Research Hypothesis In Psychology: Types, & Examples
Saul Mcleod, PhD
Editor-in-Chief for Simply Psychology
BSc (Hons) Psychology, MRes, PhD, University of Manchester
Saul Mcleod, PhD., is a qualified psychology teacher with over 18 years of experience in further and higher education. He has been published in peer-reviewed journals, including the Journal of Clinical Psychology.
Learn about our Editorial Process
Olivia Guy-Evans, MSc
Associate Editor for Simply Psychology
BSc (Hons) Psychology, MSc Psychology of Education
Olivia Guy-Evans is a writer and associate editor for Simply Psychology. She has previously worked in healthcare and educational sectors.
On This Page:
A research hypothesis, in its plural form “hypotheses,” is a specific, testable prediction about the anticipated results of a study, established at its outset. It is a key component of the scientific method .
Hypotheses connect theory to data and guide the research process towards expanding scientific understanding

Some key points about hypotheses:
- A hypothesis expresses an expected pattern or relationship. It connects the variables under investigation.
- It is stated in clear, precise terms before any data collection or analysis occurs. This makes the hypothesis testable.
- A hypothesis must be falsifiable. It should be possible, even if unlikely in practice, to collect data that disconfirms rather than supports the hypothesis.
- Hypotheses guide research. Scientists design studies to explicitly evaluate hypotheses about how nature works.
- For a hypothesis to be valid, it must be testable against empirical evidence. The evidence can then confirm or disprove the testable predictions.
- Hypotheses are informed by background knowledge and observation, but go beyond what is already known to propose an explanation of how or why something occurs.
Predictions typically arise from a thorough knowledge of the research literature, curiosity about real-world problems or implications, and integrating this to advance theory. They build on existing literature while providing new insight.
Types of Research Hypotheses
Alternative hypothesis.
The research hypothesis is often called the alternative or experimental hypothesis in experimental research.
It typically suggests a potential relationship between two key variables: the independent variable, which the researcher manipulates, and the dependent variable, which is measured based on those changes.
The alternative hypothesis states a relationship exists between the two variables being studied (one variable affects the other).
A hypothesis is a testable statement or prediction about the relationship between two or more variables. It is a key component of the scientific method. Some key points about hypotheses:
- Important hypotheses lead to predictions that can be tested empirically. The evidence can then confirm or disprove the testable predictions.
In summary, a hypothesis is a precise, testable statement of what researchers expect to happen in a study and why. Hypotheses connect theory to data and guide the research process towards expanding scientific understanding.
An experimental hypothesis predicts what change(s) will occur in the dependent variable when the independent variable is manipulated.
It states that the results are not due to chance and are significant in supporting the theory being investigated.
The alternative hypothesis can be directional, indicating a specific direction of the effect, or non-directional, suggesting a difference without specifying its nature. It’s what researchers aim to support or demonstrate through their study.
Null Hypothesis
The null hypothesis states no relationship exists between the two variables being studied (one variable does not affect the other). There will be no changes in the dependent variable due to manipulating the independent variable.
It states results are due to chance and are not significant in supporting the idea being investigated.
The null hypothesis, positing no effect or relationship, is a foundational contrast to the research hypothesis in scientific inquiry. It establishes a baseline for statistical testing, promoting objectivity by initiating research from a neutral stance.
Many statistical methods are tailored to test the null hypothesis, determining the likelihood of observed results if no true effect exists.
This dual-hypothesis approach provides clarity, ensuring that research intentions are explicit, and fosters consistency across scientific studies, enhancing the standardization and interpretability of research outcomes.
Nondirectional Hypothesis
A non-directional hypothesis, also known as a two-tailed hypothesis, predicts that there is a difference or relationship between two variables but does not specify the direction of this relationship.
It merely indicates that a change or effect will occur without predicting which group will have higher or lower values.
For example, “There is a difference in performance between Group A and Group B” is a non-directional hypothesis.
Directional Hypothesis
A directional (one-tailed) hypothesis predicts the nature of the effect of the independent variable on the dependent variable. It predicts in which direction the change will take place. (i.e., greater, smaller, less, more)
It specifies whether one variable is greater, lesser, or different from another, rather than just indicating that there’s a difference without specifying its nature.
For example, “Exercise increases weight loss” is a directional hypothesis.
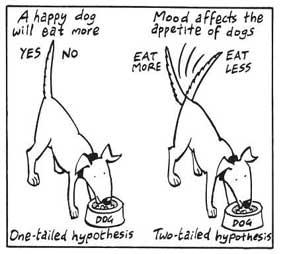
Falsifiability
The Falsification Principle, proposed by Karl Popper , is a way of demarcating science from non-science. It suggests that for a theory or hypothesis to be considered scientific, it must be testable and irrefutable.
Falsifiability emphasizes that scientific claims shouldn’t just be confirmable but should also have the potential to be proven wrong.
It means that there should exist some potential evidence or experiment that could prove the proposition false.
However many confirming instances exist for a theory, it only takes one counter observation to falsify it. For example, the hypothesis that “all swans are white,” can be falsified by observing a black swan.
For Popper, science should attempt to disprove a theory rather than attempt to continually provide evidence to support a research hypothesis.
Can a Hypothesis be Proven?
Hypotheses make probabilistic predictions. They state the expected outcome if a particular relationship exists. However, a study result supporting a hypothesis does not definitively prove it is true.
All studies have limitations. There may be unknown confounding factors or issues that limit the certainty of conclusions. Additional studies may yield different results.
In science, hypotheses can realistically only be supported with some degree of confidence, not proven. The process of science is to incrementally accumulate evidence for and against hypothesized relationships in an ongoing pursuit of better models and explanations that best fit the empirical data. But hypotheses remain open to revision and rejection if that is where the evidence leads.
- Disproving a hypothesis is definitive. Solid disconfirmatory evidence will falsify a hypothesis and require altering or discarding it based on the evidence.
- However, confirming evidence is always open to revision. Other explanations may account for the same results, and additional or contradictory evidence may emerge over time.
We can never 100% prove the alternative hypothesis. Instead, we see if we can disprove, or reject the null hypothesis.
If we reject the null hypothesis, this doesn’t mean that our alternative hypothesis is correct but does support the alternative/experimental hypothesis.
Upon analysis of the results, an alternative hypothesis can be rejected or supported, but it can never be proven to be correct. We must avoid any reference to results proving a theory as this implies 100% certainty, and there is always a chance that evidence may exist which could refute a theory.
How to Write a Hypothesis
- Identify variables . The researcher manipulates the independent variable and the dependent variable is the measured outcome.
- Operationalized the variables being investigated . Operationalization of a hypothesis refers to the process of making the variables physically measurable or testable, e.g. if you are about to study aggression, you might count the number of punches given by participants.
- Decide on a direction for your prediction . If there is evidence in the literature to support a specific effect of the independent variable on the dependent variable, write a directional (one-tailed) hypothesis. If there are limited or ambiguous findings in the literature regarding the effect of the independent variable on the dependent variable, write a non-directional (two-tailed) hypothesis.
- Make it Testable : Ensure your hypothesis can be tested through experimentation or observation. It should be possible to prove it false (principle of falsifiability).
- Clear & concise language . A strong hypothesis is concise (typically one to two sentences long), and formulated using clear and straightforward language, ensuring it’s easily understood and testable.
Consider a hypothesis many teachers might subscribe to: students work better on Monday morning than on Friday afternoon (IV=Day, DV= Standard of work).
Now, if we decide to study this by giving the same group of students a lesson on a Monday morning and a Friday afternoon and then measuring their immediate recall of the material covered in each session, we would end up with the following:
- The alternative hypothesis states that students will recall significantly more information on a Monday morning than on a Friday afternoon.
- The null hypothesis states that there will be no significant difference in the amount recalled on a Monday morning compared to a Friday afternoon. Any difference will be due to chance or confounding factors.
More Examples
- Memory : Participants exposed to classical music during study sessions will recall more items from a list than those who studied in silence.
- Social Psychology : Individuals who frequently engage in social media use will report higher levels of perceived social isolation compared to those who use it infrequently.
- Developmental Psychology : Children who engage in regular imaginative play have better problem-solving skills than those who don’t.
- Clinical Psychology : Cognitive-behavioral therapy will be more effective in reducing symptoms of anxiety over a 6-month period compared to traditional talk therapy.
- Cognitive Psychology : Individuals who multitask between various electronic devices will have shorter attention spans on focused tasks than those who single-task.
- Health Psychology : Patients who practice mindfulness meditation will experience lower levels of chronic pain compared to those who don’t meditate.
- Organizational Psychology : Employees in open-plan offices will report higher levels of stress than those in private offices.
- Behavioral Psychology : Rats rewarded with food after pressing a lever will press it more frequently than rats who receive no reward.

Related Articles
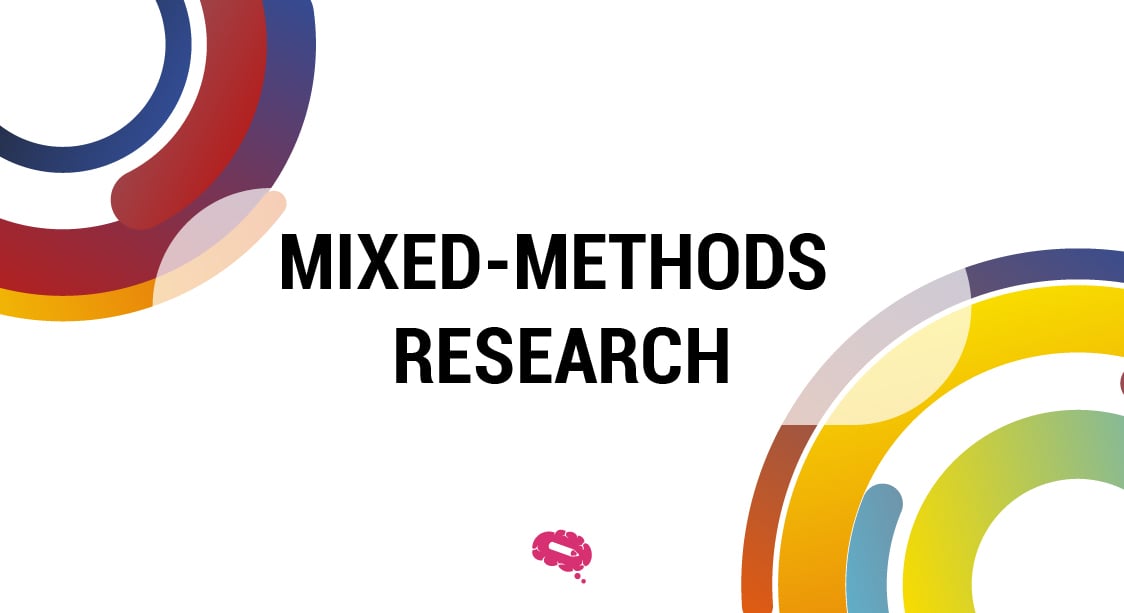
Research Methodology
Mixed Methods Research
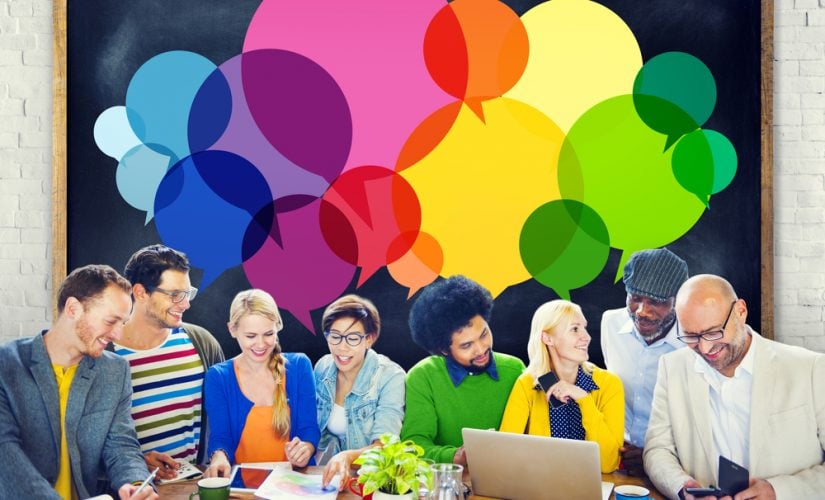
Conversation Analysis
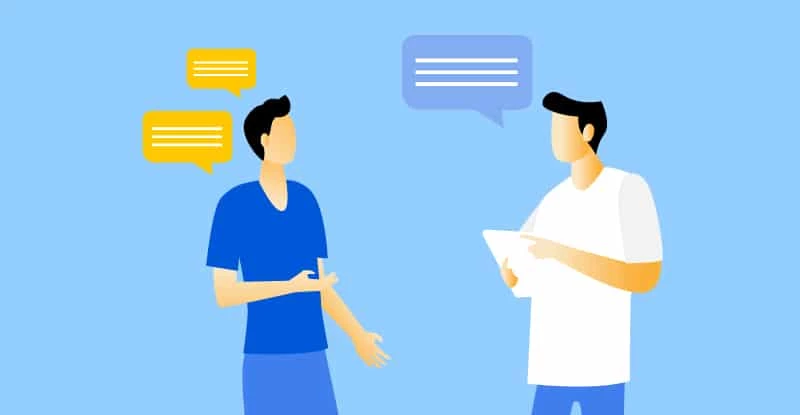
Discourse Analysis
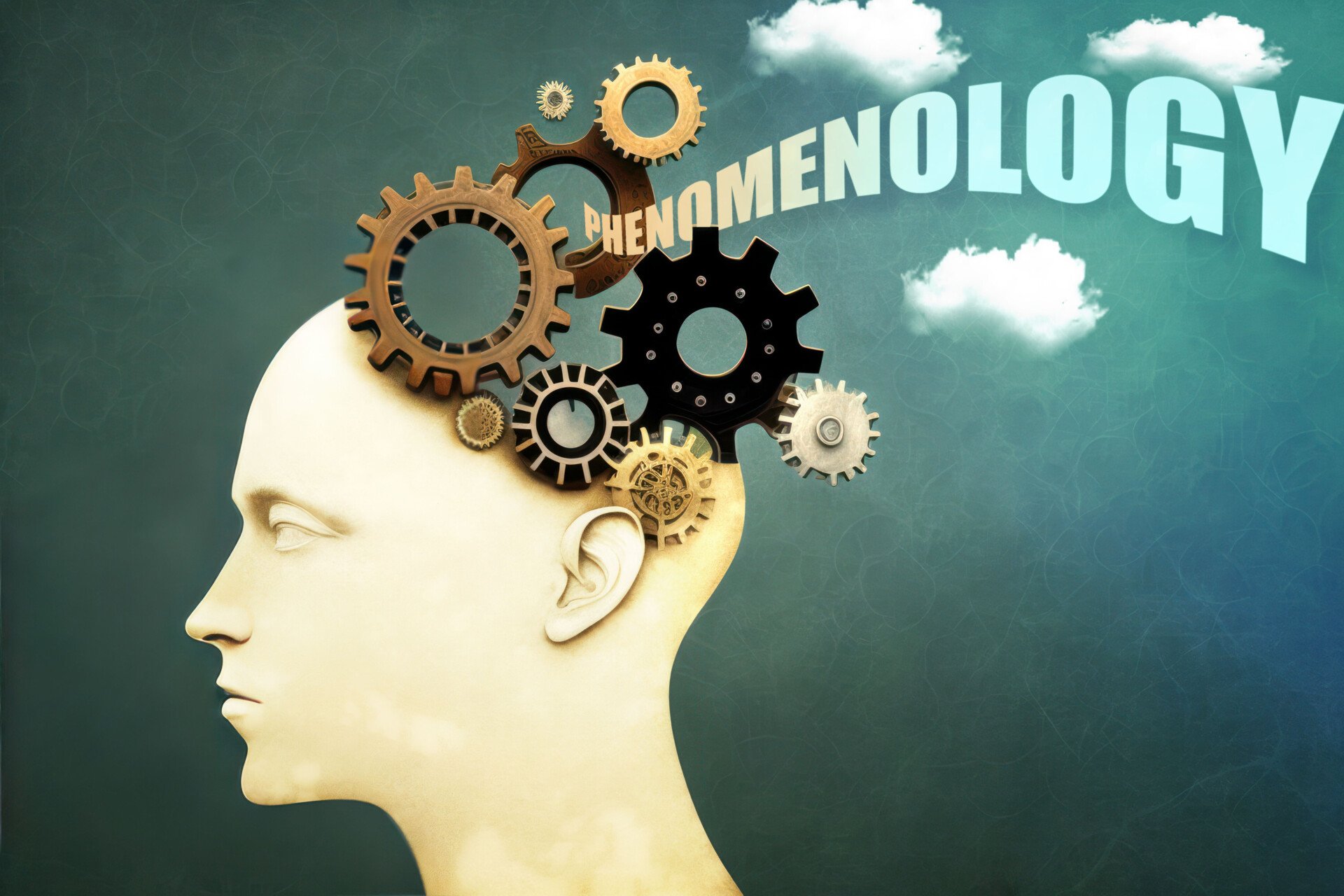
Phenomenology In Qualitative Research
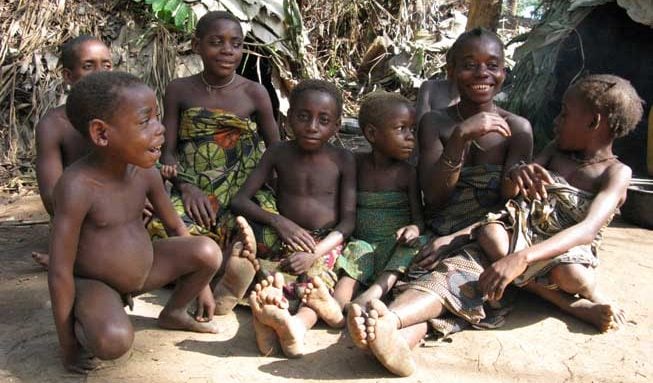
Ethnography In Qualitative Research
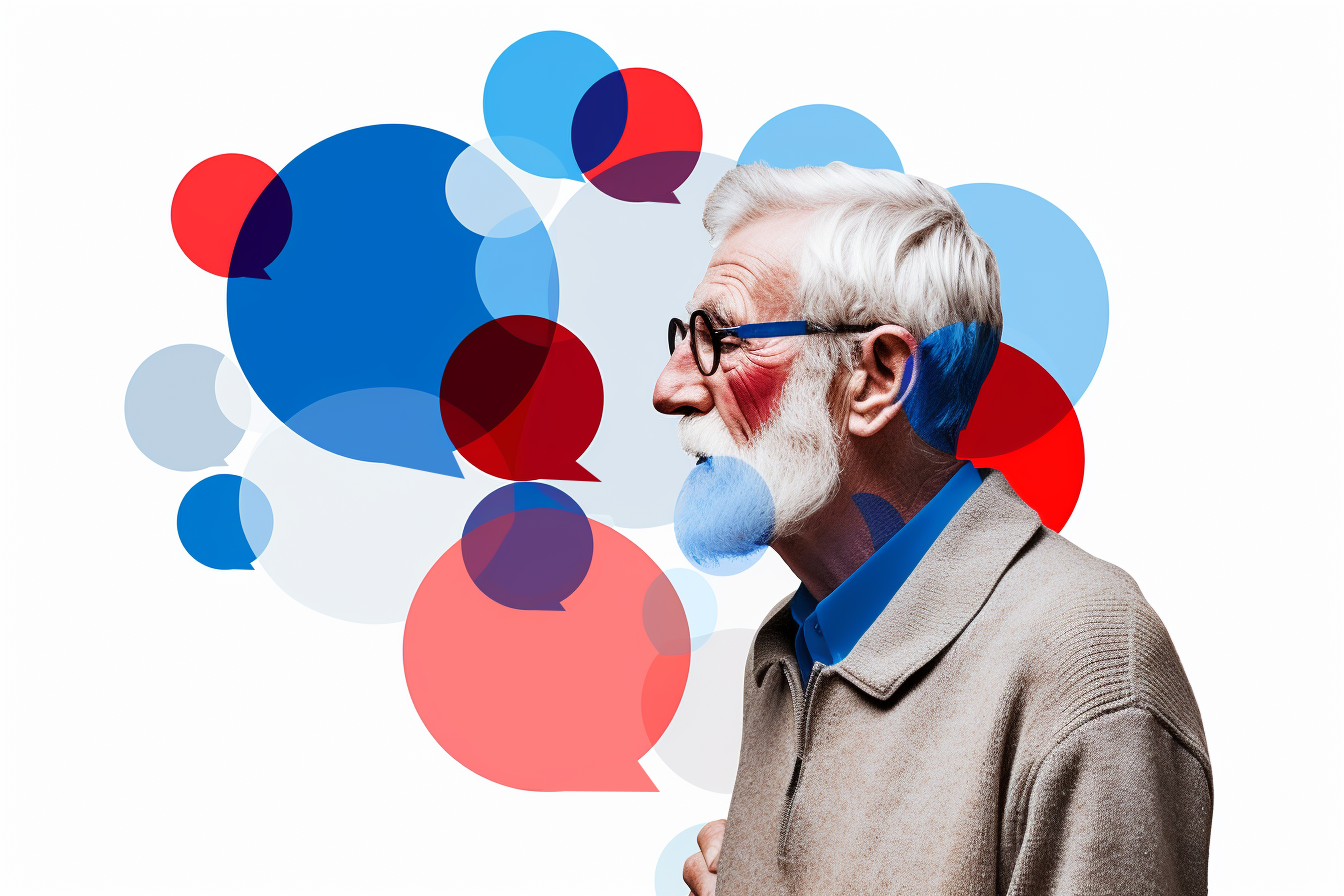
Narrative Analysis In Qualitative Research
Directional and non-directional hypothesis: A Comprehensive Guide
Karolina Konopka
Customer support manager
Want to talk with us?
In the world of research and statistical analysis, hypotheses play a crucial role in formulating and testing scientific claims. Understanding the differences between directional and non-directional hypothesis is essential for designing sound experiments and drawing accurate conclusions. Whether you’re a student, researcher, or simply curious about the foundations of hypothesis testing, this guide will equip you with the knowledge and tools to navigate this fundamental aspect of scientific inquiry.
Understanding Directional Hypothesis
Understanding directional hypotheses is crucial for conducting hypothesis-driven research, as they guide the selection of appropriate statistical tests and aid in the interpretation of results. By incorporating directional hypotheses, researchers can make more precise predictions, contribute to scientific knowledge, and advance their fields of study.
Definition of directional hypothesis
Directional hypotheses, also known as one-tailed hypotheses, are statements in research that make specific predictions about the direction of a relationship or difference between variables. Unlike non-directional hypotheses, which simply state that there is a relationship or difference without specifying its direction, directional hypotheses provide a focused and precise expectation.
A directional hypothesis predicts either a positive or negative relationship between variables or predicts that one group will perform better than another. It asserts a specific direction of effect or outcome. For example, a directional hypothesis could state that “increased exposure to sunlight will lead to an improvement in mood” or “participants who receive the experimental treatment will exhibit higher levels of cognitive performance compared to the control group.”
Directional hypotheses are formulated based on existing theory, prior research, or logical reasoning, and they guide the researcher’s expectations and analysis. They allow for more targeted predictions and enable researchers to test specific hypotheses using appropriate statistical tests.
The role of directional hypothesis in research
Directional hypotheses also play a significant role in research surveys. Let’s explore their role specifically in the context of survey research:
- Objective-driven surveys : Directional hypotheses help align survey research with specific objectives. By formulating directional hypotheses, researchers can focus on gathering data that directly addresses the predicted relationship or difference between variables of interest.
- Question design and measurement : Directional hypotheses guide the design of survey question types and the selection of appropriate measurement scales. They ensure that the questions are tailored to capture the specific aspects related to the predicted direction, enabling researchers to obtain more targeted and relevant data from survey respondents.
- Data analysis and interpretation : Directional hypotheses assist in data analysis by directing researchers towards appropriate statistical tests and methods. Researchers can analyze the survey data to specifically test the predicted relationship or difference, enhancing the accuracy and reliability of their findings. The results can then be interpreted within the context of the directional hypothesis, providing more meaningful insights.
- Practical implications and decision-making : Directional hypotheses in surveys often have practical implications. When the predicted relationship or difference is confirmed, it informs decision-making processes, program development, or interventions. The survey findings based on directional hypotheses can guide organizations, policymakers, or practitioners in making informed choices to achieve desired outcomes.
- Replication and further research : Directional hypotheses in survey research contribute to the replication and extension of studies. Researchers can replicate the survey with different populations or contexts to assess the generalizability of the predicted relationships. Furthermore, if the directional hypothesis is supported, it encourages further research to explore underlying mechanisms or boundary conditions.
By incorporating directional hypotheses in survey research, researchers can align their objectives, design effective surveys, conduct focused data analysis, and derive practical insights. They provide a framework for organizing survey research and contribute to the accumulation of knowledge in the field.
Examples of research questions for directional hypothesis
Here are some examples of research questions that lend themselves to directional hypotheses:
- Does increased daily exercise lead to a decrease in body weight among sedentary adults?
- Is there a positive relationship between study hours and academic performance among college students?
- Does exposure to violent video games result in an increase in aggressive behavior among adolescents?
- Does the implementation of a mindfulness-based intervention lead to a reduction in stress levels among working professionals?
- Is there a difference in customer satisfaction between Product A and Product B, with Product A expected to have higher satisfaction ratings?
- Does the use of social media influence self-esteem levels, with higher social media usage associated with lower self-esteem?
- Is there a negative relationship between job satisfaction and employee turnover, indicating that lower job satisfaction leads to higher turnover rates?
- Does the administration of a specific medication result in a decrease in symptoms among individuals with a particular medical condition?
- Does increased access to early childhood education lead to improved cognitive development in preschool-aged children?
- Is there a difference in purchase intention between advertisements with celebrity endorsements and advertisements without, with celebrity endorsements expected to have a higher impact?
These research questions generate specific predictions about the direction of the relationship or difference between variables and can be tested using appropriate research methods and statistical analyses.
Definition of non-directional hypothesis
Non-directional hypotheses, also known as two-tailed hypotheses, are statements in research that indicate the presence of a relationship or difference between variables without specifying the direction of the effect. Instead of making predictions about the specific direction of the relationship or difference, non-directional hypotheses simply state that there is an association or distinction between the variables of interest.
Non-directional hypotheses are often used when there is no prior theoretical basis or clear expectation about the direction of the relationship. They leave the possibility open for either a positive or negative relationship, or for both groups to differ in some way without specifying which group will perform better or worse.
Advantages and utility of non-directional hypothesis
Non-directional hypotheses in survey s offer several advantages and utilities, providing flexibility and comprehensive analysis of survey data. Here are some of the key advantages and utilities of using non-directional hypotheses in surveys:
- Exploration of Relationships : Non-directional hypotheses allow researchers to explore and examine relationships between variables without assuming a specific direction. This is particularly useful in surveys where the relationship between variables may not be well-known or there may be conflicting evidence regarding the direction of the effect.
- Flexibility in Question Design : With non-directional hypotheses, survey questions can be designed to measure the relationship between variables without being biased towards a particular outcome. This flexibility allows researchers to collect data and analyze the results more objectively.
- Open to Unexpected Findings : Non-directional hypotheses enable researchers to be open to unexpected or surprising findings in survey data. By not committing to a specific direction of the effect, researchers can identify and explore relationships that may not have been initially anticipated, leading to new insights and discoveries.
- Comprehensive Analysis : Non-directional hypotheses promote comprehensive analysis of survey data by considering the possibility of an effect in either direction. Researchers can assess the magnitude and significance of relationships without limiting their analysis to only one possible outcome.
- S tatistical Validity : Non-directional hypotheses in surveys allow for the use of two-tailed statistical tests, which provide a more conservative and robust assessment of significance. Two-tailed tests consider both positive and negative deviations from the null hypothesis, ensuring accurate and reliable statistical analysis of survey data.
- Exploratory Research : Non-directional hypotheses are particularly useful in exploratory research, where the goal is to gather initial insights and generate hypotheses. Surveys with non-directional hypotheses can help researchers explore various relationships and identify patterns that can guide further research or hypothesis development.
It is worth noting that the choice between directional and non-directional hypotheses in surveys depends on the research objectives, existing knowledge, and the specific variables being investigated. Researchers should carefully consider the advantages and limitations of each approach and select the one that aligns best with their research goals and survey design.
- Share with others
- Twitter Twitter Icon
- LinkedIn LinkedIn Icon
Related posts
Top 8 email survey tools to boost your feedback, what is an ai survey question generator – how to use it, how to implement nps surveys: a step-by-step guide, 15 best website survey questions to ask your visitors, how to write a good survey introduction, 7 best ai survey generators, get answers today.
- No credit card required
- No time limit on Free plan
You can modify this template in every possible way.
All templates work great on every device.
Directional vs Non-Directional Hypothesis: Key Difference
In statistics, a directional hypothesis, also known as a one-tailed hypothesis, is a type of hypothesis that predicts the direction of the relationship between variables or the direction of the difference between groups.

