Cognitive Flexibility: The Multitool for Problem-Solving
By GGI Insights | April 8, 2024

Table of contents

In this article, we will explore the various aspects of cognitive flexibility and how it can be harnessed as a multitool for problem-solving.
Neurological Foundations
Cognitive flexibility is deeply rooted in the intricate workings of the brain. Neuroplasticity, the brain's ability to reorganize itself by forming new neural connections, plays a crucial role in the development and enhancement of cognitive flexibility. By constantly rewiring and adapting, the brain becomes more nimble and adaptable in tackling complex tasks and finding solutions. Lifelong learning stimulates this neuroplasticity, fostering continued growth and adaptability of the brain. Delayed gratification is also an aspect of this adaptability, as it requires the brain to prioritize long-term rewards over immediate pleasures, strengthening cognitive control and decision-making abilities.
One key aspect of neuroplasticity is the brain's ability to learn from failure . Each time we encounter failure, our brain restructures itself, learning from the experience and adapting for future encounters. This neuroplastic response to failure is essential for the development of cognitive flexibility. Self-reflection in these moments is crucial as it allows individuals to critically analyze their experiences, gaining deeper insights into their cognitive processes and patterns.
Neuroplasticity is a captivating phenomenon that enables the brain to reshape itself in response to experiences and learning. It is not a static and inflexible organ, but rather a dynamic and adaptable structure that can undergo growth and change throughout our lives. This extraordinary capacity of the brain to rewire itself is what empowers us to acquire new skills, embrace new environments, and overcome challenges with a growth mindset .
Plasticity and Pathways
The brain's plasticity allows for the creation of new pathways, enabling information to flow more freely and efficiently between different regions. This enhanced connectivity fosters cognitive flexibility by facilitating the integration of diverse perspectives and promoting the exploration of alternative solutions. It empowers individuals to break free from ingrained thought patterns and engage in more adaptive and flexible thinking. A s part of this dynamic process, a feedback loop is created, where the outcomes of our actions and decisions feed back into our brain, influencing future responses and enhancing our adaptability. Self-awareness is a key factor in this loop, as it helps individuals recognize and understand the impact of their thoughts and actions on their cognitive development.
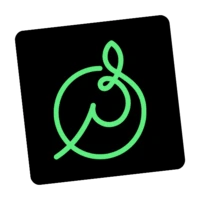
Unlock your business's full potential with gardenpatch. Their team of strategists specializes in transforming your operations for maximum efficiency and growth. Click here to drive growth through efficient operations!
Imagine the brain as a vast network of interconnected roads, with each road representing a neural pathway. In a rigid and inflexible brain, these roads would be narrow and limited, restricting the flow of information. However, in a brain with high plasticity, these roads are wide and well-connected, allowing for a seamless exchange of ideas and information. This feedback loop , constantly reinforced through new experiences and learning, is a fundamental aspect of cognitive flexibility, allowing for continual adaptation and growth.
As we encounter new experiences and challenges, the brain starts to forge new pathways, like building new roads to connect previously isolated regions. This process not only strengthens existing connections but also creates new ones, expanding the brain's capacity for cognitive flexibility. It's like opening up new avenues of exploration and possibilities within our minds.
Neuroplasticity is not limited to a specific age or stage of life. While the brain's plasticity is most pronounced during childhood, it continues to be present throughout adulthood. This means that we have the potential to enhance our cognitive flexibility at any age by actively engaging in activities that stimulate the brain, such as learning new skills, solving puzzles, or engaging in creative endeavors.
By embracing the concept of neuroplasticity and understanding its role in cognitive flexibility, we can appreciate the remarkable adaptability of the human brain. It is a testament to our brain's capacity for growth and change, offering us endless opportunities to expand our thinking, challenge our assumptions, and approach problems from different angles.
Tools for Enhancement
There are various strategies and techniques that can be employed to enhance cognitive flexibility and tap into its problem-solving potential.
Cognitive flexibility, the ability to adapt and shift thinking in response to changing circumstances, is a valuable skill that can be developed and improved over time. It allows individuals to approach problems from different angles, consider multiple perspectives, and generate creative solutions.
Mind Maps to Lateral Thinking
Mind maps, visual representations of ideas and concepts, are effective tools for stimulating cognitive flexibility. By visually connecting different ideas, mind maps encourage nonlinear thinking and facilitate the exploration of alternative connections and solutions.
When creating a mind map, individuals can start with a central idea and branch out to related concepts, allowing their thoughts to flow freely. This process encourages the brain to make new associations and connections, expanding cognitive flexibility. As the mind map grows, it becomes a visual representation of the individual's thought process, capturing the complexity and richness of their thinking.
Practicing lateral thinking, a deliberate effort to approach problems from unconventional angles, helps stretch cognitive flexibility and unlock innovative problem-solving strategies. This technique encourages individuals to break free from traditional thought patterns and explore new possibilities.
By intentionally challenging assumptions and exploring alternative perspectives, individuals can expand their cognitive flexibility and discover unique solutions to complex problems. Lateral thinking encourages individuals to ask "what if" questions, consider different scenarios, and explore uncharted territories of thought.
Lateral thinking can be practiced through various techniques, such as brainstorming, role-playing, and using provocative statements. These methods encourage individuals to think beyond the obvious and embrace ambiguity, fostering cognitive flexibility and enhancing problem-solving abilities.
Mind maps and lateral thinking are powerful tools that can enhance cognitive flexibility and unlock the problem-solving potential of individuals. By visually representing ideas and exploring unconventional approaches, these techniques stimulate creative thinking and encourage the exploration of alternative solutions. Incorporating these tools into daily practice can lead to improved cognitive flexibility and a broader range of problem-solving strategies.
Flexibility in Crisis
In times of crisis or uncertainty, the ability to adapt and think flexibly becomes even more crucial. Cognitive flexibility empowers individuals to navigate unforeseen challenges and find effective solutions. Utilizing positive self-talk can significantly influence this adaptability, reinforcing the individual's confidence and ability to handle challenging situations.
When faced with a crisis, it is often the individuals who can think outside the box and embrace flexibility that emerge as problem solvers. The power of cognitive flexibility lies in its ability to enable individuals to approach challenges with adaptability and creativity. By being open to new ideas and perspectives, individuals can navigate through the complexities of a crisis and find innovative solutions.
Adaptability Case Studies
Real-life examples demonstrate the power of cognitive flexibility in dire situations. From emergency response teams making quick decisions to individuals facing unexpected obstacles, adaptability is a defining characteristic of successful problem solvers. These case studies showcase the importance of embracing flexibility and highlight the positive outcomes that can be achieved by harnessing this multitool for problem-solving.
One notable case study involves an emergency response team during a natural disaster. As they faced rapidly changing circumstances and limited resources, their cognitive flexibility allowed them to quickly assess the situation and come up with creative solutions. By adapting their strategies and thinking outside the conventional approaches, they were able to save lives and minimize the impact of the disaster.
Another example of cognitive flexibility in action is seen in individuals facing unexpected obstacles. Whether it is a sudden change in their career path or a personal setback, those who possess cognitive flexibility are better equipped to adapt and find new opportunities. They are able to reframe their perspectives, explore alternative options, and embrace change as a catalyst for growth.
Cognitive flexibility serves as the multitool for problem-solving by enabling individuals to approach challenges with adaptability and creativity. Its neurological foundations in plasticity and pathways provide a solid framework for enhancing cognitive flexibility. By utilizing strategies such as mind maps and lateral thinking, individuals can further develop and hone their cognitive flexibility skills. In times of crisis, cognitive flexibility becomes even more vital, allowing for quick adaptation and effective problem-solving. Embracing cognitive flexibility as a valuable asset in problem-solving endeavors can lead to innovative solutions and positive outcomes.
Popular Insights:
Shop with purpose at impact mart your purchase empowers positive change. thanks for being the difference.

Customer Onboarding: Proven Techniques for Seamless Client Integration
Customer journey: optimizing interactions for lasting customer loyalty, email open rates: enhancing the readership of your marketing emails, revenue: the fundamental pillar of business viability and growth, marketing strategies: craft dynamic approaches for business expansion, startup school: accelerating your path to entrepreneurial success, ecommerce marketing strategy: data-driven tactics for enhanced loyalty, hubspot crm review: a comprehensive look into what makes hubspot great, hubspot growth suite: catalyzing your company growth with integration, hubspot service hub: taking superb customer service to the next level.
Click through the PLOS taxonomy to find articles in your field.
For more information about PLOS Subject Areas, click here .
Loading metrics
Open Access
Study Protocol
Study protocol: How does cognitive flexibility relate to other executive functions and learning in healthy young adults?
Roles Investigation, Methodology, Visualization, Writing – original draft, Writing – review & editing
* E-mail: [email protected]
Affiliation Nanyang Technological University, Singapore, Singapore

Roles Writing – original draft
Roles Investigation, Methodology, Writing – original draft
Roles Conceptualization, Funding acquisition, Methodology, Writing – review & editing
Affiliation National Institutes of Health, Bethesda, Maryland, United States of America
Affiliation University of Cambridge, Cambridge, United Kingdom
Roles Investigation, Methodology, Writing – original draft, Writing – review & editing
Roles Visualization, Writing – original draft
Affiliation National Institute of Education, Singapore, Singapore
Roles Writing – original draft, Writing – review & editing
Roles Conceptualization, Funding acquisition, Methodology, Software, Writing – review & editing
- [ ... ],
¶ The member list of the CLIC Phase 1 consortium can be found in the Appendix G in S1 File .
- [ view all ]
- [ view less ]
- Ke Tong,
- Yuan Ni Chan,
- Xiaoqin Cheng,
- Bobby Cheon,
- Michelle Ellefson,
- Restria Fauziana,
- Shengchuang Feng,
- Nastassja Fischer,
- Balázs Gulyás,
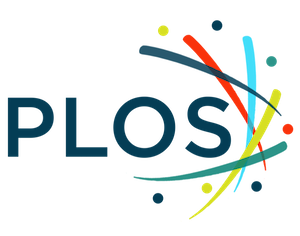
- Published: July 20, 2023
- https://doi.org/10.1371/journal.pone.0286208
- Reader Comments
Cognitive flexibility (CF) enables individuals to readily shift from one concept or mode of practice/thoughts to another in response to changes in the environment and feedback, making CF vital to optimise success in obtaining goals. However, how CF relates to other executive functions (e.g., working memory, response inhibition), mental abilities (e.g., creativity, literacy, numeracy, intelligence, structure learning), and social factors (e.g., multilingualism, tolerance of uncertainty, perceived social support, social decision-making) is less well understood. The current study aims to (1) establish the construct validity of CF in relation to other executive function skills and intelligence, and (2) elucidate specific relationships between CF, structure learning, creativity, career decision making and planning, and other life skills.
This study will recruit up to 400 healthy Singaporean young adults (age 18–30) to complete a wide range of cognitive tasks and social questionnaires/tasks. The richness of the task/questionnaire battery and within-participant administration enables us to use computational modelling and structural equation modelling to examine connections between the latent constructs of interest.
Significance and Impact
The current study is the first systematic investigation into the construct validity of CF and its interrelationship with other important cognitive skills such as learning and creativity, within an Asian context. The study will further explore the concept of CF as a non-unitary construct, a novel theoretical proposition in the field. The inclusion of a structure learning paradigm is intended to inform future development of a novel intervention paradigm to enhance CF. Finally, the results of the study will be useful for informing classroom pedagogy and the design of lifelong learning policies and curricula, as part of the wider remit of the Cambridge-NTU Centre for Lifelong Learning and Individualised Cognition (CLIC).
Citation: Tong K, Chan YN, Cheng X, Cheon B, Ellefson M, Fauziana R, et al. (2023) Study protocol: How does cognitive flexibility relate to other executive functions and learning in healthy young adults? PLoS ONE 18(7): e0286208. https://doi.org/10.1371/journal.pone.0286208
Editor: Avanti Dey, Public Library of Science, UNITED STATES
Received: May 3, 2023; Accepted: May 10, 2023; Published: July 20, 2023
This is an open access article, free of all copyright, and may be freely reproduced, distributed, transmitted, modified, built upon, or otherwise used by anyone for any lawful purpose. The work is made available under the Creative Commons CC0 public domain dedication.
Data Availability: No results are reported in the current protocol manuscript. Deidentified research data will be made publicly available when the study is completed and published.
Funding: Funding source: National Research Foundation (NRF), Singapore ( www.nrf.gov.sg ). Grant Recipient: Cambridge Centre for Advanced Research and Education in Singapore. The funders did not and will not have a role in study design, data collection and analysis, decision to publish, or preparation of the manuscript.
Competing interests: The authors have declared that no competing interests exist.
Introduction
As technology and globalisation are changing the nature of labour markets and increasing the demand for high skills levels, the need for individuals to be capable of learning new skills during their careers becomes increasingly pressing [ 1 , 2 ]. In the Singapore context, where this study will take place, Singapore’s SkillsFuture programme, promoted by the Ministries of Education, Manpower, and Trade & Industry, aptly recognises that societies need workers with the capacity for flexible behaviour. That is, the ability to adapt to change, problem-solve in new situations based on previous experience to achieve in jobs that are likely to emerge over the next few decades [ 3 ]. This individual capacity for cognitive flexibility is central to the modern digital age with its rapidly changing settings at home and work [ 4 , 5 ]. High cognitive flexibility is associated with improved wellbeing which will promote a flourishing society and will lead to improved social and workplace outcomes [ 6 , 7 ] as well as entrepreneurship and innovation [ 8 ].
As we recognise the need for flexible behaviour and transferable skills in our workforce, we need our education systems to equip citizens with the cognitive flexibility they need to develop these skills for the future [ 9 , 10 ]. However, there currently need to be more evidence-based training programmes that can effectively support and promote cognitive flexibility across the life course.
Cognitive flexibility
Cognitive Flexibility (CF) is a component of executive function (EF), along with working memory and inhibitory control [ 11 ]. Existing studies encourage the view that there is something quite specific about the flexibility construct, making it separable from the other main EF components. The evidence for dissociations of CF and other executive functions comes from psychometric studies, genetic and neuroscientific investigations, and clinical findings (for recent reviews, see [ 12 , 13 ]).
From the psychometric perspective, there is a general, well-known problem in measuring components of EF, which is that of "task impurity". Most cognitive tasks involve non-executive functions and commonly involve different loadings on its three prime components. Virtually all tasks have a working memory load, and most involve a degree of response inhibition. Importantly, Friedman and Miyake found that working memory updating has good relationships with measures of IQ (relevant to the general factor, g ), but CF did not correlate highly with IQ measures [ 14 ]. Their recent review concluded, "all the studies we reviewed found evidence that shifting was separable from updating or working memory in older children and adults" [ 11 ]. They found that when inconsistencies did arise, they tended to involve the inhibition factor. Regarding relationships of shifting with response inhibition, Blackwell et al. found that children who were better at card sorting were worse at response inhibition [ 15 ]. A similar dissociation has been found for adults raised in stressful environments [ 16 ]. Therefore, although EF has a certain unity, there is also diversity, especially concerning CF.
Given the issue of "task impurity", to effectively measure the psychological construct of cognitive flexibility (as separate from other executive functions), it is necessary to administer multiple CF tests that load on the component of interest and to adopt a latent variable approach to analysis. With this method, Friedman and Miyake showed clear evidence of the separability of CF from other EFs [ 14 ]. This study showed that tests of CF ("shifting"), inhibition, and working memory have relatively low correlations with one another (representing an overall EF construct) but correlate well within each component. However, what is unknown–and would be a novel scientific advance–is whether cognitive flexibility itself may be further fractionated into sub-components (such as relating to rule learning and exploration as distinct from executive switching) and the extent to which different CF tasks tap into these sub-skills. In the current project, we seek to address this gap in understanding the nature and measurability of the cognitive flexibility construct to generate robust operational measures of CF for our later training studies.
In contrast to working memory and inhibition control, which are highly heritable skills, cognitive flexibility (CF) may be influenced more by environmental factors and potentially beneficial from training more effectively [ 11 , 17 ]. Previous CF training interventions have used tasks that activate other executive functions, making them less precise as training tools [ 17 – 19 ]. To develop a training approach more precisely targeted at CF, we adopt the Structure Learning (SL) task, which involves identifying patterns in the presentation of stimuli without explicit feedback [ 20 – 23 ], as a foundational training paradigm (for more details about the SL task, see Methods section and Appendix D in S1 File ). The SL training approach emphasizes the emergence of flexibility through exploring and rule learning generated in dynamic environments. SL training might lead to higher-order and potentially generalizable "learning-to-learn" abilities rather than rote memorization of specific information. We hypothesize that SL performance is associated with CF measures; thus, we include SL in the task battery and carefully examine its relationship with CF and other cognitive constructs.
A growing literature demonstrates links between CF and key outcomes of interest, particularly academic performance. For example, Yeniad et al. found that associations between shifting ability and reading and maths performance were "substantial and significant" [ 24 ], and Mayes et al. found that performance on the Wisconsin Card Sort Test–a prototypical test of cognitive flexibility was one of the very few measures that predicted maths performance, after accounting for general intelligence [ 25 ]. Accumulating data from these and other studies have provided largely correlational evidence and, to a lesser extent, developmental and intervention-based evidence for links between cognitive flexibility and “real-world” outcomes. However, few studies have adequately controlled for the effects of IQ and working memory (or inhibition) or adopted a latent variable approach to elucidate the specific contribution of cognitive flexibility toward the outcomes of interest. Therefore, in this new project, we seek to better characterise the relationship between the CF construct and academic outcomes, creativity, problem-solving and socioemotional skills in the context of Singaporean young adults.
Research aim and strategy
Prior research has signified CF’s theoretical and practical importance as a vital factor in optimising success. To better characterise the CF construct and clarify CF’s cognitive and social underpinnings, we proposed the current project to investigate the relationships between CF, other executive functions, and other critical cognitive and social constructs in a Singaporean cohort. Specifically, the study aims to confirm whether there is a relative dissociation between CF and other executive functions (such as working memory and inhibition). We further aim to assess relationships between CF and other primary outcome variables, including structure learning, creativity, literacy, numeracy, and problem solving. Finally, the research aims to assess the influence of vital socio-cognitive variables (e.g., multilingualism, perceived social support, tolerance of uncertainty, and social decision-making) on these relationships. Understanding these relationships has value for translational research, such as in organisational settings (e.g., decision-making, career), and informs the need for CF training and development in the local educational curricula. The main hypotheses are summarized below.
- Latent variable analyses will show relative dissociation between cognitive flexibility and other executive functions (such as working memory and inhibition).
- Cognitive flexibility will be relatively dissociated from general intelligence.
- Cognitive flexibility will be best represented by more than one latent variable.
- Structure learning scores will be associated with at least one of the cognitive flexibility latent variables.
- Cognitive flexibility latent variable(s) will be associated with at least one primary outcome variable (creativity, literacy, numeracy, and problem solving)
- The relationship between structure learning scores and cognitive flexibility will vary significantly at different levels of each primary socio-cognitive variable (multilingualism, perceived social support, tolerance of uncertainty, and decision-making factors).
The current study is part of a large-scale international collaboration under the University of Cambridge-Nanyang Technological University Centre for Lifelong Learning and Individualised Cognition (Cambridge-NTU CLIC). CLIC launched as a flagship research programme within the National Research Foundation of Singapore’s Science of Learning initiative and aims to improve support for lifelong learning and cognitive agility. The following protocol describes an adult characterisation study which aims to investigate the construct validity of CF and CF’s relationship with other cognitive constructs of interest. Thereafter, this study will be referred to as the “CLIC adult study”, as distinct from a related study (not reported here) that will be conducted with adolescents. Results of this study will inform the development of intervention paradigms to enhance CF (i.e., through structure learning-based training). CLIC projects also include adolescent studies and intervention studies, which will be reported separately.
Methods overview
The CLIC adult study protocol will be described in three main sections: Study Preparation, Study Administration Part I (Demographics, social questionnaires, and social decision-making tasks), and Part II (Cognitive tasks). Fig 1 summarises the overall study workflow. Study preparation encompasses recruitment, eligibility screening, and consent procedures. Study Administration Part I is delivered online, including demographics, socio-cognitive questionnaires, and social decision-making tasks. Part II of the study, the cognitive task battery, is administered in a lab or a hybrid remote-guided testing setting, based on participants’ preferences and suitability for online task administration.
- PPT PowerPoint slide
- PNG larger image
- TIFF original image
Detailed documentations of all tasks and questionnaires can be found in Appendices A to F in S1 File .
https://doi.org/10.1371/journal.pone.0286208.g001
Study preparation
This study has obtained ethical approval (IRB-2021-761 "Executive Functions and Learning") from the Nanyang Technological University Institutional Review Board (IRB). Written consent from all participants will be obtained for this study.
Recruitment overview.
Participants in this study will be Singaporeans recruited from vocational institutions (such as the Institute of Technical Education), institutions of Higher Education (NTU, other local universities and polytechnics), as well as from the general working population via community sampling (e.g., community centres, clinics, via social media platforms). Interested participants will be provided with a study sign-up webpage. The recruitment phase will commence on November 22, 2021, and will continue until January 22, 2024, or until the desired sample size has been reached, whichever occurs first. Access to information that could identify individual participants will only be granted to the Principal Investigator, Co-Principal Investigators, and key research staff assigned to manage personal data, such as for contacting the participant to schedule for an experiment.
Eligibility screening and consent.
Upon signing up for the study, participants are requested via email to complete the eligibility screening questionnaire (Appendix A in S1 File ) online. Participants’ responses to the screening questionnaire will be used to determine their eligibility for this study. See Appendix A in S1 File for the detailed inclusion criteria. Eligible participants will have the study information sheet and consent form sent to them via Adobe sign. They will have their queries or concerns answered through email or a virtual meeting with a research staff before consenting to the study. Afterwards, the participant will be assigned a participant ID for the study.
Sample size.
We aim to recruit up to 400 healthy young adults aged 18 to 30 years old inclusive, to achieve a final sample size of at least N = 347. This sample size calculation is based on a meta-analysis by Yeniad et al. [ 24 ], with a data structure that is similar to the present study, suggesting a tight distribution of pairwise correlations ranging between 0.2 and 0.3 (with isolated extremes 0.09, 0.36). Based on this meta-analysis, we conservatively expect an effect size of approximately r = 0.15. Fisher’s Z transformations would hence require an N of 347 to achieve 80% power with an alpha set at 0.05. Allowing for a 15% data attrition rate, this yields a recruitment target of N = 400.
Pre-registration.
The current study protocol elaborates on our submitted pre-registration to the Open Science Framework [ 26 ].
Study administration
Table 1 summarises the planned CLIC adult study administration, which comprises of two parts: Part I will be conducted online and Part II will be conducted either entirely in a lab (Face-to-Face, F2F condition) or in a hybrid Remote-Guided Testing setting (Hybrid RGT). All participants will first complete online socio-cognitive questionnaires and social decision-making tasks in Part I (details in section on Social Factors, and in Appendix B in S1 File ). The items within each questionnaire/task will be presented in a fixed order, except for one section from the Clip-Q Singapore Language History Questionnaire.
https://doi.org/10.1371/journal.pone.0286208.t001
Part II can be administered flexibly via one of two testing modalities: Face-to-Face in-lab testing (F2F) and hybrid Remote-Guided Testing (RGT). To tackle the challenges posed by the global COVID-19 pandemic in conducting human psychological research, Leong et al. proposed a new mode of testing–Remote-Guided Testing, as a promising alternative to the traditional in-person testing mode while maintaining the quality of cognitive data collected [ 27 ]. With the easing of social distancing measures, the CLIC Cognition Team has adapted the RGT protocol originally developed in 2020 to a hybrid-RGT version in 2022, incorporating both in-person and online testing.
At the beginning of Part II, participants will complete a technical questionnaire to ascertain their eligibility for RGT (See Fig 1 . Full questionnaire can be found in Appendix C in S1 File ). The screening criteria include operating system compatibility for our testing software, minimum screen size and resolution, and wired keyboard and mouse connections. If the participant meets the necessary technical criteria, arrangements will be made to schedule them for the hybrid-RGT sessions, otherwise, they will be scheduled for F2F sessions.
After confirming the participants’ three sessions in Part II of the study, they will complete the same set of cognitive tasks. The cognitive task battery will be delivered both manually and on computerised study platforms, such as Cambridge Neuropsychological Test Automated Battery (CANTAB) ( www.cambridgecognition.com ), Inquisit 6 (Millisecond, 2019), Gorilla Experiment Builder ( www.gorilla.sc ), and iABC (iabc.psychol.cam.ac.uk/welcome), as detailed in the next section and in Appendix D in S1 File . The task orders will be deliberately randomised to control for order effects, depending on the allocation of tasks within each session. Participants will be randomly assigned to one of the three testing orders, as summarised in Appendix E in S1 File . The F2F sessions will be administered in a lab setting using a ThinkPad E14 laptop, wired mouse and earphones. The entire cognitive task battery, tested over three sessions, will take approximately 8.5–9 hours to complete. Appendix F in S1 File summarises the estimated task durations for each cognitive task.
Comprehensive task battery
To address the study’s research hypotheses, we curated a comprehensive test battery consisting of cognitive tasks, social and multilingualism questionnaires, and decision-making tasks. We aim to measure critical cognitive constructs such as cognitive flexibility, working memory, response inhibition, structure learning, general intelligence, creativity, literacy, numeracy, problem-solving, as well as socio-cognitive variables such as multilingualism, social decision-making, perceived social support, tolerance of uncertainty (need for closure and social experiences), and career decision making and planning. Fig 2 summarises the primary cognitive constructs examined within the CLIC adult study, which are further described in the following text and Appendix D in S1 File .
Appendix D in S1 File documents the full task details.
https://doi.org/10.1371/journal.pone.0286208.g002
Cognitive flexibility.
One major aim of this study is to validate the construct of cognitive flexibility (CF) as a latent variable, before establishing its relationship to structure learning (SL) and other outcome variables of interest. To characterise the CF construct, we curated five tests (see Fig 3 ). The Wisconsin Card Sort Test [ 28 ] and CANTAB Intra-/Extra-Dimensional Set Shifting task [ 29 ] probe set-shifting abilities, in which participants need to learn the rule via feedback and overcome the old rule when there is a rule change. The Trail Making Test [ 30 ] and Task Set-Switching [ 31 ] paradigm are classic assessments of executive switching abilities, in which participants need to follow the instructed rule to switch between different actions. Similar tests have been used previously to assess the CF construct [ 13 , 32 ]. A fifth test, Probabilistic Reversal Learning [ 33 ], has excellent face validity as a test of CF, but requires decision-making under uncertainty to a greater extent than the other four tests, because the task rules are probabilistic rather than deterministic. This uncertainty might offer more room for exploration and thus tap into a unique aspect of CF that could be related to implicit learning of probabilistic patterns and creativity.
For key constructs of interest, such as cognitive flexibility and creativity, we administer multiple tasks to improve measurement validity. See Appendix D in S1 File for the full task list and details.
https://doi.org/10.1371/journal.pone.0286208.g003
Structure learning.
The Structure Learning (SL) task [ 20 , 21 ] involves identifying patterns in seemingly stochastically presented symbols and making predictions based on learned patterns. On each trial, participants will see a sequence of visual symbols and will then be asked to predict which symbol they think should come next in the sequence ( Fig 3 , middle left panel). The symbol sequences are embedded with pre-defined probabilistic contingencies. Studies have shown that participants’ responses will gradually reflect those hidden probabilistic patterns through SL training, even without trial-level feedback [ 20 ].
The SL task tests a person’s ability to implicitly learn and understand the underlying structure of their environment under conditions of uncertainty and adjust to new problem-solving rules based on prior experience and current information. The current study hypothesizes that the learning strategies used in the SL task may be related to CF and aims to examine the relationship between CF and SL task performance to inform the development of future CF training interventions.
Outcome variables.
The key outcomes of interest in relation to CF include creativity, mathematics, and language skills. To assess creativity ( Fig 3 ), we will employ classical tests of convergent and divergent creativity, including the Remote Associates Test, Alternative Uses Test, Torrance Test of Creative Thinking—Figural, as well as basic verbal fluency (phonological and semantic) measures. We will also include a novel test, the Creative Foraging Game to measure explore-exploit decisions in creative foraging behaviours [ 34 ]. We will use the Woodcock Johnson IV: Tests of Achievements [ 35 ] reading (Letter-Word Identification, Sentence Reading Fluency, Passage Comprehension) and maths (Calculation, Applied Problems, Maths Facts Fluency) subtests as standardised measures of language and maths skills.
We will also examine how these cognitive factors relate to real-life, impactful, and significant decisions and phenomena. Given the increased interest in an adaptive workforce (see introduction) we focus here on career decision making in university students, as this population will soon be facing an important career and life transition to employment. We specifically examine the general phenomenon of career construction and career flexibility–i.e., the way individuals construct, adapt and change their vocational and career identity to respond to a dynamic work environment. We adopt the Career Construction Model of adaptation [ 36 , 37 ].
Social factors.
Individuals do not learn in a vacuum–the socio-cultural environment and dynamics might enhance, promote, or inhibit the development and manifestation of executive functions. This is true for more systemic environmental factors such as socio-economic status and formal education but has even been found for engagement in particular activities such as music making [ 38 – 40 ]. Indeed, social interactions with peers, elementary school social experiences, social skills intervention programs and even a very short social exchange have been associated with executive functions and their development [ 41 – 43 ]. We therefore need to characterise participants’ social context in order to understand how these might moderate the cognitive factors of interest. For the current study, we focus on four key factors for which the literature provides strong indications of a possible link with cognitive flexibility and that are relevant in the local context (see Fig 4 , which also includes complementary questionnaire measures of creativity and control variables).
See Appendix B in S1 File for the full list.
https://doi.org/10.1371/journal.pone.0286208.g004
Multilingualism is the ability of an individual speaker or a group of speakers to communicate effectively in more than one language. There is overwhelming evidence that the languages in a multilingual’s repertoire are always jointly activated even if not required in a given context. This results in constant competition and depending on the interlocutor there will be a need for the selection of the target, and inhibition of the non-target language (in monolingual contexts) or switch frequently between languages (multilinguals). These skills were originally mostly thought to link with inhibition but are more recently seen as a broader issue of executive attention, which includes most of the executive functions [ 44 ]. The Singapore government actively promotes multilingualism resulting in most people in the country being able to speak or at least understand multiple languages and the environment also presenting multilingually.
Tolerance of uncertainty refers to the extent to which someone is motivated (as opposed to being able) to engage in unconventional and novel ways of thinking and doing. We focus on social aspects here as social contexts are typically full of ambiguity, involving opposing views and unpredictable others producing feelings of uncertainty. Specifically, we examine the need for cognitive closure [ 45 ], the willingness to accept opposing views [ 46 ], beliefs about the fundamental and unchanging nature of race (racial essentialism) [ 47 ], and the extent of multicultural experiences someone has [ 48 ]. Thus, we supplement the examination of the capacity for cognitive flexibility with the motivational desire for a definite and crystallised answer, as opposed to ambiguity (need for closure), and the tendency to apply similar motivations to cognitions about social groups (racial essentialism). We also will capture people’s experience with social situations that may generate uncertainty and challenge conventions (e.g., multiculturalism, willingness to accept opposing views).
Perceived social support indicates to what extent an individual believes that they can rely on their social contacts especially during times of need. Social exchanges are built on the expectation of reciprocity–it is thought that confidence in the levels of social support may promote openness and flexibility. Singaporeans have multiple sources of social support, including an extended family, friends and others in a tight-knit community, possible larger and more diverse than in Western societies. We measure perceived social support based on individuals’ perceptions of the extent to which they have significant others, family and friends they can rely on in terms of need [ 49 , 50 ] as well as their social networks [ 51 ].
Social Decision-Making preferences reflect the way participants choose between options necessitating to take into account the preferences and strategies of other people. Multidisciplinary research early has suggested that human intellect and executive functions, to a great extent, evolve as a response to social exchanges, and especially balancing between cooperation and competition [ 52 – 54 ]. Such dynamic and intense social interactions require flexible thinking and adaptive responses to allow for mentalising, perspective taking, learning of norms and exercising strategic thinking and behaviour These preferences allow for the building of trust and reciprocity, the pillars of efficient economic exchanges [ 55 , 56 ] and entrepreneurial cognition [ 57 ]. These factors are also examined here with stylized game-theoretic approaches.
The questionnaires included some additional measurements. The expression of creativity depends not only on the creative potential (measured by the creativity tasks in Part II–see Fig 2 ) but, as well, the beliefs and motivation to be creative (“creative mindset”). We thus added questionnaires that measure these beliefs [ 58 – 62 ]. Finally, a set of control variables was added, including personality (BIG-5 and Empathy Quotient) as well as measurements of sleep quality and preferences ( Fig 4 ).
Analysis plan
Our large-scale data collection and comprehensive task battery permits the use of SEM methods to address the aforementioned "task impurity" issue [ 32 ]. Accordingly, we plan to establish measurement models of latent constructs (e.g., CF, other EFs, creativity, and social factors) and test the hypothesized relationships between the latent constructs. Further, computational modelling can reveal potentially separable cognitive processes with formal parameterised models [ 63 ], offering an in-depth look at the cognitive constructs of interest. Computationally modelled parameters from the Reinforcement Learning (RL) framework utilise trial-level data and their relationships with task feedback to separate participants’ characteristics in value updating and decision-making. RL modelling parameters thus provide insights into mechanistic links between CF and other cognitive constructs that may otherwise be hidden when investigated using surface-level behavioural indices (e.g., overall accuracy, mean response time). In the current study, tasks that involve rule-learning via feedback can be modelled using the RL framework [ 64 – 66 ]. In particular, we plan to use hierarchical Bayesian RL models to extract learning and explore-exploit related parameters from Wisconsin Card Sort Task [ 67 ], CANTAB Intra-/Extra-Dimensional Set Shifting task [ 68 ], and Probabilistic Reversal Learning task [ 69 ]. We also plan to apply task-specific computational models where appropriate, e.g., structure learning task [ 20 , 21 ] and stop signal task [ 70 ].
Significance and impact
The current study will be the first systematic large-scale investigation into the construct validity of CF and its interrelationship with other important cognitive skills such as learning and creativity, in an Asian context. A further point of novelty is that the study will offer an in-depth analysis of CF sub-components and their specific relation to other cognitive skills, in particular structure learning and creativity. The exploration of CF as a non-unitary construct will be a novel theoretical contribution to the field of executive functions. Finally, the results of the study are expected to be useful for informing classroom pedagogy and the design of lifelong learning policies and curricula, suited for the Singaporean context but also with wider applicability. Here, we will adopt the Remote-Guided Testing (RGT) approach to overcome challenges posed by any on-going COVID-19 pandemic-related restrictions. Leong et al. demonstrated equivalent data quality from the RGT method compared with lab-based settings [ 27 ]. Thereby, there is potential for the RGT method to complement traditional F2F methods in both research and clinical settings, particularly in situations where in-person meetings would be difficult or impossible. In clinical settings, remote testing methods not requiring the use of personal protective equipment such as masks may be beneficial to reduce the communication barrier between experimenter and participant [ 71 ].
CLIC is unique in its focus on translational research—bringing cognitive neuroscience knowledge into the design of learning pedagogies and lifelong learning programmes. Cognitive flexibility may be key to learning and the ability to upskill, reskill, and fit existing knowledge to ever-evolving life situations, such as job changes and environmental uncertainties [ 72 , 73 ].
The current study also has a unique potential impact on education in both school and life-long learning settings. Globalisation and rapid technological development have provided myriad opportunities for the advancement of student education and novel training in the workplace. However, these same processes will also engender new sources of uncertainty, requiring students to develop a fluid mindset to respond to accompanying challenges. Critical competencies such as creativity, curiosity, and grit are some of the traits that can help students navigate this uncertainty and embrace the challenges [ 74 , 75 ]. For example, students today are already constantly bombarded with many sources of information on the Internet, and they need to develop capacities to analyse these data systematically. Without the ability to fluidly transition between similar but competing ideas, students would effectively be perseverating on familiar interpretations of these data. Navigating through a high-density information environment requires intelligence and, more importantly, the ability to discover new patterns amid the noise and flexibly disengage with previously valued methods. Thus, cognitive flexibility is the critical skill that may be important to nurture among students and life-long learners.
Limitations and future directions
The current testing protocol is adapted for adults with testing modalities in-lab or in a standardised remote guided testing scenario. While this helps with collecting high-quality data, there are limitations when generalizing to other populations or testing scenarios. For example, our protocol needs age-appropriate adaptation when testing younger age groups (e.g., infants and adolescence), which require additional considerations in terms of testing duration, language requirement, and cognitive load; or older age groups, such as busy working professionals (that might not have the time or motivation for face to face testing) or seniors (over 65 years old with particular socio-cognitive or even physical / movement limitations).
Finally, there is potential to enhance participant motivation and engagement with the cognitive tasks through gamification, including social gamification (such as cooperative and competitive incentive schemes). Adding game elements could enhance participants’ motivation and engagement for a wide age range [ 76 – 78 ]. Applying gamification elements judiciously could lead to the creation of enjoyable and scientifically accurate cognitive evaluations. Identifying suitable elements for gamification is a secondary aim of the project, which will increase the potential translational value and impact of our findings.
Supporting information
S1 file. appendices a to g for “study protocol: how does cognitive flexibility relate to other executive functions and learning in healthy young adults”..
https://doi.org/10.1371/journal.pone.0286208.s001
Acknowledgments
We gratefully acknowledge all the CLIC Phase 1 Consortium members for their dedication and collaboration in this project. Our sincere thanks go to the external collaborators for their insightful contributions. We extend our appreciation to the student assistants for their crucial efforts. We also acknowledge our funding agencies and institutions for their financial support. Lastly, we express our gratitude to all individuals who provided valuable feedback and encouragement. We are fortunate to have collaborated with such exceptional individuals, whose collective efforts shaped the success of this research project. The complete list of consortium members, external collaborators, and student assistants can be found in the Appendix G in S1 File .
- View Article
- Google Scholar
- PubMed/NCBI
- 7. Royal Society (Great Britain) Brain. Brain Waves: Module 1 Neuroscience, Society and Policy. Royal Society; 2011.
- 9. Howells K. The future of education and skills: education 2030: the future we want [Internet]. Paris: OECD; 2018 Apr.
- 10. Nägele C, Stalder BE. Competence and the Need for Transferable Skills. In: Mulder M, editor. Competence-based Vocational and Professional Education: Bridging the Worlds of Work and Education. Cham: Springer International Publishing; 2017. p. 739–53. (Technical and Vocational Education and Training: Issues, Concerns and Prospects).
- 35. Schrank FA, Wendling BJ. The Woodcock–Johnson IV: Tests of cognitive abilities, tests of oral language, tests of achievement. In: Contemporary intellectual assessment: Theories, tests, and issues, 4th ed. New York, NY, US: The Guilford Press; 2018. p. 383–451.
- 44. Bialystok E. Bilingualism and executive function: What’s the connection? In: Bilingual cognition and language: The state of the science across its subfields. Amsterdam, Netherlands: John Benjamins Publishing Company; 2018. p. 283–305. (Studies in bilingualism).
- 45. Kruglanski AW. The psychology of closed-mindedness. New York: Psychology Press; 2004.
- 49. Cutrona CE, Russell DW. The provisions of social relationships and adaptation to stress. In: Jones WH, Perlman D, editors. Advances in personal relationships. JAI Press; 1988. p. 37–67.
- 53. Vygotsky LS. Mind in Society: Development of Higher Psychological Processes. Cole M, John-Steiner V, Scribner S, Souberman E, editors. Cambridge, MA: Harvard University Press; 1980. 176 p.
- 64. Sutton RS, Barto AG. Introduction to reinforcement learning. MIT press Cambridge; 1998.

