
An official website of the United States government
The .gov means it’s official. Federal government websites often end in .gov or .mil. Before sharing sensitive information, make sure you’re on a federal government site.
The site is secure. The https:// ensures that you are connecting to the official website and that any information you provide is encrypted and transmitted securely.
- Publications
- Account settings
Preview improvements coming to the PMC website in October 2024. Learn More or Try it out now .
- Advanced Search
- Journal List
- Front Mol Neurosci
- PMC10511750

Conflicting theories on addiction aetiology and the strengths and limitations of substance use disorder disease modelling
A lack of cross-disciplinary unanimity prevails within addiction research. Theories conceptualizing addiction through the three-stage brain disease model contest other theories that substance use disorder is of behavioural or genetic origin. The reverberations of this lack of consensus are noticeable throughout addiction research and within the foundations of disease modelling. The availability of methods to investigate substance use disorder are inconsistent and sometimes unrepresentative. This review discusses theories of addiction aetiology, available models for addiction research and the strengths and limitations of current practical experimental methods of study.
1. Introduction
Construing a universally accepted definition for addiction has been a controversial matter since deliberations arose over the first documented cases of substance abuse in the 17 th century ( Crocq, 2007 ). At this time, conflicting opinions existed on whether to categorise addiction as a sin or a disease ( Nathan et al., 2016 ). The unravelling of the cause and symptomatology of addiction has diluted morality arguments and replaced them with the concept of addiction as a brain disease (culminating in the US National Institute on Drug Abuse 1997 report) ( Heilig et al., 2021 ). However, cross-disciplinary variation in acceptance of this definition prevails. These contrasting theories make unanimity on defining addiction a constant challenge ( Hammer et al., 2013 ).
2. Theories of addiction
2.1. addiction: a brain disease.
The conceptual framework behind addiction as a brain disease is the reality that drugs of abuse have demonstrated the potential to alter the neural circuitry and molecular constituency of the brain ( Leshner, 1997 ).
The transition from acute, controlled, or occasional usage to chronic drug addiction is equally paralleled with neurochemical and molecular changes. These changes can be grouped into a three-stage addiction cycle of ‘binge/intoxication’, ‘withdrawal/negative effect’ and ‘preoccupation/anticipation’ in which distinct neural remodelling is clear within stages ( Koob and Volkow, 2010 ).
2.1.1. Binge/intoxication
All drugs of abuse result in excessive dopaminergic transmission within the brain’s reward circuitry, the mesolimbic system, which originates in the ventral tegmental area (VTA) and terminates in the nucleus accumbens (NAc) ( Adinoff, 2004 ). Aside from the mesolimbic dopaminergic system, heightened understanding of drug reward has revealed additional mechanisms influencing mesolimbic circuitry; the endogenous opioid system and the endogenous cannabinoid system ( Volkow et al., 2019 ). Other neurotransmitters, particularly glutamate, have also been proven to augment acute drug reward ( D'Souza, 2015 ). Thus, reward can be generalised as increased neurotransmission by substances of abuse within the mesolimbic system.
2.1.2. Withdrawal/negative effect
The persistent negative state experienced during drug abstinence, colloquially known as ‘antireward’, is analogous with upregulation of the corticotropin-releasing factor (CRF) system within the extended amygdala ( Koob and Le Moal, 2008 ). The result of hyperactive CRF-containing neurons in the amygdala is recruitment of the hypothalamic–pituitary–adrenal axis and brain stress systems ( Nestler, 2005 ). Other stress-related neurotransmitters including noradrenaline, dynorphin, vasopressin and substance P are also overexpressed ( Kwako and Koob, 2017 ). Activation of these stress systems correlate to the negative emotional state experienced during withdrawal ( Koob, 2009 ). Additionally, opposing neuroadaptations to reward processes occur. This results in a reduction in dopaminergic neurotransmission and therefore diminished positive response ( Feltenstein, 2008 ). Heightened stress and depreciated reward pathways amalgamate during this stage and present as withdrawal and resulting compulsion.
2.1.3. Preoccupation/anticipation
An auxiliary of the preoccupation and anticipation stage is chronic relapse ( Gutman, 2006 ). Further reorganisation of reward circuits and neuroplastic changes, predominantly localised to the prefrontal cortex (PFC), echo this phenomenon ( Koob and Simon, 2009 ; Veerappa et al., 2021 ). Alterations of the PFC including reduction of overall grey matter volume or disruption in specific regions such as the dorsolateral PFC correlate to impaired response inhibition and salience attribution ( Goldstein and Volkow, 2011 ). These neuroplastic changes result in over activation of ‘go’ systems and under activation of ‘stop’ systems, which are causative of habitual substance seeking and increases in impulsivity ( Substance Abuse and Mental Health Services Administration (US) and Office of the Surgeon General (US), 2016 ). Neural network remodelling manifests physically as intense craving and a loss of self-control.
2.1.4. Three-stage brain disease
Hence, specific brain alterations coincide with the accepted symptoms of addiction, namely abuse, withdrawal and relapse.
Discrepancies lie where molecular and neurochemical changes interrelate with the environmental factors of the individual ( O'Brien, 1997 ). In response to this, behavioural manifestations (for example conditioning) can be differentially expressed in individuals despite identical substance-induced changes to neuroplasticity ( Heyman, 1996 ). Some argue that there is heterogeneity in causation between cases, some being of brain-disease origin and others not, whilst others discount the disease theory entirely ( Pickard, 2022 ). It is these complexities that translate to interprofessional divergence when describing or modelling addiction.
2.2. Addiction: a behavioural disorder
Possibly the most prominent contention to brain disease models stems from research psychologist Gene Heyman, who argues that addiction is not a disease but a voluntary choice ( Heyman, 2009 ). Heyman’s viewpoint stemmed from psychiatric epidemiological studies and described replacement of predetermined social rules with self-centred rationalisations as the paramount explanation for addiction ( Hunt, 2010 ).
The argument did not deny the presence of proven neurobiological alterations caused by substances of abuse but denied the capability of these changes to disturb routine decision making. This was in response to findings that many individuals recover from medically defined addiction without treatment, that many recreational drug users do not become compulsive drug users and the lack of elucidated causal link between neural adaptions and addiction ( Heyman, 2013 ).
Other theories assert that the origin of substance use disorders is developmental learned behaviour, which nullifies the brain disease theory with claims that addictive neuroplasticity alterations mirror those seen in the development of deep habits generally ( Lewis, 2017 ). However, common learning-behaviour theories of habitual drug-seeking describe loss of executive control over habits after neuroplasticity changes, which is harmonious with disease-rooted theories ( Ostlund and Balleine, 2008 ).
Incentive sensitization describes the psychological form of ‘wanting’ triggered by stimulus which has been paired with neural modifications that increase incentive salience ( Bechara et al., 2019 ). This theory differentiates ‘wanting’ a reward from ‘liking’ the reward and highlights the insubstantial contribution of neural adaptations to the latter ( Berridge and Robinson, 2016 ).
Conditioning hypotheses, which are also rooted in drug-related environment stimuli to cause drug use, have a proven neurobiological basis linking dopaminergic transmission to drug-related cues and stress ( WEISS et al., 2001 ). Furthermore, the different stages of condition placed preference (CPP), a leading behavioural model, have been intrinsically linked to specific neural alterations ( Fattahi et al., 2023 ).
Models of positive and negative reinforcement (respectively drug reward and adverse withdrawal) as the origin of addiction are also supported through cognitive dysfunction mechanisms present in the three-stage addiction model ( Hogarth, 2020 ).
Within the growing psychological movement that defines instances of gambling, over-eating, sexual intercourse and gaming as behavioural addictions, neurobiological modifications have coincided with the disorders ( Chamberlain et al., 2016 ).
Earlier tendencies of theorists to combat disease-rooted arguments with behavioural origins entirely reveal the monumental challenge the field faces in amalgamating hypotheses and moving toward unanimous conclusions on aetiology. However, recent developments homogenise these conflicting theories to some extent through magnification of specific neuroadaptations paralleling specific behavioural tendencies. Both behavioural and neurobiological concepts are undeniable. What must be determined is the extent to which the theories are prevalent in the aetiology of addiction at different stages. The unravelling of neurobiological contributions to incentive salience are an appreciable example of this: neuroplasticity changes are relevant to ‘wanting’ but are of limited value in ‘liking’ behaviourally.
Another particularly compelling argument describes the need for promotion of ‘empirically based pluralism’ when considering alcohol dependence aetiology ( Kendler, 2012 ). Here, it is argued that alcohol dependence is influenced by ‘difference makers’ including but not limited to molecular and systems neuroscience; social, political, or cultural influence, and genetics. Addiction, generally, can be viewed from this comprehensive vantage point, where appreciation of the contribution of multiple factors and theories are valued.
The more interconnections made between specific brain regions and specific addictive behaviours, the more conceivable it is to relate pathology to the anomalies suggested or determine a specific origin for addictive tendencies, the easier it becomes to align theories. Lack of clarity promotes inconsistencies in investigating addiction disorder, which is further augmented through theories that addiction is a genetic disease.
2.3. Addiction: a genetic disorder
Chronic drug usage is associated with upregulation of the cyclic adenosine 3′,5′-monophosphate (cAMP) pathway and thus dysregulation of cAMP response element–binding protein (CREB) and fos- like protein isoform ΔFosB ( Nestler and Aghajanian, 1997 ). Dysregulation of these transcription factors causes alterations to gene expression ( Nestler, 2004 ). Therefore, much research centres around the specific target genes of these transcription factors and others ( Nestler, 2000 ).
Familial studies (twin/family/adoption) and association and linkage studies proved that many genes result in variance of addictive traits and hereditary vulnerability ( Crabbe, 2002 ). The recent application of genome-wide association studies (GWAS) greatly advanced our understanding of addiction genetics ( Hancock et al., 2018 ). Recently published genome scanning data has supplemented these classical genetic studies and provided researchers with reproducible chromosomal loci containing variants that could alter human addiction vulnerability ( Uhl et al., 2002 ).
Less divergence exists between theories of addiction genetics and others. For example, application of GWAS data has been pursued to investigate the underlying neurobiology of variants and their correlation to cellular processes molecular function ( Gelernter and Polimanti, 2021 ). Integration of genomics and neurobiology has contributed to a new ‘Genetically Informed Neurobiology of Addiction (GINA)’ model. The model not only entwines genetics and neuroscience but gives appreciation to environmental contributions of addiction origin. Hence, the GINA model is arguably the first of its kind in that it manifests all competing theories in a unified way. Further investigation of epigenetics could integrate genetic and behavioural theories further through unravelling environmentally induced alterations in gene expression ( Archer et al., 2012 ).
2.4. Addiction theory
A fully comprehensive literature review of all theories cannot be completed within the scope of this mini-review, but the discrepancies and concordance between multiple leading models are presented (see Figure 1 ).