The introduction of a directional hypothesis in a research study provides an overview of the specific prediction being made about the relationship between variables or the difference between groups. It sets the stage for the research question and outlines the expected direction of the findings. The introduction typically includes the following elements:
Research Context: Begin by introducing the general topic or research area that the study is focused on. Provide background information and highlight the significance of the research question.
Research Question: Clearly state the specific research question that the study aims to answer. This question should be directly related to the variables being investigated.
Previous Research: Summarize relevant literature or previous studies that have explored similar or related topics. This helps establish the existing knowledge base and provides a rationale for the hypothesis.
Hypothesis Statement: Present the directional hypothesis clearly and concisely. State the predicted relationship between variables or the expected difference between groups. For example, if studying the impact of a new teaching method on student performance, a directional hypothesis could be, “Students who receive the new teaching method will demonstrate higher test scores compared to students who receive the traditional teaching method.”
Justification: Provide a logical explanation for the directional hypothesis based on the existing literature or theoretical framework . Discuss any previous findings, theories, or empirical evidence that support the predicted direction of the relationship or difference.
Objectives: Outline the specific objectives or aims of the study, which should align with the research question and hypothesis. These objectives help guide the research process and provide a clear focus for the study.
By including these elements in the introduction of a research study, the directional hypothesis is introduced effectively, providing a clear and justified prediction about the expected outcome of the research.
When formulating a directional hypothesis, researchers make a specific prediction about the expected relationship or difference between variables. They specify whether they expect an increase or decrease in the dependent variable, or whether one group will score higher or lower than another group
What is Directional Hypothesis?
With a correlational study, a directional hypothesis states that there is a positive (or negative) correlation between two variables. When a hypothesis states the direction of the results, it is referred to as a directional (one-tailed) hypothesis; this is because it states that the results go in one direction.
Definition:
A directional hypothesis is a one-tailed hypothesis that states the direction of the difference or relationship (e.g. boys are more helpful than girls).
Research Question: Does exercise have a positive impact on mood?
Directional Hypothesis: Engaging in regular exercise will result in an increase in positive mood compared to a sedentary lifestyle.
In this example, the directional hypothesis predicts that regular exercise will have a specific effect on mood, specifically leading to an increase in positive mood. The researcher expects that individuals who engage in regular exercise will experience improvements in their overall mood compared to individuals who lead a sedentary lifestyle.
It’s important to note that this is just one example, and directional hypotheses can be formulated in various research areas and contexts. The key is to make a specific prediction about the direction of the relationship or difference between variables based on prior knowledge or theoretical considerations.
Advantages of Directional Hypothesis
There are several advantages to using a directional hypothesis in research studies. Here are a few key benefits:
Specific Prediction:
A directional hypothesis allows researchers to make a specific prediction about the expected relationship or difference between variables. This provides a clear focus for the study and helps guide the research process. It also allows for more precise interpretation of the results.
Testable and Refutable:
Directional hypotheses can be tested and either supported or refuted by empirical evidence. Researchers can design their study and select appropriate statistical tests to specifically examine the predicted direction of the relationship or difference. This enhances the rigor and validity of the research.
Efficiency and Resource Allocation:
By making a specific prediction, researchers can allocate their resources more efficiently. They can focus on collecting data and conducting analyses that directly test the directional hypothesis, rather than exploring all possible directions or relationships. This can save time, effort, and resources.
Theory Development:
Directional hypotheses contribute to the development of theories and scientific knowledge. When a directional hypothesis is supported by empirical evidence, it provides support for existing theories or helps generate new theories. This advancement in knowledge can guide future research and understanding in the field.
Practical Applications:
Directional hypotheses can have practical implications and applications. If a hypothesis predicts a specific direction of change, such as the effectiveness of a treatment or intervention, it can inform decision-making and guide practical applications in fields such as medicine, psychology, or education.
Enhanced Communication:
Directional hypotheses facilitate clearer communication of research findings. When researchers have made specific predictions about the direction of the relationship or difference, they can effectively communicate their results to both academic and non-academic audiences. This promotes better understanding and application of the research outcomes.
It’s important to note that while directional hypotheses offer advantages, they also require stronger evidence to support them compared to non-directional hypotheses. Researchers should carefully consider the research context, existing literature, and theoretical considerations before formulating a directional hypothesis.
Disadvantages of Directional Hypothesis
While directional hypotheses have their advantages, there are also some potential disadvantages to consider:
Risk of Type I Error:
Directional hypotheses increase the risk of committing a Type I error , also known as a false positive. By focusing on a specific predicted direction, researchers may overlook the possibility of an opposite or null effect. If the actual relationship or difference does not align with the predicted direction, researchers may incorrectly conclude that there is no effect when, in fact, there may be.
Narrow Focus:
Directional hypotheses restrict the scope of investigation to a specific predicted direction. This narrow focus may overlook other potential relationships, nuances, or alternative explanations. Researchers may miss valuable insights or unexpected findings by excluding other possibilities from consideration.
Limited Generalizability:
Directional hypotheses may limit the generalizability of findings. If the study supports the predicted direction, the results may only apply to the specific context and conditions outlined in the hypothesis. Generalizing the findings to different populations, settings, or variables may require further research.
Biased Interpretation:
Directional hypotheses can introduce bias in the interpretation of results. Researchers may be inclined to selectively focus on evidence that supports the predicted direction while downplaying or ignoring contradictory evidence. This can hinder objectivity and lead to biased conclusions.
Increased Sample Size Requirements:
Directional hypotheses often require larger sample sizes compared to non-directional hypotheses. This is because statistical power needs to be sufficient to detect the predicted direction with a reasonable level of confidence. Larger samples can be more time-consuming and resource-intensive to obtain.
Reduced Flexibility:
Directional hypotheses limit flexibility in data analysis and statistical testing. Researchers may feel compelled to use specific statistical tests or analytical approaches that align with the predicted direction, potentially overlooking alternative methods that may be more appropriate or informative.
It’s important to weigh these disadvantages against the specific research context and objectives when deciding whether to use a directional hypothesis. In some cases, a non-directional hypothesis may be more suitable, allowing for a more exploratory and comprehensive investigation of the research question.
Non-Directional Hypothesis:
A non-directional hypothesis, also known as a two-tailed hypothesis, is a type of hypothesis that does not specify the direction of the relationship between variables or the difference between groups. Instead of predicting a specific direction, a non-directional hypothesis suggests that there will be a significant relationship or difference, without indicating whether it will be positive or negative, higher or lower, etc.
The introduction of a non-directional hypothesis in a research study provides an overview of the general prediction being made about the relationship between variables or the difference between groups, without specifying the direction. It sets the stage for the research question and outlines the expectation of a significant relationship or difference. The introduction typically includes the following elements:
Research Context:
Begin by introducing the general topic or research area that the study is focused on. Provide background information and highlight the significance of the research question.
Research Question:
Clearly state the specific research question that the study aims to answer. This question should be directly related to the variables being investigated.
Previous Research:
Summarize relevant literature or previous studies that have explored similar or related topics. This helps establish the existing knowledge base and provides a rationale for the hypothesis.
Hypothesis Statement:
Present the non-directional hypothesis clearly and concisely. State that there is an expected relationship or difference between variables or groups without specifying the direction. For example, if studying the relationship between socioeconomic status and academic achievement, a non-directional hypothesis could be, “There is a significant relationship between socioeconomic status and academic achievement.”
Justification:
Provide a logical explanation for the non-directional hypothesis based on the existing literature or theoretical framework. Discuss any previous findings, theories, or empirical evidence that support the notion of a relationship or difference between the variables or groups.
Objectives:
Outline the specific objectives or aims of the study, which should align with the research question and hypothesis. These objectives help guide the research process and provide a clear focus for the study.
By including these elements in the introduction of a research study, the non-directional hypothesis is introduced effectively, indicating the expectation of a significant relationship or difference without specifying the direction
What is Non-directional hypothesis?
In a non-directional hypothesis, researchers acknowledge that there may be an effect or relationship between variables but do not make a specific prediction about the direction of that effect. This allows for a more exploratory approach to data analysis and interpretation
If a hypothesis does not state a direction but simply says that one factor affects another, or that there is an association or correlation between two variables then it is called a non-directional (two-tailed) hypothesis.
Research Question: Is there a relationship between social media usage and self-esteem ?
Non-Directional Hypothesis: There is a significant relationship between social media usage and self-esteem.
In this example, the non-directional hypothesis suggests that there is a relationship between social media usage and self-esteem without specifying whether higher social media usage is associated with higher or lower self-esteem. The hypothesis acknowledges the possibility of an effect but does not make a specific prediction about the direction of that effect.
It’s important to note that this is just one example, and non-directional hypotheses can be formulated in various research areas and contexts. The key is to indicate the expectation of a significant relationship or difference without specifying the direction, allowing for a more exploratory approach to data analysis and interpretation.
Advantages of Non-directional hypothesis
Non-directional hypotheses, also known as two-tailed hypotheses, offer several advantages in research studies. Here are some of the key advantages:
Flexibility in Data Analysis:
Non-directional hypotheses allow for flexibility in data analysis. Researchers are not constrained by a specific predicted direction and can explore the relationship or difference in various ways. This flexibility enables a more comprehensive examination of the data, considering both positive and negative associations or differences.
Objective and Open-Minded Approach:
Non-directional hypotheses promote an objective and open-minded approach to research. Researchers do not have preconceived notions about the direction of the relationship or difference, which helps mitigate biases in data interpretation. They can objectively analyze the data without being influenced by their initial expectations.
Comprehensive Understanding:
By not specifying the direction, non-directional hypotheses facilitate a comprehensive understanding of the relationship or difference being investigated. Researchers can explore and consider all possible outcomes, leading to a more nuanced interpretation of the findings. This broader perspective can provide deeper insights into the research question.
Greater Sensitivity:
Non-directional hypotheses can be more sensitive to detecting unexpected or surprising relationships or differences. Researchers are not solely focused on confirming a specific predicted direction, but rather on uncovering any significant association or difference. This increased sensitivity allows for the identification of novel patterns and relationships that may have been overlooked with a directional hypothesis.
Replication and Generalizability:
Non-directional hypotheses support replication studies and enhance the generalizability of findings. By not restricting the investigation to a specific predicted direction, the results can be more applicable to different populations, contexts, or conditions. This broader applicability strengthens the validity and reliability of the research.
Hypothesis Generation:
Non-directional hypotheses can serve as a foundation for generating new hypotheses and research questions. Significant findings without a specific predicted direction can lead to further investigations and the formulation of more focused directional hypotheses in subsequent studies.
It’s important to consider the specific research context and objectives when deciding between a directional or non-directional hypothesis. Non-directional hypotheses are particularly useful when researchers are exploring new areas or when there is limited existing knowledge about the relationship or difference being studied.
Disadvantages of Non-directional hypothesis
Non-directional hypotheses have their advantages, there are also some potential disadvantages to consider:
Lack of Specificity: Non-directional hypotheses do not provide a specific prediction about the direction of the relationship or difference between variables. This lack of specificity may limit the interpretability and practical implications of the findings. Stakeholders may desire clear guidance on the expected direction of the effect.
Non-directional hypotheses often require larger sample sizes compared to directional hypotheses. This is because statistical power needs to be sufficient to detect any significant relationship or difference, regardless of the direction. Obtaining larger samples can be more time-consuming, resource-intensive, and costly.
Reduced Precision:
By not specifying the direction, non-directional hypotheses may result in less precise findings. Researchers may obtain statistically significant results indicating a relationship or difference, but the lack of direction may hinder their ability to understand the practical implications or mechanism behind the effect.
Potential for Post-hoc Interpretation:
Non-directional hypotheses can increase the risk of post-hoc interpretation of results. Researchers may be tempted to selectively interpret and highlight only the significant findings that support their preconceived notions or expectations, leading to biased interpretations.
Limited Theoretical Guidance:
Non-directional hypotheses may lack theoretical guidance in terms of understanding the underlying mechanisms or causal pathways. Without a specific predicted direction, it can be challenging to develop a comprehensive theoretical framework to explain the relationship or difference being studied.
Potential Missed Opportunities:
Non-directional hypotheses may limit the exploration of specific directions or subgroups within the data. By not focusing on a specific direction, researchers may miss important nuances or interactions that could contribute to a deeper understanding of the phenomenon under investigation.
It’s important to carefully consider the research question, available literature, and research objectives when deciding whether to use a non-directional hypothesis. Depending on the context and goals of the study, a non-directional hypothesis may be appropriate, but researchers should also be aware of the potential limitations and address them accordingly in their research design and interpretation of results.
Difference between directional and non-directional hypothesis
the main difference between a directional hypothesis and a non-directional hypothesis lies in the specificity of the prediction made about the relationship between variables or the difference between groups.
Directional Hypothesis:
A directional hypothesis, also known as a one-tailed hypothesis, makes a specific prediction about the direction of the relationship or difference. It states the expected outcome, whether it is a positive or negative relationship, a higher or lower value, an increase or decrease, etc. The directional hypothesis guides the research in a focused manner, specifying the direction to be tested.
Example: “Students who receive tutoring will demonstrate higher test scores compared to students who do not receive tutoring.”
A non-directional hypothesis, also known as a two-tailed hypothesis, does not specify the direction of the relationship or difference. It acknowledges the possibility of a relationship or difference between variables without predicting a specific direction. The non-directional hypothesis allows for exploration and analysis of both positive and negative associations or differences.
Example: “There is a significant relationship between sleep quality and academic performance.”
In summary, a directional hypothesis makes a specific prediction about the direction of the relationship or difference, while a non-directional hypothesis suggests a relationship or difference without specifying the direction. The choice between the two depends on the research question, existing literature, and the researcher’s objectives. Directional hypotheses provide a focused prediction, while non-directional hypotheses allow for more exploratory analysis .
When to use Directional Hypothesis?
A directional hypothesis is appropriate to use in specific situations where researchers have a clear theoretical or empirical basis for predicting the direction of the relationship or difference between variables. Here are some scenarios where a directional hypothesis is commonly employed:
Prior Research and Theoretical Framework: When previous studies, existing theories, or established empirical evidence strongly suggest a specific direction of the relationship or difference, a directional hypothesis can be formulated. Researchers can build upon the existing knowledge base and make a focused prediction based on this prior information.
Cause-and-Effect Relationships: In studies aiming to establish cause-and-effect relationships, directional hypotheses are often used. When there is a clear theoretical understanding of the causal relationship between variables, researchers can predict the expected direction of the effect based on the proposed mechanism.
Specific Research Objectives: If the research study has specific objectives that require a clear prediction about the direction, a directional hypothesis can be appropriate. For instance, if the aim is to test the effectiveness of a particular intervention or treatment, a directional hypothesis can guide the evaluation by predicting the expected positive or negative outcome.
Practical Applications: Directional hypotheses are useful when the research findings have direct practical implications. For example, in fields such as medicine, psychology, or education, researchers may formulate directional hypotheses to predict the effects of certain interventions or treatments on patient outcomes or educational achievement.
Hypothesis-Testing Approach: Researchers who adopt a hypothesis-testing approach, where they aim to confirm or disconfirm specific predictions, often use directional hypotheses. This approach involves formulating a specific hypothesis and conducting statistical tests to determine whether the data support or refute the predicted direction of the relationship or difference.
When to use non directional hypothesis?
A non-directional hypothesis, also known as a two-tailed hypothesis, is appropriate to use in several situations where researchers do not have a specific prediction about the direction of the relationship or difference between variables. Here are some scenarios where a non-directional hypothesis is commonly employed:
Exploratory Research:
When the research aims to explore a new area or investigate a relationship that has limited prior research or theoretical guidance, a non-directional hypothesis is often used. It allows researchers to gather initial data and insights without being constrained by a specific predicted direction.
Preliminary Studies:
Non-directional hypotheses are useful in preliminary or pilot studies that seek to gather preliminary evidence and generate hypotheses for further investigation. By using a non-directional hypothesis, researchers can gather initial data to inform the development of more specific hypotheses in subsequent studies.
Neutral Expectations:
If researchers have no theoretical or empirical basis to predict the direction of the relationship or difference, a non-directional hypothesis is appropriate. This may occur in situations where there is a lack of prior research, conflicting findings, or inconclusive evidence to support a specific direction.
Comparative Studies:
In studies where the objective is to compare two or more groups or conditions, a non-directional hypothesis is commonly used. The focus is on determining whether a significant difference exists, without making specific predictions about which group or condition will have higher or lower values.
Data-Driven Approach:
When researchers adopt a data-driven or exploratory approach to analysis, non-directional hypotheses are preferred. Instead of testing specific predictions, the aim is to explore the data, identify patterns, and generate hypotheses based on the observed relationships or differences.
Hypothesis-Generating Studies:
Non-directional hypotheses are often used in studies aimed at generating new hypotheses and research questions. By exploring associations or differences without specifying the direction, researchers can identify potential relationships or factors that can serve as a basis for future research.
Strategies to improve directional and non-directional hypothesis
To improve the quality of both directional and non-directional hypotheses, researchers can employ various strategies. Here are some strategies to enhance the formulation of hypotheses:
Strategies to Improve Directional Hypotheses:
Review existing literature:.
Conduct a thorough review of relevant literature to identify previous research findings, theories, and empirical evidence related to the variables of interest. This will help inform and support the formulation of a specific directional hypothesis based on existing knowledge.
Develop a Theoretical Framework:
Build a theoretical framework that outlines the expected causal relationship between variables. The theoretical framework should provide a clear rationale for predicting the direction of the relationship based on established theories or concepts.
Conduct Pilot Studies:
Conducting pilot studies or preliminary research can provide valuable insights and data to inform the formulation of a directional hypothesis. Initial findings can help researchers identify patterns or relationships that support a specific predicted direction.
Seek Expert Input:
Seek input from experts or colleagues in the field who have expertise in the area of study. Discuss the research question and hypothesis with them to obtain valuable insights, perspectives, and feedback that can help refine and improve the directional hypothesis.
Clearly Define Variables:
Clearly define and operationalize the variables in the hypothesis to ensure precision and clarity. This will help avoid ambiguity and ensure that the hypothesis is testable and measurable.
Strategies to Improve Non-Directional Hypotheses:
Preliminary exploration:.
Conduct initial exploratory research to gather preliminary data and insights on the relationship or difference between variables. This can provide a foundation for formulating a non-directional hypothesis based on observed patterns or trends.
Analyze Existing Data:
Analyze existing datasets to identify potential relationships or differences. Exploratory data analysis techniques such as data visualization, descriptive statistics, and correlation analysis can help uncover initial insights that can guide the formulation of a non-directional hypothesis.
Use Exploratory Research Designs:
Employ exploratory research designs such as qualitative studies, case studies, or grounded theory approaches. These designs allow researchers to gather rich data and explore relationships or differences without preconceived notions about the direction.
Consider Alternative Explanations:
When formulating a non-directional hypothesis, consider alternative explanations or potential factors that may influence the relationship or difference between variables. This can help ensure a comprehensive and nuanced understanding of the phenomenon under investigation.
Refine Based on Initial Findings:
Refine the non-directional hypothesis based on initial findings and observations from exploratory analyses. These findings can guide the formulation of more specific hypotheses in subsequent studies or inform the direction of further research.
In conclusion, both directional and non-directional hypotheses have their merits and are valuable in different research contexts.
Here’s a summary of the key points regarding directional and non-directional hypotheses:
- A directional hypothesis makes a specific prediction about the direction of the relationship or difference between variables.
- It is appropriate when there is a clear theoretical or empirical basis for predicting the direction.
- Directional hypotheses provide a focused approach, guiding the research towards confirming or refuting a specific predicted direction.
- They are useful in studies where cause-and-effect relationships are being examined or when specific practical implications are desired.
- Directional hypotheses require careful consideration of prior research, theoretical frameworks, and available evidence.
- A non-directional hypothesis does not specify the direction of the relationship or difference between variables.
- It is employed when there is limited prior knowledge, conflicting findings, or a desire for exploratory analysis.
- Non-directional hypotheses allow for flexibility and open-mindedness in exploring the data, considering both positive and negative associations or differences.
- They are suitable for preliminary studies, exploratory research, or when the research question does not have a clear predicted direction.
- Non-directional hypotheses are beneficial for generating new hypotheses, replication studies, and enhancing generalizability.
In both cases, it is essential to ensure that hypotheses are clear, testable, and aligned with the research objectives. Researchers should also be open to revising and refining hypotheses based on the findings and feedback obtained during the research process. The choice between a directional and non-directional hypothesis depends on factors such as the research question, available literature, theoretical frameworks, and the specific objectives of the study. Researchers should carefully consider these factors to determine the most appropriate type of hypothesis to use in their research
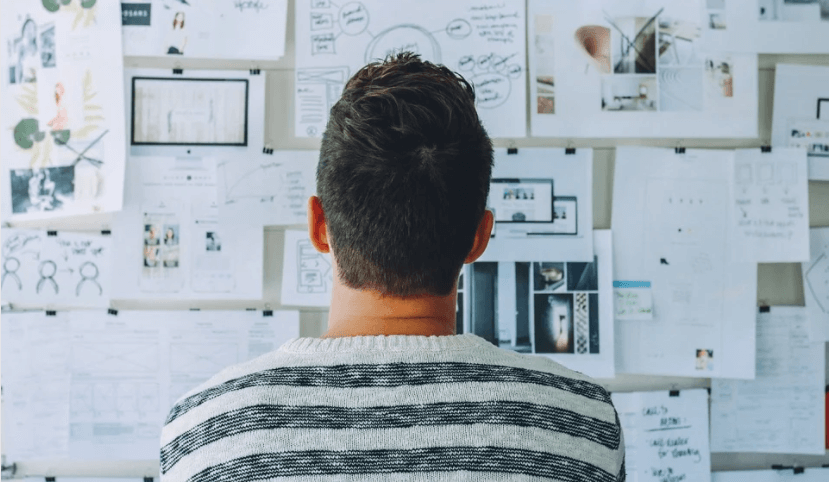
Directional vs Non-Directional Hypothesis – Collect Feedback More Effectively
To conduct a perfect survey, you should know the basics of good research . That’s why in Startquestion we would like to share with you our knowledge about basic terms connected to online surveys and feedback gathering . Knowing the basis you can create surveys and conduct research in more effective ways and thanks to this get meaningful feedback from your customers, employees, and users. That’s enough for the introduction – let’s get to work. This time we will tell you about the hypothesis .
What is a Hypothesis?
A Hypothesis can be described as a theoretical statement built upon some evidence so that it can be tested as if it is true or false. In other words, a hypothesis is a speculation or an idea, based on insufficient evidence that allows it further analysis and experimentation.
The purpose of a hypothetical statement is to work like a prediction based on studied research and to provide some estimated results before it ha happens in a real position. There can be more than one hypothesis statement involved in a research study, where you need to question and explore different aspects of a proposed research topic. Before putting your research into directional vs non-directional hypotheses, let’s have some basic knowledge.
Most often, a hypothesis describes a relation between two or more variables. It includes:
An Independent variable – One that is controlled by the researcher
Dependent Variable – The variable that the researcher observes in association with the Independent variable.
Try one of the best survey tools for free!
Start trial period without any credit card or subscription. Easily conduct your research and gather feedback via link, social media, email, and more.
Create first survey
No credit card required · Cancel any time · GDRP Compilant
How to write an effective Hypothesis?
To write an effective hypothesis follow these essential steps.
- Inquire a Question
The very first step in writing an effective hypothesis is raising a question. Outline the research question very carefully keeping your research purpose in mind. Build it in a precise and targeted way. Here you must be clear about the research question vs hypothesis. A research question is the very beginning point of writing an effective hypothesis.
Do Literature Review
Once you are done with constructing your research question, you can start the literature review. A literature review is a collection of preliminary research studies done on the same or relevant topics. There is a diversified range of literature reviews. The most common ones are academic journals but it is not confined to that. It can be anything including your research, data collection, and observation.
At this point, you can build a conceptual framework. It can be defined as a visual representation of the estimated relationship between two variables subjected to research.
Frame an Answer
After a collection of literature reviews, you can find ways how to answer the question. Expect this stage as a point where you will be able to make a stand upon what you believe might have the exact outcome of your research. You must formulate this answer statement clearly and concisely.
Build a Hypothesis
At this point, you can firmly build your hypothesis. By now, you knew the answer to your question so make a hypothesis that includes:
- Applicable Variables
- Particular Group being Studied (Who/What)
- Probable Outcome of the Experiment
Remember, your hypothesis is a calculated assumption, it has to be constructed as a sentence, not a question. This is where research question vs hypothesis starts making sense.
Refine a Hypothesis
Make necessary amendments to the constructed hypothesis keeping in mind that it has to be targeted and provable. Moreover, you might encounter certain circumstances where you will be studying the difference between one or more groups. It can be correlational research. In such instances, you must have to testify the relationships that you believe you will find in the subject variables and through this research.
Build Null Hypothesis
Certain research studies require some statistical investigation to perform a data collection. Whenever applying any scientific method to construct a hypothesis, you must have adequate knowledge of the Null Hypothesis and an Alternative hypothesis.
Null Hypothesis:
A null Hypothesis denotes that there is no statistical relationship between the subject variables. It is applicable for a single group of variables or two groups of variables. A Null Hypothesis is denoted as an H0. This is the type of hypothesis that the researcher tries to invalidate. Some of the examples of null hypotheses are:
– Hyperactivity is not associated with eating sugar.
– All roses have an equal amount of petals.
– A person’s preference for a dress is not linked to its color.
Alternative Hypothesis:
An alternative hypothesis is a statement that is simply inverse or opposite of the null hypothesis and denoted as H1. Simply saying, it is an alternative statement for the null hypothesis. The same examples will go this way as an alternative hypothesis:
– Hyperactivity is associated with eating sugar.
– All roses do not have an equal amount of petals.
– A person’s preference for a dress is linked to its color.
Start your research right now: use professional survey templates
- Brand Awareness Survey
- Survey for the thesis
- Website Evaluation Survey
See more templates
Types of Hypothesis
Apart from null and alternative hypotheses, research hypotheses can be categorized into different types. Let’s have a look at them:
Simple Hypothesis:
This type of hypothesis is used to state a relationship between a particular independent variable and only a dependent variable.
Complex Hypothesis:
A statement that states the relationship between two or more independent variables and two or more dependent variables, is termed a complex hypothesis.
Associative and Causal Hypothesis:
This type of hypothesis involves predicting that there is a point of interdependency between two variables. It says that any kind of change in one variable will cause a change in the other one. Similarly, a casual hypothesis says that a change in the dependent variable is due to some variations in the independent variable.
Directional vs non-directional hypothesis
Directional hypothesis:.
A hypothesis that is built upon a certain directional relationship between two variables and constructed upon an already existing theory, is called a directional hypothesis. To understand more about what is directional hypothesis here is an example, Girls perform better than boys (‘better than’ shows the direction predicted)
Non-directional Hypothesis:
It involves an open-ended non-directional hypothesis that predicts that the independent variable will influence the dependent variable; however, the nature or direction of a relationship between two subject variables is not defined or clear.
For Example, there will be a difference in the performance of girls & boys (Not defining what kind of difference)
As a professional, we suggest you apply a non-directional alternative hypothesis when you are not sure of the direction of the relationship. Maybe you’re observing potential gender differences on some psychological test, but you don’t know whether men or women would have the higher ratio. Normally, this would say that you are lacking practical knowledge about the proposed variables. A directional test should be more common for tests.
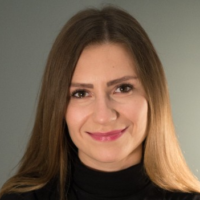
Author: Ula Kamburov-Niepewna
Updated: 18 November 2022
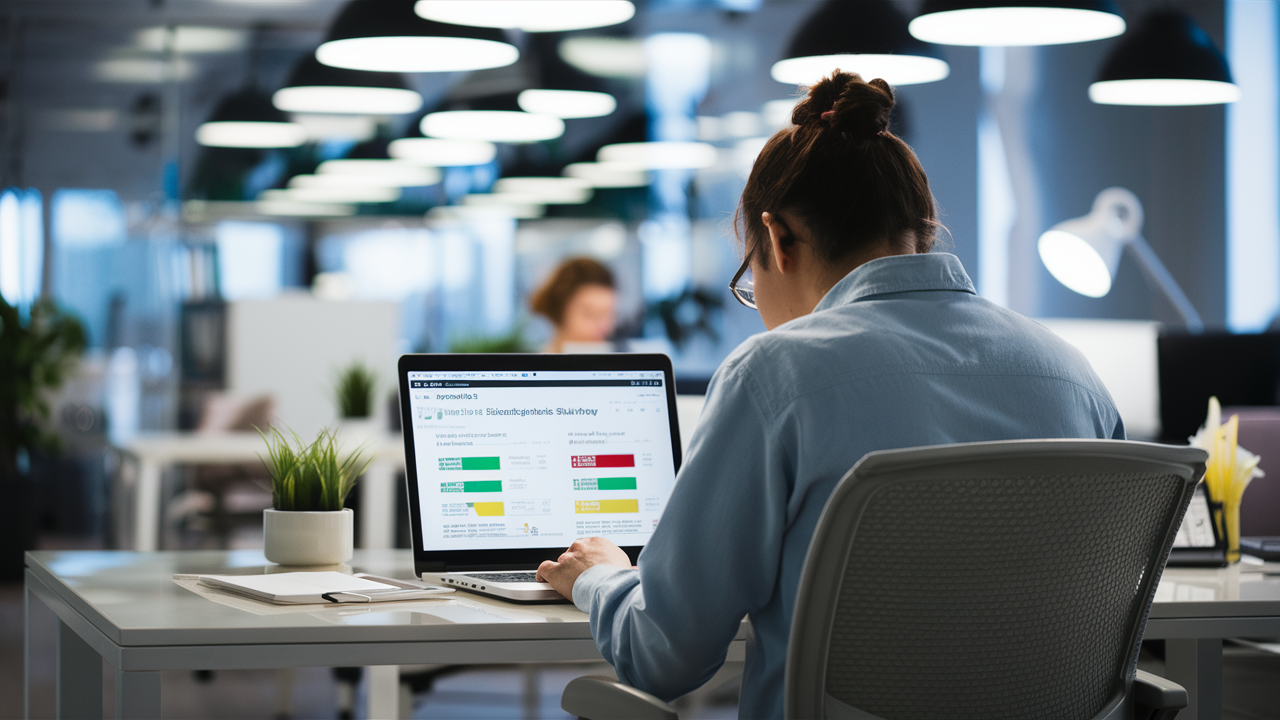
Analyzing Open Ended Survey Responses with AI – Meet Insightomat
While closed-ended questions provide quick and quantifiable data, open-ended questions unlock deeper insights into customer sentiments and behaviors. But how do you efficiently analyze the wealth of qualitative data that open-ended questions generate?
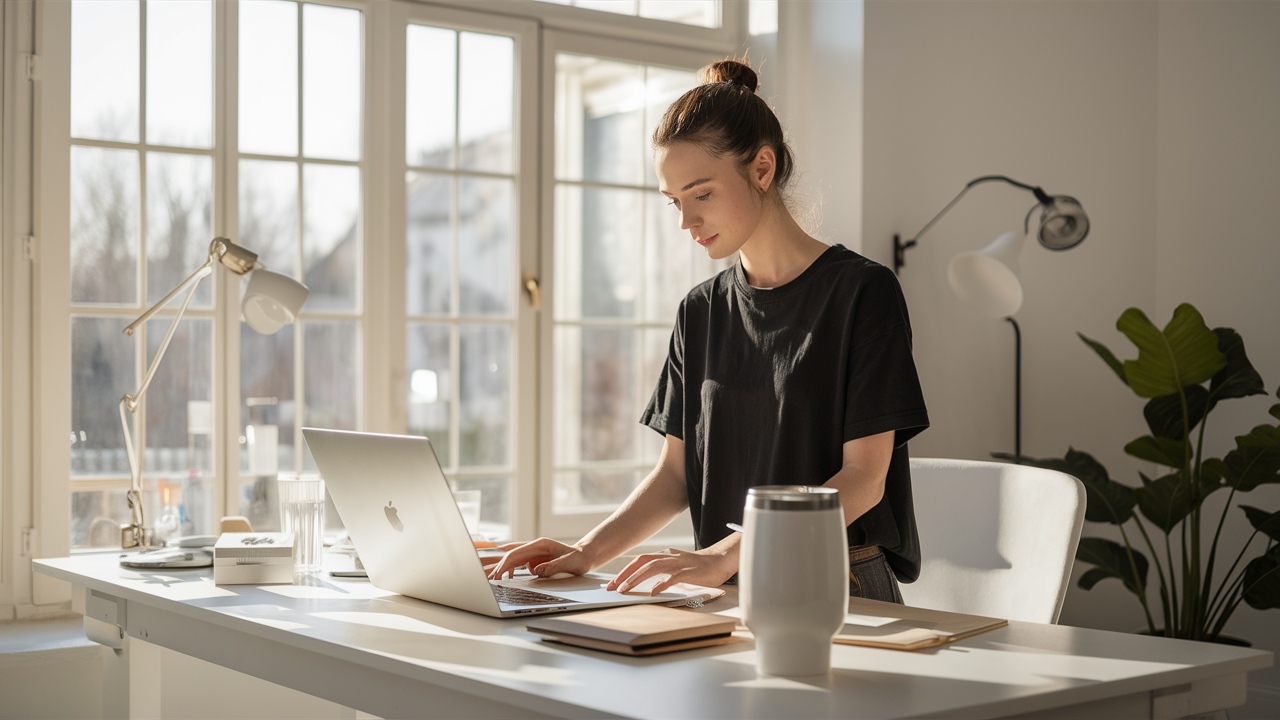
Qualitative Survey Questions with Some Examples
Understanding your customers' experiences and perceptions is crucial for enhancing your products and services. While quantitative research provides numerical insights, qualitative survey questions dive deeper, exploring the "why" behind customer behaviors.
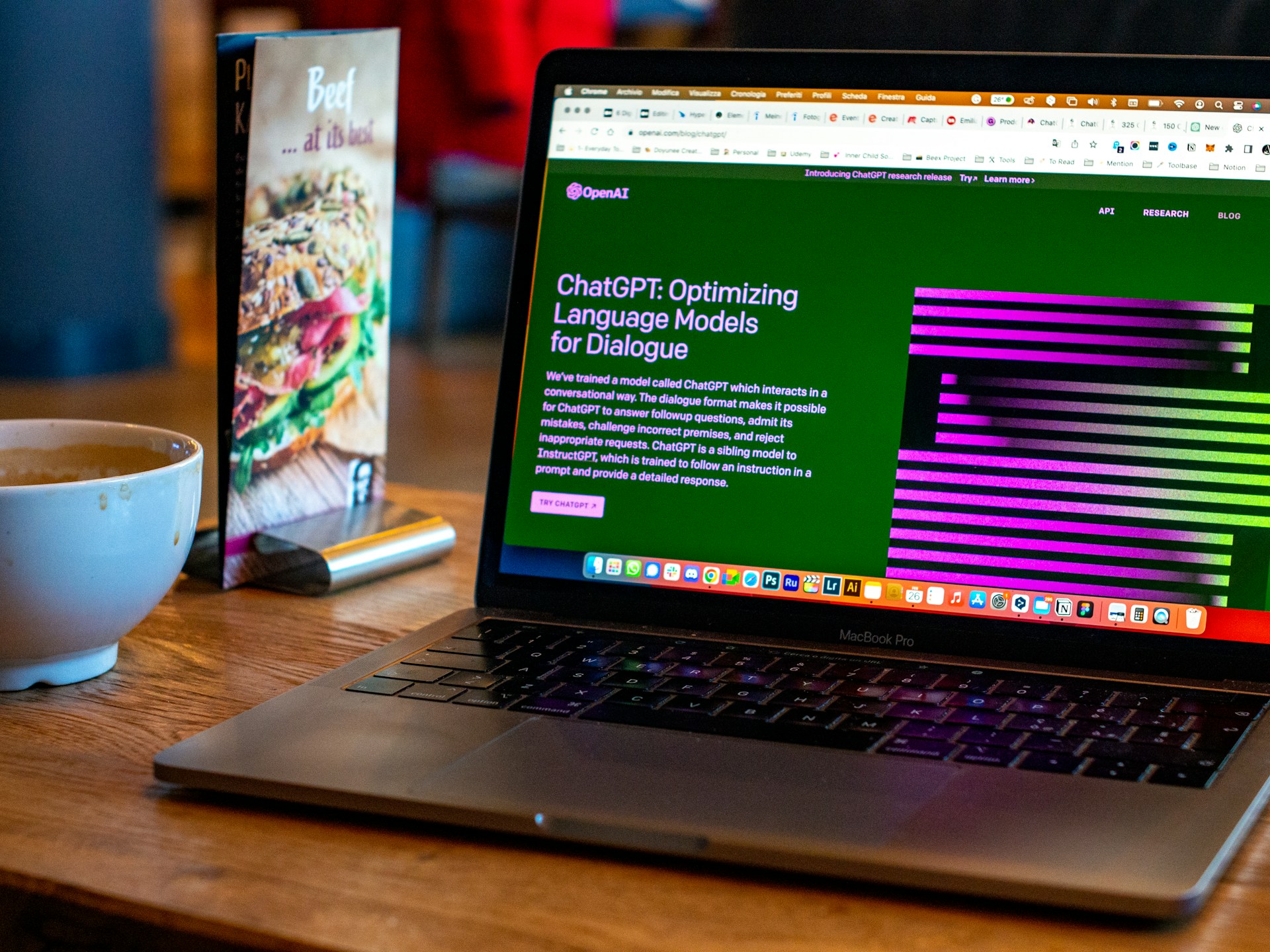
Eleven Useful ChatGPT Alternatives
ChatGPT has made quite a splash in the world of generative AI, but it's not the only game in town. Let's take a look at some of the best alternatives that could become your new go-to.
psychologyrocks
Hypotheses; directional and non-directional, what is the difference between an experimental and an alternative hypothesis.
Nothing much! If the study is a laboratory experiment then we can call the hypothesis “an experimental hypothesis”, where we make a prediction about how the IV causes an effect on the DV. If we have a non-experimental design, i.e. we are not able to manipulate the IV as in a natural or quasi-experiment , or if some other research method has been used, then we call it an “alternativehypothesis”, alternative to the null.
Directional hypothesis: A directional (or one tailed hypothesis) states which way you think the results are going to go, for example in an experimental study we might say…”Participants who have been deprived of sleep for 24 hours will have more cold symptoms in the following week after exposure to a virus than participants who have not been sleep deprived”; the hypothesis compares the two groups/conditions and states which one will ….have more/less, be quicker/slower, etc.
If we had a correlational study, the directional hypothesis would state whether we expect a positive or a negative correlation, we are stating how the two variables will be related to each other, e.g. there will be a positive correlation between the number of stressful life events experienced in the last year and the number of coughs and colds suffered, whereby the more life events you have suffered the more coughs and cold you will have had”. The directional hypothesis can also state a negative correlation, e.g. the higher the number of face-book friends, the lower the life satisfaction score “
Non-directional hypothesis: A non-directional (or two tailed hypothesis) simply states that there will be a difference between the two groups/conditions but does not say which will be greater/smaller, quicker/slower etc. Using our example above we would say “There will be a difference between the number of cold symptoms experienced in the following week after exposure to a virus for those participants who have been sleep deprived for 24 hours compared with those who have not been sleep deprived for 24 hours.”
When the study is correlational, we simply state that variables will be correlated but do not state whether the relationship will be positive or negative, e.g. there will be a significant correlation between variable A and variable B.
Null hypothesis The null hypothesis states that the alternative or experimental hypothesis is NOT the case, if your experimental hypothesis was directional you would say…
Participants who have been deprived of sleep for 24 hours will NOT have more cold symptoms in the following week after exposure to a virus than participants who have not been sleep deprived and any difference that does arise will be due to chance alone.
or with a directional correlational hypothesis….
There will NOT be a positive correlation between the number of stress life events experienced in the last year and the number of coughs and colds suffered, whereby the more life events you have suffered the more coughs and cold you will have had”
With a non-directional or two tailed hypothesis…
There will be NO difference between the number of cold symptoms experienced in the following week after exposure to a virus for those participants who have been sleep deprived for 24 hours compared with those who have not been sleep deprived for 24 hours.
or for a correlational …
there will be NO correlation between variable A and variable B.
When it comes to conducting an inferential stats test, if you have a directional hypothesis , you must do a one tailed test to find out whether your observed value is significant. If you have a non-directional hypothesis , you must do a two tailed test .
Exam Techniques/Advice
- Remember, a decent hypothesis will contain two variables, in the case of an experimental hypothesis there will be an IV and a DV; in a correlational hypothesis there will be two co-variables
- both variables need to be fully operationalised to score the marks, that is you need to be very clear and specific about what you mean by your IV and your DV; if someone wanted to repeat your study, they should be able to look at your hypothesis and know exactly what to change between the two groups/conditions and exactly what to measure (including any units/explanation of rating scales etc, e.g. “where 1 is low and 7 is high”)
- double check the question, did it ask for a directional or non-directional hypothesis?
- if you were asked for a null hypothesis, make sure you always include the phrase “and any difference/correlation (is your study experimental or correlational?) that does arise will be due to chance alone”
Practice Questions:
- Mr Faraz wants to compare the levels of attendance between his psychology group and those of Mr Simon, who teaches a different psychology group. Which of the following is a suitable directional (one tailed) hypothesis for Mr Faraz’s investigation?
A There will be a difference in the levels of attendance between the two psychology groups.
B Students’ level of attendance will be higher in Mr Faraz’s group than Mr Simon’s group.
C Any difference in the levels of attendance between the two psychology groups is due to chance.
D The level of attendance of the students will depend upon who is teaching the groups.
2. Tracy works for the local council. The council is thinking about reducing the number of people it employs to pick up litter from the street. Tracy has been asked to carry out a study to see if having the streets cleaned at less regular intervals will affect the amount of litter the public will drop. She studies a street to compare how much litter is dropped at two different times, once when it has just been cleaned and once after it has not been cleaned for a month.
Write a fully operationalised non-directional (two-tailed) hypothesis for Tracy’s study. (2)
3. Jamila is conducting a practical investigation to look at gender differences in carrying out visuo-spatial tasks. She decides to give males and females a jigsaw puzzle and will time them to see who completes it the fastest. She uses a random sample of pupils from a local school to get her participants.
(a) Write a fully operationalised directional (one tailed) hypothesis for Jamila’s study. (2) (b) Outline one strength and one weakness of the random sampling method. You may refer to Jamila’s use of this type of sampling in your answer. (4)
4. Which of the following is a non-directional (two tailed) hypothesis?
A There is a difference in driving ability with men being better drivers than women
B Women are better at concentrating on more than one thing at a time than men
C Women spend more time doing the cooking and cleaning than men
D There is a difference in the number of men and women who participate in sports
Revision Activity
writing-hypotheses-revision-sheet
Quizizz link for teachers: https://quizizz.com/admin/quiz/5bf03f51add785001bc5a09e
Share this:
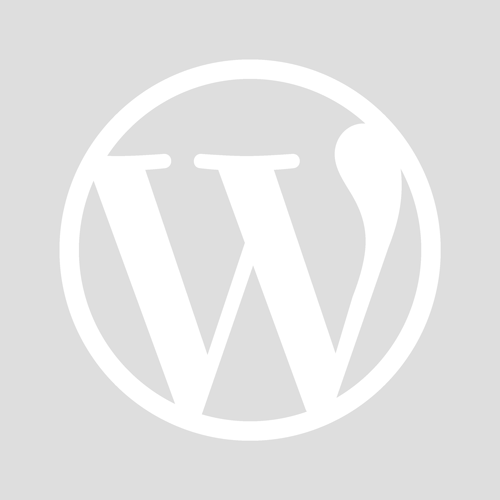
- Already have a WordPress.com account? Log in now.
- Subscribe Subscribed
- Copy shortlink
- Report this content
- View post in Reader
- Manage subscriptions
- Collapse this bar
Directional vs. Non-Directional Hypothesis in Research
In the world of research and statistical analysis, formulating hypotheses is a crucial step in the scientific process. Hypotheses guide researchers in making predictions and testing relationships between variables. When it comes to hypotheses, there are two main types: directional and non-directional.
Table of Contents
Directional Hypothesis
A directional hypothesis, also known as a one-tailed hypothesis, is formulated with a specific predicted direction of the relationship between variables. It indicates an expectation of the relationship being either positive or negative.
Example of Directional Hypothesis
Advantages of directional hypothesis, non-directional hypothesis.
Non-directional hypotheses are often used when there is insufficient prior knowledge or theoretical basis to predict the direction of the relationship. It allows for a more exploratory approach, where the researcher is open to discovering the nature of the relationship through data analysis .
Example of Non-Directional Hypothesis
Read More: Population vs Sample | Examples
Advantages of Non-Directional Hypothesis:
Difference between directional and non-directional hypotheses.
Choosing Between Directional and Non-Directional Hypotheses: The choice between a directional and non-directional hypothesis depends on the research question, existing knowledge, and theoretical background. Here are a few considerations for selecting the appropriate type of hypothesis:
Directional vs. Non-Directional Hypothesis
Aspect | Directional Hypothesis | Non-Directional Hypothesis |
---|---|---|
Specifies the expected direction of the effect | Does not specify the expected direction | |
One-tailed (focuses on one direction) | Two-tailed (considers both positive and negative effects) | |
Often based on prior research or theory | May lack prior knowledge or theoretical basis | |
Higher power to detect the specified direction | Power is divided between both directions | |
Less flexible in exploring alternative outcomes | More flexible in considering different outcomes. | |
Higher confidence in the predicted direction | Equal confidence in both positive and negative effects |
Directional hypotheses offer specific predictions about the expected direction of the relationship, whereas non-directional hypotheses allow for more exploratory investigations without preconceived notions of the direction.
Remember, hypotheses serve as a roadmap for research, and regardless of their type, they play a crucial role in scientific inquiry and the pursuit of knowledge.
Other articles
Related posts, research paper outline template: examples of structured research paper outlines, writing a research paper cover letter (example), get free access to 25 million peer-reviewed research papers, how to add social media citations in research article, clinical researcher: responsibilities, skills, and steps to pursue this career, alternative hypothesis: types and examples, causal research: examples, benefits, and practical tips, 7 types of observational studies | examples, writing an introduction for a research paper: a guide (with examples), clinical research design: elements and importance, leave a reply cancel reply.
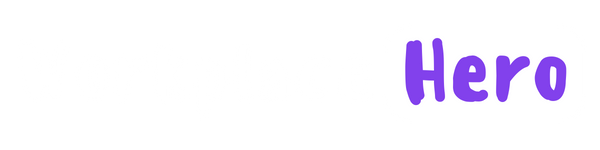
Understanding the fundamentals of a non-directional hypothesis
A crucial aspect of conducting research is formulating a hypothesis. In simple terms, a hypothesis is a statement that makes a prediction about the relationship between two or more variables. It serves as a guide for researchers to test their theories and draw conclusions. A non-directional hypothesis, also known as a two-tailed hypothesis, is a type of hypothesis that does not specify the direction of the predicted relationship between variables. This means that the researcher is not making a specific prediction and is open to the possibility of any outcome. In this article, we will delve into the fundamentals of a non-directional hypothesis and its significance in research studies.
The difference between directional and non-directional hypotheses
When conducting research, it is important to have a clear understanding of the different types of hypotheses. One key distinction to make is between directional and non-directional hypotheses.
A directional hypothesis, also known as a one-tailed hypothesis, predicts the direction of the relationship between variables. This means that the researcher has a specific prediction in mind. For example, a directional hypothesis might state that "increased exercise leads to improved cardiovascular health." In this case, the researcher is specifically predicting that increased exercise will have a positive effect on cardiovascular health.
On the other hand, a non-directional hypothesis, also known as a two-tailed hypothesis, does not specify the direction of the relationship between variables. This means that the researcher does not have a specific prediction in mind and is open to the possibility of any outcome. For example, a non-directional hypothesis might state that "there is a relationship between exercise and cardiovascular health." In this case, the researcher is simply predicting that there is some sort of relationship between the two variables, but is not specifying whether it is positive or negative.
The main difference between these two types of hypotheses lies in the level of specificity. A directional hypothesis provides a specific prediction about the relationship between variables, while a non-directional hypothesis leaves the prediction open-ended.
So why would a researcher choose to use a non-directional hypothesis? One reason is that it allows for more flexibility and open-mindedness in the research process. By not specifying a particular direction, the researcher is not constrained by preconceived notions or biases. This can lead to more objective and unbiased findings.
Another reason to use a non-directional hypothesis is when there is a lack of previous research or evidence to support a specific direction. If there is limited knowledge or conflicting results on the topic, it may be more appropriate to use a non-directional hypothesis to explore the relationship between variables without making any specific predictions.
In summary, the difference between directional and non-directional hypotheses lies in the level of specificity and prediction. While directional hypotheses specify the direction of the relationship, non-directional hypotheses leave the prediction open-ended. Non-directional hypotheses provide researchers with more flexibility and open-mindedness in the research process and can be especially useful in situations with limited previous research or conflicting evidence.
Why use a non-directional hypothesis
There are several reasons why researchers might choose to use a non-directional hypothesis in their studies. Firstly, it allows for more flexibility and open-mindedness in the research process. By not specifying a particular direction, researchers are not constrained by preconceived notions or biases. This can lead to more objective and unbiased findings, as the researcher is not influenced by their own expectations.
Another reason to use a non-directional hypothesis is when there is a lack of previous research or evidence to support a specific direction. Sometimes, researchers are exploring a new topic or are working in an area where there is limited knowledge or conflicting results. In these cases, it may be more appropriate to use a non-directional hypothesis to explore the relationship between variables without making any specific predictions. This allows for a more exploratory approach, where researchers can gather data and draw conclusions based on the evidence they find.
Additionally, using a non-directional hypothesis can help researchers avoid the problem of hindsight bias. Hindsight bias occurs when researchers interpret their results in a way that aligns with their initial expectations. By using a non-directional hypothesis, researchers are less likely to fall into this trap and can make more accurate interpretations of their data.
Overall, using a non-directional hypothesis can be beneficial in certain research scenarios. It allows for flexibility, open-mindedness, and exploration. It also helps researchers avoid bias and hindsight bias, leading to more objective and reliable findings. By using a non-directional hypothesis, researchers can approach their studies with a fresh perspective and make new discoveries in their field.
How to form a non-directional hypothesis
Forming a non-directional hypothesis is an essential step in conducting research. Unlike a directional hypothesis, which predicts the specific direction of the relationship between variables, a non-directional hypothesis leaves the prediction open-ended. This allows for more flexibility and exploration in the research process. Here are some key steps to consider when forming a non-directional hypothesis:
Identify the variables
Start by identifying the variables that you want to study. These variables should be measurable and have a logical connection to each other. For example, if you are interested in studying the relationship between exercise and cardiovascular health, your variables would be exercise and cardiovascular health.
Determine the type of relationship
Consider what type of relationship you want to explore between the variables. Are you looking for a relationship that is positive, negative, or simply any relationship? This will help guide the formation of your hypothesis. For example, if you want to explore any relationship between exercise and cardiovascular health, your hypothesis might be that there is a relationship between the two variables.
Keep it general
When writing your hypothesis, avoid specifying a particular direction of the relationship. Instead, keep it general and open-ended. This allows for more flexibility in the research process. For example, a non-directional hypothesis could state that "there is a relationship between exercise and cardiovascular health," without specifying whether it is positive or negative.
Be specific and testable
Although a non-directional hypothesis does not specify the direction of the relationship, it should still be specific and testable. This means that it should be clear enough to guide your research and allow you to gather data to support or reject the hypothesis. Make sure that your variables are clearly defined and that you have a clear plan for how you will measure them.
Consider alternative explanations
When forming a non-directional hypothesis, it is important to consider alternative explanations for the relationship between variables. This will help ensure that your hypothesis is comprehensive and takes into account different possibilities. For example, if you are studying the relationship between exercise and cardiovascular health, consider other factors that may influence cardiovascular health, such as diet or genetics.
By following these steps, you can effectively form a non-directional hypothesis for your research. This will allow you to explore the relationship between variables without being constrained by preconceived notions or biases. Remember to keep your hypothesis general, specific, and testable, and consider alternative explanations for the relationship.
Examples of non-directional hypotheses in research
When it comes to research, formulating a non-directional hypothesis can be particularly useful in certain scenarios. Let's explore some examples of non-directional hypotheses to understand how they can be applied in research studies.
"There is a relationship between sleep duration and academic performance."
This hypothesis suggests that there is some form of relationship between the amount of sleep an individual gets and their academic performance. However, it does not specify whether this relationship is positive or negative. By using a non-directional hypothesis, researchers can explore the potential impact of sleep duration on academic performance without making a specific prediction.
"There is a relationship between stress levels and job satisfaction." In this case, the hypothesis proposes that there is some form of relationship between stress levels and job satisfaction. However, it does not specify whether increased stress levels lead to decreased job satisfaction or vice versa. By using a non-directional hypothesis, researchers can examine the relationship between these variables without assuming a particular direction.
"There is a relationship between age and technology usage." This hypothesis suggests that there is some form of relationship between age and technology usage. However, it does not specify whether younger individuals are more likely to use technology or whether older individuals are more likely to use technology. By using a non-directional hypothesis, researchers can explore the potential relationship between age and technology usage without assuming a specific pattern.
These examples illustrate how non-directional hypotheses allow researchers to investigate relationships between variables without being tied to a specific prediction. This flexibility enables researchers to approach their studies with an open mind and gather objective data to draw conclusions.
By using non-directional hypotheses, researchers can explore uncharted territory, address conflicting evidence, and provide valuable insights into various fields of study. This approach promotes a more exploratory and unbiased research process, leading to more accurate and reliable findings. So, if you find yourself in a research scenario where a specific direction is unclear or the evidence is limited, consider using a non-directional hypothesis to guide your exploration.
Advantages and disadvantages of non-directional hypotheses
Non-directional hypotheses have several advantages and disadvantages that researchers should consider when conducting their studies.
One of the main advantages of using a non-directional hypothesis is the flexibility it provides in the research process. By not specifying a particular direction, researchers are not limited by preconceived notions or biases.
This allows for more open-mindedness and exploration, which can lead to new insights and discoveries. It also allows researchers to consider alternative explanations and factors that may influence the relationship between variables.
Another advantage of non-directional hypotheses is that they help researchers avoid the problem of hindsight bias. Hindsight bias occurs when researchers interpret their results in a way that aligns with their initial expectations. By using a non-directional hypothesis, researchers are less likely to fall into this trap and can make more accurate interpretations of their data. This enhances the reliability and objectivity of their findings.
Non-directional hypotheses are also useful in situations where there is limited previous research or conflicting evidence. They allow researchers to explore the relationship between variables without making any specific predictions, which can be especially valuable in new or unexplored areas of study. This approach promotes a more exploratory and unbiased research process, enabling researchers to gather data and draw conclusions based on the evidence they find.
Disadvantages
However, there are also some disadvantages to using non-directional hypotheses. One disadvantage is that they may lack specificity. Without specifying the direction of the relationship, researchers may struggle to draw clear conclusions or make specific recommendations based on their findings. This can make it challenging to apply the research to practical situations or inform decision-making processes.
Another potential disadvantage is that non-directional hypotheses can be more difficult to test statistically. Without specifying the direction of the relationship, it may be harder to determine the significance of the findings or establish causal relationships between variables. This can make it more challenging to draw meaningful and robust conclusions from the research.
In summary, non-directional hypotheses offer flexibility, open-mindedness, and exploration in the research process. They help researchers avoid biases, and hindsight bias, and provide valuable insights in situations with limited previous research or conflicting evidence. However, they may lack specificity and can be more challenging to test statistically. Researchers should carefully consider these advantages and disadvantages when deciding whether to use a non-directional hypothesis in their studies.
User Preferences
Content preview.
Arcu felis bibendum ut tristique et egestas quis:
- Ut enim ad minim veniam, quis nostrud exercitation ullamco laboris
- Duis aute irure dolor in reprehenderit in voluptate
- Excepteur sint occaecat cupidatat non proident
Keyboard Shortcuts
5.2 - writing hypotheses.
The first step in conducting a hypothesis test is to write the hypothesis statements that are going to be tested. For each test you will have a null hypothesis (\(H_0\)) and an alternative hypothesis (\(H_a\)).
When writing hypotheses there are three things that we need to know: (1) the parameter that we are testing (2) the direction of the test (non-directional, right-tailed or left-tailed), and (3) the value of the hypothesized parameter.
- At this point we can write hypotheses for a single mean (\(\mu\)), paired means(\(\mu_d\)), a single proportion (\(p\)), the difference between two independent means (\(\mu_1-\mu_2\)), the difference between two proportions (\(p_1-p_2\)), a simple linear regression slope (\(\beta\)), and a correlation (\(\rho\)).
- The research question will give us the information necessary to determine if the test is two-tailed (e.g., "different from," "not equal to"), right-tailed (e.g., "greater than," "more than"), or left-tailed (e.g., "less than," "fewer than").
- The research question will also give us the hypothesized parameter value. This is the number that goes in the hypothesis statements (i.e., \(\mu_0\) and \(p_0\)). For the difference between two groups, regression, and correlation, this value is typically 0.
Hypotheses are always written in terms of population parameters (e.g., \(p\) and \(\mu\)). The tables below display all of the possible hypotheses for the parameters that we have learned thus far. Note that the null hypothesis always includes the equality (i.e., =).
Research Question | Is the population mean different from \( \mu_{0} \)? | Is the population mean greater than \(\mu_{0}\)? | Is the population mean less than \(\mu_{0}\)? |
---|---|---|---|
Null Hypothesis, \(H_{0}\) | \(\mu=\mu_{0} \) | \(\mu=\mu_{0} \) | \(\mu=\mu_{0} \) |
Alternative Hypothesis, \(H_{a}\) | \(\mu\neq \mu_{0} \) | \(\mu> \mu_{0} \) | \(\mu<\mu_{0} \) |
Type of Hypothesis Test | Two-tailed, non-directional | Right-tailed, directional | Left-tailed, directional |
Research Question | Is there a difference in the population? | Is there a mean increase in the population? | Is there a mean decrease in the population? |
---|---|---|---|
Null Hypothesis, \(H_{0}\) | \(\mu_d=0 \) | \(\mu_d =0 \) | \(\mu_d=0 \) |
Alternative Hypothesis, \(H_{a}\) | \(\mu_d \neq 0 \) | \(\mu_d> 0 \) | \(\mu_d<0 \) |
Type of Hypothesis Test | Two-tailed, non-directional | Right-tailed, directional | Left-tailed, directional |
Research Question | Is the population proportion different from \(p_0\)? | Is the population proportion greater than \(p_0\)? | Is the population proportion less than \(p_0\)? |
---|---|---|---|
Null Hypothesis, \(H_{0}\) | \(p=p_0\) | \(p= p_0\) | \(p= p_0\) |
Alternative Hypothesis, \(H_{a}\) | \(p\neq p_0\) | \(p> p_0\) | \(p< p_0\) |
Type of Hypothesis Test | Two-tailed, non-directional | Right-tailed, directional | Left-tailed, directional |
Research Question | Are the population means different? | Is the population mean in group 1 greater than the population mean in group 2? | Is the population mean in group 1 less than the population mean in groups 2? |
---|---|---|---|
Null Hypothesis, \(H_{0}\) | \(\mu_1=\mu_2\) | \(\mu_1 = \mu_2 \) | \(\mu_1 = \mu_2 \) |
Alternative Hypothesis, \(H_{a}\) | \(\mu_1 \ne \mu_2 \) | \(\mu_1 \gt \mu_2 \) | \(\mu_1 \lt \mu_2\) |
Type of Hypothesis Test | Two-tailed, non-directional | Right-tailed, directional | Left-tailed, directional |
Research Question | Are the population proportions different? | Is the population proportion in group 1 greater than the population proportion in groups 2? | Is the population proportion in group 1 less than the population proportion in group 2? |
---|---|---|---|
Null Hypothesis, \(H_{0}\) | \(p_1 = p_2 \) | \(p_1 = p_2 \) | \(p_1 = p_2 \) |
Alternative Hypothesis, \(H_{a}\) | \(p_1 \ne p_2\) | \(p_1 \gt p_2 \) | \(p_1 \lt p_2\) |
Type of Hypothesis Test | Two-tailed, non-directional | Right-tailed, directional | Left-tailed, directional |
Research Question | Is the slope in the population different from 0? | Is the slope in the population positive? | Is the slope in the population negative? |
---|---|---|---|
Null Hypothesis, \(H_{0}\) | \(\beta =0\) | \(\beta= 0\) | \(\beta = 0\) |
Alternative Hypothesis, \(H_{a}\) | \(\beta\neq 0\) | \(\beta> 0\) | \(\beta< 0\) |
Type of Hypothesis Test | Two-tailed, non-directional | Right-tailed, directional | Left-tailed, directional |
Research Question | Is the correlation in the population different from 0? | Is the correlation in the population positive? | Is the correlation in the population negative? |
---|---|---|---|
Null Hypothesis, \(H_{0}\) | \(\rho=0\) | \(\rho= 0\) | \(\rho = 0\) |
Alternative Hypothesis, \(H_{a}\) | \(\rho \neq 0\) | \(\rho > 0\) | \(\rho< 0\) |
Type of Hypothesis Test | Two-tailed, non-directional | Right-tailed, directional | Left-tailed, directional |
Aims And Hypotheses, Directional And Non-Directional
March 7, 2021 - paper 2 psychology in context | research methods.
In Psychology, hypotheses are predictions made by the researcher about the outcome of a study. The research can chose to make a specific prediction about what they feel will happen in their research (a directional hypothesis) or they can make a ‘general,’ ‘less specific’ prediction about the outcome of their research (a non-directional hypothesis). The type of prediction that a researcher makes is usually dependent on whether or not any previous research has also investigated their research aim.
Variables Recap:
The independent variable (IV) is the variable that psychologists manipulate/change to see if changing this variable has an effect on the depen dent variable (DV).
The dependent variable (DV) is the variable that the psychologists measures (to see if the IV has had an effect).
Research/Experimental Aim(S):

