzhu Z, Chen Z. Study on the current situation and factors influencing the quality of reproductive life of infertile men. Pract Prev Med. 2023;30:21–5.
Yanhong Z, Ruimin B, Yanna CAI. Correlation of psychological stress with psychological resilience and reproductive quality of life in infertile women. Maternal Child Health Care China. 2020;35:3462–5.
Cai YN, Dong YZ. Dual mediating effects of positive and negative emotions on fertility stress and fertility quality of life among infertile men in China. Zhonghua Nan Ke Xue. 2021;27(12):1092–7.
PubMed Google Scholar
Cusatis R, Fergestrom N, Cooper A, Schoyer KD, Kruper A, Sandlow J, et al. Too much time? Time use and fertility-specific quality of life among men and women seeking specialty care for infertility. BMC Psychol. 2019;7:45.
Article PubMed PubMed Central Google Scholar
Feijing Z, Zhimin SUN, Yanan S, Yanna CAI, Yuezhi D. Impact of gender roles on fertility stress and quality of life in infertile couples. J Clin Nurs Pract. 2016;2:37–41.
Guo L. Impact of social support on quality of life of male infertility patients. Gansu Med J. 2017;36:567–8.
Wang JH. Analysis and intervention of psychological state of patients with multiple in vitro fertilization-embryo transfer failures. World Digest Recent Med Inf. 2018;18:183–.
Caiying L, Qiongfang C. Survey and analysis of fertility quality of life of infertility patients. Chin Med Sci. 2017;7:103–5.
Chao LI, Zhiyong C, Jinzhou QIN, Liqin FU. A study on the sexual stress status of men among first-time infertile couples. China Med Pharm. 2019;9:193–5.
Li G, Jiang Z, Han X, Shang X, Tian W, Kang X, et al. A moderated mediation model of perceived stress, negative emotions and mindfulness on fertility quality of life in women with recurrent pregnancy loss. Qual Life Res. 2020;29(7):1775–87.
Asazawa K, Jitsuzaki M, Mori A, Ichikawa T, Shinozaki K, Porter SE. Quality-of-life predictors for men undergoing infertility treatment in Japan. Jpn J Nurs Sci. 2019;16(3):329–41.
Download references
Acknowledgements
Not applicable.
This work was supported by National Natural Science Foundation(82260651 & 82060589)., Key Laboratory of Environmental Exposome, Xinjiang Medical University,, Xinjiang Uygur Autonomous Region "14th Five-Year" special discipline-public health and prevention medicine.
Author information
Maierhaba Abulizi and Ming Jiang contributed equally to this work.
Authors and Affiliations
School of Public Health, Xinjiang Medical University, Urumqi, Xinjiang, China
Lihuan Zhi, Xueyu Wei & Lijuan He
The First Affiliated Hospital of Xinjiang Medical University, Urumqi, Xinjiang, China
Abulizi Maierhaba, Ming Jiang & Li Wang
You can also search for this author in PubMed Google Scholar
Contributions
J M was responsible for writing substantial contributions to conception and design, W L and M A were responsible for acquisiting the data, analysis and interpretation of data, M A drafted the article and was a major contributor in writing the manuscript. HLJ and ZLH revised it critically for important intellectual content, final approval of the version to be published, All authors read and approved the final manuscript.
Corresponding authors
Correspondence to Lijuan He or Li Wang .
Ethics declarations
Ethics approval and consent to participate.
This study was approved by the Ethics Committee of the First Affiliated Hospital of Xinjiang Medical University (ethics number: 20210226-168). Informed consent for participation was obtained from all the participants and their legal guardians.
Consent for publication
Competing interests.
The authors declare no competing interests.
Additional information
Publisher’s note.
Springer Nature remains neutral with regard to jurisdictional claims in published maps and institutional affiliations.
Rights and permissions
Open Access This article is licensed under a Creative Commons Attribution 4.0 International License, which permits use, sharing, adaptation, distribution and reproduction in any medium or format, as long as you give appropriate credit to the original author(s) and the source, provide a link to the Creative Commons licence, and indicate if changes were made. The images or other third party material in this article are included in the article’s Creative Commons licence, unless indicated otherwise in a credit line to the material. If material is not included in the article’s Creative Commons licence and your intended use is not permitted by statutory regulation or exceeds the permitted use, you will need to obtain permission directly from the copyright holder. To view a copy of this licence, visit http://creativecommons.org/licenses/by/4.0/ . The Creative Commons Public Domain Dedication waiver ( http://creativecommons.org/publicdomain/zero/1.0/ ) applies to the data made available in this article, unless otherwise stated in a credit line to the data.