A summary of leading theories on addiction aetiology; the three-stage brain disease model, concepts in genetic modelling and behavioural theories of addiction, and their interrelationships.
Contrasting opinions on the causative factor of addiction between neurobiology, behaviour and genetics is commonplace in the literature across fields. Research into substance use disorder should be in lieu of the neurobiological changes associated with addiction; the interaction of these changes with the subject’s environment; the effect of these changes on behaviour; the resulting transcriptional changes, and the influence of genetics on neuroadaptation and vulnerability.
Models of addiction allowing investigation into these theories and their constituency are vital. Here, we discuss the currently available methods of research in these areas and the strengths and limitations of practical, experimental addiction modelling.
3. Models of addiction
3.1. in vivo addiction models.
In lieu of tendencies to define addiction in degrees of abstinence, animal self-administration experiments inclusive of oral and intravenous drug-taking and intracranial self-stimulation (ICSS) have been predominant in vivo models ( Gardner, 2000 ). These studies have provided valuable information on addiction sensitivities, abuse potential and neuroadaptation mechanisms (particularly within the binge and intoxication stage of the brain-disease model) ( Negus and Miller, 2014 ). Recent trends in ICSS have highlighted the strengths of this technique in developing our comprehension of drug-reward deficits, abuse-limiting effects, addictive relativity between substances of abuse and specific neurotransmission ( Kenny et al., 2018 ).
CPP and condition placed aversion (CPA) studies apply habituation, freedom of movement and conditioning to positive or negative stimuli to assess the motivation of reward or the negative effects of withdrawal ( Cunningham et al., 2006 ). The advantage of these methods is the ability to investigate drug reinforcement tendencies and draw parallels between drug use and environmental cues ( McKendrick and Graziane, 2020 ).
Within animal reinstatement experiments, the extent to which non-contingent exposure to drugs or related stimuli result in renewal of a previously drug-reinforced behaviour after extinction is measured ( Shaham et al., 2003 ). These experiments aim to disentangle the complexities of craving and relapse.
Animal models have informed our psychological and neurobiological understanding of addiction and thus driven treatment development. In the case of alcohol use disorder (AUD), for example, results from animal experiments were translated clinically to specifically licenced medications including acamprosate and naltrexone ( Spanagel, 2017 ).
Aside from this, animal studies possess a tendency to ineffectively translate to clinical situations. Some argue this lack of translatability is a consequence of ill-considered animal study designs, one example being the lack of alternative options to drug taking in classic self-administration studies ( Ahmed, 2010 ). The decision to administer a substance in this case would not illustrate the decision-making process of an addicted individual, and as such is unrepresentative. Whilst animal researchers have ameliorated this to an extent through the introduction of modern methods like alternative non-drug rewards, forced versus voluntary abstinence and punishment upon reinstatement, the issue of lack of coherence between animal and human behaviour is still prevalent ( Kramer et al., 2020 ). Some addictive criteria, such as escalation of drug use, can be efficaciously modelled in non-human animal models, whilst other addictive complexities fail in human recapitulation ( Ahmed, 2011 ).
Reasonable debate stems from the belief that animal models fundamentally fail in replicating the complexities of an addicted human brain ( Everitt et al., 2017 ). Whilst animal models have postdictive validity (as evident through application of postdictive models in buprenorphine, methadone, and nicotine replacement therapy development), they cannot feasibly be used as a model of initial addiction research or as a model on which to base treatment discovery without heavily considering the translational limitations ( Heilig et al., 2016 ).
Drosophila melanogaster use in addiction research is practical, inexpensive, and allows investigations into genetics through utilisation of their extensive homogeneity with the human genome and their ability to engage in drug related behaviours ( Devineni and Heberlein, 2010 ; Awofala, 2011 ; Kaun et al., 2012 ). However, similar difficulties in linking neural activity to behaviour or to what extent Drosophila can replicate complex human addictive characteristics prevail ( Sokolowski, 2001 ).
Animal genetic studies such as knock out (KO) experiments have similar translational limitations, though recent progress in integrating phenotypical analysis and genomic data from addiction studies across species could improve this ( Cates et al., 2019 ).
Human studies logically improve upon limitations of animal and Drosophila studies. Within human self-administration studies, the variability of addictive tendencies in patients suffering from substance use disorders can be established in research settings. Patterns of addiction characteristics such as daily drug use, bidirectionality of social factors, and drug-seeking or drug-taking behaviour depending on experimental conditions can be determined within the research setting ( Venniro et al., 2016 ).
From human brain imaging studies, assumptions on neurobiological mechanisms of addiction can be made ( Volkow et al., 2003 ). Modern imaging techniques including magnetic resonance spectroscopy (MRS), positron emission tomography (PET) and single photon emission computed tomography (SPECT) allow real-time observations of neuroplastic changes in response to drug use, and so are an undoubtedly useful method of addiction modelling ( Fowler et al., 2007 ). The difficulty with these models is the legal, ethical, and practical challenge of conducting human research, especially in the setting of addiction to illegal substances. Whilst ideal in representability, human studies are beyond the scope of many research laboratories and so the lack of an elementary, practicable and translatable model for basic and mechanistic addiction research persists.
Hence, all current in vivo models of addiction have advantages and limitations (see Table 1 ). Traditionally, in many other research themes, in vitro models can be used to allow simplistic and workable investigations (often before progression to in vivo studies). The issue within the addiction research setting, and neuroscience generally, is that in vitro modelling of complex neurological disorders presents with complications itself.
A summary of the advantages and disadvantages of platforms to model and investigate substance use disorder.
3.2. In vitro addiction models
Traditional in vitro models of addiction have relied on human post-mortem or rodent brain tissue, often used to isolate primary neurons for culture, thus the problem of accessibility or difficulties recapitulating the human brain using animal-based models persist ( Le Feber, 2019 ). A recent surge in novel in vitro preparations and techniques has provided a platform that could overcome these limitations.
Much of this research has centred around protocols allowing laboratory growth of in vitro neural cells that originate from embryonic stem cells (ESCs) or induced pluripotent stem cells (IPSCs) ( Altimus et al., 2020 ). This technique provides an in vitro model that is innately human and manipulatable depending on cell type required or the neurobiological mechanisms under investigation ( Bitar and Barry, 2020 ).
Within addiction research there are already multiple examples of IPSC application, for example in vitro investigations into cannabinoid signalling and its effect on neuronal and dopaminergic maturation ( Stanslowsky et al., 2017 ). The method provides a platform of investigating addictive neurobiology in a setting where the results are conclusively representative of human neuroadaptations owing to their human origin.
Issues with accessing post-mortem brain tissue or reliance on human subjects for in vivo experiments are reduced when using stem cells, as an exponential number of experiments can be completed in a relevant neuroscientific environment from one initial human source ( Goncalves and Przyborski, 2018 ). This is enhanced through the capability of stem cells to form three-dimensional brain organoids, as structurally accurate human brain cell types relevant to addiction can be formed in vitro for experimentation without reliance on post-mortem tissue ( Boeshore et al., 2022 ). Recent application of RNA sequencing technology to complete multiplexed morphogen screens of these organoids has provided more clarity on differentiating to specific neural subtypes, allowing replication of human neuronal cells with distinct, diverse identities for experimentation ( Amin et al., 2023 ).
Additional advantages come from comparisons of IPSCs from healthy versus addicted patients. This allows us to investigate neuroadaptations conclusively and permits investigation into genetic susceptibility or specific allele variation for investigation of both genetic and brain disease theories ( Sheng et al., 2016 ). What is particularly promising when considering the application of stem cells to investigate neurobiology and genetics is the potential of combining this with modern sequencing capabilities as GWAS or ‘omics approaches. Having readily available human tissue for genomic investigations could promote advancement of our understanding of the complex biology behind addiction by providing a platform of practical, relevant research. Hence, novel culture techniques and sequencing technologies could provide a paradigm for experimental investigations that consider each competing theory of addiction.
Limitations of IPSC or ESC application arise from issues of reproducibility when generating the models, which is partly attributed to genomic or epigenomic variability influencing differentiation and otherwise attributed to practical discrepancies ( Marchetto et al., 2011 ). The complexity of IPSC and ESC use is therefore an obstacle to broad scale application in addiction research, as is the reliability and reproducibility of the resulting terminally differentiated cell lines.
Refining protocols to reduce the weighting of this variation, for example through completing additional gain and loss of function experiments to exclude genetic variability, are essential, as is confirmation of the relevance of IPSCs in replicating addiction ( Soldner and Jaenisch, 2017 ). Studies are indicating that IPSCs or ESCs are suitable in vitro models to study substance use disorder, giving lower weighting to the latter issue ( Lieberman, 2016 ).
More straight forward protocols of differentiation include human neuroblastoma cell line SH-SY5Y to form dopaminergic neuronal-like cells are already used in in vitro molecular neuroscience ( Dravid et al., 2021 ). These models use readily accessible immortal cell lines and there are examples of use within addiction settings, but their simplicity of use translates to lower representability compared to the ability of stem cells to reproduce specific brain cell types and the same issue of variability in differentiation transpires ( Ma et al., 2015 ).
In vitro addiction modelling could allow efficient and relevant drug discovery or treatment pathways that could result in improvement of the currently substandard clinical options in addiction care, especially when combined with modern sequencing technologies ( Giri and Bader, 2015 ). However, the models are notwithstanding limitations, and the information on neurobiology or genetics gained through in vitro methods must still be considered in lieu of behavioural arguments and environmental factors.
4. Discussion
Addiction is a uniquely challenging and complicated disorder, and so it is not entirely surprising that there are interprofessional discrepancies between definitions and theories on causation. The repercussions of this ambiguity extend throughout the field of addiction and arise even within basic disease-modelling for experimentation.
In vivo experiments conducted on human studies or in vitro experiments using human brain tissue are advantageous to addiction research, allowing insights into human behaviour and the neurobiological changes associated with substance use disorder. However, limitations exist due to lack of availability, practicalities, and the financial burden of human studies.
The use of IPSCs or ESCs to recapitulate the human brain in vitro could improve upon some of the current limitations of addiction modelling and may provide a platform for practical and reproducible addiction research through forming structurally, biologically, and genetically relevant in vitro environments. Combining this method with contemporary sequencing techniques permits thorough exploration of the latter, and through epigenetic approaches could reduce disparities between these models and behavioural theories of addiction.
Appreciation of all theories of addiction aetiology and their coordination with each other is vital to improving our understanding of substance use disorder and translating this to effective treatment pathways. The lack of a practical, representative model for addiction studies is not only an obstacle to advancing this understanding, but likely a direct result of this lack of clarity.
Modern techniques have the potential to overcome the shortcomings of previously utilised methods by providing researchers with innately human, practical, and manipulatable addiction models. This could be instrumental to effective drug discovery for substance use disorder and has the potential to revolutionise the field. However, acceptance of concurrent theories in addiction aetiology must still precede a universally accepted experimental model.
Author contributions
MG: original draught preparation. SS: review and editing. All authors have read and agreed to the published version of the manuscript.
This work was funded in whole, or in part, by the Wellcome Trust [RS3684]. For the purpose of open access, the author has applied a CC BY public copyright licence to any Author Accepted Manuscript version arising from this submission.
Conflict of interest
The authors declare that the research was conducted in the absence of any commercial or financial relationships that could be construed as a potential conflict of interest.
Publisher’s note
All claims expressed in this article are solely those of the authors and do not necessarily represent those of their affiliated organizations, or those of the publisher, the editors and the reviewers. Any product that may be evaluated in this article, or claim that may be made by its manufacturer, is not guaranteed or endorsed by the publisher.
Acknowledgments
The authors would like to acknowledge all colleagues within the Biodiscovery Institute, the Translational and Radiation Biology team and the Wellcome Trust Molecular Pharmacology and Drug Discovery DTP for their constant support.
- Adinoff B. (2004). Neurobiologic processes in drug reward and addiction . Harv. Rev. Psychiatry 12 , 305–320. doi: 10.1080/10673220490910844, PMID: [ PMC free article ] [ PubMed ] [ CrossRef ] [ Google Scholar ]
- Ahmed S. H. (2010). Validation crisis in animal models of drug addiction: beyond nondisordered drug use toward drug addiction . Neurosci. Biobehav. Rev. 35 , 172–184. doi: 10.1016/j.neubiorev.2010.04.005, PMID: [ PubMed ] [ CrossRef ] [ Google Scholar ]
- Ahmed S. H. (2011). “ Escalation of drug use ” in Animal models of drug addiction . ed. Olmstead M. C. (Totowa, NJ: Humana Press; ) [ Google Scholar ]
- Altimus C. M., Marlin B. J., Charalambakis N. E., Colón-Rodriquez A., Glover E. J., Izbicki P., et al.. (2020). The next 50 years of neuroscience . J. Neurosci. 40 , 101–106. doi: 10.1523/jneurosci.0744-19.2019, PMID: [ PMC free article ] [ PubMed ] [ CrossRef ] [ Google Scholar ]
- Amin N. D., Kelley K. W., Hao J., Miura Y., Narazaki G., Li T., et al.. (2023). Generating human neural diversity with a multiplexed morphogen screen in organoids . bio Rxiv . 2023–05. doi: 10.1101/2023.05.31.541819 [ CrossRef ] [ Google Scholar ]
- Archer T., Oscar-Berman M., Blum K., Gold M. (2012). Neurogenetics and epigenetics in impulsive behaviour: impact on reward circuitry . J. Genet. Syndr. Gene. Ther. 3 :1000115. doi: 10.4172/2157-7412.1000115, PMID: [ PMC free article ] [ PubMed ] [ CrossRef ] [ Google Scholar ]
- Awofala A. A. (2011). Genetic approaches to alcohol addiction: gene expression studies and recent candidates from Drosophila . Invertebr. Neurosci. 11 , 1–7. doi: 10.1007/s10158-010-0113-y, PMID: [ PubMed ] [ CrossRef ] [ Google Scholar ]
- Bechara A., Berridge K. C., Bickel W. K., Morón J. A., Williams S. B., Stein J. S. (2019). A neurobehavioral approach to addiction: implications for the opioid epidemic and the psychology of addiction . Psychol. Sci. Public Interest 20 , 96–127. doi: 10.1177/1529100619860513, PMID: [ PMC free article ] [ PubMed ] [ CrossRef ] [ Google Scholar ]
- Berridge K. C., Robinson T. E. (2016). Liking, wanting, and the incentive-sensitization theory of addiction . Am. Psychol. 71 , 670–679. doi: 10.1037/amp0000059, PMID: [ PMC free article ] [ PubMed ] [ CrossRef ] [ Google Scholar ]
- Bitar M., Barry G. (2020). Building a human brain for research . Front. Mol. Neurosci. 13 :22. doi: 10.3389/fnmol.2020.00022, PMID: [ PMC free article ] [ PubMed ] [ CrossRef ] [ Google Scholar ]
- Boeshore K. L., Lee C.-T., Freed W. J. (2022). “ Chapter 6-Applications of human induced pluripotent stem cell and human embryonic stem cell models for substance use disorders: addiction and neurodevelopmental toxicity ” in Novel concepts in iPSC disease modeling . ed. Birbrair A. (Academic Press; ), 153–177. doi: 10.1016/B978-0-12-823882-0.00006-0 [ CrossRef ] [ Google Scholar ]
- Cates H. M., Benca-Bachman C. E., de Guglielmo G., Schoenrock S. A., Shu C., Kallupi M. (2019). National Institute on Drug Abuse genomics consortium white paper: coordinating efforts between human and animal addiction studies . Genes Brain Behav. 18 :e12577. doi: 10.1111/gbb.12577, PMID: [ PMC free article ] [ PubMed ] [ CrossRef ] [ Google Scholar ]
- Chamberlain S. R., Lochner C., Stein D. J., Goudriaan A. E., van Holst R. J., Zohar J., et al.. (2016). Behavioural addiction–a rising tide? Eur. Neuropsychopharmacol. 26 , 841–855. doi: 10.1016/j.euroneuro.2015.08.013 [ PubMed ] [ CrossRef ] [ Google Scholar ]
- Crabbe J. C. (2002). Genetic contributions to addiction . Annu. Rev. Psychol. 53 , 435–462. doi: 10.1146/annurev.psych.53.100901.135142 [ PubMed ] [ CrossRef ] [ Google Scholar ]
- Crocq M.-A. (2007). Historical and cultural aspects of man's relationship with addictive drugs . Dialogues Clin. Neurosci. 9 , 355–361. doi: 10.31887/DCNS.2007.9.4/macrocq, PMID: [ PMC free article ] [ PubMed ] [ CrossRef ] [ Google Scholar ]
- Cunningham C. L., Gremel C. M., Groblewski P. A. (2006). Drug-induced conditioned place preference and aversion in mice . Nat. Protoc. 1 , 1662–1670. doi: 10.1038/nprot.2006.279, PMID: [ PubMed ] [ CrossRef ] [ Google Scholar ]
- Devineni A. V., Heberlein U. (2010). Addiction-like behavior in Drosophila . Commun. Integr. Biol. 3 , 357–359. doi: 10.4161/cib.3.4.11885, PMID: [ PMC free article ] [ PubMed ] [ CrossRef ] [ Google Scholar ]
- Dravid A., Raos B., Svirskis D., O’Carroll S. J. (2021). Optimised techniques for highthroughput screening of differentiated SH-SY5Y cells and application for neurite outgrowth assays . Sci. Rep. 11 :23935. doi: 10.1038/s41598-021-03442-1, PMID: [ PMC free article ] [ PubMed ] [ CrossRef ] [ Google Scholar ]
- D'Souza M. S. (2015). Glutamatergic transmission in drug reward: implications for drug addiction . Front. Neurosci. 9 :404. doi: 10.3389/fnins.2015.00404, PMID: [ PMC free article ] [ PubMed ] [ CrossRef ] [ Google Scholar ]
- Everitt B. J., Giuliano C., Belin D. (2017). Addictive behaviour in experimental animals: prospects for translation . Philos. Trans. R. Soc. Lond. Ser. B Biol. Sci. 373 :20170027. doi: 10.1098/rstb.2017.0027, PMID: [ PMC free article ] [ PubMed ] [ CrossRef ] [ Google Scholar ]
- Fattahi M., Modaberi S., Eskandari K., Haghparast A. (2023). A systematic review of the local field potential adaptations during conditioned place preference task in preclinical studies . Synapse 77 :e22277. doi: 10.1002/syn.22277, PMID: [ PubMed ] [ CrossRef ] [ Google Scholar ]
- Feltenstein M. W. (2008). The neurocircuitry of addiction: an overview . Br. J. Pharmacol. 154 , 261–274. doi: 10.1038/bjp.2008.51, PMID: [ PMC free article ] [ PubMed ] [ CrossRef ] [ Google Scholar ]
- Fowler J. S., Volkow N. D., Kassed C. A., Chang L. (2007). Imaging the addicted human brain . Sci. Pract. Perspect. 3 , 4–16. doi: 10.1151/spp07324, PMID: [ PMC free article ] [ PubMed ] [ CrossRef ] [ Google Scholar ]
- Gardner E. L. (2000). What we have learned about addiction from animal models of drug self-administration . Am. J. Addict. 9 , 285–313. doi: 10.1080/10550490075004735 [ PubMed ] [ CrossRef ] [ Google Scholar ]
- Gelernter J., Polimanti R. (2021). Genetics of substance use disorders in the era of big data . Nat. Rev. Genet. 22 , 712–729. doi: 10.1038/s41576-021-00377-1, PMID: [ PMC free article ] [ PubMed ] [ CrossRef ] [ Google Scholar ]
- Giri S., Bader A. (2015). A low-cost, high-quality new drug discovery process using patient-derived induced pluripotent stem cells . Drug Discov. Today 20 , 37–49. doi: 10.1016/j.drudis.2014.10.011 [ PubMed ] [ CrossRef ] [ Google Scholar ]
- Goldstein R. Z., Volkow N. D. (2011). Dysfunction of the prefrontal cortex in addiction: neuroimaging findings and clinical implications . Nat. Rev. Neurosci. 12 , 652–669. doi: 10.1038/nrn3119, PMID: [ PMC free article ] [ PubMed ] [ CrossRef ] [ Google Scholar ]
- Goncalves K., Przyborski S. (2018). The utility of stem cells for neural regeneration . Brain Neurosci. Adv. 2 :2398212818818071. doi: 10.1177/2398212818818071 [ PMC free article ] [ PubMed ] [ CrossRef ] [ Google Scholar ]
- Gutman S. A. (2006). Why addiction has a chronic, relapsing course. The neurobiology of addiction . Occup. Ther. Ment. Health 22 , 1–29. doi: 10.1300/J004v22n02_01 [ CrossRef ] [ Google Scholar ]
- Hammer R., Dingel M., Ostergren J., Partridge B., McCormick J., Koenig B. A. (2013). Addiction: current criticism of the brain disease paradigm . AJOB Neurosci. 4 , 27–32. doi: 10.1080/21507740.2013.796328, PMID: [ PMC free article ] [ PubMed ] [ CrossRef ] [ Google Scholar ]
- Hancock D. B., Markunas C. A., Bierut L. J., Johnson E. O. (2018). Human genetics of addiction: new insights and future directions . Curr. Psychiatry Rep. 20 :8. doi: 10.1007/s11920-018-0873-3, PMID: [ PMC free article ] [ PubMed ] [ CrossRef ] [ Google Scholar ]
- Heilig M., Epstein D. H., Nader M. A., Shaham Y. (2016). Time to connect: bringing social context into addiction neuroscience . Nat. Rev. Neurosci. 17 , 592–599. doi: 10.1038/nrn.2016.67, PMID: [ PMC free article ] [ PubMed ] [ CrossRef ] [ Google Scholar ]
- Heilig M., Mac Killop J., Martinez D., et al.. (2021). Addiction as a brain disease revised: why it still matters, and the need for consilience . Neuropsychopharmacology 46 , 1715–1723. doi: 10.1038/s41386-020-00950-y, PMID: [ PMC free article ] [ PubMed ] [ CrossRef ] [ Google Scholar ]
- Heyman G. M. (1996). Resolving the contradictions of addiction . Behav. Brain Sci. 19 , 561–574. doi: 10.1017/S0140525X00042990 [ CrossRef ] [ Google Scholar ]
- Heyman GM. (2009). Addiction: a disorder of choice . Cambridge: Harvard University Press. [ Google Scholar ]
- Heyman G. (2013). Addiction and choice: theory and new data . Front. Psych. 4 :31. doi: 10.3389/fpsyt.2013.00031 [ PMC free article ] [ PubMed ] [ CrossRef ] [ Google Scholar ]
- Hogarth L. (2020). Addiction is driven by excessive goal-directed drug choice under negative affect: translational critique of habit and compulsion theory . Neuropsychopharmacology 45 , 720–735. doi: 10.1038/s41386-020-0600-8, PMID: [ PMC free article ] [ PubMed ] [ CrossRef ] [ Google Scholar ]
- Hunt D. W. (2010). A review of addiction: a disorder of choice . J. Addict. Dis. 29 , 412–413. doi: 10.1080/10550887.2010.490471 [ CrossRef ] [ Google Scholar ]
- Kaun K. R., Devineni A. V., Heberlein U. (2012). Drosophila melanogaster as a model to study drug addiction . Hum. Genet. 131 , 959–975. doi: 10.1007/s00439-012-1146-6, PMID: [ PMC free article ] [ PubMed ] [ CrossRef ] [ Google Scholar ]
- Kendler K. S. (2012). The dappled nature of causes of psychiatric illness: replacing the organic-functional/hardware-software dichotomy with empirically based pluralism . Mol. Psychiatry 17 , 377–388. doi: 10.1038/mp.2011.182 [ PMC free article ] [ PubMed ] [ CrossRef ] [ Google Scholar ]
- Kenny P. J., Hoyer D., Koob G. F. (2018). Animal models of addiction and neuropsychiatric disorders and their role in drug discovery: honoring the legacy of Athina Markou . Biol. Psychiatry. 83 , 940–946. doi: 10.1016/j.biopsych.2018.02.009, PMID: [ PubMed ] [ CrossRef ] [ Google Scholar ]
- Koob G. F. (2009). Brain stress systems in the amygdala and addiction . Brain Res. 1293 , 61–75. doi: 10.1016/j.brainres.2009.03.038, PMID: [ PMC free article ] [ PubMed ] [ CrossRef ] [ Google Scholar ]
- Koob G. F., Le Moal M. (2008). Addiction and the brain antireward system . Annu. Rev. Psychol. 59 , 29–53. doi: 10.1146/annurev.psych.59.103006.093548 [ PubMed ] [ CrossRef ] [ Google Scholar ]
- Koob G. F., Simon E. J. (2009). The neurobiology of addiction: where we have been and where we are going . J. Drug Issues 39 , 115–132. doi: 10.1177/002204260903900110, PMID: [ PMC free article ] [ PubMed ] [ CrossRef ] [ Google Scholar ]
- Koob G. F., Volkow N. D. (2010). Neurocircuitry of addiction . Neuropsychopharmacology 35 , 217–238. doi: 10.1038/npp.2009.110, PMID: [ PMC free article ] [ PubMed ] [ CrossRef ] [ Google Scholar ]
- Kramer J., Dick D. M., King A., Ray L. A., Sher K. J., Vena A., et al.. (2020). Mechanisms of alcohol addiction: bridging human and animal studies . Alcohol Alcohol. 55 , 603–607. doi: 10.1093/alcalc/agaa068, PMID: [ PMC free article ] [ PubMed ] [ CrossRef ] [ Google Scholar ]
- Kwako L. E., Koob G. F. (2017). Neuroclinical framework for the role of stress in addiction . Chronic Stress. 1 :247054701769814. doi: 10.1177/2470547017698140, PMID: [ PMC free article ] [ PubMed ] [ CrossRef ] [ Google Scholar ]
- Le Feber J. (2019). In vitro models of brain disorders . Adv Neurobiol. 22 , 19–49. doi: 10.1007/978-3-030-11135-9_2 [ PubMed ] [ CrossRef ] [ Google Scholar ]
- Leshner A. I. (1997). Addiction is a brain disease, and it matters . Science 278 , 45–47. doi: 10.1126/science.278.5335.45 [ PubMed ] [ CrossRef ] [ Google Scholar ]
- Lewis M. (2017). Addiction and the brain: development, not disease . Neuroethics 10 , 7–18. doi: 10.1007/s12152-016-9293-4, PMID: [ PMC free article ] [ PubMed ] [ CrossRef ] [ Google Scholar ]
- Lieberman R. (2016). Utilizing human induced pluripotent stem cells in the study of alcohol use disorder Doctoral Dissertations, 1035.
- Ma J., Yuan X., Qu H., Zhang J., Wang D., Sun X., et al.. (2015). The role of reactive oxygen species in morphine addiction of SH-SY5Y cells . Life Sci. 124 , 128–135. doi: 10.1016/j.lfs.2015.01.003, PMID: [ PubMed ] [ CrossRef ] [ Google Scholar ]
- Marchetto M. C., Brennand K. J., Boyer L. F., Gage F. H. (2011). Induced pluripotent stem cells (iPSCs) and neurological disease modeling: progress and promises . Hum. Mol. Genet. 20 , R109–R115. doi: 10.1093/hmg/ddr336 [ PMC free article ] [ PubMed ] [ CrossRef ] [ Google Scholar ]
- McKendrick G., Graziane N. M. (2020). Drug-induced conditioned place preference and its practical use in substance use disorder research . Front. Behav. Neurosci. 14 :582147. doi: 10.3389/fnbeh.2020.582147, PMID: [ PMC free article ] [ PubMed ] [ CrossRef ] [ Google Scholar ]
- Nathan P. E., Conrad M., Skinstad A. H. (2016). History of the concept of addiction . Annu. Rev. Clin. Psychol. 12 , 29–51. doi: 10.1146/annurev-clinpsy-021815-093546 [ PubMed ] [ CrossRef ] [ Google Scholar ]
- Negus S. S., Miller L. L. (2014). Intracranial self-stimulation to evaluate abuse potential of drugs . Pharmacol. Rev. 66 , 869–917. doi: 10.1124/pr.112.007419, PMID: [ PMC free article ] [ PubMed ] [ CrossRef ] [ Google Scholar ]
- Nestler E. J. (2000). Genes and addiction . Nat. Genet. 26 , 277–281. doi: 10.1038/8157054 [ PubMed ] [ CrossRef ] [ Google Scholar ]
- Nestler E. J. (2004). Molecular mechanisms of drug addiction . Neuropharmacology 47 , 24–32. doi: 10.1016/j.neuropharm.2004.06.031 [ PubMed ] [ CrossRef ] [ Google Scholar ]
- Nestler E. J. (2005). Is there a common molecular pathway for addiction? Nat. Neurosci. 8 , 1445–1449. doi: 10.1038/nn1578 [ PubMed ] [ CrossRef ] [ Google Scholar ]
- Nestler E. J., Aghajanian G. K. (1997). Molecular and cellular basis of addiction . Science 278 , 58–63. doi: 10.1126/science.278.5335.58 [ PubMed ] [ CrossRef ] [ Google Scholar ]
- O'Brien C. P. (1997). A range of research-based pharmacotherapies for addiction . Science 278 , 66–70. doi: 10.1126/science.278.5335.66 [ PubMed ] [ CrossRef ] [ Google Scholar ]
- Ostlund S. B., Balleine B. W. (2008). On habits and addiction: an associative analysis of compulsive drug seeking . Drug Discov. Today Dis. Models. 5 , 235–245. doi: 10.1016/j.ddmod.2009.07.004, PMID: [ PMC free article ] [ PubMed ] [ CrossRef ] [ Google Scholar ]
- Pickard H. (2022). Is addiction a brain disease? A plea for agnosticism and heterogeneity . Psychopharmacology 239 , 993–1007. doi: 10.1007/s00213-021-06013-4, PMID: [ PubMed ] [ CrossRef ] [ Google Scholar ]
- Shaham Y., Shalev U., Lu L., de Wit H., Stewart J. (2003). The reinstatement model of drug relapse: history, methodology and major findings . Psychopharmacology 168 , 3–20. doi: 10.1007/s00213-0021224-x, PMID: [ PubMed ] [ CrossRef ] [ Google Scholar ]
- Sheng Y., Filichia E., Shick E., Preston K. L., Phillips K. A., Cooperman L., et al.. (2016). Using iPSC-derived human DA neurons from opioiddependent subjects to study dopamine dynamics . Brain Behav. 6 :e00491. doi: 10.1002/brb3.491 [ PMC free article ] [ PubMed ] [ CrossRef ] [ Google Scholar ]
- Sokolowski M. B. (2001). Drosophila: genetics meets behaviour . Nat. Rev. Genet. 2 , 879–890. doi: 10.1038/35098592 [ PubMed ] [ CrossRef ] [ Google Scholar ]
- Soldner F., Jaenisch R. (2017). “ In vitro modeling of complex neurological diseases ” in Genome editing in neurosciences . eds. Jaenisch R., Zhang F., Gage F. (Cham: Springer International Publishing; ), 1–19. doi: 10.1007/978-3-319-60192-2_1 [ PubMed ] [ CrossRef ] [ Google Scholar ]
- Spanagel R. (2017). Animal models of addiction . Dialogues Clin. Neurosci. 19 , 247–258. doi: 10.31887/DCNS.2017.19.3/rspanagel, PMID: [ PMC free article ] [ PubMed ] [ CrossRef ] [ Google Scholar ]
- Stanslowsky N., Jahn K., Venneri A., Naujock M., Haase A., Martin U., et al.. (2017). Functional effects of cannabinoids during dopaminergic specification of human neural precursors derived from induced pluripotent stem cells . Addict. Biol. 22 , 1329–1342. doi: 10.1111/adb.12394, PMID: [ PubMed ] [ CrossRef ] [ Google Scholar ]
- Substance Abuse and Mental Health Services Administration (US) and Office of the Surgeon General (US) . (2016). Facing addiction in America: the surgeon general’s report on alcohol, drugs, and health . US Department of Health and Human Services: Washington, DC. [ PubMed ] [ Google Scholar ]
- Uhl G. R., Liu Q.-R., Naiman D. (2002). Substance abuse vulnerability loci: converging genome scanning data . Trends Genet. 18 , 420–425. doi: 10.1016/S01689525(02)02719-1, PMID: [ PubMed ] [ CrossRef ] [ Google Scholar ]
- Veerappa A., Pendyala G., Guda C. (2021). A systems omics-based approach to decode substance use disorders and neuroadaptations . Neurosci. Biobehav. Rev. 130 , 61–80. doi: 10.1016/j.neubiorev.2021.08.016, PMID: [ PMC free article ] [ PubMed ] [ CrossRef ] [ Google Scholar ]
- Venniro M., Caprioli D., Shaham Y. (2016). Animal models of drug relapse and craving: from drug priming-induced reinstatement to incubation of craving after voluntary abstinence . Prog. Brain. Res. , 25–52. doi: 10.1016/bs.pbr.2015.08.004 [ PubMed ] [ CrossRef ] [ Google Scholar ]
- Volkow N. D., Fowler J. S., Wang G. J. (2003). The addicted human brain: insights from imaging studies . J. Clin. Invest. 111 , 1444–1451. doi: 10.1172/jci18533, PMID: [ PMC free article ] [ PubMed ] [ CrossRef ] [ Google Scholar ]
- Volkow N. D., Michaelides M., Baler R. (2019). The neuroscience of drug reward and addiction . Physiol. Rev. 99 , 2115–2140. doi: 10.1152/physrev.00014.2018, PMID: [ PMC free article ] [ PubMed ] [ CrossRef ] [ Google Scholar ]
- Weiss F., Ciccocioppo R., Parsons L. H., Katner S., Liu X., Zorrilla E. P., et al.. (2001). Compulsive drug-seeking behavior and relapse . Ann. N. Y. Acad. Sci. 937 , 1–26. doi: 10.1111/j.1749-6632.2001.tb03556.x [ PubMed ] [ CrossRef ] [ Google Scholar ]
- Open access
- Published: 09 April 2018
Barriers and facilitators affecting the implementation of substance use screening in primary care clinics: a qualitative study of patients, providers, and staff
- Jennifer McNeely 1 , 2 ,
- Pritika C. Kumar 1 ,
- Traci Rieckmann 3 ,
- Erica Sedlander 1 ,
- Sarah Farkas 4 ,
- Christine Chollak 1 ,
- Joseph L. Kannry 5 ,
- Aida Vega 5 ,
- Eva A. Waite 5 ,
- Lauren A. Peccoralo 5 ,
- Richard N. Rosenthal 6 ,
- Dennis McCarty 7 &
- John Rotrosen 4
Addiction Science & Clinical Practice volume 13 , Article number: 8 ( 2018 ) Cite this article
21k Accesses
149 Citations
11 Altmetric
Metrics details
Alcohol and drug use are leading causes of morbidity and mortality that frequently go unidentified in medical settings. As part of a multi-phase study to implement electronic health record-integrated substance use screening in primary care clinics, we interviewed key clinical stakeholders to identify current substance use screening practices, barriers to screening, and recommendations for its implementation.
Focus groups and individual interviews were conducted with 67 stakeholders, including patients, primary care providers (faculty and resident physicians), nurses, and medical assistants, in two urban academic health systems. Themes were identified using an inductive approach, revised through an iterative process, and mapped to the Knowledge to Action (KTA) framework, which guides the implementation of new clinical practices (Graham et al. in J Contin Educ Health Prof 26(1):13–24, 2006 ).
Factors affecting implementation based on KTA elements were identified from participant narratives. Identifying the problem: Participants consistently agreed that having knowledge of a patient’s substance use is important because of its impacts on health and medical care, that substance use is not properly identified in medical settings currently, and that universal screening is the best approach. Assessing barriers: Patients expressed concerns about consequences of disclosing substance use, confidentiality, and the individual’s own reluctance to acknowledge a substance use problem. Barriers identified by providers included individual-level factors such as lack of clinical knowledge and training, as well as systems-level factors including time pressure, resources, lack of space, and difficulty accessing addiction treatment. Adapting to the local context: Most patients and providers stated that the primary care provider should play a key role in substance use screening and interventions. Opinions diverged regarding the optimal approach to delivering screening, although most preferred a patient self-administered approach. Many providers reported that taking effective action once unhealthy substance use is identified is crucial.
Conclusions
Participants expressed support for substance use screening as a valuable part of medical care, and identified individual-level as well as systems-level barriers to its implementation. These findings suggest that screening programs should clearly communicate the goals of screening to patients and proactively counteract stigma, address staff concerns regarding time and workflow, and provide education as well as treatment resources to primary care providers.
Alcohol and drug use are among the top ten causes of preventable death in the United States [ 1 , 2 , 3 , 4 ], but substance use disorders (SUDs) are greatly under-treated in the specialty addiction treatment system [ 5 ], and under-recognized in medical settings [ 6 , 7 ]. Screening for alcohol use in adult primary care settings is recommended by the United States Preventive Services Task Force (USPSTF) and ranks as the third highest prevention priority for adults in the U.S [ 8 , 9 , 10 , 11 , 12 , 13 ]. The U.S. Surgeon General’s report on addiction recommends screening for other drug use as well as alcohol, which is the current approach of federally-funded ‘screening, brief intervention, and referral to treatment (SBIRT)’ programs [ 14 , 15 ]. Yet despite over a decade of concerted efforts to integrate substance use screening and interventions into mainstream medical care [ 16 ], primary care patients are rarely screened, assessed or treated for SUDs [ 6 , 7 , 17 , 18 , 19 , 20 , 21 , 22 ].
There is a rich literature on barriers to implementing screening for substance use in primary care [ 17 , 18 , 19 , 20 ]. In today’s busy primary care practices, the time required for screening, challenges of integrating it into the clinical workflow, and poor quality of screening are the primary barriers. Most of the research documenting barriers to screening has been conducted with primary care physicians, but current primary care practice emphasizes a team-based approach and patient-centered care, and successful screening approaches typically involve other members of the care team performing the screening [ 23 , 24 ]. The perspectives of non-physician clinical staff and patients are thus critical to developing effective implementation strategies, but have been underrepresented in the screening literature.
We sought to gain an understanding of substance use screening from a diversity of clinical stakeholders, as part of a multi-site study of the National Institute on Drug Abuse (NIDA) Clinical Trials Network (CTN). The overarching goal of this CTN study is to implement substance use screening using the NIDA CTN Common Data Elements (CDEs) in primary care clinics. The CDEs are validated substance use screening tools that have been selected for integration into electronic health records (EHRs) based on their accuracy and feasibility in medical settings [ 25 , 26 ].
The conceptual model for the study is the Knowledge to Action (KTA) framework, which informs the selection and implementation of new clinical practices. Implementation models and frameworks can both guide the introduction of new practices, and inform our understanding of why the introduction of a given practice or intervention may succeed or fail [ 27 ]. While there is now a wealth of experience with implementing SBIRT through demonstration projects, it remains challenging to explain why certain approaches may have been successful in some settings but not in others, or to arrive at a set of key elements that are generally needed for the effective introduction of screening into health care settings [ 24 ]. The KTA framework was developed by implementation researchers based on a synthesis of 31 theories for planned action [ 28 , 29 ]. It is considered a process model, meaning that it can be used to describe or guide the translation of research into practice [ 27 ]. Our study activities focus on the KTA ‘action cycle,’ and consist of adapting, implementing, and evaluating the use of substance use screening tools. This paper presents results of the initial phase of the larger CTN study, and used qualitative methods to identify current substance use screening practices, barriers to screening, and recommendations for its implementation in primary care clinics.
Focus groups and individual interviews with clinical stakeholders were conducted to inform the development of EHR-integrated substance use screening tools, and strategies for their implementation in primary care clinics participating in the CTN study. A qualitative approach was used because we sought to gain an understanding of the attitudes of medical providers, clinical staff, and patients toward screening, and to elicit recommendations for introducing screening into these clinical sites. Focus groups elicited group norms and attitudes from each category of stakeholders, while individual interviews gathered more detailed information about the logistics of introducing EHR-integrated screening, and gave an in-depth understanding of primary care provider attitudes and perceived barriers. The study was approved by the Institutional Review Boards of NYU School of Medicine, Icahn School of Medicine at Mt. Sinai, and Oregon Health and & Science University.
The majority of data collection was done in one New York City health system that serves as the lead site for this CTN study, and has two participating primary care clinics. To gain additional insight from practitioners with greater SBIRT experience, we also conducted interviews at a second study site located in Portland, Oregon. Oregon introduced screening and brief intervention for alcohol and drug use in 2013 as an incentive measure for Coordinated Care Organizations (CCOs), under the state’s health care transformation [ 30 , 31 ]. Additionally, some of the Oregon participants for this study had interacted with a federally-funded SBIRT training program based at Oregon Health & Science University.
These urban, academic medical centers were chosen as sites for the CTN study because they have large networks of primary care practices, serve as training sites for multidisciplinary healthcare providers, are geographically diverse, and use Epic EHR software (Epic Systems Corporation). Epic is one of the most widely-utilized EHRs in the U.S. [ 32 ], and we chose Epic-using health systems in order to facilitate knowledge transfer between the study sites and enable future broad dissemination of the clinical tools that we plan to develop. Participating clinics within each health system were selected with a goal of providing a diversity of primary care practice settings. The two New York City sites were internal medicine clinics; one teaching practice that serves primarily a safety net patient population (NY-1), and one faculty practice (NY-2). The Portland (OR) sites were a family medicine and an internal medicine practice. At the time of the study, none of the sites had initiated systematic screening for alcohol or drug use.
Participants
Focus groups and individual interviews were conducted between June 2015 and February 2016, with a total of 67 participants. Medical providers were primary care providers (PCPs); medical assistants (MAs); or registered nurses (RNs). Our medical provider sample included both faculty and residents; although non-MD primary care providers (including nurse practitioners and Doctors of Osteopathy) were eligible to participate, our sample was composed entirely of MDs. Patients were individuals currently receiving care in one of the participating adult primary care clinics. A total of 11 focus groups were conducted at the New York clinics with faculty PCPs (two groups), residents (one group), MAs (two groups), RNs (one group), and patients (five groups). Because medical residents provided care at only one of the clinics, only one session was held with residents. At the smaller of the two New York clinics, RNs and MAs were combined into a single group. At the Oregon site, scheduling difficulties made it unfeasible to schedule and recruit participants for focus groups, and so only individual interviews were conducted.
Eight individual interviews were conducted with PCPs in Oregon (n = 6) and New York (n = 2). Individuals were selected for interviews using a critical case sampling approach [ 33 , 34 ]. In New York, the interviews occurred after the focus groups, and were used to provide more in-depth exploration of questions or themes that were raised during the focus group sessions. The two PCPs selected for individual interviews were full-time faculty providers who had participated in a focus group and articulated good understanding of the clinic’s operations. In Oregon, PCPs were individuals who were selected because of their SBIRT or health informatics experience, and were current full-time clinical faculty (n = 4) or primary care clinician informaticists (n = 2) at the study clinics.
To encourage participation, focus groups were held during regular scheduled meeting times and interviews were conducted on-site and during regular clinic hours. For focus groups with medical staff, all eligible individuals were invited by email to attend. For focus groups with patients, clinical staff distributed fliers with information about the group to patients presenting for care, and directed individuals who were interested to speak with a research assistant. All adult patients who were fluent in English were eligible to participate. Patients’ medical providers were not involved in recruitment, and were not informed regarding participation of their patients in the study. All participants received an IRB-approved written information sheet, and the interviewer verbally summarized its content and solicited questions prior to each session. All participants and gave verbal consent to the interview and audio recording. Participants were not asked to review transcripts or provide feedback on our findings.
Methodology
The semi-structured interviews sought to evaluate constructs from the Knowledge to Action (KTA) framework that guide selection and implementation of new clinical practices; in this case, the inclusion of substance use screening in primary care clinics. Interview guides were developed by the CTN study Lead Investigator and followed the themes from the action phase of the KTA framework: identifying the problem; assessing barriers; and adapting to the local context. Interview guides (included as Additional file 1 ) covered views on the purpose, value, and acceptability of screening for substance use, current screening practices, opinions regarding providers’ knowledge about substance use, and recommendations about the frequency and content of screening as well as how to integrate it into the clinical workflow. Interview guides for medical providers, staff, and patients covered the same general topics, but were tailored to the participant group (for example, interviews with patients addressed answering screening questions, while interviews with providers addressed delivering screening, receiving results, and clinical workflow). For medical providers, focus group and individual interview guides were similar, but individual interviews gave emphasis to personal experiences and attitudes, while focus groups emphasized current practices in the clinic and group attitudes regarding screening.
Data collection
Focus groups and interviews were conducted between June and November, 2015. The New York focus groups and interviews were conducted on-site at the clinics by the Lead Investigator (JM), who is a practicing PCP at a different New York City medical center. The interviews in Oregon were conducted by a psychologist (TR) who was the local site lead. Both interviewers have experience conducting qualitative health services research. Interviews were conducted in English. The average length of the focus groups and interviews was 45–60 min and all sessions were audio recorded. For each focus group, at least one member of the research staff observed the group and took field notes, while for individual interviews field notes were written by the interviewer following the session. Participants were given a small monetary amount for participation (staff received $50, patients received $20). Payments were calibrated to the amounts that are typically used for research in these clinical sites, and were higher for staff because they may have needed to take time away from paid clinical work in order to participate. Following each interview or focus group, participants were asked to complete a form collecting demographic information.
Data analysis
Interviews were transcribed verbatim from the audio recording, leaving out any names. A research assistant who attended all of the focus groups verified the transcripts by comparing them to the audio recordings. Transcripts were entered into Atlas ti (7.0) software for data management and analysis. Field notes were reviewed when needed by the interviewers (JM and TR) to facilitate recall, but were not included in the analysis.
Two researchers (PC and ES) reviewed each focus group and interview transcript. The initial review was done independently, and then the codes that each researcher had developed were discussed and redundancies were eliminated to arrive at an initial codebook. Transcripts were analyzed using thematic analysis; an inductive approach designed to identify and examine emerging themes from conceptual data that involves coding qualitative data using a list of themes found in the transcripts [ 35 ]. Initial codes and categories were developed to identify theoretically important concepts of the KTA. Emerging themes were mapped to the domains and constructs of the KTA after multiple readings of the transcripts. Several discussions were conducted among researchers (PCK, ES and JM) involved in the coding and analysis about the appropriateness of each code. Disagreements around codes, themes and subthemes were resolved by discussion among the team members and going back to the original transcripts and field notes. The final coding scheme consisted of 6 primary themes and 46 subthemes for provider transcripts and 4 primary themes and 38 subthemes for patient transcripts. Three researchers (PCK, ES and JM) concluded that thematic saturation was reached when no additional themes related to the KTA domains were emerging from the data.
Using the jointly developed codebook, ES coded all transcripts. Blinded to the coding of ES, PK coded a randomly selected 13 transcripts (20%) to establish inter-rater reliability. The Kappa coefficient for inter-rater reliability was 0.75 which is interpreted as excellent in qualitative literature [ 36 ]. In a final step, the themes and subthemes were aggregated into matrices, organized by the KTA domains, containing the most representative quotes from each interview. Matrix analyses visually display the range of related responses for each theme, to ensure that both majority and outlier responses are considered [ 37 ]. For example, the matrix for the theme ‘identifying the problem’ had columns named for each subtheme, and rows named for each interview type; cells were filled with quotes from the interviews that captured the subtheme.
Demographic characteristics of the 67 participants are included in Table 1 . Among the PCP participants, 29 were faculty and 5 were internal medicine residents. Two PCPs participated in both a focus group and an individual interview. A total of 15 medical assistants and 3 registered nurses participated. Most of the patient participants were over 45 years of age and female, and were primarily recruited from the New York teaching practice (NY-1 clinic).
Our results are described in three broad categories, based on the KTA framework. The first category, ‘identifying the problem,’ contains themes related to the value of screening and current practices. The second, ‘assessing barriers,’ includes themes related to individual-level and systems-level barriers to screening. The third category, ‘adapting to the local context,’ consists of recommendations from participants about implementing screening.
Identifying the problem
All stakeholder groups felt that identifying substance use is important for providing appropriate medical care. At the time of the study, none of the participating clinics had implemented a systematic approach to alcohol or drug screening, though they did have systems in place for tobacco screening. In this setting, participants felt that unhealthy alcohol and drug use among patients may go unidentified.
Left to the discretion of the provider, screening is not regularly done
Providers said that they asked about substance use during some visits, particularly during a patient’s initial visit to the clinic or when pursuing a specific health complaint that may be alcohol- or drug-related. Drawbacks to this approach noted by providers were that the information may not be accurate, because patterns of substance use can change over time, or that patients may not feel comfortable disclosing substance use in an initial meeting with the provider. Participants noted that some patients may never be asked about alcohol or drug use, because screening depends on the provider, the patient’s other medical problems, and the amount of time available.
Screening has value for medical care
Overwhelmingly, patients and providers agreed that substance use screening has value in primary care. Providers stated that knowing about a patient’s substance use is critical to understanding their overall state of health, and that it impacts their care for other conditions.
Some PCPs talked about substance use being similar to other social determinants of health that impact disease risk and response to treatment, ‘like whether they have a job and a place to live’ (NY-1 Faculty MD focus group). Many providers discussed specific ways in which knowledge of substance use could affect their diagnoses, prescribing decisions, and care plans. As summarized by one PCP:
I do think it is probably both important and necessary, because I guess for two reasons. One, we are a point of intervention for something that might not otherwise be identified or discussed in somebody’s life. But I also think it can be destructive. And I think people often don’t know where to talk about it. So I think it’s important to ask to give people that opportunity, but also to better understand who you’re working with and whether that’s part of why it’s difficult to care for other identified disease processes. NY-1 Faculty MD interview
Similar views were held by MAs and patients, who agreed that substance use affects overall health, and that medical providers need to know about substance use in order to provide good care. As stated by one MA: “Well, that would give the doctor information on how to take care of the patient, direct them to where they have to go if they need help, and help them with their care” (NY-2 MA focus group). Patients discussed that it was important for their medical providers knowing about substance use, to allow them to make accurate diagnoses and provide appropriate treatment. As one patient said, “And, yes, I want them to know everything about me so I can get the best possible diagnosis I can possibly get” (NY-1 Patient focus group).
In addition to providing overall knowledge about the patient’s health, providers and patients also talked about specific ways in which screening for substance use adds value to the clinical encounter. Screening was felt to be a way of signaling to patients that talking about substance use is permissible, thus opening the door to a conversation that would not have otherwise have happened. Participants also noted that identifying substance use is a necessary first step toward helping patients with substance problems. Providers stated that primary care visits present an opportunity to identify and intervene upon unhealthy use. One expression of these views is from a PCP focus group:
Some patients actually want to be helped. And they may not ask for help unless you bring it up. So sometimes that’s why they’re actually here. But they won’t tell you that. [Another participant, agreeing] “Patients don’t…I mean, most patients won’t bring it up themselves.” NY-1 Faculty MD focus group
Another way in which screening was thought to add value was by providing a ‘teachable moment’ for educating patients about substance use and related harms. This potential benefit was raised by both patients and PCPs, who felt that patients may not understand that their substance use is a health risk until they are specifically asked about it. As stated by a patient:
Some people don’t know how to ask for help. Or sometimes they don’t even realize that they need help. So maybe just talking about it with their doctor, you might not even realize how much you really do drink or how much you really do smoke unless someone asks you, oh, so how many drinks, you know… Like, how many drinks would you say that you had in a week? And if you’re somebody that’s like, oh, I don’t have a problem. But if you think about it and be like, well, I have about ten drinks a week, you may begin to think like maybe I do have a problem. So, you know, it keeps that… It makes doctors and patients actually talk about their issues…. NY-1 Patient focus group
Assessing barriers to screening for substance use
Participants identified both individual-level and systems-level barriers to implementing substance use screening in primary care settings. Individual-level factors for patients included feeling uncomfortable disclosing substance use because they fear a negative reaction from their provider, concerns about confidentiality, and not being ready to discuss their substance use. Individual-level factors for providers included their knowledge and capacity to respond effectively to substance use. Systems-level factors included limitations imposed by the clinic’s physical environment and lack of time.
Individual level barriers
Patients worry about how medical providers will react.
Patients and providers stated that patients may be uncomfortable disclosing substance use out of fear of being judged by their provider. They noted that the quality of the patient-provider relationship is an important determinant of whether patients will feel comfortable disclosing substance use. This viewpoint is captured in the following patient’s statement:
You know, people are so afraid, I think sometimes of being judged. That sometimes it just creates this impediment in regards to being as forthcoming as you possibly can. I think the relationship [with the physician] is really the foundation… NY-2 Patient focus group
Some patients expressed concern about unforeseen consequences of screening, if providers were to react negatively to a patient’s disclosure of substance use. One patient speculated that if they felt uncomfortable with their provider’s reaction it could impact their engagement in care, although they did not see this as a barrier for all patients.
Even, like, your doctor, it’s like, well, maybe they’ll look at me differently now. And then I’m uncomfortable coming. And then, so it could create this kind of like spiral of paranoia, you know. And then being uncomfortable and then feeling like, oh, now I have to leave. And like it could not end so well. You know what I mean? But then there’s people who I think would be totally honest. NY-2 Patient focus group
Patients are concerned about having substance use information in the medical record
Patients also expressed concerns about substance use information appearing in their medical record, and how that could affect the care they receive from other providers. Some patients felt that having substance use information in their medical record could potentially impact their job, insurance payments for medical care, and providers’ willingness to prescribe some medications (such as controlled substances). This overall unease with documentation of substance use was captured in the words of one patient:
…with the substance use being judged or, you know, against I guess societal expectations or whatever, I just think that that could also pose…you know, have someone feeling like I’m not too comfortable, you know, with that being in the records. NY-2 Patient focus group
Providers were sensitive to patients’ concerns about substance use information appearing in the medical record. One of the Oregon physicians with health information technology expertise discussed interacting with other healthcare providers who feel that it may not be appropriate to fully integrate substance use information into the EHR. A number of providers expressed heightened concern about documenting substance use, especially now that patients are often able to view their own medical records. This view is summarized here by one MA, but was similarly expressed by PCPs who worry about how patients will react to seeing substance use documented in their chart.
And it doesn’t say confidential or whatever. So people are very worried. And it actually puts us at major risk when we start documenting all this stuff, that the patients will come back and say.. ‘you said that I drank, you know, five bottles a day and I’m an alcoholic.’ They read these things. NY-2 MA focus group
Substance use is viewed differently from other medical conditions
Patients talked about the stigma of substance use, and some felt that the patient gets blamed for having a substance use disorder. While this was not a theme in the provider interviews, patients were sensitive to how a patient with a substance use disorder could feel accused of bringing the condition upon themselves, and worried about how this would impact their treatment. As expressed by one patient:
I would think it would be something about substance use, because there’s a stigma attached to it. Like, the fact that you maybe had cancer or you had heart disease, like you could say, well, that’s not my fault. Like that’s something that happened to me. And I overcame this, right? As opposed to the way the world looks at substance abuse as ‘this is your fault, you did this to yourself’ type of mentality. So I think that it would definitely be a difference between your previous medical conditions and substance abuse. NY-2 Patient focus group
Patients may not be ready to disclose substance use
Patients and providers discussed that an individual’s own reluctance to acknowledge having a substance use problem, even to themselves, can pose a barrier to screening. Many participants stated that patients need to be ready to be honest with themselves about their substance use before they can be expected to disclose it to their medical providers. A related theme was that patients who are not ready and willing to receive help will not disclose substance use, and for these patients screening may be ineffective. This perspective was summarized by a patient:
And so if a person’s willing to get honest and truthful about where they’re at in their station in life….Then it’s good. But if the person is still addicted and deny their drug use or alcoholism or whatever it is, you know, the screening, I don’t know how effective that would be. NY-1 Patient focus group
Provider knowledge and training
Both faculty and resident PCPs identified knowledge deficits as a barrier to providing screening and interventions to address substance use. Several providers stated that although they received training in addressing other behavioral health conditions, such as smoking cessation and depression treatment, they had less knowledge about alcohol and drug use. As a result, they felt poorly prepared to address substance use when it was identified. Knowledge was discussed as both a lack of education about substance use and as a lack of familiarity with assessing patients and linking them to appropriate treatment. As stated by one faculty PCP:
So that’s a barrier for me, is like my own lack of knowledge of what’s out there or what’s reliable and good, and what the culture of those places is [so] that I can even advise my patients, [for example] ‘Hey, I think this would be a good fit for you because,’ or ‘You might want to try to this first instead because.’ And I think that’s important when somebody is making a choice to try to do something like that. NY-2 Faculty MD focus group
Although a small number of PCPs in our sample noted that they had received specialized training in substance use and felt comfortable with substance use interventions, they still felt that they would benefit from additional hands-on teaching, such as having a coach to observe their clinical interactions and offer feedback.
Residents in particular expressed discomfort with not knowing how to address a positive screening result, because they do not know how to treat it themselves and are unfamiliar with the treatment resources available in their clinic or in the community. As one resident noted, they may be knowledgeable about treating tobacco use, and feel confident with smoking cessation counseling, but they feel poorly prepared to intervene if a patient has alcohol or drug use.
I think for smoking I have a pretty good idea of like options for my patients. And I counsel them fairly often about it. And, you know, there’s like medication options and like all that sort of stuff. But for alcohol and drugs, like I have no idea like where to send them if they screen positive. NY-1 Resident MD focus group
Patients themselves also expressed concern that providers may not have the proper training or knowledge to work with patients who report substance use. Some discussed substance use as a condition that requires specialized care, and one patient equated expecting physicians to address substance use with asking a cardiologist ‘about what’s going on with your foot.’ While they were skeptical about doctors being able to intervene, patients did express more confidence in their ability to make referrals for treatment. As one patient stated,
But I don’t believe that they know how to handle that. They would refer you to someone. But I don’t think they would know what to do. NY-2 Patient focus group
PCPs may fail to address a positive screening result
Medical assistants expressed concern that primary care providers would not respond appropriately to patients with substance use problems. This would make them feel less comfortable screening, because they were not confident that it would ultimately help the patient.
Because I feel like if he’ll tell me, a patient, oh yeah, I’m an alcoholic, I need help. Like she said with the depression, here’s a box of tissues, sit outside. And then the doctor doesn’t address it. And he says, ‘I told you I had this problem and nothing was done.’ And I’m sorry, but then we have care coordinators, navigators, like all these resources but they feel nobody’s there to help them. So like we’re in the middle. Then you feel bad. Here they come again. This is the second time you ask me this question, and they didn’t help me the first time so I’m not going to answer. We’ve had that. NY-2 MA focus group
System-level barriers
A number of systems-level barriers were identified by providers. The main barriers identified were lack of space and privacy in the clinic, poor access to treatment for patients with substance use disorders, and the time pressures of primary care visits.
Lack of privacy
Medical assistants felt strongly that screening for substance use should be done individually and in a private room. Many reported that they did not have enough privacy in their workspace, and that screening under these conditions would be disrespectful to patients. One MA expressed that screening in their current space would make patients angry.
The space is so tight. And the space is so limited… She used to have…I don’t know if you still do, two chairs in the same room, two patients at the same time, two MAs working. How are you going to ask that kind of question to a patient? They’re going to fly, right, they’re going to be very angry. I mean, that’s something very private. NY-1 MA focus group
Lack of treatment resources for patients with substance use problems
Medical providers stated that better systems are necessary for delivering effective treatment to patients with SUDs, and that this needs to be in place before initiating a screening program. Several providers mentioned that they do not know where to refer patients, and that they lack resources in the clinic to help patients with substance use problems. As one resident expressed, it is discouraging for providers to feel that they cannot connect patients to appropriate care.
And I feel like the limitations on where we can refer people afterwards. Like, well, you don’t quality for inpatient rehab. So I guess, what are we going to do? Try to go to AA? It didn’t really feel like we were providing that much of a service… NY-1 Resident MD focus group
Most providers and patients discussed substance use treatment as something that happens outside the primary care clinic, and requires referral to a community-based program. However, some providers expressed hope that there would be more primary care-integrated treatment in the future, particularly with the increased use of medication for addiction treatment. This perspective was summarized by one of the Oregon PCPs:
It doesn’t do any good to screen for anything that you don’t have a treatment for or can’t access a treatment for, and I think there is hope as medicated medication assisted treatments expand into primary care. You know, the providers will have more tools available to them. Hopefully, we’ll have more counseling resources as a result of the Affordable Care Act and mental health parity in primary care, but right now most primary care clinics don’t. OR Faculty MD interview