How it works
Transform your enterprise with the scalable mindsets, skills, & behavior change that drive performance.
Explore how BetterUp connects to your core business systems.
We pair AI with the latest in human-centered coaching to drive powerful, lasting learning and behavior change.
Build leaders that accelerate team performance and engagement.
Unlock performance potential at scale with AI-powered curated growth journeys.
Build resilience, well-being and agility to drive performance across your entire enterprise.
Transform your business, starting with your sales leaders.
Unlock business impact from the top with executive coaching.
Foster a culture of inclusion and belonging.
Accelerate the performance and potential of your agencies and employees.
See how innovative organizations use BetterUp to build a thriving workforce.
Discover how BetterUp measurably impacts key business outcomes for organizations like yours.
A demo is the first step to transforming your business. Meet with us to develop a plan for attaining your goals.

- What is coaching?
Learn how 1:1 coaching works, who its for, and if it's right for you.
Accelerate your personal and professional growth with the expert guidance of a BetterUp Coach.
Types of Coaching
Navigate career transitions, accelerate your professional growth, and achieve your career goals with expert coaching.
Enhance your communication skills for better personal and professional relationships, with tailored coaching that focuses on your needs.
Find balance, resilience, and well-being in all areas of your life with holistic coaching designed to empower you.
Discover your perfect match : Take our 5-minute assessment and let us pair you with one of our top Coaches tailored just for you.

Best practices, research, and tools to fuel individual and business growth.
View on-demand BetterUp events and learn about upcoming live discussions.
The latest insights and ideas for building a high-performing workplace.
- BetterUp Briefing
The online magazine that helps you understand tomorrow's workforce trends, today.
Innovative research featured in peer-reviewed journals, press, and more.
Founded in 2022 to deepen the understanding of the intersection of well-being, purpose, and performance
We're on a mission to help everyone live with clarity, purpose, and passion.
Join us and create impactful change.
Read the buzz about BetterUp.
Meet the leadership that's passionate about empowering your workforce.

For Business
For Individuals
What is cognitive flexibility, and why does it matter?

Transform your life
Make meaningful changes and become the best version of yourself. BetterUp's professional Coaches are here to support your personal growth journey.

Jump to section
What is cognitive flexibility?
Why is cognitive flexibility necessary, 3 cognitive flexibility examples, what does cognitive inflexibility mean, how to improve your cognitive flexibility, 3 tools and tests to measure your cognitive flexibility.
Build your cognitive flexibility
Have you ever worked with someone who can focus on multiple high-stakes tasks at once with relative ease?
Or someone who can come up with a novel idea under the pressure of a deadline?
These are all examples that help illustrate the definition of cognitive flexibility.
Cognitive flexibility is key for success in the workplace, but also in everyday life. It allows for flexible thinking to adapt to various situations.
Let’s explore the cognitive flexibility definition and how you can improve your own flexibility.
Sometimes known as cognitive shifting, cognitive flexibility is all about your brain’s ability to adapt to new, changing, or unplanned events.
Cognitive flexibility is also the ability to switch from one way of thinking to another. This is also known as task switching.

Think about it this way. You shift your body to change direction. You also shift your car into a new lane to avoid danger.
You can also learn to shift your thinking process to become more adaptable to the situation at hand. This is a prime example of cognitive flexibility.
Cognitive flexibility is important both on a micro and a macro scale in the workplace. It allows you to juggle multiple concepts at once and improve your cognitive function.
You use cognitive flexibility without realizing it on a daily basis. This happens when you multitask or when you switch from task to task.
It also happens when you interact with other people and when you go from talking to a customer to your peers.
Without mental flexibility, you’d be unable to ‘switch’ your brain from situation to situation.
It’d be difficult to concentrate on a task and perform it adequately. It’s a necessary cognitive process for productivity.
On a more macro scale, people also exhibit cognitive flexibility when thinking about a:
- Product within an industry
- Person within a team
- Single step forward when solving a complex problem
Your brain can shift from “zoomed in” to the micro (the product) to “zoomed out” to the macro (the industry).
As a result, cognitive flexibility allows you to solve problems creatively , adapt to curveballs, and act appropriately in varying situations. This is because you’re able to see from a different perspective.

So what can this look like in real situations? Here are three examples that illustrate mental flexibility.
What’s for dinner: you planned a recipe for tonight’s dinner but find you’re missing an ingredient when you get ready to cook. Cognitive flexibility will allow you to consider your options and improvise a new recipe instead of getting upset.
Your friend suddenly stops talking to you: with cognitive flexibility, you can think about why they’re acting this way. It allows you to consider their point of view and analyze the possibilities from every angle.
Someone gets sick for an event: let’s say a key volunteer for a charity event gets sick. Cognitive flexibility will allow you to consider all the options to adjust quickly. You’ll think of other people you can call. Or you’ll find ways to adjust the event with the volunteers you currently have.

The opposite of cognitive flexibility is cognitive rigidity or cognitive inflexibility.
Think about the way water moves. Water in its liquid state is similar to cognitive flexibility. But water in its frozen state is similar to cognitive rigidity. When water travels, it has the capacity to find many different paths. This is true for small streams, raging rivers, or dropped water in your kitchen.
If you’ve ever noticed how a water leak moves, you’ve seen this in action. The water will flow in several directions. It will find endless ways to surpass obstacles and continue flowing. Water follows the path of least resistance or the most efficient path for it to take.
Ice, on the other hand, is rigid. If it meets an obstacle, it cannot move past it until it melts. You can’t easily force something that’s rigid to be more fluid.
When you’re flexible, you have the cognitive ability to find more paths to a solution. You can see from multiple perspectives.
On the other hand, if you have rigid thinking, you may struggle to solve problems.
But even if you struggle with cognitive flexibility, you can work to improve this skill. Just like ice, you can melt back into water with a little bit of heat or pressure.
There’s no doubt that cognitive flexibility takes mental energy.
Think about what it feels like to go from a conversation with a toddler to a conversation with a manager. It can take a few moments to get in the right frame of mind and adapt your style to the different audiences.
Even switching between two adults can be difficult depending on the individual differences between them.
In a recent study , researchers tested the problem-solving abilities of capuchin and rhesus monkeys. They also performed the same test on humans.
100% of the monkeys demonstrated cognitive flexibility by finding a shortcut. But only 60% of humans did the same.
Practicing cognitive flexibility can create new neural pathways in your brain and improve your cognitive flexibility skill. This makes it easier to practice divergent thinking and creative problem-solving.
Here are some ways you can improve your cognitive flexibility so that you can approach a tough situation in a different way:
1. Start small
One way to practice cognitive flexibility is to introduce it in small, low-stakes ways in your life. You can expose yourself to new situations and different contexts without going too far outside of your comfort zone.
Here’s an example: the next time you order a meal at your favorite restaurant, pick something from your top three meals instead of ordering your first choice.
Imagine if the menu changes or if they’re out of your favorite food for the evening. By taking small flexible steps, you’ll start opening up to other options when you need to practice flexibility.
You’ll also become more open to trying new restaurants and new experiences.
Even if you start small, you can start improving your cognitive flexibility.
In a recent study, researchers taught rats to drive small cars. They learned that:
- The rats were more open to new challenges after learning the basics
- Rats’ stress levels went down once they mastered driving
- Richer environments led to faster learning
Like the rats, if you open yourself up to new experiences and challenges, you’ll be more open to experiencing more.
2. Build your empathy muscles
Understanding others’ experiences, processes, routines, and methods all help you build cognitive flexibility.
That’s because it helps you get out of the mentality that your way is the only way to go.
Struggling to build your empathy? Try reading fiction to see a story from someone else’s point of view.
You can also start approaching other people at work with your challenges. Ask them how they would approach a problem. Make sure you listen actively when they give their explanation.

Maybe you won’t agree with your coworker’s approach. But that’s not the point of the exercise. Doing this helps you see from their point of view.
Plus, listening can help improve your empathy and make you a better learner.
You’ll start to see that there are several ways you can approach one problem. You’ll also grow your knowledge by listening to others.
3. Interrupt and redirect your thoughts
This tactic is for people who tend to go down rabbit holes with negative thoughts about themselves. Catastrophizing is a common display of cognitive rigidity.
Here’s an example. Have you ever experienced something negative and then start telling yourself you’re a failure and that you’ll never get anything right?
You can start thinking you’ll get fired over one mistake and that you’ll never get another job opportunity.
In five seconds flat, your brain already reaches the point of thinking: “I’m a failure, and I will always be.”
When this happens, you can practice redirecting your thoughts. Be mindful of what you are thinking and interrupt the thought spiraling through your mind. Change the topic to something else entirely.
This can be easier said than done. To help you get there, get up and change your scenery. You can take a walk around the block, go on your lunch break earlier than usual, or go see one of your peers to ask about their day.
Think of it as pressing “pause” on your thoughts. You’re pressuring your brain to stop worrying , redirect, and focus on something else. This is an act of cognitive flexibility.
The more you do it, the easier it’ll become.
4. Ask yourself what else might be true
You can try this tip for yourself. But this is also a great tactic for managers to use when interacting with employees.
You can use this if one of your employees is stuck, frustrated, or a bit stubborn.
Ask them, “What else might be true?” Make sure you do this in a gentle and kind way. This will help them take a broader look at a situation. It will encourage them to consider other perspectives and look at other possible options.
For example, if an employee is upset about a canceled client meeting, they might say:
“The client canceled, and I bet they’re going with our competitor. I knew we should have priced it differently.”
Your job as a manager is to urge them to think about what else might be true. Maybe the client got sick. Maybe something else came up that you don’t know about. Maybe the support staff forgot to book a conference room.
There are so many alternative explanations. Urging your employees to think this way enhances not only their cognitive flexibility skills, but also their strategic thinking skills.

