

Case Study vs. Experiment: What's the Difference?

Key Differences
Comparison chart, generalization, preferred fields, case study and experiment definitions, when is a case study used, what is an experiment, how do case studies gather data, can case studies be generalized, what fields commonly use case studies, what type of data do experiments produce, can a case study include quantitative data, what is a case study, what is the main purpose of an experiment, is bias a concern in case studies, are experiments replicable, in which fields are experiments predominant, how do experiments impact scientific knowledge, what is a longitudinal case study, can experiments be conducted in natural settings, what is the role of control in experiments, how long can a case study last, what ethical considerations are involved in experiments, can case studies be used to test hypotheses, how is replicability ensured in experiments.

Trending Comparisons
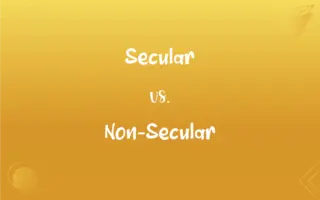
Popular Comparisons
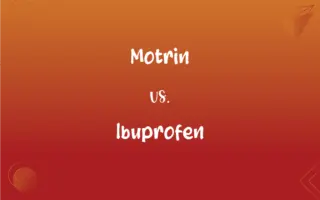
New Comparisons

- En español – ExME
- Em português – EME
An introduction to different types of study design
Posted on 6th April 2021 by Hadi Abbas
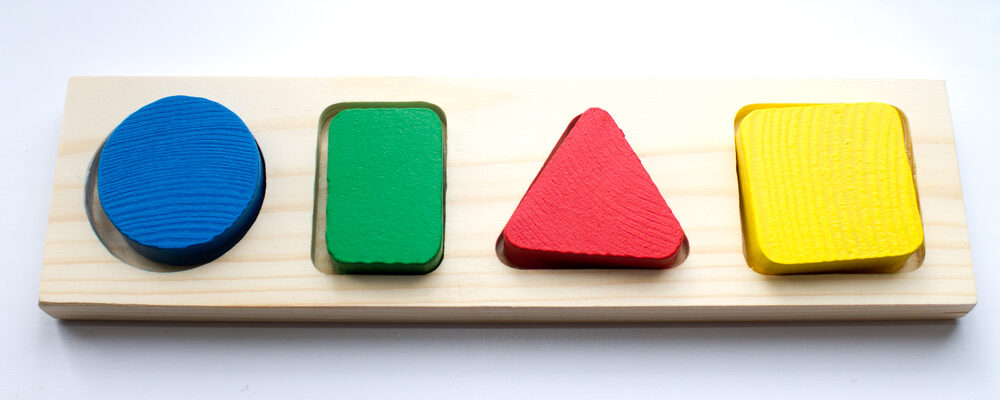
Study designs are the set of methods and procedures used to collect and analyze data in a study.
Broadly speaking, there are 2 types of study designs: descriptive studies and analytical studies.
Descriptive studies
- Describes specific characteristics in a population of interest
- The most common forms are case reports and case series
- In a case report, we discuss our experience with the patient’s symptoms, signs, diagnosis, and treatment
- In a case series, several patients with similar experiences are grouped.
Analytical Studies
Analytical studies are of 2 types: observational and experimental.
Observational studies are studies that we conduct without any intervention or experiment. In those studies, we purely observe the outcomes. On the other hand, in experimental studies, we conduct experiments and interventions.
Observational studies
Observational studies include many subtypes. Below, I will discuss the most common designs.
Cross-sectional study:
- This design is transverse where we take a specific sample at a specific time without any follow-up
- It allows us to calculate the frequency of disease ( p revalence ) or the frequency of a risk factor
- This design is easy to conduct
- For example – if we want to know the prevalence of migraine in a population, we can conduct a cross-sectional study whereby we take a sample from the population and calculate the number of patients with migraine headaches.
Cohort study:
- We conduct this study by comparing two samples from the population: one sample with a risk factor while the other lacks this risk factor
- It shows us the risk of developing the disease in individuals with the risk factor compared to those without the risk factor ( RR = relative risk )
- Prospective : we follow the individuals in the future to know who will develop the disease
- Retrospective : we look to the past to know who developed the disease (e.g. using medical records)
- This design is the strongest among the observational studies
- For example – to find out the relative risk of developing chronic obstructive pulmonary disease (COPD) among smokers, we take a sample including smokers and non-smokers. Then, we calculate the number of individuals with COPD among both.
Case-Control Study:
- We conduct this study by comparing 2 groups: one group with the disease (cases) and another group without the disease (controls)
- This design is always retrospective
- We aim to find out the odds of having a risk factor or an exposure if an individual has a specific disease (Odds ratio)
- Relatively easy to conduct
- For example – we want to study the odds of being a smoker among hypertensive patients compared to normotensive ones. To do so, we choose a group of patients diagnosed with hypertension and another group that serves as the control (normal blood pressure). Then we study their smoking history to find out if there is a correlation.
Experimental Studies
- Also known as interventional studies
- Can involve animals and humans
- Pre-clinical trials involve animals
- Clinical trials are experimental studies involving humans
- In clinical trials, we study the effect of an intervention compared to another intervention or placebo. As an example, I have listed the four phases of a drug trial:
I: We aim to assess the safety of the drug ( is it safe ? )
II: We aim to assess the efficacy of the drug ( does it work ? )
III: We want to know if this drug is better than the old treatment ( is it better ? )
IV: We follow-up to detect long-term side effects ( can it stay in the market ? )
- In randomized controlled trials, one group of participants receives the control, while the other receives the tested drug/intervention. Those studies are the best way to evaluate the efficacy of a treatment.
Finally, the figure below will help you with your understanding of different types of study designs.
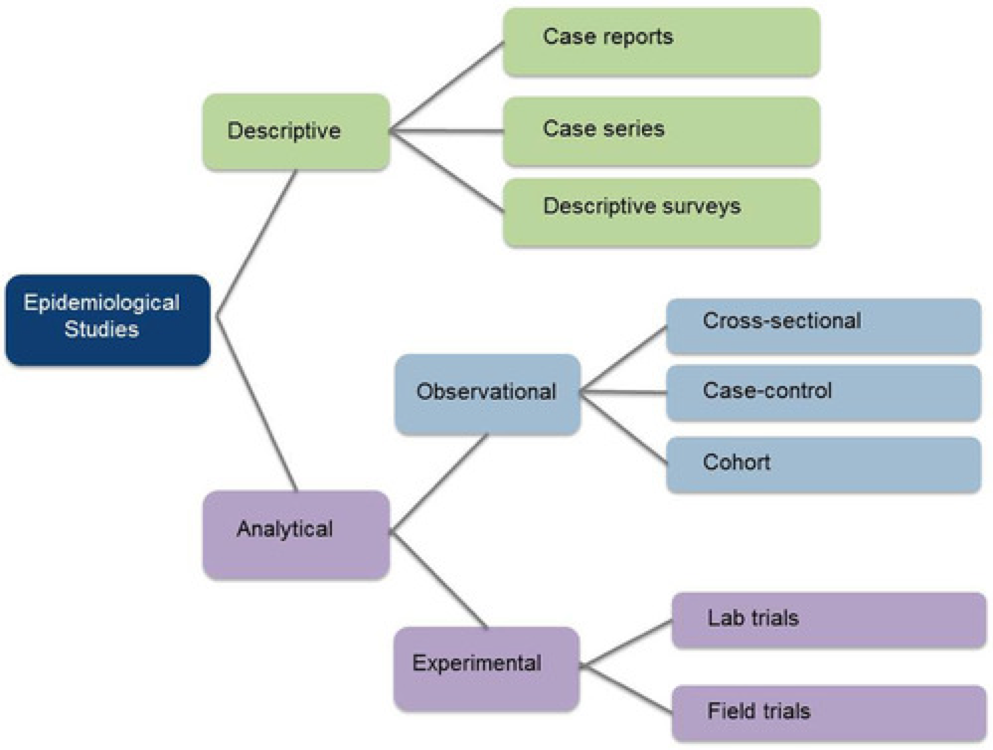
References (pdf)
You may also be interested in the following blogs for further reading:
An introduction to randomized controlled trials
Case-control and cohort studies: a brief overview
Cohort studies: prospective and retrospective designs
Prevalence vs Incidence: what is the difference?
Leave a Reply Cancel reply
Your email address will not be published. Required fields are marked *
Save my name, email, and website in this browser for the next time I comment.
No Comments on An introduction to different types of study design
you are amazing one!! if I get you I’m working with you! I’m student from Ethiopian higher education. health sciences student
Very informative and easy understandable
You are my kind of doctor. Do not lose sight of your objective.
Wow very erll explained and easy to understand
I’m Khamisu Habibu community health officer student from Abubakar Tafawa Balewa university teaching hospital Bauchi, Nigeria, I really appreciate your write up and you have make it clear for the learner. thank you
well understood,thank you so much
Well understood…thanks
Simply explained. Thank You.
Thanks a lot for this nice informative article which help me to understand different study designs that I felt difficult before
That’s lovely to hear, Mona, thank you for letting the author know how useful this was. If there are any other particular topics you think would be useful to you, and are not already on the website, please do let us know.
it is very informative and useful.
thank you statistician
Fabulous to hear, thank you John.
Thanks for this information
Thanks so much for this information….I have clearly known the types of study design Thanks
That’s so good to hear, Mirembe, thank you for letting the author know.
Very helpful article!! U have simplified everything for easy understanding
I’m a health science major currently taking statistics for health care workers…this is a challenging class…thanks for the simified feedback.
That’s good to hear this has helped you. Hopefully you will find some of the other blogs useful too. If you see any topics that are missing from the website, please do let us know!
Hello. I liked your presentation, the fact that you ranked them clearly is very helpful to understand for people like me who is a novelist researcher. However, I was expecting to read much more about the Experimental studies. So please direct me if you already have or will one day. Thank you
Dear Ay. My sincere apologies for not responding to your comment sooner. You may find it useful to filter the blogs by the topic of ‘Study design and research methods’ – here is a link to that filter: https://s4be.cochrane.org/blog/topic/study-design/ This will cover more detail about experimental studies. Or have a look on our library page for further resources there – you’ll find that on the ‘Resources’ drop down from the home page.
However, if there are specific things you feel you would like to learn about experimental studies, that are missing from the website, it would be great if you could let me know too. Thank you, and best of luck. Emma
Great job Mr Hadi. I advise you to prepare and study for the Australian Medical Board Exams as soon as you finish your undergrad study in Lebanon. Good luck and hope we can meet sometime in the future. Regards ;)
You have give a good explaination of what am looking for. However, references am not sure of where to get them from.
Subscribe to our newsletter
You will receive our monthly newsletter and free access to Trip Premium.
Related Articles
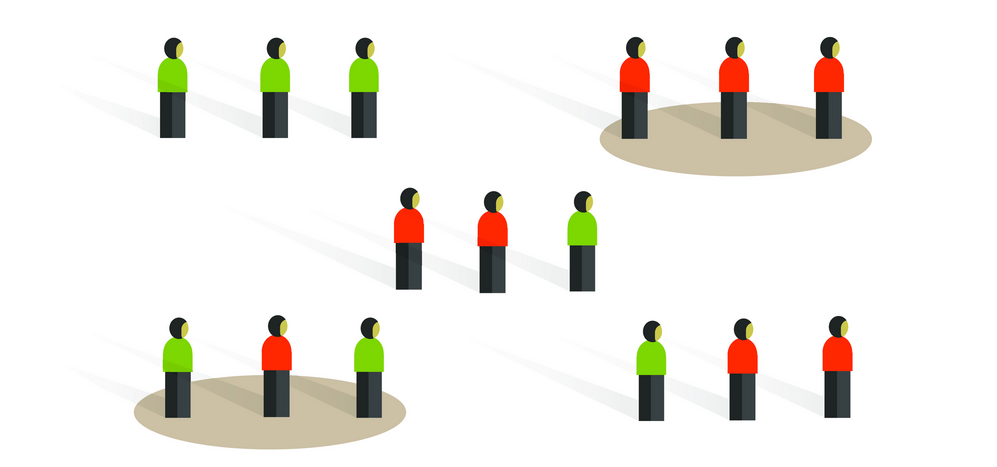
Cluster Randomized Trials: Concepts
This blog summarizes the concepts of cluster randomization, and the logistical and statistical considerations while designing a cluster randomized controlled trial.
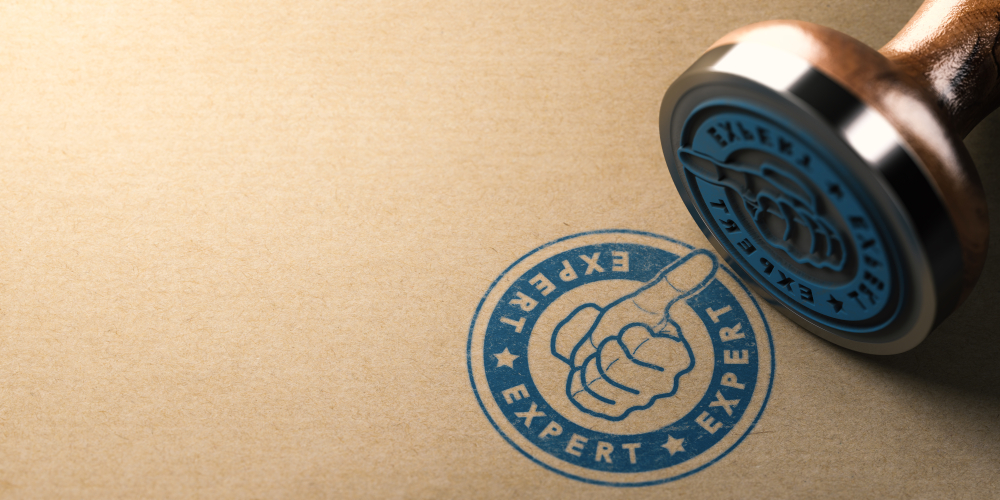
Expertise-based Randomized Controlled Trials
This blog summarizes the concepts of Expertise-based randomized controlled trials with a focus on the advantages and challenges associated with this type of study.
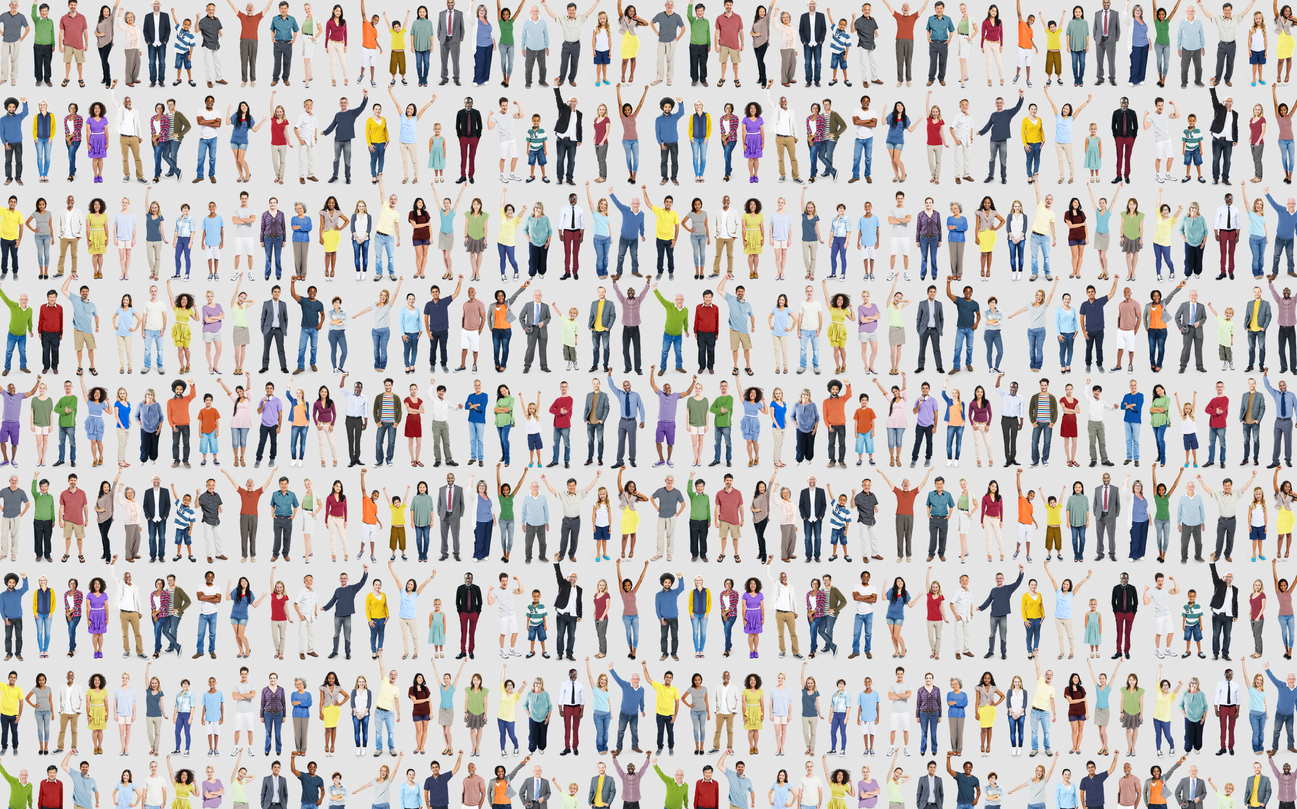
A well-designed cohort study can provide powerful results. This blog introduces prospective and retrospective cohort studies, discussing the advantages, disadvantages and use of these type of study designs.
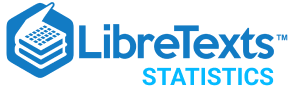
- school Campus Bookshelves
- menu_book Bookshelves
- perm_media Learning Objects
- login Login
- how_to_reg Request Instructor Account
- hub Instructor Commons
- Download Page (PDF)
- Download Full Book (PDF)
- Periodic Table
- Physics Constants
- Scientific Calculator
- Reference & Cite
- Tools expand_more
- Readability
selected template will load here
This action is not available.
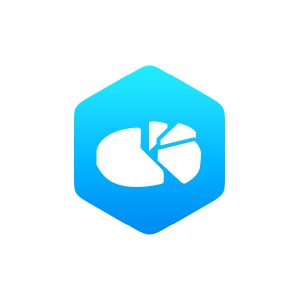
2.5: Experimental and Non-experimental Research
- Last updated
- Save as PDF
- Page ID 3948
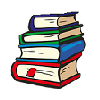
- Danielle Navarro
- University of New South Wales
One of the big distinctions that you should be aware of is the distinction between “experimental research” and “non-experimental research”. When we make this distinction, what we’re really talking about is the degree of control that the researcher exercises over the people and events in the study.
Experimental research
The key features of experimental research is that the researcher controls all aspects of the study, especially what participants experience during the study. In particular, the researcher manipulates or varies the predictor variables (IVs), and then allows the outcome variable (DV) to vary naturally. The idea here is to deliberately vary the predictors (IVs) to see if they have any causal effects on the outcomes. Moreover, in order to ensure that there’s no chance that something other than the predictor variables is causing the outcomes, everything else is kept constant or is in some other way “balanced” to ensure that they have no effect on the results. In practice, it’s almost impossible to think of everything else that might have an influence on the outcome of an experiment, much less keep it constant. The standard solution to this is randomisation: that is, we randomly assign people to different groups, and then give each group a different treatment (i.e., assign them different values of the predictor variables). We’ll talk more about randomisation later in this course, but for now, it’s enough to say that what randomisation does is minimise (but not eliminate) the chances that there are any systematic difference between groups.
Let’s consider a very simple, completely unrealistic and grossly unethical example. Suppose you wanted to find out if smoking causes lung cancer. One way to do this would be to find people who smoke and people who don’t smoke, and look to see if smokers have a higher rate of lung cancer. This is not a proper experiment, since the researcher doesn’t have a lot of control over who is and isn’t a smoker. And this really matters: for instance, it might be that people who choose to smoke cigarettes also tend to have poor diets, or maybe they tend to work in asbestos mines, or whatever. The point here is that the groups (smokers and non-smokers) actually differ on lots of things, not just smoking. So it might be that the higher incidence of lung cancer among smokers is caused by something else, not by smoking per se. In technical terms, these other things (e.g. diet) are called “confounds”, and we’ll talk about those in just a moment.
In the meantime, let’s now consider what a proper experiment might look like. Recall that our concern was that smokers and non-smokers might differ in lots of ways. The solution, as long as you have no ethics, is to control who smokes and who doesn’t. Specifically, if we randomly divide participants into two groups, and force half of them to become smokers, then it’s very unlikely that the groups will differ in any respect other than the fact that half of them smoke. That way, if our smoking group gets cancer at a higher rate than the non-smoking group, then we can feel pretty confident that (a) smoking does cause cancer and (b) we’re murderers.
Non-experimental research
Non-experimental research is a broad term that covers “any study in which the researcher doesn’t have quite as much control as they do in an experiment”. Obviously, control is something that scientists like to have, but as the previous example illustrates, there are lots of situations in which you can’t or shouldn’t try to obtain that control. Since it’s grossly unethical (and almost certainly criminal) to force people to smoke in order to find out if they get cancer, this is a good example of a situation in which you really shouldn’t try to obtain experimental control. But there are other reasons too. Even leaving aside the ethical issues, our “smoking experiment” does have a few other issues. For instance, when I suggested that we “force” half of the people to become smokers, I must have been talking about starting with a sample of non-smokers, and then forcing them to become smokers. While this sounds like the kind of solid, evil experimental design that a mad scientist would love, it might not be a very sound way of investigating the effect in the real world. For instance, suppose that smoking only causes lung cancer when people have poor diets, and suppose also that people who normally smoke do tend to have poor diets. However, since the “smokers” in our experiment aren’t “natural” smokers (i.e., we forced non-smokers to become smokers; they didn’t take on all of the other normal, real life characteristics that smokers might tend to possess) they probably have better diets. As such, in this silly example they wouldn’t get lung cancer, and our experiment will fail, because it violates the structure of the “natural” world (the technical name for this is an “artifactual” result; see later).
One distinction worth making between two types of non-experimental research is the difference be- tween quasi-experimental research and case studies. The example I discussed earlier – in which we wanted to examine incidence of lung cancer among smokers and non-smokers, without trying to control who smokes and who doesn’t – is a quasi-experimental design. That is, it’s the same as an experiment, but we don’t control the predictors (IVs). We can still use statistics to analyse the results, it’s just that we have to be a lot more careful.
The alternative approach, case studies, aims to provide a very detailed description of one or a few instances. In general, you can’t use statistics to analyse the results of case studies, and it’s usually very hard to draw any general conclusions about “people in general” from a few isolated examples. However, case studies are very useful in some situations. Firstly, there are situations where you don’t have any alternative: neuropsychology has this issue a lot. Sometimes, you just can’t find a lot of people with brain damage in a specific area, so the only thing you can do is describe those cases that you do have in as much detail and with as much care as you can. However, there’s also some genuine advantages to case studies: because you don’t have as many people to study, you have the ability to invest lots of time and effort trying to understand the specific factors at play in each case. This is a very valuable thing to do. As a consequence, case studies can complement the more statistically-oriented approaches that you see in experimental and quasi-experimental designs. We won’t talk much about case studies in these lectures, but they are nevertheless very valuable tools!
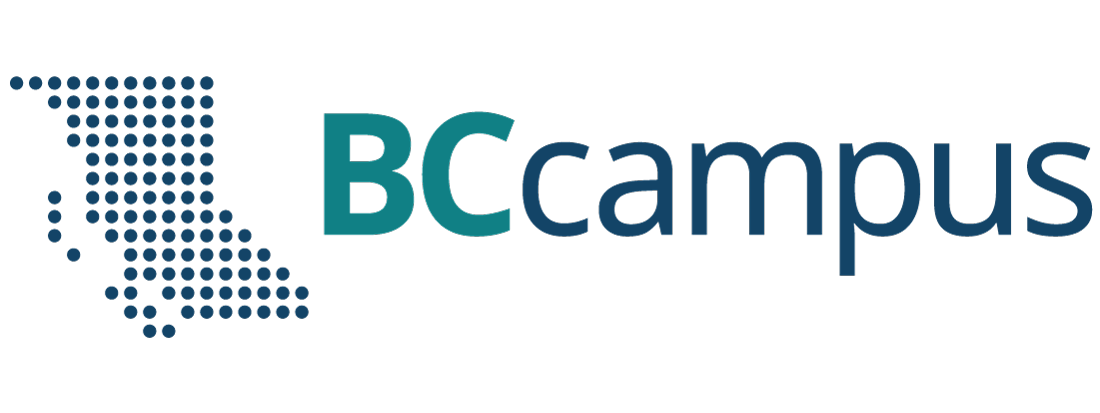
Want to create or adapt books like this? Learn more about how Pressbooks supports open publishing practices.
Chapter 3. Psychological Science
3.2 Psychologists Use Descriptive, Correlational, and Experimental Research Designs to Understand Behaviour
Learning objectives.
- Differentiate the goals of descriptive, correlational, and experimental research designs and explain the advantages and disadvantages of each.
- Explain the goals of descriptive research and the statistical techniques used to interpret it.
- Summarize the uses of correlational research and describe why correlational research cannot be used to infer causality.
- Review the procedures of experimental research and explain how it can be used to draw causal inferences.
Psychologists agree that if their ideas and theories about human behaviour are to be taken seriously, they must be backed up by data. However, the research of different psychologists is designed with different goals in mind, and the different goals require different approaches. These varying approaches, summarized in Table 3.2, are known as research designs . A research design is the specific method a researcher uses to collect, analyze, and interpret data . Psychologists use three major types of research designs in their research, and each provides an essential avenue for scientific investigation. Descriptive research is research designed to provide a snapshot of the current state of affairs . Correlational research is research designed to discover relationships among variables and to allow the prediction of future events from present knowledge . Experimental research is research in which initial equivalence among research participants in more than one group is created, followed by a manipulation of a given experience for these groups and a measurement of the influence of the manipulation . Each of the three research designs varies according to its strengths and limitations, and it is important to understand how each differs.
Descriptive Research: Assessing the Current State of Affairs
Descriptive research is designed to create a snapshot of the current thoughts, feelings, or behaviour of individuals. This section reviews three types of descriptive research : case studies , surveys , and naturalistic observation (Figure 3.4).
Sometimes the data in a descriptive research project are based on only a small set of individuals, often only one person or a single small group. These research designs are known as case studies — descriptive records of one or more individual’s experiences and behaviour . Sometimes case studies involve ordinary individuals, as when developmental psychologist Jean Piaget used his observation of his own children to develop his stage theory of cognitive development. More frequently, case studies are conducted on individuals who have unusual or abnormal experiences or characteristics or who find themselves in particularly difficult or stressful situations. The assumption is that by carefully studying individuals who are socially marginal, who are experiencing unusual situations, or who are going through a difficult phase in their lives, we can learn something about human nature.
Sigmund Freud was a master of using the psychological difficulties of individuals to draw conclusions about basic psychological processes. Freud wrote case studies of some of his most interesting patients and used these careful examinations to develop his important theories of personality. One classic example is Freud’s description of “Little Hans,” a child whose fear of horses the psychoanalyst interpreted in terms of repressed sexual impulses and the Oedipus complex (Freud, 1909/1964).
Another well-known case study is Phineas Gage, a man whose thoughts and emotions were extensively studied by cognitive psychologists after a railroad spike was blasted through his skull in an accident. Although there are questions about the interpretation of this case study (Kotowicz, 2007), it did provide early evidence that the brain’s frontal lobe is involved in emotion and morality (Damasio et al., 2005). An interesting example of a case study in clinical psychology is described by Rokeach (1964), who investigated in detail the beliefs of and interactions among three patients with schizophrenia, all of whom were convinced they were Jesus Christ.
In other cases the data from descriptive research projects come in the form of a survey — a measure administered through either an interview or a written questionnaire to get a picture of the beliefs or behaviours of a sample of people of interest . The people chosen to participate in the research (known as the sample) are selected to be representative of all the people that the researcher wishes to know about (the population). In election polls, for instance, a sample is taken from the population of all “likely voters” in the upcoming elections.
The results of surveys may sometimes be rather mundane, such as “Nine out of 10 doctors prefer Tymenocin” or “The median income in the city of Hamilton is $46,712.” Yet other times (particularly in discussions of social behaviour), the results can be shocking: “More than 40,000 people are killed by gunfire in the United States every year” or “More than 60% of women between the ages of 50 and 60 suffer from depression.” Descriptive research is frequently used by psychologists to get an estimate of the prevalence (or incidence ) of psychological disorders.
A final type of descriptive research — known as naturalistic observation — is research based on the observation of everyday events . For instance, a developmental psychologist who watches children on a playground and describes what they say to each other while they play is conducting descriptive research, as is a biopsychologist who observes animals in their natural habitats. One example of observational research involves a systematic procedure known as the strange situation , used to get a picture of how adults and young children interact. The data that are collected in the strange situation are systematically coded in a coding sheet such as that shown in Table 3.3.
The results of descriptive research projects are analyzed using descriptive statistics — numbers that summarize the distribution of scores on a measured variable . Most variables have distributions similar to that shown in Figure 3.5 where most of the scores are located near the centre of the distribution, and the distribution is symmetrical and bell-shaped. A data distribution that is shaped like a bell is known as a normal distribution .
A distribution can be described in terms of its central tendency — that is, the point in the distribution around which the data are centred — and its dispersion, or spread . The arithmetic average, or arithmetic mean , symbolized by the letter M , is the most commonly used measure of central tendency . It is computed by calculating the sum of all the scores of the variable and dividing this sum by the number of participants in the distribution (denoted by the letter N ). In the data presented in Figure 3.5 the mean height of the students is 67.12 inches (170.5 cm). The sample mean is usually indicated by the letter M .
In some cases, however, the data distribution is not symmetrical. This occurs when there are one or more extreme scores (known as outliers ) at one end of the distribution. Consider, for instance, the variable of family income (see Figure 3.6), which includes an outlier (a value of $3,800,000). In this case the mean is not a good measure of central tendency. Although it appears from Figure 3.6 that the central tendency of the family income variable should be around $70,000, the mean family income is actually $223,960. The single very extreme income has a disproportionate impact on the mean, resulting in a value that does not well represent the central tendency.
The median is used as an alternative measure of central tendency when distributions are not symmetrical. The median is the score in the center of the distribution, meaning that 50% of the scores are greater than the median and 50% of the scores are less than the median . In our case, the median household income ($73,000) is a much better indication of central tendency than is the mean household income ($223,960).
A final measure of central tendency, known as the mode , represents the value that occurs most frequently in the distribution . You can see from Figure 3.6 that the mode for the family income variable is $93,000 (it occurs four times).
In addition to summarizing the central tendency of a distribution, descriptive statistics convey information about how the scores of the variable are spread around the central tendency. Dispersion refers to the extent to which the scores are all tightly clustered around the central tendency , as seen in Figure 3.7.
Or they may be more spread out away from it, as seen in Figure 3.8.
One simple measure of dispersion is to find the largest (the maximum ) and the smallest (the minimum ) observed values of the variable and to compute the range of the variable as the maximum observed score minus the minimum observed score. You can check that the range of the height variable in Figure 3.5 is 72 – 62 = 10. The standard deviation , symbolized as s , is the most commonly used measure of dispersion . Distributions with a larger standard deviation have more spread. The standard deviation of the height variable is s = 2.74, and the standard deviation of the family income variable is s = $745,337.
An advantage of descriptive research is that it attempts to capture the complexity of everyday behaviour. Case studies provide detailed information about a single person or a small group of people, surveys capture the thoughts or reported behaviours of a large population of people, and naturalistic observation objectively records the behaviour of people or animals as it occurs naturally. Thus descriptive research is used to provide a relatively complete understanding of what is currently happening.
Despite these advantages, descriptive research has a distinct disadvantage in that, although it allows us to get an idea of what is currently happening, it is usually limited to static pictures. Although descriptions of particular experiences may be interesting, they are not always transferable to other individuals in other situations, nor do they tell us exactly why specific behaviours or events occurred. For instance, descriptions of individuals who have suffered a stressful event, such as a war or an earthquake, can be used to understand the individuals’ reactions to the event but cannot tell us anything about the long-term effects of the stress. And because there is no comparison group that did not experience the stressful situation, we cannot know what these individuals would be like if they hadn’t had the stressful experience.
Correlational Research: Seeking Relationships among Variables
In contrast to descriptive research, which is designed primarily to provide static pictures, correlational research involves the measurement of two or more relevant variables and an assessment of the relationship between or among those variables. For instance, the variables of height and weight are systematically related (correlated) because taller people generally weigh more than shorter people. In the same way, study time and memory errors are also related, because the more time a person is given to study a list of words, the fewer errors he or she will make. When there are two variables in the research design, one of them is called the predictor variable and the other the outcome variable . The research design can be visualized as shown in Figure 3.9, where the curved arrow represents the expected correlation between these two variables.
One way of organizing the data from a correlational study with two variables is to graph the values of each of the measured variables using a scatter plot . As you can see in Figure 3.10 a scatter plot is a visual image of the relationship between two variables . A point is plotted for each individual at the intersection of his or her scores for the two variables. When the association between the variables on the scatter plot can be easily approximated with a straight line , as in parts (a) and (b) of Figure 3.10 the variables are said to have a linear relationship .
When the straight line indicates that individuals who have above-average values for one variable also tend to have above-average values for the other variable , as in part (a), the relationship is said to be positive linear . Examples of positive linear relationships include those between height and weight, between education and income, and between age and mathematical abilities in children. In each case, people who score higher on one of the variables also tend to score higher on the other variable. Negative linear relationships , in contrast, as shown in part (b), occur when above-average values for one variable tend to be associated with below-average values for the other variable. Examples of negative linear relationships include those between the age of a child and the number of diapers the child uses, and between practice on and errors made on a learning task. In these cases, people who score higher on one of the variables tend to score lower on the other variable.
Relationships between variables that cannot be described with a straight line are known as nonlinear relationships . Part (c) of Figure 3.10 shows a common pattern in which the distribution of the points is essentially random. In this case there is no relationship at all between the two variables, and they are said to be independent . Parts (d) and (e) of Figure 3.10 show patterns of association in which, although there is an association, the points are not well described by a single straight line. For instance, part (d) shows the type of relationship that frequently occurs between anxiety and performance. Increases in anxiety from low to moderate levels are associated with performance increases, whereas increases in anxiety from moderate to high levels are associated with decreases in performance. Relationships that change in direction and thus are not described by a single straight line are called curvilinear relationships .
The most common statistical measure of the strength of linear relationships among variables is the Pearson correlation coefficient , which is symbolized by the letter r . The value of the correlation coefficient ranges from r = –1.00 to r = +1.00. The direction of the linear relationship is indicated by the sign of the correlation coefficient. Positive values of r (such as r = .54 or r = .67) indicate that the relationship is positive linear (i.e., the pattern of the dots on the scatter plot runs from the lower left to the upper right), whereas negative values of r (such as r = –.30 or r = –.72) indicate negative linear relationships (i.e., the dots run from the upper left to the lower right). The strength of the linear relationship is indexed by the distance of the correlation coefficient from zero (its absolute value). For instance, r = –.54 is a stronger relationship than r = .30, and r = .72 is a stronger relationship than r = –.57. Because the Pearson correlation coefficient only measures linear relationships, variables that have curvilinear relationships are not well described by r , and the observed correlation will be close to zero.
It is also possible to study relationships among more than two measures at the same time. A research design in which more than one predictor variable is used to predict a single outcome variable is analyzed through multiple regression (Aiken & West, 1991). Multiple regression is a statistical technique, based on correlation coefficients among variables, that allows predicting a single outcome variable from more than one predictor variable . For instance, Figure 3.11 shows a multiple regression analysis in which three predictor variables (Salary, job satisfaction, and years employed) are used to predict a single outcome (job performance). The use of multiple regression analysis shows an important advantage of correlational research designs — they can be used to make predictions about a person’s likely score on an outcome variable (e.g., job performance) based on knowledge of other variables.
An important limitation of correlational research designs is that they cannot be used to draw conclusions about the causal relationships among the measured variables. Consider, for instance, a researcher who has hypothesized that viewing violent behaviour will cause increased aggressive play in children. He has collected, from a sample of Grade 4 children, a measure of how many violent television shows each child views during the week, as well as a measure of how aggressively each child plays on the school playground. From his collected data, the researcher discovers a positive correlation between the two measured variables.
Although this positive correlation appears to support the researcher’s hypothesis, it cannot be taken to indicate that viewing violent television causes aggressive behaviour. Although the researcher is tempted to assume that viewing violent television causes aggressive play, there are other possibilities. One alternative possibility is that the causal direction is exactly opposite from what has been hypothesized. Perhaps children who have behaved aggressively at school develop residual excitement that leads them to want to watch violent television shows at home (Figure 3.13):
Although this possibility may seem less likely, there is no way to rule out the possibility of such reverse causation on the basis of this observed correlation. It is also possible that both causal directions are operating and that the two variables cause each other (Figure 3.14).
Still another possible explanation for the observed correlation is that it has been produced by the presence of a common-causal variable (also known as a third variable ). A common-causal variable is a variable that is not part of the research hypothesis but that causes both the predictor and the outcome variable and thus produces the observed correlation between them . In our example, a potential common-causal variable is the discipline style of the children’s parents. Parents who use a harsh and punitive discipline style may produce children who like to watch violent television and who also behave aggressively in comparison to children whose parents use less harsh discipline (Figure 3.15)
In this case, television viewing and aggressive play would be positively correlated (as indicated by the curved arrow between them), even though neither one caused the other but they were both caused by the discipline style of the parents (the straight arrows). When the predictor and outcome variables are both caused by a common-causal variable, the observed relationship between them is said to be spurious . A spurious relationship is a relationship between two variables in which a common-causal variable produces and “explains away” the relationship . If effects of the common-causal variable were taken away, or controlled for, the relationship between the predictor and outcome variables would disappear. In the example, the relationship between aggression and television viewing might be spurious because by controlling for the effect of the parents’ disciplining style, the relationship between television viewing and aggressive behaviour might go away.
Common-causal variables in correlational research designs can be thought of as mystery variables because, as they have not been measured, their presence and identity are usually unknown to the researcher. Since it is not possible to measure every variable that could cause both the predictor and outcome variables, the existence of an unknown common-causal variable is always a possibility. For this reason, we are left with the basic limitation of correlational research: correlation does not demonstrate causation. It is important that when you read about correlational research projects, you keep in mind the possibility of spurious relationships, and be sure to interpret the findings appropriately. Although correlational research is sometimes reported as demonstrating causality without any mention being made of the possibility of reverse causation or common-causal variables, informed consumers of research, like you, are aware of these interpretational problems.
In sum, correlational research designs have both strengths and limitations. One strength is that they can be used when experimental research is not possible because the predictor variables cannot be manipulated. Correlational designs also have the advantage of allowing the researcher to study behaviour as it occurs in everyday life. And we can also use correlational designs to make predictions — for instance, to predict from the scores on their battery of tests the success of job trainees during a training session. But we cannot use such correlational information to determine whether the training caused better job performance. For that, researchers rely on experiments.
Experimental Research: Understanding the Causes of Behaviour
The goal of experimental research design is to provide more definitive conclusions about the causal relationships among the variables in the research hypothesis than is available from correlational designs. In an experimental research design, the variables of interest are called the independent variable (or variables ) and the dependent variable . The independent variable in an experiment is the causing variable that is created (manipulated) by the experimenter . The dependent variable in an experiment is a measured variable that is expected to be influenced by the experimental manipulation . The research hypothesis suggests that the manipulated independent variable or variables will cause changes in the measured dependent variables. We can diagram the research hypothesis by using an arrow that points in one direction. This demonstrates the expected direction of causality (Figure 3.16):
Research Focus: Video Games and Aggression
Consider an experiment conducted by Anderson and Dill (2000). The study was designed to test the hypothesis that viewing violent video games would increase aggressive behaviour. In this research, male and female undergraduates from Iowa State University were given a chance to play with either a violent video game (Wolfenstein 3D) or a nonviolent video game (Myst). During the experimental session, the participants played their assigned video games for 15 minutes. Then, after the play, each participant played a competitive game with an opponent in which the participant could deliver blasts of white noise through the earphones of the opponent. The operational definition of the dependent variable (aggressive behaviour) was the level and duration of noise delivered to the opponent. The design of the experiment is shown in Figure 3.17
Two advantages of the experimental research design are (a) the assurance that the independent variable (also known as the experimental manipulation ) occurs prior to the measured dependent variable, and (b) the creation of initial equivalence between the conditions of the experiment (in this case by using random assignment to conditions).
Experimental designs have two very nice features. For one, they guarantee that the independent variable occurs prior to the measurement of the dependent variable. This eliminates the possibility of reverse causation. Second, the influence of common-causal variables is controlled, and thus eliminated, by creating initial equivalence among the participants in each of the experimental conditions before the manipulation occurs.
The most common method of creating equivalence among the experimental conditions is through random assignment to conditions, a procedure in which the condition that each participant is assigned to is determined through a random process, such as drawing numbers out of an envelope or using a random number table . Anderson and Dill first randomly assigned about 100 participants to each of their two groups (Group A and Group B). Because they used random assignment to conditions, they could be confident that, before the experimental manipulation occurred, the students in Group A were, on average, equivalent to the students in Group B on every possible variable, including variables that are likely to be related to aggression, such as parental discipline style, peer relationships, hormone levels, diet — and in fact everything else.
Then, after they had created initial equivalence, Anderson and Dill created the experimental manipulation — they had the participants in Group A play the violent game and the participants in Group B play the nonviolent game. Then they compared the dependent variable (the white noise blasts) between the two groups, finding that the students who had viewed the violent video game gave significantly longer noise blasts than did the students who had played the nonviolent game.
Anderson and Dill had from the outset created initial equivalence between the groups. This initial equivalence allowed them to observe differences in the white noise levels between the two groups after the experimental manipulation, leading to the conclusion that it was the independent variable (and not some other variable) that caused these differences. The idea is that the only thing that was different between the students in the two groups was the video game they had played.
Despite the advantage of determining causation, experiments do have limitations. One is that they are often conducted in laboratory situations rather than in the everyday lives of people. Therefore, we do not know whether results that we find in a laboratory setting will necessarily hold up in everyday life. Second, and more important, is that some of the most interesting and key social variables cannot be experimentally manipulated. If we want to study the influence of the size of a mob on the destructiveness of its behaviour, or to compare the personality characteristics of people who join suicide cults with those of people who do not join such cults, these relationships must be assessed using correlational designs, because it is simply not possible to experimentally manipulate these variables.
Key Takeaways
- Descriptive, correlational, and experimental research designs are used to collect and analyze data.
- Descriptive designs include case studies, surveys, and naturalistic observation. The goal of these designs is to get a picture of the current thoughts, feelings, or behaviours in a given group of people. Descriptive research is summarized using descriptive statistics.
- Correlational research designs measure two or more relevant variables and assess a relationship between or among them. The variables may be presented on a scatter plot to visually show the relationships. The Pearson Correlation Coefficient ( r ) is a measure of the strength of linear relationship between two variables.
- Common-causal variables may cause both the predictor and outcome variable in a correlational design, producing a spurious relationship. The possibility of common-causal variables makes it impossible to draw causal conclusions from correlational research designs.
- Experimental research involves the manipulation of an independent variable and the measurement of a dependent variable. Random assignment to conditions is normally used to create initial equivalence between the groups, allowing researchers to draw causal conclusions.
Exercises and Critical Thinking
- There is a negative correlation between the row that a student sits in in a large class (when the rows are numbered from front to back) and his or her final grade in the class. Do you think this represents a causal relationship or a spurious relationship, and why?
- Think of two variables (other than those mentioned in this book) that are likely to be correlated, but in which the correlation is probably spurious. What is the likely common-causal variable that is producing the relationship?
- Imagine a researcher wants to test the hypothesis that participating in psychotherapy will cause a decrease in reported anxiety. Describe the type of research design the investigator might use to draw this conclusion. What would be the independent and dependent variables in the research?
Image Attributions
Figure 3.4: “ Reading newspaper ” by Alaskan Dude (http://commons.wikimedia.org/wiki/File:Reading_newspaper.jpg) is licensed under CC BY 2.0
Aiken, L., & West, S. (1991). Multiple regression: Testing and interpreting interactions . Newbury Park, CA: Sage.
Ainsworth, M. S., Blehar, M. C., Waters, E., & Wall, S. (1978). Patterns of attachment: A psychological study of the strange situation . Hillsdale, NJ: Lawrence Erlbaum Associates.
Anderson, C. A., & Dill, K. E. (2000). Video games and aggressive thoughts, feelings, and behavior in the laboratory and in life. Journal of Personality and Social Psychology, 78 (4), 772–790.
Damasio, H., Grabowski, T., Frank, R., Galaburda, A. M., Damasio, A. R., Cacioppo, J. T., & Berntson, G. G. (2005). The return of Phineas Gage: Clues about the brain from the skull of a famous patient. In Social neuroscience: Key readings. (pp. 21–28). New York, NY: Psychology Press.
Freud, S. (1909/1964). Analysis of phobia in a five-year-old boy. In E. A. Southwell & M. Merbaum (Eds.), Personality: Readings in theory and research (pp. 3–32). Belmont, CA: Wadsworth. (Original work published 1909).
Kotowicz, Z. (2007). The strange case of Phineas Gage. History of the Human Sciences, 20 (1), 115–131.
Rokeach, M. (1964). The three Christs of Ypsilanti: A psychological study . New York, NY: Knopf.
Stangor, C. (2011). Research methods for the behavioural sciences (4th ed.). Mountain View, CA: Cengage.
Long Descriptions
Figure 3.6 long description: There are 25 families. 24 families have an income between $44,000 and $111,000 and one family has an income of $3,800,000. The mean income is $223,960 while the median income is $73,000. [Return to Figure 3.6]
Figure 3.10 long description: Types of scatter plots.
- Positive linear, r=positive .82. The plots on the graph form a rough line that runs from lower left to upper right.
- Negative linear, r=negative .70. The plots on the graph form a rough line that runs from upper left to lower right.
- Independent, r=0.00. The plots on the graph are spread out around the centre.
- Curvilinear, r=0.00. The plots of the graph form a rough line that goes up and then down like a hill.
- Curvilinear, r=0.00. The plots on the graph for a rough line that goes down and then up like a ditch.
[Return to Figure 3.10]
Introduction to Psychology - 1st Canadian Edition Copyright © 2014 by Jennifer Walinga and Charles Stangor is licensed under a Creative Commons Attribution-NonCommercial-ShareAlike 4.0 International License , except where otherwise noted.
Share This Book
Observational vs. Experimental Study: A Comprehensive Guide
Explore the fundamental disparities between experimental and observational studies in this comprehensive guide by Santos Research Center, Corp. Uncover concepts such as control group, random sample, cohort studies, response variable, and explanatory variable that shape the foundation of these methodologies. Discover the significance of randomized controlled trials and case control studies, examining causal relationships and the role of dependent variables and independent variables in research designs.
This enlightening exploration also delves into the meticulous scientific study process, involving survey members, systematic reviews, and statistical analyses. Investigate the careful balance of control group and treatment group dynamics, highlighting how researchers meticulously assign variables and analyze statistical patterns to discern meaningful insights. From dissecting issues like lung cancer to understanding sleep patterns, this guide emphasizes the precision of controlled experiments and controlled trials, where variables are isolated and scrutinized, paving the way for a deeper comprehension of the world through empirical research.
Introduction to Observational and Experimental Studies
These two studies are the cornerstones of scientific inquiry, each offering a distinct approach to unraveling the mysteries of the natural world.
Observational studies allow us to observe, document, and gather data without direct intervention. They provide a means to explore real-world scenarios and trends, making them valuable when manipulating variables is not feasible or ethical. From surveys to meticulous observations, these studies shed light on existing conditions and relationships.
Experimental studies , in contrast, put researchers in the driver's seat. They involve the deliberate manipulation of variables to understand their impact on specific outcomes. By controlling the conditions, experimental studies establish causal relationships, answering questions of causality with precision. This approach is pivotal for hypothesis testing and informed decision-making.
At Santos Research Center, Corp., we recognize the importance of both observational and experimental studies. We employ these methodologies in our diverse research projects to ensure the highest quality of scientific investigation and to answer a wide range of research questions.
Observational Studies: A Closer Look
In our exploration of research methodologies, let's zoom in on observational research studies—an essential facet of scientific inquiry that we at Santos Research Center, Corp., expertly employ in our diverse research projects.
What is an Observational Study?
Observational research studies involve the passive observation of subjects without any intervention or manipulation by researchers. These studies are designed to scrutinize the relationships between variables and test subjects, uncover patterns, and draw conclusions grounded in real-world data.
Researchers refrain from interfering with the natural course of events in controlled experiment. Instead, they meticulously gather data by keenly observing and documenting information about the test subjects and their surroundings. This approach permits the examination of variables that cannot be ethically or feasibly manipulated, making it particularly valuable in certain research scenarios.
Types of Observational Studies
Now, let's delve into the various forms that observational studies can take, each with its distinct characteristics and applications.
Cohort Studies: A cohort study is a type of observational study that entails tracking one group of individuals over an extended period. Its primary goal is to identify potential causes or risk factors for specific outcomes or treatment group. Cohort studies provide valuable insights into the development of conditions or diseases and the factors that influence them.
Case-Control Studies: Case-control studies, on the other hand, involve the comparison of individuals with a particular condition or outcome to those without it (the control group). These studies aim to discern potential causal factors or associations that may have contributed to the development of the condition under investigation.
Cross-Sectional Studies: Cross-sectional studies take a snapshot of a diverse group of individuals at a single point in time. By collecting data from this snapshot, researchers gain insights into the prevalence of a specific condition or the relationships between variables at that precise moment. Cross-sectional studies are often used to assess the health status of the different groups within a population or explore the interplay between various factors.
Advantages and Limitations of Observational Studies
Observational studies, as we've explored, are a vital pillar of scientific research, offering unique insights into real-world phenomena. In this section, we will dissect the advantages and limitations that characterize these studies, shedding light on the intricacies that researchers grapple with when employing this methodology.
Advantages: One of the paramount advantages of observational studies lies in their utilization of real-world data. Unlike controlled experiments that operate in artificial settings, observational studies embrace the complexities of the natural world. This approach enables researchers to capture genuine behaviors, patterns, and occurrences as they unfold. As a result, the data collected reflects the intricacies of real-life scenarios, making it highly relevant and applicable to diverse settings and populations.
Moreover, in a randomized controlled trial, researchers looked to randomly assign participants to a group. Observational studies excel in their capacity to examine long-term trends. By observing one group of subjects over extended periods, research scientists gain the ability to track developments, trends, and shifts in behavior or outcomes. This longitudinal perspective is invaluable when studying phenomena that evolve gradually, such as chronic diseases, societal changes, or environmental shifts. It allows for the detection of subtle nuances that may be missed in shorter-term investigations.
Limitations: However, like any research methodology, observational studies are not without their limitations. One significant challenge of statistical study lies in the potential for biases. Since researchers do not intervene in the subjects' experiences, various biases can creep into the data collection process. These biases may arise from participant self-reporting, observer bias, or selection bias in random sample, among others. Careful design and rigorous data analysis are crucial for mitigating these biases.
Another limitation is the presence of confounding variables. In observational studies, it can be challenging to isolate the effect of a specific variable from the myriad of other factors at play. These confounding variables can obscure the true relationship between the variables of interest, making it difficult to establish causation definitively. Research scientists must employ statistical techniques to control for or adjust these confounding variables.
Additionally, observational studies face constraints in their ability to establish causation. While they can identify associations and correlations between variables, they cannot prove causality or causal relationship. Establishing causation typically requires controlled experiments where researchers can manipulate independent variables systematically. In observational studies, researchers can only infer potential causation based on the observed associations.
Experimental Studies: Delving Deeper
In the intricate landscape of scientific research, we now turn our gaze toward experimental studies—a dynamic and powerful method that Santos Research Center, Corp. skillfully employs in our pursuit of knowledge.
What is an Experimental Study?
While some studies observe and gather data passively, experimental studies take a more proactive approach. Here, researchers actively introduce an intervention or treatment to an experiment group study its effects on one or more variables. This methodology empowers researchers to manipulate independent variables deliberately and examine their direct impact on dependent variables.
Experimental research are distinguished by their exceptional ability to establish cause-and-effect relationships. This invaluable characteristic allows researchers to unlock the mysteries of how one variable influences another, offering profound insights into the scientific questions at hand. Within the controlled environment of an experimental study, researchers can systematically test hypotheses, shedding light on complex phenomena.
Key Features of Experimental Studies
Central to statistical analysis, the rigor and reliability of experimental studies are several key features that ensure the validity of their findings.
Randomized Controlled Trials: Randomization is a critical element in experimental studies, as it ensures that subjects are assigned to groups in a random assignment. This randomly assigned allocation minimizes the risk of unintentional biases and confounding variables, strengthening the credibility of the study's outcomes.
Control Groups: Control groups play a pivotal role in experimental studies by serving as a baseline for comparison. They enable researchers to assess the true impact of the intervention being studied. By comparing the outcomes of the intervention group to those of survey members of the control group, researchers can discern whether the intervention caused the observed changes.
Blinding: Both single-blind and double-blind techniques are employed in experimental studies to prevent biases from influencing the study or controlled trial's outcomes. Single-blind studies keep either the subjects or the researchers unaware of certain aspects of the study, while double-blind studies extend this blindness to both parties, enhancing the objectivity of the study.
These key features work in concert to uphold the integrity and trustworthiness of the results generated through experimental studies.
Advantages and Limitations of Experimental Studies
As with any research methodology, this one comes with its unique set of advantages and limitations.
Advantages: These studies offer the distinct advantage of establishing causal relationships between two or more variables together. The controlled environment allows researchers to exert authority over variables, ensuring that changes in the dependent variable can be attributed to the independent variable. This meticulous control results in high-quality, reliable data that can significantly contribute to scientific knowledge.
Limitations: However, experimental ones are not without their challenges. They may raise ethical concerns, particularly when the interventions involve potential risks to subjects. Additionally, their controlled nature can limit their real-world applicability, as the conditions in experiments may not accurately mirror those in the natural world. Moreover, executing an experimental study in randomized controlled, often demands substantial resources, with other variables including time, funding, and personnel.
Observational vs Experimental: A Side-by-Side Comparison
Having previously examined observational and experimental studies individually, we now embark on a side-by-side comparison to illuminate the key distinctions and commonalities between these foundational research approaches.
Key Differences and Notable Similarities
Methodologies
- Observational Studies : Characterized by passive observation, where researchers collect data without direct intervention, allowing the natural course of events to unfold.
- Experimental Studies : Involve active intervention, where researchers deliberately manipulate variables to discern their impact on specific outcomes, ensuring control over the experimental conditions.
- Observational Studies : Designed to identify patterns, correlations, and associations within existing data, shedding light on relationships within real-world settings.
- Experimental Studies : Geared toward establishing causality by determining the cause-and-effect relationships between variables, often in controlled laboratory environments.
- Observational Studies : Yield real-world data, reflecting the complexities and nuances of natural phenomena.
- Experimental Studies : Generate controlled data, allowing for precise analysis and the establishment of clear causal connections.
Observational studies excel at exploring associations and uncovering patterns within the intricacies of real-world settings, while experimental studies shine as the gold standard for discerning cause-and-effect relationships through meticulous control and manipulation in controlled environments. Understanding these differences and similarities empowers researchers to choose the most appropriate method for their specific research objectives.
When to Use Which: Practical Applications
The decision to employ either observational or experimental studies hinges on the research objectives at hand and the available resources. Observational studies prove invaluable when variable manipulation is impractical or ethically challenging, making them ideal for delving into long-term trends and uncovering intricate associations between certain variables (response variable or explanatory variable). On the other hand, experimental studies emerge as indispensable tools when the aim is to definitively establish causation and methodically control variables.
At Santos Research Center, Corp., our approach to both scientific study and methodology is characterized by meticulous consideration of the specific research goals. We recognize that the quality of outcomes hinges on selecting the most appropriate method of research study. Our unwavering commitment to employing both observational and experimental research studies further underscores our dedication to advancing scientific knowledge across diverse domains.
Conclusion: The Synergy of Experimental and Observational Studies in Research
In conclusion, both observational and experimental studies are integral to scientific research, offering complementary approaches with unique strengths and limitations. At Santos Research Center, Corp., we leverage these methodologies to contribute meaningfully to the scientific community.
Explore our projects and initiatives at Santos Research Center, Corp. by visiting our website or contacting us at (813) 249-9100, where our unwavering commitment to rigorous research practices and advancing scientific knowledge awaits.
Recent Posts
Learn everything about Respiratory Syncytial Virus (RSV), from symptoms and diagnosis to treatment and prevention. Stay informed and protect your health with...
Discover key insights on Alzheimer's disease, including symptoms, stages, and care tips. Learn how to manage the condition and find out how you can...
Discover expert insights on migraines, from symptoms and causes to management strategies, and learn about our specialized support at Santos Research Center.
Explore our in-depth guide on UTIs, covering everything from symptoms and causes to effective treatments, and learn how to manage and prevent urinary tract infections.
Your definitive guide to COVID symptoms. Dive deep into the signs of COVID-19, understand the new variants, and get answers to your most pressing questions.
Unravel the differences between observational and experimental studies. Dive into the intricacies of each method and discover their unique applications in research.
Discover the different types of clinical trials and their significance in advancing medical science. Dive deep into the world of clinical research with Santos Research Center.
Santos Research Center, Corp. is a research facility conducting paid clinical trials, in partnership with major pharmaceutical companies & CROs. We work with patients from across the Tampa Bay area.
Contact Details
Navigation menu.
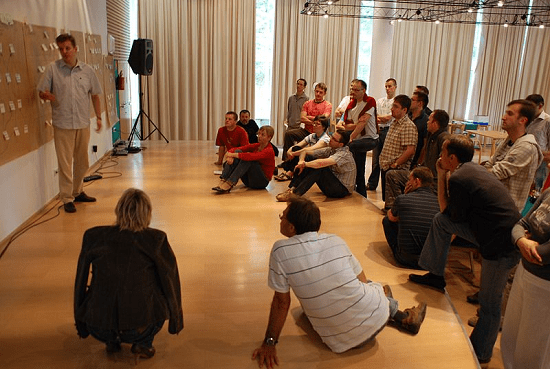
Distinguishing Between Case Studies & Experiments