Providers who worked in clinics that had a social worker or behavioral health specialist saw them as a valuable member of the care team, but stated that insufficient resources are devoted to integrated care for substance use in the primary care setting. Specifically, one provider noted that their clinic has one substance use counselor for ‘several thousand patients in internal medicine,’ and that despite the high level of need, the funding for this position is constantly in jeopardy because the counselor is unable to bill for services.
Overwhelmingly, medical providers and staff identified lack of time as a barrier to addressing substance use in primary care. They noted that visits are brief, and that there are usually multiple competing priorities, such as the patient’s chief complaint and other medical conditions. An example was given by one physician:
And I’m thinking of one person in particular who comes in, and by the time we talked about his chronic pain and primary hyper- pulmonary hypertension, neuropathy and fall risk and depression, I rarely have time to get to his substance use screening issues. Even though I’ve been taking care of him for thirteen years now and I know that he has a remote history of alcohol use disorder, I rarely, in a single visit, will get to that. OR Faculty MD interview
Relatedly, some providers felt that discussing substance use felt like ‘opening a can of worms’ that would be difficult to address. Some suggested that being able to bring patients back for a visit that focused just on substance use would help, but also noted that their schedules were usually so full that this may not be possible. These concerns were expressed by faculty and resident PCPs, and captured here in the words of a resident:
I think one of the other things is we’re a little afraid of substance abuse in the sense of that, if it comes up I feel like in order to really deal with it there are a lot of psychosocial issues that are behind it. Like people have a lot of co-morbid mental illness. And those are just difficult and very time consuming issues for us. So you really need like a dedicated visit when that comes up. NY-1 Resident MD focus group
Adapting knowledge to the local context
Participants made a number of recommendations for how to implement screening in their practices. There was broad agreement across stakeholder groups on some aspects of the screening approach, but differences of opinion regarding other aspects, such as who among the clinical staff should deliver screening.
What substances should screening address?
Screening should encompass tobacco, alcohol and other drugs.
There was broad consensus across stakeholder groups that screening should address all classes of substance use, rather than being confined to tobacco or alcohol alone. There were no participants who voiced a different opinion on this question. Some MDs additionally noted that screening should capture the full spectrum of severity, to identify patients with unhealthy use as well as more severe substance use disorders.
Should screening be universal or targeted?
Universal screening.
Most participants were in favor of universal screening, and stated that targeted screening is likely to miss patients who have unhealthy substance use, and would be less acceptable to patients. Some patients and MAs voiced strong opinions that targeted screening could be discriminatory. One patient equated it with a ‘stop and frisk’ approach to policing, which has been criticized for targeting minority populations in NYC. These views that targeted screening may be less accurate, and that it could be less comfortable for patients who feel singled out for screening, were summarized by one patient in a focus group:
Let’s suppose they ask you if you’re on drugs, if you’re doing this, what are you doing, but they don’t ask the other one then what would…I will be thinking why he would only ask me and not him? You understand what I’m trying to say? I mean, you can’t just, you know, sit there and say, okay, you look like a drinker, you look like a smoker, you know whatever. NY-1 Patient focus group
Targeted screening
While universal screening was favored by most providers, some noted that screening all patients is inefficient, and could crowd out higher value care. They noted that universal screening may not be feasible given the time limitations in primary care. In one of the MA focus groups, it was mentioned that screening older patients (over the age of 70 years) may not be necessary, since they are less likely to have substance use problems. As one provider stated,
Inherently I would think, yeah, generalized screening. But then if we are thinking within the context of primary care visit, now am I doing that at the loss of something else, other problems? And, yes, everything is important. And if I do preventative services it will be five hours a visit. So here’s where I pick and choose. I wanted the universal screening. But what gives more bang for my buck? NY-1 Faculty MD focus group
How frequently should screening occur?
Annual screening.
PCPs and MAs commented on the frequency of screening. The majority felt that screening once per year is most appropriate, and stated that annual screening is typical for other conditions. Some recommended that screening be done as part of an annual visit, though they also acknowledged that not all patients will have a dedicated annual visit for preventive care.
I think it would be great if it was a routine part of general health screening. So every time you get your annual exam, you know, we ask about exercise. We ask about nutrition. We ask about psychosocial factors. And we include alcohol, substance abuse, depression screening in all of that. OR Faculty MD interview
More than once a year
A minority of participants felt that screening should occur more frequently, and possibly at every visit, because substance use behaviors can change over time. They expressed concern that substance use can escalate quickly, and that limiting screening to once per year could result in missed opportunities for early intervention with respect to new or resurgent substance use. This view was expressed by a MA, who stated:
I do think it should be more than once a year. Because you could go do through something midyear and then go see the doctor and that question doesn’t pop up. And then you’re depressed, and then it’s not being taken care of and something serious later could happen. NY-2 Medical Assistant
How should screening be administered?
Patient self-administered screening.
There was some discrepancy of opinion regarding how screening should be administered, but the majority of participants preferred a self-administered approach. Self-administered screening was favored by providers and staff because it could save time. There was also a perception that patients would be more comfortable disclosing substance use if they did not have to report it face-to-face. This view is illustrated in the following comment from a Registered Nurse:
If I’m the patient, I think I’ll be more truthful just checking it on the form rather than a medical assistant asking me. It’s kind of like if I’m taking drugs then I’ll be embarrassed telling her. NY-1 RN focus group
Face-to-face screening
A minority of participants preferred that screening be done in person, while also noting that the patient’s trust in the person asking the questions is important. Some participants, including patients and providers, expressed concern over recording substance use related information in writing, and felt it would be more comfortable to talk about it than putting it down on a form. As expressed by one patient:
Because sometimes you don’t know where that form is going. You want it to be real personal. I want to talk to my doctor… I think that when people put it down on paper it becomes real. NY-1 Patient focus group
There was also a broader discussion in some groups that medical providers spend too much time looking at the EHR, and that this makes care less personal and can be a barrier to developing patient-provider relationships. As expressed by a MA, this was a reason for preferring face-to-face screening:
Sometimes even these patients complain that the doctors are so busy with the computer, you know writing things while they’re being seen…you know seeing the patient. So I think it’s more personal when you have that contact face to face to discuss what your issues are. NY-2 MA focus group
Who should administer screening, if it is done face-to-face?
There were differences of opinion regarding who in the clinic should administer screening. Among MDs, most preferred that screening be done by ancillary staff in the clinic, and usually identified screening as a MA role. In the participating clinical sites, it was common practice for the MAs to deliver depression screening, and substance use screening was perceived as being similar. For the minority of PCPs who believed that screening should be administered by physicians, reasons included that the MAs are already quite burdened, variation in the quality of screening delivered by MAs, and beliefs that patients have greater trust in their physicians than in other members of the care team. They also noted that because it is ultimately the provider who needs to address a positive screen, they may want to ask the questions themselves. Concerns about the quality of MA-delivered screening were expressed by one NY physician:
That’s how…is the MA doing it? Is the MA looking at the screen and just asking the questions and not making eye contact and just checking off the boxes? ..Unless, again, it’s like you know which MA has done it. And you know what they’re like and what that rapport is… NY-2 Faculty MD focus group
In contradistinction to the opinion of most PCPs, the majority of MAs and patients stated a preference for PCPs to deliver screening, if it is to be administered face-to-face. They felt that PCP screening was more likely to be accurate, and would give patients an opportunity to discuss or clarify their responses with the provider. Some patients felt that their physician was better able to interpret their body language and engage them in conversation about their responses. MAs felt that patients would be more comfortable giving sensitive information only to their PCP. Some patients also worried that information could be lost or distorted if it was collected by the MA and then passed along to the PCP. This viewpoint is captured in a statement from one patient:
And then sometimes it can also be a communication breakdown between the medical assistant and getting it to the doctor. I would be worried that my doctor wouldn’t get it the way I would deliver it. NY-1 Patient focus group
When should screening occur?
Prior to the medical encounter.
Most participants felt that screening should be done prior to the medical encounter, either in the waiting room or before the patient arrives in clinic. A number of providers noted that the most preferred option would be for screening to be completed electronically, ideally through the patient portal while the patient is at home, and then integrated into the EHR prior to the visit.
What I would want to do is all the questionnaires would be done online. As much as can be done before the appointment would be put on the tablet. So they would update their medication list online. They would do all their questionnaires. So they would upgrade all their information. And it would all be done and ready to go for the MA and the provider. So that would be my ideal world. OR Faculty MD interview
During the clinic visit, in the exam room
Patients and MAs discussed the timing and location of screening during course of the medical visit, and did not bring up the possibility of pre-visit screening. Some expressed concerns about privacy, and felt screening was best performed in the exam room, and not in the waiting area. Patients and MAs were less likely than MDs to discuss electronic approaches to screening. Some expressed pessimism about the feasibility or acceptability of electronic screening for patients, particularly those who are older or less familiar with computers. As one patient stated:
I’d rather do it on paper. Or if I’m not capable, have someone verbally ask me. I don’t like computers. I’m not going to get into that. I wouldn’t answer the questions if it was through the computer. That’s me. And I find that a lot of my friends, the seniors, prefer the paperwork or someone to ask them, like a receptionist or something, you know. NY-1 Patient focus group
Through interviews with key clinical stakeholders, this study characterized current substance use screening practices, barriers to screening, and recommendations for its implementation in primary care clinics. Our approach is unique in capturing the views of patients, as well as those of primary care providers, residents, MAs, and RNs. To be successful in practice, screening must be acceptable to all of these groups. By including participants from two health systems that differ markedly both in their geography, their health care environment, and their experience with SBIRT, we captured diverse views.
In the KTA framework, essential early steps in implementation are to identify, address barriers, and adapt knowledge to the local context. This process is important for guiding implementation strategies. Over the past decade, many attempts to introduce screening and brief intervention (SBI) have faced challenges in their implementation, or have not been sustained in the absence of grant funding [ 24 , 38 , 39 , 40 , 41 ]. We considered these interviews, which were conducted as the initial phase of a larger implementation study, to be essential for designing a screening strategy that would address as many stakeholder concerns as possible, and thus be more likely to be adopted and sustained.
Participants from all stakeholder groups felt strongly that screening for substance use is important for the quality and safety of medical care, and is an essential part of the patient’s medical history. In order to make accurate diagnoses, manage other medical conditions, and provide appropriate preventive care, it is important for medical providers to know about a patient’s substance use [ 42 ]. This is a benefit of screening that is frequently overlooked in current discussions about the efficacy of SBIRT programs for reducing drug use. Current USPSTF guidelines recommend alcohol SBI for adults in primary care settings (Grade B recommendation) [ 13 ], but the evidence is considered insufficient to support a similar recommendation for drugs [ 43 ]. Recent clinical trials of SBI have had mixed results, with two studies demonstrating no impact on drug consumption [ 44 , 45 ], and one study showing short-term reductions [ 46 ]. Notably, the study by Gelberg et al. that did show reductions in drug use [ 46 ] employed patient self-administered screening and provided PCPs with a prompt and decision support encouraging them to offer very brief advice. This approach could address some of the important barriers identified by participants in our study, including patient fears about how providers will react, and provider lack of time and knowledge to intervene on unhealthy substance use. While more research is needed on primary care-based approaches to identifying and effectively addressing drug use [ 47 ], this does not diminish the importance of screening’s role in informing clinical care for other health problems.
Importantly, we captured the views of patients on primary care-integrated screening and interventions. While there is a rich literature on medical provider attitudes in this area [ 48 , 49 , 50 , 51 , 52 , 53 , 54 , 55 , 56 ], much less is known about patient attitudes [ 57 , 58 ]. One study in a Colorado health system, conducted by Rahm et al., interviewed primary care stakeholders, including patients as well as PCPs and clinical staff, about SBIRT implementation [ 58 ]. Similar to the views of patients captured in our interviews, patients in the Colorado study endorsed universal screening as part of routine care, felt that it could help open up patient-provider discussions about substance use, and expressed concerns about the confidentiality of this information when documented in the EHR. Our patient interviews also identified a number of additional individual-level barriers to screening, including fear of the medical provider’s reaction, and the stigma associated with SUDs, which patients felt were not treated like other medical conditions. These concerns were similarly expressed by primary care patients in one prior study, also conducted in New York City [ 57 ], which found that patients considered substance use to be highly stigmatized, and felt that patients would only share this information with trusted primary care providers.
Providers have stated concerns about patients being uncomfortable with screening in previous studies evaluating screening implementation efforts [ 41 , 59 ], but patient voices on this subject have not previously been well articulated. While some patients may feel comfortable raising the subject of substance use [ 51 ], our interviews indicate that many will not disclose this information unless they are reassured that they will not feel ‘judged’ by their providers or experience negative consequences from doing so. Our findings are consistent with previous literature showing that substance use disorders are among the most severely stigmatized health conditions [ 60 ]. This is particularly concerning in light of evidence that patients who feel stigmatized by providers have poor treatment outcomes [ 61 , 62 ].
An additional theme from our patient interviews, which has not been highlighted in the screening implementation literature, is that patients lack confidence in their medical providers’ ability to effectively address substance use. In the face of stigma and expectations of negative repercussions from disclosing substance use, patients need to feel that there is benefit to doing so. This benefit can only be realized if medical providers are prepared to deliver appropriate medical care and treatment interventions to patients with unhealthy substance use. While more research on patient attitudes toward screening is needed, our findings point to the importance of articulating the value and purpose of screening to patients, informing patients about exactly how their substance use information will be used, and addressing perceived negative attitudes and lack of knowledge among medical providers and staff as being highly important to the success of any screening program.
Our interviews with providers reiterated many of the individual- and systems-level barriers that have been found in prior research [ 48 , 49 , 50 , 51 , 52 , 53 , 54 , 55 , 56 , 63 ]. Medical providers, including PCPs and MAs, repeatedly raised lack of time and competing demands during the primary care visit, and expressed concerns that physicians lack knowledge about substance use and addiction. Perhaps related to lack of knowledge about the spectrum of substance use that is typically identified via primary care screening, providers in our study seemed to conflate a positive screen with a need for addiction treatment, which would be accomplished by making a referral for specialty care. Providers voiced frustration about lack of access to treatment resources, and not knowing how to refer patients to treatment programs, but there was almost no mention of the potential role of PCPs themselves in providing treatment for substance use disorders. This finding echoes another recent primary care study, which found that PCPs frequently believed that treatment for alcohol use disorder requires intensive counseling resources and that specialty care is most effective [ 56 ]. More focused efforts are needed to engage patients in treatment, including primary care-based SUD treatment (e.g., office-based opioid and alcohol pharmacotherapy) or the use of on-site behavioral health providers who can assess and engage patients, and make warm hand-offs to specialty care. Our study indicates that having effective approaches to delivering treatment is important for cultivating PCP support for a substance use screening program.
Limitations
Our study has some limitations. While we included sites from very different parts of the U.S., both were academic medical centers located in urban areas. Individuals from rural areas, or in smaller community primary care practices, may have different views on substance use screening. Most interviews were done at the New York site, and we were not able to conduct any patient interviews or focus groups in Oregon, which gave us a more limited picture of stakeholder attitudes from this health system. PCPs in our sample included both residents and faculty, but all were physicians. This reflects the characteristics of our sites, in which the majority of primary care providers, and all individuals identified as having SBIRT or HIT expertise, were MDs. Licensed providers with different training, such as nurse practitioners or Doctors of Osteopathy, may have different views about screening. While we consider the inclusion of patients to be an important strength of the study, it proved difficult to recruit younger patients, and so their views may be underrepresented. This could be important, because younger patients may have higher rates of substance use, and greater concerns about issues such as the impact of screening on their employment or health insurance. Interviews were conducted only in English, which also excluded some patients. Because our objective was to capture the views of a general primary care patient population, we have little representation of patients with current alcohol and drug use disorders. Our interviews were conducted by the study PI (in New York) or the site Lead Investigator (in Oregon), which has the potential to introduce social desirability bias. However, we did not see direct evidence of this in the interviews, in which many participants spoke critically about the current system of care and about substance use screening and interventions.
Finally, we found that stigma and negative attitudes toward substance use were common themes in our interviews, particularly in the patient focus groups. While the KTA framework includes individual attitudes as ‘barriers to knowledge use,’ being a process model it focuses less on the factors that underlie negative attitudes, or on how attitudes may change through the process of implementing a new practice. Employing an additional theoretical model that more explicitly explores individual attitudes and behavior change may have been useful for fully exploring these themes.
This qualitative study can inform the design of substance use screening programs in primary care practices. Based on our findings, we have designed and are now testing a strategy that seeks to optimally utilize existing staff and resources to deliver screening in the participating clinics. We are implementing validated brief screening questionnaires, administered annually to all patients, using a patient self-administered approach when possible. Screening results will be paired with EHR-integrated clinical decision support to assist primary care providers in conducting a brief counseling intervention. We have improved the system for linking patients with high-risk substance use to care by identifying a clear process for referring patients to the clinic’s existing social workers, training the social workers in brief intervention, and improving their knowledge of referral sources for addiction treatment. We are hopeful that educating medical providers about substance use and interventions will begin to address providers’ negative attitudes toward patients with unhealthy alcohol and drug use, but more work is likely needed to address stigmatizing beliefs among providers and clinical staff. To directly address patient concerns about stigma, we plan to use signage and consistent language to communicate to patients that screening is universal, and is part of routine medical care.
Our findings provide general guidance regarding key elements of a screening implementation approach for primary care settings. Screening programs should clearly communicate the goals of screening to patients and seek to proactively counteract stigma, address staff concerns regarding time and workflow, and provide education as well as treatment resources to primary care providers. Without a significant infusion of resources (to support, for example, longer primary care visits, or more behavioral health staff), no screening strategy will be able to address all of the barriers that were identified. Patient concerns about the confidentiality of their screening results, and about how their providers will react, remain as important challenges to screening in medical settings. However, our understanding of stakeholder views can inform substance use screening implementation efforts by identifying important barriers that need to be acknowledged, and addressed to the extent possible, when initiating a screening program.
Abbreviations
Coordinated Care Organization
Clinical Trials Network
electronic health record
Knowledge to Action (implementation framework)
medical assistant
medical doctor
National Institute on Drug Abuse
primary care provider
principal investigator
registered nurse
screening and brief intervention
screening, brief intervention, and referral to treatment
United States Preventive Services Task Force
Dowell D, et al. Contribution of opioid-involved poisoning to the change in life expectancy in the United States, 2000–2015. JAMA 2017;318(11):1065–7.
Horgan, CM, Skwara KC, Strickler G. Substance abuse: the nation’s number one health problem. Robert Wood Johnson Foundation and Schneider Institute for Health Policy, 2001.
Mokdad AH, Marks JS, Stroup DF, Gerberding JL. Actual causes of death in the United States, 2000. JAMA. 2004;291(10):1238–45.
Article PubMed Google Scholar
McGinnis JM, Foege WH. Actual causes of death in the United States. JAMA. 1993;270(18):2207–12.
Article CAS PubMed Google Scholar
Substance Abuse and Mental Health Services Administration. NSDUH data review: receipt of services for substance use and mental health issues among adults: results from the 2016 national survey on drug use and health. Rockville: Substance Abuse and Mental Health Services Administration; 2017. https://www.samhsa.gov/data/sites/default/files/NSDUH-DR-FFR2-2016/NSDUH-DR-FFR2-2016.pdf . Accessed 6 Feb 2018.
Edlund MJ, Unutzer J, Wells KB. Clinician screening and treatment of alcohol, drug, and mental problems in primary care: results from healthcare for communities. Med Care. 2004;42(12):1158–66.
Rehm J, Anderson P, Manthey J, Shield KD, Struzzo P, Wojnar M, et al. Alcohol use disorders in primary health care: what do we know and where do we go? Alcohol Alcohol. 2016;51(4):422–7.
O’Donnell A, Anderson P, Newbury-Birch D, Schulte B, Schmidt C, Reimer J, et al. The impact of brief alcohol interventions in primary healthcare: a systematic review of reviews. Alcohol Alcohol. 2014;49(1):66–78.
Solberg LI, Maciosek MV, Edwards NM. Primary care intervention to reduce alcohol misuse ranking its health impact and cost effectiveness. Am J Prev Med. 2008;34(2):143–52.
Whitlock EP, Polen MR, Green CA, Orleans T, Klein J. Behavioral counseling interventions in primary care to reduce risky/harmful alcohol use by adults: a summary of the evidence for the U.S. Preventive Services Task Force. Ann Intern Med. 2004;140(7):557–68.
Maciosek MV, Coffield AB, Edwards NM, Flottemesch TJ, Goodman MJ, Solberg LI. Priorities among effective clinical preventive services: results of a systematic review and analysis. Am J Prev Med. 2006;31(1):52–61.
Kaner EF, Dickinson HO, Beyer F, Pienaar E, Schlesinger C, Campbell F, et al. The effectiveness of brief alcohol interventions in primary care settings: a systematic review. Drug and Alcohol Review. 2009;28(3):301–23.
Moyer VA. Screening and behavioral counseling interventions in primary care to reduce alcohol misuse: U.S. preventive services task force recommendation statement. Ann Intern Med. 2013;159(3):210–8.
PubMed Google Scholar
SBIRT: Screening, Brief Intervention, and Referral to Treatment 2014 [cited 13 Oct 2014]. http://www.integration.samhsa.gov/clinical-practice/SBIRT . Accessed 14 July 2017.
U.S. Department of Health and Human Services (HHS), Office of the Surgeon General, Facing Addiction in America: The Surgeon General’s Report on Alcohol, Drugs, and Health. Washington; 2016.
Babor TF, McRee BG, Kassebaum PA, Grimaldi PL, Ahmed K, Bray J. Screening, Brief Intervention, and Referral to Treatment (SBIRT): toward a public health approach to the management of substance abuse. Subst Abuse. 2007;28(3):7–30.
Article Google Scholar
Nilsen P. Brief alcohol intervention—where to from here? Challenges remain for research and practice. Addiction (Abingdon, England). 2010;105(6):954–9.
Nilsen P, Aalto M, Bendtsen P, Seppa K. Effectiveness of strategies to implement brief alcohol intervention in primary healthcare. A systematic review. Scand J Prim Health Care. 2006;24(1):5–15.
Kaner E. Brief alcohol intervention: time for translational research. Addiction (Abingdon, England). 2010;105(6):960–1.
Babor TF, Higgins-Biddle JC. Alcohol screening and brief intervention: dissemination strategies for medical practice and public health. Addiction. 2000;95(5):677–86.
Rieckmann T, Abraham A, Zwick J, Rasplica C, McCarty D. A longitudinal study of state strategies and policies to accelerate evidence-based practices in the context of systems transformation. Health Serv Res. 2015;50(4):1125–45.
Garnick DW, Horgan CM, Merrick EL, Hoyt A. Identification and treatment of mental and substance use conditions: health plans strategies. Med Care. 2007;45(11):1060–7.
Mertens JR, Chi FW, Weisner CM, Satre DD, Ross TB, Allen S, et al. Physician versus non-physician delivery of alcohol screening, brief intervention and referral to treatment in adult primary care: the ADVISe cluster randomized controlled implementation trial. Addict Sci Clin Pract. 2015;10(1):26.
Article PubMed PubMed Central Google Scholar
Williams EC, Johnson ML, Lapham GT, Caldeiro RM, Chew L, Fletcher GS, et al. Strategies to implement alcohol screening and brief intervention in primary care settings: a structured literature review. Psychol Addict Behav. 2011;25(2):206–14.
NIDA CTN Common Data Elements. https://cde.drugabuse.gov/ . Accessed 14 July 2017.
Ghitza UE, Gore-Langton RE, Lindblad R, Shide D, Subramaniam G, Tai B. Common data elements for substance use disorders in electronic health records: the NIDA Clinical Trials Network experience. Addiction. 2013;108(1):3–8.
Nilsen P. Making sense of implementation theories, models and frameworks. Implement Sci. 2015;10:53.
Graham ID, Logan J, Harrison MB, Straus SE, Tetroe J, Caswell W, et al. Lost in knowledge translation: time for a map? J Contin Educ Health Prof. 2006;26(1):13–24.
Kastner M, Straus SE. Application of the Knowledge-to-Action and Medical Research Council frameworks in the development of an osteoporosis clinical decision support tool. J Clin Epidemiol. 2012;65(11):1163–70. https://doi.org/10.1016/j.jclinepi.2012.04.011 .
Oregon Health Authority. 2013 Quality Pool Reference Instructions. http://www.oregon.gov/oha/analytics/CCOData/Reference%20Instructions.pdf . Accessed 3 Mar 2017.
Rieckmann T, Renfro S, McCarty D, Baker R, McConnell KJ. Quality metrics and systems transformation: are we advancing alcohol and drug screening in primary care? Health Serv Res. 2017;. https://doi.org/10.1111/1475-6773.12716 .
Medscape. Medscape EHR report 2016. http://www.medscape.com.ezproxy.med.nyu.edu/features/slideshow/public/ehr2016 . Accessed 7 July 2017.
Patton MQ. Qualitative research and evaluation methods. 3rd ed. Thousand Oaks: Sage; 2002.
Google Scholar
Patton MQ. Enhancing the quality and credibility of qualitative analysis. Health Serv Res. 1999;5(34):1189–208.
Boyatzis RE. Thematic analysis and code development: transforming qualitative information. London: Sage; 1998.
Fleiss JL. Statistical methods for rates and proportions. 2nd ed. New York: Wiley; 1981.
Averill JB. Matrix analysis as a complementary analytic strategy in qualitative inquiry. Qual Health Res. 2002;12:855–66.
Kaner E, Bland M, Cassidy P, Coulton S, Deluca P, Drummond C, et al. Screening and brief interventions for hazardous and harmful alcohol use in primary care: a cluster randomised controlled trial protocol. BMC Public Health. 2009;9:287. https://doi.org/10.1186/471-2458-9-287 .
Hilbink M, Voerman G, van Beurden I, Penninx B, Laurant M. A randomized controlled trial of a tailored primary care program to reverse excessive alcohol consumption. J Am Board Fam Med. 2012;25(5):712–22.
van Beurden I, Anderson P, Akkermans RP, Grol RP, Wensing M, Laurant MG. Involvement of general practitioners in managing alcohol problems: a randomized controlled trial of a tailored improvement programme. Addiction (Abingdon, England). 2012;107(9):1601–11.
Williams EC, Achtmeyer CE, Young JP, Rittmueller SE, Ludman EJ, Lapham GT, et al. Local implementation of alcohol screening and brief intervention at five Veterans Health Administration primary care clinics: perspectives of clinical and administrative staff. J Subst Abuse Treat. 2016;60:27–35.
Berger D, Bradley KA. Primary care management of alcohol misuse. Med Clin N Am. 2015;99(5):989–1016.
U.S. Preventive Services Task Force. Screening for Illicit Drug Use: U.S. Preventive Services Task Force Recommendation Statement. 2008. https://www.uspreventiveservicestaskforce.org/Page/Document/UpdateSummaryFinal/drug-use-illicit-screening . Accessed 14 July 2017.
Roy-Byrne P, Bumgardner K, Krupski A, Dunn C, Ries R, Donovan D, et al. Brief intervention for problem drug use in safety-net primary care settings a randomized clinical trial. J Am Med Assoc. 2014;312(5):492–501.
Saitz R, Palfai TPA, Cheng DM, Alford DP, Bernstein JA, Lloyd-Travaglini CA, et al. Screening and brief intervention for drug use in primary care the ASPIRE randomized clinical trial. J Am Med Assoc. 2014;312(5):502–13.
Gelberg L, Andersen RM, Afifi AA, Leake BD, Arangua L, Vahidi M, et al. Project QUIT (Quit Using Drugs Intervention Trial): a randomized controlled trial of a primary care-based multi-component brief intervention to reduce risky drug use. Addiction (Abingdon, England). 2015;110(11):1777–90.
Hingson R, Compton WM. Screening and brief intervention and referral to treatment for drug use in primary care: back to the drawing board. JAMA. 2014;312(5):488–9. https://doi.org/10.1001/jama.2014.7863 .
Sterling S, Kline-Simon AH, Wibbelsman C, Wong A, Weisner C. Screening for adolescent alcohol and drug use in pediatric health-care settings: predictors and implications for practice and policy. Addict Sci Clin Pract. 2012;7(1):13.
Friedmann PD, McCullough D, Saitz R. Screening and intervention for illicit drug abuse: a national survey of primary care physicians and psychiatrists. Arch Intern Med. 2001;161(2):248–51.
Friedmann PD, McCullough D, Chin MH, Saitz R. Screening and intervention for alcohol problems. A national survey of primary care physicians and psychiatrists. J Gen Intern Med. 2000;15(2):84–91.
Article CAS PubMed PubMed Central Google Scholar
McCormick KA, Cochran NE, Back AL, Merrill JO, Williams EC, Bradley KA. How primary care providers talk to patients about alcohol: a qualitative study. J Gen Intern Med. 2006;21(9):966–72.
Spandorfer JM, Israel Y, Turner BJ. Primary care physicians’ views on screening and management of alcohol abuse: inconsistencies with national guidelines. J Fam Pract. 1999;48(11):899–902.
CAS PubMed Google Scholar
Johnson M, Jackson R, Guillaume L, Meier P, Goyder E. Barriers and facilitators to implementing screening and brief intervention for alcohol misuse: a systematic review of qualitative evidence. J Public Health (Oxf). 2011;33(3):412–21. https://doi.org/10.1093/pubmed/fdq095 .
Article CAS Google Scholar
Aira M, Kauhanen J, Larivaara P, Rautio P. Factors influencing inquiry about patients’ alcohol consumption by primary health care physicians: qualitative semi-structured interview study. Fam Pract. 2003;20(3):270–5.
Yoast RA, Wilford BB, Hayashi SW. Encouraging physicians to screen for and intervene in substance use disorders: obstacles and strategies for change. J Addict Dis. 2008;27(3):77–97. https://doi.org/10.1080/10550880802122687 .
Williams EC, Achtmeyer CE, Young JP, Berger D, Curran G, Bradley KA, et al. Barriers to and facilitators of alcohol use disorder pharmacotherapy in primary care: a qualitative study in five VA clinics. J Gen Intern Med. 2017. https://doi.org/10.1007/s11606-017-4202-z .
Spear SE, Shedlin M, Gilberti B, Fiellin M, McNeely J. Feasibility and acceptability of an audio computer-assisted self-interview version of the Alcohol, Smoking and Substance Involvement Screening Test (ASSIST) in primary care patients. Subst Abuse. 2016;37(2):299–305.
Rahm AK, Boggs JM, Martin C, Price DW, Beck A, Backer TE, et al. Facilitators and barriers to implementing Screening, Brief Intervention, and Referral to Treatment (SBIRT) in primary care in integrated health care settings. Subst Abuse. 2015;36(3):281–8.
Williams EC, Achtmeyer CE, Thomas RM, Grossbard JR, Lapham GT, Chavez LJ, et al. Factors underlying quality problems with alcohol screening prompted by a clinical reminder in primary care: a multi-site qualitative study. J Gen Intern Med. 2015.
Schomerus G, Lucht M, Holzinger A, Matschinger H, Carta MG, Angermeyer MC. The stigma of alcohol dependence compared with other mental disorders: a review of population studies. Alcohol Alcohol. 2011;46(2):105–12.
Brener L, von Hippel W, von Hippel C, Resnick I, Treloar C. Perceptions of discriminatory treatment by staff as predictors of drug treatment completion: utility of a mixed methods approach. Drug Alcohol Rev. 2010;29(5):491–7.
Luoma JB, Twohig MP, Waltz T, Hayes SC, Roget N, Padilla M, et al. An investigation of stigma in individuals receiving treatment for substance abuse. Addict Behav. 2007;32(7):1331–46.
Anderson P, Laurant M, Kaner E, Wensing M, Grol R. Engaging general practitioners in the management of hazardous and harmful alcohol consumption: results of a meta-analysis. J Stud Alcohol. 2004;65(2):191–9.
Download references
Authors’ contributions
JM, TR, JK, RNR, DM, JR: Conception and design. JM, TR, ES, PCK: Analysis and interpretation of the data. All authors: Drafting and revision of the manuscript. SF, CC, AV, EAW, LP: Administrative, technical, or logistic support. JM, PCK, SF, CC, AV, EAW, LP: Collection of data. All authors read and approved the final manuscript.
Acknowledgements
The authors wish to acknowledge Melanie Harris, Marie Payment, Patricia Novo, Carol Cushing, and Carmen Rosa, and for their assistance with the study.
Competing interests
The authors declare that they have no competing interests
Availability of data and materials
The data collected and analyzed for the current study are available from the corresponding author on reasonable request.
Consent for publication
This publication was reveiwed and approved by the NIDA CTN Publications Committee.
Ethics approval and consent to participate
The study was approved by the Institutional Review Boards of NYU School of Medicine, Icahn School of Medicine at Mt. Sinai, and Oregon Health and & Science University.
Supported by National Institute on Drug Abuse cooperative awards: U10DA013035, UG1DA013035, and UG1DA015815.
Publisher’s Note
Springer Nature remains neutral with regard to jurisdictional claims in published maps and institutional affiliations.
Author information
Authors and affiliations.
Department of Population Health, New York University School of Medicine, 550 First Avenue, VZ30 6th Floor, New York, NY, 10016, USA
Jennifer McNeely, Pritika C. Kumar, Erica Sedlander & Christine Chollak
Division of General Internal Medicine, Department of Medicine, New York University School of Medicine, 550 First Avenue, New York, NY, 10016, USA
Jennifer McNeely
Greenfield Health and Department of Psychiatry, Oregon Health and Science University, 9450 SW Barnes Suite 100, Portland, OR, 97225, USA
Traci Rieckmann
Department of Psychiatry, New York University School of Medicine, One Park Avenue, 8th Floor, New York, NY, 10016, USA
Sarah Farkas & John Rotrosen
Division of General Internal Medicine, Department of Medicine, Icahn School of Medicine at Mt. Sinai, One Gustave L. Levy Place, New York, NY, 10029, USA
Joseph L. Kannry, Aida Vega, Eva A. Waite & Lauren A. Peccoralo
Department of Psychiatry, Icahn School of Medicine at Mt. Sinai, 1090 Amsterdam Avenue, New York, NY, 10025, USA
Richard N. Rosenthal
OHSU-PSU School of Public Health, Oregon Health and Science University, 3181 SW Sam Jackson Park Road, Portland, OR, 97239, USA
Dennis McCarty
You can also search for this author in PubMed Google Scholar
Corresponding author
Correspondence to Jennifer McNeely .
Additional file
Additional file 1..
Interview Guides.
Rights and permissions
Open Access This article is distributed under the terms of the Creative Commons Attribution 4.0 International License ( http://creativecommons.org/licenses/by/4.0/ ), which permits unrestricted use, distribution, and reproduction in any medium, provided you give appropriate credit to the original author(s) and the source, provide a link to the Creative Commons license, and indicate if changes were made. The Creative Commons Public Domain Dedication waiver ( http://creativecommons.org/publicdomain/zero/1.0/ ) applies to the data made available in this article, unless otherwise stated.
Reprints and permissions
About this article
Cite this article.
McNeely, J., Kumar, P.C., Rieckmann, T. et al. Barriers and facilitators affecting the implementation of substance use screening in primary care clinics: a qualitative study of patients, providers, and staff. Addict Sci Clin Pract 13 , 8 (2018). https://doi.org/10.1186/s13722-018-0110-8
Download citation
Received : 23 July 2017
Accepted : 30 January 2018
Published : 09 April 2018
DOI : https://doi.org/10.1186/s13722-018-0110-8
Share this article
Anyone you share the following link with will be able to read this content:
Sorry, a shareable link is not currently available for this article.
Provided by the Springer Nature SharedIt content-sharing initiative
- Substance use disorders
- Drug abuse screening
- Alcohol screening
- Alcohol abuse
- Primary care
- Qualitative research
Addiction Science & Clinical Practice
ISSN: 1940-0640
- Submission enquiries: [email protected]
- General enquiries: [email protected]
Advertisement
Dweck’s Mindset Theory Applied to Addictions: a Scoping Review
- Emotion and Addiction (K Morie, Section Editor)
- Published: 19 July 2022
- Volume 9 , pages 133–150, ( 2022 )
Cite this article
- Sacha Parada ORCID: orcid.org/0000-0003-2628-0293 1 ,
- Eve Legrand 1 ,
- Elsa Taschini 2 ,
- Xavier Laqueille 2 &
- Jean-François Verlhiac 1
1195 Accesses
3 Citations
Explore all metrics
Purpose of review
Mindset theory has been applied to a wide range of educational and health topics, and more recently, to addictions. The present preregistered scoping review was conducted, following the PRISMA-ScR guidelines, in order to answer three questions: (1) To what extent has mindset theory literature been linked to addiction research (including substance addiction and behavioral addiction)? (2) What interventional tools based on mindset theory have been used, in this context, in order to improve patients’ health, and to what effects? (3) What gaps and limitations exist in today’s literature, and where to guide future research in order to properly assess the effects of mindset theory on addictions?
Recent findings
Database search included APA PsychINFO and PubMed, and a manual search was also conducted. A total of 1903 documents were reviewed. Screening processes resulted in the inclusion of 18 articles. Analysis revealed that the field is still in a nascent state. More research is needed in order to properly evaluate the effect of mindset theory on addictions. Mindset interventions and manipulations have seldom been implemented. Most studies used cross-sectional designs, preventing from making causal inferences. Studies were also limited by the use of self-report data. Questions were raised concerning the hypothesis of similarity and specificity between mindset and addiction, and the place of mindset theory within known addiction frameworks.
The present scoping review points out that more research, using intervention procedure and objective data collection, should be conducted in order to properly assess the impact of mindset theory on addictions.
This is a preview of subscription content, log in via an institution to check access.
Access this article
Price includes VAT (Russian Federation)
Instant access to the full article PDF.
Rent this article via DeepDyve
Institutional subscriptions
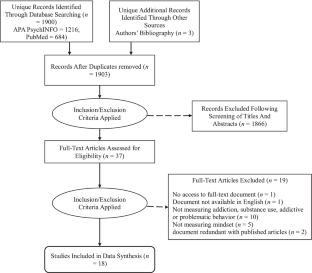
Similar content being viewed by others
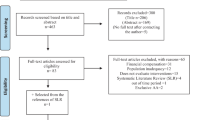
Structured programs for the self-management of substance addiction consequences in outpatient services: A scoping review
Paulo Seabra, Gabriella Boska, … Carlos Sequeira
Motivational Interviewing, Behaviour Change in Addiction Treatment
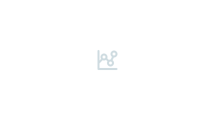
Addiction and Volitional Abilities: Stakeholders’ Understandings and their Ethical and Practical Implications
Marianne Rochette, Matthew Valiquette, … Eric Racine
Data availability
Data regarding this review (i.e., Zotero Database) is available at: https://osf.io/35fa6/?view_only=dba2a59fa0a44e3e9c36ebaeb38f608c
Papers of particular interest, published recently, have been highlighted as: • Of importance
Dweck CS. Mindsets and human nature: promoting change in the Middle East, the schoolyard, the racial divide, and willpower. Am Psychol. 2012;67(8):614–22. https://doi.org/10.1037/a0029783 .
Article PubMed Google Scholar
Dweck CS, Leggett EL. A social-cognitive approach to motivation and personality. Psychol Rev. 1988;95(2):256–73. https://doi.org/10.1037/0033-295X.95.2.256 .
Article Google Scholar
Yeager DS, Hanselman P, Walton GM, Murray JS, Crosnoe R, Muller C, Tipton E, Schneider B, Hulleman CS, Hinojosa CP, Paunesku D, Romero C, Flint K, Roberts A, Trott J, Iachan R, Buontempo J, Yang SM, Carvalho CM, … Dweck CS. A national experiment reveals where a growth mindset improves achievement. Nature , 2019; 573 (7774), 364–369. https://doi.org/10.1038/s41586-019-1466-y
Burnette JL. Implicit theories of body weight: entity beliefs can weigh you down. Pers Soc Psychol Bull. 2010;36(3):410–22. https://doi.org/10.1177/0146167209359768 .
Burnette JL, Finkel EJ. Buffering against weight gain following dieting setbacks: an implicit theory intervention. J Exp Soc Psychol. 2012;48(3):721–5. https://doi.org/10.1016/j.jesp.2011.12.020 .
Schleider J, Weisz J. A single-session growth mindset intervention for adolescent anxiety and depression: 9-month outcomes of a randomized trial. J Child Psychol Psychiatry. 2018;59(2):160–70. https://doi.org/10.1111/jcpp.12811 .
Burnette JL, Knouse LE, Vavra DT, O’Boyle E, Brooks MA. Growth mindsets and psychological distress: a meta-analysis. Clin Psychol Rev. 2020;77:101816. https://doi.org/10.1016/j.cpr.2020.101816 .
• Burnette JL, Forsyth RB, Desmarais SL, Hoyt CL. Mindsets of addiction: implications for treatment intentions. J Soc Clin Psychol. 2019;38(5):367–94. https://doi.org/10.1521/jscp.2019.38.5.367 . This study is one of the first to properly develop the rationale between mindset theory and addiction and to propose a mindset manipulation in relation with addiction outcomes .
Sridharan V, Shoda Y, Heffner JL, Bricker J. Addiction mindsets and psychological processes of quitting smoking. Subst Use Misuse. 2019;54(7):1086–95. https://doi.org/10.1080/10826084.2018.1555259 .
Article PubMed PubMed Central Google Scholar
Wang C, Luo J, Nie P, Wang D. Growth mindset can reduce the adverse effect of substance use on adolescent reasoning. Front Psychol. 2019;10. https://doi.org/10.3389/fpsyg.2019.01852 .
Chatters R, Cooper K, Day E, Knight M, Lagundoye O, Wong R, Kaltenthaler E. Psychological and psychosocial interventions for cannabis cessation in adults: a systematic review. Addiction Research & Theory. 2016;24(2):93–110. https://doi.org/10.3109/16066359.2015.1073719 .
Sileo KM, Miller AP, Wagman JA, Kiene SM. Psychosocial interventions for reducing alcohol consumption in sub-Saharan African settings: a systematic review and meta-analysis. Addiction. 2021;116(3):457–73. https://doi.org/10.1111/add.15227 .
Crescenzo FD, Ciabattini M, D’Alò GL, Giorgi RD, Giovane CD, Cassar C, Janiri L, Clark N, Ostacher MJ, Cipriani A. Comparative efficacy and acceptability of psychosocial interventions for individuals with cocaine and amphetamine addiction: a systematic review and network meta-analysis. PLoS Med. 2018;15(12):e1002715. https://doi.org/10.1371/journal.pmed.1002715 .
Quilty LC, Wardell JD, Thiruchselvam T, Keough MT, Hendershot CS. Brief interventions for problem gambling: a meta-analysis. PLoS ONE. 2019;14(4):1–17. https://doi.org/10.1371/journal.pone.0214502 .
Article CAS Google Scholar
Wild TC, Hammal F, Hancock M, Bartlett NT, Gladwin KK, Adams D, Loverock A, Hodgins DC. Forty-eight years of research on psychosocial interventions in the treatment of opioid use disorder: a scoping review. Drug Alcohol Depend. 2021;218:108434. https://doi.org/10.1016/j.drugalcdep.2020.108434 .
Article CAS PubMed Google Scholar
American Psychiatric Association (2013) Diagnostic and statistical manual of mental disorders (5th ed.). https://doi.org/10.1176/appi.books.9780890425596
Grant JE, Chamberlain SR. Expanding the definition of addiction: DSM-5 vs. ICD-11. CNS Spectrums. 2016;21(4):300–3. https://doi.org/10.1017/S1092852916000183 .
Momen NC, Plana-Ripoll O, Agerbo E, Benros ME, Børglum AD, Christensen MK, Dalsgaard S, Degenhardt L, Jonge de P, Debost J-CPG, Fenger-Grøn M, Gunn JM, Iburg KM, Kessing LV, Kessler RC, Laursen TM, Lim CCW, Mors O, Mortensen PB … McGrath JJ. Association between mental disorders and subsequent medical conditions. N Engl J Med. 2020; https://doi.org/10.1056/NEJMoa1915784 .
Plana-Ripoll O, Pedersen CB, Holtz Y, Benros ME, Dalsgaard S, Jonge de P, Fan CC, Degenhardt L, Ganna A, Greve AN, Gunn J, Iburg KM, Kessing LV, Lee BK, Lim CCW, Mors O, Nordentoft M, Prior A, Roest AM … McGrath JJ. Exploring comorbidity within mental disorders among a Danish national population. JAMA Psychiat. 2019; 76(3) 259-270. https://doi.org/10.1001/jamapsychiatry.2018.3658 .
Santucci K. Psychiatric disease and drug abuse. Curr Opin Pediatr. 2012;24(2):233–7. https://doi.org/10.1097/MOP.0b013e3283504fbf .
Lim SS, Vos T, Flaxman AD, Danaei G, Shibuya K, Adair-Rohani H, Amann M, Anderson HR, Andrews KG, Aryee M, Atkinson C, Bacchus LJ, Bahalim AN, Balakrishnan K, Balmes J, Barker-Collo S, Baxter A, Bell ML, Blore JD … Memish ZA. A comparative risk assessment of burden of disease and injury attributable to 67 risk factors and risk factor clusters in 21 regions, 1990–2010: a systematic analysis for the Global Burden of Disease Study 2010. Lancet (London, England). 2012;380(9859): 2224-2260. https://doi.org/10.1016/S0140-6736(12)61766-8 .
Peacock A, Leung J, Larney S, Colledge S, Hickman M, Rehm J, Giovino GA, West R, Hall W, Griffiths P, Ali R, Gowing L, Marsden J, Ferrari AJ, Grebely J, Farrell M, Degenhardt L. Global statistics on alcohol, tobacco and illicit drug use: 2017 status report. Addiction. 2018;113(10):1905–26. https://doi.org/10.1111/add.14234 .
West R, McEwen A, Bolling K, Owen L. Smoking cessation and smoking patterns in the general population: a 1-year follow-up. Addiction (Abingdon, England). 2001;96(6):891–902. https://doi.org/10.1046/j.1360-0443.2001.96689110.x .
Holden C. ‘Behavioral’ addictions: do they exist? Science. 2001;294(5544):980–2. https://doi.org/10.1126/science.294.5544.980 .
Marks I. Behavioural (non-chemical) addictions. Br J Addict. 1990;85(11):1389–94. https://doi.org/10.1111/j.1360-0443.1990.tb01618.x .
• Shaffer, H. J., & Shaffer, P. M. (2016). Behavioral addiction. In Encyclopedia of Mental Health (pp. 135–142). Elsevier. https://doi.org/10.1016/B978-0-12-397045-9.00077-X This paper argues for the inclusion of a broad range of problematic behaviors as behavioral addictions .
Hyman SE, Malenka RC, Nestler EJ. Neural mechanisms of addiction: the role of reward-related learning and memory. Annu Rev Neurosci. 2006;29(1):565–98. https://doi.org/10.1146/annurev.neuro.29.051605.113009 .
Ujhelyi Gomez K, Goodwin L, Jackson L, Jones A, Chisholm A, Rose AK. Are psychosocial interventions effective in reducing alcohol consumption during pregnancy and motherhood? A systematic review and meta-analysis. Addiction. 2021;116(7):1638–63. https://doi.org/10.1111/add.15296 .
Sisk VF, Burgoyne AP, Sun J, Butler JL, Macnamara BN. To what extent and under which circumstances are growth mind-sets important to academic achievement? Two meta-analyses. Psychological Science. 2018;29(4):549–71. https://doi.org/10.1177/0956797617739704 .
Blackwell LS, Trzesniewski KH, Dweck CS. Implicit theories of intelligence predict achievement across an adolescent transition: a longitudinal study and an intervention. Child Dev. 2007;78(1):246–63. https://doi.org/10.1111/j.1467-8624.2007.00995.x .
Burnette JL, O’Boyle EH, VanEpps EM, Pollack JM, Finkel EJ. Mind-sets matter: a meta-analytic review of implicit theories and self-regulation. Psychol Bull. 2013;139(3):655–701. https://doi.org/10.1037/a0029531 .
Claro S, Paunesku D, Dweck CS. Growth mindset tempers the effects of poverty on academic achievement. Proc Natl Acad Sci. 2016;113(31):8664–8. https://doi.org/10.1073/pnas.1608207113 .
Article CAS PubMed PubMed Central Google Scholar
Yeager DS, Johnson R, Spitzer BJ, Trzesniewski KH, Powers J, Dweck CS. The far-reaching effects of believing people can change: implicit theories of personality shape stress, health, and achievement during adolescence. J Pers Soc Psychol. 2014;106(6):867–84. https://doi.org/10.1037/a0036335 .
Parada S, Verlhiac J-F. Growth mindset intervention among French university students, and its articulation with proactive coping strategies. Educ Psychol. 2021;1–20. https://doi.org/10.1080/01443410.2021.1917519 .
• Sridharan V, Shoda Y, Heffner J, Bricker J. A pilot randomized controlled trial of a web-based growth mindset intervention to enhance the effectiveness of a smartphone app for smoking cessation. JMIR Mhealth Uhealth. 2019;7(7):e14602. https://doi.org/10.2196/14602 . This study is one of the first to propose a growth mindset intervention and assess its effects on addiction outcomes .
Michie S, Richardson M, Johnston M, Abraham C, Francis J, Hardeman W, Eccles MP, Cane J, Wood CE. The behavior change technique taxonomy (v1) of 93 hierarchically clustered techniques: building an international consensus for the reporting of behavior change interventions. Ann Behav Med. 2013;46(1):81–95. https://doi.org/10.1007/s12160-013-9486-6 .
Tricco AC, Lillie E, Zarin W, O’Brien KK, Colquhoun H, Levac D, Moher D, Peters MDJ, Horsley T, Weeks L, Hempel S, Akl EA, Chang C, McGowan J, Stewart L, Hartling L, Aldcroft A, Wilson MG, Garritty C … Straus SE. PRISMA extension for scoping reviews (PRISMA-ScR): checklist and explanation. Ann Intern Med. 2018;169(7)467-473. https://doi.org/10.7326/M18-0850 .
Arksey H, O’Malley L. Scoping studies: towards a methodological framework. Int J Soc Res Methodol. 2005;8(1):19–32. https://doi.org/10.1080/1364557032000119616 .
Levac D, Colquhoun H, O’Brien KK. Scoping studies: advancing the methodology. Implement Sci. 2010;5(1):69. https://doi.org/10.1186/1748-5908-5-69 .
Lindgren KP, Burnette JL, Hoyt CL, Peterson KP, Neighbors C. Growth mindsets of alcoholism buffer against deleterious effects of drinking identity on problem drinking over time. Alcohol Clin Exp Res. 2020;44(1):233–43. https://doi.org/10.1111/acer.14237 .
Thai CL, Rice EL, Taber JM, Kaufman AR, Klein WMP. Implicit theories of smoking and association with interest in quitting among current smokers. J Behav Med. 2020;43(4):544–52. https://doi.org/10.1007/s10865-019-00058-9 .
Bőthe B, Baumgartner C, Schaub MP, Demetrovics Z, Orosz G. Hands-off: feasibility and preliminary results of a two-armed randomized controlled trial of a web-based self-help tool to reduce problematic pornography use. J Behav Addict, 2021;1(aop). https://doi.org/10.1556/2006.2021.00070 .
Bőthe B, Tóth-Király I, Demetrovics Z, Orosz G. The pervasive role of sex mindset: beliefs about the malleability of sexual life is linked to higher levels of relationship satisfaction and sexual satisfaction and lower levels of problematic pornography use. Pers Individ Differ. 2017;117:15-22.psyh. https://doi.org/10.1016/j.paid.2017.05.030 .
Champagne J, Gardner B, Dommett EJ. Modelling predictors of UK undergraduates’ attitudes towards smart drugs. Trends in Neuroscience and Education. 2019;14:33–9. https://doi.org/10.1016/j.tine.2019.02.001 .
Fitz CC. Thinking it can get better makes it better: lay theories of prejudice and substance use willingness among undergraduate students of color (2015–99020–350; Issues 7-B(E)) 2015 [ProQuest Information & Learning]. psyh. https://search.ebscohost.com/login.aspx?direct=true&db=psyh&AN=2015-99020-350&lang=fr&site=ehost-live . Accessed 7 Feb 2022.
Fitz CC, Kaufman A, Moore PJ. Lay theories of smoking and young adult nonsmokers’ and smokers’ smoking expectations. J Health Psychol. 2015;20(4):438–45. https://doi.org/10.1177/1359105313502694 .
Grand AM. Implicit beliefs about the malleability of substance abuse: implications for treatment motivation and outcome (2001–95006–085; Issues 9-B) 2001 [ProQuest Information & Learning]. psyh. https://search.ebscohost.com/login.aspx?direct=true&db=psyh&AN=2001-95006-085&lang=fr&site=ehost-live . Accessed 7 Feb 2022.
Japutra A, Song Z. Mindsets, shopping motivations and compulsive buying: insights from China. J Consum Behav. 2020. https://doi.org/10.1002/cb.1821 .
Johnson VD. Growth mindset as a predictor of smoking cessation (2010–99011–153; Issues 7-A) 2010 [ProQuest Information & Learning]. Psyh. https://search.ebscohost.com/login.aspx?direct=true&db=psyh&AN=2010-99011-153&lang=fr&site=ehost-live . Accessed 7 Feb 2022.
Lindgren KP, Baldwin SA, Ramirez JJ, Olin CC, Peterson KP, Wiers RW, Teachman BA, Norris J, Kaysen D, Neighbors C. Self-control, implicit alcohol associations, and the (lack of) prediction of consumption in an alcohol taste test with college student heavy episodic drinkers. PLoS ONE. 2019;14(1):e0209940. https://doi.org/10.1371/journal.pone.0209940 .
Schroder HS, Dawood S, Yalch MM, Donnellan MB, Moser JS. Evaluating the domain specificity of mental health–related mind-sets. Social Psychological and Personality Science. 2016;7(6):508–20. https://doi.org/10.1177/1948550616644657 .
Thai CL, Coa KI, Kaufman AR. Implicit theories of smoking and association with current smoking status. J Health Psychol. 2018;23(9):1234–9. https://doi.org/10.1177/1359105316648483 .
Zeldman A, Ryan RM, Fiscella K. Motivation, autonomy support, and entity beliefs: their role in methadone maintenance treatment. J Soc Clin Psychol. 2004;23(5):675-696.psyh. https://doi.org/10.1521/jscp.23.5.675.50744 .
Paunesku D, Walton GM, Romero C, Smith EN, Yeager DS, Dweck CS. Mind-set interventions are a scalable treatment for academic underachievement. Psychol Sci. 2015;26(6):784–93. https://doi.org/10.1177/0956797615571017 .
Yeager DS, Romero C, Paunesku D, Hulleman CS, Schneider B, Hinojosa C, Lee HY, O’Brien J, Flint K, Roberts A, Trott J, Greene D, Walton GM, Dweck CS. Using design thinking to improve psychological interventions: the case of the growth mindset during the transition to high school. J Educ Psychol. 2016;108(3):374–91. https://doi.org/10.1037/edu0000098 .
Dweck CS (1999) Self-theories: Their role in motivation, personality, and development. Psychology Press
Dweck CS, Chiu C, Hong Y. Implicit theories and their role in judgments and reactions: a word from two perspectives. Psychol Inq. 1995;6(4):267–85. https://doi.org/10.1207/s15327965pli0604_1 .
McLellan AT, Kushner H, Metzger D, Peters R, Smith I, Grissom G, Pettinati H, Argeriou M. The fifth edition of the addiction severity index. J Subst Abuse Treat. 1992;9(3):199–213. https://doi.org/10.1016/0740-5472(92)90062-s .
Collins RL, Parks GA, Marlatt GA. Social determinants of alcohol consumption: the effects of social interaction and model status on the self-administration of alcohol. J Consult Clin Psychol. 1985;53(2):189–200. https://doi.org/10.1037/0022-006X.53.2.189 .
World Health Organization. AUDIT: the Alcohol Use Disorders Identification Test : guidelines for use in primary health care (WHO/MSD/MSB/01.6a). World Health Organization. 200 1. https://apps.who.int/iris/handle/10665/67205 . Accessed 14 Mar 2022.
White HR, Labouvie EW. Towards the assessment of adolescent problem drinking. J Stud Alcohol. 1989;50(1):30–7. https://doi.org/10.15288/jsa.1989.50.30 .
Pilkonis PA, Yu L, Colditz J, Dodds N, Johnston KL, Maihoefer C, Stover AM, Daley DC, McCarty D. Item banks for alcohol use from the patient-reported outcomes measurement information system (PROMIS®): use, consequences, and expectancies. Drug Alcohol Depend. 2013;130(1–3):167–77. https://doi.org/10.1016/j.drugalcdep.2012.11.002 .
Brown RL, Rounds LA. Conjoint screening questionnaires for alcohol and other drug abuse: criterion validity in a primary care practice. Wis Med J. 1995;94(3):135–40.
CAS PubMed Google Scholar
Siu AFY. Validation of the substance use risk profile scale for adolescents in Hong Kong. J Psychoeduc Assess. 2011;29(1):75–83. https://doi.org/10.1177/0734282910362044 .
Heatherton TF, Kozlowski LT, Frecker RC, Fagerström KO. The Fagerström test for nicotine dependence: a revision of the Fagerström tolerance questionnaire. Br J Addict. 1991;86(9):1119–27. https://doi.org/10.1111/j.1360-0443.1991.tb01879.x .
Bőthe B, Tóth-Király I, Zsila Á, Griffiths MD, Demetrovics Z, Orosz G. The development of the problematic pornography consumption scale (PPCS). J Sex Res. 2018;55(3):395–406. https://doi.org/10.1080/00224499.2017.1291798 .
Ridgway NM, Kukar-Kinney M, Monroe KB (2008) An expanded conceptualization and a new measure of compulsive buying . https://doi.org/10.1086/591108 .
Desmarais SL, Van Dorn RA, Sellers BG, Young MS, Swartz MS. Accuracy of self-report, biological tests, collateral reports and clinician ratings in identifying substance use disorders among adults with schizophrenia. Psychol Addict Behav. 2013;27(3):774–87. https://doi.org/10.1037/a0031256 .
Hughes JS. Support for the domain specificity of implicit beliefs about persons, intelligence, and morality. Personality Individ Differ. 2015;86:195–203. https://doi.org/10.1016/j.paid.2015.05.042 .
Ajzen I. Constructing a TpB questionnaire: conceptual and methodological considerations. Undefined. 2002 https://www.semanticscholar.org/paper/Constructing-a-TpB-Questionnaire%3A-Conceptual-and-Ajzen/6074b33b529ea56c175095872fa40798f8141867 . Accessed 17 Mar 2022.
Volkow ND, Koob GF, McLellan AT. Neurobiologic advances from the brain disease model of addiction. N Engl J Med. 2016;374(4):363–71. https://doi.org/10.1056/NEJMra1511480 .
Volkow ND, Koob G. Brain disease model of addiction: why is it so controversial? The Lancet Psychiatry. 2015;2(8):677–9. https://doi.org/10.1016/S2215-0366(15)00236-9 .
Heather N, Best D, Kawalek A, Field M, Lewis M, Rotgers F, Wiers RW, Heim D. Challenging the brain disease model of addiction: European launch of the addiction theory network. Addiction Research & Theory. 2018;26(4):249–55. https://doi.org/10.1080/16066359.2017.1399659 .
Leshner AI. Addiction is a brain disease, and it matters. Science (New York, NY). 1997;278(5335):45–7. https://doi.org/10.1126/science.278.5335.45 .
Satel S, Lilienfeld SO. Addiction and the brain-disease fallacy. Front Psych. 2014;4:141. https://doi.org/10.3389/fpsyt.2013.00141 .
Lebowitz MS, Appelbaum PS. Beneficial and detrimental effects of genetic explanations for addiction. Int J Soc Psychiatry. 2017;63(8):717–23. https://doi.org/10.1177/0020764017737573 .
Wiens TK, Walker LJ. The chronic disease concept of addiction: helpful or harmful? Addiction Research & Theory. 2015;23(4):309–21. https://doi.org/10.3109/16066359.2014.987760 .
Heyman GM, Mims V. What addicts can teach us about addiction: a natural history approach. In Addiction and Choice: Oxford University Press; 2016. https://doi.org/10.1093/acprof:oso/9780198727224.003.0021 .
Book Google Scholar
Kourgiantakis T, Ashcroft R. Family-focused practices in addictions: a scoping review protocol. BMJ Open. 2018;8(1):e019433. https://doi.org/10.1136/bmjopen-2017-019433 .
Download references
This research was supported by the National Cancer Institute ( Institut National du Cancer—INCa_16214 ).
Author information
Authors and affiliations.
Université Paris Nanterre, Nanterre, France
Sacha Parada, Eve Legrand & Jean-François Verlhiac
Hôpital Sainte-Anne, service d’addictologie, Paris, France
Elsa Taschini & Xavier Laqueille
You can also search for this author in PubMed Google Scholar
Contributions
Sacha Parada had the original idea for the article, Sacha Parada and Jean-François Verlhiac performed the literature search and data analysis, Sacha Parada drafted the article, Eve Legrand critically revised the work, Elsa Taschini, Xavier Laqueille and Jean-François Verlhiac reviewed and validated the final draft.
Corresponding author
Correspondence to Sacha Parada .
Ethics declarations
Ethical approval.
This research was exempted of the Ethics Committee Approval due to the nature of the paper (scoping review).
Conflict of Interest
The authors have no conflict of interest to declare. Authors certify that they have no affiliations with or involvement in any organization or entity with any financial interest or non-financial interest in the subject matter or materials discussed in this manuscript.
Preregistration
This study’s design and analysis plan were preregistered; see https://osf.io/m6fdt/
Additional information
Publisher's note.
Springer Nature remains neutral with regard to jurisdictional claims in published maps and institutional affiliations.
This article is part of the Topical Collection on Emotion and Addiction
Public health significance statements: This review found that mindset theory and interventions are increasingly being studied in relation to substance and behavior addiction. Results on addiction outcomes are encouraging, but more research is needed to fully assess their impact.
Rights and permissions
Reprints and permissions
About this article
Parada, S., Legrand, E., Taschini, E. et al. Dweck’s Mindset Theory Applied to Addictions: a Scoping Review. Curr Addict Rep 9 , 133–150 (2022). https://doi.org/10.1007/s40429-022-00427-6
Download citation
Accepted : 01 July 2022
Published : 19 July 2022
Issue Date : September 2022
DOI : https://doi.org/10.1007/s40429-022-00427-6
Share this article
Anyone you share the following link with will be able to read this content:
Sorry, a shareable link is not currently available for this article.
Provided by the Springer Nature SharedIt content-sharing initiative
- Mindset theory
- Behavioral addiction
- Scoping review
- Find a journal
- Publish with us
- Track your research
Methodological and Conceptual Limitations in Exercise Addiction Research
Affiliations.
- 1 Institute of Health Promotion and Sport Sciences, Eötvös Loránd University, Budapest, Hungary.
- 2 Psychology Division, Nottingham Trent University, Nottingham, United Kingdom.
- 3 Department of Physical Education, Sport & Human Movement, Autonomous University of Madrid, Campus Cantoblanco, Madrid, Spain.
- 4 Institute of Psychology, Eötvös Loránd University, Budapest, Hungary.
- PMID: 26339214
- PMCID: PMC4553651
The aim of this brief analytical review is to highlight and disentangle research dilemmas in the field of exercise addiction. Research examining exercise addiction is primarily based on self-reports, obtained by questionnaires (incorporating psychometrically validated instruments), and interviews, which provide a range of risk scores rather than diagnosis. Survey methodology indicates that the prevalence of risk for exercise addiction is approximately 3 percent among the exercising population. Several studies have reported a substantially greater prevalence of risk for exercise addiction in elite athletes compared to those who exercise for leisure. However, elite athletes may assign a different interpretation to the assessment tools than leisure exercisers. The present paper examines the: 1) discrepancies in the classification of exercise addiction; 2) inconsistent reporting of exercise addiction prevalence; and 3) varied interpretation of exercise addiction diagnostic tools. It is concluded that there is the need for consistent terminology, to follow-up results derived from exercise addiction instruments with interviews, and to follow a theory-driven rationale in this area of research.
Keywords: athlete; behavioral addiction; commitment to exercise; exerciser; methodology.
Publication types
- Behavior, Addictive / epidemiology*
- Behavior, Addictive / physiopathology*
- Biomedical Research / methods*
- Research Design*
- Sports Medicine / methods
- Terminology as Topic*
- Privacy Policy
Buy Me a Coffee