Want to know where you stand regarding cognitive flexibility? Here is how you can perform the test on yourself.
The Cognitive Control and Flexibility Questionnaire
This questionnaire was developed in the context of research completed in 2018.
Here are statements the researchers developed. Your job is to decide if these statements are true or false for you:
- I get easily distracted by upsetting thoughts or feelings.
- My thoughts and emotions interfere with my ability to concentrate.
- I have a hard time managing my emotions.
- It’s hard for me to shift my attention away from negative thoughts or feelings.
- I feel like I lose control over my thoughts and emotions.
- It is easy for me to ignore distracting thoughts.
- It’s difficult to let go of intrusive thoughts or emotions.
- I find it easy to set aside unpleasant thoughts or emotions.
- I can remain in control of my thoughts and emotions.
- I take the time to think of more than one way to resolve the problem.
- I approach the situation from multiple angles.
- I consider the situation from multiple viewpoints before responding.
- I take the time to see things from different perspectives before reacting.
- I take the time to think of several ways to best cope with the situation before acting.
- I weigh out my options before choosing how to take action.
- I manage my thoughts or feelings by reframing the situation.
- I control my thoughts and feelings by putting the situation into context.
- I can easily think of multiple coping options before deciding how to respond.
The Cognitive Flexibility Scale
Just like the previous cognitive flexibility test, this is another research-backed tool to help you with cognitive flexibility.
You can run a demo of the test to see what results you get for yourself.
Flanker test and stroop test
Flanker and Stroop tests were developed in the ’70s, but they’re still used today to evaluate cognitive flexibility.
They involve using colors or arrows to see how well you can react to change.
You can test yourself here and practice reading the colors to improve your cognitive flexibility.
Or, you can run a more complete version of the flanker test by using the demo available here . It may take some practice to get used to how this test works.
Cognitive flexibility requires practice in the small moments of your everyday life.
If you want to improve your own cognitive flexibility, you can practice at work or at home.
When you get upset or feel stuck, remember to give yourself some grace. It takes practice to develop cognitive flexibility.
If you still experience getting stuck, it’s normal. Pause and breathe for a few seconds and consider what else might be possible in your situation.
With the right coaching, you can build your cognitive flexibility even faster. Try BetterUp today to see how you and your team can build cognitive flexibility together.
Lauren Miller
BetterUp Fellow Coach
What is emotional well-being? 8 ways to improve your mental health
Are you reaching your full potential a guide to personal development, why self-management is key to success and how to improve yours, what is self-preservation 5 skills for achieving it, physical well-being and health: what it is and how to achieve it, what is determination develop traits you need to succeed, how to walk the freeing path of believing in yourself, 13 imposter syndrome books from psychologists and coaches, why the best time to drink coffee is not when you wake up, similar articles, why employee flexibility is the new workplace watchword, cognitive bias: what it is and how to overcome it, learn how to cope: 6 factors that promote resilience, developing cognitive empathy to become a better coworker, how to become a life coach and start a promising career, what is cognitive dissonance and how do you reduce it, why workplace flexibility matters and 4 ways to offer it, developing psychological flexibility, why cognitive agility matters, stay connected with betterup, get our newsletter, event invites, plus product insights and research..
3100 E 5th Street, Suite 350 Austin, TX 78702
- Platform Overview
- Integrations
- Powered by AI
- BetterUp Lead
- BetterUp Manage™
- BetterUp Care™
- Sales Performance
- Diversity & Inclusion
- Case Studies
- Why BetterUp?
- About Coaching
- Find your Coach
- Career Coaching
- Communication Coaching
- Life Coaching
- News and Press
- Leadership Team
- Become a BetterUp Coach
- BetterUp Labs
- Center for Purpose & Performance
- Leadership Training
- Business Coaching
- Contact Support
- Contact Sales
- Privacy Policy
- Acceptable Use Policy
- Trust & Security
- Cookie Preferences
Cognitive flexibility and belonging among university students: mediating role of adaptation
- Published: 28 March 2023
- Volume 43 , pages 3234–3242, ( 2024 )
Cite this article
- Aliye Ateş-Ös ORCID: orcid.org/0000-0002-0854-3486 1 &
- Nergüz Bulut-Serin 1
643 Accesses
2 Citations
Explore all metrics
A number of studies have explored the potential links between adaptation, cognitive flexibility, and school belonging. Although existing studies show that cognitive flexibility is associated with belonging, the current study suggests that cognitive flexibility increases belongingness with an indirect effect of adaptability. Also, adaptive behavior has been considered as a mediating variable as it is effective in re-establishing the order as a result of the deterioration of the established balance. Therefore, it is aimed to study the mediating role of adaptation for cognitive flexibility and the sense of belonging to the university. The sample of the study consists of 421 university students who participated voluntarily (290 women and 131 men; aged between 18 and 32 years). Participants were asked to fill out a questionnaire package consisting of cognitive flexibility, adaptation, and belongingness. Structural equation modeling was used to analyze the data. The effect of cognitive flexibility on the belongingness of university students was mediated by adaptation. The results highlight the importance of academic adaptation in university life. Moreover, it was found university students who reported greater cognitive flexibility have a better sense of belonging. Accordingly, some suggestions were proposed for high school educators, university administrators, and researchers.
This is a preview of subscription content, log in via an institution to check access.
Access this article
Price includes VAT (Russian Federation)
Instant access to the full article PDF.
Rent this article via DeepDyve
Institutional subscriptions
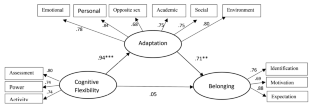
Similar content being viewed by others
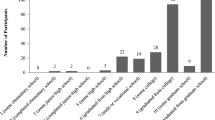
Sense of Belonging and Student Engagement: A Daily Study of First- and Continuing-Generation College Students
Cari Gillen-O’Neel
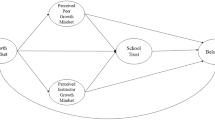
Belonging in STEM: Growth Mindset as a Filter of Contextual Cues
C. Lee Williams, Quinn Hirschi, … Josipa Roksa
Initial Development and Validation of the School Belongingness Scale
Gokmen Arslan & Erdinc Duru
Data Availability
The datasets generated during the current study are available on request from the corresponding author.
Allen, K. A., Slaten, C. D., Arslan, G., Roffey, S., Craig, H., & Vella-Brodrick, D. A. (2021). School belonging: The importance of student and teacher relationships. The Palgrave handbook of positive education (pp. 525–550). Cham: Palgrave Macmillan. https://doi.org/10.1007/978-3-030-64537-3_21
Chapter Google Scholar
Allen, K. A., Gray, D. L., Baumeister, R. F., et al. (2022). The need to Belong: A deep dive into the Origins, Implications, and future of a foundational construct. Educ Psychol Rev , 34 , 1133–1156. https://doi.org/10.1007/s10648-021-09633-6
Article PubMed Google Scholar
Aladağ, M., Kağnıcı, D., Tuna, M., & Tezer, E. (2003). Üniversite Yaşam Ölçeği: Ölçek Geliştirme ve Yapı Geçerliliği Üzerine Bir Çalışma. Turkish Psychological Counseling and Guidance Journal , 2(20), 41–47. Retrieved from https://dergipark.org.tr/tr/pub/tpdrd/issue/21440/229865
Altunkol, F. (2011). Üniversite öğrencilerinin bilişsel esneklikleri ile algılanan stres düzeyleri arasında ilişkinin incelenmesi. Yayımlanmamış yüksek lisans tezi, Çukurova Üniversitesi, Adana https://acikbilim.yok.gov.tr/handle/20.500.12812/132692
Alzubi, E. M., Attiat, M. M., & Al-Adamat, O. A. (2022). Systemic intelligence predictors of cognitive flexibility and cognitive holding power among university students. Cypriot Journal of Educational Science , 17 (2), 491–505. https://doi.org/10.18844/cjes.v17i2.6835
Article Google Scholar
American Psychological Association. (n.d.). Adaptive behavior. In APA dictionary of psychology. Retrieved May 18 (2019). from https://dictionary.apa.org/adaptive-behavior
Amerstorfer, C. M. and Freiin, & von Münster-Kistner, C. (2021). Student perceptions of academic engagement and student-teacher relationships in problem-based learning. Front. Psychol . 12:713057. doi: https://doi.org/10.3389/fpsyg.2021.713057
Arslan, G. (2021). School belongingness, well-being, and mental health among adolescents: Exploring the role of loneliness. Australian Journal of Psychology , 73 (1), 70–80. https://doi.org/10.1080/00049530.2021.1904499
Asıcı, E., & Sarı, H. I. (2021). A proposed model to explain happiness in College students: The Roles of Perceived Parenting Styles, emotional Self-Efficacy, and forgiveness. Journal of Adult Development, 28 (4), 332–345.
Bandura, A. (1986). Social foundations of thought and action: A social cognitive theory . Englewood Cliffs, NJ: Prentice-Hall.
Google Scholar
Bakırcıoğlu, R. (2012). Eğitim ve psikoloji sözlüğü . Ankara: Anı Yayıncılık.
Baumeister, R. F., & Leary, M. R. (1995). The need to belong: Desire for interpersonal attachments as a fundamental human motivation. Psychological Bulletin , 117 (3), 497–529. https://doi.org/10.1037/0033-2909.117.3.497
Article CAS PubMed Google Scholar
Baytemir, K., Akın-Kösterelioğlu, M., & Kösterelioğlu, İ. (2015). Algılanan Okul Yaşantıları Ölçeğinin Türkçeye Uyarlanması: Geçerlik ve Güvenirlik Çalışması. Çankırı Karatekin Üniversitesi Sosyal Bilimler Enstitüsü Dergisi, 6(2) , 597–608. Retrieved from https://dergipark.org.tr/tr/pub/jiss/issue/25891/272826
Bellici, N. (2015). Ortaokul öğrencilerinde okula bağlanmanın çeşitli değişkenler açısından incelenmesi. Abant İzzet Baysal Üniversitesi Eğitim Fakültesi Dergisi, 15(1), 48–65. Retrieved from https://dergipark.org.tr/tr/download/article-file/17047
Bilgin, M. (2009). Developing a cognitive flexibility scale: Validity and reliability studies. Social Behavior and Personality: an international journal , 37 (3), 343–353. https://doi.org/10.2224/sbp.2009.37.3.343
Browne, M. W., & Cudeck, R. (1993). Alternative ways of assessing model fit. In K. A. Bollen, & J. S. Long (Eds.), Testing structural equation models (pp. 136–162). Sage.
Cañas, J. J., Quesada, J. F., Antolí, A., & Fajardo, I. (2003). Cognitive flexibility and adaptability to environmental changes in dynamic complex problem-solving tasks. Ergonomics , 46 , 482. https://doi.org/10.1080/0014013031000061640
Cañas, J. J., Fajardo, I., & Salmeron, L. (2006). Cognitive flexibility. International encyclopedia of ergonomics and human factors , 1 (3), 297–301. https://doi.org/10.13140/2.1.4439.6326
Capps, M. A. (2003). Characteristics of a sense of belonging and its relationship to academic achievement of students in selected middle schools in region IV and VI educational service centers. Unpublished Phd Thesis. Texas: A&M University.
Coulacoglou, C., & Saklofske, D. H. (2017). Executive function, theory of mind, and adaptive behavior. Psychomterics and Psychological assessment. Principles and applications , 91–119. https://doi.org/10.1016/B978-0-12-802219-1.00018-3
Çuhadaroğlu, A. (2013). Bilişsel esnekliğin yordayıcıları. Cumhuriyet Uluslararası Eğitim Dergisi , 2 (1), 86–101.
Dajani, D. R., & Uddin, L. Q. (2015). Demystifying cognitive flexibility: Implications for clinical and developmental neuroscience. Trends in neurosciences , 38 (9), 571–578. https://doi.org/10.1016/j.tins.2015.07.003
Article CAS PubMed PubMed Central Google Scholar
Demirtaş, A. S. (2019). Stresli durumlarda bilişsel kontrol ve bilişsel esneklik: Bir ölçek uyarlama çalışması. Psikoloji Çalışmaları - Studies in Psychology , 39 (2), 345–368. https://doi.org/10.26650/SP2019-0028
Dennis, J. P., & Vander Wal, J. S. (2010). The cognitive flexibility inventory: Instrument development and estimates of reliability and validity. Cognitive therapy and research , 34 (3), 241–253. https://doi.org/10.1007/s10608-009-9276-4
Doğan-Laçin, B. G., & Yalçın, İ. (2015). Predictive roles of self-efficacy and coping strategies in cognitive flexibility among university students. Postgraduate Thesis, Ankara, Ankara University, Educational Sciences. https://doi.org/10.16986/huje.2018037424
Encina, Y., & Berger, C. (2021). Civic Behavior and sense of belonging at School: The moderating role of School Climate. Child Ind Res , 14 , 1453–1477. https://doi.org/10.1007/s12187-021-09809-0
Evli, M., & Şimşek, N. (2018). Stresle Başetmede Geliştirilebilir Bir Boyut: Öğrenilmiş Güçlülük. Journal of Contemporary Medicine , 8 (4), 377–380. https://doi.org/10.16899/gopctd.415035
Gray, D. L., Hope, E., & Byrd, C. M. (2020). Why Black adolescents are vulnerable at school and how schools can provide opportunities to belong to fix it. Policy Insights from the Behavioral and Brain Sciences , 7 (1), 3–9. https://doi.org/10.1177/2372732219868744
Hu, L. T., & Bentler, P. M. (1999). Cutof criteria for ft indexes in covariance structure analysis: Conventional criteria versus new alternatives. Structural Equation Modeling: A Multidisciplinary Journal , 6 (1), 1–55. https://doi.org/10.1080/10705519909540118
Jensen, F. E., & Nutt, A. E. (2015). The teenage brain: A neuroscientist’s survival guide to raising adolescents and young adults First edition. New York, NY: Harper, an imprint of Harper Collins Publishers.
Karaman, Ö. (2013). Öğrencilerin Öğrenim Gördükleri Üniversiteye Ait Olma Gereksinimlerinin İncelenmesi. Yayınlanmamış Doktora Tezi. İnönü Üniv. Eğitim Bilimleri Enst. Eğitim Bilimleri Böl . Malatya: Psikolojik Danışma ve Rehberlik Anabilim Dalı.
Kline, R. B. (2016). Principles and practice of structural equation modeling (4th ed.). New York, NY: Guilford Press.
Kösterelioğlu, M. A. (2021). Self-leadership perception and emotional intelligence as the predictors of cognitive flexibility. problems of education in the 21st century , 79 (5), 700–715. https://doi.org/10.33225/pec/21.79.700
Kuyumcu, B., & Kirazcı, F. (2020). The Link Between Cognitive Flexibility and Educational Stress Among High School Students: Mediation Through Perception of Teacher Acceptance. Turkish Psychological Counseling and Guidance Journal, 10 (58), 515–530. Retrieved from https://dergipark.org.tr/tr/pub/tpdrd/issue/57025/802046
Leary, M. R. (2021). Emotional reactions to threats to acceptance and belonging: A retrospective look at the big picture. Australian Journal of Psychology , 73 (1), 4–11. https://doi.org/10.1080/00049530.2021.1883410
Article MathSciNet Google Scholar
Mardia, K. V., & Foster, K. (1983). Omnibus tests of multiformity based on skewness and kurtosis. Communications in Statistics - Theory and Methods, 12 , 207–221. https://doi.org/10.1080/03610928308828452
Martin, M. M., & Anderson, C. M. (1998). The cognitive flexibility scale: Three validity studies. Communication Reports , 11 (1), 1–9. https://doi.org/10.1080/08934219809367680
Moore, A., & Malinowski, P. (2009). Meditation, mindfulness and cognitive flexibility. Consciousness and cognition , 18 (1), 176–186. https://doi.org/10.1016/j.concog.2008.12.008
Murphy, F. C., Michael, A., & Sahakian, B. J. (2012). Emotion modulates cognitive flexibility in patients with major depression. Psychological medicine , 42 (7), 1373–1382. https://doi.org/10.1017/S0033291711002418
Öz, S. (2012). Ergenlerin cinsiyet, sosyo-ekonomik ve öğrenim kademesi düzeylerine göre bilişsel esneklik, uyum ve kaygı puanları arasındaki ilişkinin incelenmesi. Yayınlanmamış yüksek lisans tezi . Adana: Çukurova Üniversitesi Sosyal Bilimler Enstitüsü.
Özkalp, E. (2003). Örgütsel Davranış . Eskişehir: Anadolu Universitesi Yayınları.
Piaget, J. (1970). Piaget’s theory. In P. Mussen (Ed.), Handbook of child psychology . New York, NY, USA: Wiley.
Pillow, D. R., Malone, G. P., & Hale, W. J. (2015). The need to belong and its association with fully satisfying relationships: A tale of two measures. Personality and individual differences , 74 , 259–264. https://doi.org/10.1016/j.paid.2014.10.031
Article PubMed PubMed Central Google Scholar
Pittman, L. D., & Richmond, A. (2007). Academic and psychological functioning in late adolescence: The importance of school belonging. The Journal of Experimental Education , 75 (4), 270–290. https://doi.org/10.3200/JEXE.75.4.270-292
Podsakoff, P. M., MacKenzie, S. B., Lee, J. Y., & Podsakoff, N. P. (2003). Common method biases in behavioral research: A critical review of the literature and recommended remedies. Journal of Applied Psychology , 88 (5), 879–903.
Preiss, D., D (2022). Metacognition, mind Wandering, and cognitive flexibility: Understanding Creativity. Journal of Intelligence , 10 , 69. https://doi.org/10.3390/jintelligence10030069
Reyes, M. E., Cruz, R., & Meza, I. (2023). University membership through immersive virtual environments and a sense of belongingness. Interactive Learning Environments , 1–13. https://doi.org/10.1080/10494820.2023.2165509
Robinson, O. C. (2014). Sampling in interview-based qualitative research: A theoretical and practical guide. Qualitative research in psychology , 11 (1), 25–41.
Robson, C. (2002). Real World Research: A resource for Social scientists and practitioner researchers . Oxford: Blackwell Publishers Ltd.
Schumacker, R. E., & Lomax, R. G. (2004). A beginner’s guide to structural equation modeling. 2nd Edition, Lawrence Erlbaum Associates, Mahwah.
Shamionov, R. M., Grigoryeva, M. V., Grinina, E. S., Sozonnik, A. V., & Bolshakova, A. S. (2023). Subjective assessments of the Pandemic Situation and Academic Adaptation of University students. OBM Neurobiology , 7 (1), 18. https://doi.org/10.21926/obm.neurobiol.2301150
Shamionov, R. M., Grigoryeva, M. V., Grinina, E. S., & Sozonnik, A. V. (2020). Characteristics of academic adaptation and subjective well-being in University students with chronic Diseases. European Journal of Investigation in Health Psychology and Education , 10 (3), 816–831. https://doi.org/10.3390/ejihpe10030059
Shrout, P. E., & Bolger, N. (2002). Mediation in experimental and nonexperimental studies: New procedures and recommendations. Psychological methods , 7 (4), 422. https://doi.org/10.1037/1082-989X.7.4.422
Smith, C. A., & Konik, J. (2022). Who is satisfied with life? Personality, cognitive flexibility, and life satisfaction. Current Psychology , 41 , 9019–9026. https://doi.org/10.1007/s12144-021-01359-6
Spiro, R. J., Feltovich, P. J., Jacobson, M. J., & ve Coulson, R. L. (1992). Cognitive flexibility, constructivism, and hypertext: Random access instruction for advanced knowledge acquisition in ill-structured domains. In T. M. Duffy, & D. ve Jonassen (Eds.), Constructivism and the technology of instruction: A conversation (s.57–75) . Hillsdale, NJ: Erlbaum.
Stevens, M. (2009). Kingdom of children. Culture and controversy in the Homeschooling Movement . Princeton: Princeton University Press. https://doi.org/10.1515/9781400824809
Book Google Scholar
Sürücü, M., & Bacanlı, F. (2010). Üniversiteye Uyumun Psikolojik Dayanıklılık ve Demografik Değişkenlere Göre İncelenmesi. Gazi Eğitim Fakültesi Dergisi, Cilt 30, Sayı 2, 375–396. Retrieved from https://dergipark.org.tr/tr/pub/gefad/issue/6741/90627
Tabachnick, B. G., & Fidell, L. S. (2007). Experimental designs using ANOVA (Vol. 724). Belmont, CA: Thomson/Brooks/Cole.
Turkington, D., Dudley, R., Warman, D. M., & Beck, A. T. (2006). Cognitive-behavioral therapy for schizophrenia: A review. Focus , 10 (2), 5–233.
Uddin, L. Q. (2021). Cognitive and behavioural flexibility: Neural mechanisms and clinical considerations. Nature Reviews Neuroscience , 22 , 167–179. https://doi.org/10.1038/s41583-021-00428-w
Vargas-Madriz, L. F., & Konishi, C. (2021). The relationship between social support and student academic involvement: The mediating role of school belonging. Canadian Journal of School Psychology , 36 (4), 290–303. https://doi.org/10.1177/08295735211034713
Yu, B., Mak, A. S., & Bodycott, P. (2021). Psychological and academic adaptation of mainland Chinese students in Hong Kong universities [Article]. Studies in Higher Education, 46 (8), 1552–1564. https://doi.org/10.1080/03075079.2019.1693991
Download references
This current study was not supported by a foundation or person.
Author information
Authors and affiliations.
Education Faculty, Department of Guidance and Psychological Counseling, European University of Lefke. Lefke/Northern Cyprus, Mersin, TR-10, 99010, Turkey
Aliye Ateş-Ös & Nergüz Bulut-Serin
You can also search for this author in PubMed Google Scholar
Corresponding author
Correspondence to Aliye Ateş-Ös .
Ethics declarations
Conflict of interest.
The authors declare that they have no confict of interest.
Ethics approval
The study was approved by the Ethics Committee of European University of Lefke (reference number, UEK/51/02/1920/06) and the author(s) complied with all ethical standards.
Informed consent
All participants confirmed informed consent.
Additional information
Publisher’s note.
Springer Nature remains neutral with regard to jurisdictional claims in published maps and institutional affiliations.
This article is a part of the first author’s Ph.D. thesis supervised by the second author. Cognitive flexibility and belonging among university students: Mediating role of adaptation.
Rights and permissions
Springer Nature or its licensor (e.g. a society or other partner) holds exclusive rights to this article under a publishing agreement with the author(s) or other rightsholder(s); author self-archiving of the accepted manuscript version of this article is solely governed by the terms of such publishing agreement and applicable law.
Reprints and permissions
About this article
Ateş-Ös, A., Bulut-Serin, N. Cognitive flexibility and belonging among university students: mediating role of adaptation. Curr Psychol 43 , 3234–3242 (2024). https://doi.org/10.1007/s12144-023-04577-2
Download citation
Accepted : 17 March 2023
Published : 28 March 2023
Issue Date : January 2024
DOI : https://doi.org/10.1007/s12144-023-04577-2
Share this article
Anyone you share the following link with will be able to read this content:
Sorry, a shareable link is not currently available for this article.
Provided by the Springer Nature SharedIt content-sharing initiative
- Cognitive flexibility
- Structural equation model
- Find a journal
- Publish with us
- Track your research
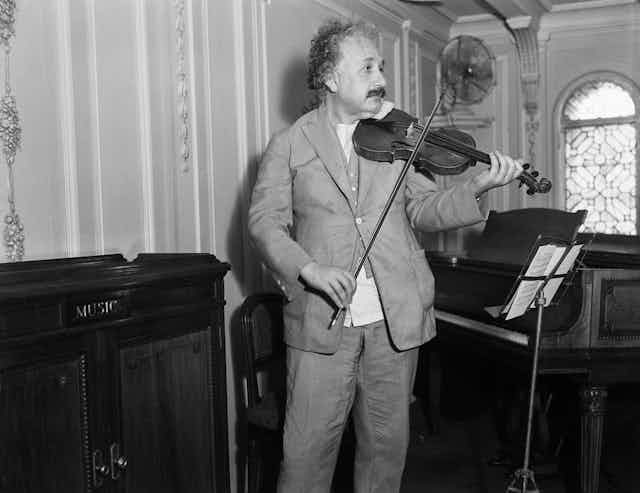
IQ tests can’t measure it, but ‘cognitive flexibility’ is key to learning and creativity
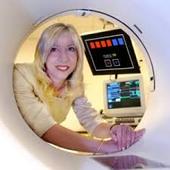
Professor of Clinical Neuropsychology, University of Cambridge
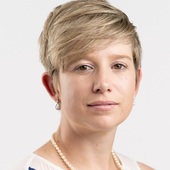
Postdoctoral Research Associate, Cognitive Neuroscience, University of Cambridge
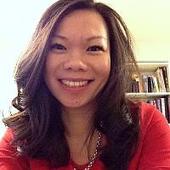
Assistant Professor of Psychology, Nanyang Technological University
Disclosure statement
Barbara Jacquelyn Sahakian receives funding from the Wellcome Trust, the Leverhulme Foundation and the Lundbeck Foundation. Her research is conducted within the NIHR MedTech and In vitro diagnostic Co-operative (MIC) and the NIHR Cambridge Biomedical Research Centre (BRC) Mental Health and Neurodegeneration Themes. She consults for Cambridge Cognition. The University of Cambridge and Nanyang Technological University Centre for Lifelong Learning and Individualised Cognition (CLIC) research project is funded by the National Research Foundation, Prime Minister's Office, Singapore under its Campus for Research Excellence and Technological Enterprise (CREATE) programme.
Christelle Langley is funded by the Wellcome Trust.
Victoria Leong receives funding from the Ministry of Education, Singapore and the Centre for Lifelong Learning and Individualised Cognition (CLIC). CLIC is supported by the National Research Foundation, Prime Minister’s Office, Singapore under its Campus for Research Excellence and Technological Enterprise (CREATE) programme.
University of Cambridge provides funding as a member of The Conversation UK.
View all partners
- Bahasa Indonesia
IQ is often hailed as a crucial driver of success, particularly in fields such as science, innovation and technology. In fact, many people have an endless fascination with the IQ scores of famous people. But the truth is that some of the greatest achievements by our species have primarily relied on qualities such as creativity, imagination, curiosity and empathy.
You can listen to more articles from The Conversation, narrated by Noa, here .
Many of these traits are embedded in what scientists call “cognitive flexibility” – a skill that enables us to switch between different concepts, or to adapt behaviour to achieve goals in a novel or changing environment. It is essentially about learning to learn and being able to be flexible about the way you learn. This includes changing strategies for optimal decision-making. In our ongoing research, we are trying to work out how people can best boost their cognitive flexibility.
Cognitive flexibility provides us with the ability to see that what we are doing is not leading to success and to make the appropriate changes to achieve it. If you normally take the same route to work, but there are now roadworks on your usual route, what do you do? Some people remain rigid and stick to the original plan, despite the delay. More flexible people adapt to the unexpected event and problem-solve to find a solution.
Cognitive flexibility may have affected how people coped with the pandemic lockdowns, which produced new challenges around work and schooling. Some of us found it easier than others to adapt our routines to do many activities from home. Such flexible people may also have changed these routines from time to time, trying to find better and more varied ways of going about their day. Others, however, struggled and ultimately became more rigid in their thinking. They stuck to the same routine activities, with little flexibility or change.
Huge advantages
Flexible thinking is key to creativity – in other words, the ability to think of new ideas, make novel connections between ideas, and make new inventions. It also supports academic and work skills such as problem solving. That said, unlike working memory – how much you can remember at a certain time – it is largely independent of IQ, or “ crystallised intelligence ”. For example, many visual artists may be of average intelligence, but highly creative and have produced masterpieces.
Contrary to many people’s beliefs, creativity is also important in science and innovation. For example, we have discovered that entrepreneurs who have created multiple companies are more cognitively flexible than managers of a similar age and IQ.
So does cognitive flexibility make people smarter in a way that isn’t always captured on IQ tests? We know that it leads to better “ cold cognition ”, which is non-emotional or “rational” thinking, throughout the lifespan. For example, for children it leads to better reading abilities and better school performance .
It can also help protect against a number of biases, such as confirmation bias. That’s because people who are cognitively flexible are better at recognising potential faults in themselves and using strategies to overcome these faults.
Cognitive flexibility is also associated with higher resilience to negative life events , as well as better quality of life in older individuals. It can even be beneficial in emotional and social cognition: studies have shown that cognitive flexibility has a strong link to the ability to understand the emotions , thoughts and intentions of others.
The opposite of cognitive flexibility is cognitive rigidity, which is found in a number of mental health disorders including obsessive-compulsive disorder , major depressive disorder and autism spectrum disorder .
Neuroimaging studies have shown that cognitive flexibility is dependent on a network of frontal and “striatal” brain regions. The frontal regions are associated with higher cognitive processes such as decision-making and problem solving. The striatal regions are instead linked with reward and motivation.
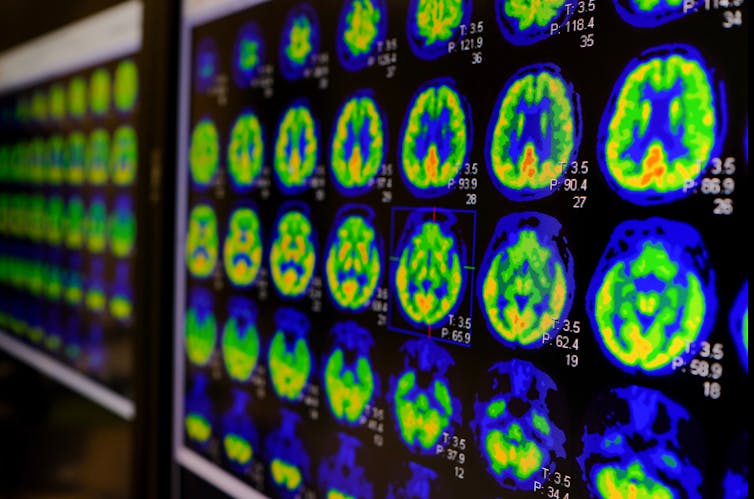
There are a number of ways to objectively assess people’s cognitive flexibility, including the Wisconsin Card Sorting Test and the CANTAB Intra-Extra Dimensional Set Shift Task .
Boosting flexibility
The good news is that it seems you can train cognitive flexibility. Cognitive behavioural therapy (CBT), for example, is an evidence-based psychological therapy which helps people change their patterns of thoughts and behaviour. For example, a person with depression who has not been contacted by a friend in a week may attribute this to the friend no longer liking them. In CBT, the goal is to reconstruct their thinking to consider more flexible options, such as the friend being busy or unable to contact them.
Structure learning – the ability to extract information about the structure of a complex environment and decipher initially incomprehensible streams of sensory information – is another potential way forward. We know that this type of learning involves similar frontal and striatal brain regions as cognitive flexibility.
In a collaboration between the University of Cambridge and Nanyang Technological University, we are currently working on a “real world” experiment to determine whether structural learning can actually lead to improved cognitive flexibility.
Studies have shown the benefits of training cognitive flexibility, for example in children with autism. After training cognitive flexibility, the children showed not only improved performance on cognitive tasks, but also improved social interaction and communication. In addition, cognitive flexibility training has been shown to be beneficial for children without autism and in older adults .
As we come out of the pandemic, we will need to ensure that in teaching and training new skills, people also learn to be cognitively flexible in their thinking. This will provide them with greater resilience and wellbeing in the future .
Cognitive flexibility is essential for society to flourish . It can help maximise the potential of individuals to create innovative ideas and creative inventions. Ultimately, it is such qualities we need to solve the big challenges of today, including global warming, preservation of the natural world, clean and sustainable energy and food security.
Professors Trevor Robbins , Annabel Chen and Zoe Kourtzi also contributed to this article.
- Intelligence
- Theory of mind
- Audio narrated

Project Officer, Student Volunteer Program

Audience Development Coordinator (fixed-term maternity cover)


Lecturer (Hindi-Urdu)

Director, Defence and Security

Opportunities with the new CIEHF

An official website of the United States government
The .gov means it’s official. Federal government websites often end in .gov or .mil. Before sharing sensitive information, make sure you’re on a federal government site.
The site is secure. The https:// ensures that you are connecting to the official website and that any information you provide is encrypted and transmitted securely.
- Publications
- Account settings
Preview improvements coming to the PMC website in October 2024. Learn More or Try it out now .
- Advanced Search
- Journal List

Intelligence IS Cognitive Flexibility: Why Multilevel Models of Within-Individual Processes Are Needed to Realise This
Damian p. birney.
1 School of Psychology, University of Sydney, Sydney 2006, Australia
Jens F. Beckmann
2 School of Education, Durham University, Durham DH1 1TA, UK; [email protected]
Associated Data
Not applicable.
Despite substantial evidence for the link between an individual’s intelligence and successful life outcomes, questions about what defines intelligence have remained the focus of heated dispute. The most common approach to understanding intelligence has been to investigate what performance on tests of intellect is and is not associated with. This psychometric approach, based on correlations and factor analysis is deficient. In this review, we aim to substantiate why classic psychometrics which focus on between-person accounts will necessarily provide a limited account of intelligence until theoretical considerations of within-person accounts are incorporated. First, we consider the impact of entrenched psychometric presumptions that support the status quo and impede alternative views. Second, we review the importance of process-theories, which are critical for any serious attempt to build a within-person account of intelligence. Third, features of dynamic tasks are reviewed, and we outline how static tasks can be modified to target within-person processes. Finally, we explain how multilevel models are conceptually and psychometrically well-suited to building and testing within-individual notions of intelligence, which at its core, we argue is cognitive flexibility. We conclude by describing an application of these ideas in the context of microworlds as a case study.
1. Introduction
One of the least disputed claims in psychology is the link between an individual’s intelligence and successful life outcomes, particularly in academia and work ( Gottfredson 1997 , 2018 ; Mackintosh 2011 ; Sternberg et al. 2000 ). Paradoxically, some of the most disputed claims in psychology concern how to define and operationalise intelligence ( Gottfredson 2018 ; Horn and Noll 1994 ). The solution to the definition-operationalisation problem has less to do with filling some sparsity of theorising, there is much to draw from ( Sternberg 2020 ). Instead, we are hamstrung by psychometric methods that are at once too flexible, too constrained, and too disconnected from substantive theory. We advocate for approaches to intelligence that are directed at within-individual processes, rather than at between-individual comparisons because they are fundamentally closer to the conceptual notion of adaptivity. Adaptivity in complex and novel situations requires rapid and flexible encoding, representation, and manipulation of relations between aspects of the physical and mental world ( Beckmann 2014 ). Our aim in this review is first to explicate a notion of intelligence in which the conceptualisation and operationalisation are jointly integrated, aligned, and directly related to how one successfully adapts to changing demands of the environment or task from one situation to the next. Second, we aim to demonstrate why a multilevel, analytic framework is critical to achieve this. To distinguish our notion from the status quo, particularly ‘g’ and Fluid Intelligence ( Gf ), we use the term “intelligence as cognitive flexibility”. We do so more as a placeholder because if it had not already lost most of its meaning ( Gottfredson 2018 ), the term intelligence would better serve our intentions.
The focus on managing changing demand is consistent with common definitions of fluid intelligence, defined as entailing “deliberate but flexible control of attention to solve novel ‘on the spot’ problems that cannot be performed by relying exclusively on previously learned habits, schemas, and scripts” ( Schneider and McGrew 2012, p. 111 ). Yet, whether one accepts this definition or another, in practice it is primarily between-individual accounts which dominate the operationalisation of virtually all variants of intelligence, Gf included. As we will argue, this first serves to relegate the identification of flexibility to unnecessarily indirect inference from tests that do not require adaptation whatsoever, and second, as demonstrated by others, it relies on a somewhat dubious extrapolation of the ergodic assumption , that causal inferences from between-individual models map directly on to within-individual mechanisms ( Borsboom et al. 2003 ; Molenaar 2004 , 2013 ).
The mechanisms of intelligence most theories draw on relates to those extensively studied by cognitive psychologists, such as memory, attention, switching, inhibitory control, and relational binding, as well as higher-order concepts such as working memory and reasoning. De Boeck et al. ( 2020 ) argue that while there was early promise in the decomposition of reasoning tasks into such component processes to investigate process correlates of intelligence (for instance, Sternberg 1977a , 1977b ), these innovations were ultimately not pursued, in part because of the emerging domination of factor analysis in theory development. That is, while these cognitive psychology constructs tend to have articulated process accounts, they were not the panacea to the conceptualisation-operationalisation misalignment of intelligence hoped for. Translating these process-focused constructs into assessments, sometimes referred to as elementary cognitive tasks (ECTs), has psychometric challenges which the traditional latent variable (psychometric) approach to intellectual abilities cannot resolve alone ( Goecke et al. 2021 ).
In this review, we aim to substantiate why the classic psychometric approach will always necessarily provide a limited account of intelligence and what might be done to redress this. The paper is structured in four parts. In Part 1 we consider the implications of three common but theoretically dubious practices that have become entrenched and serve to reinforce the status quo while impeding alternative views and potential progress. In Part 2 we review the importance of process-theories, which are critical for any serious attempt to build a within-person account of intelligence. In Part 3 we explicate the distinction between typical static tasks and dynamic tasks, which are by design focused on within-individual processes, and outline how the former can be modified to approximate the latter. Finally, in Part 4 we explain how multilevel, mixed effects analytic approaches both are conceptually and psychometrically well-suited to building and testing within-individual notions of intelligence—to narrowing the theory-operationalisation gap. We conclude by describing an application of these ideas as a case study.
We reflect on these four aspects because they are relevant to any proposition that aims to explicate a more authentic and dynamic definition of intelligence. There is a subtle but important difference between a proposition that we should take dynamic processes seriously, and a claim that traditional psychometrics are not well suited to achieve this. We necessarily address these psychometric issues in Part 1 because they are, or at least should be, the pillars of operationalisation and measurement ( Birney et al. 2022 ; Michell 1990 ).
2. Part 1: Building a Case for Intelligence as Cognitive Flexibility
2.1. entrenched assumptions.
Across the course of the history of intelligence theorising, a number of presumptions have worked their way into the collective consciousness and are now considered “knowns” ( Neisser et al. 1996 ). Many of these, we believe, have become largely dogmatic, unquestionable “facts”. We consider three; (a) the supposition of stability, (b) the belief that factor analysis of correlations alone can reveal true latent processes and attributes within the individual, and (c) the view that observed variables (i.e., test scores) must be manifestations of these latent processes, rather than seriously considering that tests scores are formative causes of latent variables. That these are typically assumptions necessary to simplify psychometric modelling, rather than being core, testable theoretical tenets, has been known for some time. A small but increasingly vocal collective are questioning not only the validity of these “knowns”, but also critically reflecting on the limitations of their utility in providing a greater understanding of intelligence (e.g., Bollen and Diamantopoulos 2017 ; Borsboom 2015 ; Conway et al. 2021 ; De Boeck et al. 2020 ; Kovacs and Conway 2016 ; Molenaar 2013 ; van der Maas et al. 2017 ).
2.1.1. Supposition of Stability
Whereas personality assessments tend to focus on typical levels, intelligence tests aim at assessing maximal performance levels ( Neisser et al. 1996 ). From this, Goff and Ackerman ( 1992, p. 538 ) suggested that the use of intelligence tests actually implies “the existence of a stable or permanent capability”. We are not arguing against the goal of assessing maximal performance, because it largely reflects what researchers and educators intentionally set out to assess going at least as far back as Binet ( 1905 )—a correlate of a nascent aptitude or cognitive potential. However, the assumption of inherent stability as a psychometric criterion, realised by concepts like test–retest reliability, is ostensibly antithetical to the notion of within-individual variability, including learning and development, and over time this has led to a set of psychometric practices well-suited to stable attributes but not systematically varying ones. In other words, if the starting assumption for mapping the assessment of a given set of intellectual attributes is that there is no or minimal within-individual variability, then stability-focused assessment and validation methods will evolve accordingly. As a result, “successful” measurement, so defined, not only risks becoming dissociated from the conceptual understanding of cognitive capabilities, our conceptual understanding may be skewed to fit our measurement assumptions.
These types of limitations of traditional psychometrics have long been recognized as overly restrictive in areas where assessment of dynamic processes is of interest, for instance, Dynamic Testing ( Grigorenko and Sternberg 1998 ; Guthke and Beckmann 2000 ), complex-problem solving ( Beckmann et al. 2017 ; Dörner and Funke 2017 ), and more recently cognitive flexibility ( Beckmann 2014 ). The point here is that the extant psychometric principles of best-test design are often challenged by constructs that are by definition dynamic, fluid, and complexly determined by transient or volatile contextual and intra-personal factors. This is what needs to be redressed.
2.1.2. The Ergodic Assumption: History Tells Us Correlations Are Not Enough; Logic Tells Us They Never Were
The individual-differences approach to the investigation of psychological attributes generally, and intellectual abilities specifically, has long been known to be incomplete without a consideration of process-oriented accounts ( Cronbach 1957 ; Deary 2001 ; van der Maas et al. 2017 ). Lohman and Ippel ( 1993, p. 41 ) citing Cronbach ( 1957 ), McNemar (1964), Spearman (1927) and others, concluded that a major reason why the individual differences approach to the study of intelligence “… was unable to achieve one of its central goals: the identification of mental processes that underlie intelligent functioning”, was because “… a research program dominated by factor analysis of test intercorrelations was incapable of producing an explanatory theory of human intelligence”.
In his presidential address to the annual meeting of the Psychometric Society, Guttman ( 1971 ) contrasted the purpose of observation in the psychometric testing tradition, which was (and generally still is) to compare individuals, with his proposed, amended purpose which was to assess the structure of relationships among observations . In effect, Guttman was arguing that if one wishes to better understand the processes of intelligence, one needs to take a distinctively within-individual perspective. Lohman and Ippel ( 1993, p. 42 ) went further and suggested that the general idea of test theory as applied statistics (i.e., psychometrics) has not only hampered the development of structural theories for the measurement of processes, but actually precluded it (see also, Deary 2001 ; Molenaar 2004 ). Borsboom et al. ( 2003 ) later made the compelling argument “that between-subjects models do not imply, test, or support causal accounts that are valid at the individual level.” (p. 214). Additionally, that therefore, within-individual level processing must be explicitly incorporated in measurement models in order to substantively link between-subject models of intellect with what is happening at the level of the individual ( Borsboom et al. 2004 ). As we will elaborate on in a later section (Part 4), like others (e.g., De Boeck et al. 2020 ), we see promise in multilevel (mixed-effects) models (MLM) for linking theory and measurement.
The claim that the structure observed at a between-individual level exists at the level of an individual is referred to as the Ergodic Assumption ( Molenaar 2004 , 2013 ). As explicated formally by Molenaar ( 2004 , 2013 ), when there is substantial heterogeneity across individuals, or in other words, when stationarity of means and covariances does not exist across time/occasions, as is true for biological systems, including that of humans, the likelihood of the ergodic assumption being true is vanishingly low. The implication of this for the current discussion (and the field in general) is that the majority of between-individual conceptualisations of intelligence, such as that represented by the Cattell-Horn-Carroll (CHC) hierarchical taxonomy ( Carroll 1993 ; Schneider and McGrew 2012 ) of human abilities, probably do not hold for most individuals. It is conceivable to say, Damian’s inductive, quantitative, and verbal attributes (narrow CHC factors) covary differently relative to Jens’; that is, their CHC “factor structures” are different. When we assess between-person CHC factors, such as inductive reasoning, quantitative reasoning, and verbal comprehension, we are making the unstated supposition that each of these attributes exists uniquely within the person we are assessing. We are certainly doing so when we plot the person’s profile of derived scores as indices of CHC factors, and then interpret their strengths and weaknesses. This is precisely the ergodic assumption as it is realised in practice. In fact, Molenaar ( 2004, p. 215 ) concludes that for nonergodic processes “there is no scientifically respectable alternative but to study the structures of [within-individual variability] and [between-individual variability] for their own sake”. Of course, there are subdisciplines of researchers who have devoted considerable energies to each. Cronbach ( 1957 ) referred to them as experimentalists and correlationalists and argued that there will always remain questions that “Nature will never answer until our two disciplines ask [them] in a single voice” (p. 683).
2.1.3. Ontological Status of Reflective vs. Causal- and Composite-Formative Concepts
The common factor-analytic/SEM model on which CHC is based is a reflective one, where individual differences in observed variables (and latent variables in hierarchical models) are considered effect-indicators of the latent attribute of interest 1 . That is, the variance in scores on the observed indicators represents effects that are caused by the latent variable. An alternative is to consider causal formative models, where observed variables (and latent variables) are cause-indicators . Here, variation in the resulting latent variable is caused by the indicators. Thus in formative models, the latent variable represents the indicators’ shared contribution in some collective way ( Bollen and Diamantopoulos 2017 ; Kovacs and Conway 2016 ).
Formative models have typically not been broadly adopted by intelligence researchers (cf., Kovacs and Conway 2016 ), in spite of the fact they have been known since at least the 1960s (see Blalock, H.M, 1963, cited in Bollen and Diamantopoulos 2017 ). Bollen and Diamantopoulos ( 2017 ) suggest this is in part due to an historical entrenchment of thinking in terms of reflective models. This is not particularly surprising since theorisation is typically targeted at individual-centred processes that are intuitively reflective in nature, but such claims should be tested, not assumed. Bollen and Diamantopoulos review seven common criticism presented against the appropriateness of using formative indicators. They conclude each criticism is either invalid or represents issues shared by reflective indicators. Importantly for our purposes, the authors demarcate the difference between causal -formative and composite -formative indicators in terms of conceptual-unity, a distinction they argue is often ignored or misunderstood. When corrected, this leads to a straightforward discounting of the core criticisms and their basic tenets. Bollen and Diamantopoulos ( 2017 ) demonstrate that latent variables derived from models of causal-formative indicators which have what they refer to as conceptual unity , can be considered as measures 2 , analogous to reflective latent variables. Conceptual unity exists when each indicator matches “the idea embodied by the concept” (p. 584). How precisely this is achieved is not clear; it is an aspect of the theorising needing further explication. However, according to Bollen and Diamantopoulos, composite-formative indicators do not require conceptual unity, and therefore composite variables are not measures, they are not latent variables, and neither are the indicators causes of the composite variable. Composite variables may have utility as a summary of the multiple variables in a predictive sense but not an explanatory one.
The demarcation between a composite vs. causal indicator is difficult to resolve. The identification of trait-complexes ( Ackerman et al. 2013 ) present a potentially illustrative case in point. Ackerman and Heggestad ( 1997 ) proposed that there are four trait-complexes, two of which are represented in Figure 1 (left panel), that each encompass an overlapping set of different traits from the domains of personality, abilities, and interests (additional trait-complexes were subsequently included, see Ackerman et al. 2013). “Validity” of trait-complexes is purportedly evidenced by their differential prediction of domain-specific knowledge acquisition. For instance, the intellectual/cultural trait-complex was captured by Gc and ideational fluency abilities, artistic and investigative interests, and absorption, openness, and typical-intellectual engagement personality dimensions.