Case Study vs Experiment
Case studies and experiments are two distinct research methods used across various disciplines, providing researchers with the ability to study and analyze a subject through different approaches. This variety in research methods allows the researcher to gather both qualitative and quantitative data, cross-check the data, and assign greater validity to the conclusions and overall findings of the research. A case study is a research method in which the researcher explores the subject in depth, while an experiment is a research method where two specific groups or variables are used to test a hypothesis. This article will examine the differences between case study and experiment further.
What is a Case Study?
A case study is a research method where an individual, event, or significant place is studied in depth. In the case of an individual, the researcher studies the person’s life history, which can include important days or special experiences. The case study method is used in various social sciences such as sociology, anthropology, and psychology. Through a case study, the researcher can identify and understand the subjective experiences of an individual regarding a specific topic. For example, a researcher studying the impact of second rape on the lives of rape victims can conduct several case studies to understand the subjective experiences of individuals and social mechanisms that contribute to this phenomenon. The case study is a qualitative research method that can be subjective.
What is an Experiment?
An experiment, unlike a case study, can be classified as a quantitative research method, as it provides statistically significant data and an objective, empirical approach. Experiments are primarily used in natural sciences, as they allow the scientist to control variables. In social sciences, controlling variables can be challenging and may lead to faulty conclusions. In an experiment, there are mainly two variables: the independent variable and the dependent variable. The researcher tries to test their hypothesis by manipulating these variables. There are different types of experiments, such as laboratory experiments (conducted in laboratories where conditions can be strictly controlled) and natural experiments (which take place in real-life settings). As seen, case study methods and experiments are very different from one another. However, most researchers prefer to use triangulation when conducting research to minimize biases.
Key Takeaways
- Case studies are in-depth explorations of a subject, providing qualitative data, while experiments test hypotheses by manipulating variables, providing quantitative data.
- Experiments are primarily used in natural sciences, whereas case studies are primarily used in social sciences.
- Experiments involve testing the correlation between two variables (independent and dependent), while case studies focus on exploring a subject in depth without testing correlations between variables.
LEAVE A REPLY Cancel reply
Save my name, email, and website in this browser for the next time I comment.
Related Articles
Difference between power & authority, distinguishing could of & could have, distinguishing pixie & bob haircuts, distinguishing between debate & discussion, distinguishing between dialogue & conversation, distinguishing between a present & a gift, distinguishing between will & can, distinguishing between up & upon.
Case Study vs. Single-Case Experimental Designs
What's the difference.
Case study and single-case experimental designs are both research methods used in psychology and other social sciences to investigate individual cases or subjects. However, they differ in their approach and purpose. Case studies involve in-depth examination of a single case, such as an individual, group, or organization, to gain a comprehensive understanding of the phenomenon being studied. On the other hand, single-case experimental designs focus on studying the effects of an intervention or treatment on a single subject over time. These designs use repeated measures and control conditions to establish cause-and-effect relationships. While case studies provide rich qualitative data, single-case experimental designs offer more rigorous experimental control and allow for the evaluation of treatment effectiveness.
Further Detail
Introduction.
When conducting research in various fields, it is essential to choose the appropriate study design to answer research questions effectively. Two commonly used designs are case study and single-case experimental designs. While both approaches aim to provide valuable insights into specific phenomena, they differ in several key attributes. This article will compare and contrast the attributes of case study and single-case experimental designs, highlighting their strengths and limitations.
Definition and Purpose
A case study is an in-depth investigation of a particular individual, group, or event. It involves collecting and analyzing qualitative or quantitative data to gain a comprehensive understanding of the subject under study. Case studies are often used to explore complex phenomena, generate hypotheses, or provide detailed descriptions of unique cases.
On the other hand, single-case experimental designs are a type of research design that focuses on studying a single individual or a small group over time. These designs involve manipulating an independent variable and measuring its effects on a dependent variable. Single-case experimental designs are particularly useful for examining cause-and-effect relationships and evaluating the effectiveness of interventions or treatments.
Data Collection and Analysis
In terms of data collection, case studies rely on various sources such as interviews, observations, documents, and artifacts. Researchers often employ multiple methods to gather rich and diverse data, allowing for a comprehensive analysis of the case. The data collected in case studies are typically qualitative in nature, although quantitative data may also be included.
In contrast, single-case experimental designs primarily rely on quantitative data collection methods. Researchers use standardized measures and instruments to collect data on the dependent variable before, during, and after the manipulation of the independent variable. This allows for a systematic analysis of the effects of the intervention or treatment on the individual or group being studied.
Generalizability
One of the key differences between case studies and single-case experimental designs is their generalizability. Case studies are often conducted on unique or rare cases, making it challenging to generalize the findings to a larger population. The focus of case studies is on providing detailed insights into specific cases rather than making broad generalizations.
On the other hand, single-case experimental designs aim to establish causal relationships and can provide evidence for generalizability. By systematically manipulating the independent variable and measuring its effects on the dependent variable, researchers can draw conclusions about the effectiveness of interventions or treatments that may be applicable to similar cases or populations.
Internal Validity
Internal validity refers to the extent to which a study accurately measures the cause-and-effect relationship between variables. In case studies, establishing internal validity can be challenging due to the lack of control over extraneous variables. The presence of multiple data sources and the potential for subjective interpretation may also introduce bias.
In contrast, single-case experimental designs prioritize internal validity by employing rigorous control over extraneous variables. Researchers carefully design the intervention or treatment, implement it consistently, and measure the dependent variable under controlled conditions. This allows for a more confident determination of the causal relationship between the independent and dependent variables.
Time and Resources
Case studies often require significant time and resources due to their in-depth nature. Researchers need to spend considerable time collecting and analyzing data from various sources, conducting interviews, and immersing themselves in the case. Additionally, case studies may involve multiple researchers or a research team, further increasing the required resources.
On the other hand, single-case experimental designs can be more time and resource-efficient. Since they focus on a single individual or a small group, data collection and analysis can be more streamlined. Researchers can also implement interventions or treatments in a controlled manner, reducing the time and resources needed for data collection.
Ethical Considerations
Both case studies and single-case experimental designs require researchers to consider ethical implications. In case studies, researchers must ensure the privacy and confidentiality of the individuals or groups being studied. Informed consent and ethical guidelines for data collection and analysis should be followed to protect the rights and well-being of the participants.
Similarly, in single-case experimental designs, researchers must consider ethical considerations when implementing interventions or treatments. The well-being and safety of the individual or group being studied should be prioritized, and informed consent should be obtained. Additionally, researchers should carefully monitor and evaluate the potential risks and benefits associated with the intervention or treatment.
Case studies and single-case experimental designs are valuable research approaches that offer unique insights into specific phenomena. While case studies provide in-depth descriptions and exploratory analyses of individual cases, single-case experimental designs focus on establishing causal relationships and evaluating interventions or treatments. Researchers should carefully consider the attributes and goals of their study when choosing between these two designs, ensuring that the selected approach aligns with their research questions and objectives.
Comparisons may contain inaccurate information about people, places, or facts. Please report any issues.
Have a language expert improve your writing
Run a free plagiarism check in 10 minutes, automatically generate references for free.
- Knowledge Base
- Methodology
- Case Study | Definition, Examples & Methods
Case Study | Definition, Examples & Methods
Published on 5 May 2022 by Shona McCombes . Revised on 30 January 2023.
A case study is a detailed study of a specific subject, such as a person, group, place, event, organisation, or phenomenon. Case studies are commonly used in social, educational, clinical, and business research.
A case study research design usually involves qualitative methods , but quantitative methods are sometimes also used. Case studies are good for describing , comparing, evaluating, and understanding different aspects of a research problem .
Table of contents
When to do a case study, step 1: select a case, step 2: build a theoretical framework, step 3: collect your data, step 4: describe and analyse the case.
A case study is an appropriate research design when you want to gain concrete, contextual, in-depth knowledge about a specific real-world subject. It allows you to explore the key characteristics, meanings, and implications of the case.
Case studies are often a good choice in a thesis or dissertation . They keep your project focused and manageable when you don’t have the time or resources to do large-scale research.
You might use just one complex case study where you explore a single subject in depth, or conduct multiple case studies to compare and illuminate different aspects of your research problem.
Prevent plagiarism, run a free check.
Once you have developed your problem statement and research questions , you should be ready to choose the specific case that you want to focus on. A good case study should have the potential to:
- Provide new or unexpected insights into the subject
- Challenge or complicate existing assumptions and theories
- Propose practical courses of action to resolve a problem
- Open up new directions for future research
Unlike quantitative or experimental research, a strong case study does not require a random or representative sample. In fact, case studies often deliberately focus on unusual, neglected, or outlying cases which may shed new light on the research problem.
If you find yourself aiming to simultaneously investigate and solve an issue, consider conducting action research . As its name suggests, action research conducts research and takes action at the same time, and is highly iterative and flexible.
However, you can also choose a more common or representative case to exemplify a particular category, experience, or phenomenon.
While case studies focus more on concrete details than general theories, they should usually have some connection with theory in the field. This way the case study is not just an isolated description, but is integrated into existing knowledge about the topic. It might aim to:
- Exemplify a theory by showing how it explains the case under investigation
- Expand on a theory by uncovering new concepts and ideas that need to be incorporated
- Challenge a theory by exploring an outlier case that doesn’t fit with established assumptions
To ensure that your analysis of the case has a solid academic grounding, you should conduct a literature review of sources related to the topic and develop a theoretical framework . This means identifying key concepts and theories to guide your analysis and interpretation.
There are many different research methods you can use to collect data on your subject. Case studies tend to focus on qualitative data using methods such as interviews, observations, and analysis of primary and secondary sources (e.g., newspaper articles, photographs, official records). Sometimes a case study will also collect quantitative data .
The aim is to gain as thorough an understanding as possible of the case and its context.
In writing up the case study, you need to bring together all the relevant aspects to give as complete a picture as possible of the subject.
How you report your findings depends on the type of research you are doing. Some case studies are structured like a standard scientific paper or thesis, with separate sections or chapters for the methods , results , and discussion .
Others are written in a more narrative style, aiming to explore the case from various angles and analyse its meanings and implications (for example, by using textual analysis or discourse analysis ).
In all cases, though, make sure to give contextual details about the case, connect it back to the literature and theory, and discuss how it fits into wider patterns or debates.
Cite this Scribbr article
If you want to cite this source, you can copy and paste the citation or click the ‘Cite this Scribbr article’ button to automatically add the citation to our free Reference Generator.
McCombes, S. (2023, January 30). Case Study | Definition, Examples & Methods. Scribbr. Retrieved 29 April 2024, from https://www.scribbr.co.uk/research-methods/case-studies/
Is this article helpful?
Shona McCombes
Other students also liked, correlational research | guide, design & examples, a quick guide to experimental design | 5 steps & examples, descriptive research design | definition, methods & examples.

Want to create or adapt books like this? Learn more about how Pressbooks supports open publishing practices.
2.2 Psychologists Use Descriptive, Correlational, and Experimental Research Designs to Understand Behavior
Learning objectives.
- Differentiate the goals of descriptive, correlational, and experimental research designs and explain the advantages and disadvantages of each.
- Explain the goals of descriptive research and the statistical techniques used to interpret it.
- Summarize the uses of correlational research and describe why correlational research cannot be used to infer causality.
- Review the procedures of experimental research and explain how it can be used to draw causal inferences.
Psychologists agree that if their ideas and theories about human behavior are to be taken seriously, they must be backed up by data. However, the research of different psychologists is designed with different goals in mind, and the different goals require different approaches. These varying approaches, summarized in Table 2.2 “Characteristics of the Three Research Designs” , are known as research designs . A research design is the specific method a researcher uses to collect, analyze, and interpret data . Psychologists use three major types of research designs in their research, and each provides an essential avenue for scientific investigation. Descriptive research is research designed to provide a snapshot of the current state of affairs . Correlational research is research designed to discover relationships among variables and to allow the prediction of future events from present knowledge . Experimental research is research in which initial equivalence among research participants in more than one group is created, followed by a manipulation of a given experience for these groups and a measurement of the influence of the manipulation . Each of the three research designs varies according to its strengths and limitations, and it is important to understand how each differs.
Table 2.2 Characteristics of the Three Research Designs
Stangor, C. (2011). Research methods for the behavioral sciences (4th ed.). Mountain View, CA: Cengage.
Descriptive Research: Assessing the Current State of Affairs
Descriptive research is designed to create a snapshot of the current thoughts, feelings, or behavior of individuals. This section reviews three types of descriptive research: case studies , surveys , and naturalistic observation .
Sometimes the data in a descriptive research project are based on only a small set of individuals, often only one person or a single small group. These research designs are known as case studies — descriptive records of one or more individual’s experiences and behavior . Sometimes case studies involve ordinary individuals, as when developmental psychologist Jean Piaget used his observation of his own children to develop his stage theory of cognitive development. More frequently, case studies are conducted on individuals who have unusual or abnormal experiences or characteristics or who find themselves in particularly difficult or stressful situations. The assumption is that by carefully studying individuals who are socially marginal, who are experiencing unusual situations, or who are going through a difficult phase in their lives, we can learn something about human nature.
Sigmund Freud was a master of using the psychological difficulties of individuals to draw conclusions about basic psychological processes. Freud wrote case studies of some of his most interesting patients and used these careful examinations to develop his important theories of personality. One classic example is Freud’s description of “Little Hans,” a child whose fear of horses the psychoanalyst interpreted in terms of repressed sexual impulses and the Oedipus complex (Freud (1909/1964).

Political polls reported in newspapers and on the Internet are descriptive research designs that provide snapshots of the likely voting behavior of a population.
Another well-known case study is Phineas Gage, a man whose thoughts and emotions were extensively studied by cognitive psychologists after a railroad spike was blasted through his skull in an accident. Although there is question about the interpretation of this case study (Kotowicz, 2007), it did provide early evidence that the brain’s frontal lobe is involved in emotion and morality (Damasio et al., 2005). An interesting example of a case study in clinical psychology is described by Rokeach (1964), who investigated in detail the beliefs and interactions among three patients with schizophrenia, all of whom were convinced they were Jesus Christ.
In other cases the data from descriptive research projects come in the form of a survey — a measure administered through either an interview or a written questionnaire to get a picture of the beliefs or behaviors of a sample of people of interest . The people chosen to participate in the research (known as the sample ) are selected to be representative of all the people that the researcher wishes to know about (the population ). In election polls, for instance, a sample is taken from the population of all “likely voters” in the upcoming elections.
The results of surveys may sometimes be rather mundane, such as “Nine out of ten doctors prefer Tymenocin,” or “The median income in Montgomery County is $36,712.” Yet other times (particularly in discussions of social behavior), the results can be shocking: “More than 40,000 people are killed by gunfire in the United States every year,” or “More than 60% of women between the ages of 50 and 60 suffer from depression.” Descriptive research is frequently used by psychologists to get an estimate of the prevalence (or incidence ) of psychological disorders.
A final type of descriptive research—known as naturalistic observation —is research based on the observation of everyday events . For instance, a developmental psychologist who watches children on a playground and describes what they say to each other while they play is conducting descriptive research, as is a biopsychologist who observes animals in their natural habitats. One example of observational research involves a systematic procedure known as the strange situation , used to get a picture of how adults and young children interact. The data that are collected in the strange situation are systematically coded in a coding sheet such as that shown in Table 2.3 “Sample Coding Form Used to Assess Child’s and Mother’s Behavior in the Strange Situation” .
Table 2.3 Sample Coding Form Used to Assess Child’s and Mother’s Behavior in the Strange Situation
The results of descriptive research projects are analyzed using descriptive statistics — numbers that summarize the distribution of scores on a measured variable . Most variables have distributions similar to that shown in Figure 2.5 “Height Distribution” , where most of the scores are located near the center of the distribution, and the distribution is symmetrical and bell-shaped. A data distribution that is shaped like a bell is known as a normal distribution .
Table 2.4 Height and Family Income for 25 Students
Figure 2.5 Height Distribution
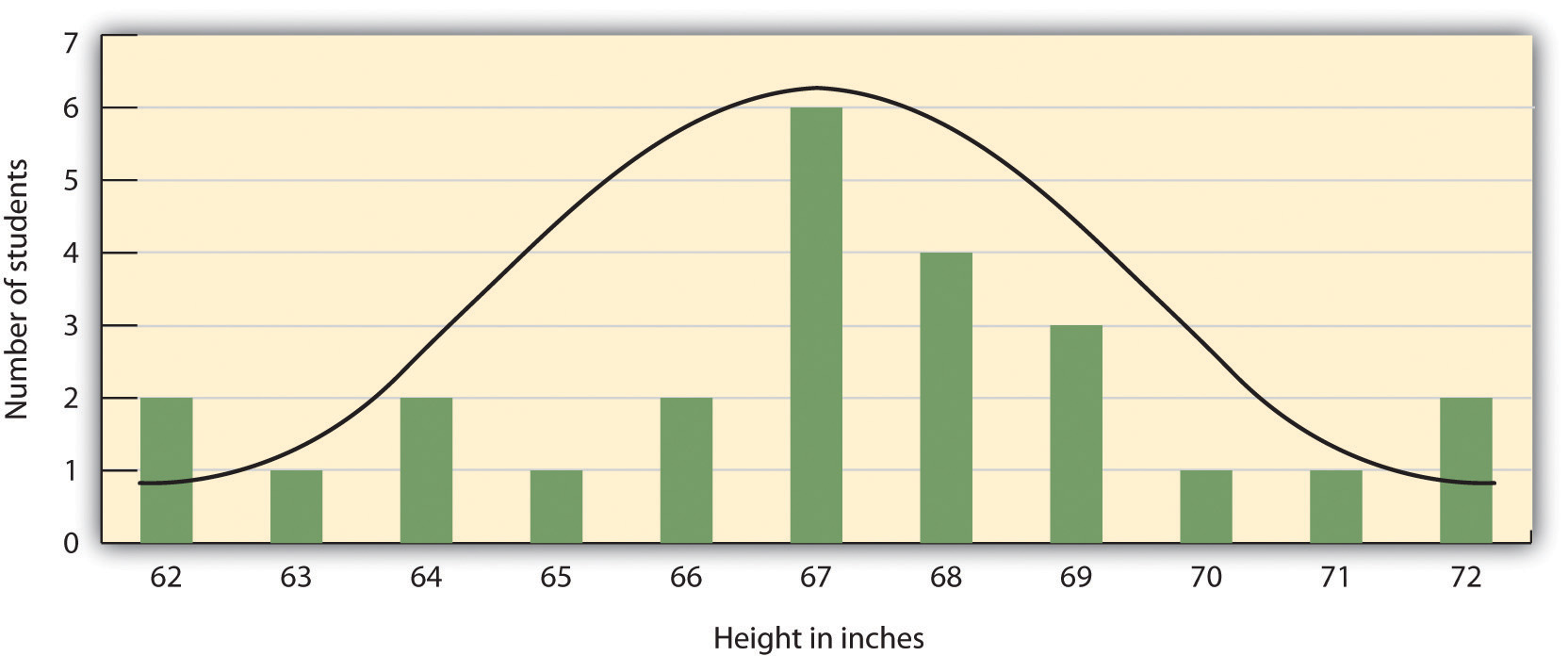
The distribution of the heights of the students in a class will form a normal distribution. In this sample the mean ( M ) = 67.12 and the standard deviation ( s ) = 2.74.
A distribution can be described in terms of its central tendency —that is, the point in the distribution around which the data are centered—and its dispersion , or spread. The arithmetic average, or arithmetic mean , is the most commonly used measure of central tendency . It is computed by calculating the sum of all the scores of the variable and dividing this sum by the number of participants in the distribution (denoted by the letter N ). In the data presented in Figure 2.5 “Height Distribution” , the mean height of the students is 67.12 inches. The sample mean is usually indicated by the letter M .
In some cases, however, the data distribution is not symmetrical. This occurs when there are one or more extreme scores (known as outliers ) at one end of the distribution. Consider, for instance, the variable of family income (see Figure 2.6 “Family Income Distribution” ), which includes an outlier (a value of $3,800,000). In this case the mean is not a good measure of central tendency. Although it appears from Figure 2.6 “Family Income Distribution” that the central tendency of the family income variable should be around $70,000, the mean family income is actually $223,960. The single very extreme income has a disproportionate impact on the mean, resulting in a value that does not well represent the central tendency.
The median is used as an alternative measure of central tendency when distributions are not symmetrical. The median is the score in the center of the distribution, meaning that 50% of the scores are greater than the median and 50% of the scores are less than the median . In our case, the median household income ($73,000) is a much better indication of central tendency than is the mean household income ($223,960).
Figure 2.6 Family Income Distribution
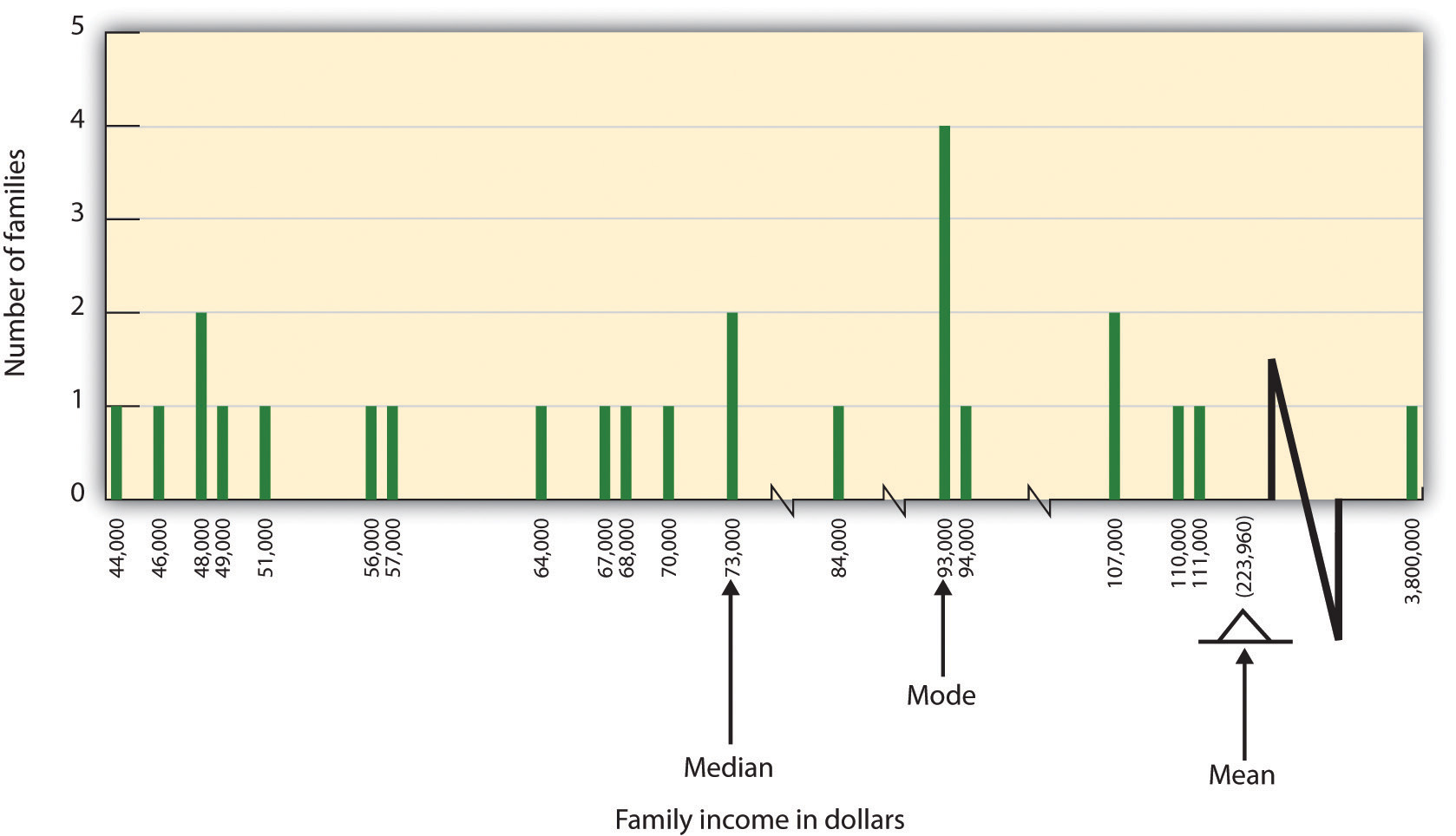
The distribution of family incomes is likely to be nonsymmetrical because some incomes can be very large in comparison to most incomes. In this case the median or the mode is a better indicator of central tendency than is the mean.
A final measure of central tendency, known as the mode , represents the value that occurs most frequently in the distribution . You can see from Figure 2.6 “Family Income Distribution” that the mode for the family income variable is $93,000 (it occurs four times).
In addition to summarizing the central tendency of a distribution, descriptive statistics convey information about how the scores of the variable are spread around the central tendency. Dispersion refers to the extent to which the scores are all tightly clustered around the central tendency, like this:
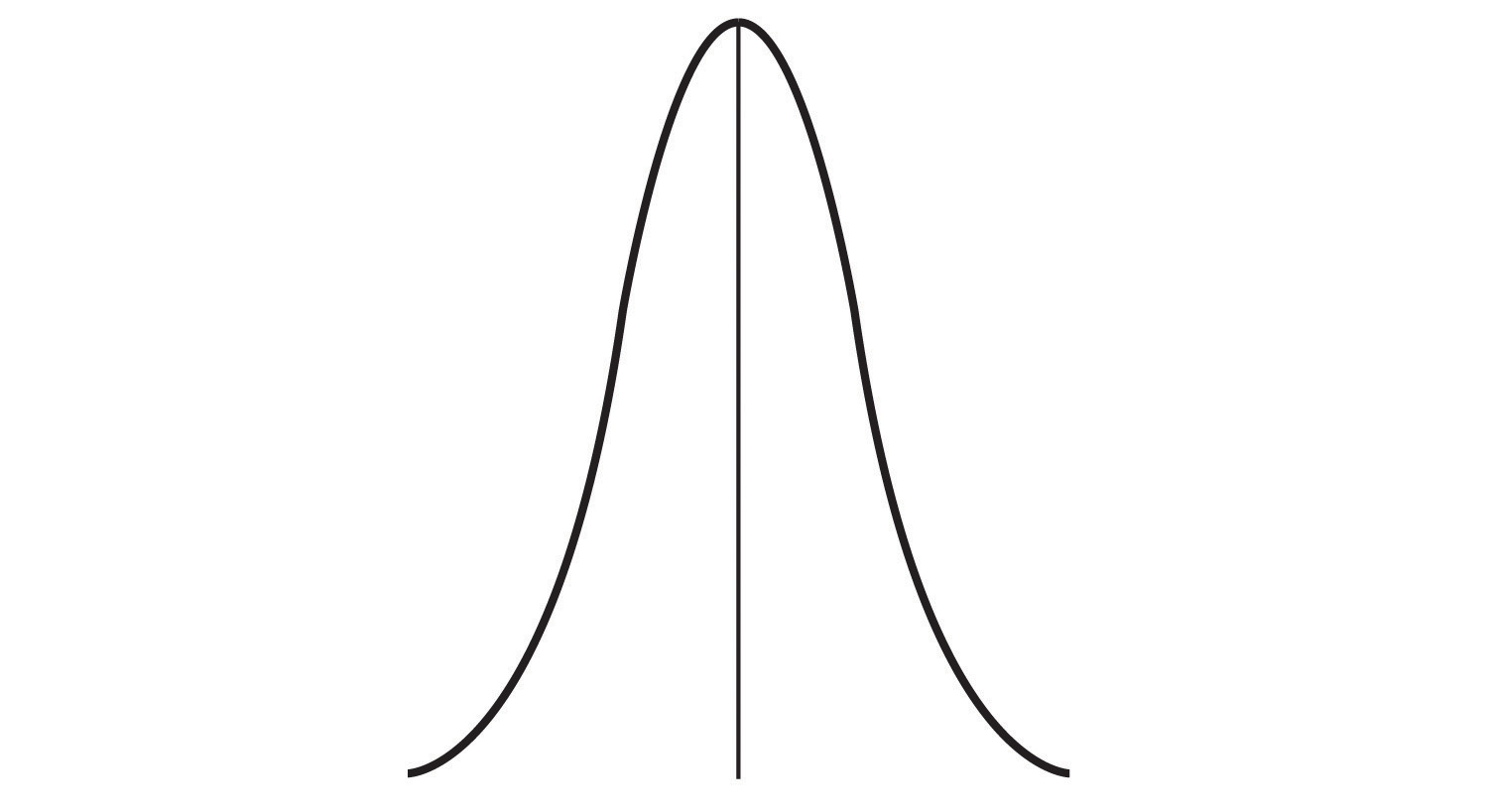
Or they may be more spread out away from it, like this:
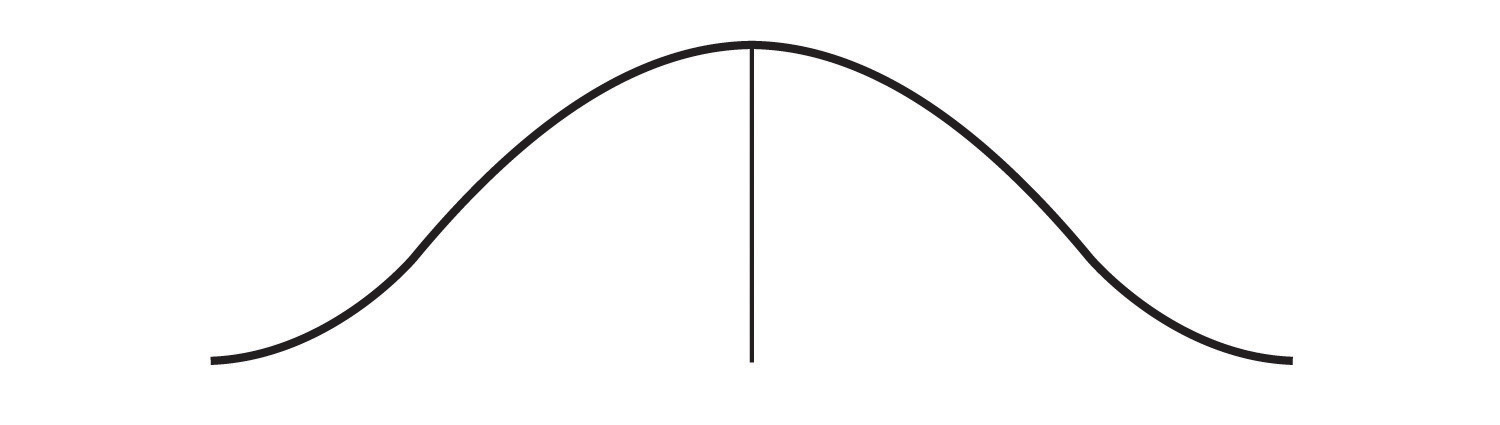
One simple measure of dispersion is to find the largest (the maximum ) and the smallest (the minimum ) observed values of the variable and to compute the range of the variable as the maximum observed score minus the minimum observed score. You can check that the range of the height variable in Figure 2.5 “Height Distribution” is 72 – 62 = 10. The standard deviation , symbolized as s , is the most commonly used measure of dispersion . Distributions with a larger standard deviation have more spread. The standard deviation of the height variable is s = 2.74, and the standard deviation of the family income variable is s = $745,337.
An advantage of descriptive research is that it attempts to capture the complexity of everyday behavior. Case studies provide detailed information about a single person or a small group of people, surveys capture the thoughts or reported behaviors of a large population of people, and naturalistic observation objectively records the behavior of people or animals as it occurs naturally. Thus descriptive research is used to provide a relatively complete understanding of what is currently happening.
Despite these advantages, descriptive research has a distinct disadvantage in that, although it allows us to get an idea of what is currently happening, it is usually limited to static pictures. Although descriptions of particular experiences may be interesting, they are not always transferable to other individuals in other situations, nor do they tell us exactly why specific behaviors or events occurred. For instance, descriptions of individuals who have suffered a stressful event, such as a war or an earthquake, can be used to understand the individuals’ reactions to the event but cannot tell us anything about the long-term effects of the stress. And because there is no comparison group that did not experience the stressful situation, we cannot know what these individuals would be like if they hadn’t had the stressful experience.
Correlational Research: Seeking Relationships Among Variables
In contrast to descriptive research, which is designed primarily to provide static pictures, correlational research involves the measurement of two or more relevant variables and an assessment of the relationship between or among those variables. For instance, the variables of height and weight are systematically related (correlated) because taller people generally weigh more than shorter people. In the same way, study time and memory errors are also related, because the more time a person is given to study a list of words, the fewer errors he or she will make. When there are two variables in the research design, one of them is called the predictor variable and the other the outcome variable . The research design can be visualized like this, where the curved arrow represents the expected correlation between the two variables:
Figure 2.2.2