An aim is a clear and precise statement of the purpose of the study. It is a statement of why a research study is taking place. This should include what is being studied and what the study is trying to achieve. (e.g. “This study aims to investigate the effects of alcohol on reaction times”.
Hypotheses:
This is a testable statement that predicts what the researcher expects to happen in their research. The research study itself is therefore a means of testing whether or not the hypothesis is supported by the findings. If the findings do support the hypothesis then the hypothesis can be retained (i.e., accepted), but if not, then it must be rejected.

(1) Directional Hypothesis: states that the IV will have an effect on the DV and what that effect will be (the direction of results). For example, eating smarties will significantly improve an individual’s dancing ability. When writing a directional hypothesis, it is important that you state exactly how the IV will influence the DV.
(3) A Null Hypothesis: states that the IV will have no significant effect on the DV, for example, ‘eating smarties will have no effect in an individuals dancing ability.’

An official website of the United States government
The .gov means it’s official. Federal government websites often end in .gov or .mil. Before sharing sensitive information, make sure you’re on a federal government site.
The site is secure. The https:// ensures that you are connecting to the official website and that any information you provide is encrypted and transmitted securely.
- Publications
- Account settings
Preview improvements coming to the PMC website in October 2024. Learn More or Try it out now .
- Advanced Search
- Journal List
- J Korean Med Sci
- v.37(16); 2022 Apr 25

A Practical Guide to Writing Quantitative and Qualitative Research Questions and Hypotheses in Scholarly Articles
Edward barroga.
1 Department of General Education, Graduate School of Nursing Science, St. Luke’s International University, Tokyo, Japan.
Glafera Janet Matanguihan
2 Department of Biological Sciences, Messiah University, Mechanicsburg, PA, USA.
The development of research questions and the subsequent hypotheses are prerequisites to defining the main research purpose and specific objectives of a study. Consequently, these objectives determine the study design and research outcome. The development of research questions is a process based on knowledge of current trends, cutting-edge studies, and technological advances in the research field. Excellent research questions are focused and require a comprehensive literature search and in-depth understanding of the problem being investigated. Initially, research questions may be written as descriptive questions which could be developed into inferential questions. These questions must be specific and concise to provide a clear foundation for developing hypotheses. Hypotheses are more formal predictions about the research outcomes. These specify the possible results that may or may not be expected regarding the relationship between groups. Thus, research questions and hypotheses clarify the main purpose and specific objectives of the study, which in turn dictate the design of the study, its direction, and outcome. Studies developed from good research questions and hypotheses will have trustworthy outcomes with wide-ranging social and health implications.
INTRODUCTION
Scientific research is usually initiated by posing evidenced-based research questions which are then explicitly restated as hypotheses. 1 , 2 The hypotheses provide directions to guide the study, solutions, explanations, and expected results. 3 , 4 Both research questions and hypotheses are essentially formulated based on conventional theories and real-world processes, which allow the inception of novel studies and the ethical testing of ideas. 5 , 6
It is crucial to have knowledge of both quantitative and qualitative research 2 as both types of research involve writing research questions and hypotheses. 7 However, these crucial elements of research are sometimes overlooked; if not overlooked, then framed without the forethought and meticulous attention it needs. Planning and careful consideration are needed when developing quantitative or qualitative research, particularly when conceptualizing research questions and hypotheses. 4
There is a continuing need to support researchers in the creation of innovative research questions and hypotheses, as well as for journal articles that carefully review these elements. 1 When research questions and hypotheses are not carefully thought of, unethical studies and poor outcomes usually ensue. Carefully formulated research questions and hypotheses define well-founded objectives, which in turn determine the appropriate design, course, and outcome of the study. This article then aims to discuss in detail the various aspects of crafting research questions and hypotheses, with the goal of guiding researchers as they develop their own. Examples from the authors and peer-reviewed scientific articles in the healthcare field are provided to illustrate key points.
DEFINITIONS AND RELATIONSHIP OF RESEARCH QUESTIONS AND HYPOTHESES
A research question is what a study aims to answer after data analysis and interpretation. The answer is written in length in the discussion section of the paper. Thus, the research question gives a preview of the different parts and variables of the study meant to address the problem posed in the research question. 1 An excellent research question clarifies the research writing while facilitating understanding of the research topic, objective, scope, and limitations of the study. 5
On the other hand, a research hypothesis is an educated statement of an expected outcome. This statement is based on background research and current knowledge. 8 , 9 The research hypothesis makes a specific prediction about a new phenomenon 10 or a formal statement on the expected relationship between an independent variable and a dependent variable. 3 , 11 It provides a tentative answer to the research question to be tested or explored. 4
Hypotheses employ reasoning to predict a theory-based outcome. 10 These can also be developed from theories by focusing on components of theories that have not yet been observed. 10 The validity of hypotheses is often based on the testability of the prediction made in a reproducible experiment. 8
Conversely, hypotheses can also be rephrased as research questions. Several hypotheses based on existing theories and knowledge may be needed to answer a research question. Developing ethical research questions and hypotheses creates a research design that has logical relationships among variables. These relationships serve as a solid foundation for the conduct of the study. 4 , 11 Haphazardly constructed research questions can result in poorly formulated hypotheses and improper study designs, leading to unreliable results. Thus, the formulations of relevant research questions and verifiable hypotheses are crucial when beginning research. 12
CHARACTERISTICS OF GOOD RESEARCH QUESTIONS AND HYPOTHESES
Excellent research questions are specific and focused. These integrate collective data and observations to confirm or refute the subsequent hypotheses. Well-constructed hypotheses are based on previous reports and verify the research context. These are realistic, in-depth, sufficiently complex, and reproducible. More importantly, these hypotheses can be addressed and tested. 13
There are several characteristics of well-developed hypotheses. Good hypotheses are 1) empirically testable 7 , 10 , 11 , 13 ; 2) backed by preliminary evidence 9 ; 3) testable by ethical research 7 , 9 ; 4) based on original ideas 9 ; 5) have evidenced-based logical reasoning 10 ; and 6) can be predicted. 11 Good hypotheses can infer ethical and positive implications, indicating the presence of a relationship or effect relevant to the research theme. 7 , 11 These are initially developed from a general theory and branch into specific hypotheses by deductive reasoning. In the absence of a theory to base the hypotheses, inductive reasoning based on specific observations or findings form more general hypotheses. 10
TYPES OF RESEARCH QUESTIONS AND HYPOTHESES
Research questions and hypotheses are developed according to the type of research, which can be broadly classified into quantitative and qualitative research. We provide a summary of the types of research questions and hypotheses under quantitative and qualitative research categories in Table 1 .
Quantitative research questions | Quantitative research hypotheses |
---|---|
Descriptive research questions | Simple hypothesis |
Comparative research questions | Complex hypothesis |
Relationship research questions | Directional hypothesis |
Non-directional hypothesis | |
Associative hypothesis | |
Causal hypothesis | |
Null hypothesis | |
Alternative hypothesis | |
Working hypothesis | |
Statistical hypothesis | |
Logical hypothesis | |
Hypothesis-testing | |
Qualitative research questions | Qualitative research hypotheses |
Contextual research questions | Hypothesis-generating |
Descriptive research questions | |
Evaluation research questions | |
Explanatory research questions | |
Exploratory research questions | |
Generative research questions | |
Ideological research questions | |
Ethnographic research questions | |
Phenomenological research questions | |
Grounded theory questions | |
Qualitative case study questions |
Research questions in quantitative research
In quantitative research, research questions inquire about the relationships among variables being investigated and are usually framed at the start of the study. These are precise and typically linked to the subject population, dependent and independent variables, and research design. 1 Research questions may also attempt to describe the behavior of a population in relation to one or more variables, or describe the characteristics of variables to be measured ( descriptive research questions ). 1 , 5 , 14 These questions may also aim to discover differences between groups within the context of an outcome variable ( comparative research questions ), 1 , 5 , 14 or elucidate trends and interactions among variables ( relationship research questions ). 1 , 5 We provide examples of descriptive, comparative, and relationship research questions in quantitative research in Table 2 .
Quantitative research questions | |
---|---|
Descriptive research question | |
- Measures responses of subjects to variables | |
- Presents variables to measure, analyze, or assess | |
What is the proportion of resident doctors in the hospital who have mastered ultrasonography (response of subjects to a variable) as a diagnostic technique in their clinical training? | |
Comparative research question | |
- Clarifies difference between one group with outcome variable and another group without outcome variable | |
Is there a difference in the reduction of lung metastasis in osteosarcoma patients who received the vitamin D adjunctive therapy (group with outcome variable) compared with osteosarcoma patients who did not receive the vitamin D adjunctive therapy (group without outcome variable)? | |
- Compares the effects of variables | |
How does the vitamin D analogue 22-Oxacalcitriol (variable 1) mimic the antiproliferative activity of 1,25-Dihydroxyvitamin D (variable 2) in osteosarcoma cells? | |
Relationship research question | |
- Defines trends, association, relationships, or interactions between dependent variable and independent variable | |
Is there a relationship between the number of medical student suicide (dependent variable) and the level of medical student stress (independent variable) in Japan during the first wave of the COVID-19 pandemic? |
Hypotheses in quantitative research
In quantitative research, hypotheses predict the expected relationships among variables. 15 Relationships among variables that can be predicted include 1) between a single dependent variable and a single independent variable ( simple hypothesis ) or 2) between two or more independent and dependent variables ( complex hypothesis ). 4 , 11 Hypotheses may also specify the expected direction to be followed and imply an intellectual commitment to a particular outcome ( directional hypothesis ) 4 . On the other hand, hypotheses may not predict the exact direction and are used in the absence of a theory, or when findings contradict previous studies ( non-directional hypothesis ). 4 In addition, hypotheses can 1) define interdependency between variables ( associative hypothesis ), 4 2) propose an effect on the dependent variable from manipulation of the independent variable ( causal hypothesis ), 4 3) state a negative relationship between two variables ( null hypothesis ), 4 , 11 , 15 4) replace the working hypothesis if rejected ( alternative hypothesis ), 15 explain the relationship of phenomena to possibly generate a theory ( working hypothesis ), 11 5) involve quantifiable variables that can be tested statistically ( statistical hypothesis ), 11 6) or express a relationship whose interlinks can be verified logically ( logical hypothesis ). 11 We provide examples of simple, complex, directional, non-directional, associative, causal, null, alternative, working, statistical, and logical hypotheses in quantitative research, as well as the definition of quantitative hypothesis-testing research in Table 3 .
Quantitative research hypotheses | |
---|---|
Simple hypothesis | |
- Predicts relationship between single dependent variable and single independent variable | |
If the dose of the new medication (single independent variable) is high, blood pressure (single dependent variable) is lowered. | |
Complex hypothesis | |
- Foretells relationship between two or more independent and dependent variables | |
The higher the use of anticancer drugs, radiation therapy, and adjunctive agents (3 independent variables), the higher would be the survival rate (1 dependent variable). | |
Directional hypothesis | |
- Identifies study direction based on theory towards particular outcome to clarify relationship between variables | |
Privately funded research projects will have a larger international scope (study direction) than publicly funded research projects. | |
Non-directional hypothesis | |
- Nature of relationship between two variables or exact study direction is not identified | |
- Does not involve a theory | |
Women and men are different in terms of helpfulness. (Exact study direction is not identified) | |
Associative hypothesis | |
- Describes variable interdependency | |
- Change in one variable causes change in another variable | |
A larger number of people vaccinated against COVID-19 in the region (change in independent variable) will reduce the region’s incidence of COVID-19 infection (change in dependent variable). | |
Causal hypothesis | |
- An effect on dependent variable is predicted from manipulation of independent variable | |
A change into a high-fiber diet (independent variable) will reduce the blood sugar level (dependent variable) of the patient. | |
Null hypothesis | |
- A negative statement indicating no relationship or difference between 2 variables | |
There is no significant difference in the severity of pulmonary metastases between the new drug (variable 1) and the current drug (variable 2). | |
Alternative hypothesis | |
- Following a null hypothesis, an alternative hypothesis predicts a relationship between 2 study variables | |
The new drug (variable 1) is better on average in reducing the level of pain from pulmonary metastasis than the current drug (variable 2). | |
Working hypothesis | |
- A hypothesis that is initially accepted for further research to produce a feasible theory | |
Dairy cows fed with concentrates of different formulations will produce different amounts of milk. | |
Statistical hypothesis | |
- Assumption about the value of population parameter or relationship among several population characteristics | |
- Validity tested by a statistical experiment or analysis | |
The mean recovery rate from COVID-19 infection (value of population parameter) is not significantly different between population 1 and population 2. | |
There is a positive correlation between the level of stress at the workplace and the number of suicides (population characteristics) among working people in Japan. | |
Logical hypothesis | |
- Offers or proposes an explanation with limited or no extensive evidence | |
If healthcare workers provide more educational programs about contraception methods, the number of adolescent pregnancies will be less. | |
Hypothesis-testing (Quantitative hypothesis-testing research) | |
- Quantitative research uses deductive reasoning. | |
- This involves the formation of a hypothesis, collection of data in the investigation of the problem, analysis and use of the data from the investigation, and drawing of conclusions to validate or nullify the hypotheses. |
Research questions in qualitative research
Unlike research questions in quantitative research, research questions in qualitative research are usually continuously reviewed and reformulated. The central question and associated subquestions are stated more than the hypotheses. 15 The central question broadly explores a complex set of factors surrounding the central phenomenon, aiming to present the varied perspectives of participants. 15
There are varied goals for which qualitative research questions are developed. These questions can function in several ways, such as to 1) identify and describe existing conditions ( contextual research question s); 2) describe a phenomenon ( descriptive research questions ); 3) assess the effectiveness of existing methods, protocols, theories, or procedures ( evaluation research questions ); 4) examine a phenomenon or analyze the reasons or relationships between subjects or phenomena ( explanatory research questions ); or 5) focus on unknown aspects of a particular topic ( exploratory research questions ). 5 In addition, some qualitative research questions provide new ideas for the development of theories and actions ( generative research questions ) or advance specific ideologies of a position ( ideological research questions ). 1 Other qualitative research questions may build on a body of existing literature and become working guidelines ( ethnographic research questions ). Research questions may also be broadly stated without specific reference to the existing literature or a typology of questions ( phenomenological research questions ), may be directed towards generating a theory of some process ( grounded theory questions ), or may address a description of the case and the emerging themes ( qualitative case study questions ). 15 We provide examples of contextual, descriptive, evaluation, explanatory, exploratory, generative, ideological, ethnographic, phenomenological, grounded theory, and qualitative case study research questions in qualitative research in Table 4 , and the definition of qualitative hypothesis-generating research in Table 5 .
Qualitative research questions | |
---|---|
Contextual research question | |
- Ask the nature of what already exists | |
- Individuals or groups function to further clarify and understand the natural context of real-world problems | |
What are the experiences of nurses working night shifts in healthcare during the COVID-19 pandemic? (natural context of real-world problems) | |
Descriptive research question | |
- Aims to describe a phenomenon | |
What are the different forms of disrespect and abuse (phenomenon) experienced by Tanzanian women when giving birth in healthcare facilities? | |
Evaluation research question | |
- Examines the effectiveness of existing practice or accepted frameworks | |
How effective are decision aids (effectiveness of existing practice) in helping decide whether to give birth at home or in a healthcare facility? | |
Explanatory research question | |
- Clarifies a previously studied phenomenon and explains why it occurs | |
Why is there an increase in teenage pregnancy (phenomenon) in Tanzania? | |
Exploratory research question | |
- Explores areas that have not been fully investigated to have a deeper understanding of the research problem | |
What factors affect the mental health of medical students (areas that have not yet been fully investigated) during the COVID-19 pandemic? | |
Generative research question | |
- Develops an in-depth understanding of people’s behavior by asking ‘how would’ or ‘what if’ to identify problems and find solutions | |
How would the extensive research experience of the behavior of new staff impact the success of the novel drug initiative? | |
Ideological research question | |
- Aims to advance specific ideas or ideologies of a position | |
Are Japanese nurses who volunteer in remote African hospitals able to promote humanized care of patients (specific ideas or ideologies) in the areas of safe patient environment, respect of patient privacy, and provision of accurate information related to health and care? | |
Ethnographic research question | |
- Clarifies peoples’ nature, activities, their interactions, and the outcomes of their actions in specific settings | |
What are the demographic characteristics, rehabilitative treatments, community interactions, and disease outcomes (nature, activities, their interactions, and the outcomes) of people in China who are suffering from pneumoconiosis? | |
Phenomenological research question | |
- Knows more about the phenomena that have impacted an individual | |
What are the lived experiences of parents who have been living with and caring for children with a diagnosis of autism? (phenomena that have impacted an individual) | |
Grounded theory question | |
- Focuses on social processes asking about what happens and how people interact, or uncovering social relationships and behaviors of groups | |
What are the problems that pregnant adolescents face in terms of social and cultural norms (social processes), and how can these be addressed? | |
Qualitative case study question | |
- Assesses a phenomenon using different sources of data to answer “why” and “how” questions | |
- Considers how the phenomenon is influenced by its contextual situation. | |
How does quitting work and assuming the role of a full-time mother (phenomenon assessed) change the lives of women in Japan? |
Qualitative research hypotheses | |
---|---|
Hypothesis-generating (Qualitative hypothesis-generating research) | |
- Qualitative research uses inductive reasoning. | |
- This involves data collection from study participants or the literature regarding a phenomenon of interest, using the collected data to develop a formal hypothesis, and using the formal hypothesis as a framework for testing the hypothesis. | |
- Qualitative exploratory studies explore areas deeper, clarifying subjective experience and allowing formulation of a formal hypothesis potentially testable in a future quantitative approach. |
Qualitative studies usually pose at least one central research question and several subquestions starting with How or What . These research questions use exploratory verbs such as explore or describe . These also focus on one central phenomenon of interest, and may mention the participants and research site. 15
Hypotheses in qualitative research
Hypotheses in qualitative research are stated in the form of a clear statement concerning the problem to be investigated. Unlike in quantitative research where hypotheses are usually developed to be tested, qualitative research can lead to both hypothesis-testing and hypothesis-generating outcomes. 2 When studies require both quantitative and qualitative research questions, this suggests an integrative process between both research methods wherein a single mixed-methods research question can be developed. 1
FRAMEWORKS FOR DEVELOPING RESEARCH QUESTIONS AND HYPOTHESES
Research questions followed by hypotheses should be developed before the start of the study. 1 , 12 , 14 It is crucial to develop feasible research questions on a topic that is interesting to both the researcher and the scientific community. This can be achieved by a meticulous review of previous and current studies to establish a novel topic. Specific areas are subsequently focused on to generate ethical research questions. The relevance of the research questions is evaluated in terms of clarity of the resulting data, specificity of the methodology, objectivity of the outcome, depth of the research, and impact of the study. 1 , 5 These aspects constitute the FINER criteria (i.e., Feasible, Interesting, Novel, Ethical, and Relevant). 1 Clarity and effectiveness are achieved if research questions meet the FINER criteria. In addition to the FINER criteria, Ratan et al. described focus, complexity, novelty, feasibility, and measurability for evaluating the effectiveness of research questions. 14
The PICOT and PEO frameworks are also used when developing research questions. 1 The following elements are addressed in these frameworks, PICOT: P-population/patients/problem, I-intervention or indicator being studied, C-comparison group, O-outcome of interest, and T-timeframe of the study; PEO: P-population being studied, E-exposure to preexisting conditions, and O-outcome of interest. 1 Research questions are also considered good if these meet the “FINERMAPS” framework: Feasible, Interesting, Novel, Ethical, Relevant, Manageable, Appropriate, Potential value/publishable, and Systematic. 14
As we indicated earlier, research questions and hypotheses that are not carefully formulated result in unethical studies or poor outcomes. To illustrate this, we provide some examples of ambiguous research question and hypotheses that result in unclear and weak research objectives in quantitative research ( Table 6 ) 16 and qualitative research ( Table 7 ) 17 , and how to transform these ambiguous research question(s) and hypothesis(es) into clear and good statements.
Variables | Unclear and weak statement (Statement 1) | Clear and good statement (Statement 2) | Points to avoid |
---|---|---|---|
Research question | Which is more effective between smoke moxibustion and smokeless moxibustion? | “Moreover, regarding smoke moxibustion versus smokeless moxibustion, it remains unclear which is more effective, safe, and acceptable to pregnant women, and whether there is any difference in the amount of heat generated.” | 1) Vague and unfocused questions |
2) Closed questions simply answerable by yes or no | |||
3) Questions requiring a simple choice | |||
Hypothesis | The smoke moxibustion group will have higher cephalic presentation. | “Hypothesis 1. The smoke moxibustion stick group (SM group) and smokeless moxibustion stick group (-SLM group) will have higher rates of cephalic presentation after treatment than the control group. | 1) Unverifiable hypotheses |
Hypothesis 2. The SM group and SLM group will have higher rates of cephalic presentation at birth than the control group. | 2) Incompletely stated groups of comparison | ||
Hypothesis 3. There will be no significant differences in the well-being of the mother and child among the three groups in terms of the following outcomes: premature birth, premature rupture of membranes (PROM) at < 37 weeks, Apgar score < 7 at 5 min, umbilical cord blood pH < 7.1, admission to neonatal intensive care unit (NICU), and intrauterine fetal death.” | 3) Insufficiently described variables or outcomes | ||
Research objective | To determine which is more effective between smoke moxibustion and smokeless moxibustion. | “The specific aims of this pilot study were (a) to compare the effects of smoke moxibustion and smokeless moxibustion treatments with the control group as a possible supplement to ECV for converting breech presentation to cephalic presentation and increasing adherence to the newly obtained cephalic position, and (b) to assess the effects of these treatments on the well-being of the mother and child.” | 1) Poor understanding of the research question and hypotheses |
2) Insufficient description of population, variables, or study outcomes |
a These statements were composed for comparison and illustrative purposes only.
b These statements are direct quotes from Higashihara and Horiuchi. 16
Variables | Unclear and weak statement (Statement 1) | Clear and good statement (Statement 2) | Points to avoid |
---|---|---|---|
Research question | Does disrespect and abuse (D&A) occur in childbirth in Tanzania? | How does disrespect and abuse (D&A) occur and what are the types of physical and psychological abuses observed in midwives’ actual care during facility-based childbirth in urban Tanzania? | 1) Ambiguous or oversimplistic questions |
2) Questions unverifiable by data collection and analysis | |||
Hypothesis | Disrespect and abuse (D&A) occur in childbirth in Tanzania. | Hypothesis 1: Several types of physical and psychological abuse by midwives in actual care occur during facility-based childbirth in urban Tanzania. | 1) Statements simply expressing facts |
Hypothesis 2: Weak nursing and midwifery management contribute to the D&A of women during facility-based childbirth in urban Tanzania. | 2) Insufficiently described concepts or variables | ||
Research objective | To describe disrespect and abuse (D&A) in childbirth in Tanzania. | “This study aimed to describe from actual observations the respectful and disrespectful care received by women from midwives during their labor period in two hospitals in urban Tanzania.” | 1) Statements unrelated to the research question and hypotheses |
2) Unattainable or unexplorable objectives |
a This statement is a direct quote from Shimoda et al. 17
The other statements were composed for comparison and illustrative purposes only.
CONSTRUCTING RESEARCH QUESTIONS AND HYPOTHESES
To construct effective research questions and hypotheses, it is very important to 1) clarify the background and 2) identify the research problem at the outset of the research, within a specific timeframe. 9 Then, 3) review or conduct preliminary research to collect all available knowledge about the possible research questions by studying theories and previous studies. 18 Afterwards, 4) construct research questions to investigate the research problem. Identify variables to be accessed from the research questions 4 and make operational definitions of constructs from the research problem and questions. Thereafter, 5) construct specific deductive or inductive predictions in the form of hypotheses. 4 Finally, 6) state the study aims . This general flow for constructing effective research questions and hypotheses prior to conducting research is shown in Fig. 1 .

