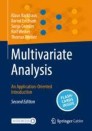
Multivariate Analysis pp 381–452 Cite as

Factor Analysis
- Klaus Backhaus 6 ,
- Bernd Erichson 7 ,
- Sonja Gensler 8 ,
- Rolf Weiber 9 &
- Thomas Weiber 10
- First Online: 29 June 2023
1007 Accesses
1 Altmetric
The explorative factor analysis is a procedure of multivariate analysis which aims at identifying structures in large sets of variables. Large sets of variables are often characterized by the fact that as the number of variables increases, it may be assumed that more and more variables are correlated. The exploratory factor analysis aims to structure the relationships in a large set of variables to the extent that it identifies groups of variables that are highly correlated with each other and separates them from less correlated groups. The groups of highly correlated variables are called factors. Apart from the structuring function, factor analysis is also used for data reduction. At the end of the chapter, there is also a brief outlook on confirmatory factor analysis, in which predefined factor structures are examined.
This is a preview of subscription content, log in via an institution .
Buying options
- Available as PDF
- Read on any device
- Instant download
- Own it forever
- Available as EPUB and PDF
- Compact, lightweight edition
- Dispatched in 3 to 5 business days
- Free shipping worldwide - see info
Tax calculation will be finalised at checkout
Purchases are for personal use only
Correlations form the basis of factor analysis. For readers who are not sufficiently familiar with the term, the concept of correlations is explained in detail in Sect. 1.2.2 .
On the website www.multivariate-methods.info , we provide supplementary material (e.g., Excel files) to deepen the reader’s understanding of the methodology.
Standardized variables have an average of 0 and a variance of 1. For the standardization of variables see also Sect. 1.2.1 .
For a brief summary of the basics of statistical testing see Sect. 1.3 .
The determinant of the correlation matrix in the application example is det = 0.001. Furthermore, ln (0.00096) = −6.9485.
If a variable x j is transformed into a standardized variable z j , the mean value of z j = 0 and the variance of z j = 1. This results in a considerable simplification in the representation of the following relationships. See the explanations on standardization in Sect. 1.2.1 .
Please note that example 3 does not correspond to the application example in Sect. 7.2.1 .
Standardized variables with a unit variance of 1 are assumed. For the decomposition of the variance of an output variable, see also the explanations in Sect. 7.2.2.4.2 and especially Fig. 7.13 .
Remember that we use standardized variables and therefore the variance of each variable is 1 and the total variance in the data set is 5.
Mathematically the eigenvalues are calculated first (this is a standard problem of mathematics) and then the components or factors are derived.
On the problem of interpreting factors or main components, also compare Sect. 7.2.3 and the case study in Sect. 7.3.3.4 .
In contrast, the case study shows major differences between PCA and PAF (cf. Sect. 7.3.3.4 ).
In contrast to PAF, the aim of the ML, GLS and ULS methods is to determine the factor loadings in such a way that the difference between the empirical correlation matrix ( R ) and the model-theoretical correlation matrix ( \({\hat{\mathbf{R}}}\) ) is minimal. In alpha factorization, Cronbach’s alpha is maximized, and image factorization is based on the image of a variable. The listed procedures are all implemented in SPSS, with PCA included as a further extraction procedure (see Fig. 7.21 ).
Note that PCA, in contrast, requires a collective term for correlating variables. Cf. the presentation on the problem of the interpretation of factors in Sect. 7.2.3 and the notes on the case study in Sect. 7.3.3.4 .
In the case study, the same data set is used as for discriminant analysis (Chap. 4 ), logistic regression (Chap. 5 ) and cluster analysis (Chap. 8 8). This is to better illustrate the similarities and differences between the different variants of the method.
Missing values are a frequent and unfortunately unavoidable problem when conducting surveys (e.g. because people cannot or do not want to answer some of the questions, or as a result of mistakes by the interviewer). The handling of missing values in empirical studies is discussed in Sect. 1.5.2 .
In the following the same data set is used as in the case study of discriminant analysis (Chap. 4 ), logistic regression (Chap. 5 ) and cluster analysis (Chap. 8 ). Thus, it is easier to demonstrate the similarities and differences between the methods.
By reducing the number of variables to 9, the number of valid cases in the case study changes to 117.
Cluster analysis is the central methodological instrument for identifying similarly perceived objects. The cluster analysis presented in this book (cf. Chap. 8 ) is also based on the data set used in this case study (Table 7.24 ) and confirms the result of a two-cluster solution that is emerging here.