Reprints and permissions
About this article
Cite this article.
Maierhaba, A., Jiang, M., Zhi, L. et al. A pathway study of factors influencing quality of fertility life. BMC Public Health 24 , 1045 (2024). https://doi.org/10.1186/s12889-024-18386-0
Download citation
Received : 23 November 2023
Accepted : 19 March 2024
Published : 15 April 2024
DOI : https://doi.org/10.1186/s12889-024-18386-0
Share this article
Anyone you share the following link with will be able to read this content:
Sorry, a shareable link is not currently available for this article.
Provided by the Springer Nature SharedIt content-sharing initiative
- Infertility
- Fertility quality of life
- Influencing factors
- Pathway analysis
BMC Public Health
ISSN: 1471-2458
- Submission enquiries: [email protected]
- General enquiries: [email protected]
Measles — United States, January 1, 2020–March 28, 2024
Weekly / April 11, 2024 / 73(14);295–300
Adria D. Mathis, MSPH 1 ; Kelley Raines, MPH 1 ; Nina B. Masters, PhD 1 ; Thomas D. Filardo, MD 1 ; Gimin Kim, MS 1 ; Stephen N. Crooke, PhD 1 ; Bettina Bankamp, PhD 1 ; Paul A. Rota, PhD 1 ; David E. Sugerman, MD 1 ( View author affiliations )
What is already known about this topic?
Although endemic U.S. measles was declared eliminated in 2000, measles importations continue to occur. Prolonged outbreaks during 2019 threatened the U.S. measles elimination status.
What is added by this report?
During January 1, 2020–March 28, 2024, a total of 338 U.S. measles cases were reported; 29% of these cases occurred during the first quarter of 2024, almost all in persons who were unvaccinated or whose vaccination status was unknown. As of the end of 2023, U.S. measles elimination status was maintained.
What are the implications for public health practice?
Risk for widespread U.S. measles transmission remains low because of high population immunity. Enhanced efforts are needed to increase routine U.S. vaccination coverage, encourage vaccination before international travel, identify communities at risk for measles transmission, and rapidly investigate suspected measles cases to reduce cases and complications of measles.
- Article PDF
- Full Issue PDF
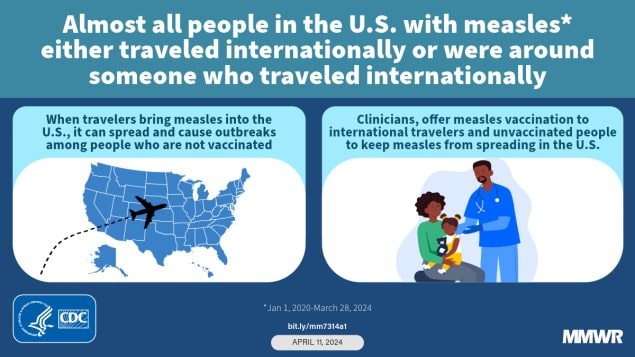
Measles is a highly infectious febrile rash illness and was declared eliminated in the United States in 2000. However, measles importations continue to occur, and U.S. measles elimination status was threatened in 2019 as the result of two prolonged outbreaks among undervaccinated communities in New York and New York City. To assess U.S. measles elimination status after the 2019 outbreaks and to provide context to understand more recent increases in measles cases, CDC analyzed epidemiologic and laboratory surveillance data and the performance of the U.S. measles surveillance system after these outbreaks. During January 1, 2020–March 28, 2024, CDC was notified of 338 confirmed measles cases; 97 (29%) of these cases occurred during the first quarter of 2024, representing a more than seventeenfold increase over the mean number of cases reported during the first quarter of 2020–2023. Among the 338 reported cases, the median patient age was 3 years (range = 0–64 years); 309 (91%) patients were unvaccinated or had unknown vaccination status, and 336 case investigations included information on ≥80% of critical surveillance indicators. During 2020–2023, the longest transmission chain lasted 63 days. As of the end of 2023, because of the absence of sustained measles virus transmission for 12 consecutive months in the presence of a well-performing surveillance system, U.S. measles elimination status was maintained. Risk for widespread U.S. measles transmission remains low because of high population immunity. However, because of the increase in cases during the first quarter of 2024, additional activities are needed to increase U.S. routine measles, mumps, and rubella vaccination coverage, especially among close-knit and undervaccinated communities. These activities include encouraging vaccination before international travel and rapidly investigating suspected measles cases.