Research questions are used more frequently in qualitative research than objectives or hypotheses. 3 These questions seek to discover, understand, explore or describe experiences by asking “What” or “How.” The questions are open-ended to elicit a description rather than to relate variables or compare groups. The questions are continually reviewed, reformulated, and changed during the qualitative study. 3 Research questions are also used more frequently in survey projects than hypotheses in experiments in quantitative research to compare variables and their relationships.
Hypotheses are constructed based on the variables identified and as an if-then statement, following the template, ‘If a specific action is taken, then a certain outcome is expected.’ At this stage, some ideas regarding expectations from the research to be conducted must be drawn. 18 Then, the variables to be manipulated (independent) and influenced (dependent) are defined. 4 Thereafter, the hypothesis is stated and refined, and reproducible data tailored to the hypothesis are identified, collected, and analyzed. 4 The hypotheses must be testable and specific, 18 and should describe the variables and their relationships, the specific group being studied, and the predicted research outcome. 18 Hypotheses construction involves a testable proposition to be deduced from theory, and independent and dependent variables to be separated and measured separately. 3 Therefore, good hypotheses must be based on good research questions constructed at the start of a study or trial. 12
In summary, research questions are constructed after establishing the background of the study. Hypotheses are then developed based on the research questions. Thus, it is crucial to have excellent research questions to generate superior hypotheses. In turn, these would determine the research objectives and the design of the study, and ultimately, the outcome of the research. 12 Algorithms for building research questions and hypotheses are shown in Fig. 2 for quantitative research and in Fig. 3 for qualitative research.

EXAMPLES OF RESEARCH QUESTIONS FROM PUBLISHED ARTICLES
- EXAMPLE 1. Descriptive research question (quantitative research)
- - Presents research variables to be assessed (distinct phenotypes and subphenotypes)
- “BACKGROUND: Since COVID-19 was identified, its clinical and biological heterogeneity has been recognized. Identifying COVID-19 phenotypes might help guide basic, clinical, and translational research efforts.
- RESEARCH QUESTION: Does the clinical spectrum of patients with COVID-19 contain distinct phenotypes and subphenotypes? ” 19
- EXAMPLE 2. Relationship research question (quantitative research)
- - Shows interactions between dependent variable (static postural control) and independent variable (peripheral visual field loss)
- “Background: Integration of visual, vestibular, and proprioceptive sensations contributes to postural control. People with peripheral visual field loss have serious postural instability. However, the directional specificity of postural stability and sensory reweighting caused by gradual peripheral visual field loss remain unclear.
- Research question: What are the effects of peripheral visual field loss on static postural control ?” 20
- EXAMPLE 3. Comparative research question (quantitative research)
- - Clarifies the difference among groups with an outcome variable (patients enrolled in COMPERA with moderate PH or severe PH in COPD) and another group without the outcome variable (patients with idiopathic pulmonary arterial hypertension (IPAH))
- “BACKGROUND: Pulmonary hypertension (PH) in COPD is a poorly investigated clinical condition.
- RESEARCH QUESTION: Which factors determine the outcome of PH in COPD?
- STUDY DESIGN AND METHODS: We analyzed the characteristics and outcome of patients enrolled in the Comparative, Prospective Registry of Newly Initiated Therapies for Pulmonary Hypertension (COMPERA) with moderate or severe PH in COPD as defined during the 6th PH World Symposium who received medical therapy for PH and compared them with patients with idiopathic pulmonary arterial hypertension (IPAH) .” 21
- EXAMPLE 4. Exploratory research question (qualitative research)
- - Explores areas that have not been fully investigated (perspectives of families and children who receive care in clinic-based child obesity treatment) to have a deeper understanding of the research problem
- “Problem: Interventions for children with obesity lead to only modest improvements in BMI and long-term outcomes, and data are limited on the perspectives of families of children with obesity in clinic-based treatment. This scoping review seeks to answer the question: What is known about the perspectives of families and children who receive care in clinic-based child obesity treatment? This review aims to explore the scope of perspectives reported by families of children with obesity who have received individualized outpatient clinic-based obesity treatment.” 22
- EXAMPLE 5. Relationship research question (quantitative research)
- - Defines interactions between dependent variable (use of ankle strategies) and independent variable (changes in muscle tone)
- “Background: To maintain an upright standing posture against external disturbances, the human body mainly employs two types of postural control strategies: “ankle strategy” and “hip strategy.” While it has been reported that the magnitude of the disturbance alters the use of postural control strategies, it has not been elucidated how the level of muscle tone, one of the crucial parameters of bodily function, determines the use of each strategy. We have previously confirmed using forward dynamics simulations of human musculoskeletal models that an increased muscle tone promotes the use of ankle strategies. The objective of the present study was to experimentally evaluate a hypothesis: an increased muscle tone promotes the use of ankle strategies. Research question: Do changes in the muscle tone affect the use of ankle strategies ?” 23
EXAMPLES OF HYPOTHESES IN PUBLISHED ARTICLES
- EXAMPLE 1. Working hypothesis (quantitative research)
- - A hypothesis that is initially accepted for further research to produce a feasible theory
- “As fever may have benefit in shortening the duration of viral illness, it is plausible to hypothesize that the antipyretic efficacy of ibuprofen may be hindering the benefits of a fever response when taken during the early stages of COVID-19 illness .” 24
- “In conclusion, it is plausible to hypothesize that the antipyretic efficacy of ibuprofen may be hindering the benefits of a fever response . The difference in perceived safety of these agents in COVID-19 illness could be related to the more potent efficacy to reduce fever with ibuprofen compared to acetaminophen. Compelling data on the benefit of fever warrant further research and review to determine when to treat or withhold ibuprofen for early stage fever for COVID-19 and other related viral illnesses .” 24
- EXAMPLE 2. Exploratory hypothesis (qualitative research)
- - Explores particular areas deeper to clarify subjective experience and develop a formal hypothesis potentially testable in a future quantitative approach
- “We hypothesized that when thinking about a past experience of help-seeking, a self distancing prompt would cause increased help-seeking intentions and more favorable help-seeking outcome expectations .” 25
- “Conclusion
- Although a priori hypotheses were not supported, further research is warranted as results indicate the potential for using self-distancing approaches to increasing help-seeking among some people with depressive symptomatology.” 25
- EXAMPLE 3. Hypothesis-generating research to establish a framework for hypothesis testing (qualitative research)
- “We hypothesize that compassionate care is beneficial for patients (better outcomes), healthcare systems and payers (lower costs), and healthcare providers (lower burnout). ” 26
- Compassionomics is the branch of knowledge and scientific study of the effects of compassionate healthcare. Our main hypotheses are that compassionate healthcare is beneficial for (1) patients, by improving clinical outcomes, (2) healthcare systems and payers, by supporting financial sustainability, and (3) HCPs, by lowering burnout and promoting resilience and well-being. The purpose of this paper is to establish a scientific framework for testing the hypotheses above . If these hypotheses are confirmed through rigorous research, compassionomics will belong in the science of evidence-based medicine, with major implications for all healthcare domains.” 26
- EXAMPLE 4. Statistical hypothesis (quantitative research)
- - An assumption is made about the relationship among several population characteristics ( gender differences in sociodemographic and clinical characteristics of adults with ADHD ). Validity is tested by statistical experiment or analysis ( chi-square test, Students t-test, and logistic regression analysis)
- “Our research investigated gender differences in sociodemographic and clinical characteristics of adults with ADHD in a Japanese clinical sample. Due to unique Japanese cultural ideals and expectations of women's behavior that are in opposition to ADHD symptoms, we hypothesized that women with ADHD experience more difficulties and present more dysfunctions than men . We tested the following hypotheses: first, women with ADHD have more comorbidities than men with ADHD; second, women with ADHD experience more social hardships than men, such as having less full-time employment and being more likely to be divorced.” 27
- “Statistical Analysis
- ( text omitted ) Between-gender comparisons were made using the chi-squared test for categorical variables and Students t-test for continuous variables…( text omitted ). A logistic regression analysis was performed for employment status, marital status, and comorbidity to evaluate the independent effects of gender on these dependent variables.” 27
EXAMPLES OF HYPOTHESIS AS WRITTEN IN PUBLISHED ARTICLES IN RELATION TO OTHER PARTS
- EXAMPLE 1. Background, hypotheses, and aims are provided
- “Pregnant women need skilled care during pregnancy and childbirth, but that skilled care is often delayed in some countries …( text omitted ). The focused antenatal care (FANC) model of WHO recommends that nurses provide information or counseling to all pregnant women …( text omitted ). Job aids are visual support materials that provide the right kind of information using graphics and words in a simple and yet effective manner. When nurses are not highly trained or have many work details to attend to, these job aids can serve as a content reminder for the nurses and can be used for educating their patients (Jennings, Yebadokpo, Affo, & Agbogbe, 2010) ( text omitted ). Importantly, additional evidence is needed to confirm how job aids can further improve the quality of ANC counseling by health workers in maternal care …( text omitted )” 28
- “ This has led us to hypothesize that the quality of ANC counseling would be better if supported by job aids. Consequently, a better quality of ANC counseling is expected to produce higher levels of awareness concerning the danger signs of pregnancy and a more favorable impression of the caring behavior of nurses .” 28
- “This study aimed to examine the differences in the responses of pregnant women to a job aid-supported intervention during ANC visit in terms of 1) their understanding of the danger signs of pregnancy and 2) their impression of the caring behaviors of nurses to pregnant women in rural Tanzania.” 28
- EXAMPLE 2. Background, hypotheses, and aims are provided
- “We conducted a two-arm randomized controlled trial (RCT) to evaluate and compare changes in salivary cortisol and oxytocin levels of first-time pregnant women between experimental and control groups. The women in the experimental group touched and held an infant for 30 min (experimental intervention protocol), whereas those in the control group watched a DVD movie of an infant (control intervention protocol). The primary outcome was salivary cortisol level and the secondary outcome was salivary oxytocin level.” 29
- “ We hypothesize that at 30 min after touching and holding an infant, the salivary cortisol level will significantly decrease and the salivary oxytocin level will increase in the experimental group compared with the control group .” 29
- EXAMPLE 3. Background, aim, and hypothesis are provided
- “In countries where the maternal mortality ratio remains high, antenatal education to increase Birth Preparedness and Complication Readiness (BPCR) is considered one of the top priorities [1]. BPCR includes birth plans during the antenatal period, such as the birthplace, birth attendant, transportation, health facility for complications, expenses, and birth materials, as well as family coordination to achieve such birth plans. In Tanzania, although increasing, only about half of all pregnant women attend an antenatal clinic more than four times [4]. Moreover, the information provided during antenatal care (ANC) is insufficient. In the resource-poor settings, antenatal group education is a potential approach because of the limited time for individual counseling at antenatal clinics.” 30
- “This study aimed to evaluate an antenatal group education program among pregnant women and their families with respect to birth-preparedness and maternal and infant outcomes in rural villages of Tanzania.” 30
- “ The study hypothesis was if Tanzanian pregnant women and their families received a family-oriented antenatal group education, they would (1) have a higher level of BPCR, (2) attend antenatal clinic four or more times, (3) give birth in a health facility, (4) have less complications of women at birth, and (5) have less complications and deaths of infants than those who did not receive the education .” 30
Research questions and hypotheses are crucial components to any type of research, whether quantitative or qualitative. These questions should be developed at the very beginning of the study. Excellent research questions lead to superior hypotheses, which, like a compass, set the direction of research, and can often determine the successful conduct of the study. Many research studies have floundered because the development of research questions and subsequent hypotheses was not given the thought and meticulous attention needed. The development of research questions and hypotheses is an iterative process based on extensive knowledge of the literature and insightful grasp of the knowledge gap. Focused, concise, and specific research questions provide a strong foundation for constructing hypotheses which serve as formal predictions about the research outcomes. Research questions and hypotheses are crucial elements of research that should not be overlooked. They should be carefully thought of and constructed when planning research. This avoids unethical studies and poor outcomes by defining well-founded objectives that determine the design, course, and outcome of the study.
Disclosure: The authors have no potential conflicts of interest to disclose.
Author Contributions:
- Conceptualization: Barroga E, Matanguihan GJ.
- Methodology: Barroga E, Matanguihan GJ.
- Writing - original draft: Barroga E, Matanguihan GJ.
- Writing - review & editing: Barroga E, Matanguihan GJ.
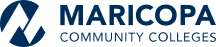
10 Chapter 10: Hypothesis Testing with Z
Setting up the hypotheses.
When setting up the hypotheses with z, the parameter is associated with a sample mean (in the previous chapter examples the parameters for the null used 0). Using z is an occasion in which the null hypothesis is a value other than 0. For example, if we are working with mothers in the U.S. whose children are at risk of low birth weight, we can use 7.47 pounds, the average birth weight in the US, as our null value and test for differences against that. For now, we will focus on testing a value of a single mean against what we expect from the population.
Using birthweight as an example, our null hypothesis takes the form: H 0 : μ = 7.47 Notice that we are testing the value for μ, the population parameter, NOT the sample statistic ̅X (or M). We are referring to the data right now in raw form (we have not standardized it using z yet). Again, using inferential statistics, we are interested in understanding the population, drawing from our sample observations. For the research question, we have a mean value from the sample to use, we have specific data is – it is observed and used as a comparison for a set point.
As mentioned earlier, the alternative hypothesis is simply the reverse of the null hypothesis, and there are three options, depending on where we expect the difference to lie. We will set the criteria for rejecting the null hypothesis based on the directionality (greater than, less than, or not equal to) of the alternative.
If we expect our obtained sample mean to be above or below the null hypothesis value (knowing which direction), we set a directional hypothesis. O ur alternative hypothesis takes the form based on the research question itself. In our example with birthweight, this could be presented as H A : μ > 7.47 or H A : μ < 7.47.
Note that we should only use a directional hypothesis if we have a good reason, based on prior observations or research, to suspect a particular direction. When we do not know the direction, such as when we are entering a new area of research, we use a non-directional alternative hypothesis. In our birthweight example, this could be set as H A : μ ≠ 7.47
In working with data for this course we will need to set a critical value of the test statistic for alpha (α) for use of test statistic tables in the back of the book. This is determining the critical rejection region that has a set critical value based on α.
Determining Critical Value from α
We set alpha (α) before collecting data in order to determine whether or not we should reject the null hypothesis. We set this value beforehand to avoid biasing ourselves by viewing our results and then determining what criteria we should use.
When a research hypothesis predicts an effect but does not predict a direction for the effect, it is called a non-directional hypothesis . To test the significance of a non-directional hypothesis, we have to consider the possibility that the sample could be extreme at either tail of the comparison distribution. We call this a two-tailed test .
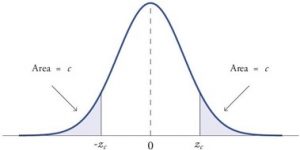
Figure 1. showing a 2-tail test for non-directional hypothesis for z for area C is the critical rejection region.
When a research hypothesis predicts a direction for the effect, it is called a directional hypothesis . To test the significance of a directional hypothesis, we have to consider the possibility that the sample could be extreme at one-tail of the comparison distribution. We call this a one-tailed test .
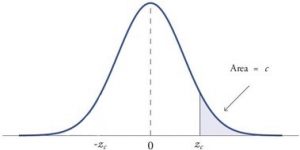
Figure 2. showing a 1-tail test for a directional hypothesis (predicting an increase) for z for area C is the critical rejection region.
Determining Cutoff Scores with Two-Tailed Tests
Typically we specify an α level before analyzing the data. If the data analysis results in a probability value below the α level, then the null hypothesis is rejected; if it is not, then the null hypothesis is not rejected. In other words, if our data produce values that meet or exceed this threshold, then we have sufficient evidence to reject the null hypothesis ; if not, we fail to reject the null (we never “accept” the null). According to this perspective, if a result is significant, then it does not matter how significant it is. Moreover, if it is not significant, then it does not matter how close to being significant it is. Therefore, if the 0.05 level is being used, then probability values of 0.049 and 0.001 are treated identically. Similarly, probability values of 0.06 and 0.34 are treated identically. Note we will discuss ways to address effect size (which is related to this challenge of NHST).
When setting the probability value, there is a special complication in a two-tailed test. We have to divide the significance percentage between the two tails. For example, with a 5% significance level, we reject the null hypothesis only if the sample is so extreme that it is in either the top 2.5% or the bottom 2.5% of the comparison distribution. This keeps the overall level of significance at a total of 5%. A one-tailed test does have such an extreme value but with a one-tailed test only one side of the distribution is considered.
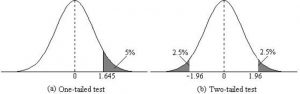
Figure 3. Critical value differences in one and two-tail tests. Photo Credit
Let’s re view th e set critical values for Z.
We discussed z-scores and probability in chapter 8. If we revisit the z-score for 5% and 1%, we can identify the critical regions for the critical rejection areas from the unit standard normal table.
- A two-tailed test at the 5% level has a critical boundary Z score of +1.96 and -1.96
- A one-tailed test at the 5% level has a critical boundary Z score of +1.64 or -1.64
- A two-tailed test at the 1% level has a critical boundary Z score of +2.58 and -2.58
- A one-tailed test at the 1% level has a critical boundary Z score of +2.33 or -2.33.
Review: Critical values, p-values, and significance level
There are two criteria we use to assess whether our data meet the thresholds established by our chosen significance level, and they both have to do with our discussions of probability and distributions. Recall that probability refers to the likelihood of an event, given some situation or set of conditions. In hypothesis testing, that situation is the assumption that the null hypothesis value is the correct value, or that there is no effec t. The value laid out in H 0 is our condition under which we interpret our results. To reject this assumption, and thereby reject the null hypothesis, we need results that would be very unlikely if the null was true.
Now recall that values of z which fall in the tails of the standard normal distribution represent unlikely values. That is, the proportion of the area under the curve as or more extreme than z is very small as we get into the tails of the distribution. Our significance level corresponds to the area under the tail that is exactly equal to α: if we use our normal criterion of α = .05, then 5% of the area under the curve becomes what we call the rejection region (also called the critical region) of the distribution. This is illustrated in Figure 4.
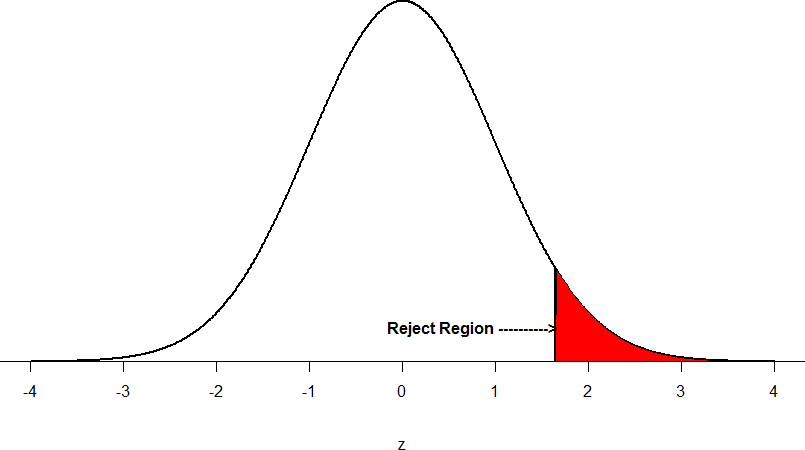
Figure 4: The rejection region for a one-tailed test
The shaded rejection region takes us 5% of the area under the curve. Any result which falls in that region is sufficient evidence to reject the null hypothesis.
The rejection region is bounded by a specific z-value, as is any area under the curve. In hypothesis testing, the value corresponding to a specific rejection region is called the critical value, z crit (“z-crit”) or z* (hence the other name “critical region”). Finding the critical value works exactly the same as finding the z-score corresponding to any area under the curve like we did in Unit 1. If we go to the normal table, we will find that the z-score corresponding to 5% of the area under the curve is equal to 1.645 (z = 1.64 corresponds to 0.0405 and z = 1.65 corresponds to 0.0495, so .05 is exactly in between them) if we go to the right and -1.645 if we go to the left. The direction must be determined by your alternative hypothesis, and drawing then shading the distribution is helpful for keeping directionality straight.
Suppose, however, that we want to do a non-directional test. We need to put the critical region in both tails, but we don’t want to increase the overall size of the rejection region (for reasons we will see later). To do this, we simply split it in half so that an equal proportion of the area under the curve falls in each tail’s rejection region. For α = .05, this means 2.5% of the area is in each tail, which, based on the z-table, corresponds to critical values of z* = ±1.96. This is shown in Figure 5.
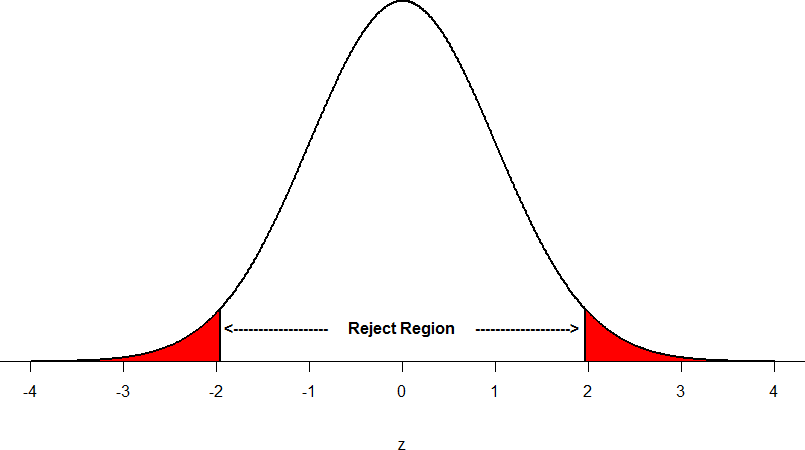
Figure 5: Two-tailed rejection region
Thus, any z-score falling outside ±1.96 (greater than 1.96 in absolute value) falls in the rejection region. When we use z-scores in this way, the obtained value of z (sometimes called z-obtained) is something known as a test statistic, which is simply an inferential statistic used to test a null hypothesis.
Calculate the test statistic: Z
Now that we understand setting up the hypothesis and determining the outcome, let’s examine hypothesis testing with z! The next step is to carry out the study and get the actual results for our sample. Central to hypothesis test is comparison of the population and sample means. To make our calculation and determine where the sample is in the hypothesized distribution we calculate the Z for the sample data.
Make a decision
To decide whether to reject the null hypothesis, we compare our sample’s Z score to the Z score that marks our critical boundary. If our sample Z score falls inside the rejection region of the comparison distribution (is greater than the z-score critical boundary) we reject the null hypothesis.
The formula for our z- statistic has not changed:
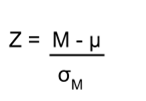
To formally test our hypothesis, we compare our obtained z-statistic to our critical z-value. If z obt > z crit , that means it falls in the rejection region (to see why, draw a line for z = 2.5 on Figure 1 or Figure 2) and so we reject H 0 . If z obt < z crit , we fail to reject. Remember that as z gets larger, the corresponding area under the curve beyond z gets smaller. Thus, the proportion, or p-value, will be smaller than the area for α, and if the area is smaller, the probability gets smaller. Specifically, the probability of obtaining that result, or a more extreme result, under the condition that the null hypothesis is true gets smaller.
Conversely, if we fail to reject, we know that the proportion will be larger than α because the z-statistic will not be as far into the tail. This is illustrated for a one- tailed test in Figure 6.
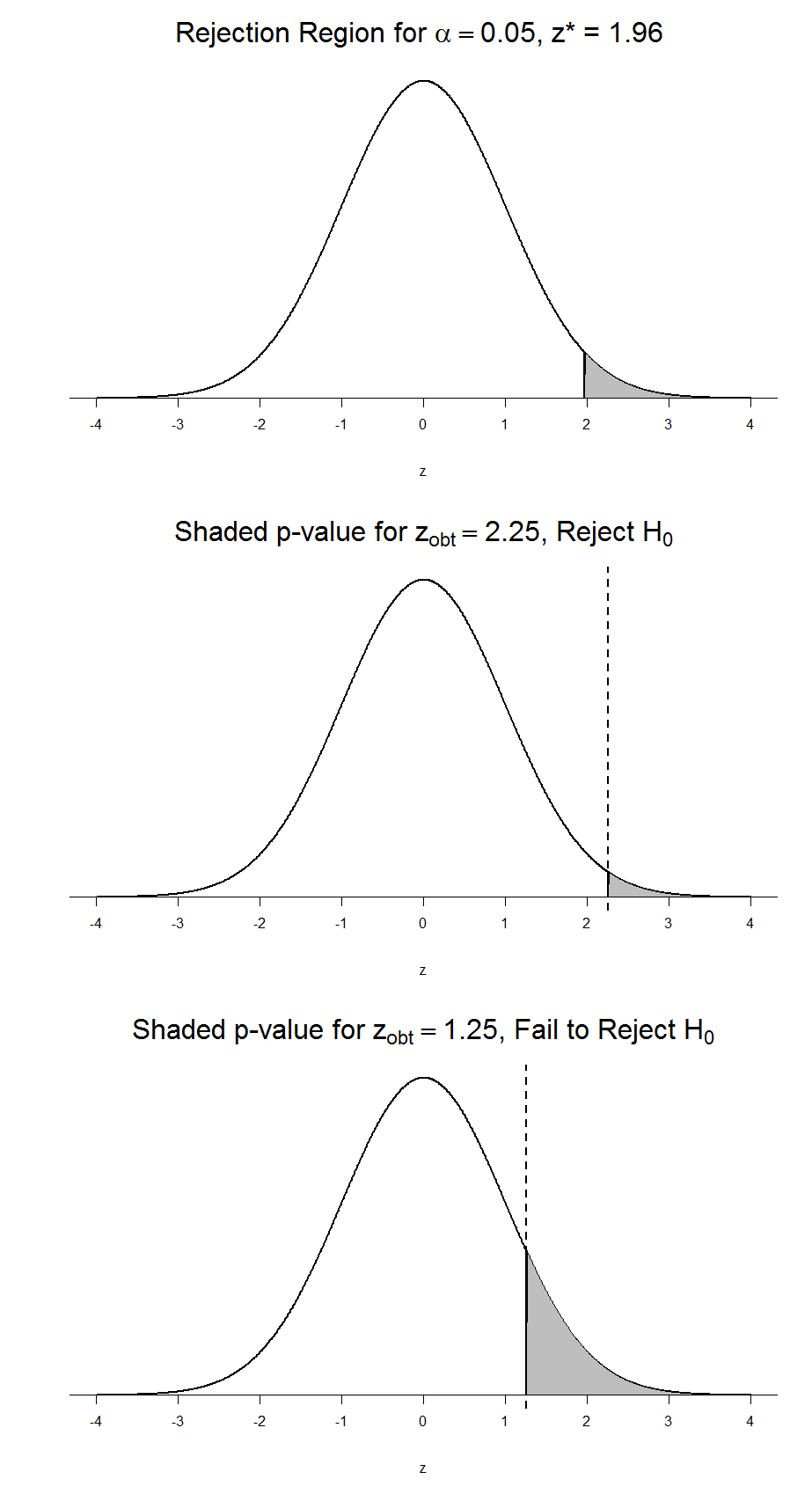
Figure 6. Relation between α, z obt , and p
When the null hypothesis is rejected, the effect is said to be statistically significant . Do not confuse statistical significance with practical significance. A small effect can be highly significant if the sample size is large enough.
Why does the word “significant” in the phrase “statistically significant” mean something so different from other uses of the word? Interestingly, this is because the meaning of “significant” in everyday language has changed. It turns out that when the procedures for hypothesis testing were developed, something was “significant” if it signified something. Thus, finding that an effect is statistically significant signifies that the effect is real and not due to chance. Over the years, the meaning of “significant” changed, leading to the potential misinterpretation.
Review: Steps of the Hypothesis Testing Process
The process of testing hypotheses follows a simple four-step procedure. This process will be what we use for the remained of the textbook and course, and though the hypothesis and statistics we use will change, this process will not.
Step 1: State the Hypotheses
Your hypotheses are the first thing you need to lay out. Otherwise, there is nothing to test! You have to state the null hypothesis (which is what we test) and the alternative hypothesis (which is what we expect). These should be stated mathematically as they were presented above AND in words, explaining in normal English what each one means in terms of the research question.
Step 2: Find the Critical Values
Next, we formally lay out the criteria we will use to test our hypotheses. There are two pieces of information that inform our critical values: α, which determines how much of the area under the curve composes our rejection region, and the directionality of the test, which determines where the region will be.