One way of organizing the data from a correlational study with two variables is to graph the values of each of the measured variables using a scatter plot . As you can see in Figure 2.10 “Examples of Scatter Plots” , a scatter plot is a visual image of the relationship between two variables . A point is plotted for each individual at the intersection of his or her scores for the two variables. When the association between the variables on the scatter plot can be easily approximated with a straight line, as in parts (a) and (b) of Figure 2.10 “Examples of Scatter Plots” , the variables are said to have a linear relationship .
When the straight line indicates that individuals who have above-average values for one variable also tend to have above-average values for the other variable, as in part (a), the relationship is said to be positive linear . Examples of positive linear relationships include those between height and weight, between education and income, and between age and mathematical abilities in children. In each case people who score higher on one of the variables also tend to score higher on the other variable. Negative linear relationships , in contrast, as shown in part (b), occur when above-average values for one variable tend to be associated with below-average values for the other variable. Examples of negative linear relationships include those between the age of a child and the number of diapers the child uses, and between practice on and errors made on a learning task. In these cases people who score higher on one of the variables tend to score lower on the other variable.
Relationships between variables that cannot be described with a straight line are known as nonlinear relationships . Part (c) of Figure 2.10 “Examples of Scatter Plots” shows a common pattern in which the distribution of the points is essentially random. In this case there is no relationship at all between the two variables, and they are said to be independent . Parts (d) and (e) of Figure 2.10 “Examples of Scatter Plots” show patterns of association in which, although there is an association, the points are not well described by a single straight line. For instance, part (d) shows the type of relationship that frequently occurs between anxiety and performance. Increases in anxiety from low to moderate levels are associated with performance increases, whereas increases in anxiety from moderate to high levels are associated with decreases in performance. Relationships that change in direction and thus are not described by a single straight line are called curvilinear relationships .
Figure 2.10 Examples of Scatter Plots
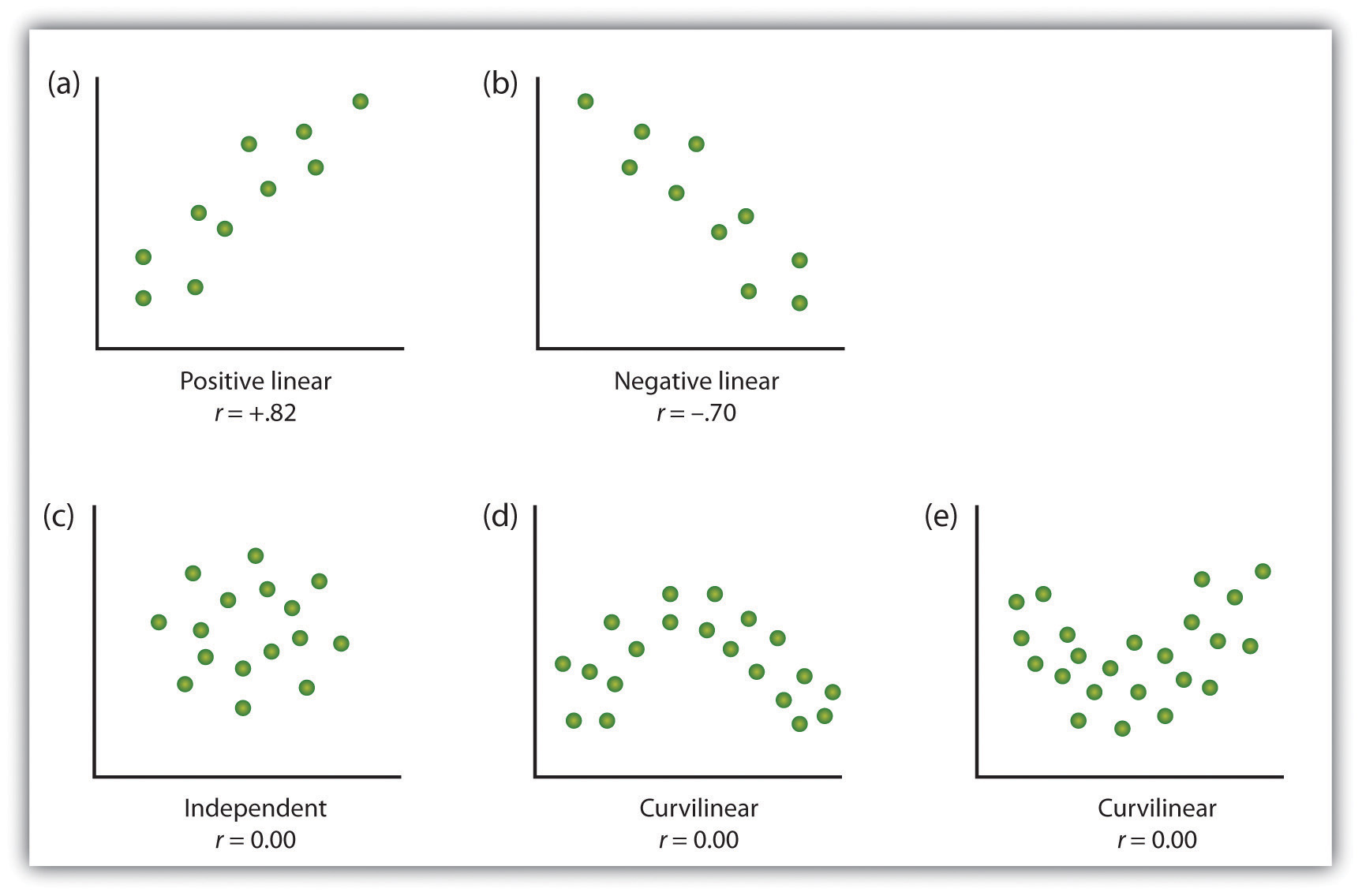
Some examples of relationships between two variables as shown in scatter plots. Note that the Pearson correlation coefficient ( r ) between variables that have curvilinear relationships will likely be close to zero.
Adapted from Stangor, C. (2011). Research methods for the behavioral sciences (4th ed.). Mountain View, CA: Cengage.
The most common statistical measure of the strength of linear relationships among variables is the Pearson correlation coefficient , which is symbolized by the letter r . The value of the correlation coefficient ranges from r = –1.00 to r = +1.00. The direction of the linear relationship is indicated by the sign of the correlation coefficient. Positive values of r (such as r = .54 or r = .67) indicate that the relationship is positive linear (i.e., the pattern of the dots on the scatter plot runs from the lower left to the upper right), whereas negative values of r (such as r = –.30 or r = –.72) indicate negative linear relationships (i.e., the dots run from the upper left to the lower right). The strength of the linear relationship is indexed by the distance of the correlation coefficient from zero (its absolute value). For instance, r = –.54 is a stronger relationship than r = .30, and r = .72 is a stronger relationship than r = –.57. Because the Pearson correlation coefficient only measures linear relationships, variables that have curvilinear relationships are not well described by r , and the observed correlation will be close to zero.
It is also possible to study relationships among more than two measures at the same time. A research design in which more than one predictor variable is used to predict a single outcome variable is analyzed through multiple regression (Aiken & West, 1991). Multiple regression is a statistical technique, based on correlation coefficients among variables, that allows predicting a single outcome variable from more than one predictor variable . For instance, Figure 2.11 “Prediction of Job Performance From Three Predictor Variables” shows a multiple regression analysis in which three predictor variables are used to predict a single outcome. The use of multiple regression analysis shows an important advantage of correlational research designs—they can be used to make predictions about a person’s likely score on an outcome variable (e.g., job performance) based on knowledge of other variables.
Figure 2.11 Prediction of Job Performance From Three Predictor Variables
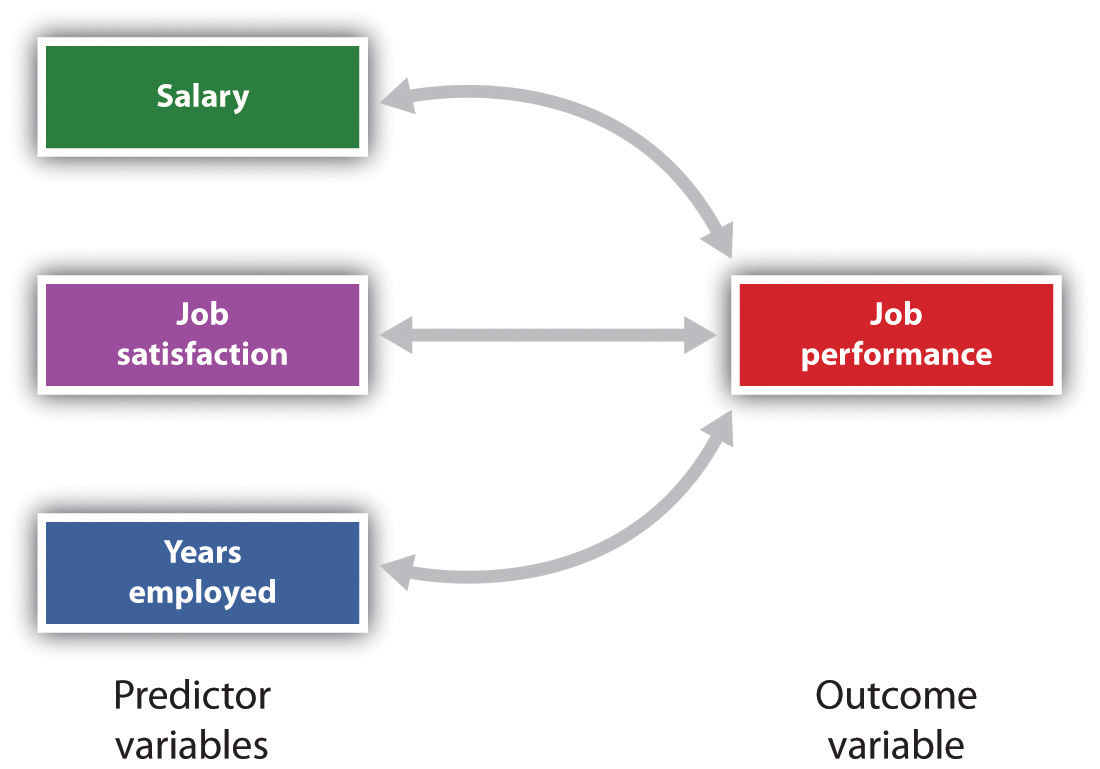
Multiple regression allows scientists to predict the scores on a single outcome variable using more than one predictor variable.
An important limitation of correlational research designs is that they cannot be used to draw conclusions about the causal relationships among the measured variables. Consider, for instance, a researcher who has hypothesized that viewing violent behavior will cause increased aggressive play in children. He has collected, from a sample of fourth-grade children, a measure of how many violent television shows each child views during the week, as well as a measure of how aggressively each child plays on the school playground. From his collected data, the researcher discovers a positive correlation between the two measured variables.
Although this positive correlation appears to support the researcher’s hypothesis, it cannot be taken to indicate that viewing violent television causes aggressive behavior. Although the researcher is tempted to assume that viewing violent television causes aggressive play,

there are other possibilities. One alternate possibility is that the causal direction is exactly opposite from what has been hypothesized. Perhaps children who have behaved aggressively at school develop residual excitement that leads them to want to watch violent television shows at home:

Although this possibility may seem less likely, there is no way to rule out the possibility of such reverse causation on the basis of this observed correlation. It is also possible that both causal directions are operating and that the two variables cause each other:

Still another possible explanation for the observed correlation is that it has been produced by the presence of a common-causal variable (also known as a third variable ). A common-causal variable is a variable that is not part of the research hypothesis but that causes both the predictor and the outcome variable and thus produces the observed correlation between them . In our example a potential common-causal variable is the discipline style of the children’s parents. Parents who use a harsh and punitive discipline style may produce children who both like to watch violent television and who behave aggressively in comparison to children whose parents use less harsh discipline:

In this case, television viewing and aggressive play would be positively correlated (as indicated by the curved arrow between them), even though neither one caused the other but they were both caused by the discipline style of the parents (the straight arrows). When the predictor and outcome variables are both caused by a common-causal variable, the observed relationship between them is said to be spurious . A spurious relationship is a relationship between two variables in which a common-causal variable produces and “explains away” the relationship . If effects of the common-causal variable were taken away, or controlled for, the relationship between the predictor and outcome variables would disappear. In the example the relationship between aggression and television viewing might be spurious because by controlling for the effect of the parents’ disciplining style, the relationship between television viewing and aggressive behavior might go away.
Common-causal variables in correlational research designs can be thought of as “mystery” variables because, as they have not been measured, their presence and identity are usually unknown to the researcher. Since it is not possible to measure every variable that could cause both the predictor and outcome variables, the existence of an unknown common-causal variable is always a possibility. For this reason, we are left with the basic limitation of correlational research: Correlation does not demonstrate causation. It is important that when you read about correlational research projects, you keep in mind the possibility of spurious relationships, and be sure to interpret the findings appropriately. Although correlational research is sometimes reported as demonstrating causality without any mention being made of the possibility of reverse causation or common-causal variables, informed consumers of research, like you, are aware of these interpretational problems.
In sum, correlational research designs have both strengths and limitations. One strength is that they can be used when experimental research is not possible because the predictor variables cannot be manipulated. Correlational designs also have the advantage of allowing the researcher to study behavior as it occurs in everyday life. And we can also use correlational designs to make predictions—for instance, to predict from the scores on their battery of tests the success of job trainees during a training session. But we cannot use such correlational information to determine whether the training caused better job performance. For that, researchers rely on experiments.
Experimental Research: Understanding the Causes of Behavior
The goal of experimental research design is to provide more definitive conclusions about the causal relationships among the variables in the research hypothesis than is available from correlational designs. In an experimental research design, the variables of interest are called the independent variable (or variables ) and the dependent variable . The independent variable in an experiment is the causing variable that is created (manipulated) by the experimenter . The dependent variable in an experiment is a measured variable that is expected to be influenced by the experimental manipulation . The research hypothesis suggests that the manipulated independent variable or variables will cause changes in the measured dependent variables. We can diagram the research hypothesis by using an arrow that points in one direction. This demonstrates the expected direction of causality:
Figure 2.2.3

Research Focus: Video Games and Aggression
Consider an experiment conducted by Anderson and Dill (2000). The study was designed to test the hypothesis that viewing violent video games would increase aggressive behavior. In this research, male and female undergraduates from Iowa State University were given a chance to play with either a violent video game (Wolfenstein 3D) or a nonviolent video game (Myst). During the experimental session, the participants played their assigned video games for 15 minutes. Then, after the play, each participant played a competitive game with an opponent in which the participant could deliver blasts of white noise through the earphones of the opponent. The operational definition of the dependent variable (aggressive behavior) was the level and duration of noise delivered to the opponent. The design of the experiment is shown in Figure 2.17 “An Experimental Research Design” .
Figure 2.17 An Experimental Research Design
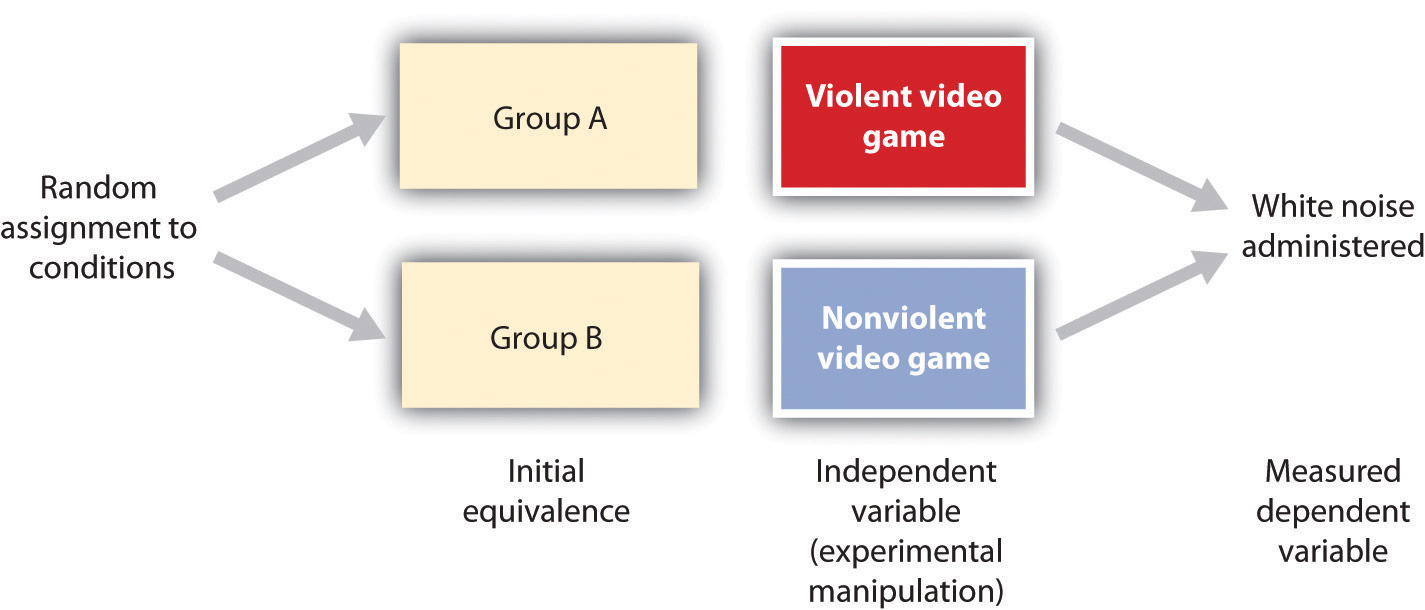
Two advantages of the experimental research design are (1) the assurance that the independent variable (also known as the experimental manipulation) occurs prior to the measured dependent variable, and (2) the creation of initial equivalence between the conditions of the experiment (in this case by using random assignment to conditions).
Experimental designs have two very nice features. For one, they guarantee that the independent variable occurs prior to the measurement of the dependent variable. This eliminates the possibility of reverse causation. Second, the influence of common-causal variables is controlled, and thus eliminated, by creating initial equivalence among the participants in each of the experimental conditions before the manipulation occurs.
The most common method of creating equivalence among the experimental conditions is through random assignment to conditions , a procedure in which the condition that each participant is assigned to is determined through a random process, such as drawing numbers out of an envelope or using a random number table . Anderson and Dill first randomly assigned about 100 participants to each of their two groups (Group A and Group B). Because they used random assignment to conditions, they could be confident that, before the experimental manipulation occurred, the students in Group A were, on average, equivalent to the students in Group B on every possible variable, including variables that are likely to be related to aggression, such as parental discipline style, peer relationships, hormone levels, diet—and in fact everything else.
Then, after they had created initial equivalence, Anderson and Dill created the experimental manipulation—they had the participants in Group A play the violent game and the participants in Group B play the nonviolent game. Then they compared the dependent variable (the white noise blasts) between the two groups, finding that the students who had viewed the violent video game gave significantly longer noise blasts than did the students who had played the nonviolent game.
Anderson and Dill had from the outset created initial equivalence between the groups. This initial equivalence allowed them to observe differences in the white noise levels between the two groups after the experimental manipulation, leading to the conclusion that it was the independent variable (and not some other variable) that caused these differences. The idea is that the only thing that was different between the students in the two groups was the video game they had played.
Despite the advantage of determining causation, experiments do have limitations. One is that they are often conducted in laboratory situations rather than in the everyday lives of people. Therefore, we do not know whether results that we find in a laboratory setting will necessarily hold up in everyday life. Second, and more important, is that some of the most interesting and key social variables cannot be experimentally manipulated. If we want to study the influence of the size of a mob on the destructiveness of its behavior, or to compare the personality characteristics of people who join suicide cults with those of people who do not join such cults, these relationships must be assessed using correlational designs, because it is simply not possible to experimentally manipulate these variables.
Key Takeaways
- Descriptive, correlational, and experimental research designs are used to collect and analyze data.
- Descriptive designs include case studies, surveys, and naturalistic observation. The goal of these designs is to get a picture of the current thoughts, feelings, or behaviors in a given group of people. Descriptive research is summarized using descriptive statistics.
- Correlational research designs measure two or more relevant variables and assess a relationship between or among them. The variables may be presented on a scatter plot to visually show the relationships. The Pearson Correlation Coefficient ( r ) is a measure of the strength of linear relationship between two variables.
- Common-causal variables may cause both the predictor and outcome variable in a correlational design, producing a spurious relationship. The possibility of common-causal variables makes it impossible to draw causal conclusions from correlational research designs.
- Experimental research involves the manipulation of an independent variable and the measurement of a dependent variable. Random assignment to conditions is normally used to create initial equivalence between the groups, allowing researchers to draw causal conclusions.
Exercises and Critical Thinking
- There is a negative correlation between the row that a student sits in in a large class (when the rows are numbered from front to back) and his or her final grade in the class. Do you think this represents a causal relationship or a spurious relationship, and why?
- Think of two variables (other than those mentioned in this book) that are likely to be correlated, but in which the correlation is probably spurious. What is the likely common-causal variable that is producing the relationship?
- Imagine a researcher wants to test the hypothesis that participating in psychotherapy will cause a decrease in reported anxiety. Describe the type of research design the investigator might use to draw this conclusion. What would be the independent and dependent variables in the research?
Aiken, L., & West, S. (1991). Multiple regression: Testing and interpreting interactions . Newbury Park, CA: Sage.
Ainsworth, M. S., Blehar, M. C., Waters, E., & Wall, S. (1978). Patterns of attachment: A psychological study of the strange situation . Hillsdale, NJ: Lawrence Erlbaum Associates.
Anderson, C. A., & Dill, K. E. (2000). Video games and aggressive thoughts, feelings, and behavior in the laboratory and in life. Journal of Personality and Social Psychology, 78 (4), 772–790.
Damasio, H., Grabowski, T., Frank, R., Galaburda, A. M., Damasio, A. R., Cacioppo, J. T., & Berntson, G. G. (2005). The return of Phineas Gage: Clues about the brain from the skull of a famous patient. In Social neuroscience: Key readings. (pp. 21–28). New York, NY: Psychology Press.
Freud, S. (1964). Analysis of phobia in a five-year-old boy. In E. A. Southwell & M. Merbaum (Eds.), Personality: Readings in theory and research (pp. 3–32). Belmont, CA: Wadsworth. (Original work published 1909)
Kotowicz, Z. (2007). The strange case of Phineas Gage. History of the Human Sciences, 20 (1), 115–131.
Rokeach, M. (1964). The three Christs of Ypsilanti: A psychological study . New York, NY: Knopf.
Introduction to Psychology Copyright © 2015 by University of Minnesota is licensed under a Creative Commons Attribution-NonCommercial-ShareAlike 4.0 International License , except where otherwise noted.
Experimental Studies and Observational Studies
- Reference work entry
- First Online: 01 January 2022
- pp 1748–1756
- Cite this reference work entry
- Martin Pinquart 3
742 Accesses
1 Citations
Experimental studies: Experiments, Randomized controlled trials (RCTs) ; Observational studies: Non-experimental studies, Non-manipulation studies, Naturalistic studies
Definitions
The experimental study is a powerful methodology for testing causal relations between one or more explanatory variables (i.e., independent variables) and one or more outcome variables (i.e., dependent variable). In order to accomplish this goal, experiments have to meet three basic criteria: (a) experimental manipulation (variation) of the independent variable(s), (b) randomization – the participants are randomly assigned to one of the experimental conditions, and (c) experimental control for the effect of third variables by eliminating them or keeping them constant.
In observational studies, investigators observe or assess individuals without manipulation or intervention. Observational studies are used for assessing the mean levels, the natural variation, and the structure of variables, as well as...
This is a preview of subscription content, log in via an institution to check access.