Schematic representation of the Intellectual/Cultural and Social trait-complexes proposed by Ackerman and Heggestad ( 1997 ). Left panel describes theoretical account; Right panel represents a reflective model of the intellectual/cultural trait-complex.
For instance, indicators of Openness (i.e., items) have conceptual unity necessary (but not sufficient) for measurement, because they are bound by the definition of the openness concept. However, although Ackerman et al. ( 2013 ) modelled trait-complexes as reflective latent traits as represented in Figure 1 , it is reasonable to question whether they are formative (and therefore the purple arrows in Figure 1 should point to the trait-complex, rather than from it). If they are formative, then the next question is whether the indicators (i.e., personality, interests, and ability factors) together have sufficient conceptual unity necessary for the resulting trait-complexes to serve as latent variables (i.e., are causal-formative) or not (i.e., are composite-formative).
According to Bollen and Diamantopoulos ( 2017 ), while there are tests to determine whether a concept is likely reflective or formative, whether one treats a concept (such as a trait-complex) as causal- or composite-formative is an ex ante decision the researcher makes via an empirically substantiated theoretical claim 3 .
Our previous attempts at conceptualising cognitive flexibility as a meta-competency ( Yu et al. 2019 ) has similar formative features. In this work, we surmised that there is a case for considering cognitive flexibility as a meta-competency to unify cognitive, conative (e.g., meta-cognitive) and situational dependencies, rather than thinking of cognitive flexibility simply as a facet of a broader flexibility attribute, as it is frequently conceived. Like the argument for trait-complexes, flexibility as a meta-competency is framed as a formative concept, but one that is probably composite in nature. The reason for classifying it as such, is that the theoretical boundaries for the meta-competency are still to be fully mapped and measurement properties still need to be better understood. Currently as it stands, while its indicators are internally coherent and (historically) considered reflective, as a set they lack sufficient conceptual unity.
The notion of Complex Problem Solving ( Dörner and Funke 2017 ) also has many features of a composite-formative model. This is evident when one considers how it is conceptually defined, as demonstrated in the excerpt from Dörner and Funke ( 2017, p. 6 ) in Figure 2 . We highlight 13 distinct components that relate to the theory of complex problem solving. Whether these components have sufficient conceptual unity to be anything other than composite-formative is not a statistical question, but rather intrinsically a theoretical and empirical one. That is, the ontological status cannot be assumed.

Excerpt from Dörner and Funke ( 2017, p. 6 ) showing distinct components (our enumeration and underlining) likely to define a composite-formative variable in the Bollen and Diamantopoulos ( 2017 ) framework.
Thinking more broadly, one might further postulate that other “intelligences”, like practical intelligence or cultural intelligence, or even operational intelligence, coined by Dörner ( 1986, p. 290 ) in relation to complex problem-solving competencies, and defined as “the factors that determine the cognitive processes commonly labelled as flexibility, foresight, circumspection, systematic planning…”, are similarly defined conceptually with formative characteristics. This is not to disparage these or our own theories and models as being of lesser worth, it is simply being true to our understanding of the nature of the concept under investigation 4 . In summing up their commentary, Bollen and Diamantopoulos ( 2017 ) conclude that it does not matter too much whether the ontological basis of our theories are reflective or formative, the important scientific point is that researchers carefully “define their concept, choose corresponding indicators, and consider whether the indicators depend on or influence the latent variable” (p. 594, our emphasis). In our view ontological considerations are critical. This is because the proliferation of new latent variables unthinkingly assumed to be reflective, has obscured rather than illuminated our understanding of underlying processes.
To conclude this section, we make note of Process Overlap Theory (POT), a recent causal-formative account of intelligence ( Kovacs and Conway 2016 ). According to Conway et al. ( 2021, p. 1 ) much of the motivation for POT is a growing dissatisfaction with the impediment to theory building caused by the disconnect between psychometrics and psychological theories, and problematic inferences related to the status of latent variables. Their argument is that the typical latent variable account, based in reflective SEM models where the latent variable is assumed to causally determine (i.e., is manifested in) individual differences in observed test scores, overlooks the real possibility that the emergence of a latent variable from such statistical approaches is an epiphenomenon of the fact that different tasks share different common processes, as represented statistically by causal-formative models. This is consistent with the work of van der Maas et al. ( 2006 ) who demonstrated that reciprocal mutualism between processes sufficiently explains positive manifold without the need to introduce a reflective latent attribute, such as ‘g’. Importantly however, Fried ( 2020 ) has demonstrated that network models are not necessarily differentiable from reflective models in terms of explained variance. Thus, simply moving to a formative account (or even a network one) is not sufficient. The burden now rests with the researcher to explicate the specific processes entailed.
2.2. Summary of Part 1: Why Intelligence Theorising Has Survived However, Failed to Thrive
In Part 1 we have presented a review of a small selection of entrenched assumptions that have stymied intelligence theorizing. In doing so, the central point of our argument is that we have focused for too long on between-individual comparisons and too willingly tolerated inconsideration of within-person accounts.
Psychometric tests of intelligence have great utility in predicting interesting (and important) outcomes, and pragmatically the common-factor analyses of correlations works well in this regard. One might be tempted to therefore ask, what are the implications of not redressing the limitations reviewed in Part 1? This is our response so far. First, if we do not question the supposition of stability, we risk over-looking (and not assessing) adaptive, situation-contingent, within-person differences. This risks limiting our understanding of the dynamic features of intelligent behaviour in applied settings, such as work and education. Second, we reviewed analyses that demonstrate assuming within-person accounts follow from between-person theories, that is, assuming ergodicity, is logically untenable. The ergodic claim assumes stationarity of means and covariances across time within the individual, and this is largely untenable in practice, further contributing to the argument for testing the supposition of stability. Third, we reminded readers that the between-person theories themselves are often based on an untested assumption of reflective models, that differences in the indicators are caused by differences in the latent variable (arrows going from the latent variable to the indicators). The alternative, formative claim, that indicators are causing differences in the latent variable (arrows going to the latent variable from the indicators) is rarely tested, and when it is, the respective models often account for as much variance as reflective models, so the choice can easily be driven by pragmatism and inertia.
When we scratch the surface, it is apparent that between-person models of intellect have little explanatory value and thus their pragmatic benefit and descriptive utility rests on a theoretically shallow house of cards. To address the challenges presented by these and other types of entrenched assumptions, we need a grounded process theory of intelligence. In the following we map out some of the requirements needed for a within-person approach, admittedly in somewhat of a selective way.
3. Part 2: Requirements for A Within-Person Approach to Intelligence
“ It is true that the components of individual differences have often been interpreted in terms of cognitive processes, but such an interpretation does not logically follow. The interpretation is necessarily a post hoc interpretation based on the assumptions that processes are directly reflected in individual differences in performances and that correlation between performances defining a factor indicates that a common process is involved. ” ( De Boeck et al. 2020, p. 58 )
3.1. Process-Oriented Accounts
Following the arguments of Molenaar ( 2004 , 2013 ) and Borsboom et al. ( 2003 , 2004 ), the ergodic assumption in psychology is tenuous at best, and all between-person models are variously imperfect accounts of what is likely to be occurring within an individual. Taking the call for the study of within-individual variability in its own right seriously ( Molenaar 2004 ), where does one begin to map out a process-oriented account? The obvious choice is with working memory, and we will consider what current conceptualisations of working-memory theory have to offer. However, it turns out the notion of complexity is a compelling first place to start because of its already deep links with intelligence theory.
3.1.1. Complexity as the “Ingredient” Process of Intelligence
Theorising within the psychometric intelligence tradition is not completely devoid of attempts to understand processes. Arguably the most developed is based on the notion of complexity , and the observation that performances on tasks, occupations, and work that are more complex, broadly defined, tend to be more highly correlated with intelligence. The ensuing supposition is that intelligence entails a capacity to deal with complexity ( Gottfredson 2018 ). Following from this, an independent indicator of complexity is changes in correlations with, or loadings on, measures of intelligence that are concomitant with changes in task complexity ( Arend et al. 2003 ; Spilsbury et al. 1990 ; Stankov and Cregan 1993 ), but all else being equal, not with changes in difficulty generated by other task features ( Birney and Bowman 2009 ; Stankov 2000 ). Birney ( 2002 ) referred to this criterion as psychometric complexity .
Complexity vs. Difficulty
To understand why complexity is of such value in the conceptualisation and assessment of intelligence, it is necessary to take a brief diversion to distinguish it from difficulty ( Beckmann et al. 2017 ). Difficulty is atheoretical, in that a rank-ordering of test items that are solved by fewer and fewer people tells us little about what make items difficult, just as correlations alone, we will argue, tell us little about complexity. Difficulty is a statistical concept captured by indices such as the proportion of people who answer an intelligence test item correctly. Complexity is a cause for the difficulty one experiences, in that it is a consequence of the cognitive processes demanded of the task at hand.
While complexity is often equated to difficulty, there are certainly tasks that are not difficult yet predictive of intelligence. For instance, the well-known, perceptual inspection time task ( Deary 2001 ) appears to impose minimal storage or processing load, yet is a good predictor of Gf . Similarly, performance on the relational monitoring task ( Bateman et al. 2019 ; Chuderski 2014 ) is highly predictive of Gf , but the reasoning and memory demands are ostensibly minimal. Complexity is more nuanced and entails systematic manipulations based on a structural process hypothesis regarding differential demand on ability ( Lohman and Ippel 1993 ). That is, complexity is a causal-formative concept that is indexed by performance across task manipulations that have conceptual unity. It is conceptualised first and foremost as a quality that is determined by the cognitive demands that characteristics of the task and the situation impose, and because of this, it is psychologically substantive. Accordingly, manipulations monotonically ordered by complexity are manipulations of monotonically increasing demand on the psychological attribute ( Birney et al. 2019 ). Only in a truly pure, unidimensional task will the complexity continuum coincide with the difficulty continuum. Of course, such tasks do not exist. However, with careful, theory-driven task analyses, the parameters of complexity can be formalised and investigated ( Beckmann 2010 ; Birney and Bowman 2009 ; Ecker et al. 2010 ; Goecke et al. 2021 ; Halford et al. 1998 ).
Differential complexity correlations are a plausible, necessary criterion of an increase in cognitive demand. However, there are some statistical and theoretical challenges to be flagged. Statistically, by definition, the magnitude of a correlation coefficient is influenced by the upper-bound variance of their component measures, and variances in ability tasks are influenced by statistical difficulty. Due to restrictions of range, all else equal, tasks that are of average statistical difficulty will have a higher upper-bound variance than both easier or more difficult tasks, attenuating correlations in both the latter cases. In practice, easier and harder tasks may appear “less” complex than they really are. Whether the “shrinkage” of random-effects in multilevel models (which we describe in Part 4) serves to bring extreme observations toward the fixed-effect (i.e., toward the mean intercept or slope), or the “task purification” of latent variable SEM models are useful ways to address this statistical limitation needs further investigation.
Theoretically, once again, appropriateness of complexity correlations assume we have a sufficiently detailed process-account of the latent attribute to inform a causal statement of how the complexity manipulation demands a concomitant investment of concordant intellectual processes ( Sternberg 1977b , 1980 ). That is, while we have a theoretical cause (complexity) and a way to assess its effect (correlations), alone it provides little understanding of antecedents—anything that leads to increased correlations with intelligence is presumably a complexity manipulation. In response to this ambiguity, an early approach to incorporate theory was to consider performance under competing task conditions ( Fogarty and Stankov 1982 ) or by increasing the number of mental permutations required to successfully solve a set of reasoning tasks (e.g., Schweizer 1996 ; Schweizer and Koch 2002 ; Stankov 2000 ; Stankov and Crawford 1993 ). Such manipulations were shown to also lead to increases in correlations with Gf , and hence was presented as further evidence of the importance of complexity.
Birney et al. ( 2019 ) defined psychometric complexity more formally and generally as the extent to which within-individual differences in task performance across theoretically substantive complexity manipulations differ as a function of between-individual differences in that attribute. In multilevel models, this is a cross-level interaction. That this is the case, explicates a possible conceptual definition, operationalisation, and assessment of intelligence as cognitive flexibility that is formally aligned and testable within a common methodological framework. We discuss this further in Part 4.
3.1.2. Working-Memory Accounts of Intelligence
Investigations of processes in individual differences research has had a strong focus on understanding mechanisms underlying working memory (WM) in and of itself (e.g., Ecker et al. 2010 ; Goecke et al. 2021 ; Oberauer and Lewandowsky 2016 ), or as a set of processes common to both WM and Gf (e.g., Ackerman et al. 2005 ; Engle et al. 1999 ; Oberauer et al. 2007 ; Shipstead et al. 2016 ). What is common in many of the studies and approaches described in the rest of this section is the combined experimental-correlational methodology—basic processes are proposed, operationalised as individual differences variables and “measured”, and then “validated” as incremental predictors of the latent attribute (e.g., WMC or Gf ). The latent variables representing these attributes are defined and operationalised using the traditional reflective procedures we have described. The supposition is that the more variance the proposed processes predict in the latent WM or Gf variable, the more we know about working memory or intelligence. The view we advocate is that this approach, while rightminded in explicating process accounts, is incomplete.
In terms of WM-focused studies, consider for instance Ecker et al. ( 2010 ), who sought to map processes underlying working memory updating. Following a task analysis of a set of commonly used updating tasks, they identified three component processes, retrieval, transformation, and substitution. Using a modified version of the memory updating task, they manipulated the absence or presence of each component experimentally, and used multilevel, mixed-effects modelling to test theoretically specified contrast hypotheses (this is similar to the costs approach used by Bateman and Birney ( 2019 ) to identify a link between relational integration demand and Gf , which we will describe shortly). Ecker et al. first demonstrated that the WM updating components were distinct and additive in predicting task response times and accuracy (there were no observed interactions between the components). In the second part of the Ecker et al. study, a bi-factor SEM model tested and confirmed differential associations of the three WM updating components with an independently defined (reflective) latent WMC factor.
In a recent study investigating the role of the working-memory binding hypothesis, Goecke et al. ( 2021 ) combined an experimental manipulation of complexity of elementary cognitive tasks (ECTs), also using a bi-factor SEM approach to identify the mechanisms underlying binding demands (e.g., more stimulus-response mappings = greater binding demand) on working memory capacity. This was achieved in a three-step process. First, given ECT performance is typically differentiated more by response latency rather than accuracy, performance indices were derived using drift diffusion modelling. In total, standardized drift rates were derived for 12 indicators, 3 speed tasks (change-detection, stimulus comparison, substitution) by 2 modalities (selected from either letter, figure, or number modality) by two binding complexity levels (low and high). Second, a bi-factor SEM was run where all 12 indicators were freely allowed to load on a general speed factor, and only the six high complexity binding indicators defined the specific binding factor. Third, these two process factors were then regressed on an independently derived WMC latent factor. The results suggest that both the general and high-binding factors were comparable and significant unique predictors of WMC, together explaining 66.5% of the variance in the latent WMC factor.
In terms of combined Gf and WM studies, Unsworth and Engle ( 2007b ) for instance reported a complexity effect with Gf in simple-span tasks using a combined experimental/individual-differences approach. The authors demonstrated that as the number of to-be-recalled elements increases in simple-span memory tasks to supra-span levels, determinants of performance become more like complex-span WM tasks, in that there was an emergence of a monotonic increase in correlations with Gf as a function of list-length. Shipstead et al. ( 2016 ), building on this and other extensive theorising (e.g., Engle 2002 ; Engle et al. 1999 ; Unsworth and Engle 2007a ), proposed that the link between WM and Gf has to do with the engagement of executive attention for maintenance and disengagement processes of information held in the focus of attention. Importantly in the Shipstead et al. ( 2016 ) conceptualisation, these executive processes do not simply covary with Gf , but rather are ontological to both WM and Gf. This is such that Gf and WM tasks require executive attention of both maintenance and disengagement, but to different degrees. They argue disengagement is more critical to Gf tasks, whereas maintenance is more critical for WM tasks. Additional work has investigated a range of different WM tasks and their relations to Gf , such as inhibition of lure trials in the updating n-back task ( Burgess et al. 2011 ; Gray et al. 2003 ).
While WM processes are important aspects in Gf tasks, they are not the only aspects important to intelligence. For instance, Sternberg ( 1977a ) identified encoding, mapping, and application processes (“components” in his parlance) underlying analogical reasoning. From a task analysis perspective, understanding reasoning and novelty processing is also important, and theories of complexity in terms of processing capacity limits (e.g., Halford et al. 1998 ) are well positioned to progress further investigations ( Birney and Bowman 2009 ).
3.1.3. Relational Binding and Integration Accounts of Intelligence
One way of thinking about how processing capacity limits are related to complexity is in terms of relational binding and relational integration demand. Oberauer and colleagues (e.g., Oberauer 2021 ; Oberauer et al. 2000 ) suggest a set of working-memory mechanisms by which a coordinate system binds relational information between content (say, for instance, a mountain and mole hill) and contextual information (a size comparison) to facilitate action on a specific mental representation to derive a response (e.g., the mountain is larger). Limitation on accessibility of chunks is determined by constraints on the capacity of the focus of attention and priming in the region of direct access ( Oberauer 2013 ).
Relational integration and precursor processes associated with relational binding are also thought to underly the associations between WM and Gf. We have used relational complexity (RC) theory to parameterise the cognitive demand of relational integration ( Bateman and Birney 2019 ; Bateman et al. 2019 ; Birney and Bowman 2009 ; Birney et al. 2012 ; Gabales and Birney 2011 ). RC theory is based on the premise that the limits of WM can be understood in terms of the complexity of to-be-instantiated relations ( Birney and Halford 2002 ; Halford and Wilson 1980 ; Halford et al. 1998 ; Halford et al. 2010 ). A binary relation entails two arguments, as in the relational concept: LARGER-THAN(mountain, mole hill). A relation is instantiated through the binding of a value to an argument-slot, such as “mountain” to the larger-than argument; and separately “mole hill” to the implied smaller-than argument-slot. The relation exists only in its integrated form. It is thought that the typical limit of human capacity is a quaternary relation, an example of which according to Halford et al. ( 2007 ), are proportional analogies in the form of A:B :: C:?.
Application of RC theory led to the development and validation of a class of relational integration measures known as Latin Square Tasks (LST) ( Birney et al. 2006 ). A Latin Square entails a k × k matrix with k different element types distributed such that each element exists only once in each row and column. Experimental manipulations of partially completed LS are in terms of (a) relational complexity (relational integration of 2, 3, or 4 dimensions) and storage load (number of interim solutions to be maintained) ( Birney and Bowman 2009 ; Birney et al. 2006 ); (b) presentation format (with and without time-limits) ( Hearne et al. 2019 ) (c) dynamic-completion (recording of non-target-cells as external-memory aid to mitigate memory demand and isolate binding) ( Bateman et al. 2017 ); and LST dimensionality (4 × 4 LST, requiring only a shape response, and a 5 × 5 Greco-LST which superimposes two LSTs integrating shape and colour) ( Birney et al. 2012 ; Gabales and Birney 2011 ). Each of these within-task manipulations were theoretically designed to tap specific aspects of Gf ; they have been shown to be differentially and incrementally predictive to varying extents.
RC has also been useful to inform manipulations of relational binding in cognitive processing load in the Arithmetic Chain Task (ACT) ( Bateman and Birney 2019 ) and the Swaps task ( Bateman 2020 ; Stankov 2000 ), where systematicity plays out differently in each, giving further insights into underlying within-individual mechanisms. For each trial in the experimental conditions of the ACT, participants are given 6s to study a to-be-recalled mapping of letters to numbers (Screen1: A = 2, B = 4, C = 1). They are then given new mappings that are either in a systematic order (Screen2: X = A, Y = B, Z = C) or a random order (e.g., X = B, Y = C, Z = A), and need to use this derived mapping of numbers on to X, Y and Z to complete a chain of simple arithmetic (Screen3:, e.g., 5 − 4 + X + 2 − Y + Z = ?). Systematicity inherent in natural-ordering facilitates chunking of relationally bound elements (ABC = 241 = XYZ), which aids number recall to complete the arithmetic. Random (or non-systematic) ordering stymies chunking (ABC = 241 = ZXY). Using multilevel models, the within-individual cost of performance in the non-systematic condition (relative to a control condition with no mappings) was shown to be moderated by Gf , but not for the systematic condition ( Bateman and Birney 2019 ). The interpretation is that sensitivity to systematicity and capacity to build strong flexible bindings in disordered contexts (ABC = 241 = ZXY) is an important Gf process.
The Swaps task requires mental permutation and updating and presents participants with a letter triplet (e.g., JKL) with instructions to mentally rearrange or ‘swap’ the positions of letters (e.g., Swap 1 and 2; then Swap 3 and 2) and report the final ordering (i.e., KLJ). As indicated previously, Stankov and Cregan ( 1993 ) have demonstrated the greater the number of mental permutations the higher the correlation with Gf . Bateman ( 2020 ) modified the Swaps task to target binding systematicity designed to emerge over the multiple swaps required within items. For example, given [TQXBL] the required solution path with swap instructions is: Initial order [TQXBL]; Swap 1 with 2 = [QTXBL]; swap 3 with 2 = [QXTBL]; swap 1 with 3 = final order [TXQBL]. The intended systematicity is that B and L can be chunked because they are never swapped and this is not pointed out to participants; and sensitivity to this facilitates performance. Based on the ACT findings of Bateman and Birney ( 2019 ), one might predict that performance in the intuitively more difficult, non-systematic condition would be more predictive of Gf . However, preliminary data provided by Bateman ( 2020 ) indicated the opposite—performance was moderated by Gf when systematicity was present , but not when it was absent. This suggests that sensitivity to systematicity over time is also a feature of Gf .
As a relevant aside, the notion of fluid intelligence comprising the ability to utilise structure (where and when available) in conjunction with the result of poorer performance in the non-systematic condition resonates with findings in relation to the so-called semanticity effect in complex problem solving ( Beckmann 1994 ; Beckmann et al. 2017 ; Beckmann and Goode 2013 ). Here, the presence of semantically laden labels for system variables negatively affects knowledge acquisition as well as system control performance. This effect is caused by relying on a false sense of familiarity which is triggered by the variable labels rather than systematically testing assumptions. In other words, the apparent lack of systematicity when interacting with the system results in not utilising available cognitive resources, which is reflected in lower correlations between Gf and CPS performance shown under high semanticity conditions in contrast to CPS performance shown under low semanticity conditions.
Together, the ACT and the Swaps data support conceptual definitions of Gf as entailing both a capacity for binding sensitivity to systematicity and managing disorder through building and maintaining strong yet flexible bindings. The standard between-person approach tells us that both tasks correlate with Gf to the same extent ( r ~ 0.40); the within-individual approach provides additional insights by suggesting they do so for different reasons, supporting our argument that understanding within-individual processes is critical to intelligence as cognitive flexibility.
3.2. Summary of Part 2: Why WM Theory Is Important to Within-Person Process Accounts
In Part 2, we outlined the historical importance of the concept of “complexity” in intelligence theorising and made a distinction between difficulty as a statistical entity and complexity as a theoretical concept. While there are pragmatic challenges in operationalising this distinction, we alluded to the promise of MLM, when clearly specified process accounts are incorporated into the operationalisation. In this respect, we reviewed seminal process accounts of WM in relation to fluid intelligence, and more recent advances in terms of the cognitive models that formalise the role of relational binding and integration. In particular, we highlighted exemplar research that has incorporated process-accounts in SEM modelling (e.g., bi-factor analyses). The core point is that because of the limitations outlined in Part 1, process accounts are needed for any theory that wishes to take within-individual differences seriously. In our view, the process accounts reviewed in this section provide an excellent place to start.
4. Part 3: Theory through Task Analysis
While the work so far presented certainly takes a process account, there are two issues left unaddressed. First, the tasks investigated are not dynamic and nor do they necessarily allow for within-task adaptation to changing conditions. Second, the “validity” criterion used are predominantly non-dynamic measures of WM and Gf . To validate an operationalisation of intelligence as cognitive flexibility in a traditional way (i.e., through statistical associations), one needs an appropriate dynamic criterion measure. The standard approach would be to predict a real-world outcome where “cognitive flexibility” is assumed to be required, and to then check for incremental prediction of this outcome over and above classic measures of Gf . This is the approach used for validating CPS tasks, and other “alternative” measures of intelligence. This seems conceptually the right thing to do, however defining what is appropriate is not straightforward, although the necessary steps are clear. First, one must resist the pragmatics of relying on readily available quantified criteria (i.e., statistical association) without reflection on their conceptual and operational quality. If one relies on such atheoretical approaches there are two possible outcomes: (1) there is a correlation of some size and we happily conclude we have valid “measurement”, or (2) there is no (or unsatisfactory) correlation, and conclude the criterion was not good enough, but that our “measurement” might be saved from negative evidence while we search for the right criterion. A more systematic approach is needed. In response to these sort of challenges, we begin by distinguishing between features and dimensions that differentiate static vs. dynamic tasks, and consider how the former might be modified to emulate the latter.
4.1. Static Tasks
Static assessment tasks have several common characteristics. They (a) focus on the accuracy or speed of a one-off response; (b) follow classic psychometric principles closely, particularly the notion of item stability as the foundation of measurement consistency and test development; (c) assume local independence of items, whereby items are ostensibly interchangeable ( Pedhazuer and Schmelkin 1991 ), and (d) item-specific feedback is not provided (as this would jeopardise (b) and (c)). Due to these properties, performance in static tests is typically operationalised as an aggregate of item accuracy (e.g., proportion of correct items) or response time. Whilst static tasks may be psychometrically desirable, they are conceptually inadequate when it comes to dynamic concepts such as intelligence as cognitive flexibility. Static tasks can be made dynamic by focussing on the variability (in accuracy/speed) caused by systematic within-task manipulations. This can be achieved in a number of ways, we discuss two general approaches that entail (a) redesigning tasks to entail structured within-task manipulations, and (b) through interposition of idiosyncratic information to the existing task.
4.1.1. Theoretically Substantiated Within-Task Manipulation
When items are designed to be differentially sensitive to the structure of specific underlying cognitive processes, they are fundamental and not interchangeable in relation to items of a specifically, different type. Performance is conceptualised as a function of this predefined structural relationship, the simplest being a relation of difference. This is a standard approach for identifying processes as we have already outlined (e.g., Ecker et al. 2010 ). One’s capacity to learn can also be modelled as changes in performance from one item to the next in linear and non-linear ways, controlling for other task and person characteristics—that is, item-order is the relational structure. Using an MLM approach, Birney et al. ( 2017 ) investigated correlates of performance and item-order experience trajectories across the 36 items of Raven’s Advanced Progressive Matrices test. Similar approaches to item-order effects have been conducted by Schweizer and colleagues (e.g., Schweizer 2009 ; Schweizer et al. 2015 ). The relational structure can also be variable and nuanced. For instance, using Bayesian methods, Cripps et al. ( 2016 ) separately and jointly modelled the probability of an individual to spiral monotonically into poorer performance during a natural decision-making task, which are sometimes referred to as microworlds, if and when they reached an idiosyncratic motivational threshold (as opposed to an ability threshold). Birney et al. ( 2021 ) report on preliminary work extending Cripps et al.’s to model spiral and recovery trajectories in the n-back task.
4.1.2. Within-Task “Interposition”
Static tests can also be made more dynamic through interposition of information during a task that intentionally serves to focus problem solving on one or more item characteristics. This can be in the form of feedback, such as simple accuracy feedback, or a more specific strategy/hint, such as “consider how colours change” in a series-completion task. Provision of feedback designed to change performance is one of the defining features of the dynamic testing paradigm ( Guthke and Beckmann 2000 ), but other forms of prompting may also change the way people approach problems. While the intention of such manipulations is to focus assessment on dynamic processes rather than static ones, an important theoretical implication of interpositions is that they may impact the validity of the assessment in unintended ways ( Birney et al. 2022 ; Double and Birney 2019 ). Careful theorising and experimentation are necessary to ensure validity claims can be defended. Our approach is to base interposition manipulations on a process account of intelligence as cognitive flexibility.
4.2. Dynamic Tasks
The main characteristic of dynamic tasks—as they have been employed in the context of complex problem-solving research and the assessment of learning ability—is their operational focus on within-person performance variability. The definition of Dynamic Testing, for instance, characterises it as a methodological approach to psychometric assessment that uses systematic variations of task characteristics or situational characteristics in the presentation of test items with the intention to evoke intra-individual variability in test performance ( Beckmann 2014 ; Elliott et al. 2018 ; Guthke and Beckmann 2000 ). In so-called learning tests the dynamic nature of assessment is realised by providing test takers with the opportunity to demonstrate their receptiveness to scaffolded, error-specific thinking prompts after an incorrect response to a test item. Complex problem solving can also be conceptualised as dynamic testing ( Beckmann 2014 ) as it also embodies various forms of dynamics. These include (a) the feature of system feedback (e.g., whether the system state changes towards the set goal state as a consequence of the problem solver’s intervention), (b) the implementation of so-called autonomic changes in the system behaviour (i.e., the state of system variables changes independently from the problem solvers inputs), but also (c) the necessity for knowledge-acquisition (rule-learning) on which subsequent system control (rule application) relies (Goode and Beckmann 2010).
In short, dynamic tasks have two or more dimensions of performance, entail fluid and divergent processes, and are multi-phasic (rather than multi-dimensional) across time/occasion and across the external (task context) and internal (cognitive process) problem-space. Dynamic processes are present to some extent in existing flexibility and switching tasks ( Miyake and Friedman 2012 ), but as we have just outlined, are arguably better represented in complex problem solving (CPS) and microworld tasks ( Dörner and Funke 2017 ; Funke et al. 2017 ), which as also argued above, may have a formative nature as complex-problem solving competencies. We consider each of these paradigms next.
4.2.1. Set-Switching and Card Sorting
The well-known set-switching paradigm entails learning and applying a set of conditional rules. For instance, the screen location of a stimulus (left/right) might be associated with a Y/N response conditional on a particular stimulus feature (colour/shape), for example: “Y if stimulus is on left and green, else N; Y if stimulus is on right and circle, else N”. Performance requires rule-set acquisition, conditional response-switching, and inhibition (e.g., not pressing Y when a green square is on the right). Performance is a function of a response-latency cost for switch trials relative to repeat trials. While the basic cognitive psychology switching research tends not to consider individual differences (cf., Ravizza and Carter 2008 ), it has been useful as a metaphor of higher level shifting of perspectives, as might be necessary in novelty processing ( Beckmann 2014 ; Diamond 2013 ), or as formative indicators for higher level flexibility concepts. The Wisconsin Card Sorting Task requires one to sort cards one at a time based on a core attribute (colour, shape, numerosity). Unlike set-switching, the sorting rule is not known in advance, rather it needs to be deduced from feedback. This rule (say, sort by colour) will persist across multiple trials and then change without forewarning to a different rule (say, sort by shape). Preservative sorting in the face of negative feedback indicates a lack of cognitive flexibility. Recent computational modelling research has shown the diagnostic value of deriving alternative assessment metrics from well-known neuropsychological tasks, such as these. For instance, Steinke and Kopp ( 2020 ) demonstrated that a reconceptualisation of Wisconsin Card Sorting Test metrics show promise in clinically differentiating Parkinson and ALS conditions. It is important to note that while parameterizing task performance using computational methods can lead to effective prediction/diagnosis, it is not given they will also lead to sufficient theoretical understanding necessary to design interventions.
4.2.2. Complex Problem Solving (CPS) and Microworlds
CPS tasks present participants with an explicit opportunity to acquire knowledge and to control and manage changes in a complex system by allowing direct experimentation ( Dörner and Funke 2017 ; Funke 1998 ). CPS tasks vary from high-fidelity microworld simulations with many inputs and outputs (e.g., flight simulators), to “ minimal complex systems ” (MCS) which present the simplest possible interaction of variables (ie, deterministic and linear) ( Funke et al. 2017 ). CPS tasks having conceptual links with intelligence and decades of successful application in training and education ( Wood et al. 2009 ). However, they are often discounted as intelligence measures because of the challenge in extracting psychometrically reliable and valid performance indicators that correlate sufficiently with static tests of intelligence ( Beckmann and Guthke 1995 ; Greiff et al. 2015 ; Stadler et al. 2015 ). Consistent with others ( Funke et al. 2017 ), we argue that emphasis on classic psychometric qualities has led to an advocacy for MCS-like tasks, a reduction in multi-phasic task complexity, and questionable validity as tests of “true” CPS ability ( Beckmann et al. 2017 ). As indicated previously, it is feasible that typical summary scores from CPS represent a composite-formative concept, and according to Bollen and Diamantopoulos ( 2017 ) are not measures. This is not necessarily an insurmountable problem. We have argued that a sufficiently detailed task analysis and experimental manipulations, causal and effect-based concepts can be specified and extracted as measures ( Beckmann 2019 ; Birney et al. 2018 ).
4.3. Summary of Part 3: Why Task-Analysis Is Important
A tacit “known” we have not previously mentioned is the mantra that one should “validate” new measures of intelligence by assessing how well they correlate with existing ones. This not only leads to new tests functioning much like old ones, but also results in theoretical inertia; our understanding of intelligence and how to measure it does not progress as rapidly as it could. To bring operationalisations of intelligence in line with conceptualisations, we must stretch beyond the status quo (which we have outlined in Parts 1 and 2). With this as our overarching goal, in Part 3 we reviewed features common to existing static and dynamic assessment tasks. We surmised that static tasks are, inter-alia, characterised by one-off measures and local independence of items, whereas dynamic tasks are characterised by having multiple dimensions of performance across items that have dependences across multiple occasions, and often entail feedback. The latter is conceptual closer to our proposed within-individual conceptualisation of intelligence, however, as we pointed out, dynamic tasks present challenges to standard psychometric methods that seems to have reinforced pragmatism and inaction. In the next section we describe how multilevel models (also known as latent-growth models) can address these challenges.
5. Part 4: A Case for Multilevel Models in Intelligence Research
As we have suggested above, with careful, theory-driven task analyses, the parameters of complexity can be formalised and investigated ( Birney and Bowman 2009 ; Ecker et al. 2010 ; Goecke et al. 2021 ; Halford et al. 1998 ). Multilevel models (MLM) are well-suited for this in that they provide a means to explicate a definition, operationalisation, and assessment of cognitive flexibility that is formally aligned and testable within a common model. Such formalisations facilitate statistical analyses, but are also a priori critical for theoretical developments ( Navarro 2021 ). The goal of this final section is to explain how MLM might be used as a theoretical framework for intelligence as cognitive flexibility.
5.1. Cognitive Flexibility as Contingent Level 1 Variability in MLM Models
In considering a within-person account of intelligence, there are a number of sources of variability to consider. Variability at the level of the sample (as a proxy for the population, i.e., Level 2 between individuals), variability at the level of the individual (Level 1, within-individual), and cross-level variability. These can be represented as random effects in a multilevel model. An example of a regression approach is represented below, although SEM formalisations are of course comparable ( Brose et al. 2021 ).
where, Y i j = observation i for individual j .
In this two-level model, π 0 j represents the mean score (i.e., an intercept) for individual j across all occasions i (when X and Z are centred); whereas π 1 j and π 2 j represent the change in Y , as a function of X and Z, respectively , also observed at level 1 (i.e., slopes). Here, we make a distinction between two different types of level 1 variables, X and Z . X is a variable that varies by occasion (i) and individual (j), such as a participant’s rating of confidence or perceived task demand for the given occasion, hence the subscripting, X ij . Z on the other hand, is a variable that changes by occasion (i) only; it is constant for all individuals for that occasion and accordingly subscripted as Z i . An example is an item feature, such as item complexity manipulation, presented in a constant order for everyone, or a variable such as time. While in practice these variables are typically treated as equivalent statistically, in terms of cognitive flexibility they are conceptually different. The model could be extended (with subscripts updated) to capture person × task × situation interactions ( Beckmann 2010 ) by adding a clustering level, such that we have observation Y ijk , where individual i (now at level 3) under situation j ( Z , now at level 2) attempts task manipulation k ( X , now at level 1), but for illustrative purposes we stay with the two-level conceptualisation.
Variability in the individuals’ π 0 j , π 1 j , and π 2 j parameters is considered at level 2 (in Equations (2)–(4), respectively). β 01 represents the change in the individuals’ mean scores as a function of W , a variable that differs between people; and β 11 and β 21 , respectively represent the change in the individuals’ X and Z slope parameters, also as a function of W. Accordingly, β 11 and β 21 are cross-level interaction parameters. For completeness, β 00 , β 10 , and β 20 represent the sample’s average mean and slope (conditional on level 1 and level 2 variables). One might also be tempted to make a distinction between types of level 2 variables analogous to that made between X and Z . For instance, W might reflect inherent individual differences, such as age or conscientiousness, whereas V (Equation (2)) might represent a factor external to the individual, such as a between-condition manipulation (e.g., group 1 gets contextualised feedback, and group 2 gets generic feedback). While the latter is of potential scientific interest and allows for experimental group comparisons for the purpose of, say, validating an operationalisation of cognitive flexibility, our focus here is specifically on within-person processes and how they might differ from one person to another. Accordingly, this type of between-condition comparison is not a factor directly of relevance in building a conceptualisation and measure of cognitive flexibility.
5.1.1. Within- and Between-Individual Parameters of Intelligence as Flexibility
We postulate that cognitive flexibility can be conceived as level 1 variability in (intellectual) behaviour ( Y ij ) that has level 1 contingency. That is, as a behavioural response to X and Z factors as just described. Π 1 and π 2 are contingency parameters, potentially conditional on level 2 influences. The contingency parameters represent how one’s responses change as a function of variation in the problem-space (broadly defined in terms of X and Z factors). X and Z are exemplar triggers in the problem-space for a dynamic response. The magnitude of such responses is indexed by the contingency parameters, and these might be moderated by specific characteristics of the individual. For instance, someone already predisposed to novelty (such as someone high in the openness personality dimension) may not require an as extreme contingent response as someone low in openness; their higher levels of openness might mitigate the flexibility needed when confronted with X and Z factors. This between-person moderation of level 1 contingencies is represented by W parameters, specifically in our representation by β 11 and β 21 . The β 0 intercept parameters reflect group/population mean levels of the contingency parameters. However, simply because the β s are between-person parameters, this does not mean they are not relevant to a conceptualisation of within-person flexibility. The moderation effect just described, demonstrates that these between-person parameters are critical because they serve to contextualise individual responses, the Y ij , more fully. Table 1 presents a selection of possibly relevant level 1 contingent factors and level 2 moderators of these.
Examples contingent (Level 1) and moderating (Level 2) indicators of cognitive flexibility.
The contingent variables can be conceived as either person-centred ( X ) or task/situation centred ( Z ), although each idiosyncratically impact the person’s response. The X factors are contemporaneous to the response in some way, but conceptually distinct from it. For instance, confidence in accuracy ratings are retrospective to a response, whereas state personality is antecedent to a response, but in both cases, they are distinct and idiosyncratically experienced by the individual. On the other hand, the Z factors are germane to the required response, and while they might differ from one occasion to another, they are objectively the same for all people, such as the binding complexity of an item. People are likely to differ in their response to the complexity (i.e., between-individual differences), and this variability is captured in the random-effects of the respective π contingency parameter.
5.1.2. Statistical Advantages of MLM
Multilevel models are considered to resolve reliability concerns about using difference scores ( Draheim et al. 2021 ), allowing contrasts between conditions of, say, higher vs. lower complexity ( Birney et al. 2017 ; Conway et al. 2021 ; Frischkorn and von Bastian 2021 ). There are also other methodological concerns related to using correlation-based criteria that MLM is well positioned to address. Low complexity tends to be associated with higher accuracy (indicating lower levels of experienced difficulty) and a small number of potential solution paths, which by definition lead to ceiling effects and consequently to lower reliability. Higher complexity items tend to have lower accuracy, and a larger number of potential (and perceived) solution paths, which might introduce a combination of floor effects and multidimensionality 6 , also resulting in lower reliability. Having the basis for the correlation-criterion of psychometric complexity to “work” across more than a small range of complexity levels is challenging, particularly since the extremes often define the scope of interest. Within LMER models, shrinkage of random-effects toward fixed-effects ( Gelman et al. 2012 ) has the potential to address this to some extent, although more research is needed to understand the boundaries. An alternative approach is to adopt a binary perspective, where the process is required (present) or not (absent). Ecker et al. ( 2010 ), Bateman and Birney ( 2019 ), and Birney et al. ( 2018 ) have each used this effectively under different conditions.
5.2. Microworld Contingency Parameters as Indicators of Cognitive Flexibility: A Case Study
Using multilevel models, our previous work ( Birney et al. 2018 ) suggests that judicious manipulations of microworld parameters offer potential to derive indicators of decisional and reasoning processes underlying intelligence, that can be isolated from other factors. Although the study was not designed to operationalise intelligence as cognitive flexibility in the way we conceive of it here, the LMER application of parameters derived from this work exemplifies our current approach. In this study, participants were tasked with maintaining a dynamic (changing) inventory at an ideal level by managing outflow via staffing decisions over 30 simulated weeks (see Figure 3 ).