Step 3: Compute the Test Statistic
Once we have our hypotheses and the standards we use to test them, we can collect data and calculate our test statistic, in this case z . This step is where the vast majority of differences in future chapters will arise: different tests used for different data are calculated in different ways, but the way we use and interpret them remains the same.
Step 4: Make the Decision
Finally, once we have our obtained test statistic, we can compare it to our critical value and decide whether we should reject or fail to reject the null hypothesis. When we do this, we must interpret the decision in relation to our research question, stating what we concluded, what we based our conclusion on, and the specific statistics we obtained.
Example: Movie Popcorn
Let’s see how hypothesis testing works in action by working through an example. Say that a movie theater owner likes to keep a very close eye on how much popcorn goes into each bag sold, so he knows that the average bag has 8 cups of popcorn and that this varies a little bit, about half a cup. That is, the known population mean is μ = 8.00 and the known population standard deviation is σ =0.50. The owner wants to make sure that the newest employee is filling bags correctly, so over the course of a week he randomly assesses 25 bags filled by the employee to test for a difference (n = 25). He doesn’t want bags overfilled or under filled, so he looks for differences in both directions. This scenario has all of the information we need to begin our hypothesis testing procedure.
Our manager is looking for a difference in the mean cups of popcorn bags compared to the population mean of 8. We will need both a null and an alternative hypothesis written both mathematically and in words. We’ll always start with the null hypothesis:
H 0 : There is no difference in the cups of popcorn bags from this employee H 0 : μ = 8.00
Notice that we phrase the hypothesis in terms of the population parameter μ, which in this case would be the true average cups of bags filled by the new employee.
Our assumption of no difference, the null hypothesis, is that this mean is exactly
the same as the known population mean value we want it to match, 8.00. Now let’s do the alternative:
H A : There is a difference in the cups of popcorn bags from this employee H A : μ ≠ 8.00
In this case, we don’t know if the bags will be too full or not full enough, so we do a two-tailed alternative hypothesis that there is a difference.
Our critical values are based on two things: the directionality of the test and the level of significance. We decided in step 1 that a two-tailed test is the appropriate directionality. We were given no information about the level of significance, so we assume that α = 0.05 is what we will use. As stated earlier in the chapter, the critical values for a two-tailed z-test at α = 0.05 are z* = ±1.96. This will be the criteria we use to test our hypothesis. We can now draw out our distribution so we can visualize the rejection region and make sure it makes sense
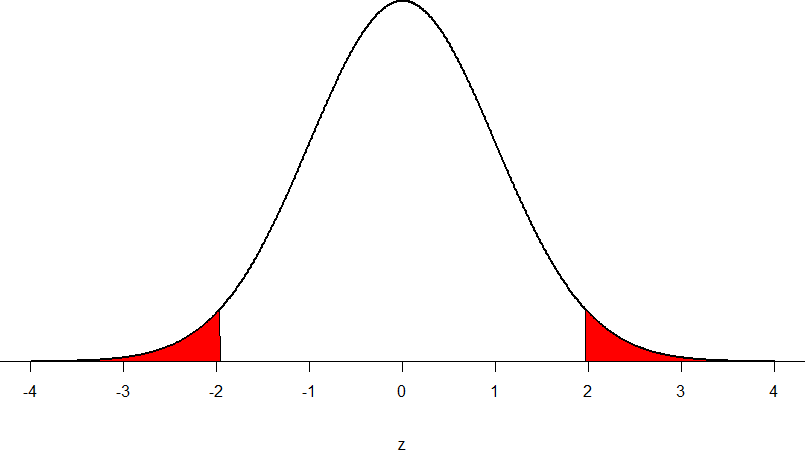
Figure 7: Rejection region for z* = ±1.96
Step 3: Calculate the Test Statistic
Now we come to our formal calculations. Let’s say that the manager collects data and finds that the average cups of this employee’s popcorn bags is ̅X = 7.75 cups. We can now plug this value, along with the values presented in the original problem, into our equation for z:
So our test statistic is z = -2.50, which we can draw onto our rejection region distribution:
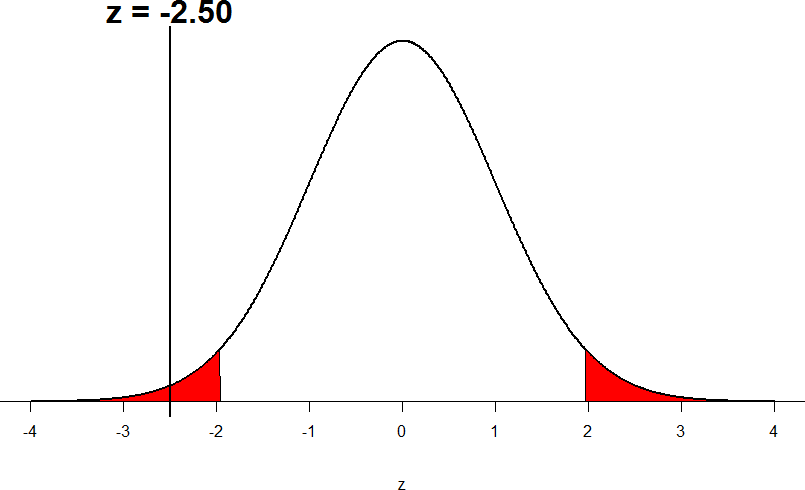
Figure 8: Test statistic location
Looking at Figure 5, we can see that our obtained z-statistic falls in the rejection region. We can also directly compare it to our critical value: in terms of absolute value, -2.50 > -1.96, so we reject the null hypothesis. We can now write our conclusion:
When we write our conclusion, we write out the words to communicate what it actually means, but we also include the average sample size we calculated (the exact location doesn’t matter, just somewhere that flows naturally and makes sense) and the z-statistic and p-value. We don’t know the exact p-value, but we do know that because we rejected the null, it must be less than α.
Effect Size
When we reject the null hypothesis, we are stating that the difference we found was statistically significant, but we have mentioned several times that this tells us nothing about practical significance. To get an idea of the actual size of what we found, we can compute a new statistic called an effect size. Effect sizes give us an idea of how large, important, or meaningful a statistically significant effect is.
For mean differences like we calculated here, our effect size is Cohen’s d :
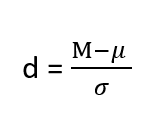
Effect sizes are incredibly useful and provide important information and clarification that overcomes some of the weakness of hypothesis testing. Whenever you find a significant result, you should always calculate an effect size
d | Interpretation |
---|---|
0.0 – 0.2 | negligible |
0.2 – 0.5 | small |
0.5 – 0.8 | medium |
0.8 – | large |
Table 1. Interpretation of Cohen’s d
Example: Office Temperature
Let’s do another example to solidify our understanding. Let’s say that the office building you work in is supposed to be kept at 74 degree Fahrenheit but is allowed
to vary by 1 degree in either direction. You suspect that, as a cost saving measure, the temperature was secretly set higher. You set up a formal way to test your hypothesis.
You start by laying out the null hypothesis:
H 0 : There is no difference in the average building temperature H 0 : μ = 74
Next you state the alternative hypothesis. You have reason to suspect a specific direction of change, so you make a one-tailed test:
H A : The average building temperature is higher than claimed H A : μ > 74
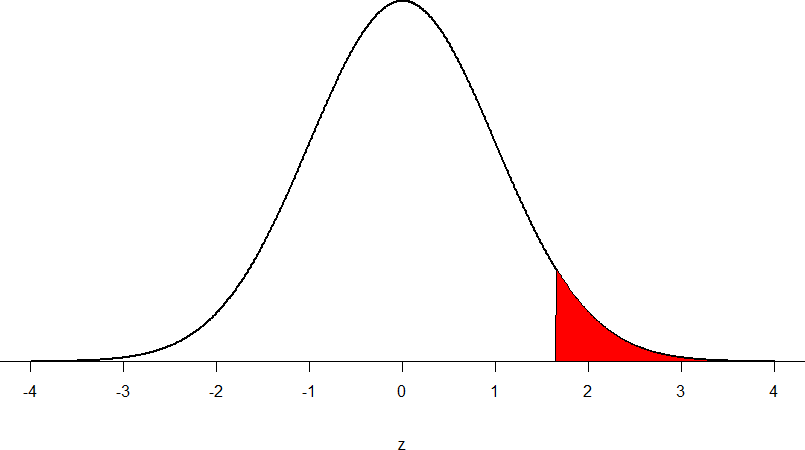
Now that you have everything set up, you spend one week collecting temperature data:
Day | Temp |
Monday | 77 |
Tuesday | 76 |
Wednesday | 74 |
Thursday | 78 |
Friday | 78 |
You calculate the average of these scores to be 𝑋̅ = 76.6 degrees. You use this to calculate the test statistic, using μ = 74 (the supposed average temperature), σ = 1.00 (how much the temperature should vary), and n = 5 (how many data points you collected):
z = 76.60 − 74.00 = 2.60 = 5.78
1.00/√5 0.45
This value falls so far into the tail that it cannot even be plotted on the distribution!
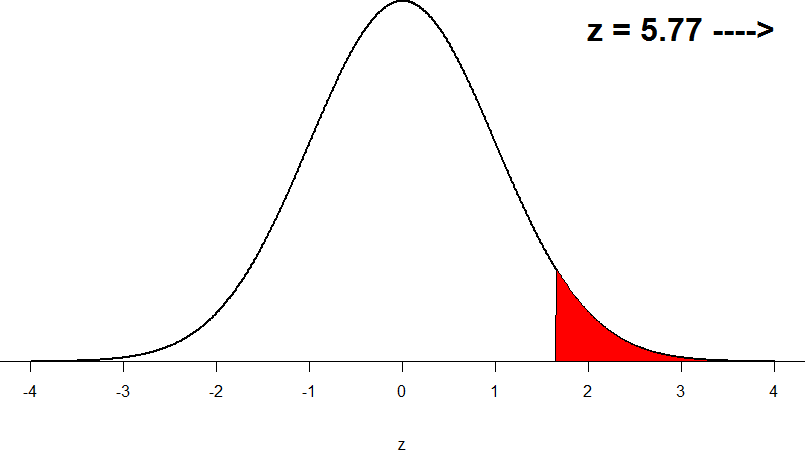
Figure 7: Obtained z-statistic
You compare your obtained z-statistic, z = 5.77, to the critical value, z* = 1.645, and find that z > z*. Therefore you reject the null hypothesis, concluding: Based on 5 observations, the average temperature (𝑋̅ = 76.6 degrees) is statistically significantly higher than it is supposed to be, z = 5.77, p < .05.
d = (76.60-74.00)/ 1= 2.60
The effect size you calculate is definitely large, meaning someone has some explaining to do!
Example: Different Significance Level
First, let’s take a look at an example phrased in generic terms, rather than in the context of a specific research question, to see the individual pieces one more time. This time, however, we will use a stricter significance level, α = 0.01, to test the hypothesis.
We will use 60 as an arbitrary null hypothesis value: H 0 : The average score does not differ from the population H 0 : μ = 50
We will assume a two-tailed test: H A : The average score does differ H A : μ ≠ 50
We have seen the critical values for z-tests at α = 0.05 levels of significance several times. To find the values for α = 0.01, we will go to the standard normal table and find the z-score cutting of 0.005 (0.01 divided by 2 for a two-tailed test) of the area in the tail, which is z crit * = ±2.575. Notice that this cutoff is much higher than it was for α = 0.05. This is because we need much less of the area in the tail, so we need to go very far out to find the cutoff. As a result, this will require a much larger effect or much larger sample size in order to reject the null hypothesis.
We can now calculate our test statistic. The average of 10 scores is M = 60.40 with a µ = 60. We will use σ = 10 as our known population standard deviation. From this information, we calculate our z-statistic as:
Our obtained z-statistic, z = 0.13, is very small. It is much less than our critical value of 2.575. Thus, this time, we fail to reject the null hypothesis. Our conclusion would look something like:
Notice two things about the end of the conclusion. First, we wrote that p is greater than instead of p is less than, like we did in the previous two examples. This is because we failed to reject the null hypothesis. We don’t know exactly what the p- value is, but we know it must be larger than the α level we used to test our hypothesis. Second, we used 0.01 instead of the usual 0.05, because this time we tested at a different level. The number you compare to the p-value should always be the significance level you test at. Because we did not detect a statistically significant effect, we do not need to calculate an effect size. Note: some statisticians will suggest to always calculate effects size as a possibility of Type II error. Although insignificant, calculating d = (60.4-60)/10 = .04 which suggests no effect (and not a possibility of Type II error).
Review Considerations in Hypothesis Testing
Errors in hypothesis testing.
Keep in mind that rejecting the null hypothesis is not an all-or-nothing decision. The Type I error rate is affected by the α level: the lower the α level the lower the Type I error rate. It might seem that α is the probability of a Type I error. However, this is not correct. Instead, α is the probability of a Type I error given that the null hypothesis is true. If the null hypothesis is false, then it is impossible to make a Type I error. The second type of error that can be made in significance testing is failing to reject a false null hypothesis. This kind of error is called a Type II error.
Statistical Power
The statistical power of a research design is the probability of rejecting the null hypothesis given the sample size and expected relationship strength. Statistical power is the complement of the probability of committing a Type II error. Clearly, researchers should be interested in the power of their research designs if they want to avoid making Type II errors. In particular, they should make sure their research design has adequate power before collecting data. A common guideline is that a power of .80 is adequate. This means that there is an 80% chance of rejecting the null hypothesis for the expected relationship strength.
Given that statistical power depends primarily on relationship strength and sample size, there are essentially two steps you can take to increase statistical power: increase the strength of the relationship or increase the sample size. Increasing the strength of the relationship can sometimes be accomplished by using a stronger manipulation or by more carefully controlling extraneous variables to reduce the amount of noise in the data (e.g., by using a within-subjects design rather than a between-subjects design). The usual strategy, however, is to increase the sample size. For any expected relationship strength, there will always be some sample large enough to achieve adequate power.
Inferential statistics uses data from a sample of individuals to reach conclusions about the whole population. The degree to which our inferences are valid depends upon how we selected the sample (sampling technique) and the characteristics (parameters) of population data. Statistical analyses assume that sample(s) and population(s) meet certain conditions called statistical assumptions.
It is easy to check assumptions when using statistical software and it is important as a researcher to check for violations; if violations of statistical assumptions are not appropriately addressed then results may be interpreted incorrectly.
Learning Objectives
Having read the chapter, students should be able to:
- Conduct a hypothesis test using a z-score statistics, locating critical region, and make a statistical decision including.
- Explain the purpose of measuring effect size and power, and be able to compute Cohen’s d.
Exercises – Ch. 10
- List the main steps for hypothesis testing with the z-statistic. When and why do you calculate an effect size?
- z = 1.99, two-tailed test at α = 0.05
- z = 1.99, two-tailed test at α = 0.01
- z = 1.99, one-tailed test at α = 0.05
- You are part of a trivia team and have tracked your team’s performance since you started playing, so you know that your scores are normally distributed with μ = 78 and σ = 12. Recently, a new person joined the team, and you think the scores have gotten better. Use hypothesis testing to see if the average score has improved based on the following 8 weeks’ worth of score data: 82, 74, 62, 68, 79, 94, 90, 81, 80.
- A study examines self-esteem and depression in teenagers. A sample of 25 teens with a low self-esteem are given the Beck Depression Inventory. The average score for the group is 20.9. For the general population, the average score is 18.3 with σ = 12. Use a two-tail test with α = 0.05 to examine whether teenagers with low self-esteem show significant differences in depression.
- You get hired as a server at a local restaurant, and the manager tells you that servers’ tips are $42 on average but vary about $12 (μ = 42, σ = 12). You decide to track your tips to see if you make a different amount, but because this is your first job as a server, you don’t know if you will make more or less in tips. After working 16 shifts, you find that your average nightly amount is $44.50 from tips. Test for a difference between this value and the population mean at the α = 0.05 level of significance.
Answers to Odd- Numbered Exercises – Ch. 10
1. List hypotheses. Determine critical region. Calculate z. Compare z to critical region. Draw Conclusion. We calculate an effect size when we find a statistically significant result to see if our result is practically meaningful or important
5. Step 1: H 0 : μ = 42 “My average tips does not differ from other servers”, H A : μ ≠ 42 “My average tips do differ from others”
Introduction to Statistics for Psychology Copyright © 2021 by Alisa Beyer is licensed under a Creative Commons Attribution-NonCommercial-ShareAlike 4.0 International License , except where otherwise noted.
Share This Book
Educational resources and simple solutions for your research journey
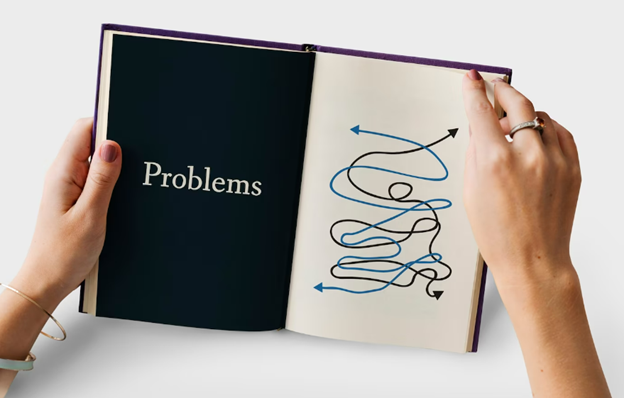
What is a Research Hypothesis: How to Write it, Types, and Examples
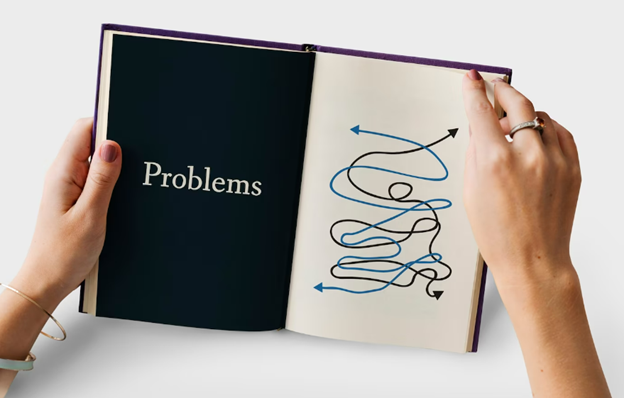
Any research begins with a research question and a research hypothesis . A research question alone may not suffice to design the experiment(s) needed to answer it. A hypothesis is central to the scientific method. But what is a hypothesis ? A hypothesis is a testable statement that proposes a possible explanation to a phenomenon, and it may include a prediction. Next, you may ask what is a research hypothesis ? Simply put, a research hypothesis is a prediction or educated guess about the relationship between the variables that you want to investigate.
It is important to be thorough when developing your research hypothesis. Shortcomings in the framing of a hypothesis can affect the study design and the results. A better understanding of the research hypothesis definition and characteristics of a good hypothesis will make it easier for you to develop your own hypothesis for your research. Let’s dive in to know more about the types of research hypothesis , how to write a research hypothesis , and some research hypothesis examples .
Table of Contents
What is a hypothesis ?
A hypothesis is based on the existing body of knowledge in a study area. Framed before the data are collected, a hypothesis states the tentative relationship between independent and dependent variables, along with a prediction of the outcome.
What is a research hypothesis ?
Young researchers starting out their journey are usually brimming with questions like “ What is a hypothesis ?” “ What is a research hypothesis ?” “How can I write a good research hypothesis ?”
A research hypothesis is a statement that proposes a possible explanation for an observable phenomenon or pattern. It guides the direction of a study and predicts the outcome of the investigation. A research hypothesis is testable, i.e., it can be supported or disproven through experimentation or observation.
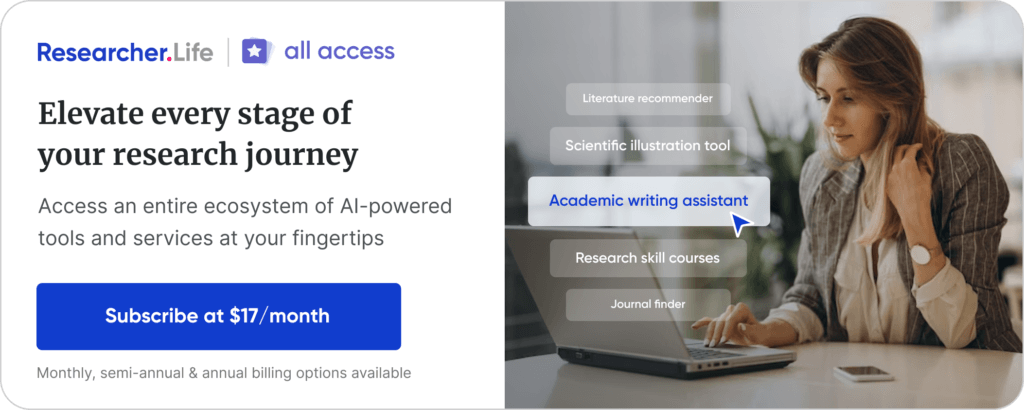
Characteristics of a good hypothesis
Here are the characteristics of a good hypothesis :
- Clearly formulated and free of language errors and ambiguity
- Concise and not unnecessarily verbose
- Has clearly defined variables
- Testable and stated in a way that allows for it to be disproven
- Can be tested using a research design that is feasible, ethical, and practical
- Specific and relevant to the research problem
- Rooted in a thorough literature search
- Can generate new knowledge or understanding.
How to create an effective research hypothesis
A study begins with the formulation of a research question. A researcher then performs background research. This background information forms the basis for building a good research hypothesis . The researcher then performs experiments, collects, and analyzes the data, interprets the findings, and ultimately, determines if the findings support or negate the original hypothesis.
Let’s look at each step for creating an effective, testable, and good research hypothesis :
- Identify a research problem or question: Start by identifying a specific research problem.
- Review the literature: Conduct an in-depth review of the existing literature related to the research problem to grasp the current knowledge and gaps in the field.
- Formulate a clear and testable hypothesis : Based on the research question, use existing knowledge to form a clear and testable hypothesis . The hypothesis should state a predicted relationship between two or more variables that can be measured and manipulated. Improve the original draft till it is clear and meaningful.
- State the null hypothesis: The null hypothesis is a statement that there is no relationship between the variables you are studying.
- Define the population and sample: Clearly define the population you are studying and the sample you will be using for your research.
- Select appropriate methods for testing the hypothesis: Select appropriate research methods, such as experiments, surveys, or observational studies, which will allow you to test your research hypothesis .
Remember that creating a research hypothesis is an iterative process, i.e., you might have to revise it based on the data you collect. You may need to test and reject several hypotheses before answering the research problem.
How to write a research hypothesis
When you start writing a research hypothesis , you use an “if–then” statement format, which states the predicted relationship between two or more variables. Clearly identify the independent variables (the variables being changed) and the dependent variables (the variables being measured), as well as the population you are studying. Review and revise your hypothesis as needed.
An example of a research hypothesis in this format is as follows:
“ If [athletes] follow [cold water showers daily], then their [endurance] increases.”
Population: athletes
Independent variable: daily cold water showers
Dependent variable: endurance
You may have understood the characteristics of a good hypothesis . But note that a research hypothesis is not always confirmed; a researcher should be prepared to accept or reject the hypothesis based on the study findings.
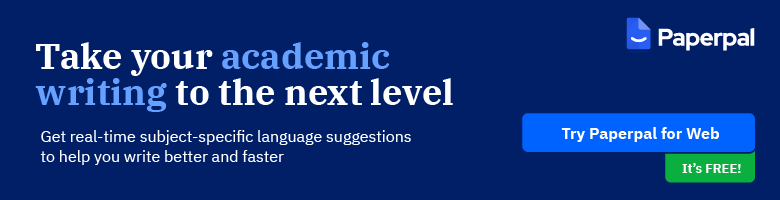
Research hypothesis checklist
Following from above, here is a 10-point checklist for a good research hypothesis :
- Testable: A research hypothesis should be able to be tested via experimentation or observation.
- Specific: A research hypothesis should clearly state the relationship between the variables being studied.
- Based on prior research: A research hypothesis should be based on existing knowledge and previous research in the field.
- Falsifiable: A research hypothesis should be able to be disproven through testing.
- Clear and concise: A research hypothesis should be stated in a clear and concise manner.
- Logical: A research hypothesis should be logical and consistent with current understanding of the subject.
- Relevant: A research hypothesis should be relevant to the research question and objectives.
- Feasible: A research hypothesis should be feasible to test within the scope of the study.
- Reflects the population: A research hypothesis should consider the population or sample being studied.
- Uncomplicated: A good research hypothesis is written in a way that is easy for the target audience to understand.
By following this research hypothesis checklist , you will be able to create a research hypothesis that is strong, well-constructed, and more likely to yield meaningful results.
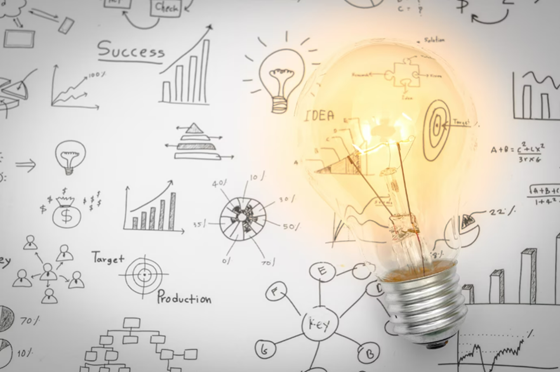
Types of research hypothesis
Different types of research hypothesis are used in scientific research:
1. Null hypothesis:
A null hypothesis states that there is no change in the dependent variable due to changes to the independent variable. This means that the results are due to chance and are not significant. A null hypothesis is denoted as H0 and is stated as the opposite of what the alternative hypothesis states.
Example: “ The newly identified virus is not zoonotic .”
2. Alternative hypothesis:
This states that there is a significant difference or relationship between the variables being studied. It is denoted as H1 or Ha and is usually accepted or rejected in favor of the null hypothesis.
Example: “ The newly identified virus is zoonotic .”
3. Directional hypothesis :
This specifies the direction of the relationship or difference between variables; therefore, it tends to use terms like increase, decrease, positive, negative, more, or less.
Example: “ The inclusion of intervention X decreases infant mortality compared to the original treatment .”
4. Non-directional hypothesis:
While it does not predict the exact direction or nature of the relationship between the two variables, a non-directional hypothesis states the existence of a relationship or difference between variables but not the direction, nature, or magnitude of the relationship. A non-directional hypothesis may be used when there is no underlying theory or when findings contradict previous research.
Example, “ Cats and dogs differ in the amount of affection they express .”
5. Simple hypothesis :
A simple hypothesis only predicts the relationship between one independent and another independent variable.
Example: “ Applying sunscreen every day slows skin aging .”
6 . Complex hypothesis :
A complex hypothesis states the relationship or difference between two or more independent and dependent variables.
Example: “ Applying sunscreen every day slows skin aging, reduces sun burn, and reduces the chances of skin cancer .” (Here, the three dependent variables are slowing skin aging, reducing sun burn, and reducing the chances of skin cancer.)
7. Associative hypothesis:
An associative hypothesis states that a change in one variable results in the change of the other variable. The associative hypothesis defines interdependency between variables.
Example: “ There is a positive association between physical activity levels and overall health .”
8 . Causal hypothesis:
A causal hypothesis proposes a cause-and-effect interaction between variables.
Example: “ Long-term alcohol use causes liver damage .”
Note that some of the types of research hypothesis mentioned above might overlap. The types of hypothesis chosen will depend on the research question and the objective of the study.
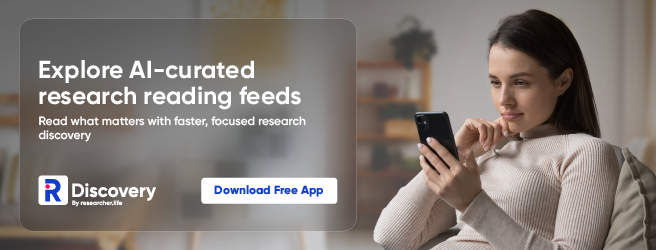
Research hypothesis examples
Here are some good research hypothesis examples :
“The use of a specific type of therapy will lead to a reduction in symptoms of depression in individuals with a history of major depressive disorder.”
“Providing educational interventions on healthy eating habits will result in weight loss in overweight individuals.”
“Plants that are exposed to certain types of music will grow taller than those that are not exposed to music.”
“The use of the plant growth regulator X will lead to an increase in the number of flowers produced by plants.”
Characteristics that make a research hypothesis weak are unclear variables, unoriginality, being too general or too vague, and being untestable. A weak hypothesis leads to weak research and improper methods.
Some bad research hypothesis examples (and the reasons why they are “bad”) are as follows:
“This study will show that treatment X is better than any other treatment . ” (This statement is not testable, too broad, and does not consider other treatments that may be effective.)
“This study will prove that this type of therapy is effective for all mental disorders . ” (This statement is too broad and not testable as mental disorders are complex and different disorders may respond differently to different types of therapy.)
“Plants can communicate with each other through telepathy . ” (This statement is not testable and lacks a scientific basis.)
Importance of testable hypothesis
If a research hypothesis is not testable, the results will not prove or disprove anything meaningful. The conclusions will be vague at best. A testable hypothesis helps a researcher focus on the study outcome and understand the implication of the question and the different variables involved. A testable hypothesis helps a researcher make precise predictions based on prior research.
To be considered testable, there must be a way to prove that the hypothesis is true or false; further, the results of the hypothesis must be reproducible.
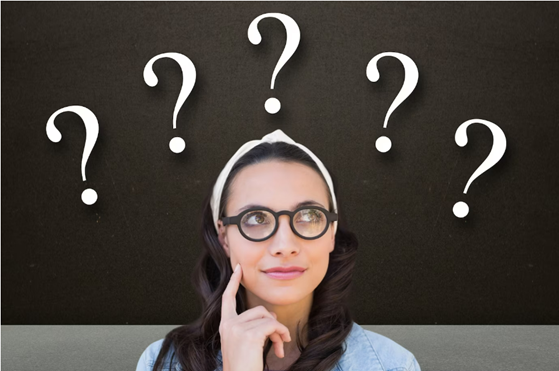
Frequently Asked Questions (FAQs) on research hypothesis
1. What is the difference between research question and research hypothesis ?
A research question defines the problem and helps outline the study objective(s). It is an open-ended statement that is exploratory or probing in nature. Therefore, it does not make predictions or assumptions. It helps a researcher identify what information to collect. A research hypothesis , however, is a specific, testable prediction about the relationship between variables. Accordingly, it guides the study design and data analysis approach.
2. When to reject null hypothesis ?
A null hypothesis should be rejected when the evidence from a statistical test shows that it is unlikely to be true. This happens when the test statistic (e.g., p -value) is less than the defined significance level (e.g., 0.05). Rejecting the null hypothesis does not necessarily mean that the alternative hypothesis is true; it simply means that the evidence found is not compatible with the null hypothesis.
3. How can I be sure my hypothesis is testable?
A testable hypothesis should be specific and measurable, and it should state a clear relationship between variables that can be tested with data. To ensure that your hypothesis is testable, consider the following:
- Clearly define the key variables in your hypothesis. You should be able to measure and manipulate these variables in a way that allows you to test the hypothesis.
- The hypothesis should predict a specific outcome or relationship between variables that can be measured or quantified.
- You should be able to collect the necessary data within the constraints of your study.
- It should be possible for other researchers to replicate your study, using the same methods and variables.
- Your hypothesis should be testable by using appropriate statistical analysis techniques, so you can draw conclusions, and make inferences about the population from the sample data.
- The hypothesis should be able to be disproven or rejected through the collection of data.
4. How do I revise my research hypothesis if my data does not support it?
If your data does not support your research hypothesis , you will need to revise it or develop a new one. You should examine your data carefully and identify any patterns or anomalies, re-examine your research question, and/or revisit your theory to look for any alternative explanations for your results. Based on your review of the data, literature, and theories, modify your research hypothesis to better align it with the results you obtained. Use your revised hypothesis to guide your research design and data collection. It is important to remain objective throughout the process.
5. I am performing exploratory research. Do I need to formulate a research hypothesis?
As opposed to “confirmatory” research, where a researcher has some idea about the relationship between the variables under investigation, exploratory research (or hypothesis-generating research) looks into a completely new topic about which limited information is available. Therefore, the researcher will not have any prior hypotheses. In such cases, a researcher will need to develop a post-hoc hypothesis. A post-hoc research hypothesis is generated after these results are known.
6. How is a research hypothesis different from a research question?
A research question is an inquiry about a specific topic or phenomenon, typically expressed as a question. It seeks to explore and understand a particular aspect of the research subject. In contrast, a research hypothesis is a specific statement or prediction that suggests an expected relationship between variables. It is formulated based on existing knowledge or theories and guides the research design and data analysis.
7. Can a research hypothesis change during the research process?
Yes, research hypotheses can change during the research process. As researchers collect and analyze data, new insights and information may emerge that require modification or refinement of the initial hypotheses. This can be due to unexpected findings, limitations in the original hypotheses, or the need to explore additional dimensions of the research topic. Flexibility is crucial in research, allowing for adaptation and adjustment of hypotheses to align with the evolving understanding of the subject matter.
8. How many hypotheses should be included in a research study?
The number of research hypotheses in a research study varies depending on the nature and scope of the research. It is not necessary to have multiple hypotheses in every study. Some studies may have only one primary hypothesis, while others may have several related hypotheses. The number of hypotheses should be determined based on the research objectives, research questions, and the complexity of the research topic. It is important to ensure that the hypotheses are focused, testable, and directly related to the research aims.
9. Can research hypotheses be used in qualitative research?
Yes, research hypotheses can be used in qualitative research, although they are more commonly associated with quantitative research. In qualitative research, hypotheses may be formulated as tentative or exploratory statements that guide the investigation. Instead of testing hypotheses through statistical analysis, qualitative researchers may use the hypotheses to guide data collection and analysis, seeking to uncover patterns, themes, or relationships within the qualitative data. The emphasis in qualitative research is often on generating insights and understanding rather than confirming or rejecting specific research hypotheses through statistical testing.
Researcher.Life is a subscription-based platform that unifies the best AI tools and services designed to speed up, simplify, and streamline every step of a researcher’s journey. The Researcher.Life All Access Pack is a one-of-a-kind subscription that unlocks full access to an AI writing assistant, literature recommender, journal finder, scientific illustration tool, and exclusive discounts on professional publication services from Editage.
Based on 21+ years of experience in academia, Researcher.Life All Access empowers researchers to put their best research forward and move closer to success. Explore our top AI Tools pack, AI Tools + Publication Services pack, or Build Your Own Plan. Find everything a researcher needs to succeed, all in one place – Get All Access now starting at just $17 a month !
Related Posts