Introduction
Measles is a highly infectious acute, febrile rash illness with a >90% secondary attack rate among susceptible contacts ( 1 ). High national 2-dose coverage with the measles, mumps, and rubella (MMR) vaccine led to the declaration of U.S. measles elimination* in 2000 ( 2 ). However, this elimination status was threatened in 2019 because of two prolonged outbreaks among undervaccinated communities in New York and New York City; these outbreaks accounted for 29% of all reported cases during 2001–2019 ( 2 ). To assess U.S. measles elimination status after the 2019 outbreaks and to provide context for understanding more recent increases in measles cases in 2024, † CDC assessed the epidemiologic and laboratory-based surveillance of measles in the United States and the performance of the U.S. measles surveillance system during January 1, 2020–March 28, 2024.
Reporting and Classification of Measles Cases
Confirmed measles cases § ( 1 ) are reported to CDC by state health departments through the National Notifiable Disease Surveillance System and directly (by email or telephone) to the National Center for Immunization and Respiratory Diseases. Measles cases are classified by the Council of State and Territorial Epidemiologists as import-associated if they were internationally imported, epidemiologically linked to an imported case, or had viral genetic evidence of an imported measles genotype ( 1 ); cases with no epidemiologic or virologic link to an imported case are classified as having an unknown source ( 1 ). For this analysis, unique sequences were defined as those differing by at least one nucleotide in the N-450 sequence (the 450 nucleotides encoding the carboxyl-terminal 150 nucleoprotein amino acids) based on the standard World Health Organization (WHO) recommendations for describing sequence variants ¶ ( 3 ). Unvaccinated patients were classified as eligible for vaccination if they were not vaccinated according to Advisory Committee on Immunization Practices recommendations ( 4 ). A well-performing surveillance system was defined as one with ≥80% of cases meeting each of the following three criteria: classified as import-associated, reported with complete information on at least eight of 10 critical surveillance indicators (i.e., place of residence, sex, age, occurrence of fever and rash, date of rash onset, vaccination status, travel history, hospitalization, transmission setting, and whether the case was outbreak-related) ( 5 ), and laboratory-confirmed.
Assessment of Chains of Transmission
Cases were classified into chains of transmission on the basis of known epidemiologic linkages: isolated (single) cases, two-case chains (two epidemiologically linked cases), and outbreaks (three or more epidemiologically linked cases). The potential for missed cases within two-case chains and outbreaks was assessed by measuring the interval between measles rash onset dates in each chain; chains with more than one maximum incubation period (21 days) between cases could indicate a missing case in the chain. This activity was reviewed by CDC, deemed not research, and was conducted consistent with applicable federal law and CDC policy.**
Reported Measles Cases and Outbreaks
CDC was notified of 338 confirmed measles cases with rash onset during January 1, 2020–March 28, 2024 ( Figure ); cases occurred in 30 jurisdictions. During 2020, 12 of 13 cases preceded the commencement of COVID-19 mitigation efforts in March 2020. Among the 170 cases reported during 2021 and 2022, 133 (78%) were associated with distinct outbreaks: 47 (96%) of 49 cases in 2021 occurred among Afghan evacuees temporarily housed at U.S. military bases during Operation Allies Welcome, and 86 (71%) of 121 cases in 2022 were associated with an outbreak in central Ohio. During 2023, 28 (48%) of 58 cases were associated with four outbreaks. As of March 28, 2024, a total of 97 cases have been reported in 2024, representing 29% of all 338 measles cases reported during January 1, 2020–March 28, 2024, and more than a seventeenfold increase over the mean number of cases reported during the first quarter of 2020–2023 (five cases).
Characteristics of Reported Measles Cases
The median patient age was 3 years (range = 0–64 years); more than one half of cases (191; 58%) occurred in persons aged 16 months–19 years ( Table ). Overall, 309 (91%) patients were unvaccinated (68%) or had unknown vaccination status (23%); 29 (9%) had previously received ≥1 MMR vaccine dose. Among the 309 cases among unvaccinated persons or persons with unknown vaccination status, 259 (84%) patients were eligible for vaccination, 40 (13%) were aged 6–11 months and therefore not recommended for routine MMR vaccination, and 10 (3%) were ineligible for MMR because they were aged <6 months. †† Among 155 (46%) hospitalized measles patients, 109 (70%) cases occurred in persons aged <5 years; 142 (92%) hospitalized patients were unvaccinated or had unknown vaccination status. No measles-associated deaths were reported to CDC.