Access this chapter
Institutional subscriptions
Atalay K, Barrett GF (2015) The impact of age pension eligibility age on retirement and program dependence: evidence from an Australian experiment. Rev Econ Stat 97:71–87. https://doi.org/10.1162/REST_a_00443
Article Google Scholar
Bergeman L, Boker SM (eds) (2016) Methodological issues in aging research. Psychology Press, Hove
Google Scholar
Byrkes CR, Bielak AMA (under review) Evaluation of publication bias and statistical power in gerontological psychology. Manuscript submitted for publication
Campbell DT, Stanley JC (1966) Experimental and quasi-experimental designs for research. Rand-McNally, Chicago
Carpenter D (2010) Reputation and power: organizational image and pharmaceutical regulation at the FDA. Princeton University Press, Princeton
Cavanaugh JC, Blanchard-Fields F (2019) Adult development and aging, 8th edn. Cengage, Boston
Fölster M, Hess U, Hühnel I et al (2015) Age-related response bias in the decoding of sad facial expressions. Behav Sci 5:443–460. https://doi.org/10.3390/bs5040443
Freund AM, Isaacowitz DM (2013) Beyond age comparisons: a plea for the use of a modified Brunswikian approach to experimental designs in the study of adult development and aging. Hum Dev 56:351–371. https://doi.org/10.1159/000357177
Haslam C, Morton TA, Haslam A et al (2012) “When the age is in, the wit is out”: age-related self-categorization and deficit expectations reduce performance on clinical tests used in dementia assessment. Psychol Aging 27:778–784. https://doi.org/10.1037/a0027754
Institute for Social Research (2018) The health and retirement study. Aging in the 21st century: Challenges and opportunities for americans. Survey Research Center, University of Michigan
Jung J (1971) The experimenter’s dilemma. Harper & Row, New York
Leary MR (2001) Introduction to behavioral research methods, 3rd edn. Allyn & Bacon, Boston
Lindenberger U, Scherer H, Baltes PB (2001) The strong connection between sensory and cognitive performance in old age: not due to sensory acuity reductions operating during cognitive assessment. Psychol Aging 16:196–205. https://doi.org/10.1037//0882-7974.16.2.196
Löckenhoff CE, Carstensen LL (2004) Socioemotional selectivity theory, aging, and health: the increasingly delicate balance between regulating emotions and making tough choices. J Pers 72:1395–1424. https://doi.org/10.1111/j.1467-6494.2004.00301.x
Maxwell SE (2015) Is psychology suffering from a replication crisis? What does “failure to replicate” really mean? Am Psychol 70:487–498. https://doi.org/10.1037/a0039400
Menard S (2002) Longitudinal research (2nd ed.). Sage, Thousand Oaks, CA
Mitchell SJ, Scheibye-Knudsen M, Longo DL et al (2015) Animal models of aging research: implications for human aging and age-related diseases. Ann Rev Anim Biosci 3:283–303. https://doi.org/10.1146/annurev-animal-022114-110829
Moher D (1998) CONSORT: an evolving tool to help improve the quality of reports of randomized controlled trials. JAMA 279:1489–1491. https://doi.org/10.1001/jama.279.18.1489
Oxford Centre for Evidence-Based Medicine (2011) OCEBM levels of evidence working group. The Oxford Levels of Evidence 2. Available at: https://www.cebm.net/category/ebm-resources/loe/ . Retrieved 2018-12-12
Patten ML, Newhart M (2018) Understanding research methods: an overview of the essentials, 10th edn. Routledge, New York
Piccinin AM, Muniz G, Sparks C et al (2011) An evaluation of analytical approaches for understanding change in cognition in the context of aging and health. J Geront 66B(S1):i36–i49. https://doi.org/10.1093/geronb/gbr038
Pinquart M, Silbereisen RK (2006) Socioemotional selectivity in cancer patients. Psychol Aging 21:419–423. https://doi.org/10.1037/0882-7974.21.2.419
Redman LM, Ravussin E (2011) Caloric restriction in humans: impact on physiological, psychological, and behavioral outcomes. Antioxid Redox Signal 14:275–287. https://doi.org/10.1089/ars.2010.3253
Rutter M (2007) Proceeding from observed correlation to causal inference: the use of natural experiments. Perspect Psychol Sci 2:377–395. https://doi.org/10.1111/j.1745-6916.2007.00050.x
Schaie W, Caskle CI (2005) Methodological issues in aging research. In: Teti D (ed) Handbook of research methods in developmental science. Blackwell, Malden, pp 21–39
Shadish WR, Cook TD, Campbell DT (2002) Experimental and quasi-experimental designs for generalized causal inference. Houghton Mifflin, Boston
Sonnega A, Faul JD, Ofstedal MB et al (2014) Cohort profile: the health and retirement study (HRS). Int J Epidemiol 43:576–585. https://doi.org/10.1093/ije/dyu067
Weil J (2017) Research design in aging and social gerontology: quantitative, qualitative, and mixed methods. Routledge, New York
Download references
Author information
Authors and affiliations.
Psychology, Philipps University, Marburg, Germany
Martin Pinquart
You can also search for this author in PubMed Google Scholar
Corresponding author
Correspondence to Martin Pinquart .
Editor information
Editors and affiliations.
Population Division, Department of Economics and Social Affairs, United Nations, New York, NY, USA
Department of Population Health Sciences, Department of Sociology, Duke University, Durham, NC, USA
Matthew E. Dupre
Section Editor information
Department of Sociology and Center for Population Health and Aging, Duke University, Durham, NC, USA
Kenneth C. Land
Department of Sociology, University of Kentucky, Lexington, KY, USA
Anthony R. Bardo
Rights and permissions
Reprints and permissions
Copyright information
© 2021 Springer Nature Switzerland AG
About this entry
Cite this entry.
Pinquart, M. (2021). Experimental Studies and Observational Studies. In: Gu, D., Dupre, M.E. (eds) Encyclopedia of Gerontology and Population Aging. Springer, Cham. https://doi.org/10.1007/978-3-030-22009-9_573
Download citation
DOI : https://doi.org/10.1007/978-3-030-22009-9_573
Published : 24 May 2022
Publisher Name : Springer, Cham
Print ISBN : 978-3-030-22008-2
Online ISBN : 978-3-030-22009-9
eBook Packages : Social Sciences Reference Module Humanities and Social Sciences Reference Module Business, Economics and Social Sciences
Share this entry
Anyone you share the following link with will be able to read this content:
Sorry, a shareable link is not currently available for this article.
Provided by the Springer Nature SharedIt content-sharing initiative
- Publish with us
Policies and ethics
- Find a journal
- Track your research
- Key Differences
Know the Differences & Comparisons
Difference Between Survey and Experiment
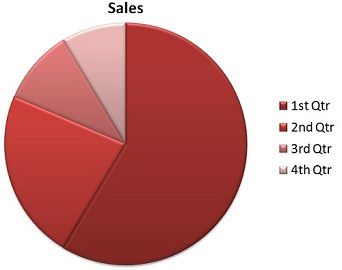
While surveys collected data, provided by the informants, experiments test various premises by trial and error method. This article attempts to shed light on the difference between survey and experiment, have a look.
Content: Survey Vs Experiment
Comparison chart, definition of survey.
By the term survey, we mean a method of securing information relating to the variable under study from all or a specified number of respondents of the universe. It may be a sample survey or a census survey. This method relies on the questioning of the informants on a specific subject. Survey follows structured form of data collection, in which a formal questionnaire is prepared, and the questions are asked in a predefined order.
Informants are asked questions concerning their behaviour, attitude, motivation, demographic, lifestyle characteristics, etc. through observation, direct communication with them over telephone/mail or personal interview. Questions are asked verbally to the respondents, i.e. in writing or by way of computer. The answer of the respondents is obtained in the same form.
Definition of Experiment
The term experiment means a systematic and logical scientific procedure in which one or more independent variables under test are manipulated, and any change on one or more dependent variable is measured while controlling for the effect of the extraneous variable. Here extraneous variable is an independent variable which is not associated with the objective of study but may affect the response of test units.
In an experiment, the investigator attempts to observe the outcome of the experiment conducted by him intentionally, to test the hypothesis or to discover something or to demonstrate a known fact. An experiment aims at drawing conclusions concerning the factor on the study group and making inferences from sample to larger population of interest.
Key Differences Between Survey and Experiment
The differences between survey and experiment can be drawn clearly on the following grounds:
- A technique of gathering information regarding a variable under study, from the respondents of the population, is called survey. A scientific procedure wherein the factor under study is isolated to test hypothesis is called an experiment.
- Surveys are performed when the research is of descriptive nature, whereas in the case of experiments are conducted in experimental research.
- The survey samples are large as the response rate is low, especially when the survey is conducted through mailed questionnaire. On the other hand, samples required in the case of experiments is relatively small.
- Surveys are considered suitable for social and behavioural science. As against this, experiments are an important characteristic of physical and natural sciences.
- Field research refers to the research conducted outside the laboratory or workplace. Surveys are the best example of field research. On the contrary, Experiment is an example of laboratory research. A laboratory research is nothing but research carried on inside the room equipped with scientific tools and equipment.
- In surveys, the data collection methods employed can either be observation, interview, questionnaire, or case study. As opposed to experiment, the data is obtained through several readings of the experiment.
While survey studies the possible relationship between data and unknown variable, experiments determine the relationship. Further, Correlation analysis is vital in surveys, as in social and business surveys, the interest of the researcher rests in understanding and controlling relationships between variables. Unlike experiments, where casual analysis is significant.
You Might Also Like:
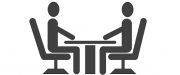
sanjay kumar yadav says
November 17, 2016 at 1:08 am
Ishika says
September 9, 2017 at 9:30 pm
The article was quite helpful… Thank you.
May 21, 2018 at 3:26 pm
Can you develop your Application for Android
Surbhi S says
May 21, 2018 at 4:21 pm
Yeah, we will develop android app soon.
October 31, 2018 at 12:32 am
If I was doing an experiment with Poverty and Education level, which do you think would be more appropriate for me?
Thanks, Chris
Ndaware M.M says
January 7, 2021 at 2:29 am
So interested,
Victoria Addington says
May 18, 2023 at 5:31 pm
Thank you for explaining the topic
Leave a Reply Cancel reply
Your email address will not be published. Required fields are marked *
Save my name, email, and website in this browser for the next time I comment.
- - Google Chrome
Intended for healthcare professionals
- Access provided by Google Indexer
- My email alerts
- BMA member login
- Username * Password * Forgot your log in details? Need to activate BMA Member Log In Log in via OpenAthens Log in via your institution

Search form
- Advanced search
- Search responses
- Search blogs
- Efficacy of psilocybin...
Efficacy of psilocybin for treating symptoms of depression: systematic review and meta-analysis
Linked editorial.
Psilocybin for depression
- Related content
- Peer review
- Athina-Marina Metaxa , masters graduate researcher 1 ,
- Mike Clarke , professor 2
- 1 Nuffield Department of Primary Care Health Sciences, University of Oxford, Oxford OX2 6GG, UK
- 2 Northern Ireland Methodology Hub, Centre for Public Health, ICS-A Royal Hospitals, Belfast, Ireland, UK
- Correspondence to: A-M Metaxa athina.metaxa{at}hmc.ox.ac.uk (or @Athina_Metaxa12 on X)
- Accepted 6 March 2024
Objective To determine the efficacy of psilocybin as an antidepressant compared with placebo or non-psychoactive drugs.
Design Systematic review and meta-analysis.
Data sources Five electronic databases of published literature (Cochrane Central Register of Controlled Trials, Medline, Embase, Science Citation Index and Conference Proceedings Citation Index, and PsycInfo) and four databases of unpublished and international literature (ClinicalTrials.gov, WHO International Clinical Trials Registry Platform, ProQuest Dissertations and Theses Global, and PsycEXTRA), and handsearching of reference lists, conference proceedings, and abstracts.
Data synthesis and study quality Information on potential treatment effect moderators was extracted, including depression type (primary or secondary), previous use of psychedelics, psilocybin dosage, type of outcome measure (clinician rated or self-reported), and personal characteristics (eg, age, sex). Data were synthesised using a random effects meta-analysis model, and observed heterogeneity and the effect of covariates were investigated with subgroup analyses and metaregression. Hedges’ g was used as a measure of treatment effect size, to account for small sample effects and substantial differences between the included studies’ sample sizes. Study quality was appraised using Cochrane’s Risk of Bias 2 tool, and the quality of the aggregated evidence was evaluated using GRADE guidelines.
Eligibility criteria Randomised trials in which psilocybin was administered as a standalone treatment for adults with clinically significant symptoms of depression and change in symptoms was measured using a validated clinician rated or self-report scale. Studies with directive psychotherapy were included if the psychotherapeutic component was present in both experimental and control conditions. Participants with depression regardless of comorbidities (eg, cancer) were eligible.
Results Meta-analysis on 436 participants (228 female participants), average age 36-60 years, from seven of the nine included studies showed a significant benefit of psilocybin (Hedges’ g=1.64, 95% confidence interval (CI) 0.55 to 2.73, P<0.001) on change in depression scores compared with comparator treatment. Subgroup analyses and metaregressions indicated that having secondary depression (Hedges’ g=3.25, 95% CI 0.97 to 5.53), being assessed with self-report depression scales such as the Beck depression inventory (3.25, 0.97 to 5.53), and older age and previous use of psychedelics (metaregression coefficient 0.16, 95% CI 0.08 to 0.24 and 4.2, 1.5 to 6.9, respectively) were correlated with greater improvements in symptoms. All studies had a low risk of bias, but the change from baseline metric was associated with high heterogeneity and a statistically significant risk of small study bias, resulting in a low certainty of evidence rating.
Conclusion Treatment effects of psilocybin were significantly larger among patients with secondary depression, when self-report scales were used to measure symptoms of depression, and when participants had previously used psychedelics. Further research is thus required to delineate the influence of expectancy effects, moderating factors, and treatment delivery on the efficacy of psilocybin as an antidepressant.
Systematic review registration PROSPERO CRD42023388065.

- Download figure
- Open in new tab
- Download powerpoint
Introduction
Depression affects an estimated 300 million people around the world, an increase of nearly 20% over the past decade. 1 Worldwide, depression is also the leading cause of disability. 2
Drugs for depression are widely available but these seem to have limited efficacy, can have serious adverse effects, and are associated with low patient adherence. 3 4 Importantly, the treatment effects of antidepressant drugs do not appear until 4-7 weeks after the start of treatment, and remission of symptoms can take months. 4 5 Additionally, the likelihood of relapse is high, with 40-60% of people with depression experiencing a further depressive episode, and the chance of relapse increasing with each subsequent episode. 6 7
Since the early 2000s, the naturally occurring serotonergic hallucinogen psilocybin, found in several species of mushrooms, has been widely discussed as a potential treatment for depression. 8 9 Psilocybin’s mechanism of action differs from that of classic selective serotonin reuptake inhibitors (SSRIs) and might improve the treatment response rate, decrease time to improvement of symptoms, and prevent relapse post-remission. Moreover, more recent assessments of harm have consistently reported that psilocybin generally has low addictive potential and toxicity and that it can be administered safely under clinical supervision. 10
The renewed interest in psilocybin’s antidepressive effects led to several clinical trials on treatment resistant depression, 11 12 major depressive disorder, 13 and depression related to physical illness. 14 15 16 17 These trials mostly reported positive efficacy findings, showing reductions in symptoms of depression within a few hours to a few days after one dose or two doses of psilocybin. 11 12 13 16 17 18 These studies reported only minimal adverse effects, however, and drug harm assessments in healthy volunteers indicated that psilocybin does not induce physiological toxicity, is not addictive, and does not lead to withdrawal. 19 20 Nevertheless, these findings should be interpreted with caution owing to the small sample sizes and open label design of some of these studies. 11 21
Several systematic reviews and meta-analyses since the early 2000s have investigated the use of psilocybin to treat symptoms of depression. Most found encouraging results, but as well as people with depression some included healthy volunteers, 22 and most combined data from studies of multiple serotonergic psychedelics, 23 24 25 even though each compound has unique neurobiological effects and mechanisms of action. 26 27 28 Furthermore, many systematic reviews included non-randomised studies and studies in which psilocybin was tested in conjunction with psychotherapeutic interventions, 25 29 30 31 32 which made it difficult to distinguish psilocybin’s treatment effects. Most systematic reviews and meta-analyses did not consider the impact of factors that could act as moderators to psilocybin’s effects, such as type of depression (primary or secondary), previous use of psychedelics, psilocybin dosage, type of outcome measure (clinician rated or self-reported), and personal characteristics (eg, age, sex). 25 26 29 30 31 32 Lastly, systematic reviews did not consider grey literature, 33 34 which might have led to a substantial overestimation of psilocybin’s efficacy as a treatment for depression. In this review we focused on randomised trials that contained an unconfounded evaluation of psilocybin in adults with symptoms of depression, regardless of country and language of publication.
In this systematic review and meta-analysis of indexed and non-indexed randomised trials we investigated the efficacy of psilocybin to treat symptoms of depression compared with placebo or non-psychoactive drugs. The protocol was registered in the International Prospective Register of Systematic Reviews (see supplementary Appendix A). The study overall did not deviate from the pre-registered protocol; one clarification was made to highlight that any non-psychedelic comparator was eligible for inclusion, including placebo, niacin, micro doses of psychedelics, and drugs that are considered the standard of care in depression (eg, SSRIs).
Inclusion and exclusion criteria
Double blind and open label randomised trials with a crossover or parallel design were eligible for inclusion. We considered only studies in humans and with a control condition, which could include any type of non -active comparator, such as placebo, niacin, or micro doses of psychedelics.
Eligible studies were those that included adults (≥18 years) with clinically significant symptoms of depression, evaluated using a clinically validated tool for depression and mood disorder outcomes. Such tools included the Beck depression inventory, Hamilton depression rating scale, Montgomery-Åsberg depression rating scale, profile of mood states, and quick inventory of depressive symptomatology. Studies of participants with symptoms of depression and comorbidities (eg, cancer) were also eligible. We excluded studies of healthy participants (without depressive symptomatology).
Eligible studies investigated the effect of psilocybin as a standalone treatment on symptoms of depression. Studies with an active psilocybin condition that involved micro dosing (ie, psilocybin <100 μg/kg, according to the commonly accepted convention 22 35 ) were excluded. We included studies with directive psychotherapy if the psychotherapeutic component was present in both the experimental and the control conditions, so that the effects of psilocybin could be distinguished from those of psychotherapy. Studies involving group therapy were also excluded. Any non-psychedelic comparator was eligible for inclusion, including placebo, niacin, and micro doses of psychedelics.
Changes in symptoms, measured by validated clinician rated or self-report scales, such as the Beck depression inventory, Hamilton depression rating scale, Montgomery-Åsberg depression rating scale, profile of mood states, and quick inventory of depressive symptomatology were considered. We excluded outcomes that were measured less than three hours after psilocybin had been administered because any reported changes could be attributed to the transient cognitive and affective effects of the substance being administered. Aside from this, outcomes were included irrespective of the time point at which measurements were taken.
Search strategy
We searched major electronic databases and trial registries of psychological and medical research, with no limits on the publication date. Databases were the Cochrane Central Register of Controlled Trials via the Cochrane Library, Embase via Ovid, Medline via Ovid, Science Citation Index and Conference Proceedings Citation Index-Science via Web of Science, and PsycInfo via Ovid. A search through multiple databases was necessary because each database includes unique journals. Supplementary Appendix B shows the search syntax used for the Cochrane Central Register of Controlled Trials, which was slightly modified to comply with the syntactic rules of the other databases.
Unpublished and grey literature were sought through registries of past and ongoing trials, databases of conference proceedings, government reports, theses, dissertations, and grant registries (eg, ClinicalTrials.gov, WHO International Clinical Trials Registry Platform, ProQuest Dissertations and Theses Global, and PsycEXTRA). The references and bibliographies of eligible studies were checked for relevant publications. The original search was done in January 2023 and updated search was performed on 10 August 2023.
Data collection, extraction, and management
The results of the literature search were imported to the Endnote X9 reference management software, and the references were imported to the Covidence platform after removal of duplicates. Two reviewers (AM and DT) independently screened the title and abstract of each reference and then screened the full text of potentially eligible references. Any disagreements about eligibility were resolved through discussion. If information was insufficient to determine eligibility, the study’s authors were contacted. The reviewers were not blinded to the studies’ authors, institutions, or journal of publication.
The PRISMA (Preferred Reporting Items for Systematic Reviews and Meta-Analyses) flow diagram shows the study selection process and reasons for excluding studies that were considered eligible for full text screening. 36
Critical appraisal of individual studies and of aggregated evidence
The methodological quality of eligible studies was assessed using the Cochrane Risk of Bias 2 tool (RoB 2) for assessing risk of bias in randomised trials. 37 In addition to the criteria specified by RoB 2, we considered the potential impact of industry funding and conflicts of interest. The overall methodological quality of the aggregated evidence was evaluated using GRADE (Grading of Recommendations, Assessment, Development and Evaluation). 38
If we found evidence of heterogeneity among the trials, then small study biases, such as publication bias, were assessed using a funnel plot and asymmetry tests (eg, Egger’s test). 39
We used a template for data extraction (see supplementary Appendix C) and summarised the extracted data in tabular form, outlining personal characteristics (age, sex, previous use of psychedelics), methodology (study design, dosage), and outcome related characteristics (mean change from baseline score on a depression questionnaire, response rates, and remission rates) of the included studies. Response conventionally refers to a 50% decrease in symptom severity based on scores on a depression rating scale, whereas remission scores are specific to a questionnaire (eg, score of ≤5 on the quick inventory of depressive symptomatology, score of ≤10 on the Montgomery-Åsberg depression rating scale, 50% or greater reduction in symptoms, score of ≤7 on the Hamilton depression rating scale, or score of ≤12 on the Beck depression inventory). Across depression scales, higher scores signify more severe symptoms of depression.
Continuous data synthesis
From each study we extracted the baseline and post-intervention means and standard deviations (SDs) of the scores between comparison groups for the depression questionnaires and calculated the mean differences and SDs of change. If means and SDs were not available for the included studies, we extracted the values from available graphs and charts using the Web Plot Digitizer application ( https://automeris.io/WebPlotDigitizer/ ). If it was not possible to calculate SDs from the graphs or charts, we generated values by converting standard errors (SEs) or confidence intervals (CIs), depending on availability, using formulas in the Cochrane Handbook (section 7.7.3.2). 40
Standardised mean differences were calculated for each study. We chose these rather than weighted mean differences because, although all the studies measured depression as the primary outcome, they did so with different questionnaires that score depression based on slightly different items. 41 If we had used weighted mean differences, any variability among studies would be assumed to reflect actual methodological or population differences and not differences in how the outcome was measured, which could be misleading. 40
The Hedges’ g effect size estimate was used because it tends to produce less biased results for studies with smaller samples (<20 participants) and when sample sizes differ substantially between studies, in contrast with Cohen’s d. 42 According to the Cochrane Handbook, the Hedges’ g effect size measure is synonymous with the standardised mean difference, 40 and the terms may be used interchangeably. Thus, a Hedges’ g of 0.2, 0.5, 0.8, or 1.2 corresponds to a small, medium, large, or very large effect, respectively. 40
Owing to variation in the participants’ personal characteristics, psilocybin dosage, type of depression investigated (primary or secondary), and type of comparators, we used a random effects model with a Hartung-Knapp-Sidik-Jonkman modification. 43 This model also allowed for heterogeneity and within study variability to be incorporated into the weighting of the results of the included studies. 44 Lastly, this model could help to generalise the findings beyond the studies and patient populations included, making the meta-analysis more clinically useful. 45 We chose the Hartung-Knapp-Sidik-Jonkman adjustment in favour of more widely used random effects models (eg, DerSimonian and Laird) because it allows for better control of type 1 errors, especially for studies with smaller samples, and provides a better estimation of between study variance by accounting for small sample sizes. 46 47
For studies in which multiple treatment groups were compared with a single placebo group, we split the placebo group to avoid multiplicity. 48 Similarly, if studies included multiple primary outcomes (eg, change in depression at three weeks and at six weeks), we split the treatment groups to account for overlapping participants. 40
Prediction intervals (PIs) were calculated and reported to show the expected effect range of a similar future study, in a different setting. In a random effects model, within study measures of variability, such as CIs, can only show the range in which the average effect size could lie, but they are not informative about the range of potential treatment effects given the heterogeneity between studies. 49 Thus, we used PIs as an indication of variation between studies.
Heterogeneity and sensitivity analysis
Statistical heterogeneity was tested using the χ 2 test (significance level P<0.1) and I 2 statistic, and heterogeneity among included studies was evaluated visually and displayed graphically using a forest plot. If substantial or considerable heterogeneity was found (I 2 ≥50% or P<0.1), 50 we considered the study design and characteristics of the included studies. Sources of heterogeneity were explored by subgroup analysis, and the potential effects on the results are discussed.
Planned sensitivity analyses to assess the effect of unpublished studies and studies at high risk of bias were not done because all included studies had been published and none were assessed as high risk of bias. Exclusion sensitivity plots were used to display graphically the impact of individual studies and to determine which studies had a particularly large influence on the results of the meta-analysis. All sensitivity analyses were carried out with Stata 16 software.
Subgroup analysis
To reduce the risk of errors caused by multiplicity and to avoid data fishing, we planned subgroup analyses a priori and limited to: (1) patient characteristics, including age and sex; (2) comorbidities, such as a serious physical condition (previous research indicates that the effects of psilocybin may be less strong for such participants, compared with participants with no comorbidities) 33 ; (3) number of doses and amount of psilocybin administered, because some previous meta-analyses found that a higher number of doses and a higher dose of psilocybin both predicted a greater reduction in symptoms of depression, 34 whereas others reported the opposite 33 ; (4) psilocybin administered alongside psychotherapeutic guidance or as a standalone treatment; (5) severity of depressive symptoms (clinical v subclinical symptomatology); (6) clinician versus patient rated scales; and (7) high versus low quality studies, as determined by RoB 2 assessment scores.
Metaregression
Given that enough studies were identified (≥10 distinct observations according to the Cochrane Handbook’s suggestion 40 ), we performed metaregression to investigate whether covariates, or potential effect modifiers, explained any of the statistical heterogeneity. The metaregression analysis was carried out using Stata 16 software.
Random effects metaregression analyses were used to determine whether continuous variables such as participants’ age, percentage of female participants, and percentage of participants who had previously used psychedelics modified the effect estimate, all of which have been implicated in differentially affecting the efficacy of psychedelics in modifying mood. 51 We chose this approach in favour of converting these continuous variables into categorical variables and conducting subgroup analyses for two primary reasons; firstly, the loss of any data and subsequent loss of statistical power would increase the risk of spurious significant associations, 51 and, secondly, no cut-offs have been agreed for these factors in literature on psychedelic interventions for mood disorders, 52 making any such divisions arbitrary and difficult to reconcile with the findings of other studies. The analyses were based on within study averages, in the absence of individual data points for each participant, with the potential for the results to be affected by aggregate bias, compromising their validity and generalisability. 53 Furthermore, a group level analysis may not be able to detect distinct interactions between the effect modifiers and participant subgroups, resulting in ecological bias. 54 As a result, this analysis should be considered exploratory.
Sensitivity analysis
A sensitivity analysis was performed to determine if choice of analysis method affected the primary findings of meta-analysis. Specifically, we reanalysed the data on change in depression score using a random effects Dersimonian and Laird model without the Hartung-Knapp-Sidik-Jonkman modification and compared the results with those of the originally used model. This comparison is particularly important in the presence of substantial heterogeneity and the potential of small study effects to influence the intervention effect estimate. 55
Patient and public involvement
Research on novel depression treatments is of great interest to both patients and the public. Although patients and members of the public were not directly involved in the planning or writing of this manuscript owing to a lack of available funding for recruitment and researcher training, patients and members of the public read the manuscript after submission.
Figure 1 presents the flow of studies through the systematic review and meta-analysis. 56 A total of 4884 titles were retrieved from the five databases of published literature, and a further 368 titles were identified from the databases of unpublished and international literature in February 2023. After the removal of duplicate records, we screened the abstracts and titles of 875 reports. A further 12 studies were added after handsearching of reference lists and conference proceedings and abstracts. Overall, nine studies totalling 436 participants were eligible. The average age of the participants ranged from 36-60 years. During an updated search on 10 August 2023, no further studies were identified.

Flow of studies in systematic review and meta-analysis
After screening of the title and abstract, 61 titles remained for full text review. Native speakers helped to translate papers in languages other than English. The most common reasons for exclusion were the inclusion of healthy volunteers, absence of control groups, and use of a survey based design rather than an experimental design. After full text screening, nine studies were eligible for inclusion, and 15 clinical trials prospectively registered or underway as of August 2023 were noted for potential future inclusion in an update of this review (see supplementary Appendix D).
We sent requests for further information to the authors of studies by Griffiths et al, 57 Barrett, 58 and Benville et al, 59 because these studies appeared to meet the inclusion criteria but were only provided as summary abstracts online. A potentially eligible poster presentation from the 58th annual meeting of the American College of Neuropsychopharmacology was identified but the lead author (Griffiths) clarified that all information from the presentation was included in the studies by Davis et al 13 and Gukasyan et al 60 ; both of which we had already deemed ineligible.
Barrett 58 reported the effects of psilocybin on the cognitive flexibility and verbal reasoning of a subset of patients with major depressive disorder from Griffith et al’s trial, 61 compared with a waitlist group, but when contacted, Barrett explained that the results were published in the study by Doss et al, 62 which we had already screened and judged ineligible (see supplementary Appendix E). Benville et al’s study 59 presented a follow-up of Ross et al’s study 17 on a subset of patients with cancer and high suicidal ideation and desire for hastened death at baseline. Measures of antidepressant effects of psilocybin treatment compared with niacin were taken before and after treatment crossover, but detailed results are not reported. Table 1 describes the characteristics of the included studies and table 2 lists the main findings of the studies.
Characteristics of included studies
- View inline
Main findings of included studies
Side effects and adverse events
Side effects reported in the included studies were minor and transient (eg, short term increases in blood pressure, headache, and anxiety), and none were coded as serious. Cahart-Harris et al noted one instance of abnormal dreams and insomnia. 63 This side effect profile is consistent with findings from other meta-analyses. 30 68 Owing to the different scales and methods used to catalogue side effects and adverse events across trials, it was not possible to combine these data quantitatively (see supplementary Appendix F).
Risk of bias
The Cochrane RoB 2 tools were used to evaluate the included studies ( table 3 ). RoB 2 for randomised trials was used for the five reports of parallel randomised trials (Carhart-Harris et al 63 and its secondary analysis Barba et al, 64 Goodwin et al 18 and its secondary analysis Goodwin et al, 65 and von Rotz et al 66 ) and RoB 2 for crossover trials was used for the four reports of crossover randomised trials (Griffiths et al, 14 Grob et al, 15 and Ross et al 17 and its follow-up Ross et al 67 ). Supplementary Appendix G provides a detailed explanation of the assessment of the included studies.
Summary risk of bias assessment of included studies, based on domains in Cochrane Risk of Bias 2 tool
Quality of included studies
Confidence in the quality of the evidence for the meta-analysis was assessed using GRADE, 38 through the GRADEpro GDT software program. Figure 2 shows the results of this assessment, along with our summary of findings.