See the general comments in Sect. 7.2.2.4 .
Child, D. (2006). The essentials of factor analysis (3rd ed.). Bloomsbury Academic.
Google Scholar
Cureton, E. E., & D’Agostino, R. B. (1993). Factor analysis: An applied approach. Erlbaum.
Dziuban, C. D. & Shirkey, E. C. (1974). When is a correlation matrix appropriate for factor analysis? Some decision rules. Psychological bulletin, 81 (6), 358.
Guttman, L. (1953). Image theory for the structure of quantitative variates. Psychometrika, 18 (4), 277–296.
Article Google Scholar
Harrington, D. (2009). Confirmatory factor analysis. Oxford University Press.
Kaiser, H. F., & Rice, J. (1974). Little jiffy, mark IV. Educational and psychological measurement, 34 (1), 111–117.
Loehlin, J. (2004). Latent variable models: An introduction to factor, path, and structural equation analysis (4th ed.). Psychology Press.
Book Google Scholar
Further Reading
Bartholomew, D. J., Knott, M., & Moustaki, I. (2011). Latent variable models and factor analysis: A unified approach (Vol. 904). Wiley.
Costello, A. B., & Osborne, J. W. (2005). Best practices in exploratory factor analysis: Four recommendations for getting the most from your analysis. Practical Assessment, Research and Evaluation, 10 (7), 1–9.
Harman, H. (1976). Modern factor analysis (3rd ed.). The University of Chicago Press.
Kaiser, H. F. (1970). A second generation little jiffy. Psychometrika, 35 (4), 401–415.
Kim, J. O., & Mueller, J. (1978). Introduction to factor analysis: What it is and how to do it. SAGE.
Stewart, D. (1981). The application and misapplication of factor analysis. Journal of Marketing Research, 18 (1), 51–62.
Tabachnick, B. G., & Fidell, L. S. (2007). Using multivariate statistics (5th ed.). Allyn & Bacon.
Thompson, B. (2004). Exploratory and confirmatory factor analysis – Understanding concepts and applications. American Psychological Association.
Yong, A. G., & Pearce, S. (2013). A beginner’s guide to factor analysis: Focusing on exploratory factor analysis. Tutorials in Quantitative Methods for Psychology, 9 (2), 79–94.
Download references
Author information
Authors and affiliations.
University of Münster, Münster, Nordrhein-Westfalen, Germany
Klaus Backhaus
Otto-von-Guericke-University Magdeburg, Magdeburg, Sachsen-Anhalt, Germany
Bernd Erichson
Sonja Gensler
University of Trier, Trier, Rheinland-Pfalz, Germany
Rolf Weiber
Munich, Bayern, Germany
Thomas Weiber
You can also search for this author in PubMed Google Scholar
Corresponding author
Correspondence to Rolf Weiber .
Rights and permissions
Reprints and permissions
Copyright information
© 2023 Springer Fachmedien Wiesbaden GmbH, part of Springer Nature
About this chapter
Cite this chapter.
Backhaus, K., Erichson, B., Gensler, S., Weiber, R., Weiber, T. (2023). Factor Analysis. In: Multivariate Analysis. Springer Gabler, Wiesbaden. https://doi.org/10.1007/978-3-658-40411-6_7
Download citation
DOI : https://doi.org/10.1007/978-3-658-40411-6_7
Published : 29 June 2023
Publisher Name : Springer Gabler, Wiesbaden
Print ISBN : 978-3-658-40410-9
Online ISBN : 978-3-658-40411-6
eBook Packages : Business and Economics (German Language)
Share this chapter
Anyone you share the following link with will be able to read this content:
Sorry, a shareable link is not currently available for this article.