Home » Limitations in Research – Types, Examples and Writing Guide
Limitations in Research – Types, Examples and Writing Guide
Table of Contents


Limitations in Research
Limitations in research refer to the factors that may affect the results, conclusions , and generalizability of a study. These limitations can arise from various sources, such as the design of the study, the sampling methods used, the measurement tools employed, and the limitations of the data analysis techniques.
Types of Limitations in Research
Types of Limitations in Research are as follows:
Sample Size Limitations
This refers to the size of the group of people or subjects that are being studied. If the sample size is too small, then the results may not be representative of the population being studied. This can lead to a lack of generalizability of the results.
Time Limitations
Time limitations can be a constraint on the research process . This could mean that the study is unable to be conducted for a long enough period of time to observe the long-term effects of an intervention, or to collect enough data to draw accurate conclusions.
Selection Bias
This refers to a type of bias that can occur when the selection of participants in a study is not random. This can lead to a biased sample that is not representative of the population being studied.
Confounding Variables
Confounding variables are factors that can influence the outcome of a study, but are not being measured or controlled for. These can lead to inaccurate conclusions or a lack of clarity in the results.
Measurement Error
This refers to inaccuracies in the measurement of variables, such as using a faulty instrument or scale. This can lead to inaccurate results or a lack of validity in the study.
Ethical Limitations
Ethical limitations refer to the ethical constraints placed on research studies. For example, certain studies may not be allowed to be conducted due to ethical concerns, such as studies that involve harm to participants.
Examples of Limitations in Research
Some Examples of Limitations in Research are as follows:
Research Title: “The Effectiveness of Machine Learning Algorithms in Predicting Customer Behavior”
Limitations:
- The study only considered a limited number of machine learning algorithms and did not explore the effectiveness of other algorithms.
- The study used a specific dataset, which may not be representative of all customer behaviors or demographics.
- The study did not consider the potential ethical implications of using machine learning algorithms in predicting customer behavior.
Research Title: “The Impact of Online Learning on Student Performance in Computer Science Courses”
- The study was conducted during the COVID-19 pandemic, which may have affected the results due to the unique circumstances of remote learning.
- The study only included students from a single university, which may limit the generalizability of the findings to other institutions.
- The study did not consider the impact of individual differences, such as prior knowledge or motivation, on student performance in online learning environments.
Research Title: “The Effect of Gamification on User Engagement in Mobile Health Applications”
- The study only tested a specific gamification strategy and did not explore the effectiveness of other gamification techniques.
- The study relied on self-reported measures of user engagement, which may be subject to social desirability bias or measurement errors.
- The study only included a specific demographic group (e.g., young adults) and may not be generalizable to other populations with different preferences or needs.
How to Write Limitations in Research
When writing about the limitations of a research study, it is important to be honest and clear about the potential weaknesses of your work. Here are some tips for writing about limitations in research:
- Identify the limitations: Start by identifying the potential limitations of your research. These may include sample size, selection bias, measurement error, or other issues that could affect the validity and reliability of your findings.
- Be honest and objective: When describing the limitations of your research, be honest and objective. Do not try to minimize or downplay the limitations, but also do not exaggerate them. Be clear and concise in your description of the limitations.
- Provide context: It is important to provide context for the limitations of your research. For example, if your sample size was small, explain why this was the case and how it may have affected your results. Providing context can help readers understand the limitations in a broader context.
- Discuss implications : Discuss the implications of the limitations for your research findings. For example, if there was a selection bias in your sample, explain how this may have affected the generalizability of your findings. This can help readers understand the limitations in terms of their impact on the overall validity of your research.
- Provide suggestions for future research : Finally, provide suggestions for future research that can address the limitations of your study. This can help readers understand how your research fits into the broader field and can provide a roadmap for future studies.
Purpose of Limitations in Research
There are several purposes of limitations in research. Here are some of the most important ones:
- To acknowledge the boundaries of the study : Limitations help to define the scope of the research project and set realistic expectations for the findings. They can help to clarify what the study is not intended to address.
- To identify potential sources of bias: Limitations can help researchers identify potential sources of bias in their research design, data collection, or analysis. This can help to improve the validity and reliability of the findings.
- To provide opportunities for future research: Limitations can highlight areas for future research and suggest avenues for further exploration. This can help to advance knowledge in a particular field.
- To demonstrate transparency and accountability: By acknowledging the limitations of their research, researchers can demonstrate transparency and accountability to their readers, peers, and funders. This can help to build trust and credibility in the research community.
- To encourage critical thinking: Limitations can encourage readers to critically evaluate the study’s findings and consider alternative explanations or interpretations. This can help to promote a more nuanced and sophisticated understanding of the topic under investigation.
When to Write Limitations in Research
Limitations should be included in research when they help to provide a more complete understanding of the study’s results and implications. A limitation is any factor that could potentially impact the accuracy, reliability, or generalizability of the study’s findings.
It is important to identify and discuss limitations in research because doing so helps to ensure that the results are interpreted appropriately and that any conclusions drawn are supported by the available evidence. Limitations can also suggest areas for future research, highlight potential biases or confounding factors that may have affected the results, and provide context for the study’s findings.
Generally, limitations should be discussed in the conclusion section of a research paper or thesis, although they may also be mentioned in other sections, such as the introduction or methods. The specific limitations that are discussed will depend on the nature of the study, the research question being investigated, and the data that was collected.
Examples of limitations that might be discussed in research include sample size limitations, data collection methods, the validity and reliability of measures used, and potential biases or confounding factors that could have affected the results. It is important to note that limitations should not be used as a justification for poor research design or methodology, but rather as a way to enhance the understanding and interpretation of the study’s findings.
Importance of Limitations in Research
Here are some reasons why limitations are important in research:
- Enhances the credibility of research: Limitations highlight the potential weaknesses and threats to validity, which helps readers to understand the scope and boundaries of the study. This improves the credibility of research by acknowledging its limitations and providing a clear picture of what can and cannot be concluded from the study.
- Facilitates replication: By highlighting the limitations, researchers can provide detailed information about the study’s methodology, data collection, and analysis. This information helps other researchers to replicate the study and test the validity of the findings, which enhances the reliability of research.
- Guides future research : Limitations provide insights into areas for future research by identifying gaps or areas that require further investigation. This can help researchers to design more comprehensive and effective studies that build on existing knowledge.
- Provides a balanced view: Limitations help to provide a balanced view of the research by highlighting both strengths and weaknesses. This ensures that readers have a clear understanding of the study’s limitations and can make informed decisions about the generalizability and applicability of the findings.
Advantages of Limitations in Research
Here are some potential advantages of limitations in research:
- Focus : Limitations can help researchers focus their study on a specific area or population, which can make the research more relevant and useful.
- Realism : Limitations can make a study more realistic by reflecting the practical constraints and challenges of conducting research in the real world.
- Innovation : Limitations can spur researchers to be more innovative and creative in their research design and methodology, as they search for ways to work around the limitations.
- Rigor : Limitations can actually increase the rigor and credibility of a study, as researchers are forced to carefully consider the potential sources of bias and error, and address them to the best of their abilities.
- Generalizability : Limitations can actually improve the generalizability of a study by ensuring that it is not overly focused on a specific sample or situation, and that the results can be applied more broadly.
About the author
Muhammad Hassan
Researcher, Academic Writer, Web developer
You may also like

How to Cite Research Paper – All Formats and...

Data Collection – Methods Types and Examples

Delimitations in Research – Types, Examples and...

Research Paper Format – Types, Examples and...

Research Process – Steps, Examples and Tips

Research Design – Types, Methods and Examples
ORIGINAL RESEARCH article
Prevalence and related factors of sleep quality among chinese undergraduates in jiangsu province: multiple models' analysis.