Imported Measles Cases
Among all 338 cases, 326 (96%) were associated with an importation; 12 (4%) had an unknown source. Among the 326 import-associated cases, 200 (61%) occurred among U.S. residents who were eligible for vaccination but who were unvaccinated or whose vaccination status was unknown. Among 93 (28%) measles cases that were directly imported from other countries, 34 (37%) occurred in foreign visitors, and 59 (63%) occurred in U.S. residents, 53 (90%) of whom were eligible for vaccination but were unvaccinated or whose vaccination status was unknown. One (2%) case in a U.S. resident occurred in a person too young for vaccination, two (3%) in persons who had previously received 1 MMR vaccine dose, and three (5%) in persons who had previously received 2 MMR vaccine doses. The most common source for internationally imported cases during the study period were the Eastern Mediterranean (48) and African (24) WHO regions. During the first quarter of 2024, a total of six internationally imported cases were reported from the European and South-East Asia WHO regions, representing a 50% increase over the mean number of importations from these regions during 2020–2023 (mean of two importations per year from each region).
Surveillance Quality Indicators
Overall, all but two of the 338 case investigations included information on ≥80% of the critical surveillance indicators; those two case investigations included information on 70% of critical surveillance indicators. Date of first case report to a health department was available for 219 (65%) case investigations; 127 (58%) cases were reported to health departments on or before the day of rash onset (IQR = 4 days before to 3 days after). Overall, 314 (93%) measles cases were laboratory confirmed, including 16 (5%) by immunoglobulin M (serologic) testing alone and 298 (95%) by real-time reverse transcription–polymerase chain reaction (rRT-PCR). Among 298 rRT-PCR–positive specimens, 221 (74%) were successfully genotyped: 177 (80%) were genotype B3, and 44 (20%) were genotype D8. Twenty-two distinct sequence identifiers (DSIds) ( 3 ) for genotype B3 and 13 DSIds for genotype D8 were detected (Supplementary Figure, https://stacks.cdc.gov/view/cdc/152776 ). The longest period of detection for any DSId was 15 weeks (DSId 8346).
Chains of Transmission
The 338 measles cases were categorized into 92 transmission chains (Table); 62 (67%) were isolated cases, 10 (11%) were two-case chains, and 20 (22%) were outbreaks of three or more cases. Seven (35%) of 20 outbreaks occurred during 2024. §§ The median outbreak size was six cases (range = three–86 cases) and median duration of transmission was 20 days (range = 6–63 days). Among the 30 two-case chains and outbreaks, more than one maximum incubation period (21 days) did not elapse between any two cases.
Because of the absence of endemic measles virus transmission for 12 consecutive months in the presence of a well-performing surveillance system, as of the end of 2023, measles elimination has been maintained in the United States. U.S. measles elimination reduces the number of cases, deaths, and costs that would occur if endemic measles transmission were reestablished. Investigation of almost all U.S. measles cases reported since January 2020 were import-associated, included complete information on critical surveillance variables, were laboratory-confirmed by rRT-PCR, and underwent genotyping; these findings indicate that the U.S. measles surveillance system is performing well. A variety of transmission chain sizes were detected, including isolated cases, suggesting that sustained measles transmission would be rapidly detected. However, the rapid increase in the number of reported measles cases during the first quarter of 2024 represents a renewed threat to elimination.
Most measles importations were cases among persons traveling to and from countries in the Eastern Mediterranean and African WHO regions; these regions experienced the highest reported measles incidence among all WHO regions during 2021–2022 ( 6 ). During November 2022–October 2023, the number of countries reporting large or disruptive outbreaks increased by 123%, from 22 to 49. Global estimates suggest that first-dose measles vaccination coverage had declined from 86% in 2019 to 83% in 2022, leaving almost 22 million children aged <1 year susceptible to measles ( 6 ).
As has been the case in previous postelimination years ( 7 ), most imported measles cases occurred among unvaccinated U.S. residents. Increasing global measles incidence and decreasing vaccination coverage will increase the risk for importations into U.S. communities, as has been observed during the first quarter of 2024, further supporting CDC’s recommendation for persons to receive MMR vaccine before international travel ( 4 ).