What is a Case Study in Research? Definition, Methods, and Examples

Take Top AI Tools for Researchers for a Spin with the Editage All Access 7-Day Pass!
- Privacy Policy

Home » What is a Hypothesis – Types, Examples and Writing Guide
What is a Hypothesis – Types, Examples and Writing Guide
Table of Contents

Definition:
Hypothesis is an educated guess or proposed explanation for a phenomenon, based on some initial observations or data. It is a tentative statement that can be tested and potentially proven or disproven through further investigation and experimentation.
Hypothesis is often used in scientific research to guide the design of experiments and the collection and analysis of data. It is an essential element of the scientific method, as it allows researchers to make predictions about the outcome of their experiments and to test those predictions to determine their accuracy.
Types of Hypothesis
Types of Hypothesis are as follows:
Research Hypothesis
A research hypothesis is a statement that predicts a relationship between variables. It is usually formulated as a specific statement that can be tested through research, and it is often used in scientific research to guide the design of experiments.
Null Hypothesis
The null hypothesis is a statement that assumes there is no significant difference or relationship between variables. It is often used as a starting point for testing the research hypothesis, and if the results of the study reject the null hypothesis, it suggests that there is a significant difference or relationship between variables.
Alternative Hypothesis
An alternative hypothesis is a statement that assumes there is a significant difference or relationship between variables. It is often used as an alternative to the null hypothesis and is tested against the null hypothesis to determine which statement is more accurate.
Directional Hypothesis
A directional hypothesis is a statement that predicts the direction of the relationship between variables. For example, a researcher might predict that increasing the amount of exercise will result in a decrease in body weight.
Non-directional Hypothesis
A non-directional hypothesis is a statement that predicts the relationship between variables but does not specify the direction. For example, a researcher might predict that there is a relationship between the amount of exercise and body weight, but they do not specify whether increasing or decreasing exercise will affect body weight.
Statistical Hypothesis
A statistical hypothesis is a statement that assumes a particular statistical model or distribution for the data. It is often used in statistical analysis to test the significance of a particular result.
Composite Hypothesis
A composite hypothesis is a statement that assumes more than one condition or outcome. It can be divided into several sub-hypotheses, each of which represents a different possible outcome.
Empirical Hypothesis
An empirical hypothesis is a statement that is based on observed phenomena or data. It is often used in scientific research to develop theories or models that explain the observed phenomena.
Simple Hypothesis
A simple hypothesis is a statement that assumes only one outcome or condition. It is often used in scientific research to test a single variable or factor.
Complex Hypothesis
A complex hypothesis is a statement that assumes multiple outcomes or conditions. It is often used in scientific research to test the effects of multiple variables or factors on a particular outcome.
Applications of Hypothesis
Hypotheses are used in various fields to guide research and make predictions about the outcomes of experiments or observations. Here are some examples of how hypotheses are applied in different fields:
- Science : In scientific research, hypotheses are used to test the validity of theories and models that explain natural phenomena. For example, a hypothesis might be formulated to test the effects of a particular variable on a natural system, such as the effects of climate change on an ecosystem.
- Medicine : In medical research, hypotheses are used to test the effectiveness of treatments and therapies for specific conditions. For example, a hypothesis might be formulated to test the effects of a new drug on a particular disease.
- Psychology : In psychology, hypotheses are used to test theories and models of human behavior and cognition. For example, a hypothesis might be formulated to test the effects of a particular stimulus on the brain or behavior.
- Sociology : In sociology, hypotheses are used to test theories and models of social phenomena, such as the effects of social structures or institutions on human behavior. For example, a hypothesis might be formulated to test the effects of income inequality on crime rates.
- Business : In business research, hypotheses are used to test the validity of theories and models that explain business phenomena, such as consumer behavior or market trends. For example, a hypothesis might be formulated to test the effects of a new marketing campaign on consumer buying behavior.
- Engineering : In engineering, hypotheses are used to test the effectiveness of new technologies or designs. For example, a hypothesis might be formulated to test the efficiency of a new solar panel design.
How to write a Hypothesis
Here are the steps to follow when writing a hypothesis:
Identify the Research Question
The first step is to identify the research question that you want to answer through your study. This question should be clear, specific, and focused. It should be something that can be investigated empirically and that has some relevance or significance in the field.
Conduct a Literature Review
Before writing your hypothesis, it’s essential to conduct a thorough literature review to understand what is already known about the topic. This will help you to identify the research gap and formulate a hypothesis that builds on existing knowledge.
Determine the Variables
The next step is to identify the variables involved in the research question. A variable is any characteristic or factor that can vary or change. There are two types of variables: independent and dependent. The independent variable is the one that is manipulated or changed by the researcher, while the dependent variable is the one that is measured or observed as a result of the independent variable.
Formulate the Hypothesis
Based on the research question and the variables involved, you can now formulate your hypothesis. A hypothesis should be a clear and concise statement that predicts the relationship between the variables. It should be testable through empirical research and based on existing theory or evidence.
Write the Null Hypothesis
The null hypothesis is the opposite of the alternative hypothesis, which is the hypothesis that you are testing. The null hypothesis states that there is no significant difference or relationship between the variables. It is important to write the null hypothesis because it allows you to compare your results with what would be expected by chance.
Refine the Hypothesis
After formulating the hypothesis, it’s important to refine it and make it more precise. This may involve clarifying the variables, specifying the direction of the relationship, or making the hypothesis more testable.
Examples of Hypothesis
Here are a few examples of hypotheses in different fields:
- Psychology : “Increased exposure to violent video games leads to increased aggressive behavior in adolescents.”
- Biology : “Higher levels of carbon dioxide in the atmosphere will lead to increased plant growth.”
- Sociology : “Individuals who grow up in households with higher socioeconomic status will have higher levels of education and income as adults.”
- Education : “Implementing a new teaching method will result in higher student achievement scores.”
- Marketing : “Customers who receive a personalized email will be more likely to make a purchase than those who receive a generic email.”
- Physics : “An increase in temperature will cause an increase in the volume of a gas, assuming all other variables remain constant.”
- Medicine : “Consuming a diet high in saturated fats will increase the risk of developing heart disease.”
Purpose of Hypothesis
The purpose of a hypothesis is to provide a testable explanation for an observed phenomenon or a prediction of a future outcome based on existing knowledge or theories. A hypothesis is an essential part of the scientific method and helps to guide the research process by providing a clear focus for investigation. It enables scientists to design experiments or studies to gather evidence and data that can support or refute the proposed explanation or prediction.
The formulation of a hypothesis is based on existing knowledge, observations, and theories, and it should be specific, testable, and falsifiable. A specific hypothesis helps to define the research question, which is important in the research process as it guides the selection of an appropriate research design and methodology. Testability of the hypothesis means that it can be proven or disproven through empirical data collection and analysis. Falsifiability means that the hypothesis should be formulated in such a way that it can be proven wrong if it is incorrect.
In addition to guiding the research process, the testing of hypotheses can lead to new discoveries and advancements in scientific knowledge. When a hypothesis is supported by the data, it can be used to develop new theories or models to explain the observed phenomenon. When a hypothesis is not supported by the data, it can help to refine existing theories or prompt the development of new hypotheses to explain the phenomenon.
When to use Hypothesis
Here are some common situations in which hypotheses are used:
- In scientific research , hypotheses are used to guide the design of experiments and to help researchers make predictions about the outcomes of those experiments.
- In social science research , hypotheses are used to test theories about human behavior, social relationships, and other phenomena.
- I n business , hypotheses can be used to guide decisions about marketing, product development, and other areas. For example, a hypothesis might be that a new product will sell well in a particular market, and this hypothesis can be tested through market research.
Characteristics of Hypothesis
Here are some common characteristics of a hypothesis:
- Testable : A hypothesis must be able to be tested through observation or experimentation. This means that it must be possible to collect data that will either support or refute the hypothesis.
- Falsifiable : A hypothesis must be able to be proven false if it is not supported by the data. If a hypothesis cannot be falsified, then it is not a scientific hypothesis.
- Clear and concise : A hypothesis should be stated in a clear and concise manner so that it can be easily understood and tested.
- Based on existing knowledge : A hypothesis should be based on existing knowledge and research in the field. It should not be based on personal beliefs or opinions.
- Specific : A hypothesis should be specific in terms of the variables being tested and the predicted outcome. This will help to ensure that the research is focused and well-designed.
- Tentative: A hypothesis is a tentative statement or assumption that requires further testing and evidence to be confirmed or refuted. It is not a final conclusion or assertion.
- Relevant : A hypothesis should be relevant to the research question or problem being studied. It should address a gap in knowledge or provide a new perspective on the issue.
Advantages of Hypothesis
Hypotheses have several advantages in scientific research and experimentation:
- Guides research: A hypothesis provides a clear and specific direction for research. It helps to focus the research question, select appropriate methods and variables, and interpret the results.
- Predictive powe r: A hypothesis makes predictions about the outcome of research, which can be tested through experimentation. This allows researchers to evaluate the validity of the hypothesis and make new discoveries.
- Facilitates communication: A hypothesis provides a common language and framework for scientists to communicate with one another about their research. This helps to facilitate the exchange of ideas and promotes collaboration.
- Efficient use of resources: A hypothesis helps researchers to use their time, resources, and funding efficiently by directing them towards specific research questions and methods that are most likely to yield results.
- Provides a basis for further research: A hypothesis that is supported by data provides a basis for further research and exploration. It can lead to new hypotheses, theories, and discoveries.
- Increases objectivity: A hypothesis can help to increase objectivity in research by providing a clear and specific framework for testing and interpreting results. This can reduce bias and increase the reliability of research findings.
Limitations of Hypothesis
Some Limitations of the Hypothesis are as follows:
- Limited to observable phenomena: Hypotheses are limited to observable phenomena and cannot account for unobservable or intangible factors. This means that some research questions may not be amenable to hypothesis testing.
- May be inaccurate or incomplete: Hypotheses are based on existing knowledge and research, which may be incomplete or inaccurate. This can lead to flawed hypotheses and erroneous conclusions.
- May be biased: Hypotheses may be biased by the researcher’s own beliefs, values, or assumptions. This can lead to selective interpretation of data and a lack of objectivity in research.
- Cannot prove causation: A hypothesis can only show a correlation between variables, but it cannot prove causation. This requires further experimentation and analysis.
- Limited to specific contexts: Hypotheses are limited to specific contexts and may not be generalizable to other situations or populations. This means that results may not be applicable in other contexts or may require further testing.
- May be affected by chance : Hypotheses may be affected by chance or random variation, which can obscure or distort the true relationship between variables.
About the author
Muhammad Hassan
Researcher, Academic Writer, Web developer
You may also like

Research Methods – Types, Examples and Guide

Data Verification – Process, Types and Examples

Dissertation – Format, Example and Template

Chapter Summary & Overview – Writing Guide...

Research Report – Example, Writing Guide and...

Research Objectives – Types, Examples and...

Non Directional Hypothesis
Ai generator.
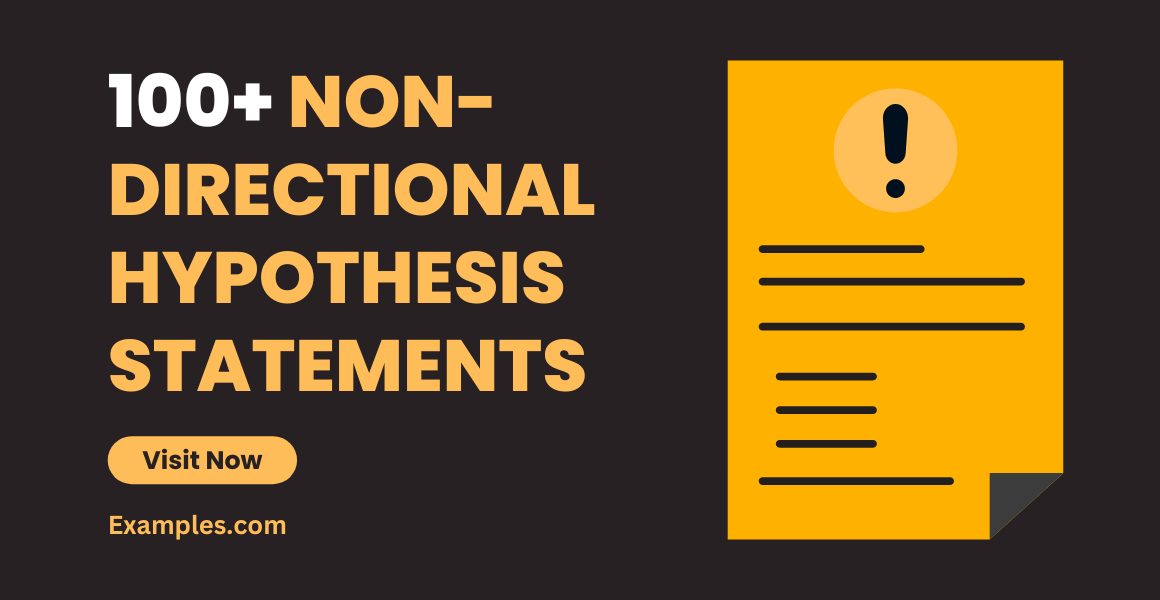
In the realm of hypothesis formulation, non-directional hypotheses offer a distinct perspective. These hypotheses suggest a relationship between variables without specifying the nature or direction of that relationship. This guide delves into non-directional hypothesis examples across various fields, outlines a step-by-step approach to crafting them, and provides expert tips to ensure your non-directional hypotheses are robust and insightful. Explore the world of Thesis statement hypotheses that explore connections without predetermined expectations.
What is the Non-Directional Hypothesis? – Definition
A non-directional hypothesis, also known as a two tailed hypothesis , is a type of hypothesis that predicts a relationship between variables without specifying the direction of that relationship. Unlike directional hypotheses that predict a specific outcome, non-directional hypotheses simply suggest that a relationship exists without indicating whether one variable will increase or decrease in response to changes in the other variable.
What is an Example of a Non-Directional Hypothesis Statement?
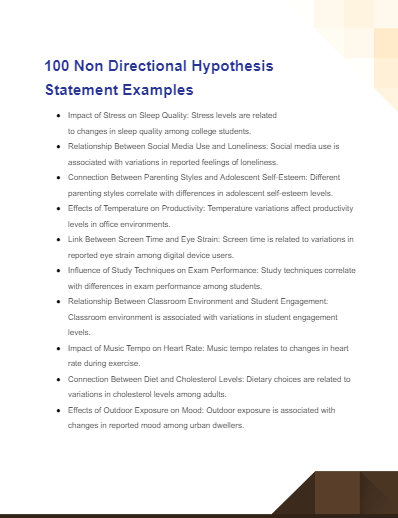
Size: 219 KB
“An increase in exercise frequency is associated with changes in weight.”
In this non-directional hypothesis, the statement suggests that a relationship exists between exercise frequency and weight changes but doesn’t specify whether increased exercise will lead to weight loss or weight gain. It leaves the direction of the relationship open for empirical investigation and data analysis.
100 Non Directional Hypothesis Statement Examples
Non-directional hypotheses explore relationships between variables without predicting the specific outcome. These simple hypothesis offer flexibility, allowing researchers to uncover unforeseen connections. Discover a range of non-directional hypothesis examples that span disciplines, enabling empirical exploration and evidence-based conclusions.
- Impact of Stress on Sleep Quality : Stress levels are related to changes in sleep quality among college students.
- Relationship Between Social Media Use and Loneliness : Social media use is associated with variations in reported feelings of loneliness.
- Connection Between Parenting Styles and Adolescent Self-Esteem : Different parenting styles correlate with differences in adolescent self-esteem levels.
- Effects of Temperature on Productivity : Temperature variations affect productivity levels in office environments.
- Link Between Screen Time and Eye Strain : Screen time is related to variations in reported eye strain among digital device users.
- Influence of Study Techniques on Exam Performance : Study techniques correlate with differences in exam performance among students.
- Relationship Between Classroom Environment and Student Engagement : Classroom environment is associated with variations in student engagement levels.
- Impact of Music Tempo on Heart Rate : Music tempo relates to changes in heart rate during exercise.
- Connection Between Diet and Cholesterol Levels : Dietary choices are related to variations in cholesterol levels among adults.
- Effects of Outdoor Exposure on Mood : Outdoor exposure is associated with changes in reported mood among urban dwellers.
- Relationship Between Personality Traits and Leadership Styles : Personality traits are associated with differences in preferred leadership styles among professionals.
- Impact of Time Management Strategies on Academic Performance : Time management strategies correlate with variations in academic performance among college students.
- Connection Between Cultural Exposure and Empathy Levels : Cultural exposure relates to changes in reported empathy levels among individuals.
- Effects of Nutrition Education on Dietary Choices : Nutrition education is associated with variations in dietary choices among adolescents.
- Link Between Social Support and Stress Levels : Social support is related to differences in reported stress levels among working adults.
- Influence of Exercise Intensity on Mood : Exercise intensity correlates with variations in reported mood among fitness enthusiasts.
- Relationship Between Parental Involvement and Academic Achievement : Parental involvement is associated with differences in academic achievement among schoolchildren.
- Impact of Sleep Duration on Cognitive Function : Sleep duration is related to changes in cognitive function among older adults.
- Connection Between Environmental Factors and Creativity : Environmental factors correlate with variations in reported creative thinking abilities among artists.
- Effects of Communication Styles on Conflict Resolution : Communication styles are associated with differences in conflict resolution outcomes among couples.
- Relationship Between Social Interaction and Life Satisfaction : Social interaction is related to variations in reported life satisfaction among elderly individuals.
- Impact of Classroom Seating Arrangements on Participation : Classroom seating arrangements correlate with differences in student participation levels.
- Connection Between Smartphone Use and Sleep Quality : Smartphone use is associated with changes in reported sleep quality among young adults.
- Effects of Mindfulness Practices on Stress Reduction : Mindfulness practices relate to variations in reported stress levels among participants.
- Link Between Gender and Communication Styles : Gender is related to differences in communication styles among individuals in group discussions.
- Influence of Advertising Exposure on Purchase Decisions : Advertising exposure correlates with variations in reported purchase decisions among consumers.
- Relationship Between Job Satisfaction and Employee Productivity : Job satisfaction is associated with differences in employee productivity levels.
- Impact of Social Support on Coping Mechanisms : Social support relates to variations in reported coping mechanisms among individuals facing challenges.
- Connection Between Classroom Environment and Student Creativity : Classroom environment is related to changes in student creativity levels.
- Effects of Exercise on Mood : Exercise is associated with variations in reported mood levels among participants.
- Relationship Between Music Preferences and Stress Levels : Music preferences are related to variations in reported stress levels among individuals.
- Impact of Nutrition Education on Food Choices : Nutrition education correlates with differences in dietary food choices among adolescents.
- Connection Between Physical Activity and Cognitive Function : Physical activity is associated with changes in cognitive function among older adults.
- Effects of Color Exposure on Mood : Color exposure relates to variations in reported mood levels among participants.
- Link Between Personality Traits and Career Choice : Personality traits are related to differences in career choices among individuals.
- Influence of Outdoor Recreation on Mental Well-being : Outdoor recreation is associated with variations in reported mental well-being among participants.
- Relationship Between Social Media Use and Self-Esteem : Social media use correlates with changes in reported self-esteem levels among young adults.
- Impact of Parenting Styles on Adolescent Risk Behavior : Parenting styles are related to variations in reported risk behaviors among adolescents.
- Connection Between Sleep Quality and Cognitive Performance : Sleep quality relates to changes in cognitive performance among students.
- Effects of Art Exposure on Creativity : Art exposure is associated with differences in reported creative thinking abilities among participants.
- Relationship Between Social Support and Mental Health : Social support is related to variations in reported mental health outcomes among individuals.
- Impact of Technology Use on Interpersonal Communication : Technology use correlates with differences in reported interpersonal communication skills among individuals.
- Connection Between Parental Attachment and Romantic Relationships : Parental attachment is associated with variations in the quality of romantic relationships among adults.
- Effects of Environmental Noise on Concentration : Environmental noise relates to changes in reported concentration levels among students.
- Link Between Music Exposure and Memory Performance : Music exposure is related to differences in memory performance among participants.
- Influence of Nutrition on Physical Fitness : Nutrition choices correlate with variations in reported physical fitness levels among athletes.
- Relationship Between Stress and Health Outcomes : Stress levels are associated with changes in reported health outcomes among individuals.
- Impact of Workplace Environment on Job Satisfaction : Workplace environment relates to differences in reported job satisfaction among employees.
- Connection Between Humor and Stress Reduction : Humor is related to variations in reported stress reduction among participants.
- Effects of Social Interaction on Emotional Well-being : Social interaction correlates with changes in reported emotional well-being among participants.
- Relationship Between Cultural Exposure and Cognitive Flexibility : Cultural exposure is related to variations in reported cognitive flexibility among individuals.
- Impact of Parent-Child Communication on Academic Achievement : Parent-child communication correlates with differences in academic achievement levels among students.
- Connection Between Personality Traits and Prosocial Behavior : Personality traits are associated with variations in reported prosocial behaviors among individuals.
- Effects of Nature Exposure on Stress Reduction : Nature exposure relates to changes in reported stress reduction among participants.
- Link Between Sleep Duration and Cognitive Performance : Sleep duration is related to differences in cognitive performance among participants.
- Influence of Social Media Use on Body Image : Social media use correlates with variations in reported body image satisfaction among young adults.
- Relationship Between Exercise and Mental Well-being : Exercise levels are associated with changes in reported mental well-being among participants.
- Impact of Cultural Competency Training on Patient Care : Cultural competency training relates to differences in patient care outcomes among healthcare professionals.
- Connection Between Perceived Social Support and Resilience : Perceived social support is related to variations in reported resilience levels among individuals.
- Effects of Environmental Factors on Mood : Environmental factors correlate with changes in reported mood levels among participants.
- Relationship Between Cultural Diversity and Team Performance : Cultural diversity is related to variations in reported team performance outcomes among professionals.
- Impact of Parental Involvement on Academic Motivation : Parental involvement correlates with differences in academic motivation levels among schoolchildren.
- Connection Between Mindfulness Practices and Anxiety Reduction : Mindfulness practices are associated with changes in reported anxiety levels among participants.
- Effects of Nutrition Education on Eating Habits : Nutrition education relates to variations in dietary eating habits among adolescents.
- Link Between Personality Traits and Learning Styles : Personality traits are related to differences in preferred learning styles among students.
- Influence of Nature Exposure on Creativity : Nature exposure correlates with variations in reported creative thinking abilities among individuals.
- Relationship Between Extracurricular Activities and Social Skills : Extracurricular activities are associated with changes in reported social skills among adolescents.
- Impact of Cultural Awareness Training on Stereotypes : Cultural awareness training relates to differences in perceived stereotypes among participants.
- Connection Between Sleep Quality and Emotional Regulation : Sleep quality is related to variations in reported emotional regulation skills among individuals.
- Effects of Music Exposure on Mood : Music exposure correlates with changes in reported mood levels among participants.
- Relationship Between Cultural Sensitivity and Cross-Cultural Communication : Cultural sensitivity is related to variations in reported cross-cultural communication skills among professionals.
- Impact of Parent-Child Bonding on Emotional Well-being : Parent-child bonding correlates with differences in reported emotional well-being levels among individuals.
- Connection Between Personality Traits and Conflict Resolution Styles : Personality traits are associated with variations in preferred conflict resolution styles among individuals.
- Effects of Mindfulness Practices on Focus and Concentration : Mindfulness practices relate to changes in reported focus and concentration levels among participants.
- Link Between Gender Identity and Career Aspirations : Gender identity is related to differences in reported career aspirations among individuals.
- Influence of Art Exposure on Emotional Expression : Art exposure correlates with variations in reported emotional expression abilities among participants.
- Relationship Between Peer Influence and Risky Behavior : Peer influence is associated with changes in reported engagement in risky behaviors among adolescents.
- Impact of Diversity Training on Workplace Harmony : Diversity training relates to differences in perceived workplace harmony among employees.
- Connection Between Sleep Patterns and Cognitive Performance : Sleep patterns are related to variations in cognitive performance among students.
- Effects of Exercise on Self-Esteem : Exercise correlates with changes in reported self-esteem levels among participants.
- Relationship Between Social Interaction and Well-being : Social interaction is related to variations in reported well-being levels among individuals.
- Impact of Parenting Styles on Adolescent Peer Relationships : Parenting styles correlate with differences in peer relationship quality among adolescents.
- Connection Between Personality Traits and Communication Effectiveness : Personality traits are associated with variations in communication effectiveness among professionals.
- Effects of Outdoor Activities on Stress Reduction : Outdoor activities relate to changes in reported stress reduction among participants.
- Link Between Music Exposure and Emotional Regulation : Music exposure is related to differences in reported emotional regulation skills among individuals.
- Influence of Family Dynamics on Academic Achievement : Family dynamics correlate with variations in academic achievement levels among students.
- Relationship Between Cultural Engagement and Empathy : Cultural engagement is associated with changes in reported empathy levels among individuals.
- Impact of Conflict Resolution Strategies on Relationship Satisfaction : Conflict resolution strategies relate to differences in reported relationship satisfaction levels among couples.
- Connection Between Sleep Quality and Physical Health : Sleep quality is related to variations in reported physical health outcomes among individuals.
- Effects of Social Support on Coping with Stress : Social support correlates with changes in reported coping strategies for stress among participants.
- Relationship Between Cultural Sensitivity and Patient Care : Cultural sensitivity is related to variations in reported patient care outcomes among healthcare professionals.
- Impact of Family Communication on Adolescent Well-being : Family communication correlates with differences in reported well-being levels among adolescents.
- Connection Between Personality Traits and Leadership Styles : Personality traits are associated with variations in preferred leadership styles among professionals.
- Effects of Nature Exposure on Attention Span : Nature exposure relates to changes in reported attention span among participants.
- Link Between Music Preference and Emotional Expression : Music preference is related to differences in reported emotional expression abilities among individuals.
- Influence of Peer Support on Academic Success : Peer support correlates with variations in reported academic success levels among students.
- Relationship Between Cultural Engagement and Creativity : Cultural engagement is associated with changes in reported creative thinking abilities among individuals.
- Impact of Conflict Resolution Skills on Relationship Satisfaction : Conflict resolution skills relate to differences in reported relationship satisfaction levels among couples.
- Connection Between Sleep Patterns and Stress Levels : Sleep patterns are related to variations in reported stress levels among individuals.
- Effects of Social Interaction on Happiness : Social interaction correlates with changes in reported happiness levels among participants.
Non-Directional Hypothesis Statement Examples for Psychology
These examples pertain to psychological studies and cover various relationships between psychological hypothesis concepts. For instance, the first example suggests that attachment styles might be related to romantic satisfaction, but it doesn’t specify whether attachment styles would increase or decrease satisfaction.
- Relationship Between Attachment Styles and Romantic Satisfaction : Attachment styles are related to variations in reported romantic satisfaction levels among individuals in psychology studies.
- Impact of Personality Traits on Career Success : Personality traits correlate with differences in reported career success outcomes among psychology study participants.
- Connection Between Parenting Styles and Adolescent Self-Esteem : Parenting styles are associated with variations in reported self-esteem levels among adolescents in psychological research.
- Effects of Social Media Use on Body Image : Social media use relates to changes in reported body image satisfaction among young adults in psychology experiments.
- Link Between Sleep Patterns and Emotional Well-being : Sleep patterns are related to differences in reported emotional well-being levels among psychology research participants.
- Influence of Mindfulness Practices on Stress Reduction : Mindfulness practices correlate with variations in reported stress reduction among psychology study participants.
- Relationship Between Social Interaction and Mental Health : Social interaction is associated with changes in reported mental health outcomes among individuals in psychology studies.
- Impact of Parent-Child Bonding on Emotional Resilience : Parent-child bonding relates to differences in reported emotional resilience levels among psychology research participants.
- Connection Between Cultural Sensitivity and Empathy : Cultural sensitivity is related to variations in reported empathy levels among individuals in psychology experiments.
- Effects of Exercise on Mood : Exercise correlates with changes in reported mood levels among psychology study participants.
Non-Directional Hypothesis Statement Examples in Research
These research hypothesis examples focus on research studies in general, covering a wide range of topics and relationships. For instance, the second example suggests that employee training might be related to workplace productivity, without indicating whether the training would lead to higher or lower productivity.
- Relationship Between Time Management and Academic Performance : Time management is related to variations in academic performance levels among research participants.
- Impact of Employee Training on Workplace Productivity : Employee training correlates with differences in reported workplace productivity outcomes among research subjects.
- Connection Between Media Exposure and Political Knowledge : Media exposure is associated with variations in reported political knowledge levels among research participants.
- Effects of Environmental Factors on Children’s Cognitive Development : Environmental factors relate to changes in reported cognitive development among research subjects.
- Link Between Parental Involvement and Student Motivation : Parental involvement is related to differences in reported student motivation levels among research participants.
- Influence of Cultural Immersion on Language Proficiency : Cultural immersion correlates with variations in reported language proficiency levels among research subjects.
- Relationship Between Leadership Styles and Team Performance : Leadership styles are associated with changes in reported team performance outcomes among research participants.
- Impact of Financial Literacy Education on Savings Habits : Financial literacy education relates to differences in reported savings habits among research subjects.
- Connection Between Stress Levels and Physical Health : Stress levels are related to variations in reported physical health outcomes among research participants.
- Effects of Music Exposure on Concentration : Music exposure correlates with changes in reported concentration levels among research subjects.
Non-Directional Hypothesis Statement Examples for Research Methodology
These examples are specific to the methods used in conducting research. The eighth example states that randomization might relate to group equivalence, but it doesn’t specify whether randomization would lead to more equivalent or less equivalent groups.
- Relationship Between Sampling Techniques and Research Validity : Sampling techniques are related to variations in research validity outcomes in studies of research methodology.
- Impact of Data Collection Methods on Data Accuracy : Data collection methods correlate with differences in reported data accuracy in research methodology experiments.
- Connection Between Research Design and Study Reproducibility : Research design is associated with variations in reported study reproducibility in research methodology studies.
- Effects of Questionnaire Format on Response Consistency : Questionnaire format relates to changes in reported response consistency in research methodology research.
- Link Between Ethical Considerations and Research Credibility : Ethical considerations are related to differences in reported research credibility in studies of research methodology.
- Influence of Measurement Scales on Data Precision : Measurement scales correlate with variations in reported data precision in research methodology experiments.
- Relationship Between Experimental Controls and Internal Validity : Experimental controls are associated with changes in internal validity outcomes in research methodology studies.
- Impact of Randomization on Group Equivalence : Randomization relates to differences in reported group equivalence in research methodology research.
- Connection Between Qualitative Data Analysis Methods and Data Richness : Qualitative data analysis methods are related to variations in reported data richness in studies of research methodology.
- Effects of Hypothesis Formulation on Research Focus : Hypothesis formulation correlates with changes in reported research focus in research methodology experiments.
These non-directional hypothesis statement examples offer insights into the diverse array of relationships explored in psychology, research, and research methodology studies, fostering empirical discovery and contributing to the advancement of knowledge across various fields.
Difference between Directional & Non-Directional Hypothesis
Directional and non-directional hypotheses are distinct approaches used in formulating hypotheses for research studies. Understanding the differences between them is essential for researchers to choose the appropriate type of causal hypothesis based on their study’s goals and prior knowledge.
- Direction: Directional hypotheses predict a specific relationship direction, while non-directional hypotheses do not specify a direction.
- Specificity: Directional hypotheses are more specific, while non-directional hypotheses are more general.
- Flexibility: Non-directional hypotheses allow for open-ended exploration, while directional hypotheses focus on confirming or refuting specific expectations.
How to Write a Non-Directional Hypothesis Statement – Step by Step Guide
- Identify Variables: Clearly define the variables you’re investigating—usually, an independent variable (the one manipulated) and a dependent variable (the one measured).
- Indicate Relationship: State that a relationship exists between the variables without predicting a specific direction.
- Use General Language: Craft the statement in a way that encompasses various possible outcomes.
- Avoid Biased Language: Do not include words that suggest a stronger effect or specific outcome for either variable.
- Connect to Research: If applicable, link the hypothesis to existing research or theories that justify exploring the relationship.
Tips for Writing a Non-Directional Hypothesis
- Start with Inquiry: Frame your hypothesis as an answer to a research question.
- Embrace Openness: Non-directional hypotheses are ideal when no strong expectation exists.
- Be Succinct: Keep the hypothesis statement concise and clear.
- Stay Neutral: Avoid implying that one variable will have a stronger impact.
- Allow Exploration: Leave room for various potential outcomes without preconceived notions.
- Tailor to Context: Ensure the hypothesis aligns with your research context and goals.
Non-directional hypotheses are particularly useful in exploratory research, where researchers aim to discover relationships without imposing specific expectations. They allow for unbiased investigation and the potential to uncover unexpected patterns or connections.
Remember that whether you choose a directional or non-directional hypothesis, both play critical roles in shaping the research process, guiding study design, data collection, and analysis. The choice depends on the research’s nature, goals, and existing knowledge in the field. You may also be interested in our science hypothesis .
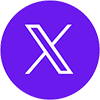
Text prompt
- Instructive
- Professional
10 Examples of Public speaking
20 Examples of Gas lighting
Live revision! Join us for our free exam revision livestreams Watch now →
Reference Library
Collections
- See what's new
- All Resources
- Student Resources
- Assessment Resources
- Teaching Resources
- CPD Courses
- Livestreams
Study notes, videos, interactive activities and more!
Psychology news, insights and enrichment
Currated collections of free resources
Browse resources by topic
- All Psychology Resources
Resource Selections
Currated lists of resources
Non-Directional Hypothesis
A non-directional hypothesis is a two-tailed hypothesis that does not predict the direction of the difference or relationship (e.g. girls and boys are different in terms of helpfulness).
- Share on Facebook
- Share on Twitter
- Share by Email
Research Methods: MCQ Revision Test 1 for AQA A Level Psychology
Topic Videos
Example Answers for Research Methods: A Level Psychology, Paper 2, June 2018 (AQA)
Exam Support
Example Answer for Question 14 Paper 2: AS Psychology, June 2017 (AQA)
Model answer for question 11 paper 2: as psychology, june 2016 (aqa), a level psychology topic quiz - research methods.
Quizzes & Activities
Our subjects
- › Criminology
- › Economics
- › Geography
- › Health & Social Care
- › Psychology
- › Sociology
- › Teaching & learning resources
- › Student revision workshops
- › Online student courses
- › CPD for teachers
- › Livestreams
- › Teaching jobs
Boston House, 214 High Street, Boston Spa, West Yorkshire, LS23 6AD Tel: 01937 848885
- › Contact us
- › Terms of use
- › Privacy & cookies
© 2002-2024 Tutor2u Limited. Company Reg no: 04489574. VAT reg no 816865400.
- The Open University
- Accessibility hub
- Guest user / Sign out
- Study with The Open University
My OpenLearn Profile
Personalise your OpenLearn profile, save your favourite content and get recognition for your learning
About this free course
Become an ou student, download this course, share this free course.
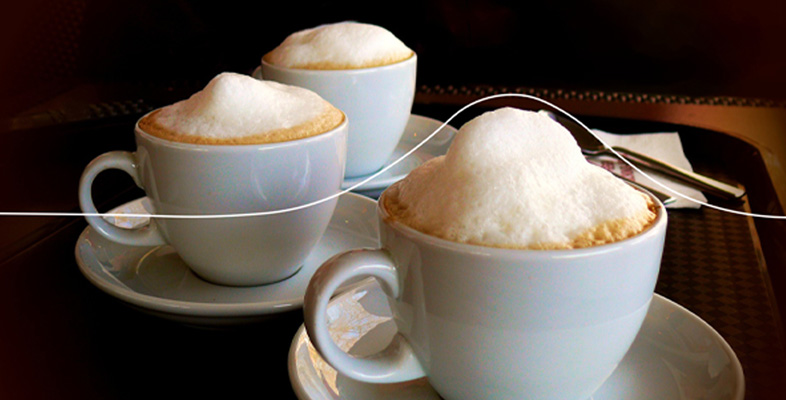
Start this free course now. Just create an account and sign in. Enrol and complete the course for a free statement of participation or digital badge if available.
4.2 Two-tailed tests
Hypotheses that have an equal (=) or not equal (≠) supposition (sign) in the statement are called non-directional hypotheses . In non-directional hypotheses, the researcher is interested in whether there is a statistically significant difference or relationship between two or more variables, but does not have any specific expectation about which group or variable will be higher or lower. For example, a non-directional hypothesis might be: ‘There is a difference in the preference for brand X between male and female consumers.’ In this hypothesis, the researcher is interested in whether there is a statistically significant difference in the preference for brand X between male and female consumers, but does not have a specific prediction about which gender will have a higher preference. The researcher may conduct a survey or experiment to collect data on the brand preference of male and female consumers and then use statistical analysis to determine whether there is a significant difference between the two groups.
Non-directional hypotheses are also known as two-tailed hypotheses. The term ‘two-tailed’ comes from the fact that the statistical test used to evaluate the hypothesis is based on the assumption that the difference or relationship could occur in either direction, resulting in two ‘tails’ in the probability distribution. Using the coffee foam example (from Activity 1), you have the following set of hypotheses:
H 0 : µ = 1cm foam
H a : µ ≠ 1cm foam
In this case, the researcher can reject the null hypothesis for the mean value that is either ‘much higher’ or ‘much lower’ than 1 cm foam. This is called a two-tailed test because the rejection region includes outcomes from both the upper and lower tails of the sample distribution when determining a decision rule. To give an illustration, if you set alpha level (α) equal to 0.05, that would give you a 95% confidence level. Then, you would reject the null hypothesis for obtained values of z 1.96 (you will look at how to calculate z-scores later in the course).
This can be plotted on a graph as shown in Figure 7.
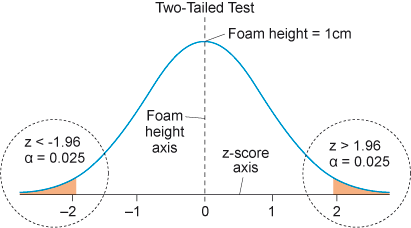
A symmetrical graph reminiscent of a bell. The x-axis is labelled ‘z-score’ and the y-axis is labelled ‘probability density’. The x-axis increases in increments of 1 from -2 to 2.
The top of the bell-shaped curve is labelled ‘Foam height = 1cm’. The graph circles the rejection regions of the null hypothesis on both sides of the bell curve. Within these circles are two areas shaded orange: beneath the curve from -2 downwards which is labelled z 1.96 and α = 0.025.
In a two-tailed hypothesis test, the null hypothesis assumes that there is no significant difference or relationship between the two groups or variables, and the alternative hypothesis suggests that there is a significant difference or relationship, but does not specify the direction of the difference or relationship.
When performing a two-tailed test, you need to determine the level of significance, which is denoted by alpha (α). The value of alpha, in this case, is 0.05. To perform a two-tailed test at a significance level of 0.05, you need to divide alpha by 2, giving a significance level of 0.025 for each distribution tail (0.05/2 = 0.025). This is done because the two-tailed test is looking for significance in either tail of the distribution. If the calculated test statistic falls in the rejection region of either tail of the distribution, then the null hypothesis is rejected and the alternative hypothesis is accepted. In this case, the researcher can conclude that there is a significant difference or relationship between the two groups or variables.
Assuming that the population follows a normal distribution, the tail located below the critical value of z = –1.96 (in a later section, you will discuss how this value was determined) and the tail above the critical value of z = +1.96 each represent a proportion of 0.025. These tails are referred to as the lower and upper tails, respectively, and they correspond to the extreme values of the distribution that are far from the central part of the bell curve. These critical values are used in a two-tailed hypothesis test to determine whether to reject or fail to reject the null hypothesis. The null hypothesis represents the default assumption that there is no significant difference between the observed data and what would be expected under a specific condition.
If the calculated test statistic falls within the critical values, then the null hypothesis cannot be rejected at the 0.05 level of significance. However, if the calculated test statistic falls outside the critical values (orange-coloured areas in Figure 7), then the null hypothesis can be rejected in favour of the alternative hypothesis, suggesting that there is evidence of a significant difference between the observed data and what would be expected under the specified condition.