Provided by the Springer Nature SharedIt content-sharing initiative
- Publish with us
Policies and ethics
- Find a journal
- Track your research
Root out friction in every digital experience, super-charge conversion rates, and optimise digital self-service
Uncover insights from any interaction, deliver AI-powered agent coaching, and reduce cost to serve
Increase revenue and loyalty with real-time insights and recommendations delivered straight to teams on the ground
Know exactly how your people feel and empower managers to improve employee engagement, productivity, and retention
Take action in the moments that matter most along the employee journey and drive bottom line growth
Whatever they’re are saying, wherever they’re saying it, know exactly what’s going on with your people
Get faster, richer insights with qual and quant tools that make powerful market research available to everyone
Run concept tests, pricing studies, prototyping + more with fast, powerful studies designed by UX research experts
Track your brand performance 24/7 and act quickly to respond to opportunities and challenges in your market
Meet the operating system for experience management
- Free Account
- For Digital
- For Customer Care
- For Human Resources
- For Researchers
- Financial Services
- All Industries
Popular Use Cases
- Customer Experience
- Employee Experience
- Employee Exit Interviews
- Net Promoter Score
- Voice of Customer
- Customer Success Hub
- Product Documentation
- Training & Certification
- XM Institute
- Popular Resources
- Customer Stories
- Market Research
- Artificial Intelligence
- Partnerships
- Marketplace
The annual gathering of the experience leaders at the world’s iconic brands building breakthrough business results.
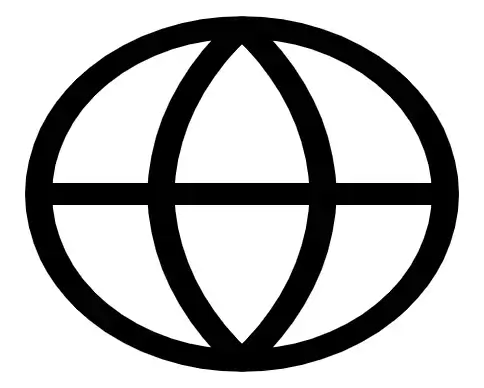
- English/AU & NZ
- Español/Europa
- Español/América Latina
- Português Brasileiro
- REQUEST DEMO
- Experience Management
- Survey Analysis
- Factor Analysis
Try Qualtrics for free
What is factor analysis and how does it simplify research findings.
17 min read There are many forms of data analysis used to report on and study survey data. Factor analysis is best when used to simplify complex data sets with many variables.
What is factor analysis?
Factor analysis is the practice of condensing many variables into just a few, so that your research data is easier to work with.
For example, a retail business trying to understand customer buying behaviours might consider variables such as ‘did the product meet your expectations?’, ‘how would you rate the value for money?’ and ‘did you find the product easily?’. Factor analysis can help condense these variables into a single factor, such as ‘customer purchase satisfaction’.
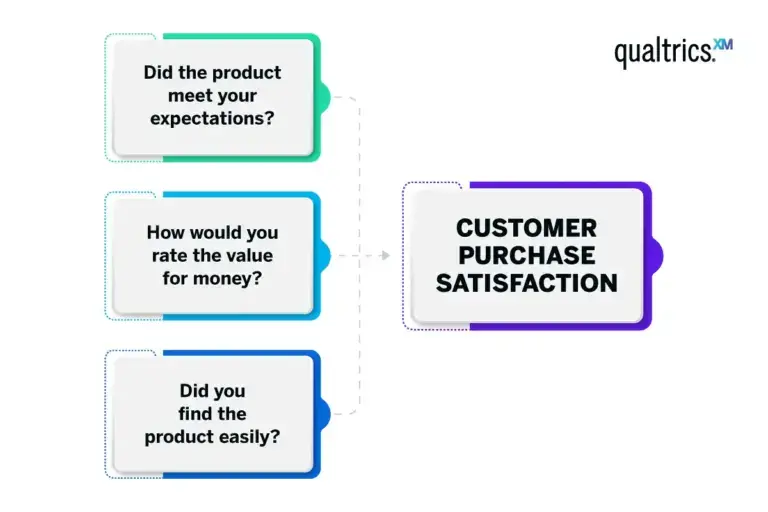
The theory is that there are deeper factors driving the underlying concepts in your data, and that you can uncover and work with them instead of dealing with the lower-level variables that cascade from them. Know that these deeper concepts aren’t necessarily immediately obvious – they might represent traits or tendencies that are hard to measure, such as extraversion or IQ.
Factor analysis is also sometimes called “dimension reduction”: you can reduce the “dimensions” of your data into one or more “super-variables,” also known as unobserved variables or latent variables. This process involves creating a factor model and often yields a factor matrix that organises the relationship between observed variables and the factors they’re associated with.
As with any kind of process that simplifies complexity, there is a trade-off between the accuracy of the data and how easy it is to work with. With factor analysis, the best solution is the one that yields a simplification that represents the true nature of your data, with minimum loss of precision. This often means finding a balance between achieving the variance explained by the model and using fewer factors to keep the model simple.