GRADE assessment outputs for outcomes investigated in meta-analysis (change in depression scores and response and remission rates). The risk in the intervention group (and its 95% CI) is based on the assumed risk in the comparison group and the relative effect of the intervention (and its 95% CI). BDI=Beck depression inventory; CI=confidence interval; GRADE=Grading of Recommendations, Assessment, Development and Evaluation; HADS-D=hospital anxiety and depression scale; HAM-D=Hamilton depression rating scale; MADRS=Montgomery-Åsberg depression rating scale; QIDS=quick inventory of depressive symptomatology; RCT=randomised controlled trial; SD=standard deviation
Meta-analyses
Continuous data, change in depression scores —Using a Hartung-Knapp-Sidik-Jonkman modified random effects meta-analysis, change in depression scores was significantly greater after treatment with psilocybin compared with active placebo. The overall Hedges’ g (1.64, 95% CI 0.55 to 2.73) indicated a large effect size favouring psilocybin ( fig 3 ). PIs were, however, wide and crossed the line of no difference (95% CI −1.72 to 5.03), indicating that there could be settings or populations in which psilocybin intervention would be less efficacious.

Forest plot for overall change in depression scores from before to after treatment. CI=confidence interval; DL=DerSimonian and Laird; HKSJ=Hartung-Knapp-Sidik-Jonkman
Exploring publication bias in continuous data —We used Egger’s test and a funnel plot to examine the possibility of small study biases, such as publication bias. Statistical significance of Egger’s test for small study effects, along with the asymmetry in the funnel plot ( fig 4 ), indicates the presence of bias against smaller studies with non-significant results, suggesting that the pooled intervention effect estimate is likely to be overestimated. 69 An alternative explanation, however, is that smaller studies conducted at the early stages of a new psychotherapeutic intervention tend to include more high risk or responsive participants, and psychotherapeutic interventions tend to be delivered more effectively in smaller trials; both of these factors can exaggerate treatment effects, resulting in funnel plot asymmetry. 70 Also, because of the relatively small number of included studies and the considerable heterogeneity observed, test power may be insufficient to distinguish real asymmetry from chance. 71 Thus, this analysis should be considered exploratory.