Directional Hypothesis: Definition and 10 Examples
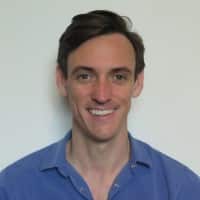
Chris Drew (PhD)
Dr. Chris Drew is the founder of the Helpful Professor. He holds a PhD in education and has published over 20 articles in scholarly journals. He is the former editor of the Journal of Learning Development in Higher Education. [Image Descriptor: Photo of Chris]
Learn about our Editorial Process
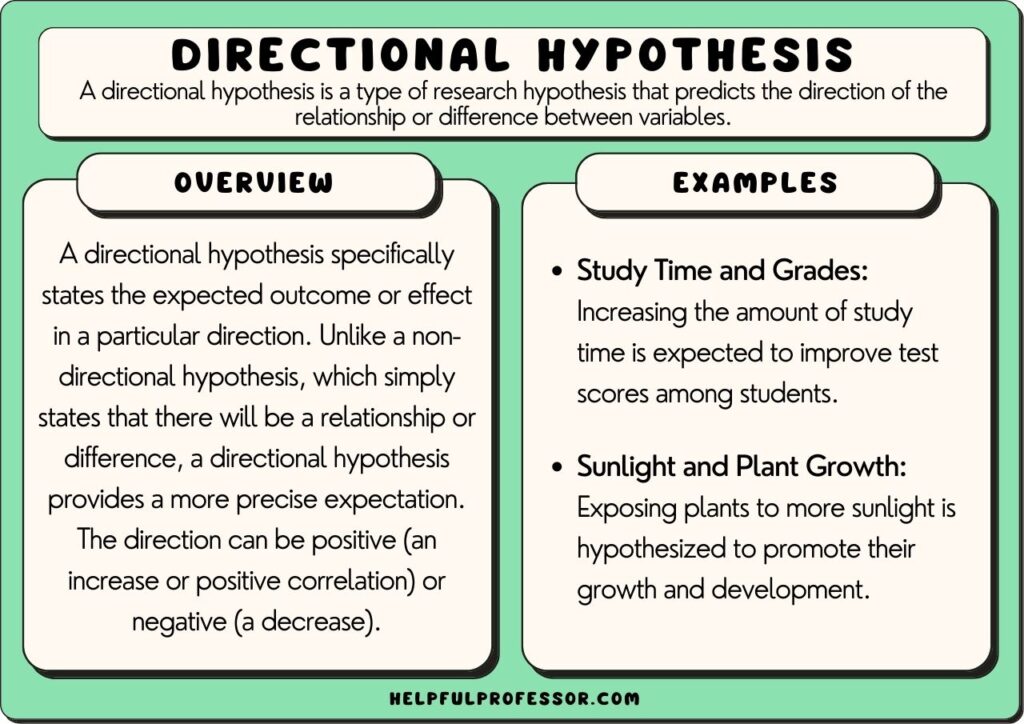
A directional hypothesis refers to a type of hypothesis used in statistical testing that predicts a particular direction of the expected relationship between two variables.
In simpler terms, a directional hypothesis is an educated, specific guess about the direction of an outcome—whether an increase, decrease, or a proclaimed difference in variable sets.
For example, in a study investigating the effects of sleep deprivation on cognitive performance, a directional hypothesis might state that as sleep deprivation (Independent Variable) increases, cognitive performance (Dependent Variable) decreases (Killgore, 2010). Such a hypothesis offers a clear, directional relationship whereby a specific increase or decrease is anticipated.
Global warming provides another notable example of a directional hypothesis. A researcher might hypothesize that as carbon dioxide (CO2) levels increase, global temperatures also increase (Thompson, 2010). In this instance, the hypothesis clearly articulates an upward trend for both variables.
In any given circumstance, it’s imperative that a directional hypothesis is grounded on solid evidence. For instance, the CO2 and global temperature relationship is based on substantial scientific evidence, and not on a random guess or mere speculation (Florides & Christodoulides, 2009).
Directional vs Non-Directional vs Null Hypotheses
A directional hypothesis is generally contrasted to a non-directional hypothesis. Here’s how they compare:
- Directional hypothesis: A directional hypothesis provides a perspective of the expected relationship between variables, predicting the direction of that relationship (either positive, negative, or a specific difference).
- Non-directional hypothesis: A non-directional hypothesis denotes the possibility of a relationship between two variables ( the independent and dependent variables ), although this hypothesis does not venture a prediction as to the direction of this relationship (Ali & Bhaskar, 2016). For example, a non-directional hypothesis might state that there exists a relationship between a person’s diet (independent variable) and their mood (dependent variable), without indicating whether improvement in diet enhances mood positively or negatively. Overall, the choice between a directional or non-directional hypothesis depends on the known or anticipated link between the variables under consideration in research studies.
Another very important type of hypothesis that we need to know about is a null hypothesis :
- Null hypothesis : The null hypothesis stands as a universality—the hypothesis that there is no observed effect in the population under study, meaning there is no association between variables (or that the differences are down to chance). For instance, a null hypothesis could be constructed around the idea that changing diet (independent variable) has no discernible effect on a person’s mood (dependent variable) (Yan & Su, 2016). This proposition is the one that we aim to disprove in an experiment.
While directional and non-directional hypotheses involve some integrated expectations about the outcomes (either distinct direction or a vague relationship), a null hypothesis operates on the premise of negating such relationships or effects.
The null hypotheses is typically proposed to be negated or disproved by statistical tests, paving way for the acceptance of an alternate hypothesis (either directional or non-directional).
Directional Hypothesis Examples
1. exercise and heart health.
Research suggests that as regular physical exercise (independent variable) increases, the risk of heart disease (dependent variable) decreases (Jakicic, Davis, Rogers, King, Marcus, Helsel, Rickman, Wahed, Belle, 2016). In this example, a directional hypothesis anticipates that the more individuals maintain routine workouts, the lesser would be their odds of developing heart-related disorders. This assumption is based on the underlying fact that routine exercise can help reduce harmful cholesterol levels, regulate blood pressure, and bring about overall health benefits. Thus, a direction – a decrease in heart disease – is expected in relation with an increase in exercise.
2. Screen Time and Sleep Quality
Another classic instance of a directional hypothesis can be seen in the relationship between the independent variable, screen time (especially before bed), and the dependent variable, sleep quality. This hypothesis predicts that as screen time before bed increases, sleep quality decreases (Chang, Aeschbach, Duffy, Czeisler, 2015). The reasoning behind this hypothesis is the disruptive effect of artificial light (especially blue light from screens) on melatonin production, a hormone needed to regulate sleep. As individuals spend more time exposed to screens before bed, it is predictably hypothesized that their sleep quality worsens.
3. Job Satisfaction and Employee Turnover
A typical scenario in organizational behavior research posits that as job satisfaction (independent variable) increases, the rate of employee turnover (dependent variable) decreases (Cheng, Jiang, & Riley, 2017). This directional hypothesis emphasizes that an increased level of job satisfaction would lead to a reduced rate of employees leaving the company. The theoretical basis for this hypothesis is that satisfied employees often tend to be more committed to the organization and are less likely to seek employment elsewhere, thus reducing turnover rates.
4. Healthy Eating and Body Weight
Healthy eating, as the independent variable, is commonly thought to influence body weight, the dependent variable, in a positive way. For example, the hypothesis might state that as consumption of healthy foods increases, an individual’s body weight decreases (Framson, Kristal, Schenk, Littman, Zeliadt, & Benitez, 2009). This projection is based on the premise that healthier foods, such as fruits and vegetables, are generally lower in calories than junk food, assisting in weight management.
5. Sun Exposure and Skin Health
The association between sun exposure (independent variable) and skin health (dependent variable) allows for a definitive hypothesis declaring that as sun exposure increases, the risk of skin damage or skin cancer increases (Whiteman, Whiteman, & Green, 2001). The premise aligns with the understanding that overexposure to the sun’s ultraviolet rays can deteriorate skin health, leading to conditions like sunburn or, in extreme cases, skin cancer.
6. Study Hours and Academic Performance
A regularly assessed relationship in academia suggests that as the number of study hours (independent variable) rises, so too does academic performance (dependent variable) (Nonis, Hudson, Logan, Ford, 2013). The hypothesis proposes a positive correlation , with an increase in study time expected to contribute to enhanced academic outcomes.
7. Screen Time and Eye Strain
It’s commonly hypothesized that as screen time (independent variable) increases, the likelihood of experiencing eye strain (dependent variable) also increases (Sheppard & Wolffsohn, 2018). This is based on the idea that prolonged engagement with digital screens—computers, tablets, or mobile phones—can cause discomfort or fatigue in the eyes, attributing to symptoms of eye strain.
8. Physical Activity and Stress Levels
In the sphere of mental health, it’s often proposed that as physical activity (independent variable) increases, levels of stress (dependent variable) decrease (Stonerock, Hoffman, Smith, Blumenthal, 2015). Regular exercise is known to stimulate the production of endorphins, the body’s natural mood elevators, helping to alleviate stress.
9. Water Consumption and Kidney Health
A common health-related hypothesis might predict that as water consumption (independent variable) increases, the risk of kidney stones (dependent variable) decreases (Curhan, Willett, Knight, & Stampfer, 2004). Here, an increase in water intake is inferred to reduce the risk of kidney stones by diluting the substances that lead to stone formation.
10. Traffic Noise and Sleep Quality
In urban planning research, it’s often supposed that as traffic noise (independent variable) increases, sleep quality (dependent variable) decreases (Muzet, 2007). Increased noise levels, particularly during the night, can result in sleep disruptions, thus, leading to poor sleep quality.
11. Sugar Consumption and Dental Health
In the field of dental health, an example might be stating as one’s sugar consumption (independent variable) increases, dental health (dependent variable) decreases (Sheiham, & James, 2014). This stems from the fact that sugar is a major factor in tooth decay, and increased consumption of sugary foods or drinks leads to a decline in dental health due to the high likelihood of cavities.
See 15 More Examples of Hypotheses Here
A directional hypothesis plays a critical role in research, paving the way for specific predicted outcomes based on the relationship between two variables. These hypotheses clearly illuminate the expected direction—the increase or decrease—of an effect. From predicting the impacts of healthy eating on body weight to forecasting the influence of screen time on sleep quality, directional hypotheses allow for targeted and strategic examination of phenomena. In essence, directional hypotheses provide the crucial path for inquiry, shaping the trajectory of research studies and ultimately aiding in the generation of insightful, relevant findings.
Ali, S., & Bhaskar, S. (2016). Basic statistical tools in research and data analysis. Indian Journal of Anaesthesia, 60 (9), 662-669. doi: https://doi.org/10.4103%2F0019-5049.190623
Chang, A. M., Aeschbach, D., Duffy, J. F., & Czeisler, C. A. (2015). Evening use of light-emitting eReaders negatively affects sleep, circadian timing, and next-morning alertness. Proceeding of the National Academy of Sciences, 112 (4), 1232-1237. doi: https://doi.org/10.1073/pnas.1418490112
Cheng, G. H. L., Jiang, D., & Riley, J. H. (2017). Organizational commitment and intrinsic motivation of regular and contractual primary school teachers in China. New Psychology, 19 (3), 316-326. Doi: https://doi.org/10.4103%2F2249-4863.184631
Curhan, G. C., Willett, W. C., Knight, E. L., & Stampfer, M. J. (2004). Dietary factors and the risk of incident kidney stones in younger women: Nurses’ Health Study II. Archives of Internal Medicine, 164 (8), 885–891.
Florides, G. A., & Christodoulides, P. (2009). Global warming and carbon dioxide through sciences. Environment international , 35 (2), 390-401. doi: https://doi.org/10.1016/j.envint.2008.07.007
Framson, C., Kristal, A. R., Schenk, J. M., Littman, A. J., Zeliadt, S., & Benitez, D. (2009). Development and validation of the mindful eating questionnaire. Journal of the American Dietetic Association, 109 (8), 1439-1444. doi: https://doi.org/10.1016/j.jada.2009.05.006
Jakicic, J. M., Davis, K. K., Rogers, R. J., King, W. C., Marcus, M. D., Helsel, D., … & Belle, S. H. (2016). Effect of wearable technology combined with a lifestyle intervention on long-term weight loss: The IDEA randomized clinical trial. JAMA, 316 (11), 1161-1171.
Khan, S., & Iqbal, N. (2013). Study of the relationship between study habits and academic achievement of students: A case of SPSS model. Higher Education Studies, 3 (1), 14-26.
Killgore, W. D. (2010). Effects of sleep deprivation on cognition. Progress in brain research , 185 , 105-129. doi: https://doi.org/10.1016/B978-0-444-53702-7.00007-5
Marczinski, C. A., & Fillmore, M. T. (2014). Dissociative antagonistic effects of caffeine on alcohol-induced impairment of behavioral control. Experimental and Clinical Psychopharmacology, 22 (4), 298–311. doi: https://psycnet.apa.org/doi/10.1037/1064-1297.11.3.228
Muzet, A. (2007). Environmental Noise, Sleep and Health. Sleep Medicine Reviews, 11 (2), 135-142. doi: https://doi.org/10.1016/j.smrv.2006.09.001
Nonis, S. A., Hudson, G. I., Logan, L. B., & Ford, C. W. (2013). Influence of perceived control over time on college students’ stress and stress-related outcomes. Research in Higher Education, 54 (5), 536-552. doi: https://doi.org/10.1023/A:1018753706925
Sheiham, A., & James, W. P. (2014). A new understanding of the relationship between sugars, dental caries and fluoride use: implications for limits on sugars consumption. Public health nutrition, 17 (10), 2176-2184. Doi: https://doi.org/10.1017/S136898001400113X
Sheppard, A. L., & Wolffsohn, J. S. (2018). Digital eye strain: prevalence, measurement and amelioration. BMJ open ophthalmology , 3 (1), e000146. doi: http://dx.doi.org/10.1136/bmjophth-2018-000146
Stonerock, G. L., Hoffman, B. M., Smith, P. J., & Blumenthal, J. A. (2015). Exercise as Treatment for Anxiety: Systematic Review and Analysis. Annals of Behavioral Medicine, 49 (4), 542–556. doi: https://doi.org/10.1007/s12160-014-9685-9
Thompson, L. G. (2010). Climate change: The evidence and our options. The Behavior Analyst , 33 , 153-170. Doi: https://doi.org/10.1007/BF03392211
Whiteman, D. C., Whiteman, C. A., & Green, A. C. (2001). Childhood sun exposure as a risk factor for melanoma: a systematic review of epidemiologic studies. Cancer Causes & Control, 12 (1), 69-82. doi: https://doi.org/10.1023/A:1008980919928
Yan, X., & Su, X. (2009). Linear regression analysis: theory and computing . New Jersey: World Scientific.
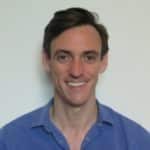
- Chris Drew (PhD) https://helpfulprofessor.com/author/chris-drew-phd/ 101 Class Group Name Ideas (for School Students)
- Chris Drew (PhD) https://helpfulprofessor.com/author/chris-drew-phd/ 19 Top Cognitive Psychology Theories (Explained)
- Chris Drew (PhD) https://helpfulprofessor.com/author/chris-drew-phd/ 119 Bloom’s Taxonomy Examples
- Chris Drew (PhD) https://helpfulprofessor.com/author/chris-drew-phd/ All 6 Levels of Understanding (on Bloom’s Taxonomy)
Leave a Comment Cancel Reply
Your email address will not be published. Required fields are marked *
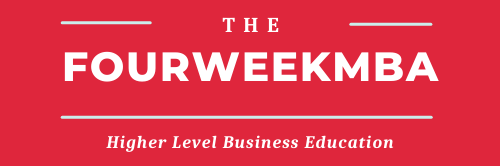
The Leading Source of Insights On Business Model Strategy & Tech Business Models

Non-Directional Hypothesis
Non-directional hypotheses, also known as null hypotheses, serve as a foundational concept in scientific research across various fields, from psychology and biology to economics and sociology. These hypotheses are essential for hypothesis testing, where researchers aim to determine whether observed data supports or contradicts their predictions. In contrast to directional hypotheses, which specify the expected direction of a relationship , non-directional hypotheses do not make explicit predictions about the direction of the relationship between variables. Instead, they focus on testing for the mere presence or absence of a relationship .
Table of Contents
Understanding Non-Directional Hypotheses
A hypothesis is a statement that outlines a testable prediction about the relationship between variables in a research study. Hypotheses are fundamental to the scientific method, guiding the design of experiments and the analysis of data. While hypotheses can take various forms, including directional hypotheses, non-directional hypotheses, or null hypotheses, serve a specific purpose in hypothesis testing.
Non-directional hypotheses , often referred to as null hypotheses, do not make explicit predictions about the direction of the relationship between variables. Instead, they state that there is no significant relationship , difference, or effect. Researchers use non-directional hypotheses when they do not have a specific expectation regarding the outcome of their study or when they aim to test for the mere presence or absence of an effect.
In summary, non-directional hypotheses:
- Do not predict the direction of the relationship between variables.
- State that there is no significant relationship , difference, or effect.
- Are used when researchers do not have specific expectations or when they want to test for the presence or absence of an effect.
The Structure of Non-Directional Hypotheses
Non-directional hypotheses typically consist of two main components: the independent variable (IV) and the dependent variable (DV). The IV is the variable that researchers manipulate or examine for its effect on the DV, which is the variable that researchers measure or observe. Here is the general structure of a non-directional hypothesis:
“There is no significant relationship /difference/effect between [IV] and [DV].”
- “There is no” indicates the absence of a predicted effect.
- “Significant” implies that the absence of the effect will be tested statistically.
- “[IV]” represents the independent variable.
- “and” connects the independent variable to the dependent variable.
- “[DV]” represents the dependent variable.
Let’s break down the structure with a few examples:
- “There is no significant relationship between the amount of rainfall and crop yield.”
- “There is no significant difference in blood pressure between participants who take Drug A and those who take a placebo.”
- “There is no significant effect of gender on test performance .”
Significance and Advantages of Non-Directional Hypotheses
Non-directional hypotheses offer several advantages in scientific research:
1. Objectivity:
- Non-directional hypotheses are objective and neutral statements that do not impose specific expectations on the outcomes of a study. This objectivity is particularly important when researchers have limited prior knowledge about the variables being studied.
2. Flexibility:
- Non-directional hypotheses are versatile and can be used in a wide range of research scenarios. They are not constrained by the need to predict a specific direction of the relationship , making them suitable for exploratory or preliminary research.
3. Rigorous Testing:
- Non-directional hypotheses undergo rigorous statistical testing to determine whether the observed results are statistically significant. This testing provides a rigorous assessment of the presence or absence of effects.
4. Comparison with Alternative Hypotheses:
- Non-directional hypotheses can be compared with alternative hypotheses, including directional hypotheses, to evaluate which hypothesis best fits the observed data. This process helps researchers refine their theories and hypotheses.
5. Hypothesis Testing:
- Non-directional hypotheses are central to hypothesis testing, a fundamental aspect of the scientific method. Hypothesis testing enables researchers to draw conclusions about the relationships between variables based on empirical evidence.
Formulating Non-Directional Hypotheses
Formulating effective non-directional hypotheses requires a systematic approach. Here are the steps to formulate a non-directional hypothesis:
1. Identify the Variables:
- Begin by identifying the independent and dependent variables in your study. These variables should be clearly defined and measurable.
2. Clarify the Research Question:
- Clearly state the research question that you aim to answer. The research question should focus on the relationship , difference, or effect you want to investigate.
3. Determine the Absence of Effect:
- Consider what it would mean for there to be no significant relationship , difference, or effect between the variables. This helps in formulating the negation that characterizes non-directional hypotheses.
4. Craft the Hypothesis:
- Use the structure mentioned earlier to craft your non-directional hypothesis. Ensure that it is clear and concise, stating that there is no significant relationship , difference, or effect between the variables.
5. Ensure Testability:
- Ensure that your non-directional hypothesis is testable. This means that you should be able to collect data and analyze it to determine whether the observed results support or contradict the hypothesis.
6. Revise and Refine:
- Review your non-directional hypothesis and refine it as needed. Seek feedback from colleagues or mentors to improve its clarity and specificity.
Examples of Non-Directional Hypotheses
Non-directional hypotheses are used in various fields of research to test for the mere presence or absence of effects. Here are some examples from different scientific disciplines:
1. Psychology:
- Research Question: Does exposure to a new teaching method affect students’ test performance ?
- Non-Directional Hypothesis: “There is no significant difference in test performance between students exposed to the new teaching method and those not exposed to it.”
2. Biology:
- Research Question: Is there a relationship between the presence of a specific gene variant and the risk of a certain disease?
- Non-Directional Hypothesis: “There is no significant relationship between the presence of the gene variant and the risk of the disease.”
3. Economics:
- Research Question: Does the introduction of a new tax policy lead to changes in consumer spending behavior?
- Non-Directional Hypothesis: “There is no significant relationship between the introduction of the new tax policy and changes in consumer spending behavior.”
4. Sociology:
- Research Question: Is there an association between parental involvement and students’ academic achievement?
- Non-Directional Hypothesis: “There is no significant relationship between parental involvement and students’ academic achievement.”
5. Environmental Science:
- Research Question: Does the level of air pollution impact respiratory health in urban areas?
- Non-Directional Hypothesis: “There is no significant relationship between the level of air pollution and respiratory health in urban areas.”
Null Hypothesis Testing
Non-directional hypotheses, also known as null hypotheses, are subjected to rigorous testing to determine whether the observed results support or contradict the hypothesis. The process of testing the null hypothesis involves collecting data, performing statistical analyses, and assessing whether the observed differences or effects are
statistically significant.
In hypothesis testing, researchers aim to reject the null hypothesis if the observed results are highly unlikely to occur by chance. If the null hypothesis is rejected, it suggests that there is a significant relationship , difference, or effect between the variables. If the null hypothesis is not rejected, it indicates that there is no significant evidence to support the presence of the hypothesized effect.
The significance level (often denoted as α) is predetermined by researchers and represents the threshold for determining statistical significance. Common significance levels include 0.05 and 0.01, indicating that researchers are willing to accept a 5% or 1% chance of making a Type I error (incorrectly rejecting a true null hypothesis).
Non-directional hypotheses, also known as null hypotheses, are fundamental to hypothesis testing and the scientific method. They allow researchers to test for the mere presence or absence of relationships, differences, or effects between variables, without making specific predictions about the direction of these relationships.
The objectivity, versatility, and rigor of null hypothesis testing make it an essential tool in scientific research across diverse disciplines. By formulating and testing non-directional hypotheses, researchers contribute to the systematic accumulation of knowledge, refine their theories, and draw meaningful conclusions about the relationships between variables.
Whether in the natural sciences, social sciences, or humanities, non-directional hypotheses remain a cornerstone of empirical research, enabling researchers to explore, question, and expand our understanding of the complex phenomena that shape our world.
Connected Thinking Frameworks
Convergent vs. Divergent Thinking

Critical Thinking

Second-Order Thinking

Lateral Thinking

Bounded Rationality

Dunning-Kruger Effect

Occam’s Razor

Lindy Effect

Antifragility

Systems Thinking

Vertical Thinking

Maslow’s Hammer

Peter Principle

Straw Man Fallacy

Streisand Effect

Recognition Heuristic

Representativeness Heuristic

Take-The-Best Heuristic

Bundling Bias

Barnum Effect

First-Principles Thinking

Ladder Of Inference

Goodhart’s Law

Six Thinking Hats Model

Mandela Effect

Crowding-Out Effect

Bandwagon Effect

Moore’s Law

Disruptive Innovation

Value Migration

Bye-Now Effect

Stereotyping

Murphy’s Law

Law of Unintended Consequences

Fundamental Attribution Error

Outcome Bias

Hindsight Bias

Read Next: Biases , Bounded Rationality , Mandela Effect , Dunning-Kruger Effect , Lindy Effect , Crowding Out Effect , Bandwagon Effect .
Main Guides:
- Business Models
- Business Strategy
- Marketing Strategy
- Business Model Innovation
- Platform Business Models
- Network Effects In A Nutshell
- Digital Business Models
More Resources

About The Author
Gennaro Cuofano
Discover more from fourweekmba.
Subscribe now to keep reading and get access to the full archive.
Type your email…
Continue reading

Statistics Made Easy
What is a Directional Hypothesis? (Definition & Examples)
A statistical hypothesis is an assumption about a population parameter . For example, we may assume that the mean height of a male in the U.S. is 70 inches.
The assumption about the height is the statistical hypothesis and the true mean height of a male in the U.S. is the population parameter .
To test whether a statistical hypothesis about a population parameter is true, we obtain a random sample from the population and perform a hypothesis test on the sample data.
Whenever we perform a hypothesis test, we always write down a null and alternative hypothesis:
- Null Hypothesis (H 0 ): The sample data occurs purely from chance.
- Alternative Hypothesis (H A ): The sample data is influenced by some non-random cause.
A hypothesis test can either contain a directional hypothesis or a non-directional hypothesis:
- Directional hypothesis: The alternative hypothesis contains the less than (“<“) or greater than (“>”) sign. This indicates that we’re testing whether or not there is a positive or negative effect.
- Non-directional hypothesis: The alternative hypothesis contains the not equal (“≠”) sign. This indicates that we’re testing whether or not there is some effect, without specifying the direction of the effect.
Note that directional hypothesis tests are also called “one-tailed” tests and non-directional hypothesis tests are also called “two-tailed” tests.
Check out the following examples to gain a better understanding of directional vs. non-directional hypothesis tests.
Example 1: Baseball Programs
A baseball coach believes a certain 4-week program will increase the mean hitting percentage of his players, which is currently 0.285.
To test this, he measures the hitting percentage of each of his players before and after participating in the program.
He then performs a hypothesis test using the following hypotheses:
- H 0 : μ = .285 (the program will have no effect on the mean hitting percentage)
- H A : μ > .285 (the program will cause mean hitting percentage to increase)
This is an example of a directional hypothesis because the alternative hypothesis contains the greater than “>” sign. The coach believes that the program will influence the mean hitting percentage of his players in a positive direction.
Example 2: Plant Growth
A biologist believes that a certain pesticide will cause plants to grow less during a one-month period than they normally do, which is currently 10 inches.
To test this, she applies the pesticide to each of the plants in her laboratory for one month.
She then performs a hypothesis test using the following hypotheses:
- H 0 : μ = 10 inches (the pesticide will have no effect on the mean plant growth)
- H A : μ < 10 inches (the pesticide will cause mean plant growth to decrease)
This is also an example of a directional hypothesis because the alternative hypothesis contains the less than “<” sign. The biologist believes that the pesticide will influence the mean plant growth in a negative direction.
Example 3: Studying Technique
A professor believes that a certain studying technique will influence the mean score that her students receive on a certain exam, but she’s unsure if it will increase or decrease the mean score, which is currently 82.
To test this, she lets each student use the studying technique for one month leading up to the exam and then administers the same exam to each of the students.
- H 0 : μ = 82 (the studying technique will have no effect on the mean exam score)
- H A : μ ≠ 82 (the studying technique will cause the mean exam score to be different than 82)
This is an example of a non-directional hypothesis because the alternative hypothesis contains the not equal “≠” sign. The professor believes that the studying technique will influence the mean exam score, but doesn’t specify whether it will cause the mean score to increase or decrease.
Additional Resources
Introduction to Hypothesis Testing Introduction to the One Sample t-test Introduction to the Two Sample t-test Introduction to the Paired Samples t-test
Featured Posts