Factor analysis isn’t a single technique, but a family of statistical methods that can be used to identify the latent factors driving observable variables. Factor analysis is commonly used in market research , as well as other disciplines like technology, medicine, sociology, field biology, education, psychology and many more.
What is a factor?
In the context of factor analysis, a factor is a hidden or underlying variable that we infer from a set of directly measurable variables.
Take ‘customer purchase satisfaction’ as an example again. This isn’t a variable you can directly ask a customer to rate, but it can be determined from the responses to correlated questions like ‘did the product meet your expectations?’, ‘how would you rate the value for money?’ and ‘did you find the product easily?’.
While not directly observable, factors are essential for providing a clearer, more streamlined understanding of data. They enable us to capture the essence of our data’s complexity, making it simpler and more manageable to work with, and without losing lots of information.
Free eBook: 2024 global market research trends report
Key concepts in factor analysis
These concepts are the foundational pillars that guide the application and interpretation of factor analysis.
Central to factor analysis, variance measures how much numerical values differ from the average. In factor analysis, you’re essentially trying to understand how underlying factors influence this variance among your variables. Some factors will explain more variance than others, meaning they more accurately represent the variables they consist of.
The eigenvalue expresses the amount of variance a factor explains. If a factor solution (unobserved or latent variables) has an eigenvalue of 1 or above, it indicates that a factor explains more variance than a single observed variable, which can be useful in reducing the number of variables in your analysis. Factors with eigenvalues less than 1 account for less variability than a single variable and are generally not included in the analysis.
Factor score
A factor score is a numeric representation that tells us how strongly each variable from the original data is related to a specific factor. Also called the component score, it can help determine which variables are most influenced by each factor and are most important for each underlying concept.
Factor loading
Factor loading is the correlation coefficient for the variable and factor. Like the factor score, factor loadings give an indication of how much of the variance in an observed variable can be explained by the factor. High factor loadings (close to 1 or -1) mean the factor strongly influences the variable.
When to use factor analysis
Factor analysis is a powerful tool when you want to simplify complex data, find hidden patterns, and set the stage for deeper, more focused analysis.
It’s typically used when you’re dealing with a large number of interconnected variables, and you want to understand the underlying structure or patterns within this data. It’s particularly useful when you suspect that these observed variables could be influenced by some hidden factors.
For example, consider a business that has collected extensive customer feedback through surveys . The survey covers a wide range of questions about product quality, pricing, customer service and more. This huge volume of data can be overwhelming, and this is where factor analysis comes in. It can help condense these numerous variables into a few meaningful factors, such as ‘product satisfaction’, ‘customer service experience’ and ‘value for money’.
Factor analysis doesn’t operate in isolation – it’s often used as a stepping stone for further analysis. For example, once you’ve identified key factors through factor analysis, you might then proceed to a cluster analysis – a method that groups your customers based on their responses to these factors. The result is a clearer understanding of different customer segments, which can then guide targeted marketing and product development strategies.
By combining factor analysis with other methodologies, you can not only make sense of your data but also gain valuable insights to drive your business decisions.
Factor analysis assumptions
Factor analysis relies on several assumptions for accurate results. Violating these assumptions may lead to factors that are hard to interpret or misleading.
Linear relationships between variables
This ensures that changes in the values of your variables are consistent.
Sufficient variables for each factor
Because if only a few variables represent a factor, it might not be identified accurately.
Adequate sample size
The larger the ratio of cases (respondents, for instance) to variables, the more reliable the analysis.
No perfect multicollinearity and singularity
No variable is a perfect linear combination of other variables, and no variable is a duplicate of another.
Relevance of the variables
There should be some correlation between variables to make a factor analysis feasible.
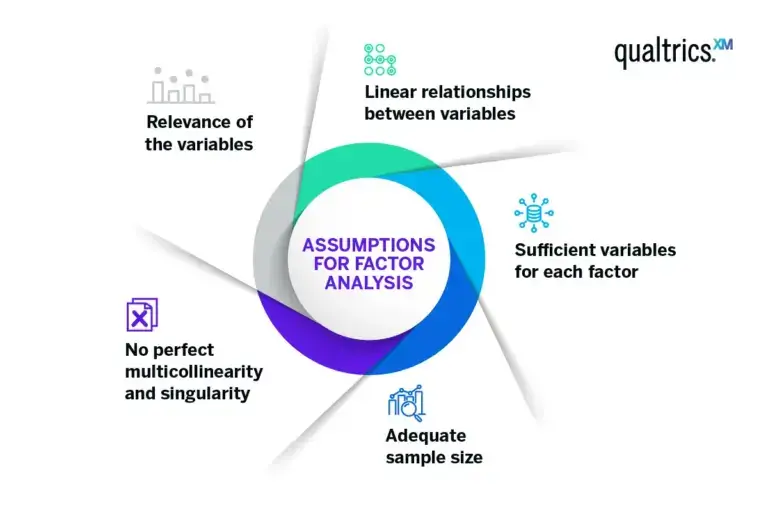
Types of factor analysis
There are two main factor analysis methods: exploratory and confirmatory. Here’s how they are used to add value to your research process.