Maintaining high national and local MMR vaccination coverage remains central to sustaining measles elimination. Risk for widespread U.S. measles transmission remains low because of high population immunity; however, national 2-dose MMR vaccination coverage has remained below the Healthy People 2030 target of 95% (the estimated population-level immunity necessary to prevent sustained measles transmission) ( 8 ) for 3 consecutive years, leaving approximately 250,000 kindergarten children susceptible to measles each year ( 9 ). Furthermore, 2-dose MMR vaccination coverage estimates in 12 states and the District of Columbia were <90%, and during the 2022–23 school year, exemption rates among kindergarten children exceeded 5% in 10 states ( 9 ). Clusters of unvaccinated persons placed communities at risk for large outbreaks, as occurred during the central Ohio outbreak in 2022: 94% of measles patients were unvaccinated and 42% were hospitalized ( 10 ). Monitoring MMR vaccination coverage at county and zip code levels could help public health agencies identify undervaccinated communities for targeted interventions to improve vaccination coverage while preparing for possible measles outbreaks. As of March 28, 2024, a total of 97 confirmed measles cases have been reported in the United States in 2024, compared with a mean of five cases during the first quarter of each year during 2020–2023. Similar to cases reported during 2020–2023, most cases reported during 2024 occurred among patients aged <20 years who were unvaccinated or whose vaccination status was unknown, and were associated with an importation. Rapid detection of cases, prompt implementation of control measures, and maintenance of high national measles vaccination coverage, including improving coverage in undervaccinated populations, is essential to preventing measles and its complications and to maintaining U.S. elimination status.
Limitations
The findings in this report are subject to at least three limitations. First, importations might have been underreported: 4% of reported cases during the study period had no known source. Second, case investigations resulting in discarded measles cases (i.e., a diagnosis of measles excluded) are not nationally reportable, which limits the ability to directly evaluate the sensitivity of measles case investigations. However, surveillance remains sufficiently sensitive to detect isolated cases and outbreaks, and robust molecular epidemiology provides further evidence supporting the absence of sustained measles transmission in the United States. Finally, the date of first case report to a health department was not available for 35% of case investigations.
Implications for Public Health Practice
The U.S. measles elimination status will continue to be threatened by global increases in measles incidence and decreases in global, national, and local measles vaccination coverage. Because of high population immunity, the risk of widespread measles transmission in the United States remains low; however, efforts are needed to increase routine MMR vaccination coverage, encourage vaccination before international travel, identify communities at risk for measles transmission, and rapidly investigate suspected measles cases to maintain elimination.
Corresponding author: Adria D. Mathis, [email protected] .
1 Division of Viral Diseases, National Center for Immunization and Respiratory Diseases, CDC.
All authors have completed and submitted the International Committee of Medical Journal Editors form for disclosure of potential conflicts of interest. Stephen N. Crooke reports institutional support from PATH. No other potential conflicts of interest were disclosed.
* Elimination is defined as the absence of endemic measles virus transmission in a defined geographic area for ≥12 months in the presence of a well-performing surveillance system.
† https://emergency.cdc.gov/han/2024/han00504.asp
§ A confirmed measles case was defined as an acute febrile rash illness with laboratory confirmation or direct epidemiologic linkage to a laboratory-confirmed case. Laboratory confirmation was defined as detection of measles virus–specific nucleic acid from a clinical specimen using real-time reverse transcription–polymerase chain reaction or a positive serologic test for measles immunoglobulin M antibody.
¶ Genotyping was performed at CDC and at the Vaccine Preventable Disease Reference Centers of the Association of Public Health Laboratories.
** 45 C.F.R. part 46.102(l)(2), 21 C.F.R. part 56; 42 U.S.C. Sect. 241(d); 5 U.S.C. Sect. 552a; 44 U.S.C. Sect. 3501 et seq.
†† MMR vaccine is not licensed for use in persons aged <6 months.
§§ At the time of this report, six measles outbreaks have ended, and one outbreak is ongoing. A measles outbreak is considered to be over when no new cases have been identified during two incubation periods (42 days) since the rash onset in the last outbreak-related case.