- 1 Key Laboratory of Human Genetics and Environmental Medicine, School of Public Health, Xuzhou Medical University, Xuzhou, China
- 2 Department of Respiratory Diseases, The Affiliated Huai'an Hospital of Xuzhou Medical University, The Second People's Hospital of Huai'an, Huai'an, Jiangsu, China
- 3 Department of Dermatology, The Affiliated Huai'an Hospital of Xuzhou Medical University, The Second People's Hospital of Huai'an, Huai'an, Jiangsu, China
- 4 Department of Biostatistics, School of Public Health, Xuzhou Medical University, Xuzhou, China
- 5 Center for Medical Statistics and Data Analysis, Xuzhou Medical University, Xuzhou, China
Background and aims: In China, a significant number of undergraduates are experiencing poor sleep quality. This study was designed to investigate the prevalence of poor sleep quality and identify associated factors among undergraduates in Jiangsu Province, China.
Methods: A total of 8,457 participants were collected in 2022 using whole-group convenience sampling. The factors studied included basic demographics, family and social support, personal lifestyles, physical and mental health, mobile phone addiction index (MPAI), and the Connor-Davidson resilience scale (CD-RISC). The Pittsburgh Sleep Quality Index (PSQI) was used to assess sleep quality. Four models, including weighted multiple linear regression, binary logistic regression, weighted linear mixed model, and logistic regression with random effects, were applied to identify associated factors for sleep quality.
Results: Of the 8,457 participants analyzed, 26.64% (2,253) were classified into the poor sleep quality group with a PSQI score >7. No significant relationship was found between sleep quality and gender, native place, economic level of family, physical exercise, dormitory light, dormitory hygiene, and amativeness matter. Risk factors for sleep quality identified by the four models included lower CD-RISC, higher MPAI, fourth grade or above, smoking, drinking, greater academic pressure, greater employment pressure, roommate sleeping late, noisy dormitory, poorer physical health status, poorer mental health status, and psychological counseling.
Conclusions: These findings provide valuable insights for university administrators, enabling them to better understand the risk factors associated with poor sleep quality in undergraduates. By identifying these factors, administrators can provide targeted intervention measures and counseling programs to improve students' sleep quality.
Introduction
Sleep is a fundamental physiological process that is essential for our overall health. It is also important for our cognitive, emotional, and physical systems ( Baranwal et al., 2023 ). In recent years, sleep quality has declined among undergraduates and received attention from the public and academics ( Wang et al., 2016 ; Ahmed et al., 2020 ; Li Y. et al., 2020 ). Unfortunately, a significant number of undergraduates are experiencing poor sleep quality. In some provinces of China, the proportion of undergraduates with poor sleep quality has crossed more than 30.0%; for example, the proportions in Jilin, Guizhou, and Hong Kong were 33.8%, 53.7%, and 57.5%, respectively ( Suen et al., 2008 ; Li Y. et al., 2020 ; Zhou et al., 2022 ). Sleep quality among undergraduate students has also been poor in other countries such as Ethiopia (57.5%) and India (51.0%) ( Lemma et al., 2012 ; Ghrouz et al., 2019 ). Some studies focusing on medical students also found a high prevalence of poor sleep quality−52.4% in Greece during COVID-19 ( Eleftheriou et al., 2021 ), 76.0% in Saudi Arabia ( Almojali et al., 2017 ), and 27.8% in Inner Mongolia Medical University of China ( Wang et al., 2016 ). In Jiangsu Province, the status of sleep quality among undergraduates is still unknown, and it would be very interesting to investigate the prevalence of poor quality and identify the associated factors in this population.
Research has indicated that sleep deprivation can lead to metabolic disorders and negative effects, such as increased metabolite levels, which can lead to poor memory, poor concentration, lower academic performance, and emotional fluctuations ( Durmer and Dinges, 2005 ; Curcio et al., 2006 ; Basner et al., 2013 ; Davies et al., 2014 ; Lo et al., 2016 ; St-Onge, 2017 ; Gerhardsson et al., 2019 ; Vaccaro et al., 2020 ). In addition, poor sleep quality can also increase the risk of developing mental health problems such as anxiety and depression ( Almojali et al., 2017 ; Shao et al., 2020 ). Given the importance of sleep in maintaining wellbeing, it is essential to understand the factors that contribute to poor sleep quality among undergraduates. Additionally, it is also imperative to identify potential solutions to improve their sleep habits and reduce the negative effects.
Some studies have shown that sleep quality among Chinese undergraduates was a result of various factors. A cross-sectional study reported a few risk factors such as poor academic performance, interpersonal relationship, skipping breakfast, and higher grades ( Wang et al., 2016 ). Another study found that being a freshman, alcohol use, gambling, exercising for more than 30 min a week on <1 day, satisfaction with parental love, and harmonious relationship with classmates were risk factors, while no learning pressure, never having self-injurious behaviors, and harmonious family relationship were protective factors ( Li Y. et al., 2020 ). A gender-specific study in China identified some risk factors for poor sleep quality that were related to weak physical condition and smoking in males, while noisy dormitory, skipping breakfast, drinking coffee, playing games, bad physical condition, and severe academic stress led to poor sleep quality in females ( Zhou et al., 2022 ). A cluster randomized-controlled trial in China showed that good dormitory sleep environments could maintain good sleep quality ( Li et al., 2022 ). In addition, the use of electronic products was also related to poor sleep quality ( Demirci et al., 2015 ; Li L. et al., 2020 ). For example, using mobile phones, computers, and other electronic products at night could affect the regulation of the sleep clock, prolong the time to fall asleep, and shorten sleep time. Besides, there is a significant amount of similar research evaluating related factors for sleep quality ( Nyer et al., 2013 ; Bi et al., 2022 ; Peltz and Rogge, 2022 ; Xian et al., 2022 ). In summary, these factors that are associated with undergraduates' sleep quality can be classified into the following categories: demographics, personal lifestyle, family support, social support, dormitory environment, physical health, and mental health.
Because of the significant social and cultural diversity between different regions in China, previous findings may not fully represent the overall sleep characteristics of the Chinese population, especially undergraduates. Thus, our study not only aimed to examine the prevalence of poor sleep among undergraduates but also provide a comprehensive understanding of the factors that contribute to poor sleep quality among undergraduates in Jiangsu Province, China. The factors examined in this study also included mobile phone addiction and psychological resilience. By enhancing our understanding of these variables, this study may also help to develop effective strategies to improve sleep quality and ultimately promote undergraduates' life quality in China.
Special attention should be paid to the four statistical methods we utilized in association analysis. Except for the usual models of multiple linear regression and binary logistic regression, two mixed effect models were adopted such as linear mixed model and logistic random effect model. All these models are variations of the generalized linear mixed model ( Bolker et al., 2009 ; Stroup, 2013 ). The mixed model is also known as the hierarchical model and contains both fixed and random effects. The random effects model represents the variability between different conditions or blocks, and they are incorporated into the model to account for the correlation between observations. In the present study, the four regression models were conducted with standard diagnostics, ensuring more accurate parameters and p -values. Various studies have utilized regression models to identify risk factors associated with poor sleep quality, but few strictly performed regression diagnostics, which can potentially result in biased results.
Participants and explanatory variables
A whole-group convenience sampling was performed in universities of Jiangsu Province using an electronic questionnaire on the Wenjuanxing platform ( www.wjx.cn ) from October to November 2022. We distributed the link and QR code of the questionnaire to the undergraduates via university teachers and a WeChat group. The questionnaire contained survey instructions explaining the online survey's purpose and significance. Each undergraduate took the survey voluntarily and had the option to withdraw at any moment. The confidentiality of data and student personal information was guaranteed. To ensure the quality and accuracy of data collection, the questionnaire was pre-tested to validate question effectiveness and comprehensibility. The sample size was thoroughly calculated based on the expected prevalence rate of sleep disorders among college students, according to the sample size calculation formula: n = z α 2 × p q / d 2 . When the significance level is α = 0.05, z α = 1.96. Let p be the expected prevalence rate and q = 1− p . Based on the preliminary survey results of this study, the estimated detection rate of sleep disorders among undergraduates is p = 16%. The allowable error is d = 0.1 × p . The estimated sample size needed for the survey is 2017 individuals.
A total of 8,587 undergraduates attended the questionnaire voluntarily, among which 130 participants with incomplete information were excluded, leading to a response rate of 98.49%. So, the final sample consisted of 8,457 participants (5,917 females and 2,540 males). Participants were all students living on campuses and enrolled in 14 majors, including literature, history, philosophy, law, economics, management, education, science, engineering, agronomy, military, art, medicine, and others.
The questions in the questionnaire were organized into continuous, categorical, and ordinal variables (see Table 1 ). In categorical and ordinal variables, some values were merged to avoid extremely small sample sizes in some subgroups. Participants who never smoked were classified as not smoking and those who smoked occasionally or often were classified as smoking. Participants who had never drunk alcohol were classified as not drinking, and those who drank alcohol occasionally or often were classified as drinking.
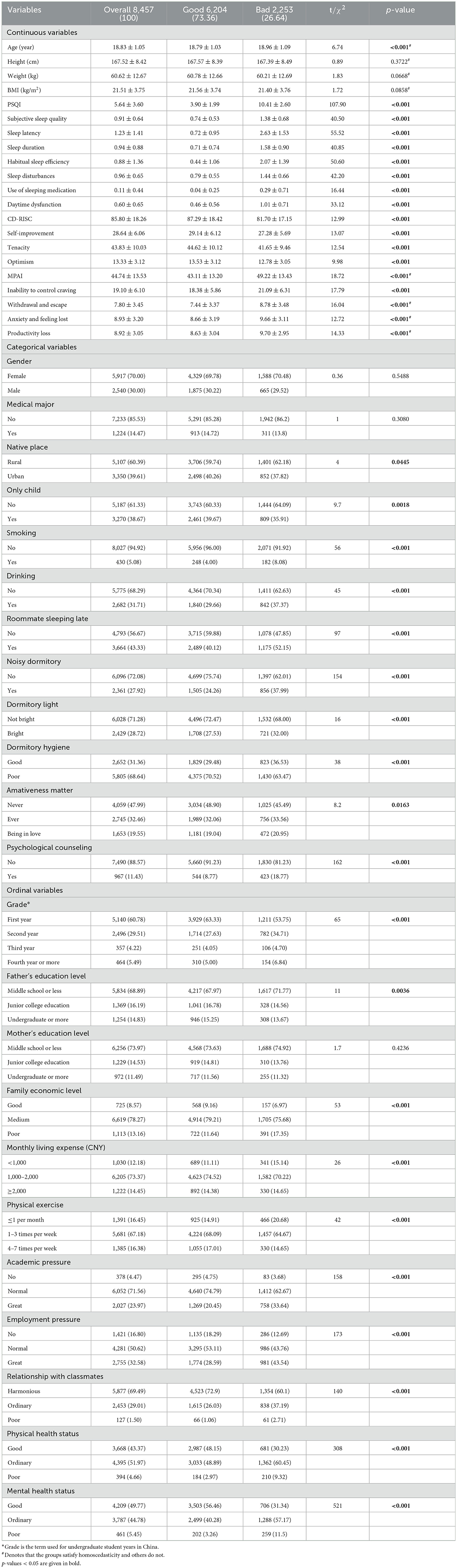
Table 1 . The prevalence of poor sleep quality across basic demographic characteristics.
Pittsburgh Sleep Quality Index
The Pittsburgh Sleep Quality Index (PSQI) ( Buysse et al., 1989 ; Chen et al., 1999 ) was adopted to assess the sleep quality of undergraduates by translating it into a Chinese version. PSQI includes 19 self-rated questions, and only the first 18 entries are used to calculate scores. These questions can be grouped into seven dimensions that can evaluate sleep quality in the last month, including subjective sleep quality, sleep latency, sleep duration, habitual sleep efficiency, sleep disturbances, use of sleeping medication, and daytime dysfunction. The final PSQI score was calculated by summing the scores from these seven dimensions. The total score ranges from 0 to 21 (0–3 for each component), with higher values indicating poor sleep quality. A final PSQI score of more than 7 indicates poor sleep quality and a score of <7 represents good sleep quality. For the PSQI conducted in this study, Cronbach's alpha was 0.877.
Mobile phone addiction index
The mobile phone addiction index (MPAI) ( Bianchi and Phillips, 2005 ), which was already translated into Chinese and validated for the Chinese population ( Li L. et al., 2020 ), was used to evaluate mobile phone addiction. The MPAI consists of 17 items quantifying four dimensions, including the inability to control craving, withdrawal and escape, anxiety and feeling lost, and productivity loss. Scores range from 1 (not at all) to 5 (always) for each item. The higher total scores indicate a higher level of mobile phone addiction. The Cronbach's alpha for the MPAI of the present study was 0.925.
Connor-Davidson resilience scale
The present study employed the Connor-Davidson resilience scale (CD-RISC) ( Connor and Davidson, 2003 ) to measure the psychological resilience of undergraduates, which reflects how the participants felt over the last month. This scale has been employed in various populations and has also been translated into Chinese ( Xu et al., 2022 ). The CD-RISC consists of 25 items, and each item's score ranges from 0 to 4, representing not true at all, rarely true, sometimes true, usually true, and true almost all the time, respectively. The final CD-RISC score was obtained by summing each score of the items, which ranged from 0 to 100, with higher scores indicating greater resilience. The Cronbach's alpha for the CD-RISC of the present study was 0.969.
Statistical analysis
The software R version 4.3.0 was used for statistical analysis. Extreme values of continuous variables were identified by the interquartile range (IQR) method. The upper and lower fences were defined as Q 3+2 IQR and Q 1 − 2 IQR . Values below (resp., above) the lower (resp., upper) fence were replaced with the lower (resp., upper) fence. For continuous variables, their central and variation trends were described by mean and standard deviation (SD), i.e., x ¯ ± s , and the means between two (resp., or more) groups were compared by t -test (resp. ANOVA) when homoscedasticity was satisfied, otherwise, Welch's t -test (resp., Welch's ANOVA) was employed. A post-hoc test was performed using Tukey's method when homoscedasticity was satisfied; otherwise, the Games-Howell test was performed. In this study, homoscedasticity was measured using Levene's test. The categorical and ordinal variables were described by frequency and constituent ratio, and the differences between groups were compared by Chi-square test. The significant level was set as 0.05.
Statistical models
Multiple linear regression model.
The associated factors for undergraduate sleep quality were identified using a linear regression model with the continuous variable PSQI score as the dependent variable, other variables as explanatory variables, and ordinal variables as continuous. The standard regression diagnostics ( Kabacoff, 2015 ) were processed after the multiple linear regression model (MLR) was implemented with the following steps: (a) Samples containing extreme values, including outliers, high leverage points, and influential points were removed. The outliers were detected if the Bonferroni adjusted p -value of the corresponding absolute studentized residuals was significant; the high leverage points were identified via the hat statistics; the influential points were determined by Cook's distance. In our model, 497 samples were removed in this step. (b) The independence of residuals was checked using the Durbin–Watson's test. In our model, independence was satisfied. (c) Multicollinearity between explanatory variables was detected through variance inflation factor (VIF). In our model, the VIF values of BMI, weight (kg), and height (cm) were all more than 4, which indicated that multicollinearity exists. Hence, weight and height were removed since BMI contains information about both. (d) The normality and homoscedasticity of residuals were checked. Our detection indicated that homoscedasticity was not satisfied. Therefore, weighted MLR was utilized to solve the heteroscedasticity problem, with weights inversely proportional to the variance of the dependent variable. The absolute values of the residuals were regressed against the fitted values, and the resulting fitted values were squared to provide the desired estimate of the variance. (e) The linearity between dependent variables and explanatory variables was checked. The performance of linearity, homoscedasticity, normality, and extreme values after diagnostics is shown in Supplementary Figure S1 . Visual inspection did not reveal any obvious deviations from linearity or normality. It is noteworthy that non-normality is less likely to be a problem when other assumptions are met since Aitken's theorem shows that the regression coefficients obtained from weighted MLR are also the best linear unbiased estimator without the assumption of normality ( Hansen, 2022 ). The linear trend test for a continuous variable was performed by transforming it into a four-valued ordinal variable by quartiles in the weighted MLR model.
Binary logistic regression model
Odds ratios (ORs) of factors associated with poor sleep quality were performed using a binary logistic regression model (BLR). The dependent variable was set as good (coded as 0) and poor (coded as 1) sleep quality based on whether the PSQI score was <7, and other variables were treated in the same way as MLR. The diagnostics ( Kabacoff, 2015 ) were processed with the following steps: (a) Extreme values were detected by the same method as MLR and 408 samples were removed. (b) Multicollinearity was detected by VIF, and weight and height were also excluded. (c) The independence of observations was performed using Durbin–Watson's test, and this assumption was satisfied. (d) The linearity between the log odds and explanatory variables was checked by regressing the log odds against the explanatory variables. The performance of linearity and extreme values after diagnostics is shown in Supplementary Figure S2 . (e) Overdispersion and events per variable (EPV) were checked ( Peduzzi et al., 1996 ). Overdispersion was accessed by the Chi-square test, but it did not exist in our model. There were 28 explanatory variables, including dummy variables, 1,897 samples with good sleep quality, and 6,152 samples with poor sleep quality. Thus, the EPV principle was satisfied. The ratio of these two outcome events was ~1:3; therefore, it can be considered as approximately balanced data. The linear trend test was performed in the same way as the aforementioned method.
Linear mixed model
The linear mixed model (LMM) is an extension of the MLR to allow both fixed and random effects, and it is particularly used in hierarchical analysis ( Stroup, 2013 ). The package “lmerTest” was adopted to implement LMM. In LMM, the continuous variable PSQI served as the dependent variable. The candidate random effect terms were checked by the likelihood ratio test. As random effects, we had intercepts for grade, smoking, drinking, physical health status, mental health status, academic pressure, employment pressure, and psychological counseling, as well as random slopes for the effect of relationship with classmates among academic pressure and employment pressure. Other variables, excluding weight and height due to multicollinearity, were set as fixed effects. No outliers were detected, and the homoscedasticity was violated. Therefore, the weighted LMM was adopted, and the weights were determined in the same way as the weighted MLR. The independence was checked using Durbin–Watson's test, and the results were satisfied. The performance of linearity, homoscedasticity, normality, and extreme values after diagnostics is shown in Supplementary Figure S3 . Visual inspection did not reveal any obvious deviations from linearity or normality.
Logistic regression with random effects
The logistic regression with random effects model (LRRE) is a type of generalized linear mixed model. The dependent variable was set as BLR. We had random intercepts for grade, smoking, drinking, physical health status, mental health status, academic pressure, employment pressure, and psychological counseling, as well as random slopes for the effect of the relationship with classmates against employment pressure and academic pressure. Other variables, excluding weight and height due to multicollinearity, were set as fixed effects. A likelihood ratio test was performed to check the significance of random effects. No outliers were detected. Independency, linearity, and overdispersion were checked in the same way as BLR, and all assumptions were satisfied. The performance of linearity after diagnostics is shown in Supplementary Figure S4 .
Prevalence of poor sleep quality
The Cronbach's alpha for the questionnaire of this study was 0.911, which indicates the internal consistency of the questionnaire was good. The sample consisted of 69.97% male and 30.03% female. Out of the 8,457 undergraduates, 6,204 (73.36%) reported having good sleep quality while 2,253 (26.64%) experienced poor sleep quality, based on the PQSI threshold value of 7. The mean PSQI score of all participants was 5.64 ± 3.60. The prevalence of poor sleep quality in undergraduates is shown in Table 1 , and the PSQI score across different characteristics is shown in Supplementary Table S1 . There was no significant difference between male (5,917) and female (2,540) students in height, weight, BMI, medical major, and non-medical major in terms of PSQI score or sleep quality. Older students and those in higher grades had a higher prevalence of poor sleep quality compared with their counterparts who were younger and in lower grades. The prevalence of poor sleep quality among students from rural areas, only-child families, and fathers with low education levels was nearly 30% higher than among students from urban areas, having siblings and fathers with high education levels. Although the PSQI scores of the three levels of mothers' education were significantly different via Welch's ANOVA, the post hoc result did not show the difference, and the constituent ratios between students with good and poor sleep quality were not significantly different. Approximately 80% of the students with either good or poor sleep quality belonged to families with medium economic levels. Similarly, more than 70% of the students with good or poor sleep quality reported a monthly living expense between 1,000 to 2,000 CNY. In terms of dormitory and personal lifestyle, the constituent ratios between students with good and poor sleep quality were all significantly different, and the corresponding PSQI scores were also significantly different. The worse these parameters were, the higher the prevalence of poor sleep quality.
Undergraduates with poor sleep quality had higher PSQI scores across all seven components compared to those with good sleep quality. The mean PSQI score for undergraduates with poor sleep quality was 10.41, which was significantly higher than their counterparts with good quality sleep ( p < 0.001). The mean scores of CD-RISC and its three components for students with poor sleep quality were all significantly lower than those with good sleep quality ( p < 0.001). The MPAI situation was the opposite.
Results of weighted MLR and BLR
The Akaike Information Criterion (AIC) of MLR and weighted MLR were 38,170.2 and 38,103.3, respectively, which indicates that weighted MLR improved the bias caused by heteroscedasticity in MLR. Table 2 presents the factors associated with PSQI scores, which were identified by weighted MLR, and the risk factors for poor sleep quality identified through BLR. The variables age, MPAI, grade, smoking, drinking, academic pressure, employment pressure, relationship with classmates, roommate sleeping late, noisy dormitory, physical health status, mental health status, and psychological counseling exhibited significant positive associations with the PSQI scores. On the other hand, BMI, CD-RISC, medical major, and father's education level demonstrated significant negative correlations with the PSQI scores. Barring BMI and relationship with classmates, the association between all other factors and PSQI was linear.
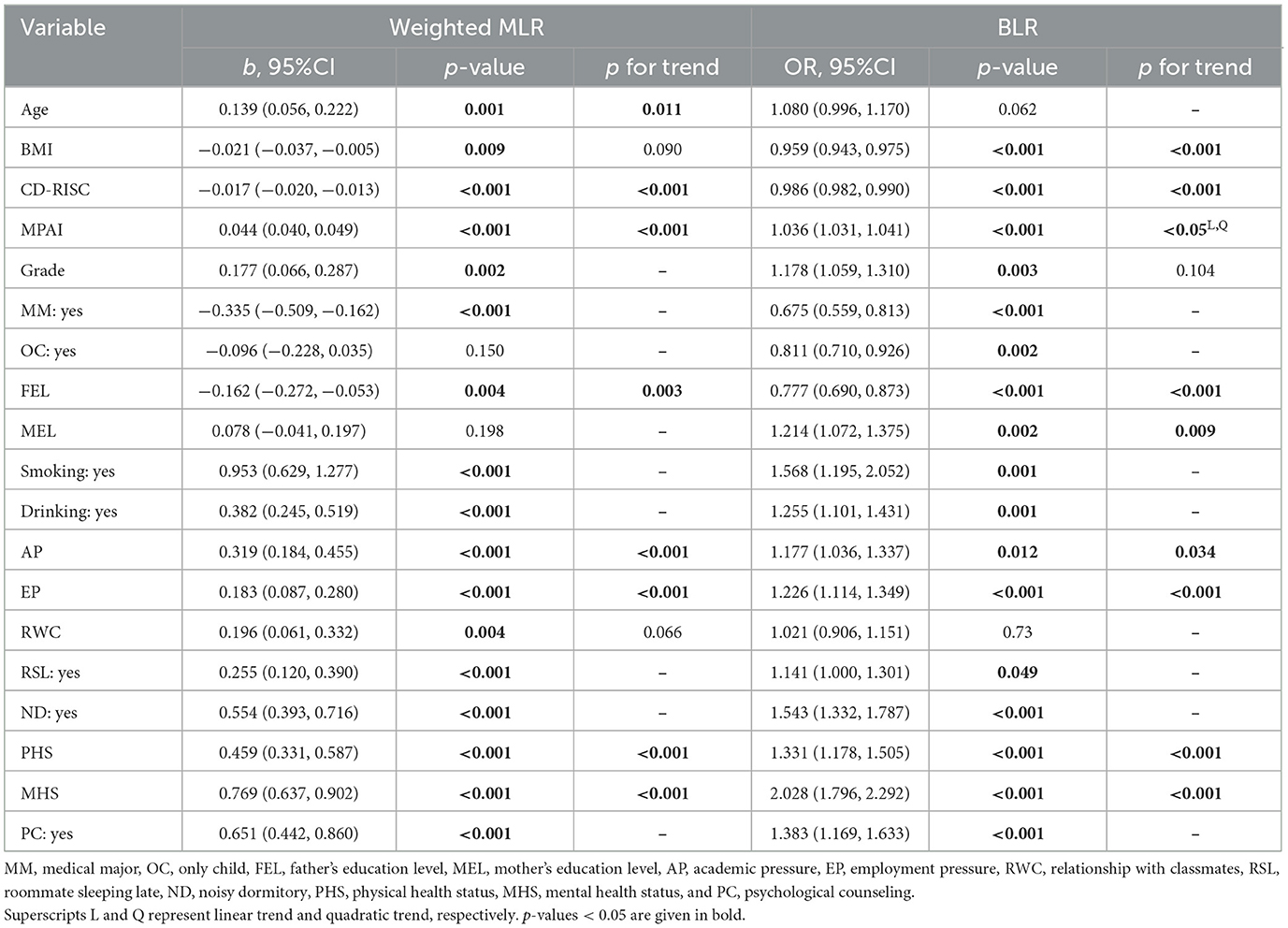
Table 2 . Risk factors for sleep quality identified by weighted MLR and BLR.
The results of BLR showed that students with higher BMI (OR = 0.959, p < 0.001), CD-RISC (OR = 0.986, p < 0.001), and father's education level (OR = 0.777, p < 0.001), had a decreased risk of poor sleep quality. Students with higher MPAI (OR = 1.036, p < 0.001), higher grades (OR = 1.178, p = 0.003), higher mother's education level (OR = 1.214, p = 0.002), greater academic pressure (OR = 1.177, p = 0.012), greater employment pressure (OR = 1.226, p < 0.001), worse physical health status (OR = 1.331, p < 0.001), and worse mental health status (OR = 2.028, p < 0.001) had an increased risk of poor sleep quality. Students who majored in medicine (OR = 0.675, p < 0.001) and were the only child in the family (OR = 0.811, p = 0.002) had a lower risk of poor sleep quality than those who did not major in medicine and were not the only child in the family. Smoking (OR = 1.568, p < 0.001), drinking (OR = 1.255, p < 0.001), and receiving psychological counseling (OR = 1.383, p < 0.001) were significantly associated with poor sleep quality. Roommates sleeping late (OR = 1.141, p = 0.049) and noisy dormitory (OR = 1.543, p < 0.001) were shown to be risk factors for poor sleep quality. The OR of mental health status was the highest, followed by smoking, noisy dormitory, psychological counseling, physical health status, and drinking. The sorted ORs of significant factors for undergraduate poor sleep quality can be found in Figure 1 . Linear trend test showed that MPAI had both significant linear and quadratic trends with respect to poor sleep quality, but no substantial distinction was observed between the two trends on visual inspection (see Supplementary Figure S4 ). Besides, there was also no linear trend between grade and poor sleep quality ( p = 0.104). The receiver operating characteristic (ROC) curve of BLR can be found in Supplementary Figure S5 , and the area under the curve (AUC) was 0.776.
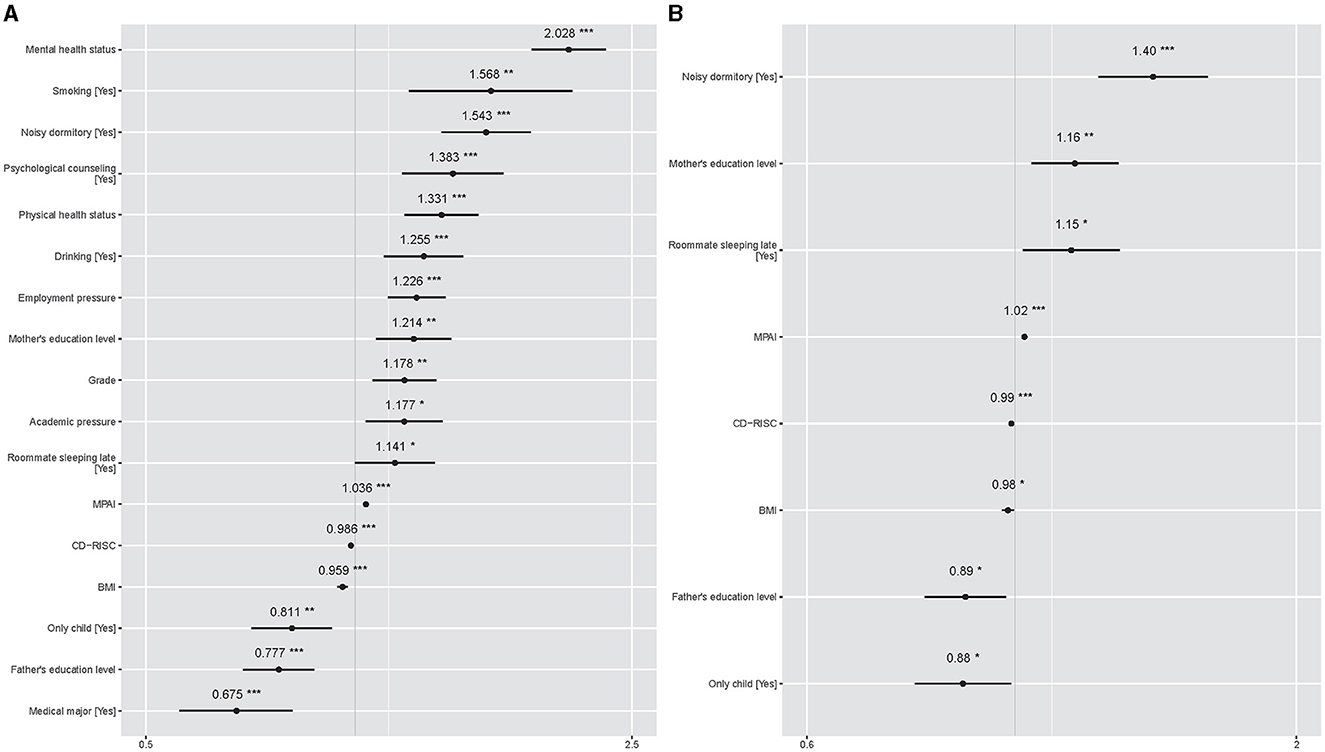
Figure 1 . Sorted ORs of significant factors for undergraduate poor sleep quality. The gray vertical solid line represents OR =1, and the short black horizontal solid line represents 95% CIs of ORs. Symbol * denotes p < 0.05, ** denotes p < 0.01, and *** denotes p < 0.001. (A) Comes from the results of BLR. (B) Comes results of LRRE.
Results of weighted LMM and LRRE
The AICs of LMM and weighted LMM were 43,857.1 and 43,554.2, respectively, which indicates that weighted LMM improved the bias caused by heteroscedasticity in LMM. Table 3 shows fixed and random effects of associated PSQI score factors identified by weighted LMM and related factors for poor sleep quality identified by LRRE. The intraclass correlation coefficients (ICCs) of the two models were 0.123 and 0.130, suggesting that 12.3% and 13.0% of the total variation in the responses were explained by subgroups. The fixed effects of age ( b = 0.142, p = 0.002) and MPAI ( b = 0.043, p < 0.001) exhibited a significant positive association with respect to PSQI scores, while the fixed effect of CD-RISC was the opposite ( b = −0.016, p < 0.001). Students with a roommate sleeping late ( b = 0.303, p < 0.001) and in a noisy dormitory ( b = 0.582, p < 0.001) had significantly higher PSQI scores at an average level compared to those without a roommate sleeping late and in a noisy dormitory. Except for age, all significant continuous factors displayed a linear trend with the PSQI score. However, an analysis of the trend graph between age and PSQI score revealed that the quadratic trend was approximately close to linearity (see Supplementary Figure S6 ). The random effects of weighted LMM explained 15.62% of the total variation. The detailed random effect values of weighted LMM can be found in Figure 2 . For random intercepts, being in the second and fourth grades, having poor mental and physical health status, receiving psychological counseling, smoking, and drinking had the effect of increasing PSQI scores. The random slopes of relationships with classmates varied across the three levels of employment pressure and academic pressure. However, the random effects of the relationship with classmates and employment pressure were not significant. In the subgroup of students without academic pressure, the intercept was −1.200, and the slope of the relationship with classmates was 0.812, suggesting that a poor relationship with classmates had a positive effect on increasing PSQI scores. In the subgroups of students with normal and great academic pressure, the intercepts were 0.496 and 0.704, respectively, indicating that these two subgroups had higher average PSQI scores compared to the subgroups of students without academic pressure. However, the slopes of relationship with classmates across the two subgroups were −0.458 and −0.353, demonstrating that a harmonious relationship with classmates had a positive effect on increasing the PSQI scores.
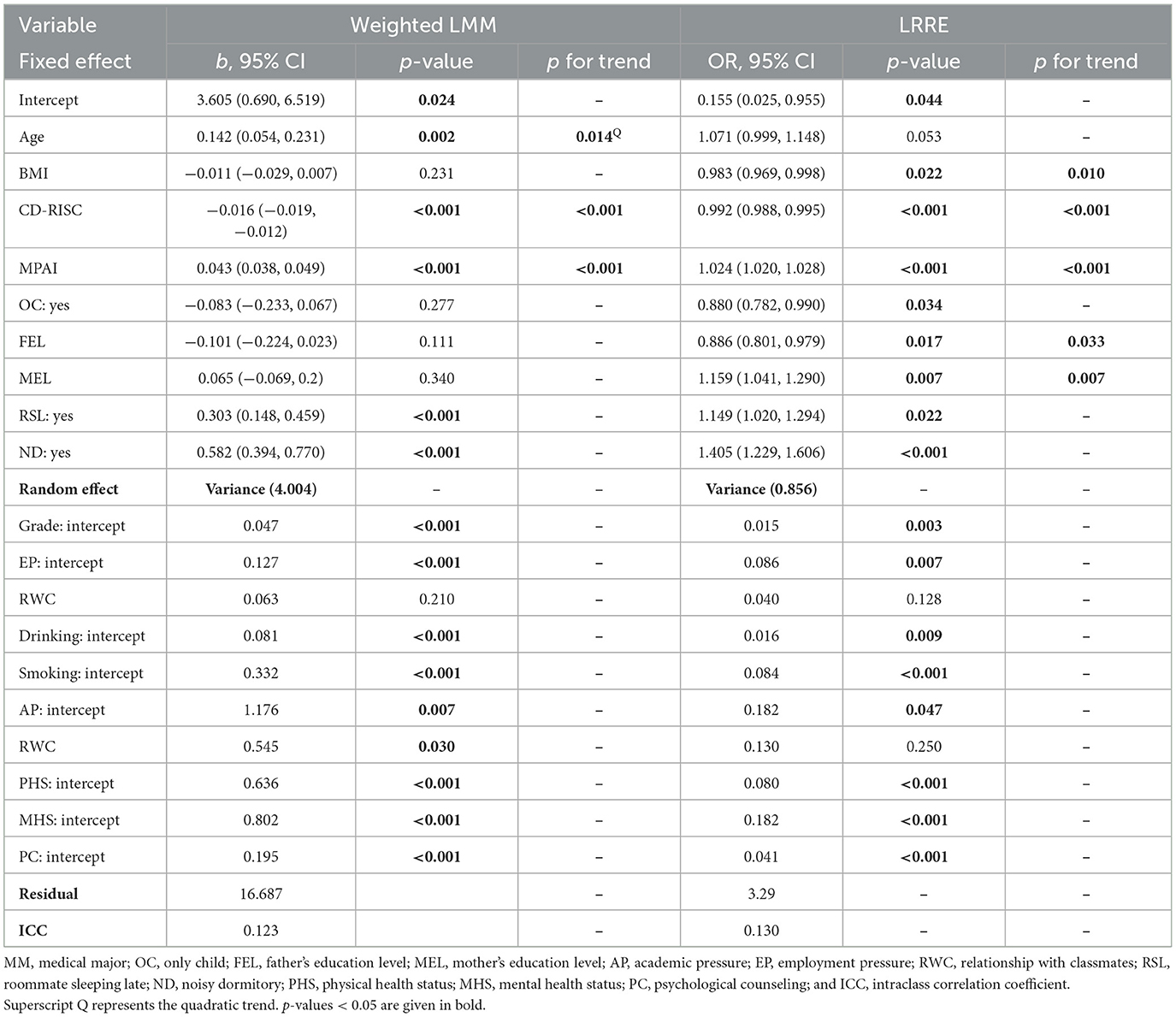
Table 3 . Fixed and random effects of factors for sleep quality by weighted LMM and LRRE.
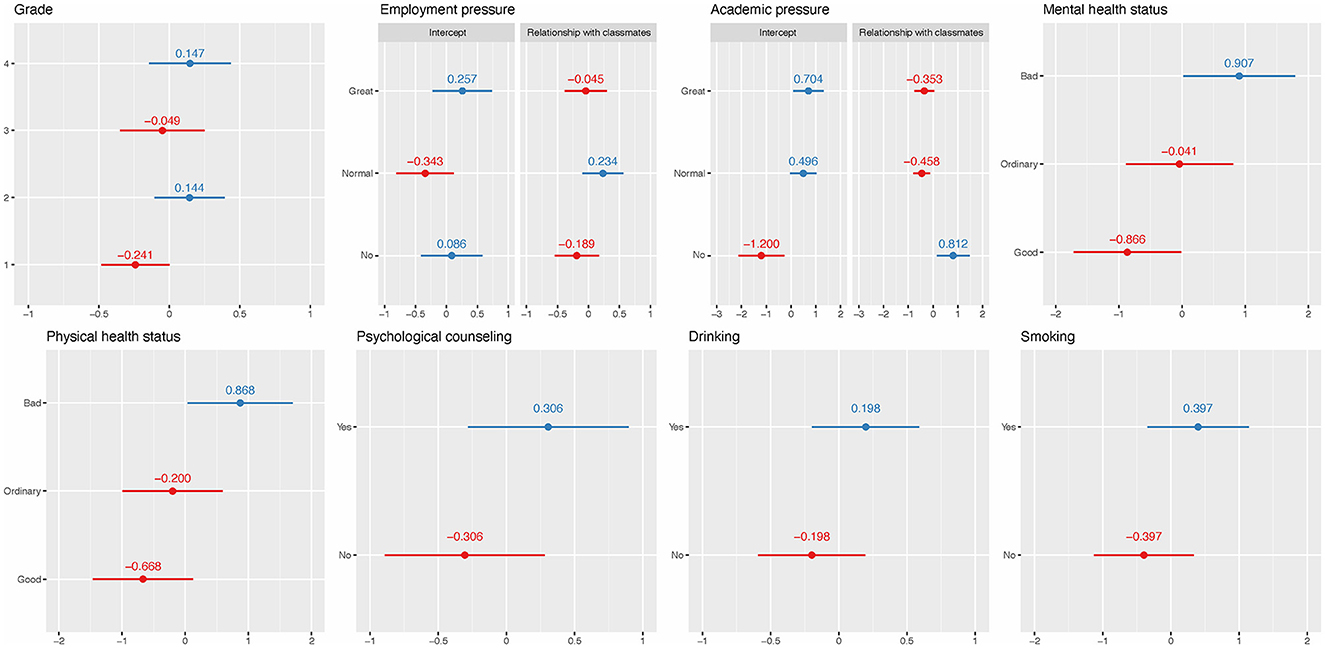
Figure 2 . Random effects of weighted LMM. The red and blue solid dots represent negative and positive effects on PSQI scores, respectively. The short horizontal solid lines represent 95% CI.
For the fixed effect results of LRRE, higher BMI (OR = 0.983, p = 0.022) and CD-RISC (OR = 0.992, p < 0.001) had a decreased risk of poor sleep quality. On the contrary, students with higher MPAI (OR = 1.024, p < 0.001) and mother's education level (OR = 1.159, p = 0.007) had an increased risk of poor sleep quality. Being the only child was a protective factor of poor sleep quality compared to not being the only child (OR = 0.88, p = 0.034). All significant continuous factors had a linear trend with respect to poor sleep quality. A higher risk was also found for students with roommates sleeping late (OR = 1.149, p = 0.022) and noisy dormitories (OR = 1.405, p < 0.001). These results are consistent with previous BLR results. In fixed effects, noisy dormitory had the highest OR, followed by mother's education level and roommates sleeping late. The sorted ORs can be found in Figure 1 . The random effects explained 20.65% of the total variation when the dataset was fitted by LRRE and their ORs, which can be found in Figure 3 . It was found that the variability in students' relationships with classmates (random slopes) did not significantly differ across the three levels of employment pressure and academic pressure. This suggests that students' relationships with classmates were consistent regardless of the degree of pressure experienced. The effects of most random intercepts were consistent with the corresponding counterpart of weighted LMM. Being in the second and fourth grades, experiencing “great” and “not having” employment pressure, poor and normal levels of mental health status, poor physical health status, receiving psychological counseling, smoking, and drinking had a positive effect on poor sleep quality. The ROC curve of LRRE can be found in Supplementary Figure S7 , and the AUC was 0.720.
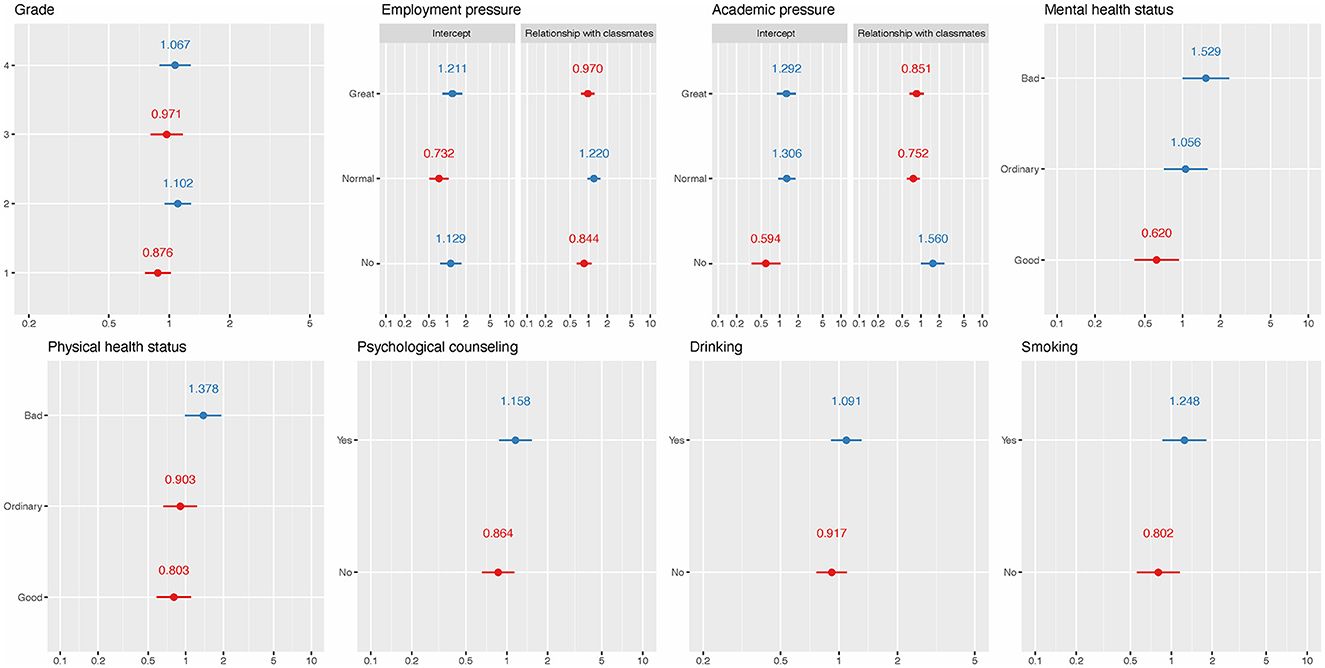
Figure 3 . ORs for random effects of LREE. The red and blue solid dots represent ORs that are <1 and >1, respectively. The short horizontal solid lines represent 95% CI.
This study investigated the prevalence and associated factors of sleep quality among undergraduates in Jiangsu Province, China. The results showed a mean PSQI score of 5.64 ± 3.60 and a prevalence of poor sleep quality of 26.64%, which is consistent with another study on medical college students in China, which showed the prevalence was 27.80% ( Wang et al., 2016 ) but lower than the results obtained in Ethiopia (55.8%) and Taiwan (33.80%) ( Kang and Chen, 2009 ; Lemma et al., 2012 ).
No significant association was found between gender and sleep quality through demographics and the four regression models. Similar results were reported in previous studies ( Lund et al., 2010 ; Wang et al., 2016 ; Li Y. et al., 2020 ). The study also found no significant correlation between sleep quality and native place, family economic level, physical exercise, dormitory light, dormitory hygiene, and amativeness matter through the four regression models. Previous studies ( Lund et al., 2010 ; Wang et al., 2016 ; Li Y. et al., 2020 ) also reported that there was no association between sleep quality and family economic level, physical exercise, and amativeness matter. Some studies showed that sleep quality might be influenced by native places ( Tang et al., 2017 ), but their cutoff values of PSQI score was not seven, and the population was not undergraduates. Our study found that there is no significant correlation between sleep quality and dormitory light brightness or dormitory hygiene; these factors were included in the cluster randomized-controlled trial described in research ( Li et al., 2022 ) to explore comprehensive environmental influences on sleep quality. The rationale for this inclusion is based on the hypothesis that environmental factors, beyond personal habits or demographic characteristics, have a significant impact on sleep quality. Although our findings and the intervention in the cited study did not demonstrate a direct impact of these factors on sleep quality, including them in the analysis will help to provide a holistic view of the environmental influences on sleep.
Since the dependent variable PSQI score in weighted MLR and weighted LMM was continuous, while the dependent variable in BLR and LRRE was dichotomous, the associated factors identified by the two types of models may differ. For example, age was significantly positively associated with PSQI score in weighted MLR and weighted LMM but was not significant in BLR and LRRE. Being the only child and mother's education level were significant in BLR and LRRE but were not in weighted MLR and weighted LMM. This may be because age can affect the PSQI score, but it has a limited impact on PSQI in the bounded range ( Basner et al., 2013 ; Demirci et al., 2015 ).
In the present study, the BLR and LRRE models identified significant associations between only child status and mother's education level with sleep quality. The effects of these factors appeared to be amplified in the dichotomous models (BLR and LRRE) than in the other models, suggesting that these variables may have a more sensitive response to categorical sleep quality measures. This phenomenon may reflect the complex relationship between these sociodemographic characteristics and individuals' daily behavioral patterns. Specifically, students who are the only child may receive more attention and resources within the family and also be subject to higher expectations and pressures from their parents, both of which may affect sleep quality.
Higher levels of maternal education may also imply better cognitive stimulation and family economic conditions, which indirectly affect the child's sleep status. On the other hand, these significant differences may be related to the family's socio-economic status and cultural background, which also affect undergraduates' performance in school or social activities and stress levels, and ultimately affect sleep quality. There was a difference in sleep quality between the only child and non-only child subgroups (see Table 1 ), but the difference in PSQI score was small (see Supplementary Table S1 ), resulting in the linear model not identifying it after adjusting for other factors. The same situation also applies to the mother's education level, in which the three levels had no difference in the PSQI score. BMI and the father's education levels were not significant in the weighted LMM but were significant in the other three models.
Relationship with classmates was significant in weighted MLR and had significant random slopes in weighted LMM but was not significant in BLR and LRRE. Some inconsistencies between weighted MLR and weighted LMM, BLR, and LRRE may be due to the different samples included. Three kinds of extreme values were removed from the weighted MLR and BLR, while LMM and LRRE had relatively few methods to detect extreme values. Weighted LMM and LRRE contained all samples since no extreme values were found in the diagnostic step.
The four regression models identified several common risk factors for sleep quality, including lower CD-RISC, higher MPAI, being in fourth grade or above, smoking, drinking, greater academic pressure, having no or great employment pressure, roommate sleeping late, noisy dormitory, poorer physical health status, poorer mental health status, and psychological counseling. Most of these results are consistent with previous studies. CD-RISC ( Bianchi and Phillips, 2005 ) measured the psychological resilience of undergraduates, and its negative association with CD-RISC was also reported by previous studies ( Li and Guo, 2023 ; Xie et al., 2023 ). A higher MPAI represents a higher level of mobile phone addiction. Mobile phone overuse may disrupt the sleep process and lead to depression. Furthermore, long-term exposure to blue light and electromagnetic fields emitted from the screen may affect melatonin levels and contribute to poor sleep quality ( Demirci et al., 2015 ). However, newer models of cell phones currently have the ability to turn off blue light, and this study did not investigate the condition with blue light turned off, so this finding may have limitations. A gender-specific study ( Zhou et al., 2022 ) found that smoking was a risk factor for poor sleep quality among males, and noisy dormitory and academic pressure were risk factors for poor sleep quality among females. A study on college students of Jilin Province ( Li Y. et al., 2020 ) found that drinking, academic pressure, and relationships with classmates were risk factors for poor sleep quality. A study on medical students ( Shao et al., 2020 ) showed that students with greater employment pressure had more anxiety symptoms, which may affect their sleep quality. A cluster randomized-controlled trial in China ( Li et al., 2022 ) showed that intervening with the sleep schedule of roommates can obtain a good sleep quality for them, suggesting that our findings of roommates sleeping late as a risk factor for poor sleep quality are consistent with it. However, there were some studies that considered low physical activity via the International Physical Activity Questionnaire-Short Form anxiety and depression scores via the Hospital Anxiety and Depression Scale as negative factors for sleep quality ( Ghrouz et al., 2019 ). Another study ( Nyer et al., 2013 ) found that students with depressive symptoms and sleep disturbance endorsed significantly more intense and frequent anxiety and poorer cognitive and physical functioning.
Being in a higher grade was a risk factor for sleep quality in weighted MLR and BLR, but only the random effects of being in the second grade and fourth grade or above were found to be positive in weighted LMM and GLMM, and the random effects of being in the first and third grades were negative. This may be due to the greater pressure faced by students in fourth grade or above, such as postgraduate entrance exams, employment, internships, and graduation thesis issues. Similarly, second grade was the year with the greatest learning pressure, and in addition to regular studies, they also needed to participate in various competitions and innovation training programs. The first grade was still in a transitional adaptation period from high school to college, while the third grade was a comfortable period after adapting to college life. The students in third grade were able to cope easily with previous pressure, and there was no new pressure similar to those in the fourth grade.
In the weighted LMM result, the slope of the relationship with classmates was positive, i.e., 0.812, in the subgroup of having no academic pressure, indicating that a poorer relationship with classmates meant a higher PSQI score. The corresponding slopes were negative, i.e., −0.458 and −0.353, in the subgroups of normal and great academic pressure, respectively, indicating that a poorer relationship with classmates meant a lower PSQI score. This may be because students who have good relationships with classmates need to spend more time dealing with communication or other issues between classmates, resulting in less rest time and high pressure. We recognize that this interpretation is speculative and based on observations from the current data set. Therefore, more comprehensive research is necessary to explore and test this hypothesis. Therefore, we call for further research to explore the complexity of the interplay between social stress, social relationships, and sleep quality.
In the weighted LMM result, the random intercept of having no employment pressure was 0.086, and its corresponding OR in the LRRE result was more than 1, which indicates that having no employment pressure had the effect of increasing the risk of poor sleep quality. This may be caused by the fact that the sample size of students with poor sleep quality and having no employment pressure was extremely low, i.e., 286. Thus, the model may not show the true effect of having no employment pressure. Another reason may be the random slopes of relationships with classmates, whose random effects were not significant.
The associated factors for sleep quality identified in various studies may differ, primarily due to several reasons. Firstly, the survey population and hierarchical structure may vary. Differences in population, region, and composition can yield diverse outcomes. Secondly, the included independent variables may differ; the same variable may have different values. Thirdly, the interaction between variables can lead to discrepancies in results. Furthermore, different models can yield different results. Even when analyzing data using the same model, different researchers may obtain distinct results due to differences in the operational methods of the model. The first two conditions are difficult to control, but we can try to avoid differences in results through standardized operations.
In the present study, four models were utilized to examine the factors associated with sleep quality. To ensure the robustness of the outcomes, we carried out rigorous diagnostics for all four models. After diagnostics, all four models obtained the best linear unbiased estimators (BLUE) or best linear unbiased predictions (BLUP) according to Gauss-Markov's theorem and Atkin's theorem ( Hansen, 2022 ). Regression diagnostics are an important step that has been overlooked by many researchers. Standard diagnostics ensure more accurate parameter estimation and p -values and ultimately improve the overall quality of the analysis. Without diagnostics, some biased results for regression models will be obtained and reported. In the weighted MLR (resp., BLR) model, we found that other levels will increase or decrease PSQI scores (resp., risk of poor sleep quality) relative to the reference level. However, we can obtain detailed effect values of each level through LMM and LRRE. To our knowledge, previous studies have not shown similar results.
Limitations
There are several limitations in the present study. Firstly, the present study selected as many candidate influencing factors as possible for sleep quality, such as family support, social support, personal lifestyle, physical health, mental health, mobile phone addiction, and psychological resilience. However, considering the length of the questionnaire, there were still some potential factors that were not included, such as coffee drinks, depression, and habits. Secondly, this study was cross-sectional, which precluded the establishment of definitive conclusions regarding the direction of causality between sleep quality and risk factors. Further, longitudinal studies are needed to investigate the causal relationships. Thirdly, all questionnaires were self-reported, highlighting the inherent limitations of self-reported measures. Lastly, there were no strict exclusion criteria in this study, which may have resulted in self-selection bias. This means that participants with anxiety and depression are characteristics that are likely to interact with the outcome under investigation, or alternatively, they may have been more likely to participate since the topic is relevant to them.
This study examined the prevalence of risk factors associated with poor sleep quality among undergraduates in Jiangsu Province, China. Our results showed a considerable prevalence of poor sleep quality among this group, with students in higher grades exhibiting a higher likelihood of experiencing poor sleep quality. The study also identified modifiable factors that correlate with poor sleep quality, including psychological resilience, mobile phone addiction, smoking, drinking, and poorer physical health. In response to the factors associated with poor sleep quality identified in our study, we advocate for university administrators to deploy systematic educational programs and interventions tailored to enhance sleep quality among students. These initiatives should directly target modifiable risk factors such as mental health status, academic and employment pressures, and suboptimal dormitory conditions. Specifically, proposed interventions include organizing workshops and seminars on sleep hygiene to educate students about the importance of good sleep practices and the physiological underpinnings of sleep. University administrators should also offer mental health services that provide counseling and stress management strategies. They should facilitate sessions on academic and time management skills to mitigate the impact of academic and employment pressures and improve dormitory living conditions through the establishment of quiet hours and better noise insulation to foster a more sleep-conducive environment. These recommendations illustrate a thoughtfully considered approach to ameliorate the sleep-related challenges faced by university students. University administrators can provide adequate psychological counseling for students to alleviate their pressures and set appropriate dormitory conventions to address dormitory-related issues.
Data availability statement
The original contributions presented in the study are included in the article/ Supplementary material , further inquiries can be directed to the corresponding authors.
Ethics statement
The studies involving humans were approved by Ethics Committee of Xuzhou Medical University. The studies were conducted in accordance with the local legislation and institutional requirements. The participants provided their written informed consent to participate in this study.
Author contributions
BH: Methodology, Writing—review & editing. WS: Data curation, Funding acquisition, Writing—review & editing. YW: Writing—review & editing. QW: Data curation, Investigation, Writing—review & editing. JL: Writing—review & editing. XX: Writing—review & editing. YH: Writing—review & editing. LX: Data curation, Funding acquisition, Methodology, Writing—original draft. DY: Conceptualization, Writing—review & editing.
The author(s) declare financial support was received for the research, authorship, and/or publication of this article. This work was supported by the National Natural Science Foundation of China (grant number 12001470), the China Postdoctoral Science Foundation (grant number 2020M671607), and the Science and Technology Development Fund project of the Affiliated Hospital of Xuzhou Medical University (grant number XYFY202245). The funders had no role in the study design, data collection and analysis, decision to publish, or preparation of the manuscript.
Conflict of interest
The authors declare that the research was conducted in the absence of any commercial or financial relationships that could be construed as a potential conflict of interest.
Publisher's note
All claims expressed in this article are solely those of the authors and do not necessarily represent those of their affiliated organizations, or those of the publisher, the editors and the reviewers. Any product that may be evaluated in this article, or claim that may be made by its manufacturer, is not guaranteed or endorsed by the publisher.
Supplementary material
The Supplementary Material for this article can be found online at: https://www.frontiersin.org/articles/10.3389/fpsyg.2024.1343186/full#supplementary-material
Supplementary Table S1. Pittsburgh Sleep Quality Index scores of undergraduates with different characteristics.
Supplementary Figure S1. The performance of linearity, homoscedasticity, extreme values, and normality after diagnostics for weighted multiple linear regression model.
Supplementary Figure S2. The performance of linearity and extreme values after diagnostics for binary logistic regression model.
Supplementary Figure S3. The performance of linearity, homoscedasticity, extreme values, and normality after diagnostics for the weighted linear mixed model.
Supplementary Figure S4. The performance of linearity after diagnostics for logistic regression with random effects model.
Supplementary Figure S5. The linear and quadratic trend between MPAI and PSQI score in weighted multiple linear regression.
Supplementary Figure S6. ROC curve of binary logistic regression model.
Supplementary Figure S7. The linear and quadratic trend between age and PSQI score in weighted linear mixed model.
Supplementary Figure S8. ROC curve of logistic regression with random effects model.
Abbreviations
PSQI, Pittsburgh sleep quality index; MPAI, mobile phone addiction index; CD-RISC, Connor-Davidson resilience scale; IQR, interquartile range; CI, confidence intervals; SD, standard deviation; ANOVA, analysis of variance; VIF, variance inflation factor; EPV, events per variable; BMI, body mass index; OR, odds ratios; MLR, multiple linear regression model; BLR, binary logistic regression model; LMM, linear mixed model; LRRE, logistic regression with random effects; AIC, Akaike Information Criterion; AUC, area under curve; ICC, intraclass correlation coefficient; BLUE, best linear unbiased estimator; BLUP, best linear unbiased prediction.
Ahmed, M. S., Khan, S., Hsan, K., Sen, L. C., Yunus, F. M., and Griffiths, M. D. (2020). Factors affecting sleep quality among the university students in Bangladesh: a cross-sectional structured interview study. Sleep Vigilance 4, 177–184. doi: 10.1007/s41782-020-00106-4
Crossref Full Text | Google Scholar
Almojali, A. I., Almalki, S. A., Alothman, A. S., Masuadi, E. M., and Alaqeel, M. K. (2017). The prevalence and association of stress with sleep quality among medical students. J. Epidemiol. Glob. Health 7, 169–174. doi: 10.1016/j.jegh.2017.04.005
PubMed Abstract | Crossref Full Text | Google Scholar
Baranwal, N., Yu, P. K., and Siegel, N. S. (2023). Sleep physiology, pathophysiology, and sleep hygiene. Prog. Cardiovasc. Dis. 77, 59–69. doi: 10.1016/j.pcad.2023.02.005
Basner, M., Rao, H., Goel, N., and Dinges, D. F. (2013). Sleep deprivation and neurobehavioral dynamics. Curr. Opin. Neurobiol. 23, 854–863. doi: 10.1016/j.conb.2013.02.008
Bi, C., Lin, H., Zhang, J., and Zhao, Z. (2022). Association between sleep quality and depression symptoms in Chinese college students during the COVID-19 lockdown period. Children 9:1237. doi: 10.3390/children9081237
Bianchi, A., and Phillips, J. G. (2005). Psychological predictors of problem mobile phone use. CyberPsychol. Behav. 8, 39–51. doi: 10.1089/cpb.2005.8.39
Bolker, B. M., Brooks, M. E., Clark, C. J., Geange, S. W., Poulsen, J. R., Stevens, M. H., et al. (2009). Generalized linear mixed models: a practical guide for ecology and evolution. Trends Ecol. Evol. 24, 127–135. doi: 10.1016/j.tree.2008.10.008
Buysse, D. J., Reynolds, C. F., Monk, T. H., Berman, S. R., and Kupfer, D. J. (1989). The Pittsburgh sleep quality index: a new instrument for psychiatric practice and research. Psychiatry Res. 28, 193–213. doi: 10.1016/0165-1781(89)90047-4
Chen, M. L., Lin, L. C., Wu, S. C., and Lin, J. G. (1999). The effectiveness of acupressure in improving the quality of sleep of institutionalized residents. J. Gerontol. A Biol. Sci. Med. Sci. 54, M389–M394. doi: 10.1093/gerona/54.8.M389
Connor, K. M., and Davidson, J. R. T. (2003). Development of a new resilience scale: the Connor-Davidson Resilience Scale (CD-RISC). Depress. Anxiety 18, 76–82. doi: 10.1002/da.10113
Curcio, G., Ferrara, M., and De Gennaro, L. (2006). Sleep loss, learning capacity and academic performance. Sleep Med. Rev. 10, 323–337. doi: 10.1016/j.smrv.2005.11.001
Davies, S. K., Ang, J. E., Revell, V. L., Holmes, B., Mann, A., Robertson, F. P., et al. (2014). Effect of sleep deprivation on the human metabolome. Proc. Natl. Acad. Sci. USA. 111, 10761–10766. doi: 10.1073/pnas.1402663111
Demirci, K., Akgönül, M., and Akpinar, A. (2015). Relationship of smartphone use severity with sleep quality, depression, and anxiety in university students. J. Behav. Addict . 4, 85–92. doi: 10.1556/2006.4.2015.010
Durmer, J. S., and Dinges, D. F. (2005). Neurocognitive consequences of sleep deprivation. Semin. Neurol. 25, 117–129. doi: 10.1055/s-2005-867080
Eleftheriou, A., Rokou, A., Arvaniti, A., Nena, E., and Steiropoulos, P. (2021). Sleep quality and mental health of medical students in Greece during the COVID-19 pandemic. Front. Public Health 9:775374. doi: 10.3389/fpubh.2021.775374
Gerhardsson, A., Åkerstedt, T., Axelsson, J., Fischer, H., Lekander, M., Schwarz, J., et al. (2019). Effect of sleep deprivation on emotional working memory. J. Sleep Res. 28:e12744. doi: 10.1111/jsr.12744
Ghrouz, A. K., Noohu, M. M., Dilshad Manzar, M., Warren Spence, D., BaHammam, A. S., and Pandi-Perumal, S. R. (2019). Physical activity and sleep quality in relation to mental health among college students. Sleep Breath. 23, 627–634. doi: 10.1007/s11325-019-01780-z
Hansen, B. E. A. (2022). modern Gauss–Markov theorem. Econometrica 90, 1283–1294. doi: 10.3982/ECTA19255
Kabacoff, R. I. (2015). R in Action: Data Analysis and Graphics with R, Second Edition . Shelter Island: Manning publications Co.
Google Scholar
Kang, J.-H., and Chen, S.-C. (2009). Effects of an irregular bedtime schedule on sleep quality, daytime sleepiness, and fatigue among university students in Taiwan. BMC Public Health 9:248. doi: 10.1186/1471-2458-9-248
Lemma, S., Gelaye, B., Berhane, Y., Worku, A., and Williams, M. A. (2012). Sleep quality and its psychological correlates among university students in Ethiopia: a cross-sectional study. BMC Psychiatry 12:237. doi: 10.1186/1471-244X-12-237
Li, L., Griffiths, M. D., Mei, S., and Niu, Z. (2020). Fear of missing out and smartphone addiction mediates the relationship between positive and negative affect and sleep quality among Chinese university students. Front. Psychiatry 11:877. doi: 10.3389/fpsyt.2020.00877
Li, M., Han, Q., Pan, Z., Wang, K., Xie, J., Zheng, B., et al. (2022). Effectiveness of multidomain dormitory environment and roommate intervention for improving sleep quality of medical college students: a cluster randomised controlled trial in China. Int. J. Environ. Res. Public Health 19:15337. doi: 10.3390/ijerph192215337
Li, Y., Bai, W., Zhu, B., Duan, R., Yu, X., Xu, W., et al. (2020). Prevalence and correlates of poor sleep quality among college students: a cross-sectional survey. Health Qual. Life Outcomes 18:210. doi: 10.1186/s12955-020-01465-2
Li, Y., and Guo, K. (2023). Research on the relationship between physical activity, sleep quality, psychological resilience, and social adaptation among Chinese college students: a cross-sectional study. Front. Psychol. 14:1104897. doi: 10.3389/fpsyg.2023.1104897
Lo, J. C., Chong, P. L. H., Ganesan, S., Leong, R. L. F., and Chee, M. W. L. (2016). Sleep deprivation increases formation of false memory. J. Sleep Res. 25, 673–682. doi: 10.1111/jsr.12436
Lund, H. G., Reider, B. D., Whiting, A. B., and Prichard, J. R. (2010). Sleep patterns and predictors of disturbed sleep in a large population of college students. J. Adolesc. Health 46, 124–132. doi: 10.1016/j.jadohealth.2009.06.016
Nyer, M., Farabaugh, A., Fehling, K., Soskin, D., Holt, D., Papakostas, G. I., et al. (2013). Relationship between sleep disturbance and depression, anxiety, and functioning in college students. Depress. Anxiety 30, 873–880. doi: 10.1002/da.22064
Peduzzi, P., Concato, J., Kemper, E., Holford, T. R., and Feinstein, A. R. A. (1996). simulation study of the number of events per variable in logistic regression analysis. J. Clin. Epidemiol. 49, 1373–1379. doi: 10.1016/S0895-4356(96)00236-3
Peltz, J., and Rogge, R. (2022). Adolescent and parent sleep quality mediates the impact of family processes on family members' psychological distress. Sleep Health 8, 73–81. doi: 10.1016/j.sleh.2021.08.009
Shao, R., He, P., Ling, B., Tan, L., Xu, L., Hou, Y., et al. (2020). Prevalence of depression and anxiety and correlations between depression, anxiety, family functioning, social support and coping styles among Chinese medical students. BMC Psychol 8:38. doi: 10.1186/s40359-020-00402-8
St-Onge, M.-P. (2017). Sleep-obesity relation: underlying mechanisms and consequences for treatment. Obes. Rev. 18(Suppl 1), 34–39. doi: 10.1111/obr.12499
Stroup, W. W. (2013). Generalized Linear Mixed Models: Modern Concepts, Methods and Applications . New York, NY: Taylor and Francis Group.
Suen, L. K. P., Hon, K. L. E., and Tam, W. W. S. (2008). Association between sleep behavior and sleep-related factors among university students in Hong Kong. Chronobiol. Int. 25, 760–775. doi: 10.1080/07420520802397186
Tang, J., Liao, Y., Kelly, B. C., Xie, L., Xiang, Y. T., Qi, C., et al. (2017). Gender and regional differences in sleep quality and insomnia: a general population-based study in Hunan province of China. Sci. Rep. 7:43690. doi: 10.1038/srep43690
Vaccaro, A., Kaplan Dor, Y., Nambara, K., Pollina, E. A., Lin, C., Greenberg, M. E., et al. (2020). Sleep loss can cause death through accumulation of reactive oxygen species in the gut. Cell 181, 1307–1328.e15. doi: 10.1016/j.cell.2020.04.049
Wang, L., Qin, P., Zhao, Y., Duan, S., Zhang, Q., Liu, Y., et al. (2016). Prevalence and risk factors of poor sleep quality among Inner Mongolia medical university students: a cross-sectional survey. Psychiatry Res. 244, 243–248. doi: 10.1016/j.psychres.2016.04.011
Xian, X., Zhang, Y., Bai, A., Zhai, X., Hu, H., Zhang, J., et al. (2022). Association between family support, stress, and sleep quality among college students during the COVID-19 online learning period. Int. J. Environ. Res. Public Health 20:248. doi: 10.3390/ijerph20010248
Xie, G., Wu, Q., Guo, X., Zhang, J., and Yin, D. (2023). Psychological resilience buffers the association between cell phone addiction and sleep quality among college students in Jiangsu Province, China. Front. Psychiatry 14:1105840. doi: 10.3389/fpsyt.2023.1105840
Xu, C., Wang, Y., Wang, Z., Li, B., Yan, C., Zhang, S., et al. (2022). Social support and coping style of medical residents in China: the mediating role of psychological resilience. Front. Psychiatry 13:888024. doi: 10.3389/fpsyt.2022.888024
Zhou, Y., Bo, S., Ruan, S., Dai, Q., Tian, Y., Shi, X., et al. (2022). Deteriorated sleep quality and influencing factors among undergraduates in northern Guizhou, China. PeerJ 10:e13833. doi: 10.7717/peerj.13833
Keywords: sleep quality, undergraduates, risk factors, Connor-Davidson resilience scale, mobile phone addiction index, Pittsburgh Sleep Quality Index
Citation: Hu B, Shen W, Wang Y, Wu Q, Li J, Xu X, Han Y, Xiao L and Yin D (2024) Prevalence and related factors of sleep quality among Chinese undergraduates in Jiangsu Province: multiple models' analysis. Front. Psychol. 15:1343186. doi: 10.3389/fpsyg.2024.1343186
Received: 23 November 2023; Accepted: 22 March 2024; Published: 10 April 2024.
Reviewed by:
Copyright © 2024 Hu, Shen, Wang, Wu, Li, Xu, Han, Xiao and Yin. This is an open-access article distributed under the terms of the Creative Commons Attribution License (CC BY) . The use, distribution or reproduction in other forums is permitted, provided the original author(s) and the copyright owner(s) are credited and that the original publication in this journal is cited, in accordance with accepted academic practice. No use, distribution or reproduction is permitted which does not comply with these terms.
*Correspondence: Dehui Yin, yindh16@xzhmu.edu.cn ; Lishun Xiao, xiaolishun@xzhmu.edu.cn
† These authors have contributed equally to this work and share first authorship
Disclaimer: All claims expressed in this article are solely those of the authors and do not necessarily represent those of their affiliated organizations, or those of the publisher, the editors and the reviewers. Any product that may be evaluated in this article or claim that may be made by its manufacturer is not guaranteed or endorsed by the publisher.
University of Utah Hospital
General questions.
- Billing & Insurance
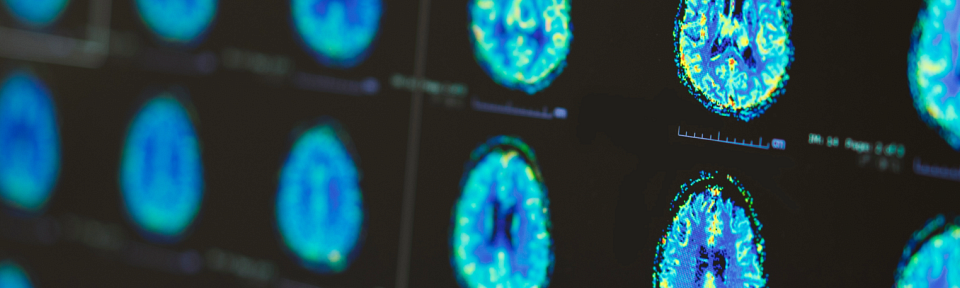
- Health Care Home
- Press Releases
University of Utah announces major funding for new addiction treatment research
Media contact:.
Patricia Brandt Manager, Public Relations and Communications, Huntsman Mental Health Institute University of Utah Health Email: Patricia.Brandt @hsc.utah.edu
Salt Lake City (April 10, 2024) - Worldwide, someone dies from drug or alcohol addiction every four minutes. Now, researchers at Huntsman Mental Health Institute at the University of Utah have been selected by Wellcome Leap to research a new treatment for substance use disorder as part of a $50 million commitment to develop innovative treatments.