Confirmatory factor analysis
In this type of analysis, the researcher starts out with a hypothesis about their data that they are looking to prove or disprove. Factor analysis will confirm – or not – where the latent variables are and how much variance they account for.
Principal component analysis (PCA) is a popular form of confirmatory factor analysis. Using this method, the researcher will run the analysis to obtain multiple possible solutions that split their data among a number of factors. Items that load onto a single particular factor are more strongly related to one another and can be grouped together by the researcher using their conceptual knowledge or pre-existing research.
Using PCA will generate a range of solutions with different numbers of factors, from simplified 1-factor solutions to higher levels of complexity. However, the fewer number of factors employed, the less variance will be accounted for in the solution.
Exploratory factor analysis
As the name suggests, exploratory factor analysis is undertaken without a hypothesis in mind. It’s an investigatory process that helps researchers understand whether associations exist between the initial variables, and if so, where they lie and how they are grouped.
How to perform factor analysis: A step-by-step guide
Performing a factor analysis involves a series of steps, often facilitated by statistical software packages like SPSS, Stata and the R programming language . Here’s a simplified overview of the process.
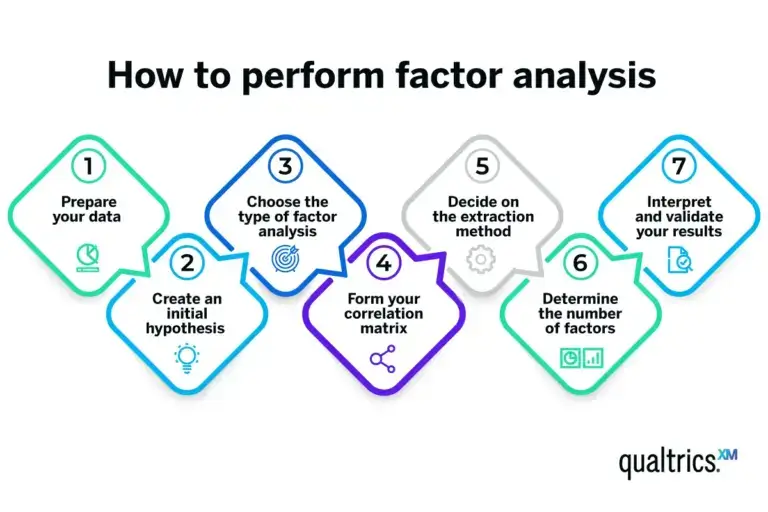
Prepare your data
Start with a dataset where each row represents a case (for example, a survey respondent), and each column is a variable you’re interested in. Ensure your data meets the assumptions necessary for factor analysis.
Create an initial hypothesis
If you have a theory about the underlying factors and their relationships with your variables, make a note of this. This hypothesis can guide your analysis, but keep in mind that the beauty of factor analysis is its ability to uncover unexpected relationships.
Choose the type of factor analysis
The most common type is exploratory factor analysis, which is used when you’re not sure what to expect. If you have a specific hypothesis about the factors, you might use confirmatory factor analysis.
Form your correlation matrix
After you’ve chosen the type of factor analysis, you’ll need to create the correlation matrix of your variables. This matrix, which shows the correlation coefficients between each pair of variables, forms the basis for the extraction of factors. This is a key step in building your factor analysis model.
Decide on the extraction method
Principal component analysis is the most commonly used extraction method. If you believe your factors are correlated, you might opt for principal axis factoring, a type of factor analysis that identifies factors based on shared variance.
Determine the number of factors
Various criteria can be used here, such as Kaiser’s criterion (eigenvalues greater than 1), the scree plot method or parallel analysis. The choice depends on your data and your goals.
Interpret and validate your results
Each factor will be associated with a set of your original variables, so label each factor based on how you interpret these associations. These labels should represent the underlying concept that ties the associated variables together.