Hey there. My name is Zach Bobbitt. I have a Masters of Science degree in Applied Statistics and I’ve worked on machine learning algorithms for professional businesses in both healthcare and retail. I’m passionate about statistics, machine learning, and data visualization and I created Statology to be a resource for both students and teachers alike. My goal with this site is to help you learn statistics through using simple terms, plenty of real-world examples, and helpful illustrations.
Leave a Reply Cancel reply
Your email address will not be published. Required fields are marked *
Join the Statology Community
Sign up to receive Statology's exclusive study resource: 100 practice problems with step-by-step solutions. Plus, get our latest insights, tutorials, and data analysis tips straight to your inbox!
By subscribing you accept Statology's Privacy Policy.
Information
- Author Services
Initiatives
You are accessing a machine-readable page. In order to be human-readable, please install an RSS reader.
All articles published by MDPI are made immediately available worldwide under an open access license. No special permission is required to reuse all or part of the article published by MDPI, including figures and tables. For articles published under an open access Creative Common CC BY license, any part of the article may be reused without permission provided that the original article is clearly cited. For more information, please refer to https://www.mdpi.com/openaccess .
Feature papers represent the most advanced research with significant potential for high impact in the field. A Feature Paper should be a substantial original Article that involves several techniques or approaches, provides an outlook for future research directions and describes possible research applications.
Feature papers are submitted upon individual invitation or recommendation by the scientific editors and must receive positive feedback from the reviewers.
Editor’s Choice articles are based on recommendations by the scientific editors of MDPI journals from around the world. Editors select a small number of articles recently published in the journal that they believe will be particularly interesting to readers, or important in the respective research area. The aim is to provide a snapshot of some of the most exciting work published in the various research areas of the journal.
Original Submission Date Received: .
- Active Journals
- Find a Journal
- Proceedings Series
- For Authors
- For Reviewers
- For Editors
- For Librarians
- For Publishers
- For Societies
- For Conference Organizers
- Open Access Policy
- Institutional Open Access Program
- Special Issues Guidelines
- Editorial Process
- Research and Publication Ethics
- Article Processing Charges
- Testimonials
- Preprints.org
- SciProfiles
- Encyclopedia
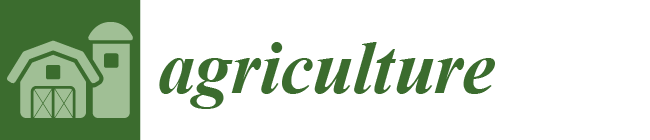
Article Menu
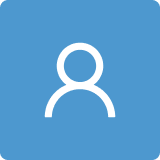
- Subscribe SciFeed
- Recommended Articles
- Google Scholar
- on Google Scholar
- Table of Contents
Find support for a specific problem in the support section of our website.
Please let us know what you think of our products and services.
Visit our dedicated information section to learn more about MDPI.
JSmol Viewer
Role of policy-supported hog insurance in promoting green total factor productivity: the case of china during 2005–2021.
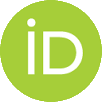
1. Introduction
2. literature review, 2.1. research on agricultural insurance, 2.2. research on gtfp, 2.3. research on the impact of agricultural insurance on gtfp, 3. policy background and theoretical analysis, 3.1. policy background on china’s policy-supported hog insurance, 3.2. theoretical analyses and research hypotheses, 3.2.1. impact analysis of hog insurance on gtfp in farming, 3.2.2. mechanism analysis of hog insurance for gtfp in farming, 4. materials and methods, 4.1. model settings, 4.1.1. sbm-ddf model with undesirable outputs, 4.1.2. multiple-time-point difference-in-differences model, 4.2. data, variables, and descriptive statistics, 5.1. parallel trend test, 5.2. baseline regression: impact of hog insurance policy on gtfp, 5.3. robustness checks, 5.3.1. placebo test, 5.3.2. preceding and lagging policy by three years, 5.3.3. lagging of control variables by one period, 6. mechanism analysis, 6.1. scale effects test, 6.2. structural effects test, 6.3. technological effects test, 7. heterogeneity analysis, 7.1. economic heterogeneity, 7.2. scale heterogeneity, 8. discussion, 9. concluding remarks and policy implications, author contributions, data availability statement, acknowledgments, conflicts of interest.
- Mapp, H.P.; Hardin, M.L.; Walker, O.L.; Persaud, T. Analysis of Risk Management Strategies for Agricultural Producers. Am. J. Agric. Econ. 1979 , 61 , 1071–1077. [ Google Scholar ] [ CrossRef ]
- Komarek, A.M.; De Pinto, A.; Smith, V.H. A review of types of risks in agriculture: What we know and what we need to know. Agric. Syst. 2020 , 178 , 102738. [ Google Scholar ] [ CrossRef ]
- Van Huis, A.; Oonincx, D.G.A.B. The environmental sustainability of insects as food and feed. A review. Agron. Sustain. Dev. 2017 , 37 , 43. [ Google Scholar ] [ CrossRef ]
- Uwizeye, A.; de Boer, I.J.M.; Opio, C.I.; Schulte, R.P.O.; Falcucci, A.; Tempio, G.; Teillard, F.; Casu, F.; Rulli, M.; Galloway, J.N.; et al. Nitrogen emissions along global livestock supply chains. Nat. Food 2020 , 1 , 437–446. [ Google Scholar ] [ CrossRef ]
- Godde, C.; Mason-D’croz, D.; Mayberry, D.; Thornton, P.; Herrero, M. Impacts of climate change on the livestock food supply chain; a review of the evidence. Glob. Food Secur. 2021 , 28 , 100488. [ Google Scholar ] [ CrossRef ] [ PubMed ]
- Tzanidakis, C.; Tzamaloukas, O.; Simitzis, P.; Panagakis, P. Precision Livestock Farming Applications (PLF) for Grazing Animals. Agriculture 2023 , 13 , 288. [ Google Scholar ] [ CrossRef ]
- Ito, S.; Kawaguchi, N.; Bosch, J.; Aguilar-Vega, C.; Sánchez-Vizcaíno, J.M. What can we learn from the five-year African swine fever epidemic in Asia? Front. Vet. Sci. 2023 , 10 , 1273417. [ Google Scholar ] [ CrossRef ] [ PubMed ]
- Benami, E.; Jin, Z.; Carter, M.R.; Ghosh, A.; Hijmans, R.J.; Hobbs, A.; Kenduiywo, B.; Lobell, D.B. Uniting remote sensing, crop modelling and economics for agricultural risk management. Nat. Rev. Earth Environ. 2021 , 2 , 140–159. [ Google Scholar ] [ CrossRef ]
- Du, X.; Ifft, J.; Lu, L.; Zilberman, D. Marketing Contracts and Crop Insurance. Am. J. Agric. Econ. 2015 , 97 , 1360–1370. [ Google Scholar ] [ CrossRef ]
- Hill, R.V.; Kumar, N.; Magnan, N.; Makhija, S.; de Nicola, F.; Spielman, D.J.; Ward, P.S. Ex ante and ex post effects of hybrid index insurance in Bangladesh. J. Dev. Econ. 2019 , 136 , 1–17. [ Google Scholar ] [ CrossRef ]
- Boyd, M.; Pai, J.; Porth, L. Livestock mortality insurance: Development and challenges. Agric. Financ. Rev. 2013 , 73 , 233–244. [ Google Scholar ] [ CrossRef ]
- Kurdyś-Kujawska, A.; Sompolska-Rzechuła, A.; Pawłowska-Tyszko, J.; Soliwoda, M. Crop Insurance, Land Productivity and the Environment: A Way forward to a Better Understanding. Agriculture 2021 , 11 , 1108. [ Google Scholar ] [ CrossRef ]
- Cole, S.A.; Xiong, W. Agricultural Insurance and Economic Development. Annu. Rev. Econ. 2017 , 9 , 235–262. [ Google Scholar ] [ CrossRef ]
- Ding, Y.; Sun, C. Does agricultural insurance promote primary industry production? Evidence from a quasi-experiment in China. Geneva Pap. Risk Insur.-Issues Pract. 2022 , 47 , 434–459. [ Google Scholar ] [ CrossRef ]
- Aravani, V.P.; Sun, H.; Yang, Z.; Liu, G.; Wang, W.; Anagnostopoulos, G.; Syriopoulos, G.; Charisiou, N.D.; Goula, M.A.; Kornaros, M.; et al. Agricultural and livestock sector’s residues in Greece & China: Comparative qualitative and quantitative characterization for assessing their potential for biogas production. Renew. Sustain. Energy Rev. 2022 , 154 , 111821. [ Google Scholar ] [ CrossRef ]
- Dumont, B.; Groot, J.C.J.; Tichit, M. Review: Make ruminants green again—How can sustainable intensification and agroecology converge for a better future? Animal 2018 , 12 , s210–s219. [ Google Scholar ] [ CrossRef ]
- Li, G. The green productivity revolution of agriculture in China from 1978 to 2008. China Econ. Q. 2014 , 13 , 537–558. [ Google Scholar ] [ CrossRef ]
- Holm-Nielsen, J.; Al Seadi, T.; Oleskowicz-Popiel, P. The future of anaerobic digestion and biogas utilization. Bioresour. Technol. 2009 , 100 , 5478–5484. [ Google Scholar ] [ CrossRef ]
- Liu, Y.; Feng, C. What drives the fluctuations of “green” productivity in China’s agricultural sector? A weighted Russell directional distance approach. Resour. Conserv. Recycl. 2019 , 147 , 201–213. [ Google Scholar ] [ CrossRef ]
- Fu, J.; Hu, J.; Cao, X. Different sources of FDI, environmental regulation and green total factor productivity. J. Int. Trade 2018 , 7 , 134–148. [ Google Scholar ] [ CrossRef ]
- Yan, L.; Meng, H.; Liu, J.; Deng, Y. Discussion on green agro-ecological capitalisation operations. World Surv. Res. 2009 , 8 , 11–14. [ Google Scholar ] [ CrossRef ]
- King, M.; Singh, A.P. Understanding farmers’ valuation of agricultural insurance: Evidence from Vietnam. Food Policy 2020 , 94 , 101861. [ Google Scholar ] [ CrossRef ]
- Claassen, R.; Langpap, C.; Wu, J. Impacts of Federal Crop Insurance on Land Use and Environmental Quality. Am. J. Agric. Econ. 2017 , 99 , 592–613. [ Google Scholar ] [ CrossRef ]
- Müller, B.; Johnson, L.; Kreuer, D. Maladaptive outcomes of climate insurance in agriculture. Glob. Environ. Change 2017 , 46 , 23–33. [ Google Scholar ] [ CrossRef ]
- Niu, Z.; Yi, F.; Chen, C. Agricultural Insurance and Agricultural Fertilizer Non-Point Source Pollution: Evidence from China’s Policy-Based Agricultural Insurance Pilot. Sustainability 2022 , 14 , 2800. [ Google Scholar ] [ CrossRef ]
- Ali, W.; Abdulai, A.; Mishra, A.K. Recent Advances in the Analyses of Demand for Agricultural Insurance in Developing and Emerging Countries. Annu. Rev. Resour. Econ. 2020 , 12 , 411–430. [ Google Scholar ] [ CrossRef ]
- Carriker, G.L.; Williams, J.R.; Barnaby, G.A.; Black, J.R. Yield and Income Risk Reduction under Alternative Crop Insurance and Disaster Assistance Designs. West. J. Agric. Econ. 1991 , 16 , 238–250. [ Google Scholar ]
- Birthal, P.S.; Hazrana, J.; Negi, D.S.; Mishra, A.K. Assessing benefits of crop insurance vis-a-vis irrigation in Indian agriculture. Food Policy 2022 , 112 , 102348. [ Google Scholar ] [ CrossRef ]
- Zhao, Y.F.; Chai, Z.; Delgado, M.S.; Preckel, P.V. An empirical analysis of the effect of crop insurance on farmers’ income: Results from Inner Mongolia in China. China Agric. Econ. Rev. 2016 , 8 , 299313. Available online: https://www.emerald.com/insight/content/doi/10.1108/CAER-05-2014-0045/full/html (accessed on 23 June 2024). [ CrossRef ]
- Budhathoki, N.K.; Lassa, J.A.; Pun, S.; Zander, K.K. Farmers’ interest and willingness-to-pay for index-based crop insurance in the lowlands of Nepal. Land Use Policy 2019 , 85 , 1–10. [ Google Scholar ] [ CrossRef ]
- Biglari, T.; Maleksaeidi, H.; Eskandari, F.; Jalali, M. Livestock insurance as a mechanism for household resilience of livestock herders to climate change: Evidence from Iran. Land Use Policy 2019 , 87 , 104043. [ Google Scholar ] [ CrossRef ]
- Rao, X.; Zhang, Y. Livestock insurance, moral hazard, and farmers’ decisions: A field experiment among hog farms in China. Geneva Pap. Risk Insur.-Issues Pract. 2020 , 45 , 134–156. [ Google Scholar ] [ CrossRef ]
- Zhang, Y.; Cao, Y.; Wang, H.H. Cheating? The Case of Producers’ Under-Reporting Behavior in Hog Insurance in China. Can. J. Agric. Econ./Rev. Can. D’agroeconomie 2018 , 66 , 489–510. [ Google Scholar ] [ CrossRef ]
- Matsuda, A.; Takahashi, K.; Ikegami, M. Direct and indirect impact of index-based livestock insurance in Southern Ethiopia. Geneva Pap. Risk Insur.-Issues Pract. 2019 , 44 , 481–502. [ Google Scholar ] [ CrossRef ]
- Amare, A.; Simane, B.; Nyangaga, J.; Defisa, A.; Hamza, D.; Gurmessa, B. Index-based livestock insurance to manage climate risks in Borena zone of southern Oromia, Ethiopia. Clim. Risk Manag. 2019 , 25 , 100191. [ Google Scholar ] [ CrossRef ]
- Fang, L.; Hu, R.; Mao, H.; Chen, S. How crop insurance influences agricultural green total factor productivity: Evidence from Chinese farmers. J. Clean. Prod. 2021 , 321 , 128977. [ Google Scholar ] [ CrossRef ]
- Wang, L.; Chang, Q.; Kong, R. Regional differences and convergence of green total factor productivity in pig breeding: Evidence from China. Front. Environ. Sci. 2023 , 11 , 1162502. [ Google Scholar ] [ CrossRef ]
- Geng, N.; Liu, Z.; Wang, X.; Meng, L.; Pan, J. Measurement of Green Total Factor Productivity and Its Spatial Convergence Test on the Pig-Breeding Industry in China. Sustainability 2022 , 14 , 13902. [ Google Scholar ] [ CrossRef ]
- Zhong, S.; Li, J.; Guo, X. Analysis on the green total factor productivity of pig breeding in China: Evidence from a meta-frontier approach. PLoS ONE 2022 , 17 , e0270549. [ Google Scholar ] [ CrossRef ]
- Long, Y.; Liu, L.; Yang, B. Different types of environmental concerns and heterogeneous influence on green total factor productivity: Evidence from Chinese provincial data. J. Clean. Prod. 2023 , 428 , 139295. [ Google Scholar ] [ CrossRef ]
- Li, G.; Jia, X.; Khan, A.A.; Khan, S.U.; Ali, M.A.S.; Luo, J. Does green finance promote agricultural green total factor productivity? Considering green credit, green investment, green securities, and carbon finance in China. Environ. Sci. Pollut. Res. 2022 , 30 , 36663–36679. [ Google Scholar ] [ CrossRef ] [ PubMed ]
- Xu, B.; Chen, W.; Zhang, G.; Wang, J.; Ping, W.; Luo, L.; Chen, J. How to achieve green growth in China’s agricultural sector. J. Clean. Prod. 2020 , 271 , 122770. [ Google Scholar ] [ CrossRef ]
- Song, Y.; Zhang, B.; Wang, J.; Kwek, K. The impact of climate change on China’s agricultural green total factor productivity. Technol. Forecast. Soc. Change 2022 , 185 , 122054. [ Google Scholar ] [ CrossRef ]
- Ahmed, N.; Hamid, Z.; Mahboob, F.; Rehman, K.U.; e Ali, M.S.; Senkus, P.; Wysokińska-Senkus, A.; Siemiński, P.; Skrzypek, A. Causal Linkage among Agricultural Insurance, Air Pollution, and Agricultural Green Total Factor Productivity in United States: Pairwise Granger Causality Approach. Agriculture 2022 , 12 , 1320. [ Google Scholar ] [ CrossRef ]
- Zhang, Z.; Mu, Y.; Hou, L. Does participation in agricultural insurance optimize factor allocation? An analysis of endogenous farmers’ insurance decision-making and its effect on production. Chin. Rural. Econ. 2018 , 10 , 53–70. [ Google Scholar ]
- Chang, H.-H.; Mishra, A.K. Chemical usage in production agriculture: Do crop insurance and off-farm work play a part? J. Environ. Manag. 2012 , 105 , 76–82. [ Google Scholar ] [ CrossRef ] [ PubMed ]
- He, J.; Zheng, X.; Rejesus, R.M.; Yorobe, J.M. Moral hazard and adverse selection effects of cost-of-production crop insurance: Evidence from the Philippines. Australian, J. Agric. Resour. Econ. 2019 , 63 , 166–197. [ Google Scholar ] [ CrossRef ]
- Sibiko, K.W.; Qaim, M. Weather index insurance, agricultural input use, and crop productivity in Kenya. Food Secur. 2020 , 12 , 151–167. [ Google Scholar ] [ CrossRef ]
- Capitanio, F.; Adinolfi, F.; Santeramo, F.G. Crop Insurance Subsidies and Environmental Externalities: Evidence from Southern Italy. Outlook Agric. 2014 , 43 , 253–258. [ Google Scholar ] [ CrossRef ]
- Bertram-Huemmer, V.; Kraehnert, K. Does Index Insurance Help Households Recover from Disaster? Evidence from IBLI Mongolia. Am. J. Agric. Econ. 2018 , 100 , 145–171. [ Google Scholar ] [ CrossRef ]
- John, F.; Toth, R.; Frank, K.; Groeneveld, J.; Müller, B. Ecological Vulnerability Through Insurance? Potential Unintended Consequences of Livestock Drought Insurance. Ecol. Econ. 2019 , 157 , 357–368. [ Google Scholar ] [ CrossRef ]
- Meng, X.; Zhou, H.; Du, L.; Shen, G. The change of agricultural environmental technology efficiency and green total factor productivity growth in China: Re-examination based on the perspective of combination of planting and breeding. Issues Agric. Econ. 2019 , 6 , 9–22. [ Google Scholar ] [ CrossRef ]
- Zhong, F.; Ning, M.; Xing, L.; Miao, Q. Does participation in agricultural insurance optimize factor allocation? An analysis of endogenous farmers’ insurance decision-making and its effect on production. China Econ. Q. 2007 , 10 , 291–308. [ Google Scholar ]
- Vigani, M.; Kathage, J. To Risk or Not to Risk? Risk Management and Farm Productivity. Am. J. Agric. Econ. 2019 , 101 , 1432–1454. [ Google Scholar ] [ CrossRef ]
- Wu, J.; Adams, R.M. Production Risk, Acreage Decisions and Implications for Revenue Insurance Programs. Can. J. Agric. Econ./Rev. Can. D’agroeconomie 2001 , 49 , 19–35. [ Google Scholar ] [ CrossRef ]
- Zhang, X.; Zhao, Y. A theoretical and empirical study on the impact of dairy cattle insurance program on the scale of dairy farming. Insur. Stud. 2017 , 2 , 40–49. [ Google Scholar ] [ CrossRef ]
- Kafle, B. Diffusion of Uncertified Organic Vegetable Farming among Small Farmers in Chitwan District, Nepal: A Case of Phoolbari Village. Int. J. Agric. Res. Rev. 2011 , 1 , 157–163. [ Google Scholar ]
- Tan, Y.; Lu, Q.; Zhang, S. Can contract farming promote farmers’ green production transition. J. Agrotech. Econ. 2022 , 7 , 16–33. [ Google Scholar ] [ CrossRef ]
- Ifft, J.; Kuethe, T.H.; Lyons, G.; Schultz, A.; Zhu, J.Y. Crop insurance’s impact on commercial bank loan volumes: Theory and evidence. Appl. Econ. Perspect. Policy 2024 , 46 , 318–337. [ Google Scholar ] [ CrossRef ]
- Rao, J.; Zhang, Y. An analysis of pollution control and utilization of manure of pig raising farms of different scales and types in China: Take LP county of Hebei Province as an example. Issues Agric. Econ. 2018 , 4 , 121–130. [ Google Scholar ] [ CrossRef ]
- Sueyoshi, T.; Zhang, R.; Qu, J.; Li, A. New concepts for environment-health measurement by data envelopment analysis and an application in China. J. Clean. Prod. 2021 , 312 , 127468. [ Google Scholar ] [ CrossRef ]
- Ma, J.; Cu, H.; Wu, B. Analysis of the Effect and Mechanism of Policy-oriented Agricultural Insurance’s Promotion on Farmers’ Income—A Quasi-natural Experimental Research on Progressive Pilots, E. Insur. Stud. 2020 , 2 , 3–18. [ Google Scholar ] [ CrossRef ]
- Tran, P.M.; Nguyen, T.; Nguyen, H.-D.; Thinh, N.A.; Lam, N.D.; Huyen, N.T.; Khuc, V.Q. Improving Green Literacy and Environmental Culture Associated with Youth Participation in the Circular Economy: A Case Study of Vietnam. Urban Sci. 2024 , 8 , 63. [ Google Scholar ] [ CrossRef ]
Click here to enlarge figure
Time | Centre |
---|---|
Policy Establishment Phase (2007–2010) | (2007) “Opinions on Promoting the Work of Policy-supported Agricultural Insurance”: Initially proposed the establishment of agricultural insurance and introduced a pioneering initiative for hog insurance, with a focus on bolstering the development of agricultural insurance. (2007) “Urgent Notice on Establishing the Hog Insurance System to Promote the Development of farming Production”: Explicitly defined the coverage responsibilities, insurance amounts, rates, and subsidy ratios for insuring breeding sows by governmental entities at various levels. (2008) “Notice on Matters Related to the Pilot Work of Subsidizing the Premium of Fattened Hog Insurance”: Selected Hunan, Sichuan, and Jilin provinces as pilot provinces to carry out the subsidy work for fattened hog insurance premiums. |
Policy Promotion Phase (2011–2015) | (2012) “Guiding Opinions on Accelerating the Development of Agricultural Insurance”: Explicitly defined the responsibilities of governments at all levels and promoted the expansion of the coverage of hog insurance. (2013) “Administrative Measures for Subsidies on Agricultural Insurance Premiums”: Clearly defined the operational procedures and subsidy standards for hog insurance, standardized operational procedures, and ensured the interests of insurance companies and farmers. (2013) “Notice on Matters Related to the Central Financial Subsidies for Agricultural Insurance Premiums in 2013”: Expanded the pilot area for premium subsidies of fattened hog insurance and increased the government’s premium subsidy ratio. |
Policy Adjustment Phase (2016–2020) | (2016) “Opinions on Deepening the Reform of Agricultural Insurance”: Proposed deepening the reform of hog insurance, optimizing the design of insurance products, and improving the level of protection. (2019) “Notice on Supporting the Stability of Hog Production and Ensuring Market Supply”: Increased the insurance amount, raising the insurance amount for breeding sows from CNY 1000–1200 to 1500, and for fattened pigs, from CNY 500–600 to CNY 800. |
Policy Enhancement Phase (2021–present) | (2021) “Opinions on Promoting the Continuous and Healthy Development of the Farming Industry”: Further advanced the livestock insurance, stabilized the insurance amount, dynamically adjusted the amount according to changes in production costs, enhanced the attractiveness of insurance products, and achieved the goal that all farms willing to be insured are insured. (2023) “Guiding Opinions on Financial Support for Comprehensively Promoting Rural Revitalization and Accelerating the Construction of a Strong Agricultural Country”: Improved the level of insurance protection and explored the development of income insurance products for livestock such as pigs and dairy cows. |
Indicator | Calculation Formula | |
---|---|---|
Input elements | Capital inputs (CNY) | Total cost of piglets, water, fuel and power, death loss, technical services, tools and materials, repair and maintenance, taxes, depreciation, premiums, management, finances, sales, and other expenses |
Feed inputs (CNY) | Total cost of concentrate feed, coarse fodder, and feed processing | |
Labor inputs (CNY) | Sum of discount for domestic labor and cost of hired labor | |
Medical and epidemic prevention inputs (CNY) | Total cost of medical care and vaccination | |
Desirable outputs | Net weight of hogs (kg) | Difference between slaughter weight and piglet weight |
Undesirable outputs | TP (t) | Elemental emission coefficient × finisher weight/reference weight × days of feeding |
TN (t) | ||
COD (t) |
Variable | Definition | Obs. | Mean | Std. Dev. | Min. | Max. |
---|---|---|---|---|---|---|
Green total factor productivity (GTFP) | Measured by SBM-DDF | 306 | 0.8798 | 0.0860 | 0.6181 | 1.0000 |
Hog insurance policy implementation (DID) | Implemented = 1; unimplemented = 0 | 306 | 0.6144 | 0.4875 | 0.0000 | 1.0000 |
GDP per capita (RGDP) | GDP/total population | 306 | 4.0060 | 2.3232 | 0.5201 | 13.8028 |
Agricultural fiscal expenditure (AFE) | Government fiscal expenditure on agriculture/total fiscal expenditure | 306 | 0.1042 | 0.0283 | 0.0218 | 0.1897 |
Government intervention intensity (GI) | Government fiscal expenditure on agriculture/GDP of primary industry | 306 | 0.1208 | 0.0544 | 0.0088 | 0.2687 |
Research and development expenditure (RD) | Fiscal expenditure on science and technology/GDP | 306 | 0.0035 | 0.0020 | 0.0003 | 0.0108 |
Environmental regulation (ER) | Investment in environmental pollution control/GDP | 306 | 0.0059 | 0.0031 | 0.0008 | 0.0162 |
Level of agricultural financial development (AFD) | Agricultural loans/GDP of agriculture, forestry, animal husbandry and fisheries | 306 | 2.0132 | 1.9803 | 0.2148 | 13.4106 |
Level of agricultural openness to the outside world (Aopen) | Export of agricultural products by region/GDP of agriculture, forestry, animal husbandry, and fisheries | 306 | 0.0401 | 0.0394 | 0.0014 | 0.1557 |
Transport accessibility (Trans) | Mileage of railways, inland waterways and roads | 306 | 1.0360 | 0.4530 | 0.1647 | 2.3167 |
Average years of schooling of people living in rural areas (Edu) | (number of people not attending school × 0 + number of people in primary school × 6 + number of people in junior high school × 9 + number of people in senior high school × 12 + number of people in tertiary education and above × 16)/population over 6 years old | 306 | 8.6810 | 0.7507 | 6.3778 | 10.1894 |
Livestock production price index (PPI) | Livestock production price index | 306 | 106.7288 | 16.5354 | 71.4000 | 157.4000 |
Farmland carrying capacity (FCC) | Cultivated land area in each province/national total cultivated land area | 306 | 0.0419 | 0.0222 | 0.0101 | 0.1345 |
Feed resources (FR) | Corn production by province/national corn production | 306 | 0.0427 | 0.0439 | 0.0003 | 0.1615 |
Scale of farming (Scale) | Total annual stock of hogs | 306 | 5451.5800 | 2630.0880 | 1183.3500 | 12849.7900 |
Planting–breeding structure (PL) | Number of farming/area of arable land for food crops | 306 | 0.4611 | 0.2295 | 0.0600 | 1.1700 |
Mechanical power of farming (MP) | Power of farming machinery | 306 | 82.0207 | 45.9551 | 12.7900 | 277.8300 |
Explained Variable: GTFP | |||
---|---|---|---|
(1) | (2) | (3) | |
DID | 0.019 *** | 0.017 ** | |
(0.007) | (0.007) | ||
pre8 | −0.003 | ||
(0.022) | |||
pre7 | 0.004 | ||
(0.019) | |||
pre6 | 0.029 * | ||
(0.016) | |||
pre5 | 0.012 | ||
(0.017) | |||
pre4 | 0.005 | ||
(0.013) | |||
pre3 | 0.016 | ||
(0.010) | |||
pre2 | 0.007 | ||
(0.010) | |||
current | 0.006 | ||
(0.009) | |||
post1 | 0.038 *** | ||
(0.012) | |||
post2 | 0.037 *** | ||
(0.012) | |||
post3 | 0.044 *** | ||
(0.012) | |||
post4 | 0.050 *** | ||
(0.013) | |||
post5 | 0.050 *** | ||
(0.014) | |||
post6 | 0.047 *** | ||
(0.015) | |||
post7 | 0.047 ** | ||
(0.020) | |||
_cons | 0.618 *** | 0.701 ** | 0.759 *** |
(0.004) | (0.303) | (0.289) | |
Control variable | NO | YES | YES |
Time-fixed effects | YES | YES | YES |
Province-fixed effects | YES | YES | YES |
N | 306 | 306 | 306 |
R2 | 0.690 | 0.706 | 0.744 |
Explained Variable: GTFP | |||
---|---|---|---|
(1) | (2) | (3) | |
DID_ahead | −0.001 | ||
(0.007) | |||
DID_delay | 0.020 *** | ||
(0.007) | |||
DID | 0.019 *** | ||
(0.007) | |||
_cons | 0.712 ** | 0.781 *** | 0.783 ** |
(0.308) | (0.300) | (0.319) | |
Control variable | YES | YES | YES |
Time-fixed effects | YES | YES | YES |
Province-fixed effects | YES | YES | YES |
N | 306 | 306 | 288 |
R2 | 0.699 | 0.708 | 0.704 |
(1) Scale | (2) PL | (3) MP | |
---|---|---|---|
DID | 0.061 *** | 0.023 *** | 0.176 *** |
(0.022) | (0.008) | (0.043) | |
_cons | 4.501 *** | −0.354 | 4.505 ** |
(1.091) | (0.364) | (2.001) | |
Control variable | YES | YES | YES |
Time-fixed effects | YES | YES | YES |
Province-fixed effects | YES | YES | YES |
N | 306 | 306 | 306 |
R2 | 0.967 | 0.965 | 0.913 |
Explained Variable: GTFP | ||||||
---|---|---|---|---|---|---|
Low-GDP | High-GDP | Free-Range | Small-Scale | Medium-Scale | Large-Scale | |
(1) | (2) | (3) | (4) | (5) | (6) | |
DID | 0.023 ** | 0.029 ** | 0.016 *** | 0.024 *** | 0.011 ** | 0.007 |
(0.009) | (0.014) | (0.006) | (0.006) | (0.005) | (0.006) | |
_cons | 0.931 ** | 0.431 | 0.400 * | 0.675 *** | 0.865 *** | 0.848 *** |
(0.394) | (0.818) | (0.209) | (0.247) | (0.245) | (0.266) | |
Control variable | YES | YES | YES | YES | YES | YES |
Time-fixed effects | YES | YES | YES | YES | YES | YES |
Province-fixed effects | YES | YES | YES | YES | YES | YES |
N | 199 | 102 | 306 | 306 | 306 | 306 |
R2 | 0.701 | 0.794 | 0.842 | 0.842 | 0.848 | 0.843 |
The statements, opinions and data contained in all publications are solely those of the individual author(s) and contributor(s) and not of MDPI and/or the editor(s). MDPI and/or the editor(s) disclaim responsibility for any injury to people or property resulting from any ideas, methods, instructions or products referred to in the content. |
Share and Cite
Wu, D.; He, S.; Qin, L.; Feng, J.; Gao, Y. Role of Policy-Supported Hog Insurance in Promoting Green Total Factor Productivity: The Case of China during 2005–2021. Agriculture 2024 , 14 , 1051. https://doi.org/10.3390/agriculture14071051
Wu D, He S, Qin L, Feng J, Gao Y. Role of Policy-Supported Hog Insurance in Promoting Green Total Factor Productivity: The Case of China during 2005–2021. Agriculture . 2024; 14(7):1051. https://doi.org/10.3390/agriculture14071051
Wu, Dongli, Shan He, Lingui Qin, Jingyue Feng, and Yu Gao. 2024. "Role of Policy-Supported Hog Insurance in Promoting Green Total Factor Productivity: The Case of China during 2005–2021" Agriculture 14, no. 7: 1051. https://doi.org/10.3390/agriculture14071051
Article Metrics
Article access statistics, further information, mdpi initiatives, follow mdpi.
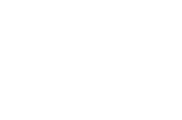
Subscribe to receive issue release notifications and newsletters from MDPI journals

IMAGES
VIDEO
COMMENTS
Examples. A research hypothesis, in its plural form "hypotheses," is a specific, testable prediction about the anticipated results of a study, established at its outset. It is a key component of the scientific method. Hypotheses connect theory to data and guide the research process towards expanding scientific understanding.
Examples of research questions for directional hypothesis. In the world of research and statistical analysis, hypotheses play a crucial role in formulating and testing scientific claims. Understanding the differences between directional and non-directional hypothesis is essential for designing sound experiments and drawing accurate conclusions.
The analysis of a nondirectional hypothesis is called a two-tailed test of significance. In a one-tailed test of significance, the hypothesis is directional, and extreme statistical values that occur in a single tail of the curve are of interest. The other two options are incorrect.
A non-directional hypothesis, also known as a two-tailed hypothesis, ... When researchers adopt a data-driven or exploratory approach to analysis, non-directional hypotheses are preferred. Instead of testing specific predictions, the aim is to explore the data, identify patterns, and generate hypotheses based on the observed relationships or ...
assume a hypothesis is directional when in fact it is non-directional. For example, everyone knows the more you revise, the better you do in exams but a hypothesis may say 'There is a difference in the exam results between those who revise a lot and those who do not revise' and this is, of course, a non-directional hypothesis. Extension task
A Null Hypothesis is denoted as an H0. This is the type of hypothesis that the researcher tries to invalidate. Some of the examples of null hypotheses are: - Hyperactivity is not associated with eating sugar. - All roses have an equal amount of petals. - A person's preference for a dress is not linked to its color.
The directional hypothesis can also state a negative correlation, e.g. the higher the number of face-book friends, the lower the life satisfaction score ". Non-directional hypothesis: A non-directional (or two tailed hypothesis) simply states that there will be a difference between the two groups/conditions but does not say which will be ...
Advantages of Non-Directional Hypothesis: Flexibility: Non-directional hypotheses provide flexibility in exploring relationships between variables without preconceived notions about the direction of the effect. Open to unexpected findings: By not specifying the direction, researchers remain open to unexpected results or alternative explanations that may emerge during the analysis.
This null hypothesis can be written as: H0: X¯ = μ H 0: X ¯ = μ. For most of this textbook, the null hypothesis is that the means of the two groups are similar. Much later, the null hypothesis will be that there is no relationship between the two groups. Either way, remember that a null hypothesis is always saying that nothing is different.
A non-directional hypothesis, also known as a two-tailed hypothesis, is a type of hypothesis that does not specify the direction of the predicted relationship between variables. This means that the researcher is not making a specific prediction and is open to the possibility of any outcome. In this article, we will delve into the fundamentals ...
5.2 - Writing Hypotheses. The first step in conducting a hypothesis test is to write the hypothesis statements that are going to be tested. For each test you will have a null hypothesis ( H 0) and an alternative hypothesis ( H a ). When writing hypotheses there are three things that we need to know: (1) the parameter that we are testing (2) the ...
Three Different Hypotheses: (1) Directional Hypothesis: states that the IV will have an effect on the DV and what that effect will be (the direction of results). For example, eating smarties will significantly improve an individual's dancing ability. When writing a directional hypothesis, it is important that you state exactly how the IV will ...
A research question is what a study aims to answer after data analysis and interpretation. The answer is written in length in the discussion section of the paper. ... Non-directional hypothesis: Associative hypothesis: Causal hypothesis: Null hypothesis: Alternative hypothesis: Working hypothesis: Statistical hypothesis: Logical hypothesis ...
To test the significance of a non-directional hypothesis, we have to consider the possibility that the sample could be extreme at either tail of the comparison distribution. We call this a two-tailed test. Figure 1. showing a 2-tail test for non-directional hypothesis for z for area C is the critical rejection region.
It seeks to explore and understand a particular aspect of the research subject. In contrast, a research hypothesis is a specific statement or prediction that suggests an expected relationship between variables. It is formulated based on existing knowledge or theories and guides the research design and data analysis. 7.
Definition: Hypothesis is an educated guess or proposed explanation for a phenomenon, based on some initial observations or data. It is a tentative statement that can be tested and potentially proven or disproven through further investigation and experimentation. Hypothesis is often used in scientific research to guide the design of experiments ...
"An increase in exercise frequency is associated with changes in weight." In this non-directional hypothesis, the statement suggests that a relationship exists between exercise frequency and weight changes but doesn't specify whether increased exercise will lead to weight loss or weight gain. It leaves the direction of the relationship open for empirical investigation and data analysis.
A Level Psychology Topic Quiz - Research Methods. Quizzes & Activities. A non-directional hypothesis is a two-tailed hypothesis that does not predict the direction of the difference or relationship (e.g. girls and boys are different in terms of helpfulness).
Non-directional hypotheses are also known as two-tailed hypotheses. The term 'two-tailed' comes from the fact that the statistical test used to evaluate the hypothesis is based on the assumption that the difference or relationship could occur in either direction, resulting in two 'tails' in the probability distribution.
The research hypothesis is needed for a sound and well-developed research study. The research hypothesis contributes to the solution of the research problem. Types of research hypotheses include inductive and deductive, directional and non-directional, and null and alternative hypotheses. Rejecting the null hypothesis and accepting the ...
Directional vs Non-Directional vs Null Hypotheses. A directional hypothesis is generally contrasted to a non-directional hypothesis.Here's how they compare: Directional hypothesis: A directional hypothesis provides a perspective of the expected relationship between variables, predicting the direction of that relationship (either positive, negative, or a specific difference).
Understanding Non-Directional Hypotheses. A hypothesis is a statement that outlines a testable prediction about the relationship between variables in a research study. Hypotheses are fundamental to the scientific method, guiding the design of experiments and the analysis of data. While hypotheses can take various forms, including directional hypotheses, non-directional hypotheses, or null ...
A statistical hypothesis is an assumption about a population parameter.For example, we may assume that the mean height of a male in the U.S. is 70 inches. The assumption about the height is the statistical hypothesis and the true mean height of a male in the U.S. is the population parameter.. To test whether a statistical hypothesis about a population parameter is true, we obtain a random ...
further analysis as potential foundation species. For example, knowing that eastern hemlock (Tsuga cana-densis) is afoundation species in eastern North American forest (Ellison, 2014), we have used codispersion analysis (Cuevas et al., 2013) to explore pairwise species co-occurrences (Buckley et al., 2016a), relation-
Hog insurance and rural environmental protection are complementary to each other. Studying the environmental effects of hog insurance is imperative for safeguarding food safety and promoting the long-term development of the agricultural insurance industry. Informed by the risk management theory and sustainable development theory, this paper constructs a theoretical framework for the impact of ...