Brian J. Mickey, MD, PhD, professor of psychiatry at Huntsman Mental Health Institute (pictured top left), will lead the team of investigators with expertise in psychiatry, biomedical engineering, neuroscience, radiology, and social work to research a new, noninvasive treatment for addiction. Co-principal investigators include Jan Kubanek, PhD , (pictured top center), and Taylor Webb, PhD (pictured top right); co-investigators include (from left to right) Eric Garland, PhD, LCSW ; Rana Jawish, MD ; Vincent Koppelmans, PhD ; and Tom Riis, PhD.
The research will be funded by the Untangling Addiction program, which is a $50 million program founded by Wellcome Leap , to develop scalable measures to assess addiction susceptibility, quantify the risks stemming from addiction, and develop innovative treatments.
“Substance use disorder is a significant global health problem, and yet the treatment options are limited,” Mickey said. “We’re developing a non-invasive intervention for preventing and treating addiction, chronic pain, and depression. This funding will help us validate and generate the data to support the next critical step: an efficacy trial to determine the effectiveness of the intervention.”
Mickey’s team will use a novel ultrasound-based device to modulate deep brain regions and behaviors associated with opioid addiction. The goal will be to ultimately develop this approach into an individually targeted therapeutic intervention for a range of addictions. “Addictions are brain illnesses that have enormous negative impact on individuals, families, and society,” Mickey said. “A major reason that addictions have been difficult to prevent—and treat—is that they are driven by dysfunction of deep brain regions that are challenging to access. Many psychiatric problems such as depression, anxiety, and addiction are caused by malfunction of brain circuits. This project is an example of our mission to understand how these neural circuits are dysregulated and to develop novel, circuit-targeted interventions that return the brain to a healthy state.”
"We are proud to bring Wellcome Leap's innovative problem-solving and funding approach to our research enterprise at the University of Utah," said Taylor Randall, President , University of Utah. "To have our mental health researchers contributing to pioneering work on addiction treatment reaffirms our commitment to improving lives through discovery."
“What makes research like this so impactful is that it brings together a variety of disciplines to help solve complex problems in mental health,” said Mark Hyman Rapaport, MD , CEO of Huntsman Mental Health Institute. “This is particularly timely news given the groundbreaking of a new translational research building on campus focused on mental health and the brain. Our nation is in a mental health crisis, but there is hope if we can think differently and work together to change this trajectory.”
About Huntsman Mental Health Institute
Huntsman Mental Health Institute at University of Utah Health brings together 75 years of patient care, research, and education into one of the nation's leading academic medical centers focused on mental health. Nestled in the campus of University of Utah, Huntsman Mental Health Institute serves the community with 1,600 faculty and staff in 20 locations providing inpatient and outpatient services for youth, teens, and adults as well as a comprehensive crisis care model which includes the nationally recognized SafeUT app and the 988 Crisis hotline for Utah. Our mission is to advance mental health knowledge, hope, and healing for all. Learn more at: HMHI.utah.edu and join the conversation on Instagram , Facebook , TikTok , X and LinkedIn .
- huntsman mental health institute
Europe PMC requires Javascript to function effectively.
Either your web browser doesn't support Javascript or it is currently turned off. In the latter case, please turn on Javascript support in your web browser and reload this page.
Search life-sciences literature (43,905,756 articles, preprints and more)
- Free full text
- Citations & impact
- Similar Articles
Methodological and Conceptual Limitations in Exercise Addiction Research.
Author information, affiliations.
- Griffiths MD 2
- de La Vega Marcos R 3
- Demetrovics Z 4
ORCIDs linked to this article
- Szabo A | 0000-0003-2788-4304
The Yale Journal of Biology and Medicine , 03 Sep 2015 , 88(3): 303-308 PMID: 26339214 PMCID: PMC4553651
Abstract
Free full text .