- Gastañaduy PA, Redd SB, Clemmons NS, et al. Measles [Chapter 7]. In: Manual for the surveillance of vaccine-preventable diseases. Atlanta, GA: US Department of Health and Human Services, CDC; 2023. https://www.cdc.gov/vaccines/pubs/surv-manual/chpt07-measles.html
- Mathis AD, Clemmons NS, Redd SB, et al. Maintenance of measles elimination status in the United States for 20 years despite increasing challenges. Clin Infect Dis 2022;75:416–24. https://doi.org/10.1093/cid/ciab979 PMID:34849648
- Williams D, Penedos A, Bankamp B, et al. Update: circulation of active genotypes of measles virus and recommendations for use of sequence analysis to monitor viral transmission. Weekly Epidemiologic Record 2022;97(39):481–92. https://reliefweb.int/report/world/weekly-epidemiological-record-wer-30-september-2022-vol-97-no-39-2022-pp-481-492-enfr
- McLean HQ, Fiebelkorn AP, Temte JL, Wallace GS; CDC. Prevention of measles, rubella, congenital rubella syndrome, and mumps, 2013: summary recommendations of the Advisory Committee on Immunization Practices (ACIP). MMWR Recomm Rep 2013;62(No. RR-4):1–34. PMID:23760231
- World Health Organization. Measles: vaccine preventable diseases surveillance standards. Geneva, Switzerland: World Health Organization; 2018. https://www.who.int/publications/m/item/vaccine-preventable-diseases-surveillance-standards-measles
- Minta AA, Ferrari M, Antoni S, et al. Progress toward measles elimination—worldwide, 2000–2022. MMWR Morb Mortal Wkly Rep 2023;72:1262–8. https://doi.org/10.15585/mmwr.mm7246a3 PMID:37971951
- Lee AD, Clemmons NS, Patel M, Gastañaduy PA. International importations of measles virus into the United States during the postelimination era, 2001–2016. J Infect Dis 2019;219:1616–23. https://doi.org/10.1093/infdis/jiy701 PMID:30535027
- Truelove SA, Graham M, Moss WJ, Metcalf CJE, Ferrari MJ, Lessler J. Characterizing the impact of spatial clustering of susceptibility for measles elimination. Vaccine 2019;37:732–41. https://doi.org/10.1016/j.vaccine.2018.12.012 PMID:30579756
- Seither R, Yusuf OB, Dramann D, Calhoun K, Mugerwa-Kasujja A, Knighton CL. Coverage with selected vaccines and exemption from school vaccine requirements among children in kindergarten—United States, 2022–23 school year. MMWR Morb Mortal Wkly Rep 2023;72:1217–24. https://doi.org/10.15585/mmwr.mm7245a2 PMID:37943705
- Tiller EC, Masters NB, Raines KL, et al. Notes from the field: measles outbreak—central Ohio, 2022–2023. MMWR Morb Mortal Wkly Rep 2023;72:847–9. https://doi.org/10.15585/mmwr.mm7231a3 PMID:37535476
FIGURE . Confirmed measles cases, by month of rash onset (N = 338) — United States, January 1, 2020–March 28, 2024
Abbreviations: IgM = immunoglobulin M; rRT-PCR = real-time reverse transcription–polymerase chain reaction; WHO = World Health Organization. * A case resulting from exposure to measles virus outside the United States as evidenced by at least some of the exposure period (7–21 days before rash onset) occurring outside the United States and rash onset occurring within 21 days of entering the United States without known exposure to measles during that time. † A case in a transmission chain epidemiologically linked to an internationally imported case. § A case for which an epidemiologic link to an internationally imported case was not identified, but for which viral sequence data indicate an imported measles genotype (i.e., a genotype that is not detected in the United States with a pattern indicative of endemic transmission). ¶ A case for which an epidemiologic or virologic link to importation or to endemic transmission within the United States cannot be established after a thorough investigation. ** Percentage is percentage of international importations. Four cases among persons who traveled to both the Eastern Mediterranean and African regions and one case in a person who traveled to both the Eastern Mediterranean and European regions were counted twice. †† Place of residence, sex, age or date of birth, fever and rash, date of rash onset, vaccination status, travel history, hospitalization, transmission setting, and whether the case was outbreak related. §§ Includes 65 cases among patients who received both positive rRT-PCR and positive IgM results. ¶¶ Percentage is percentage of total chains.
Suggested citation for this article: Mathis AD, Raines K, Masters NB, et al. Measles — United States, January 1, 2020–March 28, 2024. MMWR Morb Mortal Wkly Rep 2024;73:295–300. DOI: http://dx.doi.org/10.15585/mmwr.mm7314a1 .