Schematic representation of microworld task described by Birney et al. ( 2018 ) and experimental manipulations (E1 and E2) with exemplar trial-by-trial inventory level feedback across 30 decision periods (which defined a single “attempt”).
Complexity was experimentally manipulated along two independent dimensions intrinsic to solution, delays and outflow (these would be Z factors in Table 1 ). Delays ( Figure 3 , E1) occurred with regard to hiring and firing staff and have a knowable fixed, relational structure. A greater delay between decisions and their impact was expected to generate a concomitant increase in working memory demand. Outflow ( Figure 3 , E2) was either constant or variable (random). Variable outflow resulted in less predictable deviations from the ideal inventory level than when outflow was at a constant rate. Due to the inherent uncertainty, variable outflow was expected to make the task difficult to manage. However, for the same reasons (i.e., uncertainty), reasoning ability was expected to be less effective in mitigating this type of challenge, although we argued that there may be some strategies that might help, given sufficient motivation to attend to detail. Dynamic trial-by-trial feedback across a given block was presented to participants in graphical format (e.g., Figure 3 , right panel). The penalty score analysed as the dependent variable was calculated as a function of the trial-by-trial discrepancies between the impact of participants decisions and the ideal inventory level accumulated by the end of the block. Participants had multiple attempts under different delay and outflow conditions, and therefore experience (attempt number) was an additional performance parameter (which would also be a Z factor in Table 1 ).
Using MLM (specifically, linear mixed-effects regression), we modelled four level 1 random-effects, each conditional on the other; as represented in Figure 4 , π 0 = the intercept (mean performance), and three slopes, π 1 = attempt number (experience), π 2 = delay-effect (present vs. absent), and π 3 = outflow-effect (constant vs. variable), and considered a range of level 2 moderators of these effects as cross-level interactions. These are schematically represented in Figure 4 (full details of the analyses can be found in Birney et al. 2018 ).