Focus: Addiction
Methodological and conceptual limitations in exercise addiction research, attila szabo.
a Institute of Health Promotion and Sport Sciences, Eötvös Loránd University, Budapest, Hungary
Mark D. Griffiths
b Psychology Division, Nottingham Trent University, Nottingham, United Kingdom
Ricardo de La Vega Marcos
c Department of Physical Education, Sport & Human Movement, Autonomous University of Madrid, Campus Cantoblanco, Madrid, Spain
Barbara Mervó
d Institute of Psychology, Eötvös Loránd University, Budapest, Hungary
Zsolt Demetrovics
The aim of this brief analytical review is to highlight and disentangle research dilemmas in the field of exercise addiction. Research examining exercise addiction is primarily based on self-reports, obtained by questionnaires (incorporating psychometrically validated instruments), and interviews, which provide a range of risk scores rather than diagnosis. Survey methodology indicates that the prevalence of risk for exercise addiction is approximately 3 percent among the exercising population. Several studies have reported a substantially greater prevalence of risk for exercise addiction in elite athletes compared to those who exercise for leisure. However, elite athletes may assign a different interpretation to the assessment tools than leisure exercisers. The present paper examines the: 1) discrepancies in the classification of exercise addiction; 2) inconsistent reporting of exercise addiction prevalence; and 3) varied interpretation of exercise addiction diagnostic tools. It is concluded that there is the need for consistent terminology, to follow-up results derived from exercise addiction instruments with interviews, and to follow a theory-driven rationale in this area of research.
Introduction
While studying the effects of exercise on sleep, Baekeland [ 1 ] noted that regular exercisers experienced withdrawal symptoms when deprived of exercise. This observation pointed to the addictive nature of exercise and provided an important starting point and impetus for research into exercise addiction. Exercise addiction falls within the field of behavioral addictions, similar to gambling disorder, but due to the lack of sustained and methodologically rigorous evidence for exercise addiction as a morbidity, the disorder is not listed as a mental dysfunction in the latest (fifth) edition of the Diagnostic and Statistical Manual of Mental Disorders (DSM-5) [ 2 ]. In fact, to the authors’ knowledge, only three case studies have been reported in the literature to date [ 3 , 4 , 5 ].
Exercise addiction has been described as a morbid pattern of behavior in which the habitually exercising individual loses control over his or her exercise habits and acts compulsively, exhibits dependence, and experiences negative consequences to health as well as in his or her social and professional life. It is different from exaggerated exercise present in various eating disorders (also known as secondary exercise addiction [ 5 ]), in which the behavior represents a means of weight loss rather than addiction. The negative consequences experienced are the key factors that separate healthy from unhealthy patterns of exercise. In addition to bodily injuries and re-injuries due to exaggerated amounts of exercise without proper rest and recuperation, social and psychological hardship is also evident in exercise addiction [ 3 ]. The inability to sleep and concentrate, a sense of restlessness, and painful withdrawal symptoms (akin to substance abuse) are also daily manifestations in exercise addiction [ 3 ]. These negative consequences are so severe that they interfere with the normal daily functioning of the individual. Therefore, exercise addiction is a serious psychological morbidity that should be understood and recognized by clinicians and prevented whenever possible.
The present authors carried out an analysis of published outputs in PubMed and Google Scholar over a 3-year period (January 2011 to January 2014), and 128 publications were found that examine exercise addiction. This shows that there are approximately 40 publications per year on exercise addiction in recent years. The 128 publications appeared in 89 different journals, showing that exercise addiction is studied in a multidisciplinary field. The majority of the research comes primarily from just three developed nations ( Figure 1 ), where exercise may represent a higher-order need in contrast to the less-developed nations [ 6 ]. In spite of the growing keen interest in exercise addiction research, there are several methodological and also conceptual limitations that obscure the focus of the research and obstruct the advancement of knowledge in the area. These limiting factors delay the hope for the inclusion of the disorder in a future edition of the DSM.

Country origin of research on exercise addiction over a 3-year period (2011-2014) based on publications identified by PubMed and Google Scholar. The frequency denotes the number of papers published by scholars in the given nation over 3 years.
The present paper is organized into three main sections: 1) the discrepancies in the classification of exercise addiction; 2) the inconsistent reporting of exercise addiction prevalence; and 3) the varied interpretation of exercise addiction diagnostic tools. Through the analysis of these sections, it will be demonstrated that there is an urgent need for consistent terminology, a good reason for following up on questionnaire results with interviews, and a need to conduct theory-based research in the area.
Discrepancies in Classification of Exercise Addiction
Terminology.
To date, research investigating exercise addiction has been unfocused. For example, several terminologies are used for describing exercise addiction, including: exercise dependence [ 7 ], compulsive exercise [ 8 ], obligatory exercise [ 9 ], and exercise abuse [ 10 ]. Cook and Hausenblas [ 11 ] specifically noted that using the same scientific term to denote different situations or adopting different terms for the same situation leads to ambiguity, misconception, irreproducible results, and errors in interpretation. In spite of the fact that exercise dependence is the most often-used term ( Table 1 ), some scholars [ 12 , 13 ] have emphasized that the term addiction may be the most appropriate, because it includes both dependence and compulsion [ 14 ].
Amount of Exercise
Exercising excessively does not necessarily indicate a disorder. A further dilemma concerns the evaluation of exercise addiction, which considers the maladaptive behavior as being reflected by very high (often termed as “exaggerated”) volume of exercise. However, excessive exercise itself is not necessarily maladaptive. Athletes who become national or international competitors spend the largest proportion of their day in training or activities related to their training without having psychological problems related to their volume of exercise and/or the general stresses of their day-to-day job (something that would be experienced by most workers in whatever job they do and therefore not necessarily related to exercise addiction). They do not experience harm (apart from injuries) in their personal and social lives as a consequence of their excessive exercise behavior, because the latter is part of their expected daily routine, so they can also fulfill their other life obligations. In contrast, morbid patterns of exercise can result in personal and social harm to the individual.
Inconsistent Reporting of Exercise Addiction Prevalence
Questionnaire-based studies establish the risk for exercise addiction rather than clinical cases (diagnosis). The latter can be made using the criteria for addictive disorders listed in the DSM-5. To date, and as noted earlier, only three such cases have been reported [ 3 , 4 , 5 ]. A prominent inconsistency in the field concerns the reporting of prevalence rates for exercise addiction. For instance, Mónok et al. [ 15 ] found that the incidence of high risk for exercise addiction is 0.3 percent to 0.5 percent in the general population, while among regular exercisers it is 1.9 percent to 3.2 percent. A figure of around 3.0 percent is consistent with several empirical studies in the literature [ 13 , 16 , 17 , 18 , 19 , 20 ]. However, there are numerous reports with prevalence rates that exceed five to 10 times this figure. For example, Lejoyeux et al. [ 21 ] reported that 42 percent of a sample were diagnosed with exercise addiction (“All clients of the fitness room 18 years and older were invited to participate in the study. Three hundred subjects were included; 125 (42 percent) presented diagnostic criteria of exercise dependence.”). Apart from the issue that questionnaires do not serve diagnostic purposes (discussed below), the wide range of prevalence rates reported for the disorder raise questions about why the findings are so inconsistent.
There are probably several explanations for the wide diversity of prevalence rates for exercise addiction. First, the various assessment scales used may yield different results. Even the two most popular and well-validated instruments in the field, the Exercise Addiction Inventory (EAI) [ 22 ] and the Exercises Dependence Scale (EDS) [ 23 ] generate somewhat different, although arguably comparable, results [ 15 ]. The difference between the two instruments is that the latter is based on DSM and its revised version has 21 items, while the former is based on six common symptoms of addiction [ 13 ] and has only six items. Second, the populations sampled in various studies may not only be different (e.g., students, runners, body builders, gym users, etc.), but also, as a consequence of this diversity, may attribute different interpretation to the items in the survey instrument used [ 24 ]. Third, recent evidence shows that there are differences in the subjective interpretation of the items on the EAI between men and women, as well as across different cultures [ 24 ]. Therefore, the gender ratio in a sample and cultural component of any given investigation also may contribute to the large difference in prevalence rates. Furthermore, intense involvement with sports and exercise may influence either the interpretation and/or the actual scoring of the instruments utilized. Indeed, Szabo and Griffiths [ 19 ] showed that Sport Science undergraduates reported being at twice the risk for exercise addiction than general, habitual exercisers.
It appears that the risk for exercise addiction is influenced by several subjective and objective factors. Consideration of all known factors such as gender, athletic involvement, and all others shown to have an effect on the results may — at least in part — help bring about greater agreement in the reports of risk-prevalence of exercise addiction. Results of prior empirical research may only have been relevant to a homogeneous subgroup of exercisers. This appears to be a fact that needs to be recognized by the scholastic community before attempting to generalize the findings across the field.
Varied Interpretation of Exercise Addiction Diagnostic Tools
Need for interviews.
Instruments that assess exercise addiction do not serve as diagnostic tools for exercise addiction. The problem with sole reliance on self-report instruments in studying exercise addiction is that they yield a range of scores with values in the higher end that are interpreted as indices of risk for addiction. A score (on such instruments) may not indicate damage or detriment to individuals or those around them. If there is no harm, there is no addiction. By definition, excessive exercise is only problematic if it results in some sort of harm (injury, negligence of work, family, or other obligations that trigger negative consequences or harm in the individual's life). Therefore, data obtained using validated instruments need to be followed up with interviews and/or corroborative evidence to elaborate and confirm individual negative consequences. However, only a very small proportion of the empirical studies examining exercise addiction has complemented survey data with interviews.
Müller at al. [ 25 ] examined the level of agreement between the questionnaire-based classification of “at-risk for exercise addiction” and the results of the evaluations conducted via interviews. These authors studied 134 participants using the EDS and a structured clinical interview. The congruence between the questionnaire-based categorizations of “at-risk for exercise addiction” and the diagnosis of the disorder was examined using k-coefficients (Cohen’s kappa coefficient [ 26 ]). The findings demonstrated that the agreement between questionnaire-based and interview-based assessment was only fair to moderate, with more false positive classifications emerging from self-report instruments. The authors concluded that if the structured clinical interview is accepted to be a reliable method for the detection of the disorder, then the instruments — in this case, the EDS, which is one of the most reliable assessment tools for exercise addiction — overestimates the risk for the prevalence of exercise addiction.
The clear message of Müller et al.’s study is that without an associated interview, researchers cannot be certain of a problem in the exercise behavior of their study participants. Between the “at-risk” classification and disorder, there is an obscure gray area because it is relatively unpredictable (at the present time) as to who will develop an exercise addiction disorder from among those who were classified — via questionnaires — as being “at-risk” for the disorder. A clear borderline between “at-risk” and pathology is the evidence of harm suffered as a consequence of the (exercise) behavior. This “at-risk/disorder” borderline can only be rigorously assessed via interviews on a case-by-case basis. This is because via interviews “approaching” harm and lack of control over exercise may surface in a clearer way than via assessment scales. The mistake of not using interviews in diagnosis is a major shortcoming of the exercise addiction literature and hinders the advancement of the knowledge in the field. The present authors recommend that future studies should examine the disorder by (at the very least) interviewing those who score above a given cutoff point on a given paper-and-pencil instrument. These interviews should look not only to presence but also to the severity of the symptoms of addiction and the risk of harm to the physical and mental health of the individual. Any detrimental health effects and the endorsement of DSM-5 criteria for addictive disorders should be interpreted as markers of a morbid exercise pattern.
Issues with Using Elite Athletes as Test Subjects
Unlike athletic training, the satisfying of addictive urges cannot be scheduled. If elite athletes were addicted, they would have to exercise beyond their already demanding training schedule, which would be physically impossible. Therefore, studying exercise addiction among elite athletes could be viewed as futile. Nevertheless, researchers have begun to examine exercise addiction among elite or competitive athletes. Despite the fact that exercise addiction — if present — could represent the end of the sporting career for elite athletes, most studies that have scrutinized them have reported a significantly higher prevalence of high risk for exercise addiction than in leisure or student exercisers. Blaydon and Lindner [ 27 ] studied 203 triathletes competing at amateur (n = 133) and professional (n = 70) levels. They found that the incidence of exercise addiction ranged from 21.6 percent to 30.4 percent in the whole sample, while among the sub-sample of professional athletes, 41.4 percent were at risk for exercise addiction. Studying participants competing at different triathlon distances, Youngman [ 28 ] reported that almost 20 percent of the 1,285 triathletes studied were at risk for exercise addiction. According to the results of a study by McNamara and McCabe [ 29 ], approximately one-third of Australian elite athletes (34 percent) may be at high risk for exercise addiction. In a more recent investigation, a significant proportion of Spanish ultra-marathoners (17 percent) were classified as at high risk for exercise addiction [ 30 ]. Results with Italian athletes have shown a similar rate, with 18.3 percent of 262 competitive athletes from nine different sports (basketball, futsal, football, handball, hockey, rugby, softball, volleyball, and water polo) found to be at risk for exercise addiction [ 31 ]. A lower incidence of 7.1 percent was found in non-professional second and third division Danish soccer players [ 32 ]. However, even this “lower” figure is high, considering the much lower percentages found in the general and recreational exercising population [ 13 , 15 , 20 ].
A major conceptual concern regarding these high prevalence figures (ranging between 7 percent and 42 percent) is that if elite athletes are indeed at such a high risk for exercise addiction, then coaches, health professionals, and research scholars are dealing with a psychopathology-prone athletic population. However, this is very unlikely. To date, there is no empirical data that has recognized differences in the interpretation of the scale items by leisure exercisers and elite athletes. This issue needs systematic investigation in the future, because, conceptually, the investigation of the disorder among elite athletes does not make any rational sense.
First, by definition in exercise addiction, there is a loss of control over the behavior. Therefore, the gratification must occur when the urge compels the individual to exercise. In sports, training and competition sessions are scheduled. They do not match one’s inner urges and compulsions. Hence, the exercise-addicted athlete would need to exercise before and after training, too, which would be physically impossible. There is no scheduled addiction. The high “addiction” scores of athletes must therefore be due to a different interpretation of the instrument’s items. For example, “Exercise is the most important thing in my life” (Item 1 of the EAI) can also be interpreted as the main goal is to succeed in sporting career; thus, an endorsement of “strongly agree” (maximum score) on the questionnaire may be a sincere response from an elite athlete. Furthermore, athletes also may experience conflicts in their lives because of their training. The frequent and demanding workouts could interfere with their personal and social lives. However, like work interfering with other life activities for other people, this type of conflict is not the intra- and interpersonal conflict that is experienced by people addicted to exercise. Nevertheless, a high or maximum high score may be given for Item 2 on the EAI: “Conflicts have arisen between me and my family and/or my partner about the amount of exercise I do” at the time of assessment. Another item on the EAI is: “If I have to miss an exercise session I feel moody and irritable.” Professional athletes naturally will get irritated when they have to miss an important training session, because that may cost them participation at an upcoming competition. Further possible differences in interpretation of instrument items are illustrated in Figure 2 .

Illustration of the possible different interpretations of the statements on the EAI by maladaptive exercisers and elite athletes. Note: The key point of Figure 2 is to illustrate that different interpretations may yield equally high scores leading to erroneous conclusions.
Consequently, elite athletes demonstrate high ratings on the instruments assessing exercise addiction, not because of morbidity but because of a different interpretation associated with their high commitment to their sporting profession. Their responses may involve aspirations and visions within their strong motivation to become the best they can be in their sport. Without follow-up interviews, the athletes’ high scores on exercise addiction instruments may be a conceptual mistake. Müller et al. [ 25 ] have demonstrated that self-report instruments overestimate the prevalence of exercise addiction. In the case of elite athletes, this observation may be even more pertinent.
Conclusions and Outlook
Research examining exercise addiction is steadily growing, yet methodological and conceptual limitations hinder advancement of knowledge in the field. As yet, there is no consistency in describing addictive exercise behavior. Self-report instruments only provide a risk score, and due to inconsistent interpretations related to the nature of the studied sample (e.g., participants’ gender, athletic involvement, and cultural differences), the meaning of the self-report data is difficult to explain and/or interpret. The presumption that athletes are addicted to exercise may be wrong. Their higher scores compared to leisure exercisers may reflect different attitudes toward exercise and sport behavior that are mirrored in a different interpretation of the instrument items.
Future research should therefore use in-depth interviews with those suspected to be addicted to exercise as based on their high scores obtained on relevant instruments. Research into exercise addiction should be theoretically driven. For example, the description of behavioral disorders in the “Addictive Disorders” section of the DSM-5 is potentially useful as a model for studying exercise addiction, while also taking into consideration the common symptoms found in addictions [ 13 ]. Like all other addictions, exercise addiction may reflect an escape from a hardship along with an accessible way to overcome negative criticism, because exercise itself is a positive and socially valued behavior. The diagnosis of exercise addiction should establish harm and damage — and its cause — suffered by the individual as a consequence of the maladaptive exercise behavior.
Abbreviations
Author contributions.
AS and ZD conceived the framework of the paper and wrote the majority; MDG had conceptual and theoretical input and also wrote parts of the paper; RVM synthesized the literature, provided writing ideas and critically evaluated the final version of the manuscript; BM reviewed PubMed and Google Scholar for research trends in exercise addiction.
- Baekeland F. Exercise deprivation: sleep and psychological reactions. Arch Gen Psychiatry. 1970; 22 (4):365–369. [ Abstract ] [ Google Scholar ]
- American Psychiatric Association. Diagnostic and statistical manual of mental disorders. 5th ed. Arlington, VA: American Psychiatric Publishing; 2013. [ Google Scholar ]
- Griffiths M. Exercise addiction: a case study. Addiction Research. 1997; 5 (2):161–168. [ Google Scholar ]
- Kotbagi G, Muller I, Romo L, Kern L. Pratique problématique d’exercice physique: un cas clinique. Annales Médico-Psychologuiques Revue Psychiatrique. 2014; 172 (10):883–887. [ Google Scholar ]
- Veale CD. In: Exercise addiction: motivation for participation in sport and exercise. Anett J, Cripps B, Steinberg H, editors. Leicester, UK: The British Psychological Society; 1995. pp. 1–5. [ Google Scholar ]
- Maslow AH. Toward a psychology of being. New York: Start Publishing; 2002. [ Google Scholar ]
- Adams J. Understanding exercise dependence. J Contemp Psychother. 2009; 39 (4):231–240. [ Google Scholar ]
- Murray SB, Maguire S, Russell J, Touyz SW. The emotional regulatory features of bulimic episodes and compulsive exercise in muscle dysmorphia: a case report. Eur Eat Disord Rev. 2012; 20 (1):68–73. [ Abstract ] [ Google Scholar ]
- Thome JL, Espelage DL. Obligatory exercise and eating pathology in college females: replication and development of a structural model. Eat Behav. 2007; 8 (3):334–349. [ Abstract ] [ Google Scholar ]
- Calogero RM, Pedrotty KN. The practice and process of healthy exercise: an investigation of the treatment of exercise abuse in women with eating disorders. Eat Disord. 2004; 12 (4):273–291. [ Abstract ] [ Google Scholar ]
- Cook BJ, Hausenblas HA. The role of exercise dependence for the relationship between exercise behavior and eating pathology mediator or moderator? J Health Psychol. 2008; 13 (4):495–502. [ Abstract ] [ Google Scholar ]
- Berczik K, Szabo A, Griffiths MD, Kurimay T, Kun B, Urbán R. et al. Exercise addiction: symptoms, diagnosis, epidemiology, and etiology. Subst Use Misuse. 2012; 47 (4):403–417. [ Abstract ] [ Google Scholar ]
- Szabo A. Addiction to exercise: a symptom or a disorder? New York: Nova Science Publishers; 2010. [ Google Scholar ]
- Goodman A. Addiction: definition and implications. Brit J Addict. 1990; 85 (11):1403–1408. [ Abstract ] [ Google Scholar ]
- Mónok K, Berczik K, Urbán R, Szabo A, Griffiths MD, Farkas J. et al. Psychometric properties and concurrent validity of two exercise addiction measures: a population wide study. Psychology of Sport and Exercise. 2012; 13 (6):739–746. [ Google Scholar ]
- Allegre B, Therme P, Griffiths M. Individual factors and the context of physical activity in exercise dependence: a prospective study of ‘ultra-marathoners’ Int J Ment Health Addiction. 2007; 5 (3):233–243. [ Google Scholar ]
- Edmunds J, Ntoumanis N, Duda JL. Examining exercise dependence symptomatology from a self-determination perspective. J Health Psychol. 2006; 11 (6):887–903. [ Abstract ] [ Google Scholar ]
- Griffiths MD, Szabo A, Terry A. The exercise addiction inventory: a quick and easy screening tool for health practitioners. Brit J Sport Med. 2005; 39 (6):e30. [ Europe PMC free article ] [ Abstract ] [ Google Scholar ]
- Szabo A, Griffiths MD. Exercise addiction in British sport science students. Int J Ment Health Addiction. 2007; 5 (1):25–28. [ Google Scholar ]
- Szabo A. In: Physical activity and psychological well-being. Biddle SJH, Fox KR, Boutcher SH, editors. London: Routledge; 2000. pp. 130–153. [ Google Scholar ]
- Lejoyeux M, Avril M, Richoux C, Embouazza H, Nivoli F. Prevalence of exercise dependence and other behavioral addictions among clients of a Parisian fitness room. Compr Psychiatry. 2008; 49 (4):353–358. [ Abstract ] [ Google Scholar ]
- Terry A, Szabo A, Griffiths MD. The exercise addiction inventory: a new brief screening tool. Addiction Research & Theory. 2004; 12 (5):489–499. [ Google Scholar ]
- Hausenblas HA, Symons Downs D. How much is too much? The development and validation of the exercise dependence scale. Psychology and Health. 2002; 17 (4):387–404. [ Google Scholar ]
- Griffiths MD, Urbán R, Demetrovics Z, Lichtenstein MB, de la Vega R, Kun B. et al. A cross-cultural re-evaluation of the Exercise Addiction Inventory (EAI) in five countries. Sports Medicine – Open. 2015; 1 (1):1–5. [ Europe PMC free article ] [ Abstract ] [ Google Scholar ]
- Müller A, Cook B, Zander H, Herberg A, Müller V, de Zwaan M. Does the German version of the Exercise Dependence Scale measure exercise dependence? Psychology of Sport and Exercise. 2014; 15 (3):288–292. [ Google Scholar ]
- Carletta J. Assessing agreement on classification tasks: the kappa statistic. Computational Linguistics. 1996; 22 (2):249–254. [ Google Scholar ]
- Blaydon MJ, Lindner KJ. Eating disorders and exercise dependence in triathletes. Eat Disord. 2002; 10 (1):49–60. [ Abstract ] [ Google Scholar ]
- Youngman JD. Risk for exercise addiction: a comparison of triathletes training for sprint-, olympic-, half-Ironman-, and Ironman-distance triathlons [dissertation] [Miami FL]: University of Miami; 2007. Available from: http://scholarlyrepository.miami.edu/oa_dissertations/12/ [ Google Scholar ]
- McNamara J, McCabe MP. Striving for success or addiction? Exercise dependence among elite Australian athletes. J Sports Sci. 2012; 30 (8):755–766. [ Abstract ] [ Google Scholar ]
- Szabo A, Vega R, Ruiz-Barquín R, Rivera O. Exercise addiction in Spanish athletes: investigation of the roles of gender, social context and level of involvement. J Behav Addict. 2013; 2 (4):249–252. [ Europe PMC free article ] [ Abstract ] [ Google Scholar ]
- Costa S, Hausenblas HA, Oliva P, Cuzzocrea F, Larcan R. Perceived parental psychological control and exercise dependence symptoms in competitive athletes. Int J Ment Health Addiction. 2015; 13 (1):59–72. [ Google Scholar ]
- Lichtenstein MB, Larsen KS, Christiansen E, Støving RK, Bredahl TVG. Exercise addiction in team sport and individual sport: prevalences and validation of the exercise addiction inventory. Addiction Research & Theory. 2014; 22 (5):431–437. [ Google Scholar ]
Citations & impact
Impact metrics, citations of article over time, alternative metrics.

Article citations
Psychometric properties of the exercise addiction inventory (eai) questionnaire among physically active young adults..
Khoshro S , Farhangi MA , Jahangiry L
BMC Res Notes , 17(1):76, 14 Mar 2024
Cited by: 0 articles | PMID: 38486222 | PMCID: PMC10941438
Sporting Mind: The Interplay of Physical Activity and Psychological Health.
Martín-Rodríguez A , Gostian-Ropotin LA , Beltrán-Velasco AI , Belando-Pedreño N , Simón JA , López-Mora C , Navarro-Jiménez E , Tornero-Aguilera JF , Clemente-Suárez VJ
Sports (Basel) , 12(1):37, 22 Jan 2024
Cited by: 0 articles | PMID: 38275986 | PMCID: PMC10819297
The relationship between exercise addiction, eating disorders, and insecure attachment styles among recreational exercisers.
Lev Arey D , Sagi A , Blatt A
J Eat Disord , 11(1):131, 07 Aug 2023
Cited by: 1 article | PMID: 37550767 | PMCID: PMC10408237
Racial and Ethnic Disparities in Weight Loss Behaviors Among US College Students.
Van Dyne A , Washington N , Villodas M , Cronan T
Psychol Res Behav Manag , 16:857-873, 16 Mar 2023
Cited by: 1 article | PMID: 36960414 | PMCID: PMC10028297
The Expanded Exercise Addiction Inventory (EAI-3): Towards Reliable and International Screening of Exercise-Related Dysfunction.
Granziol U , Griffiths MD , Zou L , Yang P , Herschel HK , Junker A , Akimoto T , Stoll O , Alpay M , Aydın Z , Zandonai T , Di Lodovico L , Lichtenstein MB , Trott M , Portman RM , Schipfer M , Cook B , Cerea S , Egorov AY , [...] Szabo A
Int J Ment Health Addict , 1-27, 10 May 2023
Cited by: 0 articles | PMID: 37363769 | PMCID: PMC10171173
Similar Articles
To arrive at the top five similar articles we use a word-weighted algorithm to compare words from the Title and Abstract of each citation.
Exercise Addiction in Athletes and Leisure Exercisers: The Moderating Role of Passion.
de la Vega R , Parastatidou IS , Ruíz-Barquín R , Szabo A
J Behav Addict , 5(2):325-331, 01 Jun 2016
Cited by: 25 articles | PMID: 27363466 | PMCID: PMC5387784
Exercise addiction in adolescents and emerging adults - Validation of a youth version of the Exercise Addiction Inventory.
Lichtenstein MB , Griffiths MD , Hemmingsen SD , Støving RK
J Behav Addict , 7(1):117-125, 07 Feb 2018
Cited by: 16 articles | PMID: 29409340 | PMCID: PMC6035018
The Prevalence of Exercise Addiction Symptoms in a Sample of National Level Elite Athletes.
Lichtenstein MB , Melin AK , Szabo A , Holm L
Front Sports Act Living , 3:635418, 10 Jun 2021
Cited by: 9 articles | PMID: 34179773 | PMCID: PMC8222598
Which sports are more at risk of physical exercise addiction: A systematic review.
Di Lodovico L , Poulnais S , Gorwood P
Addict Behav , 93:257-262, 23 Dec 2018
Cited by: 31 articles | PMID: 30595420
Identifying the features of an exercise addiction: A Delphi study.
Macfarlane L , Owens G , Cruz Bdel P
J Behav Addict , 5(3):474-484, 24 Aug 2016
Cited by: 20 articles | PMID: 27554504 | PMCID: PMC5264415
Exercise addiction: symptoms, diagnosis, epidemiology, and etiology.
Berczik K , Szabó A , Griffiths MD , Kurimay T , Kun B , Urbán R , Demetrovics Z
Subst Use Misuse , 47(4):403-417, 04 Jan 2012
Cited by: 102 articles | PMID: 22216780
Europe PMC is part of the ELIXIR infrastructure
April 4, 2024