MMWR and Morbidity and Mortality Weekly Report are service marks of the U.S. Department of Health and Human Services. Use of trade names and commercial sources is for identification only and does not imply endorsement by the U.S. Department of Health and Human Services. References to non-CDC sites on the Internet are provided as a service to MMWR readers and do not constitute or imply endorsement of these organizations or their programs by CDC or the U.S. Department of Health and Human Services. CDC is not responsible for the content of pages found at these sites. URL addresses listed in MMWR were current as of the date of publication.
All HTML versions of MMWR articles are generated from final proofs through an automated process. This conversion might result in character translation or format errors in the HTML version. Users are referred to the electronic PDF version ( https://www.cdc.gov/mmwr ) and/or the original MMWR paper copy for printable versions of official text, figures, and tables.
Exit Notification / Disclaimer Policy
- The Centers for Disease Control and Prevention (CDC) cannot attest to the accuracy of a non-federal website.
- Linking to a non-federal website does not constitute an endorsement by CDC or any of its employees of the sponsors or the information and products presented on the website.
- You will be subject to the destination website's privacy policy when you follow the link.
- CDC is not responsible for Section 508 compliance (accessibility) on other federal or private website.

IMAGES
VIDEO
COMMENTS
The VR for Quality case study detailed how one professional harnessed VR to bring quality across the globe for almost no cost. Read the Case Study. Using Exploratory Data Analysis to Improve the Fresh Foods Ordering Process in Retail Stores. ... Inventory control tools were applied using DMAIC methodology. The company went from 14 major ...
outgoing quality control works for manufacturing product. There are t wo types of part was sel ected for. this case study which are huge and symmetrical parts. 85.06 seconds total inspection time ...
T oyota Quality System case study. Introduction. T oyota from the early 1960s alongside their supplier network consolidated the way in which. they were able to refine their production system ...
According to Teresa Whitacre, of international consulting firm ASQ, proper quality management also boosts a company's profitability. "Total quality management allows the company to look at their management system as a whole entity — not just an output of the quality department," she says. "Total quality means the organisation looks at ...
Conclusion: The 7 QC tools, when combined with the systematic approach of the PDCA cycle, can yield impressive results, as demonstrated in our case study. Addressing quality issues requires a ...
Quality control is a crucial aspect of any e-commerce platform, and Amazon, being one of the largest online marketplaces, has faced significant challenges regarding counterfeit and low-quality ...
Since publication in 2003 of a review 'Internal quality control: planning and implementation strategies,' 1 quality control (QC) has evolved as part of a comprehensive quality management system (QMS). The language of quality today is defined by International Standard Organization (ISO) in an effort to standardize terminology and quality management practices for world-wide applications.
The smart quality approach allows pharma companies to deploy these technologies and to integrate their quality controls in development and manufacturing. 1 (see sidebar, "Smart quality at a glance"). Well-performing manufacturing facilities have started to create paperless labs, optimize testing, automate processes, and shift testing to the ...
This case study (PDF, 1.2 MB) focuses on the successful quality improvement methods employed by a health plan to improve customer service for its members. Published by RAND, August 2007. Published by RAND, August 2007.
by by Jim Heskett. A new book by Gregory Clark identifies "labor quality" as the major enticement for capital flows that lead to economic prosperity. By defining labor quality in terms of discipline and attitudes toward work, this argument minimizes the long-term threat of outsourcing to developed economies.
Shiseido is a globally-renowned manufacturer of cosmetics and fragrances. The company carries out intensive research and development activity on a continual basis, bringing multiple new product references to market every year. In this case study we discuss why Shiseido began using CHEMUNEX® for quality control of bulk raw materials and in ...
For uie major companies in the industry, these outcomes are dependent upon the quality of sorting and loading at central loading facilities located throughout the United States. In order to improve quality control in sorting and loading, a competitive program of feedback and positive reinforcement was implemented using a baseball analog system.
This paper studies a prediction problem using time series data and machine learning algorithms. The case study is related to the quality control of bumper beams in the automotive industry. These parts are milled during the production process, and the locations of the milled holes are subject to strict tolerance limits. Machine learning models are used to predict the location of milled holes in ...
The successfulness of this system can be measured by assessing the consistency of the product quality. Coca Cola say that 'Our Company's Global Product Quality Index rating has consistently reached averages near 94 since 2007, with a 94.3 in 2010, while our Company Global Package Quality Index has steadily increased since 2007 to a 92.6 rating in 2010, our highest value to date'.