Intercorrelations and graphical representation of fixed-effects from MLM analysis of microworld performance indexed by accumulated block penalty (adapted from Birney et al. 2018, with permission from Elsevier; ref: 5356931314753). The model was of the following general form: Level 1: [ Y i j = π 0 j + π 1 j · A t t e m p t j + π 2 j · D e l a y i + π 3 j · O u t f l o w i + e i j ]; Level 2: [ π 0 j = β 00 + β 01 · M o d e r a t o r j + r 0 j ]; [ π 1 j = β 10 + β 11 · M o d e r a t o r j + r 1 j ]; [ π 2 j = β 20 + β 21 · M o d e r a t o r j + r 2 j ]; [ π 3 j = β 30 + β 31 · M o d e r a t o r j + r 3 j ]. The values by the ovals are standardized regression coefficients of the fixed-effects for each parameter ( β 00 , β 10 , β 20 , and β 30 ), averaged across the separate moderator analyses. The values by the curved arrows are the correlations between fixed-effects in a baseline model (i.e., without moderator variables). Moderators (cross-level interactions; β 01 , β 11 , β 21 , and β 31 ) included reasoning (verbal, numerical, abstract), personality (five-factor model), mindsets (goal orientations and implicit theories), and emotional intelligence (MSCEIT branches). See Birney et al. ( 2018 ) for details of additional covariates that were included.
For current purposes, there are a number of points that would benefit from some explication. First, while we could have used a SEM approach (e.g., Brose et al. 2021 ), we used a regression model. Attempts, delay, and outflow conditions were regressed on to the penalty score. Thus, the effects estimated for a given variable are conditional on all other variables in the model (as is standard for regression). Second, the fixed effects component of the analysis (i.e., β parameters, which, all else equal, are means of respective π parameters across individuals) provide weights for a linear composite which best predicts the DV (i.e., the penalty score). However, when these variables are included as random effects, the individuals’ deviations around each fixed effect is explicitly modelled as reflective latent variables, represented as ovals in Figure 4 , although in light of our current argumentation, their ontological status as such remains a supposition ( Bollen and Diamantopoulos 2017 ).
Third, in the parlance presented in this paper, π 1 , π 2 , and π 3 are within-individual contingency variables (of attempts, delay, and outflow, respectively). To explicate, consider the Attempts variable. β 10 represents the mean within-person change in penalty score contingent on number of blocks attempted, averaged across individuals and controlling for level of delay and outflow 7 . A standard interpretation is implied. The standardised regression coefficient, b 10 = −0.31 (as reported in Figure 4 ) indicates that on average, penalty scores tended to decrease with repeated attempts. Substantively, we interpreted this as a learning or experience effect. Importantly, in MLM π 1 j represents the within-individual experience contingency for each of the J individuals; and the average of these is the fixed-effect, β 10 , as just described. In this study we also considered between-individual differences variables as moderators. Although not represented in Figure 4 for simplicity, in the case of the experience contingency, verbal reasoning ability was a statistically significant moderator; the contingency effect of experience was more pronounced for those with higher verbal reasoning scores. Further details of the significant moderators of these parameters are reported in Birney et al. ( 2018 ).
If we assume for a moment that we had set this study up to operationalise cognitive flexibility, what aspect of the model would we expect cognitive flexibility to equate to? The traditional approach would suggest that performance after controlling for differences in conditions (e.g., number of attempts, and the delay/outflow effects) would best represent the essence of what is required by the task; this would be the respective mean for each person ( π 0 j ). However, the notion of cognitive flexibility that we advocate is not framed in terms of averaging across conditions or holding them constant, rather it is defined in terms of idiosyncratic (within-individual) responses to changing conditions. Thus, a model of intelligence as cognitive flexibility indexed in some way by π contingency parameters is needed. There might also be a temptation to define cognitive flexibility as the higher-order reflective factor common to all four latent variables, but this would be short-sighted and premature for the reasons we outline in this paper.
5.3. Summary of Part 4: Why Multilevel Models Are Important
The addition of within-individual process accounts of intelligence as cognitive flexibility introduces the stringent requirement for validity to be established using experimental-psychology methods. First and foremost, we should aim to develop theories for, and seek evidence of a dissociation of level 1 (within-person) process parameters based on theoretically grounded manipulations (e.g., costs and trajectories). Second, evidence of systematic level 2 variability (between-individual) in the theoretically validated level 1 parameters should be obtained. Using this MLM framework, the distinctiveness in processes and the importance of cognitive flexibility is evidenced by four effects. (1) Substantial within-individual variability in trial/item performance; (2) Systematic within-individual effects as a function of process manipulations; (3) Substantial between-individual variability in process-effects; and (4) Systematic between-individual effects of within-individual effects as a function of real-world factors where adaptivity is important. In lieu of real-world tasks , appropriately designed dynamic microworlds may be effective ( Funke et al. 2018 ), yet an arbitrary artificialness in even these tasks persists. Evidence in favour of these effects will support our supposition that our understanding of the processes underlying intelligence as cognitive flexibility can be enhanced if it is operationalised how it is conceptualised.
6. Implications and Final Considerations
During the peer-review process, anonymous reviewers, to whom we express our deep gratitude, raised some interesting discussion points, which we would like to take the opportunity to paraphrase, share, and comment on. As a caveat, and possibly case in point to the challenges our call for reform presents, the attentive reader will notice that in our responses we may have drifted into interpretations and explanations that perpetuate some of the poor practices we have criticised in this paper. For instance, we will discuss CHC factors without questioning their reflective or formative status, and in doing so, we might also be pulled up for assuming ergodicity. For the purpose of communication, we risk this inconsistency.
6.1. Beyond Fluid Intelligence: Why Flexibility Is Relevant to Intelligence Generally, and Other CHC Factors
We have framed much of our thinking in terms of fluid intelligence, so a reasonable question is whether our model of within-individual flexibility is limited to Gf , and therefore does not apply to intelligence generally? In response, we would argue that broader constructs of intelligence likely have similar within-individual conceptualisations. For instance, if one were to consider intelligence constructs such as Practical Intelligence ( Sternberg et al. 2000 ), Cultural Intelligence ( Sternberg et al. 2021 ), or even Emotional Intelligence ( Mayer and Salovey 1993 ), the notion of within-individual, contingent adaptation is central to their conceptualisation. In fact, the cognitive notion of relational integration extends quite naturally to meaning making from adaptive contingencies (i.e., relational bindings) between goals however defined in a given context and non-cognitive content (emotions, affect), possibly filtered through individual differences in personality dispositions, self-concepts, attitudes and value, and the like (as described in Section 5.1 ).
In terms of other CHC factors, some are functionally closer to elementary processes that define features of a process account (i.e., as inputs to flexibility). For instance, Jewsbury et al. ( 2016 ) demonstrated that processing (mental) speed is largely indistinguishable from the Inhibition process conceptualised in the executive function literature ( Miyake and Friedman 2012 ). Although it might be disputed, the conceptual groupings of broad factors proposed by Schneider et al. ( 2016, p. 5 , Figure 2 )— Perceptual Processing (e.g., Ga , Gv , etc.), Controlled Attention (e.g., Gf , Gwm , Gs ), Acquired Knowledge (e.g., Gc , Gq , Grw , Gr , Gl ), and Psychomotor Abilities (e.g., Gp , Gps )—further justify our expectation that the flexibility framework is not relevant to all CHC factors (see Schneider et al. for explanations of abbreviations). For instance, we can set aside Schneider et al.’s Psychomotor Abilities as outside of scope. The Controlled Attention and Perceptual Processing factors are largely process-focused as just described, or Gf which we have addressed. This leaves the Acquired Knowledge factors.
Crystalized intelligence ( Gc ) may be seen to presents an interesting challenge to our flexibility account, although if one were to accept the tenets of the Gf-Gc Investment Theory, even here the development of Gc can be mapped as a series of dynamic, within-person (goal-directed) interactions between the environment and the cognitive and affective resources (processes) one has at their disposal to deal with everyday challenges ( Ackerman 1996 ; Ziegler et al. 2012 ). The extent to which general encoding ( Gl ) and retrieval ( Gr ) factors draw on historical Gf and Gc , the same account can be applied. Thus, prima facie, we see no reason to constrain our within-person account to just fluid intelligence at this point, although this is an area ripe for investigations.
6.2. Beyond Novelty Processing: Why Flexibility Is Relevant to Routine Reasoning and Cognitive-Capacity
While our case for flexibility is relevant to the novelty aspects common to many definitions of fluid intelligence, it is reasonable to question whether the MLM-contingency framework applies to features/facets of Gf that are not inherently to do with novelty, such as routine reasoning in predictable situations, and general cognitive capability. In response, we would argue that in the scheme of one’s overall problem-solving exposure, even routine problems are opportunities to observe flexibility. First, it is interesting to note that we tend not to think of intelligence as a propensity to plod through solving routine, algorithmic problems in routine ways. In such situations we do however give credit for efficiency (e.g., quickly recognising problems are routine), coming up with better (novel) strategies, and doing so with minimal waste of resources. Recognising that a problem is a familiar one (rather than a novel one), drawing on a previously proven solution path (rather than investing effort to create a novel one), and monitoring for possible changes along the way all entails rudimentary adaptation to changing circumstances. Thus, even solution of routine, predictable problems, entails some level of flexibility.
Explanations of reasoning proper (i.e., independent of context) and general cognitive capability (i.e., what it is and how it happens) beyond descriptive accounts remains frustratingly elusive to both experimental and differential psychology. We have already outlined reasons why alone the between-person approach will not help in this regard. Cognitive psychology models, some of which we have reviewed here, go part of the way in presenting a process account of reasoning and general cognitive capability. The lazy (but likely) response is to define reasoning as an emergent property of a system of interacting basic, attentional control, relational binding, and memory processes, with cognitive capability reflected in the efficiency of such a system, often presented as a source of individual differences. Notwithstanding the myriad challenges of this account (or maybe because of them), the need for a within-individual framework of reasoning seems to be amplified rather than diminished. It is our expectation that a formalized, integrated MLM of structurally informed within- and between-person aspects of reasoning, such as the one we have proposed, may provide impetus for a renewed line of investigative efforts.
6.3. Beyond Factor-Analysis: Why Methods Matter When Studying Flexibility
While we have critiqued the use of between-person methods, we are not disputing factor analysis as a pragmatic data reduction tool, nor as a measurement tool (especially when framed as a tau equivalent measurement model). We make a distinction between factor analysis as a data-reduction tool, and structural-equation modelling (SEM) generally as a theory testing tool. Multilevel modelling of within- and between-individual differences can be achieved using a range of comparable methods and procedures, including SEM growth-curve models (e.g., ML-SEM, Brose et al. 2021 ), fixed-link SEM models ( Schweizer 2009 ), or linear mixed-effects regression procedures ( Birney et al. 2017 ; Birney et al. 2018 ). For those less familiar with the nuance of factor-analytic approaches underlying SEM (e.g., cognitive psychologist interested in individual differences), multilevel regression may be more palatable.
However, we do take issue with the dominant tendency of researchers to use reflective models as the default position without considering alternatives (as evidenced by the status quo, despite compelling arguments of their critical limitation). Furthermore, in our view, the speculation that factor analysis can purify observed test scores from error, and therefore allow one to arrive at an estimate of the supposed-to-be “true” attribute and magnitude of an effect is an unfortunate overuse of factor analysis. The same criticism would apply to an overuse of “shrinkage” in MLM regression models if this was observed to occur. Relying on sophisticated statistical tools to “purify” our measures from what are ultimately method-effects ( Birney et al. 2022 ) reflects how little we understand about the sources of impurity (e.g., unreliability or multidimensionality) in our measures ( van der Maas et al. 2017 ). We would do better to improve our measures using strong theory and better linked conceptual and empirical models, rather than make dubiously justified statistical adjustments. Doing so, we argue, requires building structural hypotheses ( Lohman and Ippel 1993 ) and taking within-person process accounts seriously. In sum, we do not have issues with correlations per se, we have issue with between-person correlations being portrayed (and then interpreted) as the only foundation for the conceptualisation and measurement of intelligence conceived as cognitive flexibility.
Finally, while we are not disputing the pragmatic utility of factor analysis, it is important to understand its mathematical foundations, even if we intend only to use the identified structure merely as a description of the covariation of some set of low-level processes. When we talk about the narrow facets of fluid ability, such as (1) induction, (2) deduction, or (3) sequential reasoning, it is easy to assume that the resulting latent variable reflects an aggregation or accumulation of the separate processes. However, mathematically, this is not the case. When each facet is added to a factor analysis, the derived common factor, which we might label Gf , is formally a statistical distillation of what is common in the facets. It is not an aggregation or accumulation of the separate facets. Therefore, when we say fluid intelligence entails, induction, deduction, and sequential reasoning, because they are the indicators (tasks) we have used to “define” the common factor, we have erred. That might be our theoretical explanation, but the common factor is nothing more and nothing less than the very precise thing these three attributes have in common. Our point is, the mathematical derivation of a Gf factor is as much an integral part of its operationalisation as are the tasks chosen. If we think otherwise, even as a first step, our methodological basis will be disconnected from the theory to an unknown extent.
6.4. Beyond the Status Quo: The Implications of Getting It Wrong
One of the implications of getting it wrong is highlighted by Fried ( 2020 ) and also by Protzko ( 2017 ). The gist of what is argued by both is that because reflective models assume the components (indicators, markers, manifest variables) are caused by the latent attribute, then the pathway to intervention by targeting indicators is logically precluded. From this sense, the latent variable is an inherent characteristic of the individual. Formative models (specifically causal-formative ones) on the other hand, where the latent variable is caused by the indicators, provide a pathway for intervention. Change the components, and the latent variable will change (that is, in formative models, the latent variable does not exist as an attribute independent of its indicators). For Fried, the impact is on indicators (symptoms) of pathology. If pathology is inherent in the individual, intervening on the symptoms is unlikely to be helpful. For Protzko, the impact is in regard to cognitive training. If intelligence is a reflective latent attribute, with WMC (say) as a reflective indicator (of the impact of intelligence on it), then training WMC is logically precluded from having an impact on intelligence.
It is interesting to note the relatively recent shift from talking about elementary cognitive tasks to elementary cognitive processes. Refined process theories are a good thing for intraindividual accounts. However, we need to be careful that we do not introduce these “processes” simply as a means to take the heat off reflective assumptions made at higher levels. In CHC framing, ECTs serve as indicators of narrow factors. If they are now processes (fundaments) in their own right, evidence for their status as reflective latent variables need to be demonstrated. Additionally, if these fundaments are reflective latent attributes, what is now the status of assumed-to-be reflective latent variables higher in the hierarchy, that is the “broad-factors”. For instance, what is the status of Gf (an abstraction of narrow ability factors) in models already made up of reflective latent processes defined at the lowest level, keeping in mind the variance distillation that occurs in factor analysis we have just pointed out. The notion of Gf as a causal-formative umbrella of lower-order reflective attributes becomes not only plausible, but possibly, logically necessary. For many, framing Gf as a formative variable is a step too far. It seems an elegant resolution may be to move beyond the simple common-process account of factor analysis, and instead invest resources into further investigations and development of time-varying network models and directed acyclic graphs ( Fried 2020 ).
6.5. Conclusion
We accept as historical fact the dominant, foundational psychometric approach to intellectual abilities as that which started more or less around the time of Charles Spearman (circa 1900) and led to the Cattell-Horn-Carroll (CHC) hierarchical taxonomy. As noted by Conway et al. ( 2021, p. 6 ), CHC is a “model of the covariance structure of cognitive abilities… but it is not a psychological theory”. Historically, establishing the validity of constructs like intelligence has been dominated by considerations of a nomological network of convergent and divergent correlations. In this conceptual analysis and review, we first considered implications of the supposition of stability as antithetical to variability, along with the ergodic claim that between-person models can be extended to within-person processes. We also considered the dominance of reflective common-factor conceptualisations and the neglect and subsequent dismissal of formative ones ( Kovacs and Conway 2016 ; van der Maas et al. 2006 ; van der Maas et al. 2017 ). We explicated causal-formative accounts, in contrast to composite-formative ones ( Bollen and Diamantopoulos 2017 ), as relevant to our goal to explicate a within-individual perspective of intelligence. This is because causal accounts put process and mechanism within the realm of direct observation through experimental manipulation and explicit process accounts, rather than leaving them to be inferred and reified from patterns of discriminant and convergent correlations after data have been collected. However, regardless of whether one studies formative or reflective concepts, or even network models, the burden of process identification is the responsibility of the researcher and cannot be delegated to statistics, no matter their levels of sophistication ( Birney et al. 2022 ).
The promise of working memory theory to provide an explanatory account of intelligence (or at least intelligence test performance) has not been missed by intelligence researchers ( Carpenter et al. 1990 ; Daneman and Carpenter 1980 ). There has been considerable debate regarding the dissociation of WM and intelligence ( Ackerman et al. 2005 ; Blair 2006 ; Guthke et al. 2003 ; Kyllonen and Christal 1990 ). While more and more refined accounts of WM processes have been developed ( Oberauer 2021 ), some we have reviewed here, these have not been matched by similarly well-honed accounts of intelligence. If anything, the limitations of traditional ways to conceptualise processes underlying intelligence and the inertial resistance to new approaches have only been amplified over time. We are of the view that much can be achieved by advancing the alignment of conceptual definitions and methodological considerations which build on modelling within-person variability.
In sum, we have four recommendations, (1) do not assume stationarity, test for it, (2) recognize within-individual (process) accounts are critical to understanding individual differences, (3) be wary of using reflective models as a starting point for theory development, and (4) multilevel models are a good for theory development, and for specifying and testing structural hypotheses regarding within-individual and between-individual differences and their moderators.
Funding Statement
This research was supported under Australian Research Council’s Discovery Projects funding scheme (project DP210101467). The views expressed herein are those of the authors and are not necessarily those of the Australian Research Council.
Author Contributions
Conceptualization, D.P.B. and J.F.B.; methodology, D.P.B. and J.F.B.; investigation, D.P.B. and J.F.B.; resources, D.P.B. and J.F.B.; writing—original draft preparation, D.P.B.; writing—review and editing, D.P.B. and J.F.B.; visualization, D.P.B.; funding acquisition, D.P.B. and J.F.B. All authors have read and agreed to the published version of the manuscript.
Institutional Review Board Statement
Informed consent statement, data availability statement, conflicts of interest.
The authors declare that the research was conducted in the absence of any commercial or financial relationships that could be construed as a potential conflict of interest.
1. The (typically) unquestioned use of the term “manifest variables” to label observed variables is testament to the assumption that individual differences in scores on these variables are the outward manifestation of concomitant individual differences in the latent attribute.
2. While we prefer to reserve the term “measure” for variables where fundamental measurement properties have been demonstrated (see Michell 1990 ), in our view, conceiving them as latent variables that happen to have a useful coding metric is more appropriate (see Birney et al. 2022 ).
3. The trait-complex example also serves to demonstrate a second point made by Bollen and Diamantopoulos ( 2017 ), that reflective latent variables (e.g., extraversion, when appropriately conceived of) can act as composite-formative indicators in other models (such as of trait-complexes).
4. This is also not to say that with greater understanding, the status of concepts will necessarily move from formative to reflective. Some concepts, maybe most, are by nature and definition, formative.
5. According to Beckmann ( 2010 ; see also Birney et al. 2016 ) within the framework of person-task-situation interactions, the situation refers to the context or circumstances in which a task is performed. It constitutes a source of complexity in addition to the processing demands posed by the task itself and therefore contributes to the overall complexity and consequently impacts performance. The user-interface, the clarity of instructions, time pressure, or the semanticity of variable labels in a CPS system are examples for such situation components.
6. Of course, multidimensionality introduces other challenges to measurement that would need to be explicated in the theoretical model.
7. As well as covariates, as mentioned previously.
Publisher’s Note: MDPI stays neutral with regard to jurisdictional claims in published maps and institutional affiliations.
- Ackerman Phillip L. A theory of adult intellectual development: Process, personality, interests, and knowledge. Intelligence. 1996; 22 :227–57. doi: 10.1016/S0160-2896(96)90016-1. [ CrossRef ] [ Google Scholar ]
- Ackerman Phillip L., Beier Margaret E., Boyle Mary O. Working memory and intelligence: The same or different constructs? Psychological Bulletin. 2005; 131 :30–60. doi: 10.1037/0033-2909.131.1.30. [ PubMed ] [ CrossRef ] [ Google Scholar ]
- Ackerman Phillip L., Heggestad Eric D. Intelligence, personality, and interests: Evidence for overlapping traits. Psychological Bulletin. 1997; 121 :219–45. doi: 10.1037/0033-2909.121.2.219. [ PubMed ] [ CrossRef ] [ Google Scholar ]
- Ackerman Phillip L., Kanfer Ruth, Beier Margaret. E. Trait complex, cognitive ability, and domain knowledge predictors of Baccalaureate success, STEM persistence, and gender differences. Journal of Educational Psychology. 2013; 105 :911–27. doi: 10.1037/a0032338. [ CrossRef ] [ Google Scholar ]
- Arend Isabel, Colom Roberto, Botella Juan, Contreras Maria José, Rubio Victor, Santacreu José. Quantifying cognitive complexity: Evidence from a reasoning task. Personality and Individual Differences. 2003; 35 :659–69. doi: 10.1016/S0191-8869(02)00243-X. [ CrossRef ] [ Google Scholar ]
- Bateman Joel E. Relational Integration in Working Memory: Determinants of Effective Task Performance and Links to Individual Differences in Fluid Intelligence. University of Sydney; Sydney: 2020. [ Google Scholar ]
- Bateman Joel E., Birney Damian P. The link between working memory and fluid intelligence is dependent on flexible bindings, not systematic access or passive retention. Acta Psychologica. 2019; 199 :1–12. doi: 10.1016/j.actpsy.2019.102893. [ PubMed ] [ CrossRef ] [ Google Scholar ]
- Bateman Joel E., Birney Damian P., Loh Vanessa. Exploring functions of working memory related to fluid intelligence: Coordination and relational integration. In: Gunzelmann Glenn, Howes Andrew, Tenbrink Thora, Davelaar Eddy J., editors. Proceedings of the 39th Annual Conference of the Cognitive Science Society. Cognitive Science Society; Austin, TX: 2017. pp. 1598–603. [ Google Scholar ]
- Bateman Joel E., Thompson Kate A., Birney Damian P. Validating the relation-monitoring task as a measure of relational integration and predictor of fluid intelligence. Memory & Cognition. 2019; 47 :1457–68. [ PubMed ] [ Google Scholar ]
- Beckmann Jens F. Lernen und komplexes Problemlösen. Ein Beitrag zur Konstruktvalidierung von Lerntests [Learning & Complex Problem Solving: A contribution to the validation of learning tests] Holos; Berlin: 1994. [ Google Scholar ]
- Beckmann Jens F. Taming a beast of burden: On some issues with the conceptualisation and operationalisation of cognitive load. Learning and Instruction. 2010; 20 :250–64. doi: 10.1016/j.learninstruc.2009.02.024. [ CrossRef ] [ Google Scholar ]
- Beckmann Jens F. The umbrella that is too wide and yet too small: Why Dynamic Testing has still not delivered on the promise that was never made. Journal of Cognitive Education and Psychology. 2014; 13 :308–23. doi: 10.1891/1945-8959.13.3.308. [ CrossRef ] [ Google Scholar ]
- Beckmann Jens F. Heigh-Ho: CPS and the seven questions—Some thoughts on contemporary Complex Problem Solving research. Journal for Dynamic Decision Making. 2019; 5 :1–5. doi: 10.11588/jddm.2019.1.69301. [ CrossRef ] [ Google Scholar ]
- Beckmann Jens F., Birney Damian P., Goode Natssia. Beyond psychometrics: The difference between difficult problem solving and complex problem solving. Frontiers in Psychology: Cognitive Science. 2017; 8 :1739. doi: 10.3389/fpsyg.2017.01739. [ PMC free article ] [ PubMed ] [ CrossRef ] [ Google Scholar ]
- Beckmann Jens F., Goode Natassi. The benefit of being naive and knowing it: The unfavourable impact of perceived context familiarity on learning in complex problem solving tasks. Instructional Science. 2013; 41 doi: 10.1007/s11251-013-9280-7. [ CrossRef ] [ Google Scholar ]
- Beckmann Jens F., Guthke Jürgen. Complex problem solving, intelligence, and learning ability. In: Frensch Peter A., Funke Joachim., editors. Complex Problem Solving: The European Perspective. Psychology Press; New York: 1995. pp. 177–200. [ Google Scholar ]
- Binet Alfred. New methods for the diagnosis of the intellectual level of subnormals. L’Année Psychologique. 1905; 12 :191–244. (Translated by Kite, Elizabeth S. (1916). The Development of Intelligence in Children . Vineland: Publications of the Training School at Vineland) [ Google Scholar ]
- Birney Damian P. Ph.D. dissertation. University of Queensland; St Lucia, Brisbane, Australia: 2002. The Measurement of Task Complexity and Cognitive Ability: Relational Complexity in Adult Reasoning. [ Google Scholar ]
- Birney Damian P., Beckmann Jens F., Beckmann Nadin. Within-individual variability of ability and learning trajectories in complex problems. In: McFarland Dennis., editor. General and Specific Mental Abilities. Cambridge Scholars Publishing; Newcastle upon Tyne: 2019. [ Google Scholar ]
- Birney Damian P., Beckmann Jens, Seah Yuan-Zhi. The eye of the beholder: Creativity ratings depend on task involvement, order and methods of evaluation, and personal characteristics of the evaluator. Learning and Individual Differences. 2016; 51 :400–8. doi: 10.1016/j.lindif.2015.07.007. [ CrossRef ] [ Google Scholar ]
- Birney Damian P., Beckmann Jens F., Beckmann Nadin, Double Kit S. Beyond the intellect: Complexity and learning trajectories in Raven’s Progressive Matrices depend on self-regulatory processes and conative dispositions. Intelligence. 2017; 61 :63–77. doi: 10.1016/j.intell.2017.01.005. [ CrossRef ] [ Google Scholar ]
- Birney Damian P., Beckmann Jens F., Beckmann Nadin, Double Kit S., Whittingham Karen. Moderators of learning and performance trajectories in microworld simulations: Too soon to give up on intellect!? Intelligence. 2018; 68 :128–40. doi: 10.1016/j.intell.2018.03.008. [ CrossRef ] [ Google Scholar ]
- Birney Damian P., Beckmann Jens F., Beckmann Nadin, Stemler Steven E. Sophisticated statistics cannot compensate for method effects if quantifiable structure is compromised. Frontiers in Psychology: Quantitative Psychology and Measurement. 2022; 13 :1–13. doi: 10.3389/fpsyg.2022.812963. [ PMC free article ] [ PubMed ] [ CrossRef ] [ Google Scholar ]
- Birney Damian P., Beckmann Jens F., Morris Richard, Cripps Sally A. Trajectories of spirals as cognitive flexibility during n-back training. International Journal of Psychophysiology. 2021; 168 :S34–S35. doi: 10.1016/j.ijpsycho.2021.07.103. [ CrossRef ] [ Google Scholar ]
- Birney Damian P., Bowman David B. An experimental-differential investigation of cognitive complexity. Psychology Science Quarterly. 2009; 51 :449–69. [ Google Scholar ]
- Birney Damian P., Bowman David B., Beckmann Jens F., Seah Yuan. Assessment of processing capacity: Latin-square task performance in a population of managers. European Journal of Psychological Assessment. 2012; 28 :216–26. doi: 10.1027/1015-5759/a000146. [ CrossRef ] [ Google Scholar ]
- Birney Damian P., Halford Graeme S. Cognitive complexity of suppositional reasoning: An application of the relational complexity metric to the knight-knave task. Thinking and Reasoning. 2002; 8 :109–34. doi: 10.1080/13546780143000161. [ CrossRef ] [ Google Scholar ]
- Birney Damian P., Halford Graeme S., Andrews Glenda. Measuring the Influence of Relational Complexity on Reasoning: The Development of the Latin Square Task. Educational and Psychological Measurement. 2006; 66 :146–71. doi: 10.1177/0013164405278570. [ CrossRef ] [ Google Scholar ]
- Blair Clancy. How similar are fluid cognition and general intelligence? A developmental neuroscience perspective on fluid cognition as an aspect of human cognitive ability. Behavioral and Brain Sciences. 2006; 29 :109–60. doi: 10.1017/S0140525X06009034. [ PubMed ] [ CrossRef ] [ Google Scholar ]
- Bollen Kenneth A., Diamantopoulos Adamantios. In defense of causal-formative indicators: A minority report. Psychological Methods. 2017; 22 :581–96. doi: 10.1037/met0000056. [ PMC free article ] [ PubMed ] [ CrossRef ] [ Google Scholar ]
- Borsboom Denny. What is causal about individual differences? : A comment on Weinberger. Theory & Psychology. 2015; 25 :362–68. [ Google Scholar ]
- Borsboom Denny, Mellenbergh Gideon J., Heerden Jaap van. The theoretical status of latent variables. Psychological Review. 2003; 110 :203–19. doi: 10.1037/0033-295X.110.2.203. [ PubMed ] [ CrossRef ] [ Google Scholar ]
- Borsboom Denny, Mellenbergh Gideon J., Heerden Jaap van. The concept of validity. Psychological Review. 2004; 111 :1061–71. doi: 10.1037/0033-295X.111.4.1061. [ PubMed ] [ CrossRef ] [ Google Scholar ]
- Brose Annette, Neubauer Andreas B., Schmiedek Florian. Integrating state dynamics and trait change: A tutorial using the example of stress reactivity and change in well-being. European Journal of Personality. 2021; 36 :180–99. doi: 10.1177/08902070211014055. [ CrossRef ] [ Google Scholar ]
- Burgess Gregory C., Gray Jeremy R., Conway Andrew R. A., Braver Todd S. Neural mechanisms of interference control underlie the relationship between fluid intelligence and working memory span. Journal of Experimental Psychology: General. 2011; 140 :674–92. doi: 10.1037/a0024695. [ PMC free article ] [ PubMed ] [ CrossRef ] [ Google Scholar ]
- Carpenter Patricia A., Just Marcel A., Shell Peter. What one intelligence test measures: A theoretical account of the processing in the raven progressive matrices test. Psychological Review. 1990; 97 :404–31. doi: 10.1037/0033-295X.97.3.404. [ PubMed ] [ CrossRef ] [ Google Scholar ]
- Carroll John B. Human Cognitive Abilities: A Survey of Factor-Analytic Studies. Cambridge University Press; Cambridge: 1993. [ Google Scholar ]
- Chuderski Adam. The relational integration task explains fluid reasoning above and beyond other working memory tasks. Memory & Cognition. 2014; 42 :448–463. doi: 10.3758/s13421-013-0366-x. [ PMC free article ] [ PubMed ] [ CrossRef ] [ Google Scholar ]
- Conway Andrew R. A., Kovacs Kristof, Hao Han, Rosales Kevin P., Snijder Jean-Paul. Individual differences in attention and intelligence: A united cognitive/psychometric approach. Journal of Intelligence. 2021; 9 :34. doi: 10.3390/jintelligence9030034. [ PMC free article ] [ PubMed ] [ CrossRef ] [ Google Scholar ]
- Cripps Edward, Wood Robert E., Beckmann Nadin, Lau John, Beckmann Jens F., Cripps Sally A. Bayesian Analysis of Individual Level Personality Dynamics [Original Research] Frontiers in Psychology. 2016; 7 :1065. doi: 10.3389/fpsyg.2016.01065. [ PMC free article ] [ PubMed ] [ CrossRef ] [ Google Scholar ]
- Cronbach Lee J. The two disciplines of scientific psychology. American Psychologist. 1957; 12 :671–84. doi: 10.1037/h0043943. [ CrossRef ] [ Google Scholar ]
- Daneman Meredyth, Carpenter Patricia A. Individual differences in working memory and reading. Journal of Verbal Learning and Verbal Behaviour. 1980; 19 :450–66. doi: 10.1016/S0022-5371(80)90312-6. [ CrossRef ] [ Google Scholar ]
- De Boeck Paul, Gore Laurence, González Trinidad, Martin Ernesto. An alternative view on the measurement of intelligence and its history. In: Sternberg Robert J., editor. The Cambridge Handbook of Intelligence. Cambridge University Press; Cambridge: 2020. pp. 44–74. [ CrossRef ] [ Google Scholar ]
- Deary Ian J. Human intelligence differences: Towards a combined experimental-differential approach. Trends in Cognitive Sciences. 2001; 5 :164–70. doi: 10.1016/S1364-6613(00)01623-5. [ PubMed ] [ CrossRef ] [ Google Scholar ]
- Diamond Adele. Executive Functions. Annual Review of Psychology. 2013; 64 :135–68. doi: 10.1146/annurev-psych-113011-143750. [ PMC free article ] [ PubMed ] [ CrossRef ] [ Google Scholar ]
- Dörner Dietrich. Diagnostik der operativen Intelligenz [Diagnosis of operative intelligence] Diagnostica. 1986; 32 :290–308. [ Google Scholar ]
- Dörner Dietrich, Funke Joachim. Complex problem solving: What It Is and what It Is not. Frontiers in Psychology. 2017; 8 :1153. doi: 10.3389/fpsyg.2017.01153. [ PMC free article ] [ PubMed ] [ CrossRef ] [ Google Scholar ]
- Double Kit S., Birney Damian P. Do confidence ratings prime confidence? Psychonomic Bulletin & Review. 2019; 26 :1035–42. doi: 10.3758/s13423-018-1553-3. [ PMC free article ] [ PubMed ] [ CrossRef ] [ Google Scholar ]
- Draheim Christopher, Tsukahara Jason S., Martin Jessie D., Mashburn Cody A., Engle Randall W. A toolbox approach to improving the measurement of attention control. Journal of Experimental Psychology: General. 2021; 150 :242–75. doi: 10.1037/xge0000783. [ PubMed ] [ CrossRef ] [ Google Scholar ]
- Ecker Ullrich K. H., Lewandowsky Stephan, Oberauer Klaus, Chee Abby E. H. The components of working memory updating: An experimental decomposition and individual differences. Journal of Experimental Psychology: Learning Memory and Cognition. 2010; 36 :170–89. doi: 10.1037/a0017891. [ PubMed ] [ CrossRef ] [ Google Scholar ]
- Elliott Julian G., Resing Wilma C. M., Beckmann Jens F. Dynamic assessment: A case of unfulfilled potential? Educational Review. 2018; 70 :7–17. doi: 10.1080/00131911.2018.1396806. [ CrossRef ] [ Google Scholar ]
- Engle Randall W. Working memory capacity as executive attention. Current Directions in Psychological Science. 2002; 11 :19–23. doi: 10.1111/1467-8721.00160. [ CrossRef ] [ Google Scholar ]
- Engle Randall W., Tuholski Stephen W., Laughlin James E., Conway Andrew R. Working memory, short-term memory, and general fluid intelligence: A latent-variable approach. Journal of Experimental Psychology: General. 1999; 128 :309–31. doi: 10.1037/0096-3445.128.3.309. [ PubMed ] [ CrossRef ] [ Google Scholar ]
- Fogarty Gerard, Stankov Lazar. Competing tasks as an index of intelligence. Personality and Individual Differences. 1982; 3 :407–22. doi: 10.1016/0191-8869(82)90006-X. [ CrossRef ] [ Google Scholar ]
- Fried Eiko. Lack of theory building and testing impedes progress in the factor and network literature. Psychological Inquiry. 2020; 31 :271–88. doi: 10.1080/1047840X.2020.1853461. [ CrossRef ] [ Google Scholar ]
- Frischkorn Gidon T., von Bastian Claudia C. In search of the executive cognitive processes proposed by Process-Overlap Theory. Journal of Intelligence. 2021; 9 :43. doi: 10.3390/jintelligence9030043. [ PMC free article ] [ PubMed ] [ CrossRef ] [ Google Scholar ]
- Funke Joachim. Computer-based testing and training with scenarios from complex problem-solving reseach: Advantages and disadvantages. International Journal of Selection and Assessment. 1998; 6 :90–96. doi: 10.1111/1468-2389.00077. [ CrossRef ] [ Google Scholar ]
- Funke Joachim, Fischer Andreas, Holt Daniel V. When less is less: Solving multiple simple problems is not complex problem solving—A comment on Greiff et al. (2015) Journal of Intelligence. 2017; 5 :5. doi: 10.3390/jintelligence5010005. [ PMC free article ] [ PubMed ] [ CrossRef ] [ Google Scholar ]
- Funke Joachim, Fischer Andreas, Holt Daniel V. Competencies for Complexity: Problem Solving in the Twenty-First Century. In: Care E., Griffin P., Wilson M., editors. Assessment and Teaching of 21st Century Skills. Educational Assessment in an Information Age. Springer; Cham: 2018. pp. 41–53. [ CrossRef ] [ Google Scholar ]
- Gabales Leonardo, Birney Damian P. Are the limits in processing and storage capacity common? Exploring the additive and interactive effects of processing and storage load in working memory. Journal of Cognitive Psychology. 2011; 23 :322–41. doi: 10.1080/20445911.2011.501330. [ CrossRef ] [ Google Scholar ]
- Gelman Andrew, Hill Jennifer, Yajima Masanao. Why we (usually) don’t have to worry about multiple comparisons. Journal of Research on Educational Effectiveness. 2012; 5 :189–211. doi: 10.1080/19345747.2011.618213. [ CrossRef ] [ Google Scholar ]
- Goecke Benjamin, Schmitz Florian, Wilhelm Oliver. Binding costs in processing efficiency as determinants of cognitive flexibility. Journal of Intelligence. 2021; 9 :18. doi: 10.3390/jintelligence9020018. [ PMC free article ] [ PubMed ] [ CrossRef ] [ Google Scholar ]
- Goff Maynard, Ackerman Phillip L. Personality-intelligence relations: Assessment of typical intellectual engagement. Journal of Educational Psychology. 1992; 84 :537–52. doi: 10.1037/0022-0663.84.4.537. [ CrossRef ] [ Google Scholar ]
- Gottfredson Linda S. Why g matters: The complexity of everyday life. Intelligence. 1997; 24 :79–732. doi: 10.1016/S0160-2896(97)90014-3. [ CrossRef ] [ Google Scholar ]
- Gottfredson Linda S. g theory: How recurring variation in human intelligence and the complexity of everyday tasks create social structure and the democratic dilemma. In: Sternberg R. J., editor. The Nature of Human Intelligence. Cambridge University Press; Cambridge: 2018. pp. 130–51. [ Google Scholar ]
- Gray Jeremy R., Chabris Christopher F., Braver Todd S. Neural mechanisms of general fluid intelligence. Nature Neuroscience. 2003; 6 :316–22. doi: 10.1038/nn1014. [ PubMed ] [ CrossRef ] [ Google Scholar ]
- Greiff Samuel, Stadler Matthias, Sonnleitner Philipp, Wolff Christian, Martin Romain. Sometimes less is more: Comparing the validity of complex problem solving measures. Intelligence. 2015; 50 :100–13. doi: 10.1016/j.intell.2015.02.007. [ CrossRef ] [ Google Scholar ]
- Grigorenko Elena L., Sternberg Robert J. Dynamic testing. Psychological Bulletin. 1998; 124 :75–111. doi: 10.1037/0033-2909.124.1.75. [ CrossRef ] [ Google Scholar ]
- Guthke Jürgen, Beckmann Jens F. The learning test concept and its application in practice. In: Lidz Carol S., Elliot Julian., editors. Dynamic Assessment: Prevailing Models and Applications (Advances in Cognition and Educational Practice) Volume 6. Elsevier Science; Oxford: 2000. pp. 17–69. [ Google Scholar ]
- Guthke Jürgen, Beckmann Jens F., Seiwald Barbara B. Wie “identisch” sind Arbeitsgedächtnis und Schlußfolgerndes Denken? [How “identical” are working memory and reasoning?] In: Krause Werner, Krause Bodo., editors. Psychologie im Kontext der Naturwissenschaften. Trafo; Berlin: 2003. pp. 149–59. [ Google Scholar ]
- Guttman Louis. Measurement as structural theory. Psychometrika. 1971; 36 :329–46. doi: 10.1007/BF02291362. [ CrossRef ] [ Google Scholar ]
- Halford Graeme S., Phillips Steven, Wilson William H., McCredden Julie E., Andrews Glenda, Birney Damian P., Baker Rosemary, Bain John D. Relational processing is fundamental to the central executive and is limited to four variables. In: Osaka Naoyuki, Logie Robert H., D’Esposito Mark., editors. The Cognitive Neuroscience of Working Memory: Behavioural and Neural Correlates. Oxford University Press; Oxford: 2007. pp. 261–80. [ Google Scholar ]
- Halford Graeme S., Wilson William H. A category theory approach to cognitive development. Cognitive Psychology. 1980; 12 :356–411. doi: 10.1016/0010-0285(80)90014-6. [ PubMed ] [ CrossRef ] [ Google Scholar ]
- Halford Graeme S., Wilson William H., Phillips Steven. Processing capacity defined by relational complexity: Implications for comparative, developmental, and cognitive psychology. Behavioral and Brain Sciences. 1998; 21 :803–31. doi: 10.1017/S0140525X98001769. [ PubMed ] [ CrossRef ] [ Google Scholar ]
- Halford Graeme S., Wilson William H., Phillips Steven. Relational knowledge: The foundation of higher cognition. Trends in Cognitive Sciences. 2010; 14 :497–505. doi: 10.1016/j.tics.2010.08.005. [ PubMed ] [ CrossRef ] [ Google Scholar ]
- Hearne Luke J., Birney Damian P., Cocchi Luca, Mattingley Jason B. The Latin Square Task as a measure of relational reasoning: A replication and assessment of reliability. European Journal of Psychological Assessment, Advance Online Publication. 2019; 36 :296. doi: 10.1027/1015-5759/a000520. [ CrossRef ] [ Google Scholar ]
- Horn John L., Noll Jennie. A system for understanding cognitive capabilities: A theory and the evidence on which it is based. In: Detterman Douglas K., editor. Theories of Intelligence. Ablex Publishing Corporation; Norwood: 1994. pp. 151–203. [ Google Scholar ]
- Jewsbury Paul A., Bowden Stephen C., Strauss Milton E. Integrating the switching, inhibition, and updating model of executive function with the Cattell—Horn—Carroll model. Journal of Experimental Psychology: General. 2016; 145 :220–45. doi: 10.1037/xge0000119. [ PubMed ] [ CrossRef ] [ Google Scholar ]
- Kovacs Kristof, Conway Aandrew R. A. Process Overlap Theory: A unified account of the general factor of intelligence. Psychological Inquiry. 2016; 27 :151–77. doi: 10.1080/1047840X.2016.1153946. [ CrossRef ] [ Google Scholar ]
- Kyllonen Patrick C., Christal Raymond E. Reasoning ability is (little more than) working-memory capacity?! Intelligence. 1990; 14 :389–433. doi: 10.1016/S0160-2896(05)80012-1. [ CrossRef ] [ Google Scholar ]
- Lohman David F., Ippel Martin J. Cognitive diagnosis: From statistically based assessment toward theory-based assessment. In: Frederiksen Norman, Mislevy Robert J., Bejar Isaac I., editors. Test Theory for a New Generation of Tests. Lawrence Erlbaum Associates; Hillsdale: 1993. pp. 41–70. [ Google Scholar ]
- Mackintosh Nicholas J. IQ and Human Intelligence. 2nd ed. Oxford University Press; Oxford: 2011. [ Google Scholar ]
- Mayer John D., Salovey Peter. The intelligence of emotional intelligence. Intelligence. 1993; 22 :89–114. doi: 10.1016/S0160-2896(96)90011-2. [ CrossRef ] [ Google Scholar ]
- Michell Joel. An Introduction to the Logic of Psychological Measurement. Lawrence Erlbaum Associates; Hillsdale: 1990. [ Google Scholar ]
- Miyake Akira, Friedman Naomi P. The nature and organization of individual differences in executive functions: Four general conclusions. Current Directions in Psychological Science. 2012; 21 :8–14. doi: 10.1177/0963721411429458. [ PMC free article ] [ PubMed ] [ CrossRef ] [ Google Scholar ]
- Molenaar Peter C. M. Manifesto on psychology as idiographic science: Bringing the person back into scientific psychology, This time forever. Measurement. 2004; 2 :201–18. doi: 10.1207/s15366359mea0204_1. [ CrossRef ] [ Google Scholar ]
- Molenaar Peter C. M. On the necessity to use person-specific data analysis approaches in psychology. European Journal of Developmental Psychology. 2013; 10 :29–39. doi: 10.1080/17405629.2012.747435. [ CrossRef ] [ Google Scholar ]
- Navarro Danielle. If mathematical psychology did not exist we might need to invent it: A comment on theory building in psychol. Perspectives on Psychological Science. 2021; 16 :707–16. doi: 10.1177/1745691620974769. [ PubMed ] [ CrossRef ] [ Google Scholar ]
- Neisser Ulric, Boodo Gweneth, Bouchard Thomas J., Jr., Boykin A. Wade, Brody Nathon, Ceci Stephen J., Halpern Diane F., Loehlin John C., Perloff Robert, Sternberg Robert J., et al. Intelligence: Knowns and unknowns. American Psychologist. 1996; 51 :77–101. doi: 10.1037/0003-066X.51.2.77. [ CrossRef ] [ Google Scholar ]
- Oberauer Klaus. The focus of attention in working memory—From metaphors to mechanisms. Frontiers in Human Neuroscience. 2013; 7 :673. doi: 10.3389/fnhum.2013.00673. [ PMC free article ] [ PubMed ] [ CrossRef ] [ Google Scholar ]
- Oberauer Klaus. Towards a theory of working memory. In: Logie Robert H., Camos Valerie, Cowan Nelson., editors. Working Memory. Oxford University Press; Oxford: 2021. [ Google Scholar ]
- Oberauer Klaus, Lewandowsky Stephan. Control of information in working memory: Encoding and removal of distractors in the complex-span paradigm. Cognition. 2016; 156 :106–28. doi: 10.1016/j.cognition.2016.08.007. [ PubMed ] [ CrossRef ] [ Google Scholar ]
- Oberauer Klaus, Suss Heinz-Martin, Schulze Ralf, Wilhelm O., Wittmann Werner. Working memory capacity—Facets of a cognitive ability construct. Personality and Individual Differences. 2000; 29 :1017–45. doi: 10.1016/S0191-8869(99)00251-2. [ CrossRef ] [ Google Scholar ]
- Oberauer Klaus, Suss Heinz-Martin, Wilhelm Oliver, Sander N. Individual differences in working memory capacity and reasoning ability. In: Conway Andrew R. A., Jarrold Christopher, Kane Mchael J., Miyake Akira, Towse John N., editors. Variation in Working Memory. Oxford University Press; New York: 2007. pp. 49–75. [ Google Scholar ]
- Pedhazuer Elazar J., Schmelkin Liora P. Measurement, Design, and Analysis: An Integrated Approach. Lawrence Erlbaum Associates; Hillsdale: 1991. [ Google Scholar ]
- Protzko John. Effects of cognitive training on the structure of intelligence. Psychonomic Bulletin & Review. 2017; 24 :1022–31. doi: 10.3758/s13423-016-1196-1. [ PubMed ] [ CrossRef ] [ Google Scholar ]
- Ravizza Susan M., Carter Cameron S. Shifting set about task switching: Behavioral and neural evidence for distinct forms of cognitive flexibility. Neuropsychologia. 2008; 46 :2924–35. doi: 10.1016/j.neuropsychologia.2008.06.006. [ PMC free article ] [ PubMed ] [ CrossRef ] [ Google Scholar ]
- Schneider W. Joel, Mayer John D., Newman Daniel A. Integrating hot and cool intelligences: Thinking broadly about broad abilities. Journal of Intelligence. 2016; 4 :1. doi: 10.3390/jintelligence4010001. [ CrossRef ] [ Google Scholar ]
- Schneider W. Joel, McGrew Kevin S. The Cattell-Horn-Carroll model of intelligence. In: Flanagan Dawn, Harrison Patti., editors. Contemporary Intellectual Assessment: Theories, Tests, and Issues. 3rd ed. Guilford; New York: 2012. pp. 99–144. [ Google Scholar ]
- Schweizer Karl. The speed-accuracy transition due to task complexity. Intelligence. 1996; 22 :115–28. doi: 10.1016/S0160-2896(96)90012-4. [ CrossRef ] [ Google Scholar ]
- Schweizer Karl. Fixed-links models for investigating experimental effects combined with processing strategies in repeated measures designs: A cognitive task as example. Methodology. 2009; 62 :217–32. doi: 10.1348/000711007X268558. [ PubMed ] [ CrossRef ] [ Google Scholar ]
- Schweizer Karl, Altmeyer Michael, Ren Xuezhu, Schreiner M.ichael. Models for the detection of deviations from the expected processing strategy in completing the items of cognitive measures. Multivariate Behavioral Research. 2015; 50 :544–54. doi: 10.1080/00273171.2015.1032397. [ PubMed ] [ CrossRef ] [ Google Scholar ]
- Schweizer Karl, Koch Wolfgang. Perceptual processes and cognitive ability. Intelligence. 2002; 142 :1–26. doi: 10.1016/S0160-2896(02)00117-4. [ CrossRef ] [ Google Scholar ]
- Shipstead Zach, Harrison Tyler L., Engle Randall W. Working memory capacity and fluid intelligence: Maintenance and disengagement. Perspectives on Psychological Science. 2016; 11 :771–79. doi: 10.1177/1745691616650647. [ PubMed ] [ CrossRef ] [ Google Scholar ]
- Spilsbury Georgina, Stankov Lazar, Roberts Richard. The effect of a test’s difficulty on its correlation with intelligence. Personality and Individual Differences. 1990; 11 :1069–77. doi: 10.1016/0191-8869(90)90135-E. [ CrossRef ] [ Google Scholar ]
- Stadler Matthias, Becker Nicolas, Gödker Markus, Leutner Detlev, Greiff Samuel. Complex problem solving and intelligence: A meta-analysis. Intelligence. 2015; 53 :92–101. doi: 10.1016/j.intell.2015.09.005. [ CrossRef ] [ Google Scholar ]
- Stankov Lazar. Complexity, metacognition and fluid intelligence. Intelligence. 2000; 28 :121–43. doi: 10.1016/S0160-2896(99)00033-1. [ CrossRef ] [ Google Scholar ]
- Stankov Lazar, Crawford John D. Ingredients of complexity in fluid intelligence. Learning and Individual Differences. 1993; 5 :73–111. doi: 10.1016/1041-6080(93)90007-F. [ CrossRef ] [ Google Scholar ]
- Stankov Lazar, Cregan Anne. Quantitative and qualitative properties of an intelligence test: Series completion. Learning and Individual Differences. 1993; 5 :137–69. doi: 10.1016/1041-6080(93)90009-H. [ CrossRef ] [ Google Scholar ]
- Steinke Alexander, Kopp Bruno. Toward a computational neuropsychology of cognitive flexibility. Brain Sciences. 2020; 12 :1000. doi: 10.3390/brainsci10121000. [ PMC free article ] [ PubMed ] [ CrossRef ] [ Google Scholar ]
- Sternberg Robert J. Component processes in analogical reasoning. Psychological Review. 1977a; 84 :353–78. doi: 10.1037/0033-295X.84.4.353. [ CrossRef ] [ Google Scholar ]
- Sternberg Robert J. Intelligence, Information Processing, and Analogical Reasoning: The Componential Analysis of Human Abilities. Lawrence Erlbaum Associates; Hillsdale: 1977b. [ Google Scholar ]
- Sternberg Robert J. Sketch of a componential subtheory of human intelligence. Behavioral and Brain Science. 1980; 3 :573–84. doi: 10.1017/S0140525X00006932. [ CrossRef ] [ Google Scholar ]
- Sternberg Robert J. In: The Cambridge Handbook of Intelligence. 2nd ed. Sternberg Robert J., editor. Cambridge University Press; Cambridge: 2020. [ CrossRef ] [ Google Scholar ]
- Sternberg Robert J., Forsythe Geroge B., Hedlund Jennifer, Horvath Joseph, Wagner Richard K., Williams Wendy M., Snook Scott, Grigorenko Elena L. Practical Intelligence in Everyday Life. Cambridge University Press; Cambridge: 2000. [ Google Scholar ]
- Sternberg Robert J., Wong Chak H., Kreisel Anastasia P. Understanding and assessing cultural intelligence: Maximum-performance and typical-performance approaches. Journal of Intelligence. 2021; 9 :45. doi: 10.3390/jintelligence9030045. [ PMC free article ] [ PubMed ] [ CrossRef ] [ Google Scholar ]
- Unsworth Nash, Engle Randall W. The nature of individual differences in working memory capacity: Active maintenance in primary memory and controlled search from secondary memory. Psychological Review. 2007a; 114 :104–32. doi: 10.1037/0033-295X.114.1.104. [ PubMed ] [ CrossRef ] [ Google Scholar ]
- Unsworth Nash, Engle Randall W. On the division of short-term and working memory: An examination of simple and complex span and their relation to higher order abilities. Psychological Bulletin. 2007b; 133 :1038–66. doi: 10.1037/0033-2909.133.6.1038. [ PubMed ] [ CrossRef ] [ Google Scholar ]
- van der Maas Han, Dolan Conor V., Grasman Raoul P. P., Wicherts Jelte M., Huizenga Hilde M., Raijmakers Maartje E. J. A dynamical model of General Intelligence: The positive manifold of intelligence by mutualism. Psychological Review. 2006; 113 :842–61. doi: 10.1037/0033-295X.113.4.842. [ PubMed ] [ CrossRef ] [ Google Scholar ]
- van der Maas Han, Kan Kees-Jan, Marsman Maarten, Stevenson Claire E. Network models for cognitive development and intelligence. Journal of Intelligence. 2017; 5 :16. doi: 10.3390/jintelligence5020016. [ PMC free article ] [ PubMed ] [ CrossRef ] [ Google Scholar ]
- Wood Robert E., Beckmann Jens F., Birney Damian P. Simulations, learning and real world capabilities. Education + Training. 2009; 51 :491–510. doi: 10.1108/00400910910987273. [ CrossRef ] [ Google Scholar ]
- Yu Calvin, Beckmann Jens F., Birney Damian P. Cognitive Flexibility as a Meta-Competency. Estudios de Psicología. 2019; 40 :563–84. doi: 10.1080/02109395.2019.1656463. [ CrossRef ] [ Google Scholar ]
- Ziegler Matthias, Danay Erik, Heene Moritz, Asendorpf Jens, Buhner Markus. Openness, fluid intelligence, and crystallized intelligence: Toward an integrative model. Journal of Research in Personality. 2012; 46 :173–83. doi: 10.1016/j.jrp.2012.01.002. [ CrossRef ] [ Google Scholar ]
Thank you for visiting nature.com. You are using a browser version with limited support for CSS. To obtain the best experience, we recommend you use a more up to date browser (or turn off compatibility mode in Internet Explorer). In the meantime, to ensure continued support, we are displaying the site without styles and JavaScript.
- View all journals
- My Account Login
- Explore content
- About the journal
- Publish with us
- Sign up for alerts
- Open access
- Published: 08 April 2024
Psychological flexibility and cognitive-affective processes in young adults’ daily lives
- Marlon Westhoff 1 ,
- Saida Heshmati 2 ,
- Björn Siepe 3 ,
- Christoph Vogelbacher 1 ,
- Joseph Ciarrochi 4 ,
- Steven C. Hayes 5 &
- Stefan G. Hofmann 1
Scientific Reports volume 14 , Article number: 8182 ( 2024 ) Cite this article
Metrics details
- Computational science
- Quality of life
Psychological flexibility plays a crucial role in how young adults adapt to their evolving cognitive and emotional landscapes. Our study investigated a core aspect of psychological flexibility in young adults: adaptive variability and maladaptive rigidity in the capacity for behavior change. We examined the interplay of these elements with cognitive-affective processes within a dynamic network, uncovering their manifestation in everyday life. Through an Ecological Momentary Assessment design, we collected intensive longitudinal data over 3 weeks from 114 young adults ages 19 to 32. Using a dynamic network approach, we assessed the temporal dynamics and individual variability in flexibility in relation to cognitive-affective processes in this sample. Rigidity exhibited the strongest directed association with other variables in the temporal network as well as highest strength centrality, demonstrating particularly strong associations to other variables in the contemporaneous network. In conclusion, the results of this study suggest that rigidity in young adults is associated with negative affect and cognitions at the same time point and the immediate future.
Introduction
Young adulthood is a pivotal stage marked by significant developmental changes, including the establishment of autonomy, transitioning into new social roles, forming intimate partnerships 1 , and evolving identity and life goals 2 . This period is also characterized by cognitive and emotional maturation 3 , presenting numerous challenges that can impact the daily psychological processes of young adults aged 18–29 years 4 , 5 . The dynamic nature of this phase provides a unique lens to examine how individuals adapt to these challenges, particularly through the lens of psychological flexibility—a key set of processes that facilitate adaptation to varying contexts 6 , 7 , 8 , 9 , 10 , 11 .
Psychological flexibility is a multidimensional concept that involves the ability to respond adaptively to internal experiences and external situations in a manner consistent with one’s values, adjusting cognitive, affective, and behavioral approaches as needed 8 . It enables young adults to constructively engage with challenging emotions and thoughts through emotional openness and cognitive flexibility 9 , 12 , 13 , influencing other psychological processes and contributing to their functional expression. Conversely, psychological inflexibility, characterized by an excessive attachment to cognitions and avoidance of distressing experiences 13 or over-attachment to supposedly positive ones can be detrimental 14 . Given the contextual nature of psychological flexibility, where internal and external environments are in a constant state of flux 8 , 9 , 10 , its role is especially salient in young adulthood—a time inherently defined by change and the need for adaptation 4 , 15 , 16 .
Given the fluid and often tumultuous nature of young adulthood, marked by significant mental health challenges 4 , 5 , the role of cognitive-affective processes in psychological flexibility warrants closer examination. Cognitive-affective processes refer to the cognitive and emotional aspects of experience and behavior. They include different thinking patterns, such as cognitive fusion, ruminative thinking, or dysfunctional thinking, which can impact how life events are perceived and given meaning. They also include the way individuals feel about their situation and deal with their emotions, for example through the development of acceptance and regulation skills. Therefore, cognitive-affective processes are fundamental to how individuals interpret information based on automatic thoughts or core assumptions 17 , manage and regulate their emotional responses 18 , 19 , and engage in decision-making that aligns with their values, for example through acceptance or cognitive defusion 20 , 21 . They are instrumental in shaping an individual’s capacity to navigate stress and adapt to novel situations 22 . The extent to which young adults can effectively confront and adapt to the developmental hurdles they face is significantly influenced by these cognitive-affective processes, which may impact their mental health 23 , 24 , 25 , 26 . To assess psychological processes, physiological measures such as heart rate data or brain correlates of psychological flexibility, e.g., associated with shift in attention or perspective taking 27 , are becoming increasingly important. These additional measures will provide complementary insights into an individual’s ability to respond flexibly to internal and external stimuli.
Previous research on psychological flexibility has notable limitations that need to be addressed. Firstly, prior studies have mostly focused on inflexibility 28 , 29 , which has overshadowed the broader, more nuanced aspects of psychological flexibility that extend beyond the mitigation of clinical distress 30 , 31 , 32 . An evolutionary perspective 33 , 34 may be useful for the simultaneous examination of both positive and negative manifestations of psychological flexibility, and for the development of assessment tools with that needed breadth 23 . This involves focusing on the dynamics of variation 29 , 35 and rigidity as key mechanisms that influence behavioral adaptability to contextual shifts 36 , 37 . Variation, characterized by healthy variability and contextual awareness, is essential for adaptive behavior 9 , 10 , 38 . In contrast, rigidity, defined as difficulty in shifting from one set way of responding to another in response to contextual events 30 , 39 , emerges as a central characteristic of mental distress 40 , 41 , 42 . Therefore, flexibility and rigidity are not merely two ends on a continuum, because both rigid and flexible responses may be adaptive and co-occur at different levels in a given time frame.
The reliance on static correlational analyses and cross-sectional designs 7 , 43 , 44 has limited the exploration of within-person changes and the temporal antecedents of maladaptive rigidity and adaptive variability. In order to uncover their potential causal pathways and intricate associations with cognitive-affective processes, research must pivot to longitudinal studies that track the temporal dynamics and individual variability of these core features of psychological flexibility. This in turn requires efficient and concise measurement of the most fundamental aspect of variability/rigidity: an individual’s capacity to change behavior. Such an approach is difficult to achieve with conventional validated questionnaires designed for cross-sectional designs that may stumble in an attempt to cover more features of psychological flexibility as a multidimensional concept.
Whereas some studies highlight the mediating role of psychological flexibility 28 , 45 , 46 , there has been an overreliance on self-report measures (e.g., Acceptance and Action Questionnaire 47 ) that may not fully capture an individual’s actual ability to modify behavior in real-world contexts (for a brief discussion, see Ciarrochi et al. 48 ). Measures of this kind are also ill-suited to longitudinal studies incorporating temporally dense and idiographic assessment, which appears to be required for a more comprehensive exploration of psychological flexibility 9 , 49 , 50 .
This study’s objective is to investigate the interplay between cognition, affect, and the capacity to change behavior in young adults during their developmental phase of young adulthood. Specifically, we aimed to answer the question: How is a core component of psychological flexibility – rigidity and variation as distinct components of the capacity to change behavior – associated with both positive and negative cognitive-affective processes in young adults’ daily lives? To achieve this, we utilized intensive longitudinal data (ILD) collected through Ecological Momentary Assessment (EMA) 51 and employed a dynamic network analytic approach 52 .
Using a dynamic network approach allows us to simultaneously analyze the associations (i.e., edges) between variables (i.e., nodes) reflecting psychological flexibility and cognitive-affective processes over time. By simultaneously considering the interplay between multiple variables, we gain deeper insights into how variables influence each other over time, providing a unique advantage in capturing the complexity of mental health 52 , 53 . By modeling reciprocal associations between rigidity/flexibility and cognitive-affective processes, rather than just unidirectional relationships, we can capture potential interactions between these processes. In addition to illustrating how the capacity for behavior change and cognitive-affective variables interact within a network, the dynamic network approach also helps identify which variables are comparatively most central. Nodes with high centrality have a comparatively stronger association with the other nodes and therefore may be of greater relevance 54 . The use of ILD may reveal temporal dependencies between variables 55 , which enhances causal inference about the relationships between psychological flexibility and both adaptive and maladaptive cognitive-affective processes 56 . The dynamic network approach addresses the inherent dynamics of variation and rigidity as key mechanisms of adaptability to contextual change, making it advantageous over static correlational analyses. This allows us to study the temporal changes and dynamic interplay of cognitive-affective processes. In addition, it considers individual variability by estimating individual networks instead of relying on aggregated group patterns. This approach enhances generalizability of our findings, as it is based on the aggregation of individual-specific data 57 , 58 .
Leveraging the dynamic network approach, we hypothesize that positive and negative processes are interconnected and mutually reinforcing, reflecting their interdependence. Consistent with prior research 23 , 59 , we anticipate that in a complex network, rigidity will have comparatively stronger contemporaneous and temporal associations with negative, rather than positive, cognitive-affective processes. At the same time, we assume that variation will have stronger contemporaneous and temporal associations with positive cognitive-affective processes. Furthermore, building on previous findings 60 , 61 , 62 , we hypothesize that rigidity will be a particularly central node compared to variation and will have a correspondingly stronger association with the other processes under investigation 47 , 61 .
We collected data with EMA 51 as part of a larger study. This study consisted of three phases: a baseline assessment, the EMA phase, and a post-assessment. For the present analyses, only data from the EMA phase were used. The EMA phase took place between September 14th 2022 and July 27th, 2023. Each individual EMA assessment spanned 21 days. Participants received prompts on their smartphones five times per day, following a semi-random schedule. Prompts were scheduled within participants’ self-reported waking hours and were delivered randomly with a normal distribution ( SD = 0.63 h) around the scheduled time, with intervals of 3 h throughout the day and at least 30 min apart. Each assessment lasted around 3.5 min, and participants were required to respond to the prompts within 120 min, after which they expired. Prompts were conducted using the Ethica Data platform, available on both iOS and Android, which participants installed on their smartphones. Participants were directed to the Qualtrics platform via Ethica to respond to relevant questions.
Participants
One hundred and twenty-five participants enrolled in the study. They were recruited through an online portal as well as flyers and posters displayed on the university campus. Eligible participants were required to be students, be over 18 years of age, and either be native German speakers or possess native-level fluency in German. Participants received reimbursement based on their response rate, with higher payment per prompt for higher response rates. The highest possible remuneration was 225 €. Eleven participants from the initial 125 were excluded from the dataset due to improper responses to survey items used for validation purposes or had too low a response rate (defined below), resulting in an analysis involving data from 114 participants. The overall mean compliance rate was 92.88% ( SD = 8.13; range 41.90–100). The study was approved by the Ethics Committee of the Department of Psychology of the Philipps-University of Marburg (Reference: 2022-22v). All participants provided informed consent after receiving complete information about the study.
Among the 114 participants included, 80 identified as female, while 34 identified as male; none identified as neither male nor female. The mean age was 23.77 ( SD = 2.52). The compliance rate among included participants was 93.54% ( SD = 5.67; range 71.43–100). The majority of participants reported being in a relationship (63%), while fewer were single (36%) or married (1%). Regarding living status, the vast majority (68%) resided in shared apartments, and 68% were bachelor students.
Time-invariant variables
Participants provided self-reported information regarding their gender, age, relationship status, living situation, and educational attainment. Descriptive statistics of the time-invariant variables can be found in Table 1 .
Time-variant variables: item selection procedure and response scale
The item selection procedure employed in this study was devised to address pertinent concerns related to dynamic psychological processes of change. The reported items are constituents of a comprehensive EMA questionnaire battery, which, in turn, forms a segment of a more extensive study comprising baseline and post-surveys. All measures used in the study are provided in the supplementary materials. For the present publication only a subset of selected items specifically relevant to our research questions are selected and listed. Complete data is accessible in the supplementary materials.
To answer relevant scientific questions, items assessing psychological flexibility, cognition, and affect were selected from the entire questionnaire battery. Items were assessed using the Process-Based Assessment Tool (PBAT) 23 . The PBAT is an item-pool designed for intensive longitudinal assessment, targeting biopsychosocial processes of change as conceptualized within the “extended evolutionary meta-model” of process-based therapy 33 . It consists of 18 items that assess cognition, affect, attention, social connection, motivation, overt behavior, physical health, retention, and flexibility/variation. Relevant to our research, the most generic component of psychological flexibility – adaptive variation and maladaptive rigidity in the capacity to change behavior – is represented by two items: “I am feeling stuck and unable to change my ineffective behavior” (rigidity) and “I am able to change my behavior, so that it helps my life” (variation). Affect was assessed with items “I am not finding an appropriate outlet for my emotions” (negative) and “I am able to experience a range of emotions appropriate to the moment” (positive). Cognition was measured using statements “My thinking is getting in the way of things that are important to me” (negative) and “I am using my thinking in ways that help me live better” (positive). Participants were asked to indicate their level of agreement with each statement based on their current feelings and behaviors on a scale from 0 to 100.
Thus in this study we focused on and measured the “bottom line” feature of psychological flexibility 63 that is particularly consistent with the contextualistic and evolutionary approach that underlies the concept 64 – can an individual display healthy forms of variation and avoid maladaptive rigidity in what they actually do. The items of the PBAT are designed to provide an efficient and concise EMA-style measure of this feature – as well as cognitive and affective domains. The PBAT represents a useful complement to more comprehensive, validated questionnaires that are more likely to be used in a cross-sectional context. The PBAT is the most specific and comprehensive measure available to assess psychological flexibility in an EMA setting.
Data analysis
Data preprocessing was performed using Python version 3.11.3 65 , time-series analyses were conducted using the statistical software R version 4.1.2 66 . The data and syntax used for the analyses are available in the supplementary materials.
Data preprocessing
To ensure data quality, we conducted several preprocessing steps. First, we ensured that only participants with a response rate ≥ 70% (i.e., at least 73 completed measurements) were included in the analyses. Additionally, care was taken to only include those prompts with a relative speed index (RSI) 63 value ≤ 2, which is a measure of completion speed and serves as an indicator of conscientious working. The RSI is computed by dividing the completion time of a prompt by the median completion time of the participant. RSI values exceeding 1 indicate faster completion, while values below 1 denote slower completion. Prompts with TIME_RSI values above 2 indicate very quick responses and should be viewed critically. Furthermore, attentiveness was assessed using a verification item (“Please just click tend to agree here”) asked at the post-assessment, along with questions regarding honest answering. Four participants were excluded from the analysis who indicated at post-assessment that they had not answered conscientiously, while two participants were excluded for providing false responses to the verification item. Additionally, two participants were excluded due to a poor compliance rate, leaving 114 participants for all further analyses. Participants were required to answer the questions and interact with the slider to progress to the next question and complete the prompt. Consequently, missing values only occurred when an entire prompt was missing by a participant due to not responding. The analysis technique explained below assumes stationarity. Therefore, the individual time series were detrended by fitting fixed-effects linear regression models to each variable, regressing out linear trends based on day number and categorical effects on the measurement per day, using an alpha of 0.05.
To avoid topological overlap of items that were selected based on theoretical premises, especially in light of positive and negative worded items assessing the same construct, we evaluated the presence of multicollinearity and the possibility of node redundancy by calculating zero-order correlations between the six variables of interest (see Fig. 1 ). Correlations among items exhibit a range from moderately to large 67 , thus reinforcing the hypothesis of interconnected psychological processes 50 . However, none of the bivariate correlations exceeded standard cut-offs thresholds for multicollinearity, signifying the absence of item redundancy (r ≥ 0.80) 68 .
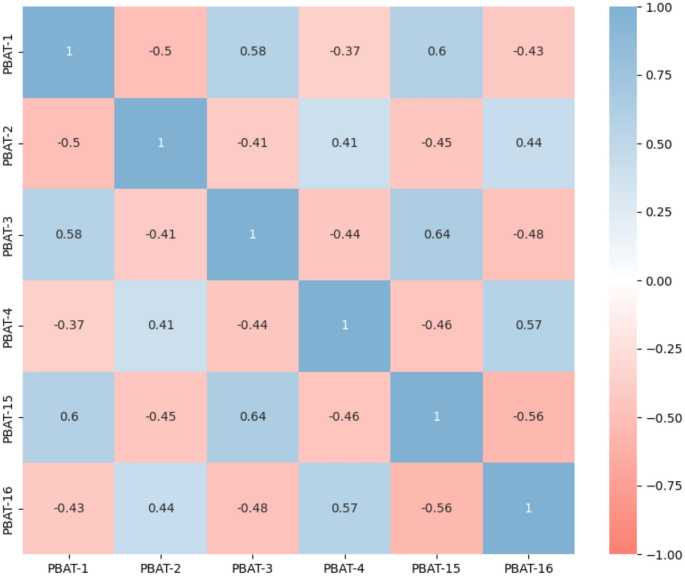
Correlation Matrix for the Items Under Investigation. Note PBAT1 = negative affect, PBAT2 = positive affect, PBAT3 = negative cognition, PBAT4 = positive cognition, PBAT15 = rigidity, PBAT16 = variation; Figure illustrates the strength of correlations between variables. Color intensity represents strength of correlation, from dark red (strong negative) to dark blue (strong positive).
Dynamic network analysis
The main objective of this study was to construct dynamic network models to investigate the dynamic associations between flexibility in the capacity to change behavior and cognitive-affective processes as young adults lived their daily lives. Networks are visually represented through graphs comprising nodes (variables) and edges (statistical relationships). Each of the processes was considered a node in the network.
For the estimation of dynamic network models, we used multilevel vector autoregression (VAR) with the R package mlVAR 69 . In a two-step procedure, mlVAR first obtains directed within-person temporal effects by performing node-wise multilevel regressions where each node is predicted by itself and all other nodes at the previous time point. The resulting temporal networks display directed edges, reflecting directed partial regression coefficients between two variables while controlling for all other relationships. In the second step, node-wise multilevel regressions with the residuals of the first step are conducted to obtain undirected contemporaneous networks. These contain partial correlations between the variables, controlled for the lag-1 temporal associations. Thickness and saturation of edges within graphical depictions indicate the strength of relationships, with positive edges shown in blue and negative edges in red. Both temporal and contemporaneous network structures were modeled for all nodes of interest.
For temporal networks, we computed node centrality using the centrality indices in-strength (IS) and out-strength (OS). In-strength centrality refers to the summation of all absolute values of the weighted edges directed towards a node, while the out-strength parameter of a node indicates the summation of the absolute values of all outgoing edges. Regarding contemporaneous networks, node centrality was determined using strength centrality. We investigated the stability of centrality measures with a case-drop bootstrap. We repeatedly dropped 20% of the sample at random 1000 times, refitted our models and recalculated the centrality measures. We then assessed the rank consistency of the nodes.
Descriptive statistics
Table 2 displays descriptive data representing the recorded processes for the group over time. Results indicate that, on average, participants experienced higher levels of positive affective and cognitive processes and variation as opposed to rigidity. Additionally, the sample tended to exhibit lower variability in their positive processes versus negative processes, as evidenced by lower standard deviations. Figure 2 illustrates the temporal progression of group processes, with both positive and negative processes exhibiting comparable patterns.
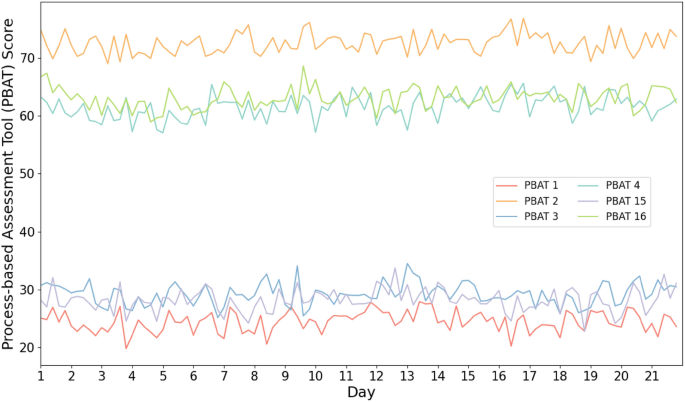
Temporal Evolution of Psychological Processes Averaged Across the Study Cohort. Note PBAT = Process-Based Assessment Tool; PBAT 1 = negative affect, PBAT 2 = positive affect, PBAT 3 = negative cognition, PBAT 4 = positive cognition, PBAT 15 = rigidity, PBAT 16 = variation.
Network model
Contemporaneous network models.
Figure 3 displays contemporaneous and temporal networks depicting the associations between psychological flexibility and cognitive-affective processes. Non-significant edges in the network were omitted from visualization. Only statistically significant relationships are described below.
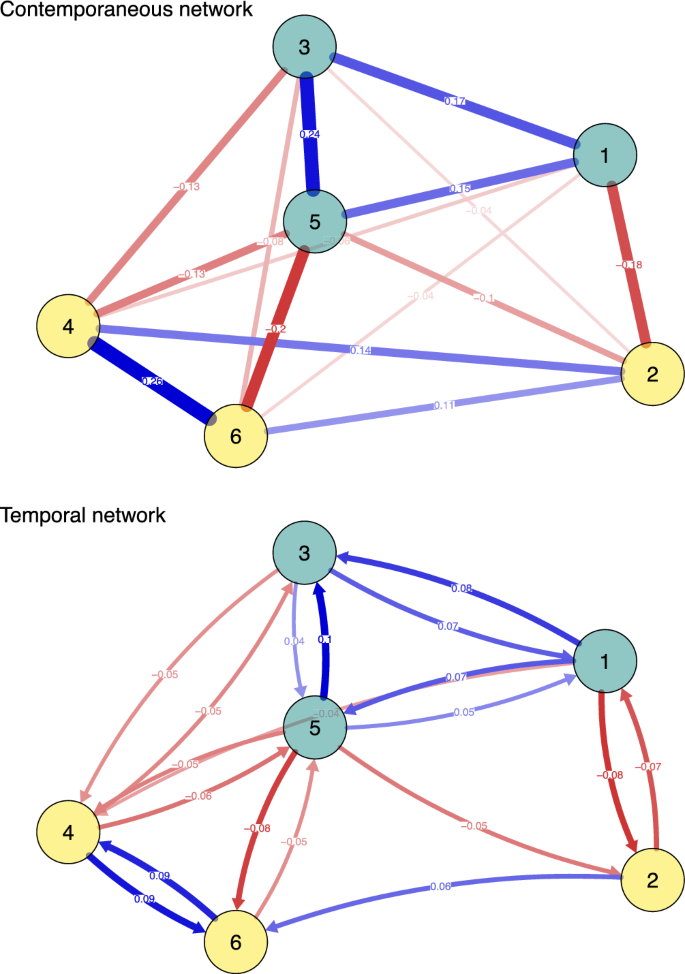
Networks of variation/rigidity, cognition, and affect. Note 1 = negative affect, 2 = positive affect, 3 = negative cognition, 4 = positive cognition, 5 = rigidity, 6 = variation; non-significant edges are omitted. In the contemporaneous network, all edges are non-directed. In the temporal network, all edges are directed. Thickness of edges indicates strength of associations. Blue edges indicate positive associations, red edges indicate negative associations.
In the contemporaneous network, which represents relationships among items at the same time point, several anticipated associations among items were identified. Notably, all nodes (i.e., psychological processes) were interconnected within the same time window (Fig. 3 ). As hypothesized, positive processes showed positive associations with each other, with a range of coefficients from r partial = 0.11 to r partial = 0.26. Negative processes were also positively related to each other, with a range of coefficients from r partial = 0.15 to r partial = 0.25. Positive processes were found to be negatively related to negative processes. Descriptively, rigidity exhibited stronger positive associations with negative processes, whereas variation showed stronger positive associations with positive processes.
In terms of centrality within the contemporaneous network, rigidity comparatively demonstrated the highest strength centrality (S = 0.83), matching our assumptions. This indicates that rigidity had comparatively the highest degree of connectivity within the contemporaneous network. Centrality estimates of the contemporaneous network can be found in the supplementary materials (Table 3). Stability analyses of the centrality indices indicated that rigidity had the highest strength centrality in all iterations of the case-drop bootstrap.
Temporal network models
In the temporal network structure, which depicts lag-1 relationships from one 3-h measurement period to the subsequent one, the results of the mlVAR network analyses revealed significant directed relationships both within and between nodes of the dimensions of processes, consistent with our assumptions (Fig. 3 ). Negative affective processes, negative cognitive processes, and rigidity were positively related to each other, with significant associations ranging from β = 0.05 to β = 0.10. This indicates that negative processes at a previous time point predicted an increased level of negative processes at the next measurement time. Regarding positive processes, bidirectional links were observed between variation and cognition, ranging from β = 0.09 to β = 0.10. Furthermore, positive affect at a previous time point predicted flexibility at the next measurement time ( β = 0.06), but not vice versa. Moreover, processes of the same construct (e.g., cognitive processes) negatively predicted each other at the next time point. Interestingly, rigidity was not only primarily related to negative cognitive-affective processes but likewise to positive processes.
Rigidity had the highest centrality in the temporal network, considering out-strength centrality (OS = 0.33), but not considering in-strength centrality (IS = 0.22). Negative cognition (IS = 0.26), and variation (IS = 0.24) comparatively showed the highest in-strength centrality, suggesting that these nodes were most associated with other nodes at earlier time points. Notably, only positive affective processes did not predict rigidity at the next time point. The centrality estimates of the temporal network can be found in Supplementary Table 3 . In the case-drop bootstrap, negative cognition had the highest in-strength centrality in 74.9% of iterations, while rigidity had the highest out-strength centrality in 88.8% of iterations.
To explore the functional relationships among a core feature of psychological flexibility – variation and rigidity in the capacity to change behavior – and positive and negative change processes (i.e., cognition, affect) in young adults, we utilized a time-series network approach. We examined both the temporal and contemporaneous network structures involving variation, rigidity, and negative and positive cognitive-affective processes.
In the temporal network, rigidity exhibited the highest out-strength. It predicted future experiences of negative cognitive-affective processes while inversely predicting future experiences of positive cognitive-affective processes as well as variation. This suggests that an individual’s overall flexibility at one point may be associated with the subsequent experiences of specific cognitive-affective processes. This finding aligns with our hypothesis and previous literature, which has underscored the pivotal role of rigidity in psychopathology 30 , 40 , 49 , 62 and aligns with our assumption that rigidity (OS = 0.33) would have a more central role compared to variation (OS = 0.14). However, this cannot be stated for in-strength, with respect to which variation (IS = 0.24) and rigidity (IS = 0.22) are roughly equal. However, the interpretation of the centrality estimates should be approached cautiously, given their similarity and the uncertainty in the estimation procedure.
Interestingly, the node representing variation showed the least connectivity with other nodes in the network. It had bidirectional connections only with rigidity and positive cognition. Positive affect also predicted variation. In view of the comparatively high in-strength, variation might be more likely to be considered an endpoint of connections in the network. Moreover, it is notable that rigidity appears to have relatively stronger outgoing connections to other variables compared to incoming ones in the temporal network. This discrepancy could give insight into the nature of rigidity. For example, rigidity may be less influenced by cognitive and affective processes than it influences them. In addition, it could act more as a starting point for processes and a central control point in the network activities. Further research is needed to explore if rigidity behaves similarly in the context of psychopathological symptoms. However, the differences between absolute centrality values are relatively small. While we have attempted to account for uncertainty, it should be noted that these differences may merely represent measurement noise.
In the contemporaneous network, nodes related to positive or negative behavior exhibited positive correlations with each other (e.g., positive cognition, affect, and variation), and nodes with opposite valence showed negative associations (e.g., positive cognition and negative affect). Furthermore, the results support our assumption that rigidity exhibits stronger associations with negative processes, whereas variation demonstrates stronger associations with positive processes. The positive associations within positive and negative processes, respectively, align with prior research, which has demonstrated robust positive associations among processes of the same valence and negative relationships between processes of opposite valence 23 , 38 , 70 , 71 .
The findings offer valuable insights into the dynamic interplay between a core feature of psychological flexibility and cognitive-affective processes in young adults. The centrality of rigidity emphasizes its potential relevance in shaping subsequent psychological experiences. The results suggest that rigidity may play a relevant role in driving the progression of psychological processes over time. Constricted psychological flexibility, characterized by feeling stuck and unable to change ineffective behaviors, may lead to subsequent maladaptive cognitive-affective processes. This underscores the potential relevance of impaired psychological flexibility for mental health.
As expected, both the contemporaneous and temporal networks display extensive connections between nodes, highlighting the highly interconnected nature of psychological processes 52 , 72 . In the temporal network, numerous feedback loops with nodes showing reciprocal connections to one another are evident. These feedback loops can contribute to system stability or instability 73 , 74 , 75 . They can lead to the stabilization and maintenance of both adaptive and maladaptive states. It is well-established that feedback loops between nodes significantly influence system stability, which may explain the persistence of mental disorders 76 , 77 . The identification of this aspect of psychological flexibility as a core process integrated within feedback loops with psychological processes such as cognition and affect holds great relevance for researchers and practitioners. Consequently, a key implication could be to prioritize the examination of this feature of psychological flexibility in future studies, given its pronounced associations with the entire system of cognitive-affective processes.
The findings of this study hold significant relevance for young adults in their everyday lives as they provide valuable insights into the relationships between psychological processes that may impact their emotions, thoughts, and behaviors. Results show that the psychological processes experienced by young adults are closely connected within short time intervals. The central role of rigidity indicates its pivotal role in shaping subsequent psychological experiences and underscores its importance in understanding mental health in this population. Young adults who tend to exhibit rigidity, feeling stuck and unable to change ineffective behaviors, may be more prone to experiencing negative psychological states, especially in light of this critical developmental period 2 , 78 , 79 . Recognizing the significance of psychological flexibility can empower young adults to cultivate more adaptive, flexible coping strategies, which may also improve mental health.
As previously mentioned, there is a need for the simultaneous examination of both positive and negative manifestations of psychological flexibility more generally and identification of its primary determinants 38 , 80 . Additionally, the development of effective measures for assessing psychological flexibility in longitudinal studies is crucial for identifying meaningful processes of change at an individual level 81 , 82 . A key objective for researchers and practitioners in clinical settings is to manipulate elements within the network to steer the system toward a trajectory leading to a desired target state 83 , 84 . This necessitates identifying elements within the network that can efficiently drive functional changes. The present study explores how rigidity and variation as features of behavior change impact cognitive-affective processes. It suggests that a more nuanced approach to understanding this core feature of psychological flexibility should be considered in future research and therapy. This newfound understanding has the potential to improve the development of more effective interventions targeting the capability to flexibly change behavior, thereby positively influencing psychological processes, such as cognition and affect. However, future studies in clinical settings will be necessary to confirm these assumptions and explore the impact of psychological flexibility in areas beyond cognition and affect as well.
One limitation of this study is the restricted sample, which consisted solely of students from a single institution. Consequently, future research should aim to explore associations between psychological flexibility, cognition, and affect in a more diverse and representative sample encompassing a broader range of mental disorders. Another limitation pertains to the reliance on self-report measures for assessing psychological constructs. Such self-report data may be influenced by social desirability or lack of awareness, potentially impacting the observed relationships’ strength. In the context of psychological flexibility, however, there are currently few alternatives to self-report ratings. In future work, it may be beneficial to consider supplementing self-report with other measures, such as physiological data (e.g., heart rate variability, metabolic flexibility, blood pressure), brain correlates, and overt behaviors while recording the context.
Furthermore, we assessed the stability of centrality measures with a case-drop bootstrap. It is unclear how well this method performs in stability assessment, and there are no clear guidelines on how to best assess uncertainty in centrality estimates in dynamic networks 85 . Another important limitation is that mlVAR is a multilevel model that assumes that network edges of different individuals come from a common distribution. It is possible that there are subgroups, or that individual-level effects are so different from one another that the use of more idiographic techniques (such as uSEM or GIMME 86 ) would be more appropriate. Ideally, such subgroup analyses would involve large data sets to find robust subgroups. As a first step, however, it seemed important to examine the dynamic network in a fashion that is more similar to current mainstream analytic practice.
While our hypothesis posits that incorporating the temporal dimension will enhance our comprehension of the temporal relationships among psychological flexibility, cognition, and affect, it is important to recognize that temporal associations and node centrality measures do not equate to causality 87 , 88 , 89 . Therefore, cautious interpretation is warranted, and future research should strive to establish causal relationships using longitudinal data and assess causal inference assumptions 90 .
Moreover, it is vital to consider that most network analyses, including the current study, operate under the assumption of a constant network structure, disregarding potential changes of mean or variance over time (i.e., stationarity). However, this assumption does not align with the dynamic nature of interventions and psychotherapeutic processes, where individuals’ experiences and responses evolve over time. To address the assumption of stationarity, future studies could employ innovative time-varying network models, capturing fluctuations in associations between variables across time points 91 , thus providing a more accurate representation of the evolving psychological processes. However, these analysis techniques need a large amount of data 92 and are therefore often infeasible in typical psychological applications. Time-varying network models become particularly pertinent when examining the experimental influence of psychological flexibility on cognitive-affective processes.
Finally, participants received a relatively high payment for participation in the EMA phase. While it was also intended to compensate for the burden of wearing wearables required for future analyses, the reimbursement was based on response rate, with higher payment per prompt for higher response rates. This could have led to lower-quality responses. However, the remuneration system was intentionally selected to produce a significant amount of data, resulting in more reliable and meaningful results. To address the risk of invalid data, strict preprocessing steps were taken as described. Additionally, participants were given the option to anonymously indicate at the end if they had responded carelessly (such as to receive higher payment). The participants were informed that their payment would not be influenced by the disclosure. Data from participants who disclosed careless responding were excluded from the analyses.
The present study contributes to the growing body of literature indicating the significance of psychological flexibility as a crucial transdiagnostic process for psychological phenomena among young adults. Overall psychological flexibility and its relation to cognitive-affective processes may play a crucial role in mental health. Future research should examine specific intervention strategies to target such maladaptive networks, such as through network control theory 93 .
Data availability
All data generated or analyzed during this study are included in this published article (and its Supplementary Information files).
Zarrett, N. & Eccles, J. The passage to adulthood: Challenges of late adolescence. New Dir. Youth Dev. 2006 , 13–28 (2006).
Article Google Scholar
Branje, S., de Moor, E. L., Spitzer, J. & Becht, A. I. Dynamics of identity development in adolescence: A decade in review. J. Res. Adolesc. 31 , 908–927 (2021).
Article PubMed PubMed Central Google Scholar
Taber-Thomas, B. & Perez-Edgar, K. Emerging Adulthood Brain Development. https://doi.org/10.1093/oxfordhb/9780199795574.013.15 . (2015).
Arnett, J. J., Žukauskienė, R. & Sugimura, K. The new life stage of emerging adulthood at ages 18–29 years: Implications for mental health. Lancet Psychiatry 1 , 569–576 (2014).
Article PubMed Google Scholar
Solmi, M. et al. Age at onset of mental disorders worldwide: Large-scale meta-analysis of 192 epidemiological studies. Mol. Psychiatry 27 , 281–295 (2022).
Article CAS PubMed Google Scholar
Dawson, D. L. & Golijani-Moghaddam, N. COVID-19: Psychological flexibility, coping, mental health, and wellbeing in the UK during the pandemic. J. Context. Behav. Sci. 17 , 126–134 (2020).
Gloster, A. T. et al. Psy-Flex: A contextually sensitive measure of psychological flexibility. J. Context. Behav. Sci. 22 , 13–23 (2021).
Hayes, S. C. Acceptance and commitment therapy, relational frame theory, and the third wave of behavioral and cognitive therapies. Behav. Therapy 35 , 639–665 (2004).
Kashdan, T. B. Psychological flexibility as a fundamental aspect of health. Clin. Psychol. Rev. 30 , 865–878 (2010).
Kashdan, T. B., Disabato, D. J., Goodman, F. R., Doorley, J. D. & McKnight, P. E. Understanding psychological flexibility: A multimethod exploration of pursuing valued goals despite the presence of distress. Psychol. Assess. 32 , 829–850 (2020).
Lucas, J. J. & Moore, K. A. Psychological flexibility: Positive implications for mental health and life satisfaction. Health Promot. Int. 35 , 312–320 (2019).
Chin, F. & Hayes, S. C. Chapter 7 - Acceptance and commitment therapy and the cognitive behavioral tradition: assumptions, model, methods, and outcomes. In The Science of Cognitive Behavioral Therapy (eds Hofmann, S. G. & Asmundson, G. J. G.) 155–173 (Academic Press, San Diego, 2017).
Chapter Google Scholar
Hayes, S. C., Strosahl, K. & Wilson, K. Acceptance and Commitment Therapy : An Experiential Approach to Behavior Change / S.C. Hayes, K.D. Strosahl, K.G. Wilson. (2011).
Sahdra, B., Ciarrochi, J. & Parker, P. Nonattachment and mindfulness: Related but distinct constructs. Psychol. Assess. 28 , 819–829 (2016).
Sawyer, S. M., Azzopardi, P. S., Wickremarathne, D. & Patton, G. C. The age of adolescence. Lancet Child Adolesc. Health 2 , 223–228 (2018).
Schulenberg, J. E., Sameroff, A. J. & Cicchetti, D. The transition to adulthood as a critical juncture in the course of psychopathology and mental health. Dev. Psychopathol. 16 , 799–806 (2004).
Hofmann, S. G., Asmundson, G. J. G. & Beck, A. T. The science of cognitive therapy. Behav. Ther. 44 , 199–212 (2013).
Gross, J. J. & Barrett, L. F. Emotion generation and emotion regulation: One or two depends on your point of view. Emot. Rev. 3 , 8–16 (2011).
Hofmann, S. G., Sawyer, A. T., Fang, A. & Asnaani, A. Emotion dysregulation model of mood and anxiety disorders. Depress. Anxiety 29 , 409–416 (2012).
Berghoff, C. R., Ritzert, T. R. & Forsyth, J. P. Value-guided action: Within-day and lagged relations of experiential avoidance, mindful awareness, and cognitive fusion in a non-clinical sample. J. Context. Behav. Sci. 10 , 19–23 (2018).
George, J. M. & Dane, E. Affect, emotion, and decision making. Organ. Behav. Human Decis. Process. 136 , 47–55 (2016).
Lindquist, K. & Barrett, L. Emotional complexity. in Emotional Complexity 513–530 (2015).
Ciarrochi, J., Sahdra, B., Hofmann, S. G. & Hayes, S. C. Developing an item pool to assess processes of change in psychological interventions: The Process-Based Assessment Tool (PBAT). J. Context. Behav. Sci. 23 , 200–213 (2022).
Fusar-Poli, P. et al. Transdiagnostic psychiatry: A systematic review. World Psychiatry 18 , 192–207 (2019).
Heinrichs, N. & Hofmann, S. G. Information processing in social phobia: A critical review. Clin. Psychol. Rev. 21 , 751–770 (2001).
Heshmati, S., DavyRomano, E., Chow, C., Doan, S. N. & Reynolds, K. D. Negative emodiversity is associated with emotional eating in adolescents: An examination of emotion dynamics in daily life. J. Adolesc. 95 , 115–130 (2023).
Benoit, R. G., Davies, D. J. & Anderson, M. C. Reducing future fears by suppressing the brain mechanisms underlying episodic simulation. Proc. Natl. Acad. Sci. 113 , E8492–E8501 (2016).
Article ADS CAS PubMed PubMed Central Google Scholar
Galhardo, R. S., Hastings, P. J. & Rosenberg, S. M. Mutation as a stress response and the regulation of evolvability. Crit. Rev. Biochem. Mol. Biol. 42 , 399–435 (2007).
Article CAS PubMed PubMed Central Google Scholar
Wagner, G. P. & Draghi, J. Evolution of evolvability. In Evolution—The Extended Synthesis (eds Pigliucci, M. & Müller, G. B.) 379–400 (The MIT Press, 2010).
Google Scholar
Morris, L. & Mansell, W. A systematic review of the relationship between rigidity/flexibility and transdiagnostic cognitive and behavioral processes that maintain psychopathology. J. Exp. Psychopathol. 9 , 2043808718779431 (2018).
Steinmetz, J.-P., Loarer, E. & Houssemand, C. Rigidity of attitudes and behaviors: A study on the validity of the concept. Individ. Differ. Res. 9 , 84–106 (2011).
Ciarrochi, J., Hayes, S. C., Oades, L. G. & Hofmann, S. G. Toward a unified framework for positive psychology interventions: Evidence-based processes of change in coaching, prevention, and training. Front Psychol. 12 , 809362 (2021).
Hayes, S. C., Hofmann, S. G. & Ciarrochi, J. A process-based approach to psychological diagnosis and treatment: The conceptual and treatment utility of an extended evolutionary meta model. Clin. Psychol. Rev. 82 , 101908 (2020).
Hayes, S. C., Hofmann, S. G. & Wilson, D. S. Clinical psychology is an applied evolutionary science. Clin. Psychol. Rev. 81 , 101892 (2020).
Hersh, M. N., Ponder, R. G., Hastings, P. J. & Rosenberg, S. M. Adaptive mutation and amplification in Escherichia coli : Two pathways of genome adaptation under stress. Res. Microbiol. 155 , 352–359 (2004).
Campbell, D. T. Blind variation and selective retention in creative thought as in other knowledge processes. Psychol. Rev. 67 , 380–400 (1960).
Skinner, B. F. Selection by consequences. Science 213 , 501–504 (1981).
Article ADS CAS PubMed Google Scholar
Hayes, S. C., Ciarrochi, J., Hofmann, S. G., Chin, F. & Sahdra, B. Evolving an idionomic approach to processes of change: Towards a unified personalized science of human improvement. Behav. Res. Therapy 156 , 104155 (2022).
Giommi, F. et al. The (In)flexible self: Psychopathology, mindfulness, and neuroscience. Int. J. Clin. Health Psychol. 23 , 100381 (2023).
Levin, M. E. et al. Exploring the relationship between experiential avoidance, alcohol use disorders, and alcohol-related problems among first-year college students. J. Am. College Health 60 , 443–448 (2012).
Levin, M. E. et al. Examining psychological inflexibility as a transdiagnostic process across psychological disorders. J. Context. Behav. Sci. 3 , 155–163 (2014).
Rawal, A., Park, R. J. & Williams, J. M. G. Rumination, experiential avoidance, and dysfunctional thinking in eating disorders. Behav. Res. Therapy 48 , 851–859 (2010).
Hailikari, T., Nieminen, J. & Asikainen, H. The ability of psychological flexibility to predict study success and its relations to cognitive attributional strategies and academic emotions. Educ. Psychol. 42 , 626–643 (2022).
Hulbert-Williams, N. J. & Storey, L. Psychological flexibility correlates with patient-reported outcomes independent of clinical or sociodemographic characteristics. Support Care Cancer 24 , 2513–2521 (2016).
Ii, T. et al. Psychological flexibility-based interventions versus first-line psychosocial interventions for substance use disorders: Systematic review and meta-analyses of randomized controlled trials. J. Context. Behav. Sci. 13 , 109–120 (2019).
Lloyd, J., Bond, F. W. & Flaxman, P. E. The value of psychological flexibility: Examining psychological mechanisms underpinning a cognitive behavioural therapy intervention for burnout. Work Stress 27 , 181–199 (2013).
Bond, F. W. et al. Preliminary psychometric properties of the acceptance and action questionnaire-II: A revised measure of psychological inflexibility and experiential avoidance. Behav. Ther. 42 , 676–688 (2011).
Ciarrochi, J., Godsell, C. & Bilich, L. Psychological flexibility as a mechanism of change in acceptance and commitment therapy. Faculty Health Behav. Sci. Pap. (Archive) 2010 , 51–75 (2010).
Doorley, J. D., Goodman, F. R., Kelso, K. C. & Kashdan, T. B. Psychological flexibility: What we know, what we do not know, and what we think we know. Soc. Personal. Psychol. Compass 14 , e12566 (2020).
Hofmann, S. G., Curtiss, J. E. & Hayes, S. C. Beyond linear mediation: Toward a dynamic network approach to study treatment processes. Clin. Psychol. Rev. 76 , 101824 (2020).
Shiffman, S., Stone, A. A. & Hufford, M. R. Ecological momentary assessment. Annu. Rev. Clin. Psychol. 4 , 1–32 (2008).
Hofmann, S. G., Curtiss, J. & McNally, R. J. A complex network perspective on clinical science. Perspect. Psychol. Sci. 11 , 597–605 (2016).
Borsboom, D. A network theory of mental disorders. World Psychiatry 16 , 5–13 (2017).
Bringmann, L. F. et al. What do centrality measures measure in psychological networks?. J. Abnorm. Psychol. 128 , 892–903 (2019).
Granger, C. W. J. Investigating causal relations by econometric models and cross-spectral methods. Econometrica 37 , 424–438 (1969).
Gates, K. M. & Molenaar, P. C. M. Group search algorithm recovers effective connectivity maps for individuals in homogeneous and heterogeneous samples. NeuroImage 63 , 310–319 (2012).
Fisher, A. J., Medaglia, J. D. & Jeronimus, B. F. Lack of group-to-individual generalizability is a threat to human subjects research. Proc. Natl. Acad. Sci. 115 , E6106–E6115 (2018).
Molenaar, P. C. M. On the necessity to use person-specific data analysis approaches in psychology. Eur. J. Dev. Psychol. 10 , 29–39 (2013).
Chen, B. et al. Basic psychological need satisfaction, need frustration, and need strength across four cultures. Motiv. Emot. 39 , 216–236 (2015).
Hayes, S. C., Strosahl, K. & Wilson, K. G. Acceptance and Commitment Therapy: An Experiential Approach to Behavior Change (Guilford Press, 2003).
Faustino, B. Transdiagnostic perspective on psychological inflexibility and emotional dysregulation. Behav. Cogn. Psychother. 49 , 233–246 (2021).
Nolen-Hoeksema, S., Wisco, B. E. & Lyubomirsky, S. Rethinking rumination. Perspect. Psychol. Sci. 3 , 400–424 (2008).
Hayes, S. C. A Liberated Mind: The Essential Guide to ACT (Random House, UK, 2019).
Ong, C. W., Ciarrochi, J., Hofmann, S. G., Karekla, M. & Hayes, S. C. Through the extended evolutionary meta-model, and what ACT found there: ACT as a process-based therapy. J. Context. Behav. Sci. 32 , 100734. https://doi.org/10.1016/j.jcbs.2024.100734 (2024).
Van Rossum, G. & Drake, F. L. Python 3 Reference Manual: (Python Documentation Manual Part 2) (CreateSpace Independent Publishing Platform, 2009).
R Core Team. R: A Language and Environment for Statistical Computing . (R Foundation for Statistical Computing, 2022).
Cohen, J. Statistical Power Analysis for the Behavioral Sciences (L. Erlbaum Associates, Hillsdale, 1988).
Berry, W. & Feldman, S. Multiple Regression in Practice (Thousand Oaks, 2023).
Epskamp, S., Waldorp, L. J., Mõttus, R. & Borsboom, D. The Gaussian graphical model in cross-sectional and time-series data. Multivar. Behav. Res. 53 , 453–480 (2018).
Ciarrochi, J. et al. A personalised-approach to identifying important determinants of well-being. Preprint at https://doi.org/10.31219/osf.io/m4zhw (2023).
Curtiss, J. E., Pinaire, M., Fulford, D., McNally, R. J. & Hofmann, S. G. Temporal and contemporaneous network structures of affect and physical activity in emotional disorders. J. Affect. Disord. 315 , 139–147 (2022).
Hayes, S. C. & Hofmann, S. G. ‘Third-wave’ cognitive and behavioral therapies and the emergence of a process-based approach to intervention in psychiatry. World Psychiatry 20 , 363–375 (2021).
Bose, E., Hravnak, M. & Sereika, S. M. Vector autoregressive (VAR) models and granger causality in time series analysis in nursing research: Dynamic changes among vital signs prior to cardiorespiratory instability events as an example. Nurs. Res. 66 , 12–19 (2017).
Curtiss, J. E., Ito, M., Takebayashi, Y. & Hofmann, S. G. Longitudinal network stability of the functional impairment of anxiety and depression. Clin. Psychol. Sci. 6 , 325–334 (2018).
Walsh, G. C., Ye, H. & Bushnell, L. G. Stability analysis of networked control systems. IEEE Trans. Control Syst. Technol. 10 , 438–446 (2002).
Scheffer, M. Foreseeing tipping points. Nature 467 , 411–412 (2010).
van de Leemput, I. A. et al. Critical slowing down as early warning for the onset and termination of depression. PNAS Proc. Natl. Acad. Sci. U. S. A. 111 , 87–92 (2014).
Article ADS PubMed Google Scholar
Hauser, T. U. On the development of OCD. Curr. Top. Behav. Neurosci. 49 , 17–30 (2021).
Larsen, B. & Luna, B. Adolescence as a neurobiological critical period for the development of higher-order cognition. Neurosci. Biobehav. Rev. 94 , 179–195 (2018).
Cherry, K. M., Hoeven, E. V., Patterson, T. S. & Lumley, M. N. Defining and measuring ‘psychological flexibility’: A narrative scoping review of diverse flexibility and rigidity constructs and perspectives. Clin. Psychol. Rev. 84 , 101973 (2021).
Bringmann, L. F. et al. A network approach to psychopathology: new insights into clinical longitudinal data. PLoS ONE 8 , e60188 (2013).
Hayes, S. C. et al. The role of the individual in the coming era of process-based therapy. Behav. Res. Ther. 117 , 40–53 (2019).
Henry, T. R., Robinaugh, D. J. & Fried, E. I. On the control of psychological networks. Psychometrika 87 , 188–213 (2022).
Article MathSciNet PubMed Google Scholar
Liu, Y.-Y., Slotine, J.-J. & Barabási, A.-L. Controllability of complex networks. Nature 473 , 167–173 (2011).
Blanchard, M. A., Contreras, A., Kalkan, R. B. & Heeren, A. Auditing the research practices and statistical analyses of the group-level temporal network approach to psychological constructs: A systematic scoping review. Behav. Res. Methods 55 , 767–787 (2023).
Yang, X. et al. Socioemotional dynamics of emotion regulation and depressive symptoms: A person-specific network approach. Complexity 2018 , 5094179 (2018).
Borsboom, D. et al. Network analysis of multivariate data in psychological science. Nat. Rev. Methods Primers 1 , 1–18 (2021).
Dablander, F. & Hinne, M. Node centrality measures are a poor substitute for causal inference. Sci. Rep. 9 , 6846 (2019).
Article ADS PubMed PubMed Central Google Scholar
Spiller, T. R. et al. On the validity of the centrality hypothesis in cross-sectional between-subject networks of psychopathology. BMC Med. 18 , 297 (2020).
Huang, D., Susser, E., Rudolph, K. E. & Keyes, K. M. Depression networks: A systematic review of the network paradigm causal assumptions. Psychol. Med. 53 , 1665–1680. https://doi.org/10.1017/S0033291723000132 (2023).
Siepe, B. et al. Temporal Dynamics of Depressive Symptomatology: An Idiographic Time Series Analysis Applying Network Models to Patients with Depressive Disorders . https://doi.org/10.31234/osf.io/hnw69 , https://osf.io/hnw69 . (2022)
Haslbeck, J. M. B., Bringmann, L. F. & Waldorp, L. J. A tutorial on estimating time-varying vector autoregressive models. Multivar. Behav. Res. 56 , 120–149 (2021).
Stocker, J. E. et al. Formalizing psychological interventions through network control theory. Sci. Rep. 13 (1), 13830 (2023).
Download references
Acknowledgements
All authors would like to thank Susanne Grünwald, Nicole Weber, and Jovan Dieckmann for their support in data acquisition. This research was supported by the Alexander von Humboldt Foundation (as part of the Alexander von Humboldt Professur of SGH), the Hessische Ministerium für Wissenschaft und Kunst (as part of the LOEWE Spitzenprofessur of SGH), and the DYNAMIC center, funded by the LOEWE program of the Hessian Ministry of Science and Arts (Grant Number: LOEWE1/16/519/03/09.001(0009)/98).
Open Access funding enabled and organized by Projekt DEAL.
Author information
Authors and affiliations.
Department of Psychology, Philipps-University of Marburg, Schulstraße 12, 35032, Marburg, Germany
Marlon Westhoff, Christoph Vogelbacher & Stefan G. Hofmann
Department of Psychology, Claremont Graduate University, Claremont, CA, USA
Saida Heshmati
Department of Psychology, Philipps-University of Marburg, Marburg, Germany
Björn Siepe
Institute for Positive Psychology and Education, Australian Catholic University, Sydney, Australia
Joseph Ciarrochi
Department of Psychology, University of Nevada Reno, Reno, NV, USA
Steven C. Hayes
You can also search for this author in PubMed Google Scholar
Contributions
M.W., S.H. and S.G.H. conceptualized the project. M.W., C.V., and S.H. curated the data. M.W., B.S. and C.V. analyzed the data. M.W., B.S. and S.H. wrote the original draft and all authors reviewed and edited the manuscript. All authors approved the final version of the article.
Corresponding author
Correspondence to Stefan G. Hofmann .
Ethics declarations
Competing interests.
The authors declare no competing interests.
Additional information
Publisher's note.
Springer Nature remains neutral with regard to jurisdictional claims in published maps and institutional affiliations.
Supplementary Information
Supplementary information 1., supplementary information 2., supplementary information 3., rights and permissions.
Open Access This article is licensed under a Creative Commons Attribution 4.0 International License, which permits use, sharing, adaptation, distribution and reproduction in any medium or format, as long as you give appropriate credit to the original author(s) and the source, provide a link to the Creative Commons licence, and indicate if changes were made. The images or other third party material in this article are included in the article's Creative Commons licence, unless indicated otherwise in a credit line to the material. If material is not included in the article's Creative Commons licence and your intended use is not permitted by statutory regulation or exceeds the permitted use, you will need to obtain permission directly from the copyright holder. To view a copy of this licence, visit http://creativecommons.org/licenses/by/4.0/ .
Reprints and permissions
About this article
Cite this article.
Westhoff, M., Heshmati, S., Siepe, B. et al. Psychological flexibility and cognitive-affective processes in young adults’ daily lives. Sci Rep 14 , 8182 (2024). https://doi.org/10.1038/s41598-024-58598-3
Download citation
Received : 12 December 2023
Accepted : 01 April 2024
Published : 08 April 2024
DOI : https://doi.org/10.1038/s41598-024-58598-3
Share this article
Anyone you share the following link with will be able to read this content:
Sorry, a shareable link is not currently available for this article.
Provided by the Springer Nature SharedIt content-sharing initiative
By submitting a comment you agree to abide by our Terms and Community Guidelines . If you find something abusive or that does not comply with our terms or guidelines please flag it as inappropriate.
Quick links
- Explore articles by subject
- Guide to authors
- Editorial policies
Sign up for the Nature Briefing newsletter — what matters in science, free to your inbox daily.