MEDIA ALERT
WHAT: "The Comfort of Recovery" quilt month-long installation and special event on Tuesday, April 9.
Art meets healing in a powerful display of resilience and recovery as the Addiction Alliance of Georgia hosts the installation of the “Comfort of Recovery" quilt throughout the month of April at the Emory Addiction Center. The captivating quilt, handcrafted by members of R2ISE to Recovery, a nonprofit recovery community organization, embodies the transformative power of connection, recovery and storytelling.
WHO: Addiction Alliance of Georgia , a partnership between Emory Healthcare and the Hazelden Betty Ford Foundation
WHEN: Installation Dates: April 1–31, 2024
Special Event: April 9; 5:30 p.m.: An evening of artistic expression and demonstration of the power of recovery, presented by R2ISE to Recovery. The “Comfort of Recovery” quilt will be on display. Light refreshments served. Please RSVP by Monday, April 8, to John Martin [email protected] .
WHERE: Emory Addiction Center (EAC), Emory Wesley Woods Hospital, 1821 Clifton Road, Suite 1200, Atlanta, GA 30329. EAC is an outpatient substance use and mental health care facility operated by the Addiction Alliance of Georgia .
ABOUT THE QUILT: Inspired by a vision to merge art and recovery, Alexia Jones, founder of R2ISE, embarked on a journey to create a tangible representation of healing. The quilt, adorned with a tree motif, symbolizes the seasons of change and interconnectedness of the recovery community. With 100 people and 200 hands contributing, its creation has united individuals of all ages, genders, races and backgrounds, fostering healing and support.
THE QUILT’S JOURNEY: From its inception, the quilt has traveled across the region sparking awareness and fostering new conversations about recovery. Displayed in various locations such as the Governor’s Office and medical schools, it serves as a beacon of hope and solidarity.
MORE INFO : This Q&A: The Comfort of Recovery. A Q&A with Alexia Jones of R2ISE is featured in Hazelden Betty Ford’s monthly Recovery.
- Brain Health Center
- News Releases
Recent News
Download emory news photo.
By downloading Emory news media, you agree to the following terms of use:
Creative Commons Attribution-NoDerivatives 4.0 International Public License
By exercising the Licensed Rights (defined below), You accept and agree to be bound by the terms and conditions of this Creative Commons Attribution-NoDerivatives 4.0 International Public License ("Public License"). To the extent this Public License may be interpreted as a contract, You are granted the Licensed Rights in consideration of Your acceptance of these terms and conditions, and the Licensor grants You such rights in consideration of benefits the Licensor receives from making the Licensed Material available under these terms and conditions.
Section 1 – Definitions.
- Adapted Material means material subject to Copyright and Similar Rights that is derived from or based upon the Licensed Material and in which the Licensed Material is translated, altered, arranged, transformed, or otherwise modified in a manner requiring permission under the Copyright and Similar Rights held by the Licensor. For purposes of this Public License, where the Licensed Material is a musical work, performance, or sound recording, Adapted Material is always produced where the Licensed Material is synched in timed relation with a moving image.
- Copyright and Similar Rights means copyright and/or similar rights closely related to copyright including, without limitation, performance, broadcast, sound recording, and Sui Generis Database Rights, without regard to how the rights are labeled or categorized. For purposes of this Public License, the rights specified in Section 2(b)(1)-(2) are not Copyright and Similar Rights.
- Effective Technological Measures means those measures that, in the absence of proper authority, may not be circumvented under laws fulfilling obligations under Article 11 of the WIPO Copyright Treaty adopted on December 20, 1996, and/or similar international agreements.
- Exceptions and Limitations means fair use, fair dealing, and/or any other exception or limitation to Copyright and Similar Rights that applies to Your use of the Licensed Material.
- Licensed Material means the artistic or literary work, database, or other material to which the Licensor applied this Public License.
- Licensed Rights means the rights granted to You subject to the terms and conditions of this Public License, which are limited to all Copyright and Similar Rights that apply to Your use of the Licensed Material and that the Licensor has authority to license.
- Licensor means the individual(s) or entity(ies) granting rights under this Public License.
- Share means to provide material to the public by any means or process that requires permission under the Licensed Rights, such as reproduction, public display, public performance, distribution, dissemination, communication, or importation, and to make material available to the public including in ways that members of the public may access the material from a place and at a time individually chosen by them.
- Sui Generis Database Rights means rights other than copyright resulting from Directive 96/9/EC of the European Parliament and of the Council of 11 March 1996 on the legal protection of databases, as amended and/or succeeded, as well as other essentially equivalent rights anywhere in the world.
- You means the individual or entity exercising the Licensed Rights under this Public License. Your has a corresponding meaning.
Section 2 – Scope.
- reproduce and Share the Licensed Material, in whole or in part; and
- produce and reproduce, but not Share, Adapted Material.
- Exceptions and Limitations . For the avoidance of doubt, where Exceptions and Limitations apply to Your use, this Public License does not apply, and You do not need to comply with its terms and conditions.
- Term . The term of this Public License is specified in Section 6(a) .
- Media and formats; technical modifications allowed . The Licensor authorizes You to exercise the Licensed Rights in all media and formats whether now known or hereafter created, and to make technical modifications necessary to do so. The Licensor waives and/or agrees not to assert any right or authority to forbid You from making technical modifications necessary to exercise the Licensed Rights, including technical modifications necessary to circumvent Effective Technological Measures. For purposes of this Public License, simply making modifications authorized by this Section 2(a)(4) never produces Adapted Material.
- Offer from the Licensor – Licensed Material . Every recipient of the Licensed Material automatically receives an offer from the Licensor to exercise the Licensed Rights under the terms and conditions of this Public License.
- No downstream restrictions . You may not offer or impose any additional or different terms or conditions on, or apply any Effective Technological Measures to, the Licensed Material if doing so restricts exercise of the Licensed Rights by any recipient of the Licensed Material.
- No endorsement . Nothing in this Public License constitutes or may be construed as permission to assert or imply that You are, or that Your use of the Licensed Material is, connected with, or sponsored, endorsed, or granted official status by, the Licensor or others designated to receive attribution as provided in Section 3(a)(1)(A)(i) .
Other rights .
- Moral rights, such as the right of integrity, are not licensed under this Public License, nor are publicity, privacy, and/or other similar personality rights; however, to the extent possible, the Licensor waives and/or agrees not to assert any such rights held by the Licensor to the limited extent necessary to allow You to exercise the Licensed Rights, but not otherwise.
- Patent and trademark rights are not licensed under this Public License.
- To the extent possible, the Licensor waives any right to collect royalties from You for the exercise of the Licensed Rights, whether directly or through a collecting society under any voluntary or waivable statutory or compulsory licensing scheme. In all other cases the Licensor expressly reserves any right to collect such royalties.
Section 3 – License Conditions.
Your exercise of the Licensed Rights is expressly made subject to the following conditions.
Attribution .
If You Share the Licensed Material, You must:
- identification of the creator(s) of the Licensed Material and any others designated to receive attribution, in any reasonable manner requested by the Licensor (including by pseudonym if designated);
- a copyright notice;
- a notice that refers to this Public License;
- a notice that refers to the disclaimer of warranties;
- a URI or hyperlink to the Licensed Material to the extent reasonably practicable;
- indicate if You modified the Licensed Material and retain an indication of any previous modifications; and
- indicate the Licensed Material is licensed under this Public License, and include the text of, or the URI or hyperlink to, this Public License.
- You may satisfy the conditions in Section 3(a)(1) in any reasonable manner based on the medium, means, and context in which You Share the Licensed Material. For example, it may be reasonable to satisfy the conditions by providing a URI or hyperlink to a resource that includes the required information.
- If requested by the Licensor, You must remove any of the information required by Section 3(a)(1)(A) to the extent reasonably practicable.
Section 4 – Sui Generis Database Rights.
Where the Licensed Rights include Sui Generis Database Rights that apply to Your use of the Licensed Material:
- for the avoidance of doubt, Section 2(a)(1) grants You the right to extract, reuse, reproduce, and Share all or a substantial portion of the contents of the database, provided You do not Share Adapted Material;
- if You include all or a substantial portion of the database contents in a database in which You have Sui Generis Database Rights, then the database in which You have Sui Generis Database Rights (but not its individual contents) is Adapted Material; and
- You must comply with the conditions in Section 3(a) if You Share all or a substantial portion of the contents of the database.
Section 5 – Disclaimer of Warranties and Limitation of Liability.
- Unless otherwise separately undertaken by the Licensor, to the extent possible, the Licensor offers the Licensed Material as-is and as-available, and makes no representations or warranties of any kind concerning the Licensed Material, whether express, implied, statutory, or other. This includes, without limitation, warranties of title, merchantability, fitness for a particular purpose, non-infringement, absence of latent or other defects, accuracy, or the presence or absence of errors, whether or not known or discoverable. Where disclaimers of warranties are not allowed in full or in part, this disclaimer may not apply to You.
- To the extent possible, in no event will the Licensor be liable to You on any legal theory (including, without limitation, negligence) or otherwise for any direct, special, indirect, incidental, consequential, punitive, exemplary, or other losses, costs, expenses, or damages arising out of this Public License or use of the Licensed Material, even if the Licensor has been advised of the possibility of such losses, costs, expenses, or damages. Where a limitation of liability is not allowed in full or in part, this limitation may not apply to You.
- The disclaimer of warranties and limitation of liability provided above shall be interpreted in a manner that, to the extent possible, most closely approximates an absolute disclaimer and waiver of all liability.
Section 6 – Term and Termination.
- This Public License applies for the term of the Copyright and Similar Rights licensed here. However, if You fail to comply with this Public License, then Your rights under this Public License terminate automatically.
Where Your right to use the Licensed Material has terminated under Section 6(a) , it reinstates:
- automatically as of the date the violation is cured, provided it is cured within 30 days of Your discovery of the violation; or
- upon express reinstatement by the Licensor.
- For the avoidance of doubt, the Licensor may also offer the Licensed Material under separate terms or conditions or stop distributing the Licensed Material at any time; however, doing so will not terminate this Public License.
- Sections 1 , 5 , 6 , 7 , and 8 survive termination of this Public License.
Section 7 – Other Terms and Conditions.
- The Licensor shall not be bound by any additional or different terms or conditions communicated by You unless expressly agreed.
- Any arrangements, understandings, or agreements regarding the Licensed Material not stated herein are separate from and independent of the terms and conditions of this Public License.
Section 8 – Interpretation.
- For the avoidance of doubt, this Public License does not, and shall not be interpreted to, reduce, limit, restrict, or impose conditions on any use of the Licensed Material that could lawfully be made without permission under this Public License.
- To the extent possible, if any provision of this Public License is deemed unenforceable, it shall be automatically reformed to the minimum extent necessary to make it enforceable. If the provision cannot be reformed, it shall be severed from this Public License without affecting the enforceability of the remaining terms and conditions.
- No term or condition of this Public License will be waived and no failure to comply consented to unless expressly agreed to by the Licensor.
- Nothing in this Public License constitutes or may be interpreted as a limitation upon, or waiver of, any privileges and immunities that apply to the Licensor or You, including from the legal processes of any jurisdiction or authority.
Creative Commons is not a party to its public licenses. Notwithstanding, Creative Commons may elect to apply one of its public licenses to material it publishes and in those instances will be considered the “Licensor.” The text of the Creative Commons public licenses is dedicated to the public domain under the CC0 Public Domain Dedication . Except for the limited purpose of indicating that material is shared under a Creative Commons public license or as otherwise permitted by the Creative Commons policies published at creativecommons.org/policies , Creative Commons does not authorize the use of the trademark “Creative Commons” or any other trademark or logo of Creative Commons without its prior written consent including, without limitation, in connection with any unauthorized modifications to any of its public licenses or any other arrangements, understandings, or agreements concerning use of licensed material. For the avoidance of doubt, this paragraph does not form part of the public licenses.

New insight into combating drug-resistant prostate cancer
New research from the University of Eastern Finland sheds light on the significance of the glucocorticoid receptor in drug-resistant prostate cancer, showing that the development of drug resistance could be prevented by limiting the activity of coregulator proteins.
Glucocorticoids regulate vital biological processes by affecting gene encoding through a DNA-binding transcription factor, namely the glucocorticoid receptor. The activity of the glucocorticoid receptor is made extensive use of in medicine because glucocorticoids have a strong anti-inflammatory effect. For this reason, synthetic glucocorticoids are one of the most prescribed drugs in the world. They are used to treat inflammatory diseases, such as rheumatoid arthritis, and as adjuvant therapy for cancer patients to alleviate the side effects of cancer therapy. In blood cancer, glucocorticoids are important drugs that limit the growth of cancer cells.
However, recent studies have shown that the glucocorticoid receptor also has an oncogenic, or cancer-promoting, effect in cancers like breast and prostate cancer. In prostate cancer, the glucocorticoid receptor can replace the activity of the androgen receptor, which is main oncogenic factor in this cancer, when its activity is inhibited by drug therapy. Thus, glucocorticoids help prostate cancer develop resistance to drug therapy.
"Due to these drug resistance and cancer-promoting effects, it is important to study how the glucocorticoid receptor functions on the cellular and molecular level in cancer," Academy Research Fellow, Docent Ville Paakinaho of the University of Eastern Finland notes.
The Paakinaho Lab has published two recent genome-wide deep sequencing studies on the subject. The first, published in Nucleic Acids Research , explored how the glucocorticoid receptor replaces the androgen receptor on the molecular level.
"This study showed that the glucocorticoid receptor can only use regulatory regions that are already active in prostate cancer cells," says Doctoral Researcher Laura Helminen of the University of Eastern Finland.
In other words, glucocorticoid receptor-mediated drug resistance emerges through these regulatory regions, and by affecting the activity of these areas, the harmful effects of glucocorticoids in prostate cancer could be prevented. Bioinformatics analyses indicated the pioneer transcription factor FOXA1 as one possible target. FOXA1 is known to have cancer-promoting properties, which is why the researchers assumed that inhibiting its activity would limit the development of glucocorticoid receptor-mediated drug-resistant prostate cancer. Surprisingly however, the effect was exactly the opposite: inhibiting the activity of FOXA1 further increased the activity of the glucocorticoid receptor -- and the development of drug resistance.
This is because FOXA1 was found to be involved in the silencing of the glucocorticoid receptor gene, and this is what increased its activity when FOXA1 was inhibited.
"Research often reveals the unexpected, and that's part of its charm," Paakinaho says.
The activity of the glucocorticoid receptor in regulatory regions can, however, be influenced in drug-resistant prostate cancer through an alternative pathway. Coregulator proteins were identified as an alternative target through which the glucocorticoid receptor affects the regulation of gene expression. These proteins include EP300 and CREBBP. Several pharmaceutical companies are developing small-molecule inhibitors targeting these proteins, and some are already being studied in patients.
In another study by the Paakinaho Lab, the researchers explored ways to inhibit glucocorticoid receptor-mediated effects by inhibiting coregulator proteins. These findings were reported in Cellular and Molecular Life Sciences.
"Silencing the EP300 and CREBBP proteins with a small-molecule inhibitor clearly prevented the activity of the glucocorticoid receptor in prostate cancer cells," Project Researcher Jasmin Huttunen of the University of Eastern Finland says.
This allowed the growth of drug-resistant prostate cancer cells to be inhibited. Furthermore, the researchers found that silencing EP300 and CREBBP also effectively inhibited the activity of the androgen receptor especially in prostate cancer cells that have an amplification of the androgen receptor gene. This amplification is found in up to half of patients with advanced prostate cancer.
Surprisingly, the EP300 and CREBBP inhibitor also inhibited the activity of FOXA1, while still preserving its ability to silence the expression of the glucocorticoid receptor gene. By using the EP300 and CREBBP inhibitor, it was possible to block the activity of FOXA1 without the development of glucocorticoid receptor-mediated drug resistance. Ultimately, inhibiting the activity of both the androgen and the glucocorticoid receptor was found to be primarily due to the limitation of FOXA1 activity. The study suggests that treatment targeting coregulator proteins could also be effective in untreated prostate cancer.
The studies were funded by the Research Council of Finland, the Sigrid Jusélius Foundation, and the Cancer Foundation Finland.
- Prostate Cancer
- Men's Health
- Breast Cancer
- Lung Cancer
- Personalized Medicine
- Diseases and Conditions
- Prostate cancer
- Drug discovery
- Personalized medicine
- Pharmaceutical company
- Drug addiction
- Antibiotic resistance
Story Source:
Materials provided by University of Eastern Finland . Note: Content may be edited for style and length.
Journal References :
- Jasmin Huttunen, Niina Aaltonen, Laura Helminen, Kirsi Rilla, Ville Paakinaho. EP300/CREBBP acetyltransferase inhibition limits steroid receptor and FOXA1 signaling in prostate cancer cells . Cellular and Molecular Life Sciences , 2024; 81 (1) DOI: 10.1007/s00018-024-05209-z
- Laura Helminen, Jasmin Huttunen, Melina Tulonen, Niina Aaltonen, Einari A Niskanen, Jorma J Palvimo, Ville Paakinaho. Chromatin accessibility and pioneer factor FOXA1 restrict glucocorticoid receptor action in prostate cancer . Nucleic Acids Research , 2024; 52 (2): 625 DOI: 10.1093/nar/gkad1126
Cite This Page :
Explore More
- Ozone Removes Mating Barriers Between Fly ...
- Parkinson's: New Theory On Origins and Spread
- Clash of Stars Solves Stellar Mystery
- Secure Quantum Computing at Home
- Ocean Currents: Collapse of Antarctic Ice ...
- Pacific Cities Much Older Than Previously ...
- The Milky Way in Ancient Egyptian Mythology
- Physical Activity Best in the Evening
- How the Body Switches out of 'Fight' Mode
- New Drug Prevents Flu-Related Lung Damage
Trending Topics
Strange & offbeat.

Making Ultra-fast Electron Measurements in Multiple Directions to Reveal the Secrets of the Aurora
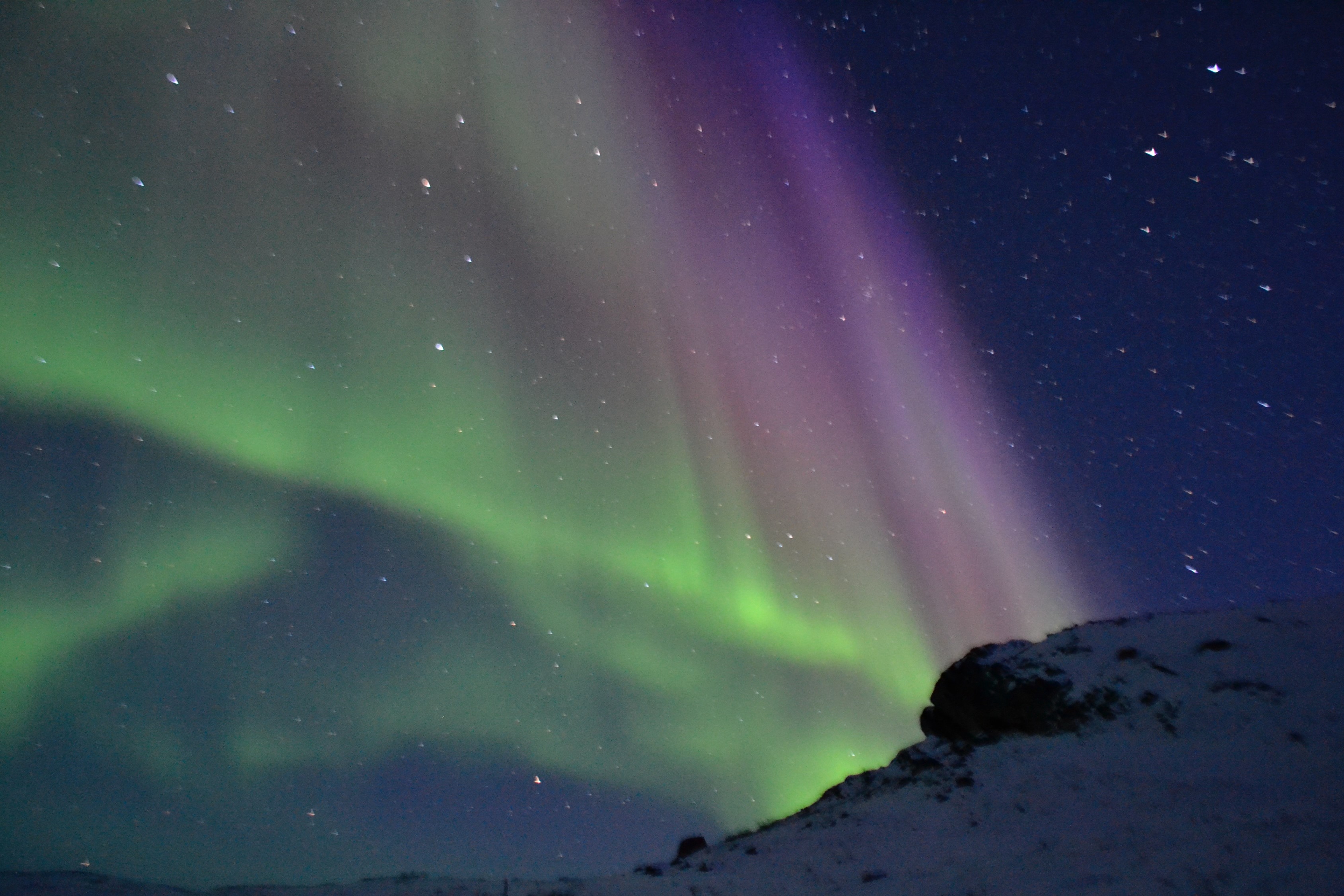
The energetic electrons that drive the aurora borealis (the northern lights) have a rich and very dynamic structure that we currently do not fully understand. Much of what we know about these electrons comes from instruments that have fundamental limitations in their ability to sample multiple energies with high time resolution. To overcome these limitations, NASA is using an innovative approach to develop instrumentation that will enhance our measurement capabilities by more than an order of magnitude—revealing a wealth of new information about the amazing physics happening within the aurora.
Typical electron instruments rely on a technique called electrostatic deflection, which requires changing a voltage to select different energies of electrons to measure. These instruments have been flown on many different space missions and have provided almost all of the in-situ electron measurements made inside the aurora. They work great when observing on timescales of seconds or even down to around a tenth of a second, but they fundamentally cannot observe down to smaller (millisecond) timescales due to the time it takes to sweep through voltages.
Ground-based optical observations of the aurora have shown that there can be rapid spatial and temporal variations that are beyond the observing capabilities of traditional electron instruments. Therefore, members of the Geophysics Laboratory at NASA’s Goddard Space Flight Center developed an instrument called the Acute Precipitating Electron Spectrometer (APES) that can measure electron precipitation within the aurora at a one millisecond cadence. APES uses a strong magnetic field inside the instrument to separate electrons with different energies onto different spatial regions of the detector. This method allows the instrument to measure the entire electron energy spectrum simultaneously at a very high rate (every 1 ms).
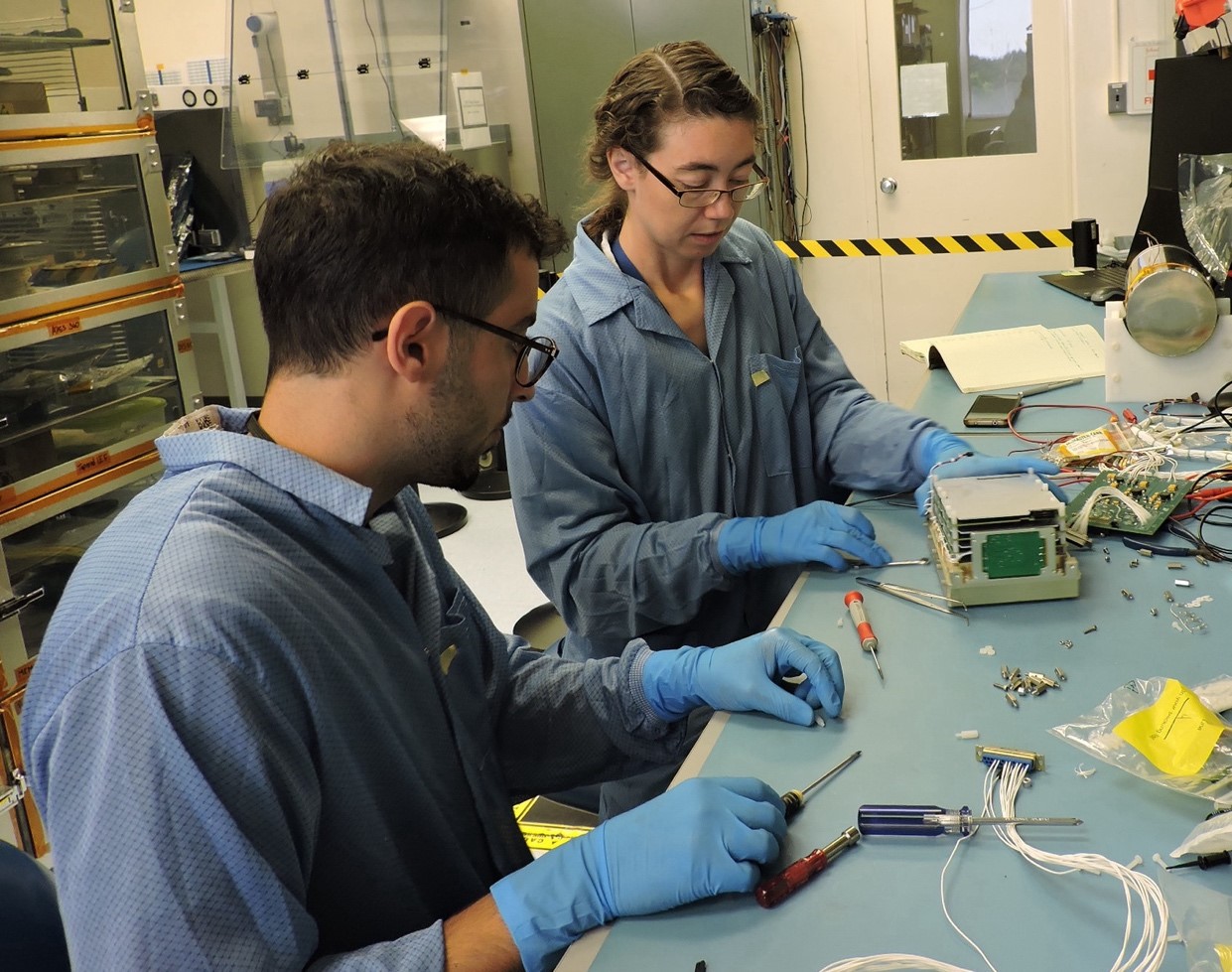
In the design of APES, one major trade-off had to be made. For the magnetic field geometry to work properly, the instrument can only observe in one direction. This concept works well if the goal is just to measure the precipitating (downgoing) electrons in the aurora that ultimately hit the atmosphere. However, we know that electrons in the aurora also move in other directions; in fact, these electrons contain a lot of information about other physical processes happening farther out in space.
To enable measurement of electrons in more than one direction, the Goddard team developed the APES-360 instrument concept. To create the APES-360 design, the team employed the same operating principles used in APES, but updated them to accommodate a multi-look direction geometry that covers a 360-degree field of view using 16 different sectors. The team had to overcome several technical challenges to develop the APES-360 concept. In particular, the electronics design had to accommodate many more anodes (charge detecting surfaces) and the associated circuitry in a small area.
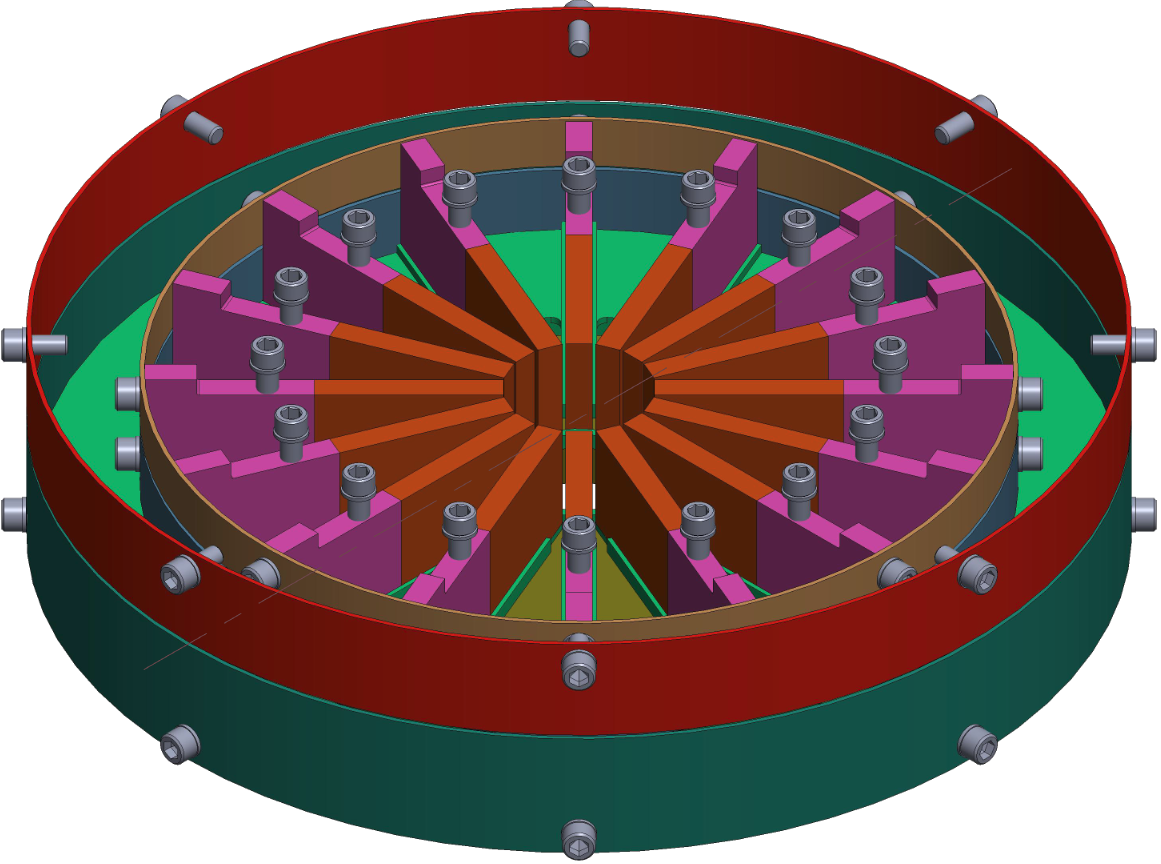
The APES-360 prototype that is currently being built will be tested and calibrated at Goddard and will fly on a sounding rocket into active aurora in the winter of 2025. This flight will provide real-life data from inside the aurora that will be used to validate the instrument performance and inform future design improvements.
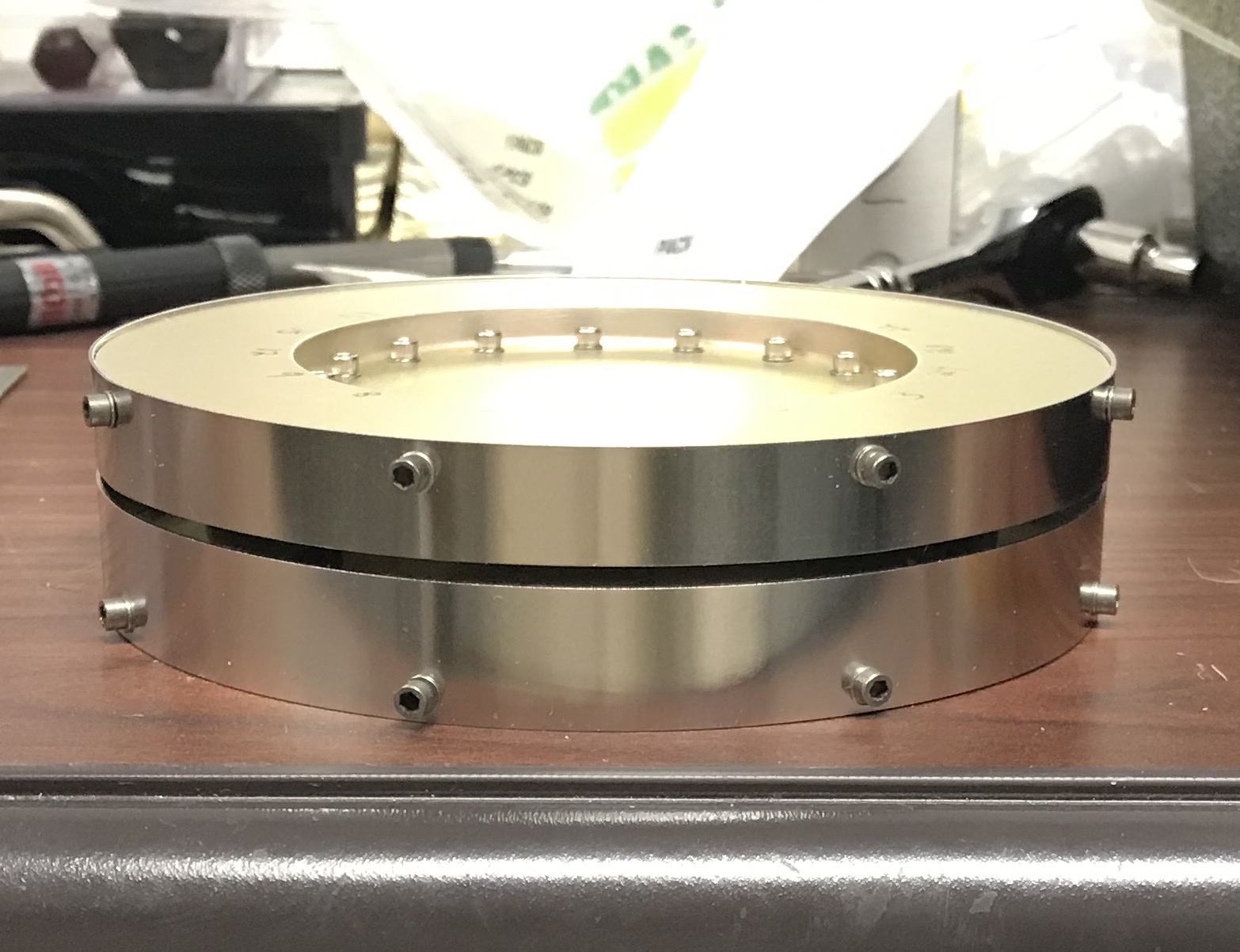
The APES-360 instrument is being designed to fit into a CubeSat form factor so that it can be used on future CubeSat missions to study the aurora. The instrument could also ultimately be flown on larger orbital missions, as well.
PROJECT LEAD:
Dr. Robert G Michell, NASA Goddard Space Flight Center (GSFC)
SPONSORING ORGANIZATIONS:
Heliophysics, Geospace Physics Laboratory (GSFC Code 673) and H-TIDeS.
Related Terms
- Heliophysics
- Science-enabling Technology
- Technology Highlights

IMAGES
VIDEO
COMMENTS
The use of IPSCs or ESCs to recapitulate the human brain in vitro could improve upon some of the current limitations of addiction modelling and may provide a platform for practical and reproducible addiction research through forming structurally, biologically, and genetically relevant in vitro environments. Combining this method with ...
This review discusses theories of addiction aetiology, available models for addiction research and the strengths and limitations of current practical experimental methods of study.
Such research has allowed us to identify structures and pathways that contribute to the neuropathology of addiction (Lynch Reference Lynch, Nicholson and Dance 2010). However, there are limitations to animal models, such as modelling vulnerability factors and complex addictive behaviours.
Alcohol and drug use are among the top ten causes of preventable death in the United States [1,2,3,4], but substance use disorders (SUDs) are greatly under-treated in the specialty addiction treatment system [], and under-recognized in medical settings [6, 7].Screening for alcohol use in adult primary care settings is recommended by the United States Preventive Services Task Force (USPSTF) and ...
As this work revealed, Dweck's mindset theory applied to addiction is still a nascent field, and the research scarce. Only 18 studies were included in the final review, among the 1903 unique citations screened. The majority of the included research were published between 2015 and 2022 (16 out of 18).
Methodological and Conceptual Limitations in Exercise Addiction Research. The aim of this brief analytical review is to highlight and disentangle research dilemmas in the field of exercise addiction. Research examining exercise addiction is primarily based on self-reports, obtained by questionnaires (incorporating psychometrically validated ...
Of the 291 papers scrutinized, only 17 (6%) reported on studies that had wholly or partially used qualitative methods. In Addiction, the proportion (at 2%) was even lower. Addiction published just three qualitative papers in 2004: Amos et al. (2004 ); Furst et al. (2004 ); and Rhodes, Davis & Judd (2004 ). Such marginality prompts uncomfortable ...
Objective: Models of addiction often posit bidirectional and dynamic associations between constructs relevant to the etiology and maintenance of addictive behaviors. The cross-lagged panel model (CLPM) is commonly used in addiction research but has been critiqued for not appropriately adjusting for between-person variance. Alternatives to the CLPM have been suggested but remain underutilized.
The present paper examines the: 1) discrepancies in the classification of exercise addiction; 2) inconsistent reporting of exercise addiction prevalence; and 3) varied interpretation of exercise ...
A lack of cross-disciplinary unanimity prevails within addiction research. Theories conceptualizing addiction through the three-stage brain disease model contest other theories that substance use disorder is of behavioural or genetic origin. The reverberations of this lack of consensus are noticeable throughout addiction research and within the foundations of disease modelling.
1. Introduction. Over the past few years, digital addiction (DA) has emerged as a significant research area due to its increasing prevalence. The prevalence of DA differs globally, varying between 8.90% in Eastern countries and 4.60% in Western countries [1].Currently, there is a lack of consensus on defining DA, including what term to use to identify it.
It is concluded that there is the need for consistent terminology, to follow-up results derived from exercise addiction instruments with interviews, and to follow a theory-driven rationale in this area of research. The aim of this brief analytical review is to highlight and disentangle research dilemmas in the field of exercise addiction. Research examining exercise addiction is primarily ...
Thus, there are still questions that remain to be addressed and specific areas warranting further research in this context. Previous reviews on PGB focused on identifying key limitations in terms of etiology and predictors (Kuss and Griffiths, 2012a), prevalence rates (Ferguson et al., 2011) and assessment (King et al., 2013; Pontes et al., 2017).
Limitations in Research. Limitations in research refer to the factors that may affect the results, conclusions, and generalizability of a study.These limitations can arise from various sources, such as the design of the study, the sampling methods used, the measurement tools employed, and the limitations of the data analysis techniques.
Limitations. There are several limitations in the present study. Firstly, the present study selected as many candidate influencing factors as possible for sleep quality, such as family support, social support, personal lifestyle, physical health, mental health, mobile phone addiction, and psychological resilience.
A Rutgers researcher has teamed up with a local high school student to build a machine-learning approach for screening current and existing drugs to determine which could potentially become effective treatments for addiction-related disorders.
Addiction is an SSA journal publishing peer-reviewed research reports on pharmalogical and behavioural addictions spanning many different disciplines. Abstract Background and aims Xylazine is a non-opioid sedative which has spread rapidly throughout the US illicit drug supply. ... LIMITATIONS. Prior to the widespread reporting in May 2023 of ...
Worldwide, someone dies from drug or alcohol addiction every four minutes. Now, researchers at Huntsman Mental Health Institute at University of Utah have been selected by Wellcome Leap to research a new treatment for substance use disorder as part of a $50 million commitment to develop innovative treatments. Brian J. Mickey, MD, PhD, Professor of Psychiatry at Huntsman Mental Health Institute ...
Contact Data CONTACT: ResearchAndMarkets.com Laura Wood,Senior Press Manager [email protected] For E.S.T Office Hours Call 1-917-300-0470 For U.S./ CAN Toll Free Call 1-800-526-8630 For ...
Blaydon and Lindner [ 27] studied 203 triathletes competing at amateur (n = 133) and professional (n = 70) levels. They found that the incidence of exercise addiction ranged from 21.6 percent to 30.4 percent in the whole sample, while among the sub-sample of professional athletes, 41.4 percent were at risk for exercise addiction.
Art meets healing in a powerful display of resilience and recovery as the Addiction Alliance of Georgia hosts the installation of the "Comfort of ... Research ; Science ; Service and Volunteering ... whether express, implied, statutory, or other. This includes, without limitation, warranties of title, merchantability, fitness for a particular ...
The crux of the issue lies in the limitations of current mental ... through voice biomarkers and concentrate their research on youths over age 18. ... opioid addiction should be pulled ...
New research sheds light on the significance of the glucocorticoid receptor in drug-resistant prostate cancer, showing that the development of drug resistance could be prevented by limiting the ...
The energetic electrons that drive the aurora borealis (the northern lights) have a rich and very dynamic structure that we currently do not fully understand. Much of what we know about these electrons comes from instruments that have fundamental limitations in their ability to sample multiple energies with high time resolution. To overcome these limitations, NASA is using an innovative ...