Manage Your Human Sigma. Organizational Development Magazine Article. John H. Fleming. Curt Coffman. James K. Harter. If sales and service organizations are to improve, they must learn to measure ...
International Journal of Science, Engineering and Technology Research (IJSETR), Volume 3, Issue 3, March 2014 A CASE STUDY OF QUALITY CONTROL CHARTS IN A MANUFACTURING INDUSTRY Fahim Ahmed Touqir1, Md. Maksudul Islam1, Lipon Kumar Sarkar2 1,2 Department of Industrial Engineering and Management 1,2 Khulna University of Engineering & Technology II.
Summary. Case Study: Connecting Cloud-based Quality Control to the Edge. Machine vision systems can recognize superficial aberrations in manufactured goods—like lines, spots, holes, color discrepancies and more—that would typically require time-consuming manual inspection. However, these systems require specialized programming and ...
A top 5 pharma company chose to implement a strong in-process testing routine strategically monitoring specific critical steps of their production process. This approach was designed to detect any microbial contamination at the earliest possible opportunity and has been key to maintaining superior control over their production processes.
For case-control studies, it is important that if matching was performed during the selection or recruitment process, the variables used as matching criteria (e.g., age, gender, race) should be controlled for in the analysis. General Guidance for Determining the Overall Quality Rating of Case-Controlled Studies
In this paper, statistical quality control of a production line has been presented using the classical method Shewhart, cumulative sum method (CUSUM) and Exponentially Weighted Moving Average (EWMA). The Shewhart technique can be utilized in controlling the process in which there are big changes in mean. The cumulative sum method is more efficient in detecting small changes in the mean. The ...
Case Studies Highlight Why Researchers Use Automated Electrophoresis Solutions. Many scientists utilize Agilent automated electrophoresis systems for sample quality control (QC) in various application workflows, including next-generation sequencing (NGS), biobanking, fragment analysis, and nucleic acid vaccine development.
Statistics. Quality control time without drive time: 5 to 10 minutes. Quality control time with drive time: 15 to 20 minutes. Golden Rule Auto Care's average: 12 minutes. KPI or goal for Golden Rule Auto Care's QC issues per month: 10% or less. This year, they have run as high as 25% and as low as 9% per month.
Quality in construction industry means that the constructed building can achieve its target in terms of workability if it meets the standards as requested. The tools to ensure the quality of the building are by having Quality Assurance (QA) and Quality Control (QC) Quality Assurance and Quality Control (QA/QC) is a tool of determining the
This study primarily aims to create an automated system for assessing the quality of 3D BIM model data, utilizing a proposed project quality control checklist. The automated system consists of four key elements: a BIM-based model, a Data Extraction and Analysis Module, a Data Storage Module, and a Data Visualization Module.
Finally, to illustrate the effectiveness of our Bayesian Max-EWMA control chart under various Loss Functions (LFs) for a Weibull process, we present a practical case study focusing on the hard ...
How cutting back infused higher quality in transaction monitoring. 11-Jul-2023 Ron V. Giammarco . About us. At EY, our purpose is building a better working world. The insights and services we provide help to create long-term value for clients, people and society, and to build trust in the capital markets. ... In this case study, learn how a ...
The COVID-19 pandemic has presented unprecedented public health challenges worldwide. Understanding the factors contributing to COVID-19 mortality is critical for effective management and intervention strategies. This study aims to unlock the predictive power of data collected from personal, clinical, preclinical, and laboratory variables through machine learning (ML) analyses.
Antimicrobial resistance is a major threat to human health. The widespread inappropriate use of antimicrobial substances drives resistance development at the individual and population level [1, 2].Infections due to resistant pathogens are responsible for a high healthcare burden and are estimated to cause over 700.000 deaths annually worldwide- a rate that is projected to rise to 10 million ...
Study population. This study was conducted using the case-control studies. Men attending the outpatient clinic of the Reproductive Fertility Centre of the First Affiliated Hospital of Xinjiang Medical University were recruited during the period of December 2020 to August 2023, with infertile men in the case group (n = 250) and men with no obvious male factor in the control group matched on a 1 ...
Introduction. Measles is a highly infectious acute, febrile rash illness with a >90% secondary attack rate among susceptible contacts (1).High national 2-dose coverage with the measles, mumps, and rubella (MMR) vaccine led to the declaration of U.S. measles elimination* in 2000 (2).However, this elimination status was threatened in 2019 because of two prolonged outbreaks among undervaccinated ...