Cognitive flexibility across the sleep-wake cycle: REM-sleep enhancement of anagram problem solving
Affiliation.
- 1 Department of Psychiatry, Harvard Medical School, 74 Fenwood Road, Boston, MA 02115, USA. [email protected]
- PMID: 12421655
- DOI: 10.1016/s0926-6410(02)00134-9
Flexible or 'fluid' cognitive processes are regarded as fundamental to problem solving and creative ability, requiring a specific neurophysiological milieu. REM-sleep dreaming is associated with creative processes and abstract reasoning with increased strength of weak associations in cognitive networks. REM sleep is also mediated by a distinctive neurophysiological profile, different to that of wake and NREM sleep. This study compared the performance of 16 subjects on a test of cognitive flexibility using anagram word puzzles following REM and NREM awakenings across the night, and waking performances during the day. REM awakenings provided a significant 32% advantage in the number of anagrams solved compared with NREM awakenings and was equal to that of wake time trials. Correlations of individual performance profiles suggest that REM sleep may offer a different mode of problem solving compared with wake and NREM. When early and late REM and NREM awakening data were separated, a dissociation was evident, with NREM task performance becoming more REM-like later in the night, while REM performance remained constant. These data suggest that the neurophysiology of REM sleep represents a brain state more amenable to flexible cognitive processing than NREM and different from that in wake, and may offer insights into the neurocognitive properties of REM-sleep dreaming.
Publication types
- Research Support, U.S. Gov't, Non-P.H.S.
- Research Support, U.S. Gov't, P.H.S.
- Cognition / physiology*
- Dreams / physiology
- Dreams / psychology
- Problem Solving*
- Sleep Stages / physiology
- Sleep, REM / physiology*
- Wakefulness / physiology*
Grants and funding
- DA11744-01A1/DA/NIDA NIH HHS/United States
- MH-48832/MH/NIMH NIH HHS/United States