Funnel plot assessing publication bias among studies measuring change in depression scores from before to after treatment. CI=confidence interval; θ IV =estimated effect size under inverse variance random effects model
Dichotomous data
We extracted response and remission rates for each group when reported directly, or imputed information when presented graphically. Two studies did not measure response or remission and thus did not contribute data for this part of the analysis. 15 18 The random effects model with a Hartung-Knapp-Sidik-Jonkman modification was used to allow for heterogeneity to be incorporated into the weighting of the included studies’ results, and to provide a better estimation of between study variance accounting for small sample sizes.
Response rate —Overall, the likelihood of psilocybin intervention leading to treatment response was about two times greater (risk ratio 2.02, 95% CI 1.33 to 3.07) than with placebo. Despite the use of different scales to measure response, the heterogeneity between studies was not significant (I 2 =25.7%, P=0.23). PIs were, however, wide and crossed the line of no difference (−0.94 to 3.88), indicating that there could be settings or populations in which psilocybin intervention would be less efficacious.
Remission rate —Overall, the likelihood of psilocybin intervention leading to remission of depression was nearly three times greater than with placebo (risk ratio 2.71, 95% CI 1.75 to 4.20). Despite the use of different scales to measure response, no statistical heterogeneity was found between studies (I 2 =0.0%, P=0.53). PIs were, however, wide and crossed the line of no difference (0.87 to 2.32), indicating that there could be settings or populations in which psilocybin intervention would be less efficacious.
Exploring publication bias in response and remission rates data —We used Egger’s test and a funnel plot to examine whether response and remission estimates were affected by small study biases. The result for Egger’s test was non-significant (P>0.05) for both response and remission estimates, and no substantial asymmetry was observed in the funnel plots, providing no indication for the presence of bias against smaller studies with non-significant results.
Heterogeneity: subgroup analyses and metaregression
Heterogeneity was considerable across studies exploring changes in depression scores (I 2 =89.7%, P<0.005), triggering subgroup analyses to explore contributory factors. Table 4 and table 5 present the results of the heterogeneity analyses (subgroup analyses and metaregression, respectively). Also see supplementary Appendix H for a more detailed description and graphical representation of these results.
Subgroup analyses to explore potential causes of heterogeneity among included studies
Metaregression analyses to explore potential causes of heterogeneity among included studies
Cumulative meta-analyses
We used cumulative meta-analyses to investigate how the overall estimates of the outcomes of interest changed as each study was added in chronological order 72 ; change in depression scores and likelihood of treatment response both increased as the percentage of participants with past use of psychedelics increased across studies, as expected based on the metaregression analysis (see supplementary Appendix I). No other significant time related patterns were found.
We reanalysed the data for change in depression scores using a random effects Dersimonian and Laird model without the Hartung-Knapp-Sidik-Jonkman modification and compared the results with those of the original model. All comparisons found to be significant using the Dersimonian and Laird model with the Hartung-Knapp-Sidik-Jonkman adjustment were also significant without the Hartung-Knapp-Sidik-Jonkman adjustment, and confidence intervals were only slightly narrower. Thus, small study effects do not appear to have played a major role in the treatment effect estimate.
Additionally, to estimate the accuracy and robustness of the estimated treatment effect, we excluded studies from the meta-analysis one by one; no important differences in the treatment effect, significance, and heterogeneity levels were observed after the exclusion of any study (see supplementary Appendix J).
In our meta-analysis we found that psilocybin use showed a significant benefit on change in depression scores compared with placebo. This is consistent with other recent meta-analyses and trials of psilocybin as a standalone treatment for depression 73 74 or in combination with psychological support. 24 25 29 30 31 32 68 75 This review adds to those finding by exploring the considerable heterogeneity across the studies, with subsequent subgroup analyses showing that the type of depression (primary or secondary) and the depression scale used (Montgomery-Åsberg depression rating scale, quick inventory of depressive symptomatology, or Beck depression inventory) had a significant differential effect on the outcome. High between study heterogeneity has been identified by some other meta-analyses of psilocybin (eg, Goldberg et al 29 ), with a higher treatment effect in studies with patients with comorbid life threatening conditions compared with patients with primary depression. 22 Although possible explanations, including personal factors (eg, patients with life threatening conditions being older) or depression related factors (eg, secondary depression being more severe than primary depression) could be considered, these hypotheses are not supported by baseline data (ie, patients with secondary depression do not differ substantially in age or symptom severity from patients with primary depression). The differential effects from assessment scales used have not been examined in other meta-analyses of psilocybin, but this review’s finding that studies using the Beck depression inventory showed a higher treatment effect than those using the Montgomery-Åsberg depression rating scale and quick inventory of depressive symptomatology is consistent with studies in the psychological literature that have shown larger treatment effects when self-report scales are used (eg, Beck depression inventory). 76 77 This finding may be because clinicians tend to overestimate the severity of depression symptoms at baseline assessments, leading to less pronounced differences between before and after treatment identified in clinician assessed scales (eg, Montgomery-Åsberg depression rating scale, quick inventory of depressive symptomatology). 78
Metaregression analyses further showed that a higher average age and a higher percentage of participants with past use of psychedelics both correlated with a greater improvement in depression scores with psilocybin use and explained a substantial amount of between study variability. However, the cumulative meta-analysis showed that the effects of age might be largely an artefact of the inclusion of one specific study, and alternative explanations are worth considering. For instance, Studerus et al 79 identified participants’ age as the only personal variable significantly associated with psilocybin response, with older participants reporting a higher “blissful state” experience. This might be because of older people’s increased experience in managing negative emotions and the decrease in 5-hydroxytryptamine type 2A receptor density associated with older age. 80 Furthermore, Rootman et al 81 reported that the cognitive performance of older participants (>55 years) improved significantly more than that of younger participants after micro dosing with psilocybin. Therefore, the higher decrease in depressive symptoms associated with older age could be attributed to a decrease in cognitive difficulties experienced by older participants.
Interestingly, a clear pattern emerged for past use of psychedelics—the higher the proportion of study participants who had used psychedelics in the past, the higher the post-psilocybin treatment effect observed. Past use of psychedelics has been proposed to create an expectancy bias among participants and amplify the positive effects of psilocybin 82 83 84 ; however, this important finding has not been examined in other meta-analyses and may highlight the role of expectancy in psilocybin research.
Limitations of this study
Generalisability of the findings of this meta-analysis was limited by the lack of racial and ethnic diversity in the included studies—more than 90% of participants were white across all included trials, resulting in a homogeneous sample that is not representative of the general population. Moreover, it was not possible to distinguish between subgroups of participants who had never used psilocybin and those who had taken psilocybin more than a year before the start of the trial, as these data were not provided in the included studies. Such a distinction would be important, as the effects of psilocybin on mood may wane within a year after being administered. 21 85 Also, how psychological support was conceptualised was inconsistent within studies of psilocybin interventions; many studies failed to clearly describe the type of psychological support participants received, and others used methods ranging from directive guidance throughout the treatment session to passive encouragement or reassurance (eg, Griffiths et al, 14 Carhart-Harris et al 63 ). The included studies also did not gather evidence on participants’ previous experiences with treatment approaches, which could influence their response to the trials’ intervention. Thus, differences between participant subgroups related to past use of psilocybin or psychotherapy may be substantial and could help interpret this study’s findings more accurately. Lastly, the use of graphical extraction software to estimate the findings of studies where exact numerical data were not available (eg, Goodwin et al, 18 Grob et al 15 ), may have affected the robustness of the analyses.
A common limitation in studies of psilocybin is the likelihood of expectancy effects augmenting the treatment effect observed. Although some studies used low dose psychedelics as comparators to deal with this problem (eg, Carhart-Harris et al, 63 Goodwin et al, 18 Griffiths et al 14 ) or used a niacin placebo that can induce effects similar to those of psilocybin (eg, Grob et al, 15 Ross et al 17 ), the extent to which these methods were effective in blinding participants is not known. Other studies have, however, reported that participants can accurately identify the study groups to which they had been assigned 70-85% of the time, 84 86 indicating a high likelihood of insufficient blinding. This is especially likely for studies in which a high proportion of participants had previously used psilocybin and other hallucinogens, making the identification of the drug’s acute effects easier (eg, Griffiths et al, 14 Grob et al, 15 Ross et al 17 ). Patients also have expectations related to the outcome of their treatment, expecting psilocybin to improve their symptoms of depression, and these positive expectancies are strong predictors of actual treatment effects. 87 88 Importantly, the effect of outcome expectations on treatment effect is particularly strong when patient reported measures are used as primary outcomes, 89 which was the case in several of the included studies (eg, Griffiths et al, 14 Grob et al, 15 Ross et al 17 ). Unfortunately, none of the included studies recorded expectations before treatment, so it is not possible to determine the extent to which this factor affected the findings.
Implications for clinical practice
Although this review’s findings are encouraging for psilocybin’s potential as an effective antidepressant, a few areas about its applicability in clinical practice remain unexplored. Firstly, it is unclear whether the protocols for psilocybin interventions in clinical trials can be reliably and safely implemented in clinical practice. In clinical trials, patients receive psilocybin in a non-traditional medical setting, such as a specially designed living room, while they may be listening to curated calming music and are isolated from most external stimuli by wearing eyeshades and external noise-cancelling earphones. A trained therapist closely supervises these sessions, and the patient usually receives one or more preparatory sessions before the treatment commences. Standardising an intervention setting with so many variables is unlikely to be achievable in routine practice, and consensus is considerably lacking on the psychotherapeutic training and accreditations needed for a therapist to deliver such treatment. 90 The combination of these elements makes this a relatively complex and expensive intervention, which could make it challenging to gain approval from regulatory agencies and to gain reimbursement from insurance companies and others. Within publicly funded healthcare systems, the high cost of treatment may make psilocybin treatment inaccessible. The high cost associated with the intervention also increases the risk that unregulated clinics may attempt to cut costs by making alterations to the protocol and the therapeutic process, 91 92 which could have detrimental effects for patients. 92 93 94 Thus, avoiding the conflation of medical and commercial interests is a primary concern that needs to be dealt with before psilocybin enters mainstream practice.
Implications for future research
More large scale randomised trials with long follow-up are needed to fully understand psilocybin’s treatment potential, and future studies should aim to recruit a more diverse population. Another factor that would make clinical trials more representative of routine practice would be to recruit patients who are currently using or have used commonly prescribed serotonergic antidepressants. Clinical trials tend to exclude such participants because many antidepressants that act on the serotonin system modulate the 5-hydroxytryptamine type 2A receptor that psilocybin primarily acts upon, with prolonged use of tricyclic antidepressants associated with more intense psychedelic experiences and use of monoamine oxidase inhibitors or SSRIs inducing weaker responses to psychedelics. 95 96 97 Investigating psilocybin in such patients would, however, provide valuable insight on how psilocybin interacts with commonly prescribed drugs for depression and would help inform clinical practice.
Minimising the influence of expectancy effects is another core problem for future studies. One strategy would be to include expectancy measures and explore the level of expectancy as a covariate in statistical analysis. Researchers should also test the effectiveness of condition masking. Another proposed solution would be to adopt a 2×2 balanced placebo design, where both the drug (psilocybin or placebo) and the instructions given to participants (told they have received psilocybin or told they have received placebo) are crossed. 98 Alternatively, clinical trials could adopt a three arm design that includes both an inactive placebo (eg, saline) and active placebo (eg, niacin, lower psylocibin dose), 98 allowing for the effects of psilocybin to be separated from those of the placebo.
Overall, future studies should explore psilocybin’s exact mechanism of treatment effectiveness and outline how its physiological effects, mystical experiences, dosage, treatment setting, psychological support, and relationship with the therapist all interact to produce a synergistic antidepressant effect. Although this may be difficult to achieve using an explanatory randomised trial design, pragmatic clinical trial designs may be better suited to psilocybin research, as their primary objective is to achieve high external validity and generalisability. Such studies may include multiple alternative treatments rather than simply an active and placebo treatment comparison (eg, psilocybin v SSRI v serotonin-noradrenaline reuptake inhibitor), and participants would be recruited from broader clinical populations. 99 100 Although such studies are usually conducted after a drug’s launch, 100 earlier use of such designs could help assess the clinical effectiveness of psilocybin more robustly and broaden patient access to a novel type of antidepressant treatment.
Conclusions
This review’s findings on psilocybin’s efficacy in reducing symptoms of depression are encouraging for its use in clinical practice as a drug intervention for patients with primary or secondary depression, particularly when combined with psychological support and administered in a supervised clinical environment. However, the highly standardised treatment setting, high cost, and lack of regulatory guidelines and legal safeguards associated with psilocybin treatment need to be dealt with before it can be established in clinical practice.
What is already known on this topic
Recent research on treatments for depression has focused on psychedelic agents that could have strong antidepressant effects without the drawbacks of classic antidepressants; psilocybin being one such substance
Over the past decade, several clinical trials, meta-analyses, and systematic reviews have investigated the use of psilocybin for symptoms of depression, and most have found that psilocybin can have antidepressant effects
Studies published to date have not investigated factors that may moderate psilocybin’s effects, including type of depression, past use of psychedelics, dosage, outcome measures, and publication biases
What this study adds
This review showed a significantly greater efficacy of psilocybin among patients with secondary depression, patients with past use of psychedelics, older patients, and studies using self-report measures for symptoms of depression
Efficacy did not appear to be homogeneous across patient types—for example, those with depression and a life threatening illness appeared to benefit more from treatment
Further research is needed to clarify the factors that maximise psilocybin’s treatment potential for symptoms of depression
Ethics statements
Ethical approval.
This study was approved by the ethics committee of the University of Oxford Nuffield Department of Medicine, which waived the need for ethical approval and the need to obtain consent for the collection, analysis, and publication of the retrospectively obtained anonymised data for this non-interventional study.
Data availability statement
The relevant aggregated data and statistical code will be made available on reasonable request to the corresponding author.
Acknowledgments
We thank DT who acted as an independent secondary reviewer during the study selection and data review process.
Contributors: AMM contributed to the design and implementation of the research, analysis of the results, and writing of the manuscript. MC was involved in planning and supervising the work and contributed to the writing of the manuscript. AMM and MC are the guarantors. The corresponding author attests that all listed authors meet authorship criteria and that no others meeting the criteria have been omitted.
Funding: None received.
Competing interests: All authors have completed the ICMJE uniform disclosure form at https://www.icmje.org/disclosure-of-interest/ and declare: no support from any organisation for the submitted work; AMM is employed by IDEA Pharma, which does consultancy work for pharmaceutical companies developing drugs for physical and mental health conditions; MC was the supervisor for AMM’s University of Oxford MSc dissertation, which forms the basis for this paper; no other relationships or activities that could appear to have influenced the submitted work.
Transparency: The corresponding author (AMM) affirms that the manuscript is an honest, accurate, and transparent account of the study being reported; that no important aspects of the study have been omitted; and that any discrepancies from the study as registered have been explained.
Dissemination to participants and related patient and public communities: To disseminate our findings and increase the impact of our research, we plan on writing several social media posts and blog posts outlining the main conclusions of our paper. These will include blog posts on the websites of the University of Oxford’s Department of Primary Care Health Sciences and Department for Continuing Education, as well as print publications, which are likely to reach a wider audience. Furthermore, we plan to present our findings and discuss them with the public in local mental health related events and conferences, which are routinely attended by patient groups and advocacy organisations.
Provenance and peer review: Not commissioned; externally peer reviewed.
This is an Open Access article distributed in accordance with the Creative Commons Attribution Non Commercial (CC BY-NC 4.0) license, which permits others to distribute, remix, adapt, build upon this work non-commercially, and license their derivative works on different terms, provided the original work is properly cited and the use is non-commercial. See: http://creativecommons.org/licenses/by-nc/4.0/ .
- ↵ World Health Organization. Depressive Disorder (Depression); 2023. https://www.who.int/news-room/fact-sheets/detail/depression .
- GBD 2017 Disease and Injury Incidence and Prevalence Collaborators
- Cipriani A ,
- Furukawa TA ,
- Salanti G ,
- Trivedi MH ,
- Wisniewski SR ,
- Mitchell AJ
- Bockting CL ,
- Hollon SD ,
- Jarrett RB ,
- Nierenberg AA ,
- Petersen TJ ,
- Páleníček T ,
- Carbonaro TM ,
- Bradstreet MP ,
- Barrett FS ,
- Carhart-Harris RL ,
- Bolstridge M ,
- Griffiths RR ,
- Johnson MW ,
- Carducci MA ,
- Danforth AL ,
- Chopra GS ,
- Kraehenmann R ,
- Preller KH ,
- Scheidegger M ,
- Goodwin GM ,
- Aaronson ST ,
- Alvarez O ,
- Bogenschutz MP ,
- Podrebarac SK ,
- Roseman L ,
- Galvão-Coelho NL ,
- Gonzalez M ,
- Dos Santos RG ,
- Osório FL ,
- Crippa JA ,
- Zuardi AW ,
- Cleare AJ ,
- Martelli C ,
- Benyamina A
- Vollenweider FX ,
- Demetriou L ,
- Carhart-Harris RL
- Timmermann C ,
- Giribaldi B ,
- Goldberg SB ,
- Nicholas CR ,
- Raison CL ,
- Irizarry R ,
- Winczura A ,
- Dimassi O ,
- Dhillon N ,
- Griffiths RR
- Castro Santos H ,
- Gama Marques J
- Moreno FA ,
- Wiegand CB ,
- Taitano EK ,
- Liberati A ,
- Tetzlaff J ,
- Altman DG ,
- PRISMA Group
- Sterne JAC ,
- Savović J ,
- Guyatt GH ,
- Schünemann HJ ,
- Tugwell P ,
- Knottnerus A
- Sterne JA ,
- Sutton AJ ,
- Ioannidis JP ,
- Higgins JPT ,
- Chandler J ,
- Borenstein M ,
- Hedges LV ,
- Higgins JP ,
- Rothstein HR
- DerSimonian R ,
- ↵ Borenstein M, Hedges L, Rothstein H. Meta-analysis: Fixed effect vs. random effects. Meta-analysis. com. 2007;1-62.
- IntHout J ,
- Rovers MM ,
- Gøtzsche PC
- Spineli LM ,
- ↵ Higgins JP, Green S. Identifying and measuring heterogeneity. Cochrane handbook for systematic reviews of interventions. 2011;5(0).
- Austin PC ,
- O’Donnell KC ,
- Mennenga SE ,
- Bogenschutz MP
- Sander SD ,
- Berlin JA ,
- Santanna J ,
- Schmid CH ,
- Szczech LA ,
- Feldman HI ,
- Anti-Lymphocyte Antibody Induction Therapy Study Group
- ↵ Iyengar S, Greenhouse J. Sensitivity analysis and diagnostics. Handbook of research synthesis and meta-analysis. Russell Sage Foundation, 2009:417-33.
- McKenzie JE ,
- Bossuyt PM ,
- ↵ Griffiths R, Barrett F, Johnson M, Mary C, Patrick F, Alan D. Psilocybin-Assisted Treatment of Major Depressive Disorder: Results From a Randomized Trial. Proceedings of the ACNP 58th Annual Meeting: Poster Session II. In Neuropsychopharmacology. 2019;44:230-384.
- ↵ Barrett F. ACNP 58th Annual Meeting: Panels, Mini-Panels and Study Groups. [Abstract.] Neuropsychopharmacology 2019;44:1-77. doi: 10.1038/s41386-019-0544-z . OpenUrl CrossRef
- Benville J ,
- Agin-Liebes G ,
- Roberts DE ,
- Gukasyan N ,
- Hurwitz ES ,
- Považan M ,
- Rosenberg MD ,
- Carhart-Harris R ,
- Buehler S ,
- Kettner H ,
- von Rotz R ,
- Schindowski EM ,
- Jungwirth J ,
- Vargas AS ,
- Barroso M ,
- Gallardo E ,
- Isojarvi J ,
- Lefebvre C ,
- Glanville J
- Sukpraprut-Braaten S ,
- Narlesky M ,
- Strayhan RC
- Prouzeau D ,
- Conejero I ,
- Voyvodic PL ,
- Becamel C ,
- Lopez-Castroman J
- Więckiewicz G ,
- Stokłosa I ,
- Gorczyca P ,
- John Mann J ,
- Currier D ,
- Zimmerman M ,
- Friedman M ,
- Boerescu DA ,
- Attiullah N
- Borgherini G ,
- Conforti D ,
- Studerus E ,
- Kometer M ,
- Vollenweider FX
- Pinborg LH ,
- Rootman JM ,
- Kryskow P ,
- Turner EH ,
- Rosenthal R
- Bershad AK ,
- Schepers ST ,
- Bremmer MP ,
- Sepeda ND ,
- Hurwitz E ,
- Horvath AO ,
- Del Re AC ,
- Flückiger C ,
- Rutherford BR ,
- Pearson C ,
- Husain SF ,
- Harris KM ,
- George JR ,
- Michaels TI ,
- Sevelius J ,
- Williams MT
- Collins A ,
- Bonson KR ,
- Buckholtz JW ,
- Yamauchi M ,
- Matsushima T ,
- Coleshill MJ ,
- Colloca L ,
- Zachariae R ,
- Colagiuri B
- Heifets BD ,
- Pratscher SD ,
- Bradley E ,
- Sugarman J ,
- Open access
- Published: 27 April 2024
The feasibility of integrating a home telehealth model for older persons living with hemodialysis
- Wanicha Pungchompoo 1 ,
- Saowaros Parinyachitta 2 ,
- Sirirat Pungchompoo 3 ,
- Warawan Udomkhwamsuk 4 &
- Panadda Suwan 5
BMC Geriatrics volume 24 , Article number: 378 ( 2024 ) Cite this article
332 Accesses
Metrics details
In Thailand, there is a rapidly increasing population of older persons living with hemodialysis (OPLWH) for whom quality of life and clinical outcomes are their main focus. This study aims to assess the feasibility of an integrated home telehealth model on quality of life and laboratory parameters of OPLWH.
In this study, the second phase of a mixed methods exploratory sequential design was conducted using a repeated measures experimental design. Participants met the inclusion criteria, which included being an OPLWH at a single hemodialysis center of one hospital in Chiang Mai province, Thailand, during the experimental period between 1 April and 30 September 2018, and willing to participate in the study. The 54 participants were purposively selected and randomly assigned to receive either an intervention ( n = 24) consisting of health education and health monitoring using a telehealth device (an iPad) and a web application, or usual care ( n = 30). The instruments included a demographic data form, which was analyzed using the chi-square test. The health-related quality of life questionnaire (the 9 - item Thai Health Status Assessment questionnaire) and blood chemistry (BUN, Cr, Hb, Hct, Alb, K, Kt/V, and nPCR) were compared and measured at baseline, and at 3 and 6 months after enrolment using independent t-test and one-way repeated measures ANOVA.
The comparison of quality of life between the two groups at the two points of repeated measurement (after 3 months) showed a statistically significant difference in mental health scores at P < 0.05 . Six months after the intervention, mean scores for health outcomes and patients’ quality of life improved; however, this change did not reach statistical significance.
Conclusions
An integrated home telehealth model implemented by a hemodialysis nurse is a feasible holistic care approach for OPLWH. However, the absence of statistical significance may partly be associated with the clinical characteristics of frailty and risk factors such as increased age, hypertension, diabetes, heart disease, longer dialysis time, and inadequacy of Kt/V. Large-scale multi-centre trials are warranted to fully examine the acceptability of the model. The duration and long-term effects of the telehealth model are also recommended for further investigation.
Patient or public contribution
The development of a home telehealth model was a collaborative process between patients, caregivers, healthcare professionals from the hemodialysis unit, and the research team.
Peer Review reports
What is already known?
In the wake of the COVID-19 pandemic, we are living in a new normal wherein many telehealth systems have been developed—for example, the use of telemedicine to prevent COVID-19.
However, little is known about whether an integrated home telehealth model might be able to promote quality of life and prevent complications for older persons undergoing hemodialysis.
What does this paper contribute to the wider global clinical community?
This paper outlines the development of a home telehealth model specifically focused on holistic care for OPLWH.
The study demonstrates increases in health-related quality of life across both physical and mental scores as a result of receiving the telehealth model. Six months after the experimental intervention, there was no statistical difference between the two groups, but the evidence revealed positive changes in mean scores for quality of life and some laboratory results compared with participants who received usual care.
An integrated holistic home telehealth model delivered by renal nurses and with interprofessional management is recommended as a feasible new clinical care model for the management of OPLWH at home. This is the first model of its kind to be implemented in Thailand.
Introduction
The structure of the world’s population is changing, with an increase in the proportion of older persons. The proportion of the global population aged 65 years or above is projected to rise from 10% in 2022 to 16% in 2050. By 2050, the number of persons aged 65 years or over, worldwide, is projected to be more than twice the number of children under age 5, and about the same as the number of children under age 12 [ 1 ]. The number of Thai older people has increased seven-fold in recent years, and by 2035, is forecast to reach 20 million [ 2 ].
More than 3 million Thais aged 60 and over have been living with chronic illness during the past five years, a statistic that illustrates the increase in chronic illness among the elderly population [ 3 ]. Chronic kidney disease (CKD), a progressive chronic disease common in older persons, is estimated to affect approximately 44% of individuals aged 65 and older [ 4 ] and more than 10% of the population worldwide [ 5 , 6 ]. End-stage renal disease (ESRD) refers to patients with an estimated glomerular filtration rate lower than 15 ml per minute per 1.73 m 2 body surface area [ 7 , 8 ]. ESRD treatment involves renal replacement therapy (RRT), which includes peritoneal dialysis (PD), hemodialysis (HD), and kidney transplantation (KT) [ 9 ]. New cases of Thais living with end-stage renal disease (ESRD) and receiving hemodialysis reached 39,398 during the period from 2017 to 2019 [ 10 ]. Patients receiving hemodialysis include those aged 45 to 64 years (41%), 65 to 74 years (24%), and 75 years and over (20%) [ 10 ]. Older persons undergoing dialysis with ESRD represent a major health and social care burden in the context of aging populations [ 4 ].
Considering this situation, nurse-led telehealth could contribute to managing some of these problems by using information and communication technologies [ 11 ]. Telehealth is a broad term encompassing the use of electronic communication to provide clinical care by replacing face-to-face visits; however, the focus here is on interactive videoconferencing, remote monitoring, mobile phones, web applications, and the internet of things [ 12 ]. Telehealth, integrating holistic and interprofessional management, is a new model of care for chronic disease management. The use of telehealth to facilitate the assessment of symptoms reported by patients themselves may also enhance effective symptom management for persons with end-stage renal disease and could provide a means to overcome identified barriers to home care, thereby improving patients’ care experiences [ 13 , 14 ].
Home telehealth can be used to deliver a range of interventions, from providing information to supporting therapeutic procedures. More timely visits may also result in earlier interventions, preventing complications and reducing unnecessary use of health services while assisting patients to manage their symptoms at home [ 15 ]. Patients who are ill or who find it difficult to travel to attend appointments with physicians can benefit from reduced travel costs; hemodialysis treatment often requires time off work and time away from family, in addition to the financial burden of transportation [ 12 ]. Remote monitoring of patients through information and communication technologies, termed “telecare” or “telehealth”, is increasingly being evidenced as a means of addressing the interest in and demands on health services, alongside more patient-focused care [ 16 ].
The conceptual framework of this study applies the continuum of home telehealth technologies, developed by Hebert, Korabek, and Scott, to examine the feasibility of using a home telehealth program for older persons living with hemodialysis (OPLWH) in Thailand [ 15 ]. These technologies can be categorized following the 3 steps of the home telehealth technologies’ continuum [ 17 ], from 1) patients initiating contact, through 2) automatic monitoring via technology, to 3) healthcare professionals initiating contact (see Fig. 1 ). Categorizing telehealth services may also be based on desirable patient outcomes and the need for services [ 15 ]. The combination of effective telehealth and a continuum of healthcare will increase understanding of health, patient outcomes, and the cost of care. Previous studies have suggested that interprofessional care management or home monitoring could improve intermediate outcomes of CKD patients [ 13 ].
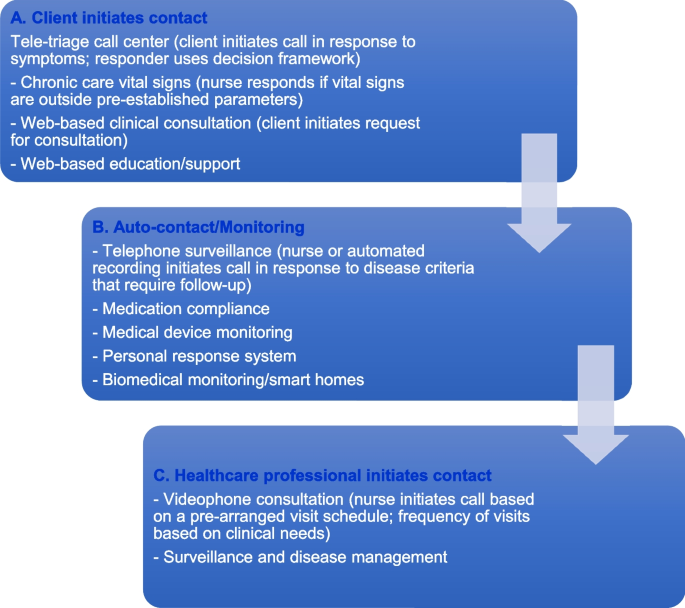
Continuum of home telehealth technologies (adapted from Hebert, Korabek, and Scott, 2006, p. 788) [ 15 ]
The effectiveness of telehealth lies in its potential for improving symptom management and quality of life for patients with long-term chronic conditions, as well as potentially reducing healthcare costs; these benefits have been demonstrated in a number of recent studies [ 9 , 12 , 14 , 18 ]. Telehealth has also been successfully implemented to encourage meaningful engagement with the care system, especially for primary outcomes among both rural and urban patients with chronic kidney disease (estimated glomerular filtration rate (eGFR) < 60 ml/min/1.73m 2 ). Telehealth and the remote monitoring of older patients living with chronic conditions may also provide a means to address geographical barriers to continuum care, thereby improving access to home dialysis, patient quality of life, and outcomes [ 12 ]. Furthermore, the impact of telehealth interventions on clinical outcomes can be reported through lower hospitalization rates and fewer clinical visits. In addition, it has been found that patients who receive telehealth were less likely to miss HD treatment sessions compared with patients who received standard care [ 19 ]. A one-year randomization control trial study, with baseline characteristics of participants including a mean age of 75.1 ± 8.1 (SD) years, found that the telehealth implementation of the Patient Aligned Care Team (PACT) was independently related to reductions in emergency department visits and hospitalization, while improving the ability to control hypertension [ 14 ]. In addition, a longitudinal cohort study confirmed that telehealth videoconferencing for kidney transplant and CKD patients with a mean age of 63.9 years (SD = 12.3 years) provided a feasible intervention for 1 year and sustainable outcomes [ 20 ]. Another qualitative study explored the perceptions of telehealth of older adults with advanced CKD, their partners, and kidney clinicians, demonstrating that telehealth may improve convenience and care partner engagement while still presenting concerns about clinical effectiveness, limitations of virtual physical examination, reduction of the patient-clinician relationship, and patient trust [ 21 ].
During the last decade, the number of OPLWH in Thailand has been increasing steadily. While improving patient outcomes and achieving higher quality care at a lower cost are two goals of adopting telehealth technology, it is necessary to better understand the underlying rationale for how and why home telehealth is effective for improving health outcomes and reducing care costs.
The overarching mixed-methods research project of which this study is a part was conducted to explore and examine the feasibility of using home telehealth for older patients living with end-stage renal disease. We conducted this study as phase 2 of a project entitled Integrating home telehealth into the holistic care: Pilot study in older persons with ESRD to examine whether integrated home telehealth with nurse oversight could be effectively implemented and whether it could improve the quality of life and clinical outcomes in OPLWH when compared with usual care.
A mixed-methods exploratory sequential design was conducted for the project entitled Integrating home telehealth into the holistic care:
Pilot study in older persons with ESRD. Mixed-method research is the combining and integrating of two research types, namely qualitative and quantitative research [ 22 , 23 ]. A description of the study carried out as phase 1 of the project has been published previously [ 24 ]. In this manuscript, we report on phase 2 of the study.
Building on the exploratory results from phase 1, this second phase focuses on gathering quantitative data in order to test or generalize the initial findings. Interaction between the qualitative and quantitative strands occurs as the researcher develops a telehealth model as an intermediate step between the two phases, building on the qualitative results and using them in the subsequent qualitative data collection. The exploratory sequential design in two phases which has been used in this study is set out below, and the scope of the research is presented (see Fig. 2 ).
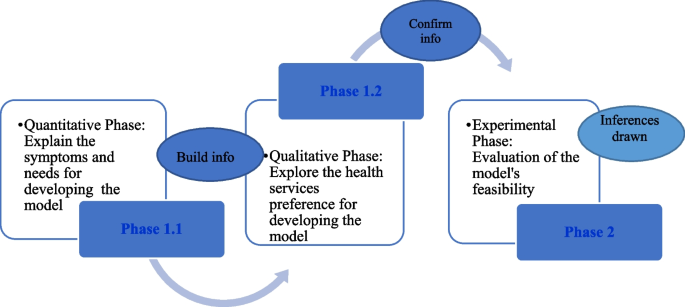
The scope of the study design
Study design and setting
The first phase of the wider mixed-methods study aimed to describe and explore symptom experiences and needs related to the integration of home telehealth into holistic care with nurse oversight for OPLWH. A survey study design with a nonprobability sampling method was employed, and OPLWH were surveyed over a six-month period (between 1 January and 30 June 2017). Participants were recruited from two hemodialysis units at two hospitals in Chiang Mai province. Purposive sampling was used to identify and recruit the participants. The instruments included the VOICES (View of Informal Carers Evaluation of Service-ESRD/Thai patients’ version) questionnaire. Descriptive statistics were used to analyse the data.
Most participants had comorbid conditions, such as hypertension or diabetes mellitus and three main physical symptoms were found (shortness of breath, and pain, and edema) while two psychological symptoms were also present (anxiety and moderate stress). Participants had experienced readmission to hospital at least twice per month. Furthermore, the majority were unable to access home care.
Next, the qualitative section of the study was conducted via in-depth interviews with 20 participants to explore the needs of participants using thematic analysis to analyze the data. The findings revealed the needs of participants related to telehealth including four dimensions: 1) symptom management at home; 2) activity and role management; 3) emotional management; and 4) spiritual support [ 24 ]. The results of the first phase were then utilized to inform and develop an effective home telehealth program for OPLWH.
Participants
In the second phase of the project, a repeated measures experimental design was implemented. Potential participants included 141 OPLWH aged 60 or over who were recruited from a single hemodialysis center at Maharaj Nakorn Chiang Mai hospital in Chiang Mai province, Thailand, during the period between 1 January 2018 and 30 March 2018. Finally, 54 participants met the inclusion criteria and were willing and able to participate in the study, as well as being capable of providing informed consent.
Inclusion and exclusion criteria
Participants were eligible to participate in the study if they were aged 60 years or over, and if they: 1) were being managed for hemodialysis and 2) had been diagnosed with end-stage renal disease stage 5 by a nephrologist. Eligible participants gave their informed consent to participate. Those who were hospitalized with acute illnesses, who had psychological or cognitive disorders, or who had physical limitations which affected their self-care were excluded. Those who were unable to meet the inclusion criteria or were not willing to participate were also excluded.
The sample calculation and randomization procedure
A simple randomization method using a 1:1 ratio was used to assign participants to either the experimental group or the control group, using the random allocation concealment method. Randomization was carried out over the telephone by the researcher who was blinded to patient identity. Although the participants could not be masked in relation to their assignment, the renal nurses caring for them were blind to the participants’ study assignment. Based on repeated measures analysis of variance, an estimated sample size of 60 (30 per group) was considered adequate to demonstrate the effects of the experimental intervention, according to previous studies [ 25 , 26 ]. However, 6 participants in the experimental group were removed because they either withdrew (3), were admitted to the intensive care unit due to co-morbid conditions (2), or died from critical renal failure (1). Thus, 54 participants remained in the study: 24 in the experimental group, and 30 in the control group. G*Power software was used to determine the sample size, and the 8 study factors were analyzed using multivariate statistics. In general, 5–20 samples per factor are preferred. However, in this study, T-test and ANOVA statistics were used. In this determination, the degree of freedom of the factor levels was 2 (3 levels of a changing time period) with two groups (a control group and an experimental group), and the patrial eta square was 0.35 according to the ANOVA results. The sample size which was calculated varied from 24 to 27 samples when we changed the number of covariance from 1 to 12 covariances and used a power of test value of 0.85. Therefore, the results showed that the sample sizes were sufficient for analysis with the ANOVA method [ 27 ]. The attrition rate for the study was 14.58% [ 25 ] (Fig. 3 ).
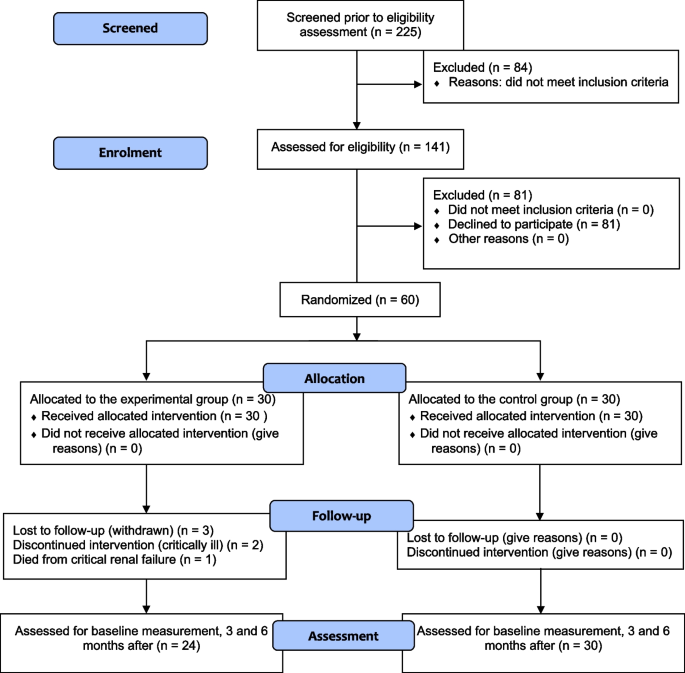
Recruitment flowchart
Intervention measures
Experimental group.
- Home telehealth model
The home telehealth model for OPLWH integrates two concepts. The first focuses on the requirement for health service provision for older persons related to their need for: the control of physical health symptoms, such as breathlessness, pain or edema; mental support and alleviating factors, such as anxiety and stress; spiritual support; clinical care, such as symptom management at home; psychological and spiritual support; regular home visits; an effective referral system; health education; and financial support [ 7 , 24 ]. The second relates to the continuum of home telehealth technologies [ 15 ] (see Fig. 4 ). The framework for our holistic home telehealth model for OPLWH operates across four dimensions: 1) health education related to chronic kidney disease and treatment; 2) the referral system; 3) online home visits; and 4) telephone/live counselling.
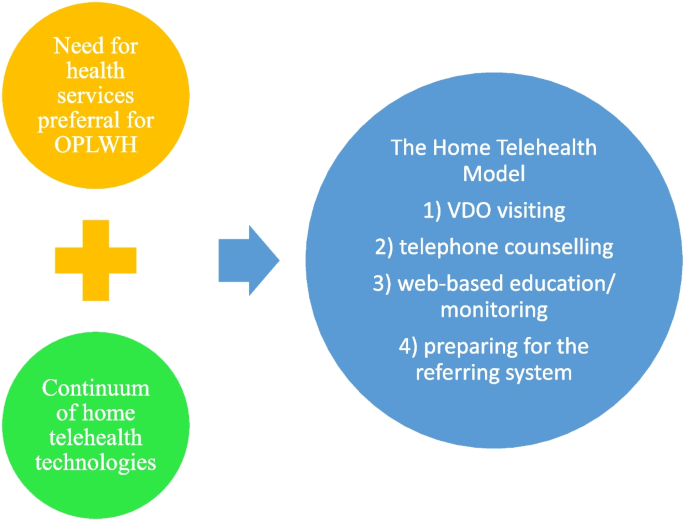
Framework of the holistic home telehealth model for OPLWH
The home telehealth model was designed and developed based on results from the first phase of the study [ 24 ]. The model was focused on video visiting, telephone counselling, web-based education and monitoring, and preparing for the referral system.
Five educational videos and a booklet were developed for participants, containing information and instructions about renal disease and treatment, food and water management, exercise and stress management, and medication management. A panel of experts made up of two renal nurse specialists, a nephrologist, and two experts in computer science evaluated the knowledge that was provided in the videos and the booklet for content validity and calibrated the web application system by testing the function of the model application’s data architecture (Fig. 5 ). This was combined with the website, which included a home page, a user panel, and an administration panel, and the mobile app, which included a user area and an expert area. The panel of experts made comments and suggestions, and the model was revised twice and returned to the panel of experts for evaluation until they were satisfied.