IMAGES
VIDEO
COMMENTS
We critically evaluate evidence for the enhancement of flexibility through cognitive training, physical activity and bilingual experience. ... flexibility and problem-solving in children with ASD 116.
With the rise of cognitive psychology, a more structured approach to the study of flexibility in problem solving was adopted. Researchers started taking an interest on the different variables that can affect subjects' (in)flexible choice of strategies and/or representations to solve particular problems.
Task switching as a marker of cognitive flexibility. Our brief review will focus on regulation of cognitive flexibility in the context of studies investigating task switching (for reviews, see Kiesel et al., 2010; Vandierendonck et al., 2010).Cognitive flexibility has also been studied using creative problem solving, or rule reversal learning paradigms, like the Wisconscin Card Sorting Test.
Introduction. Cognitive control and (cognitive) flexibility play a fundamental role in the ability to adapt to continuously changing environments and have been associated with various goal-oriented behaviors, including creativity, problem-solving, multi-tasking, and decision-making (Rolls, 2000; Ionescu, 2012; Dajani and Uddin, 2015).Cognitive control, and the processes underlying this ability ...
Cognitive flexibility is a valuable skill that can greatly enhance problem-solving abilities. With its neurological foundations rooted in plasticity and pathways, cognitive flexibility provides individuals with the tools they need to approach challenges from different angles and find creative solutions.
In this chapter, all the works presented show how contexts and contents of the situations on which our reasoning influence the expression of cognitive flexibility, which can slow down promote the adoption of a new point of view on a concept, a situation problem.
An executive function intervention known as Unstuck and On Target aims to address insistence on sameness, flexibility, goal setting and planning using a cognitive behavioural programme. This intervention has been shown to be effective for improving classroom behaviour, flexibility and problem-solving in children with ASD 116.
This ability can be acquired through experience and involves the adaptation of cognitive strategies that govern problem-solving processes. Cognitive flexibility is not limited to discrete ...
2015). In the context of problem-solving, Singer assumes cognitive flexibility in students who can propose solutions to new problems that differ from diverse solution strategies, produce novel solutions, and change previous frame of mind. Cognitive flexibility occurs when students can change ideas and approach
Cognitive Flexibility. Cognitive flexibility refers to the ability to shift attentional resources across perceptual attributes of complex, behaviorally relevant stimuli in response to changes in environmental contingencies (Robbins, 2007). ... We agree with this literature that solving a problem like "3 ...
Teaching and fostering critical thinking skills is being recognized as a key objective within educational settings. This chapter introduces critical thinking in two different ways: first as a philosophical concept and second as a psychological concept. Philosophy and psychology are the disciplines most directly concerned with critical thinking.
Cognitive flexibility will be best represented by more than one latent variable. Structure learning scores will be associated with at least one of the cognitive flexibility latent variables. Cognitive flexibility latent variable(s) will be associated with at least one primary outcome variable (creativity, literacy, numeracy, and problem solving)
Single step forward when solving a complex problem; Your brain can shift from "zoomed in" to the micro (the product) to "zoomed out" to the macro (the industry). As a result, cognitive flexibility allows you to solve problems creatively, adapt to curveballs, and act appropriately in varying situations. This is because you're able to ...
There is consensus in the literature concerning the importance of adaptation to the school for problem-solving skills (Canas et al., 2003). Thus, contemporary studies ... M. A. (2021). Self-leadership perception and emotional intelligence as the predictors of cognitive flexibility. problems of education in the 21st century, 79(5), 700-715 ...
Problem Solving / physiology*. Cognitive flexibility allows individuals to adapt to novel situations. However, this ability appears to develop slowly over the first few years of life, mediated by task complexity and opacity. We used a physically simple novel task, previously tested with nonhuman primates, to explore the developme ….
The frontal regions are associated with higher cognitive processes such as decision-making and problem solving. The striatal regions are instead linked with reward and motivation. Some people have ...
In response, we would argue that in the scheme of one's overall problem-solving exposure, even routine problems are opportunities to observe flexibility. First, it is interesting to note that we tend not to think of intelligence as a propensity to plod through solving routine, algorithmic problems in routine ways.
Cognitive Flexibility Theory and its Application to Learning Resources. Jiaming Cheng Tiffany A. Koszalka. Education, Psychology. 2016. Cognitive Flexibility Theory (CFT) suggests that deep learning requires learners to engage with new content from multiple perspectives and in flexible ways of thinking.
Psychological flexibility plays a crucial role in how young adults adapt to their evolving cognitive and emotional landscapes. Our study investigated a core aspect of psychological flexibility in ...
This paper describes one experiment and a research methodology designed to test the hypothesis that when people use a problem-solving strategy, their performance is only affected by those conditions which are relevant to that particular strategy. Subjects were 84 students who were asked to undertake 22 trials, where 16 of them had constant conditions and the last 6 had variable conditions. The ...
ability of cognitive flexibility in solving mathematical problems. Then , the results of the investigation of the completion process proved that Sub1 was able to assess the magnitude
Abstract. Flexible or 'fluid' cognitive processes are regarded as fundamental to problem solving and creative ability, requiring a specific neurophysiological milieu. REM-sleep dreaming is associated with creative processes and abstract reasoning with increased strength of weak associations in cognitive networks.
Cognitive control uses highly generalised representations and problem solving methods which are an inefficient means for producing the specialised responses of skill (Anderson, 1982 ). In other words, cognitive control is specialised for reasoning, not action control, and it is a clumsy tool to use for action control.
In classroom learning, students need mathematical cognitive flexibility to be able to solve mathematical problems with the various ideas they express. To solve the problems, they must be able to grasp the problem, see it from various points of view, and should not be rigid thinking with one solving method. In fact, the students still lack the ability to think flexibly in solving math problems ...