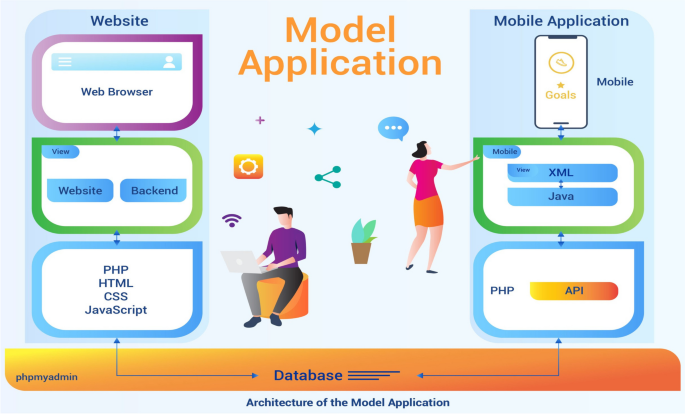
Data architecture of the model application
Finally, 3 research assistants, including an advanced practitioner nurse in hemodialysis and two hemodialysis nurses, were trained as “supporters” for assisting participants in the experimental group and also helping the researcher in implementing the intervention. The training session lasted for seven hours (a full day of training at the dialysis unit) and included a study information overview, information about the objectives and conceptual framework of the study, the instruments for recruiting potential participants, and the strategies applied to implement the model. Additionally, time was dedicated to sharing ideas, demonstrations, repeat demonstrations, and practice.
Participants and their caregivers in the experimental group received the home telehealth model in addition to standard care and were provided with a set of 1 manual and a tablet with internet connection for 6 months, containing the downloaded web application for the home telehealth model. They underwent a day of group teaching sessions, consisting of answering questions and addressing concerns as well as training in self-management skills. During each session the participants were educated about renal disease and treatment, food and water management, exercise and stress management, and medication management at home. Additionally, the participants and their caregivers were taught how to use the application, such as how to log in to the system, fill in their illness health history, record their body weight, make an online appointment, connect to Facebook, access Line to have a conversation with a hemodialysis nurse, and create a group chat with other patients. They also learned how to use video visiting, online monitoring, and telephone counselling.
The first week of group training sessions were followed by their six-month in-person participation in the home telehealth model, as well as routine online visits and telephone consulting, resulting in a total of one day per month for meeting and discussion with a hemodialysis nurse at the dialysis unit, and six months of monitoring and consultation online.
Application of the home telehealth model for OPLWH: web application
The development of the home telehealth model resulted in an application that could be used on a website or on a mobile phone and is referred to here as the web application. The website and mobile versions are connected by an application programming interface. The website creates an application programming interface and receives orders from the application. The application will then send a request for interpretation and a summary.
At the same time, the home telehealth model website is a content management system that is divided into three parts including a home page, a user panel, and an administration panel. The patient’s data from the administration panel and the web page are recalled when the patient logs in to the website linked to the user panel. The mobile application is designed to be used on a mobile phone, tablet, or iPad by connecting data from the website with the application programming interface. The mobile application site consists of two sections (user and expert). The user section is for patients to log in to make appointments and view their health history. The expert section is connected to the application programming interface, Facebook Messenger, and is controlled by a nurse or physician who checks the online appointment requests made by patients. In this section, the nurse or physician can also provide online individual counselling or group discussions (Fig. 5 ).
Control group
The control group received standard care from the nursing staff at the renal unit. Standard care for persons receiving hemodialysis in government hospital in Thailand includes routine laboratory investigations, physical examinations, health education, consultations by a renal nurse and/or a general nurse, and monthly follow-ups. After finishing the study, the participants in the control group were invited to join the home telehealth model and received copies of the set of manuals on how to manage their hemodialysis at home.
Measurement and data collection
Instruments and outcomes, the 9-item thai health status assessment instrument.
was used to evaluate the quality of life and health status of the dialysis patients [ 28 ] and is separated into two scale scores measuring physical and mental health. The instrument is composed of seven domains and two global health ratings [ 28 , 29 ]. The seven domains encourage subjects to rate their experiences with health conditions during the past month. Response choices for the seven domains are interpreted according to the perceived severity of the problems on a 5-point scale, where 1 = “very severe”, 2 = “severe”, 3 = “moderate”, 4 = “mild”, and 5 = “not at all”.
The first global question is used to compare participants’ health at the present time with their health during the preceding year. The second question compares their health with the health of others of similar age, gender, social/economic status, employment type, and lifestyle. Response choices for the two global questions included 1 = “much worse”, 2 = “a little bit worse”, 3 = “the same as”, 4 = “a little bit better”, and finally 5 = “much better”. The calculated score values range between 20 and 80 (± 3SDs); if the physical or mental health score is above 20, the scores are interpreted as equal to the general healthy Thai population. Higher calculated scores reflect better health than the general population.
The 9-item Thai Health Status Assessment Instrument is a valid and reliable quality of life measure for Thai renal replacement therapy patients. Its convergent and divergent validity have been demonstrated using the SF-36 as a concurrent measure. Its concurrent validity has also been assessed using clinical variables. Its test–retest reliability is satisfactory as the inter-class correlation coefficients are 0.79 (physical health score) and 0.78 (mental health score) [ 25 , 29 ].
After obtaining permission, we assessed the appropriateness and applicability of these instruments for our participant population. All the instruments were given to the participants in Thai language.
Demographic data
was collected at baseline. The participants were asked to provide information about their age, gender, marital status, educational level, occupation, and household income by completing the demographic data form. Also, the participants were asked about the duration of their hemodialysis treatment, while blood samples, and data related to their health status and health-related quality of life were collected at baseline (the firs week), 3 months (the last week), and 6 months (the last week).
Laboratory parameters
The laboratory parameters measured the effectiveness of the home telehealth model for older persons living with hemodialysis by comparing the significant differences between the experimental and control groups before the experiment, 3 months after, and 6 months after. The blood chemistry analyses included Blood Uria Nitrogen (BUN), Creatinine (Cr), Hemoglobin (Hb), Hematocrit (Hct), Albumin (Alb), and Potassium (K) while the adequacy of hemodialysis measurement included weekly Kt/V and Normalized protein catabolic rate (nPCR).
Data collection procedures
Purposive sampling was used to obtain a sample of 54 OPLWH, who participated in the intervention phase of the study over a period of 6 months (between 1 April 2018 to 30 September 2018). Simple randomization was used to assign participants to either the experimental or the control group. The researcher sent information letters and copies of the study protocol to the administrators of the relevant medical units to ask them for permission to collect data. The medical units had a register of OPLWH which included patients’ telephone numbers; using this register, the researcher was able to invite potential participants to take part in the study by telephone. An advanced practitioner nurse in hemodialysis and two hemodialysis nurses who were trained as “supporters” helped to recruit participants, participated in monthly meetings and discussions with researcher and participants at the hemodialysis unit, and provided monitoring and consultation online. They also helped the researcher collect laboratory parameters from medical records at the hemodialysis unit. Participants were asked to provide information about demographic data and health status assessment by completing forms and undergoing routine blood testing at baseline, 3 and 6 months. Questionnaires and measurements were used to collect data from the participants at the hemodialysis unit by the researcher. The instruments included a demographic data form, the 9 - item Thai Health Status Assessment Instrument, and blood chemistry analyses (BUN, Cr, Hb, Hct, K, Alb, Kt/V, and nPCR).
Data analysis
Data were analyzed using SPSS programme version 17. Descriptive statistics were used to analyze participants’ demographic data and clinical characteristics. Chi-square test was used to test the difference in demographic data between the two groups at baseline. The independent t-test and one-way repeated measures ANOVA were applied to compare differences in mean scores for quality of life between the two groups. One-way repeated measures ANOVA was also used to test the interaction of the Home Telehealth Model within the two groups. ANOVA was used to detect the interaction of the model and statistically significant differences in laboratory results (BUN, Cr, Hb, Hct, K, Alb, Kt/V, nPCR) between the experimental and control groups at baseline and at 3 and 6 months.
Information of participants
From Table 1 , participants’ demographic data were analyzed at baseline, and there were no differences between the experimental and control groups in terms of gender, age, marital status, religion, education level, current occupation, people living together, regular contact person, and payment of medical expenses. Of all general characteristics, only income, duration of receiving hemodialysis, and illness with other disease showed a statistical difference between the intervention and control groups at baseline.
In both groups, most participants were between 60–69 years old, and their education level was primary school. In addition, most of the participants were Buddhist. Most participants had insufficient income and received financial support from their children. However, both groups received payments from the health care coverage scheme for medical expenses.
Table 2 shows that most participants in both the experimental and control groups had received kidney replacement therapy or dialysis during the past 1–5 years. Moreover, both groups also had underlying diseases, for example hypertension, diabetes mellitus and heart disease, especially hypertension which was the highest percentage (70–80%) of the chronic diseases found in participants from each group. There were no differences in terms of demographic characteristics of the participants in receiving treatment when comparing the two groups.
Comparison of quality of life and laboratory parameters between and within the two groups
As shown in Table 3 , before the intervention, the independent t-test results showed no statistically significant difference between the two groups in mean scores of laboratory parameters ( P > 0.05). However, after 3 months, the independent t-test revealed a statistically significant difference between the two groups in the mean score of Kt/V ( P < 0.05). In addition, 6 months after the intervention, the comparison of laboratory results between the two groups found a statistically significant difference in the mean hemoglobin (Hb) score ( P < 0.05).
Table 4 displays changes in the means of patient outcomes across three time periods. The repeated measured one-way ANOVA using the General linear model and Sphericity assumed for within-subjects and between-subjects effect were performed for the multiple comparison test. The comparison between the two groups at the three points of measurement showed no statistically significant difference in either quality of life or laboratory parameters. However, after 3 months, the quality-of-life scores for the mental health dimension showed statistically significant differences between the two groups ( P < 0.05).
This study evaluated whether a hemodialysis nursing team integrated with a home telehealth model was a feasible method of holistic care delivery to maintain quality of life and improve health outcomes among OPLWH. The results confirm that the integrated intervention is feasible for improving the participants health outcomes. Telehealth and inter-professional care can be successfully implemented with meaningful engagement as part of the care system while telehealth delivered and supported by an inter-professional team has been shown to be a feasible care delivery strategy for CKD patients [ 14 ]. Advances in health technology over recent decades have kindled interest in the possibility of using home telehealth programs to extend human resources, improve access to services, and minimize the costs of care [ 15 ].
Before the COVID-19 pandemic, there were limitations related to remote care that created barriers to patient access to continuum care via telehealth, and these limitations disincentivized renal nurses to offer telehealth as an option. However, providing and receiving tele-visiting became the norm for nurses, healthcare teams, and patients when social distancing, stay-at-home, and other hospital policies were introduced in 2020 due to the COVID-19 pandemic. The utilization of the home telehealth model should be promoted and expanded for its ability to promote equal access to telehealth and the opportunity for CKD patients living with hemodialysis to receive continuing care outside hospitals. Both inpatient and outpatient practices had to quickly adapt to telehealth [ 30 ], and the development of home telehealth services has since become an essential and valuable aspect of care, allowing patients with advanced conditions to remain at home and in their own communities, although it is still the case that when they experience problems, they may feel unsure about who they can contact, or how. Nevertheless, the use of telehealth services can help to empower individuals in terms of their experiences with life-limiting illness, and the experiences of their caretakers, by facilitating the provision of real-time communication between patients and healthcare providers [ 31 ]. It can also be used to complement transitions from acute services based on patients’ needs [ 32 ].
In this study, the participants who received the home telehealth model showed no significant effects observed in terms of their quality of life or clinical outcomes over 6 months ( P > 0.05). Comparison within the intervention group between two time measurements (at baseline vs after 3 months) found statistical evidence of improvement in the mean scores of health outcomes (BUN: 53.86 ± 21.61 vs 45.34 ± 21.10; Cr: 9.67 ± 14.04 vs 6.53 ± 2.87; Hb: 10.39 ± 1.42 vs 10.95 ± 1.69; Hct: 33.02 ± 4.55 vs 34.44 ± 4.78; Alb: 4.36 ± 1.87 vs 3.91 ± 0.60; and K: 4.42 ± 0.75 vs 4.35 ± 0.79) and patients’ quality of life (physical health scores: 44.85 ± 8.09 vs 48.42 ± 8.92 and mental health scores: 45.86 ± 7.05 vs 49.89 ± 8.97). After 6 months, the comparison within the intervention group showed increases in the mean scores of Hct (34.44 ± 4.78vs 35.14 ± 8.43), Kt/V (1.59 ± 0.37 vs 1.66 ± 0.31), and nPCR (0.99 ± 0.26 vs 1.17 ± 0.73). According to the three-time measurements of quality of life and laboratory parameters, there was no statistical difference between the two groups ( P > 0.05). However, regarding the comparison using the independent t-test, after 3 months and 6 months, some of the laboratory (Kt/V and Hb) results showed statistically significant differences between the two groups ( P < 0.05) while some of the tests were not significant (BUN, Cr, Hct, K, Alb, and nPCR) ( P > 0.05). In addition, after 3 months repeated measurement, quality of life, in terms of the mental health scores, showed statistically significant differences between the two groups ( P < 0.05).
Further investigation might be needed in terms of the relationships between each laboratory result and the intervention. This finding is contradictory to the findings of previous studies. According to Minatodani, Chao, and Berman, home telehealth for high-risk dialysis patients has demonstrated improvements in the cost-effectiveness of health outcomes over 21 months [ 33 ]. In addition, a randomized controlled study on the use of home telehealth in promoting illness self-management demonstrated an improvement in health outcomes and cost-effectiveness for high-risk dialysis patients [ 34 ]. Home telehealth has also demonstrated improved health outcomes and cost-effectiveness for high-risk patients with ESRD, using remote technology for home health monitoring with support from remote care nurses [ 34 ]. Another study reported a statistically significant reduction in depression, anxiety, and stress due to telehealth interventions [ 35 ]. In contrast, the biomarkers predicted patients’ clinical outcomes. Standardized Kt/V was a significant indicator for the adequacy of the dialysis and was related to a reduction in clinical complications of dialysis patients, such as hypocalcemia, hyperphosphatemia, and anemia [ 36 ]. It was also suggested that poorer laboratory indicators demonstrated an inability to control mineral bone density and anemia [ 12 ]. The participants received hemodialysis treatment 2–3 times per week, and they needed to evaluate their hemodialysis adequacy following the KDOQI Clinical Practice Guidelines for Hemodialysis Adequacy: 2015 update [ 36 ]. Dialysis dose has been reported to have great significance for the outcome of hemodialysis treatment. Many studies have shown a relationship between dialysis dose, measured as Kt/V, and morbidity and mortality among hemodialysis patients [ 36 ]. In the case of inadequate Kt/V, patients needed to increase the dose of dialysis. In addition, the lower nPCR indicated that patients needed to increase their protein intake, or they would be at a risk for malnutrition. Patients who had low serum levels of albumin and creatinine clearance may report low quality of life and inadequacy of dialysis [ 17 , 36 ].
These results suggest that at 3 months after the intervention, the experimental group achieved a higher quality of life than the control group along both physical and mental dimensions. In the experimental group, the mean quality of life from the mental health scores showed a more positive increase than for the control group at three points of measurement. However, the success of home telehealth depends strongly on patient adherence to the prescribed program [ 6 , 17 ]. Home telehealth with remote care nurse (RCN) support can promote health behavioral change, resulting in better outcomes. Moreover, home telehealth self-monitoring with RCN support can effectively empower patients to take a more active role in their healthcare, indirectly improving quality of life for people living with chronic illness [ 18 ]. Unfortunately, we did not detect a statistically significant difference in quality of life between the two groups using repeated independent t-test and one-way repeated ANOVA. This might be because the six-month duration was not sufficient, or because the sample group was simply too small to observe a significant difference. In addition, 50% of participants in both groups were between 60–69 years old, had a low education level, needed financial support, and depended on their family members. These demographic characteristics could be indirect factors in reducing patients’ ability for maintaining adherence behavior in management with hemodialysis complications at home. Moreover, longtime management with hemodialysis and having comorbidities such as hypertension, diabetes mellitus, and heart disease can have negative impacts by increasing morbidity and mortality rates, reducing quality of life, and resulting in inadequate symptom management at home [ 24 ]. According to Table 2 , the difference in duration of receiving hemodialysis treatment, especially for patients with CKD stage 3–5, could result in poorer clinical outcomes and higher risk of emergency department visit, hospitalization, and death. This is related to the negative impacts of prolonged hemodialysis cycles for people living with ESRD, and fluctuations in terms of their cognitive and physical wellbeing, as well as in their emotional state [ 14 ]. Increased age, history of diabetes, longer dialysis vintage, and lower Kt/V were independently associated with frailty [ 37 ]. Frailty is also extremely common and influences serious clinical outcomes among older hemodialysis patients [ 37 ]. Integrating a home telehealth model with nurse oversight into holistic care for OPLWH can help them maintain their quality of life and improve their health outcomes. Moreover, the literature related to telehealth and kidney disease care has strongly reflected high patient satisfaction [ 30 ]. The results of this study show that integrating home telehealth with nurse oversight into holistic care for OPLWH has acceptable feasibility in providing a higher quality of life, as well as in terms of relative improvements in some biometric outcomes and the quality of dialysis. Therefore, it will be highly beneficial to establish such a model in renal units. However, the effectiveness of different telehealth models may need to be evaluated before implementation.
Strengths and limitations
Like any research, our study has both limitations and strengths. This is the first study of its kind in Thailand. Its main strength is the study’s implementation site, namely a hemodialysis center in an urban setting where the prevalence of OPLWH is relatively high.
On the other hand, our study had two limitations. Our relatively small sample size is likely to limit the external validity of the study. We should also recognize that the participants were OPLWH who might be unable to access particular technologies, or who may be unfamiliar with using technologies such as web applications. To solve this problem, we asked caregivers to stay with participants during the training and to be present during consultations in the 6-month intervention period (monthly routine online visits and telephone consulting), in order to help participants to access the web application and to support them when they needed help. Other studies have also found that more comprehensive interventions can require a caregiver or other person to assist patients [ 30 ].
However, those who live alone and have inadequate social support might have poorer self-management behaviors [ 17 , 38 ] and might not gain as much benefit from a telehealth model in terms of disparities in accessing telehealth for patients having lower socio-economic status. Moveover, patients with limited health literacy or geriatric symptoms, such as hearing impairment, visual problems, and fingers or bodily shaking, may not be able to operate the video calls or may not have the technical capability. In addition, it is possible that these factors affect the ability of some to have a serious discussion with clinicians during the time that they communicate via video telehealth or phone. In addition, older persons who live alone may not be able to maintain their engagement in management with self-care, medical regimens, and self-monitoring, affecting their ability to effectively report about their clinical progression [ 21 ].
Furthermore, the study was conducted at a time when third generation (3G) internet coverage was offered in Thailand. This was suitable for participants who owned modern smartphones and lived in urban areas with constant high-speed internet connection but may have presented limitations for participants in more rural areas or those who did not have access to the most up-to-date technology. To mitigate these problems, the researcher provided all participants with a mini-iPad and free Wi-Fi for 6 months so that they could regularly access the home telehealth program. Currently, fifth generation (5G) internet coverage is standard in Thailand, and Thai health policy is focusing on the digital transformation concept to ensure the vast majority of users, including the older population, are familiar with accessing internet communication in both urban and rural areas.
Implications for clinical practice
Due to limitations on renal nurses visiting patients at home and the transforming of the digital health system after the COVID-19 pandemic, older persons undergoing hemodialysis may suffer with clinical complications at home and require clinical nurse specialists to provide them with continuing renal care. Integrating a home telehealth model for this population is a feasible response, enhancing their quality of life and improving their health outcomes. The value of a home telehealth self-monitoring model with nurse oversight has been demonstrated with positive results in terms of reducing clinical complications. Despite less contact with homecare facilities due to shortages of clinical nurse specialists visiting patients at home, OPLWH experienced sustained quality of life and improved clinical outcomes after receiving the integrated home telehealth model.
In the future , renal nurses in dialysis units should emphasize the use of a telehealth model to promote the physical and mental health of their patients. Effective digital health innovations for virtual care, such as the home telehealth model implemented in this study, can provide effective solutions to the problem of a shortage of renal nurse specialists, and possibly decrease care costs for patients. Despite the study limitations, however, we recommend the use of a home telehealth model for OPLWH, based on its potential for increasing quality of life and reducing the risk of clinical complications by improving patients’ health outcomes. Further studies within different settings, populations, and cultural contexts are recommended in order to confirm the effectiveness of the home telehealth model. In addition, improving the cost effectiveness of future studies should focus on larger sample sizes, 1-year interventions, and intensive retraining sessions for patients, caregivers, and renal clinicians. An expansion of the criteria of reimbursement and regulation for patients who receive telehealth services is needed. Telephone calls may be appropriate for patients of lower socio-economic status, or those having poor internet access and low levels of technological literacy.
Our findings confirm that the implementation of a home telehealth model can yield positive changes in all aspects, including quality of life and health outcomes, for OPLWH. However, our findings are based on a short-term intervention over a six-month period. The long-term benefits of such a program should also be evaluated, and its economic impact on the delivery of healthcare services should be assessed. Our integrated holistic home telehealth model was an effective strategy to increase health-related quality of life and health outcomes over a 6-month implementation period. Our study provides evidence in favour of the feasibility of a home telehealth model for OPLWH after they have received training alongside their standard care. Such a model may potentially reduce workloads for renal nurses and improve routine care, enhancing health-related quality of life and reducing complications among OPLWH.
Availability of data and materials
The datasets used and analyzed during the current study are available from the corresponding author on reasonable request. The statistics were checked prior to submission by an expert statistician and state their name and email address.
Abbreviations
Chronic kidney disease
Renal replacement therapy
Peritoneal dialysis
- Hemodialysis
Kidney transplantation
End-stage renal disease
Older persons living with hemodialysis
Estimated glomerular filtration rate
Blood Uria Nitrogen
Normalized protein catabolicrate
A measurement of dialysis adequacy
Urea reduction ratio
United Nations Department of Economic and Social Affairs Population Division. World population prospects 2022. 2022. https://population.un.org/wpp/ . Accessed 20 Nov 2022.
Google Scholar
Knodel J, Teerawichitchainan B, Prachuabmoh V, Pothisiri W. The situation of Thailand’s older population: an update based on the 2014 survey of older persons in Thailand. Chiang Mai: HelpAge International; 2015.
National Statistics Office. Ministry of information and communication technology older persons in Thailand. 2014. http://web.nso.go.th/en/survey/age/tables_older_2014.pdf . Accessed 13 Jan 2021.
Feng L, Yap KB, Yeoh LY, Ng TP. Kidney function and cognitive and functional decline in elderly adults: findings from the Singapore longitudinal aging study. J Am Geriatr Soc. 2012;60(7):1208–14. https://doi.org/10.1111/j.1532-5415.2012.04043.x .
Article PubMed Google Scholar
Bello AK, Alrukhaimi M, Ashuntantang GE, Basnet S, Rotter RC, Douthat WG, et al. Complications of chronic kidney disease: current state, knowledge gaps, and strategy for action. Kidney Int Suppl (2011). 2017;7(2):122–9. https://doi.org/10.1016/j.kisu.2017.07.007 .
Kelly JT, Warner MM, Conley M, Reidlinger DP, Hoffmann T, Craig J, et al. Feasibility and acceptability of telehealth coaching to promote healthy eating in chronic kidney disease: a mixed-methods process evaluation. BMJ Open. 2019;9(1): e024551. https://doi.org/10.1136/bmjopen-2018-024551 .
Article PubMed PubMed Central Google Scholar
Pungchompoo W, Richardson A, Brindle LA. Experiences and needs of older people with end stage renal disease: bereaved carers perspective. Int J Palliat Nurs. 2016;22(10):490–9. https://doi.org/10.12968/ijpn.2016.22.10.490 .
Levey AS, Coresh J, Balk E, Kausz AT, Levin A, Steffes MW, et al. National Kidney Foundation practice guidelines for chronic kidney disease: evaluation, classification, and stratification. Ann Intern Med. 2003;139(2):137–47. https://doi.org/10.7326/0003-4819-139-2-200307150-00013 .
Malkina A, Tuot DS. Role of telehealth in renal replacement therapy education. Semin Dial. 2018;31(2):129–34. https://doi.org/10.1111/sdi.12663 .
Nephrology Society of Thailand. Annual reports on Thailand’s renal replacement therapy figures for the years 2016 to 2019. 2021. https://www.nephrothai.org/annual-report-thailand-renal-replacement-therapy-2007-2019-th/ . Accessed 1 May 2021.
Finet P, Le Bouquin Jeannès R, Dameron O, Gibaud B. Review of current telemedicine applications for chronic diseases. Toward a more integrated system? IRBM. 2015;36(3):133–57. https://doi.org/10.1016/j.irbm.2015.01.009 .
Article Google Scholar
Krishna VN, Managadi K, Smith M, Wallace E. Telehealth in the delivery of home dialysis care: catching up with technology. Adv Chronic Kidney Dis. 2017;24(1):12–6. https://doi.org/10.1053/j.ackd.2016.11.014 .
Margolis KL, Asche SE, Bergdall AR, Dehmer SP, Groen SE, Kadrmas HM, et al. Effect of home blood pressure telemonitoring and pharmacist management on blood pressure control: a cluster randomized clinical trial. JAMA. 2013;310(1):46–56. https://doi.org/10.1001/jama.2013.6549 .
Article CAS PubMed PubMed Central Google Scholar
Ishani A, Christopher J, Palmer D, Otterness S, Clothier B, Nugent S, et al. Telehealth by an interprofessional team in patients with CKD: a randomized controlled trial. Am J Kidney Dis. 2016;68(1):41–9. https://doi.org/10.1053/j.ajkd.2016.01.018 .
Hebert MA, Korabek B, Scott RE. Moving research into practice: a decision framework for integrating home telehealth into chronic illness care. Int J Med Inform. 2006;75(12):786–94. https://doi.org/10.1016/j.ijmedinf.2006.05.041 .
Suter P, Yueng C, Johnston D, Suter WN. Telehealth infection control: a movement toward best practice. Home Healthc Nurse. 2009;27(5):319–23. https://doi.org/10.1097/01.nhh.0000356785.85750.32 .
Arad M, Goli R, Parizad N, Vahabzadeh D, Baghaei R. Do the patient education program and nurse-led telephone follow-up improve treatment adherence in hemodialysis patients? A randomized controlled trial. BMC Nephrol. 2021;22(1):119. https://doi.org/10.1186/s12882-021-02319-9 .
Suwanrada W, Pothisiri W, Siriboon S, Bangkaew B, Milintangul C. Evaluation of the replication project of the elderly home care volunteers. Bangkok: College of Population Studies, Chulalongkorn University; 2016.
Lunney M, Lee R, Tang K, Wiebe N, Bello AK, Thomas C, et al. Impact of telehealth interventions on processes and quality of care for patients with ESRD. Am J Kidney Dis. 2018;72(4):592–600. https://doi.org/10.1053/j.ajkd.2018.02.353 .
Lambooy S, Krishnasamy R, Pollock A, Hilder G, Gray NA. Telemedicine for outpatient care of kidney transplant and CKD patients. Kidney Int Rep. 2021;6(5):1265–72. https://doi.org/10.1016/j.ekir.2021.02.016 .
Ladin K, Porteny T, Perugini JM, Gonzales KM, Aufort KE, Levine SK, et al. Perceptions of telehealth vs in-person visits among older adults with advanced kidney disease, care partners, and clinicians. JAMA Netw Open 2021;4(12). https://doi.org/10.1001/jamanetworkopen.2021.37193 .
Creswell JW, Plano Clark VL. Designing and conducting mixed methods research. 2nd ed. California: SAGE; 2011.
Creswell JW. Research Design. 4th ed. California: SAGE; 2014.
Pungchompoo W, Udomkhwamsuk W. Symptom experiences and needs of older persons living with hemodialysis in relation to integrating home telehealth into holistic end of life care: Phase I. CMU J Nat Sci. 2021;20(4):e2021081. https://doi.org/10.12982/CMUJNS.2021.081 .
Pungchompoo W, Parinyajittha S, Pungchompoo S, Kumtan P. Effectiveness of a self-management retraining program improving the quality of life of people receiving continuous ambulatory peritoneal dialysis. Nurs Health Sci. 2020;22(2):406–15. https://doi.org/10.1111/nhs.12672 .
Bowling A. Research methods in health: investigating health and health services. Buckingham, UK: Open University Press; 2002.
Kang H. Sample size determination for repeated measures design using G Power software. Anesth Pain Med. 2015;10(1):6–15. https://doi.org/10.17085/apm.2015.10.1.6 .
Cheawchanwattana A, Limwattananon C, Gross C, Limwattananon S, Tangcharoensathien V, Pongskul C, Sirivongs D. The validity of a new practical quality of life measure in patients on renal replacement therapy. J Med Assoc Thai. 2006;89(Suppl 2):S207–17.
PubMed Google Scholar
Saisunantararom W, Cheawchanwattana A, Kanjanabuch T, Buranapatana M, Chanthapasa K. Associations among spirituality, health-related quality of life, and depression in pre-dialysis chronic kidney disease patients: an exploratory analysis in Thai buddhist patients. Religions. 2015;6(4):1249–62.
Lew SQ, Sikka N. Telehealth and kidney disease care: role after the public health emergency? Clin J Am Soc Nephrol. 2021;16(12):1784–6. https://doi.org/10.2215/cjn.13651021 .
Johnston MJ, King D, Arora S, Behar N, Athanasiou T, Sevdalis N, Darzi A. Smartphones let surgeons know WhatsApp: an analysis of communication in emergency surgical teams. Am J Surg. 2015;209(1):45–51. https://doi.org/10.1016/j.amjsurg.2014.08.030 .
Roberts D, Tayler C, MacCormack D, Barwich D. Telenursing in hospice palliative care. Can Nurse. 2007;103(5):24–7.
Minatodani DE, Chao PJ, Berman SJ. Home telehealth: facilitators, barriers, and impact of nurse support among high-risk dialysis patients. Telemed J E Health. 2013;19(8):573–8. https://doi.org/10.1089/tmj.2012.0201 .
Berman SJ, Wada C, Minatodani D, Halliday T, Miyamoto R, Lindo J, Jordan PJ. Home-based preventative care in high-risk dialysis patients: a pilot study. Telemed J E Health. 2011;17(4):283–7. https://doi.org/10.1089/tmj.2010.0169 .
Kargar Jahromi M, Javadpour S, Taheri L, Poorgholami F. Effect of nurse-led telephone follow ups (tele-nursing) on depression, anxiety and stress in hemodialysis patients. Glob J Health Sci. 2015;8(3):168–73. https://doi.org/10.5539/gjhs.v8n3p168 .
Daugirdas JT, Depner TA, Inrig J, Mehrotra R, Rocco MV, Suri RS, Weiner DE. KDOQI Clinical Practice Guideline for Hemodialysis Adequacy: 2015 update. Am J Kidney Dis. 2015;66(5):884–930. https://doi.org/10.1053/j.ajkd.2015.07.015 .
Guo Y, Tian R, Ye P, Luo Y. Frailty in older patients undergoing hemodialysis and its association with all-cause mortality: a prospective cohort study. Clin Interv Aging. 2022;17:265–75.
Chen YC, Chang LC, Liu CY, Ho YF, Weng SC, Tsai TI. The roles of social support and health literacy in self-management among patients with chronic kidney disease. J Nurs Scholarsh. 2018;50(3):265–75. https://doi.org/10.1111/jnu.12377 .
Download references
Acknowledgements
This study was supported by a grant from the National Research Council of Thailand. This research was also supported by the Office of Research Administration, Chiang Mai University, for the Health Promotion, Technology and Innovation Research Group. Regarding the 9-item Thai Health Status Assessment Instrument (9-Thai), permission for its use in this study was given by Assistant Professor Dr. Areewan Cheawchanwattana. The authors would like to give special thanks to their participants who graciously gave their time to take part in this study.
Authors’ statement
The authors would like to confirm that:
1. The manuscript has not been published or submitted for publication elsewhere.
2. All authors approved the content of the manuscript and have contributed significantly to the research involved in the process of protocol development, data collection and analysis, discussion and writing for manuscript publication and process of manuscript’s revision.
3. The protocol for the research project has been approved by the Research Ethics Committees of the Institutional Review Board of Faculty of Nursing (IRB) and,
4. by the Research Ethics Committees of the Institutional Review Board of the Faculty of Medicine, Chiang Mai University, Thailand.
5. All participants gave informed consent for the research, and that their anonymity was preserved according to the Declaration of Helsinki (as revised in Brazil 2013).
6. We declare not to have any financial support or relationships that may pose a conflict of interest by disclosing any financial arrangements they have with a company whose product figures prominently in the submitted manuscript or with a company making a competing product, or any conflict relating to technology or methodology.
7. This research was funded by the National Research Council of Thailand (NRCT) and Chiang Mai University, Thailand.
8. The researchers have received permission for using the instruments utilized in the study.
This study is supported by funding from the National Research Council of Thailand (NRCT) (Grant No. 270392) and the Chiang Mai University (Grant No. R000016567, Grant No. R000029878).
Author information
Authors and affiliations.
Department of Medical Nursing, Faculty of Nursing, Chiang Mai University, Chiang Mai, Thailand
Wanicha Pungchompoo
Department of Nephrology, Faculty of Medicine, Chiang Mai University, Chiang Mai, Thailand
Saowaros Parinyachitta
Department of Industrial and Manufacturing Engineering, Faculty of Engineering, Prince of Songkla University, Songkhla, Thailand
Sirirat Pungchompoo
Department of Surgical Nursing, Faculty of Nursing, Chiang Mai University, Chiang Mai, Thailand
Warawan Udomkhwamsuk
Department of Medicine, Faculty of Medicine, Chiang Mai University, Chiang Mai, Thailand
Panadda Suwan
You can also search for this author in PubMed Google Scholar
Contributions
W.P. contributed to the concept and the design of the study, as well as provided supervision. W.P. and Sa.P. were also involved in the development of the Telehealth model and in the web-application design and development. W.P., S.P. and P.S. were involved with the data materials and data collection processing. W.P. and Si.P. provided analysis and/or interpretation for the study. W.P. and W.U. conducted a search of the literature. W.P. wrote the manuscript, and W.P. provided a critical review of the manuscript, revisions for important intellectual content, and other work. All authors read and approved of the final manuscript.
Corresponding author
Correspondence to Wanicha Pungchompoo .
Ethics declarations
Ethics approval and consent to participate.
The study protocol was approved by the Research Ethics Committee of the Institutional Review Board of the Faculty of Nursing and the Faculty of Medicine, Chiang Mai University, Thailand, Institutional Review Board No. 003–2559 (date of approval: November 30, 2016; date of expiry: November 29, 2017, and date of extension: September 2018), before the process of data collection began.
After approval was granted, the researchers informed the potential research subjects about the purpose of the research, its possible benefits, and the research process. Potential subjects were told that their participation was voluntary, that they had the right to choose whether to participate or not, and that their decision would not affect the treatment they were receiving in the hospital. They were given clear information, and they expressed their clear understanding and decision to participate before signing an informed consent form. The researchers considered each patient’s confidentiality and reassured them the data from the research results would be presented as a whole, not broken down by each individual. The researchers were available to the participants if they had any further questions.
Consent for publication
Not applicable.
Competing interests
The authors declare no competing interests.
Additional information
Publisher’s note.
Springer Nature remains neutral with regard to jurisdictional claims in published maps and institutional affiliations.
Rights and permissions
Open Access This article is licensed under a Creative Commons Attribution 4.0 International License, which permits use, sharing, adaptation, distribution and reproduction in any medium or format, as long as you give appropriate credit to the original author(s) and the source, provide a link to the Creative Commons licence, and indicate if changes were made. The images or other third party material in this article are included in the article's Creative Commons licence, unless indicated otherwise in a credit line to the material. If material is not included in the article's Creative Commons licence and your intended use is not permitted by statutory regulation or exceeds the permitted use, you will need to obtain permission directly from the copyright holder. To view a copy of this licence, visit http://creativecommons.org/licenses/by/4.0/ . The Creative Commons Public Domain Dedication waiver ( http://creativecommons.org/publicdomain/zero/1.0/ ) applies to the data made available in this article, unless otherwise stated in a credit line to the data.
Reprints and permissions
About this article
Cite this article.
Pungchompoo, W., Parinyachitta, S., Pungchompoo, S. et al. The feasibility of integrating a home telehealth model for older persons living with hemodialysis. BMC Geriatr 24 , 378 (2024). https://doi.org/10.1186/s12877-024-04981-8
Download citation
Received : 24 October 2023
Accepted : 16 April 2024
Published : 27 April 2024
DOI : https://doi.org/10.1186/s12877-024-04981-8
Share this article
Anyone you share the following link with will be able to read this content:
Sorry, a shareable link is not currently available for this article.
Provided by the Springer Nature SharedIt content-sharing initiative
- Older persons
BMC Geriatrics
ISSN: 1471-2318
- Submission enquiries: [email protected]
- General enquiries: [email protected]

IMAGES
VIDEO
COMMENTS
However, they differ in their approach and purpose. A case study involves in-depth analysis of a particular individual, group, or situation, aiming to provide a detailed understanding of a specific phenomenon. On the other hand, an experiment involves manipulating variables and observing the effects on a sample population, aiming to establish ...
Key Differences. Case studies involve a detailed examination of a specific subject, providing rich qualitative data. Experiments are structured to test hypotheses, involving variable control and manipulation. 15. In case studies, data is gathered from real-world observations, interviews, and documents. Experiments rely on quantitative data from ...
In a case series, several patients with similar experiences are grouped. Analytical Studies. Analytical studies are of 2 types: observational and experimental. Observational studies are studies that we conduct without any intervention or experiment. In those studies, we purely observe the outcomes.
Case study and research are both methods used in academic and professional settings to gather information and gain insights. However, they differ in their approach and purpose. A case study is an in-depth analysis of a specific individual, group, or situation, aiming to understand the unique characteristics and dynamics involved.
Revised on November 20, 2023. A case study is a detailed study of a specific subject, such as a person, group, place, event, organization, or phenomenon. Case studies are commonly used in social, educational, clinical, and business research. A case study research design usually involves qualitative methods, but quantitative methods are ...
The differences are apparent in terms of emphasis (e.g., more observations in ethnog-raphy, more interviews in grounded theory) and extent of data collection (e.g., only interviews in phenomenology, multiple forms in case study research to provide the in-depth case picture). At the data analysis stage, the differences are most pronounced.
You can also create a mixed methods research design that has elements of both. Descriptive research vs experimental research. Descriptive research gathers data without controlling any variables, while experimental research manipulates and controls variables to determine cause and effect.
The current conflict between case study and experimental research: A breakthrough study derives benefits from both. (ERIC Document Number ED339721). This paper describes a study that was not experimentally designed, but its major findings were generalizable to the overall population of writers in college freshman composition classes.
A case study is one of the most commonly used methodologies of social research. This article attempts to look into the various dimensions of a case study research strategy, the different epistemological strands which determine the particular case study type and approach adopted in the field, discusses the factors which can enhance the effectiveness of a case study research, and the debate ...
One distinction worth making between two types of non-experimental research is the difference be- tween quasi-experimental research and case studies. The example I discussed earlier - in which we wanted to examine incidence of lung cancer among smokers and non-smokers, without trying to control who smokes and who doesn't - is a quasi ...
Experimental research serves as a fundamental scientific method aimed at unraveling. cause-and-effect relationships between variables across various disciplines. This. paper delineates the key ...
Descriptive, correlational, and experimental research designs are used to collect and analyze data. Descriptive designs include case studies, surveys, and naturalistic observation. The goal of these designs is to get a picture of the current thoughts, feelings, or behaviours in a given group of people.
Unravel the differences between observational and experimental studies. Dive into the intricacies of each method and discover their unique applications in research. ... Case-Control Studies: Case-control studies, on the other hand, involve the comparison of individuals with a particular condition or outcome to those without it (the control ...
A case study is a research method in which the researcher explores the subject in depth, while an experiment is a research method where two specific groups or variables are used to test a hypothesis. This article will examine the differences between case study and experiment further.
One of the key differences between case studies and single-case experimental designs is their generalizability. Case studies are often conducted on unique or rare cases, making it challenging to generalize the findings to a larger population. The focus of case studies is on providing detailed insights into specific cases rather than making ...
A case study is a detailed study of a specific subject, such as a person, group, place, event, organisation, or phenomenon. Case studies are commonly used in social, educational, clinical, and business research. A case study research design usually involves qualitative methods, but quantitative methods are sometimes also used.
Actually, the term is "Sample Survey" and you may search online for it. I think the difference lies in the aim of the three types of studies, sample surveys want to get data for a parameter while observational studies and experiments want to convert some data into information, i.e., correlation and causation respectively.
Within analytic research, a further distinction can be made between experimental and observational analytic studies. 3 In experimental studies, that is, RCTs, the investigator intentionally manipulates the intervention by randomly allocating participants to the intervention or control group. 4 In contrast, in observational analytic studies, the ...
Descriptive, correlational, and experimental research designs are used to collect and analyze data. Descriptive designs include case studies, surveys, and naturalistic observation. The goal of these designs is to get a picture of the current thoughts, feelings, or behaviors in a given group of people.
Controlled experiments establish causality, whereas correlational studies only show associations between variables. In an experimental design, you manipulate an independent variable and measure its effect on a dependent variable. Other variables are controlled so they can't impact the results. In a correlational design, you measure variables ...
Different study designs are needed for the description of aging and for the analysis of the explanatory mechanisms that cause age-associated change. Scientists should use the most appropriate design to study their research questions. At a general level, observational (non-experimental) studies and experimental studies can be distinguished (Fig ...
A scientific procedure wherein the factor under study is isolated to test hypothesis is called an experiment. Surveys are performed when the research is of descriptive nature, whereas in the case of experiments are conducted in experimental research. The survey samples are large as the response rate is low, especially when the survey is ...
Differences between models and future research needs Our results suggest that climate change might become a more important driver of terrestrial biodiversity loss than land-use change by midcentury ( Figs. 1 and 2 ), in agreement with recent findings based on single metrics ( 10 ) and in contrast to an earlier review ( 6 ).
Revised on January 22, 2024. Like a true experiment, a quasi-experimental design aims to establish a cause-and-effect relationship between an independent and dependent variable. However, unlike a true experiment, a quasi-experiment does not rely on random assignment. Instead, subjects are assigned to groups based on non-random criteria.
Objective To determine the efficacy of psilocybin as an antidepressant compared with placebo or non-psychoactive drugs. Design Systematic review and meta-analysis. Data sources Five electronic databases of published literature (Cochrane Central Register of Controlled Trials, Medline, Embase, Science Citation Index and Conference Proceedings Citation Index, and PsycInfo) and four databases of ...
In Thailand, there is a rapidly increasing population of older persons living with hemodialysis (OPLWH) for whom quality of life and clinical outcomes are their main focus. This study aims to assess the feasibility of an integrated home telehealth model on quality of life and laboratory parameters of OPLWH. In this study, the second phase of a mixed methods exploratory sequential design was ...