Validation can be done through a variety of methods, like splitting your data in half and checking if both halves produce the same factors.
How factor analysis can help you
As well as giving you fewer variables to navigate, factor analysis can help you understand grouping and clustering in your input variables, since they’ll be grouped according to the latent variables.
Say you ask several questions all designed to explore different, but closely related, aspects of customer satisfaction:
- How satisfied are you with our product?
- Would you recommend our product to a friend or family member?
- How likely are you to purchase our product in the future?
But you only want one variable to represent a customer satisfaction score. One option would be to average the three question responses. Another option would be to create a factor dependent variable. This can be done by running a principal component analysis (PCA) and keeping the first principal component (also known as a factor). The advantage of a PCA over an average is that it automatically weights each of the variables in the calculation.
Say you have a list of questions and you don’t know exactly which responses will move together and which will move differently; for example, purchase barriers of potential customers. The following are possible barriers to purchase:
- Price is prohibitive
- Overall implementation costs
- We can’t reach a consensus in our organisation
- Product is not consistent with our business strategy
- I need to develop an ROI, but cannot or have not
- We are locked into a contract with another product
- The product benefits don’t outweigh the cost
- We have no reason to switch
- Our IT department cannot support your product
- We do not have sufficient technical resources
- Your product does not have a feature we require
- Other (please specify)
Factor analysis can uncover the trends of how these questions will move together. The following are loadings for 3 factors for each of the variables.
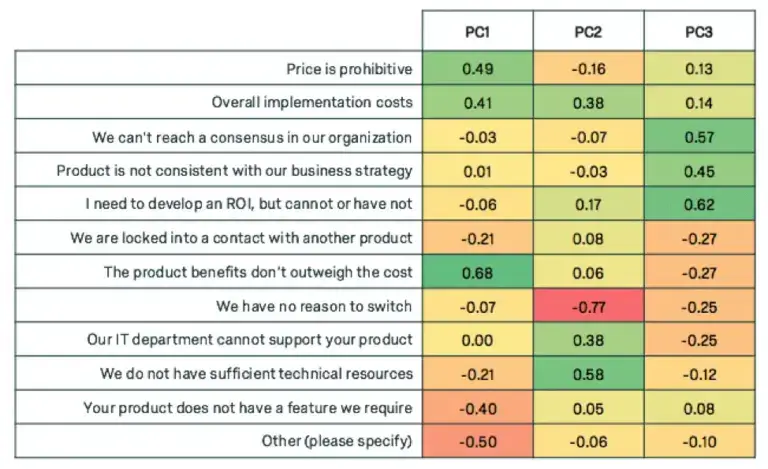
Notice how each of the principal components have high weights for a subset of the variables. Weight is used interchangeably with loading, and high weight indicates the variables that are most influential for each principal component. +0.30 is generally considered to be a heavy weight.
The first component displays heavy weights for variables related to cost, the second weights variables related to IT, and the third weights variables related to organisational factors. We can give our new super variables clever names.
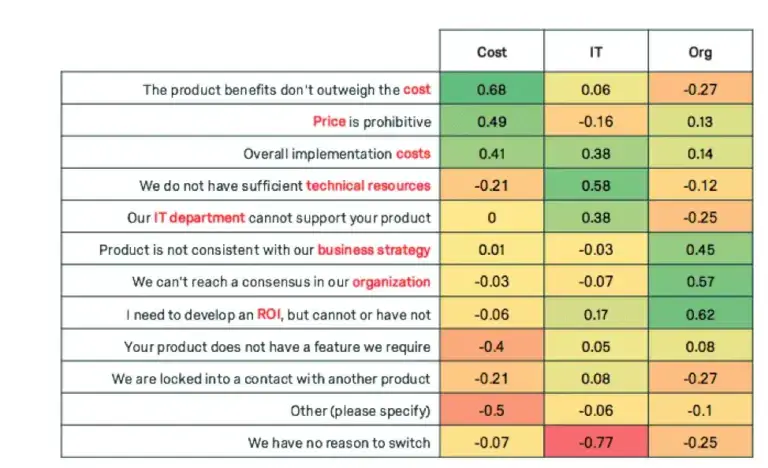
If we were to cluster the customers based on these three components, we can see some trends. Customers tend to be high in cost barriers or organisational barriers, but not both.
The red dots represent respondents who indicated they had higher organisational barriers; the green dots represent respondents who indicated they had higher cost barriers
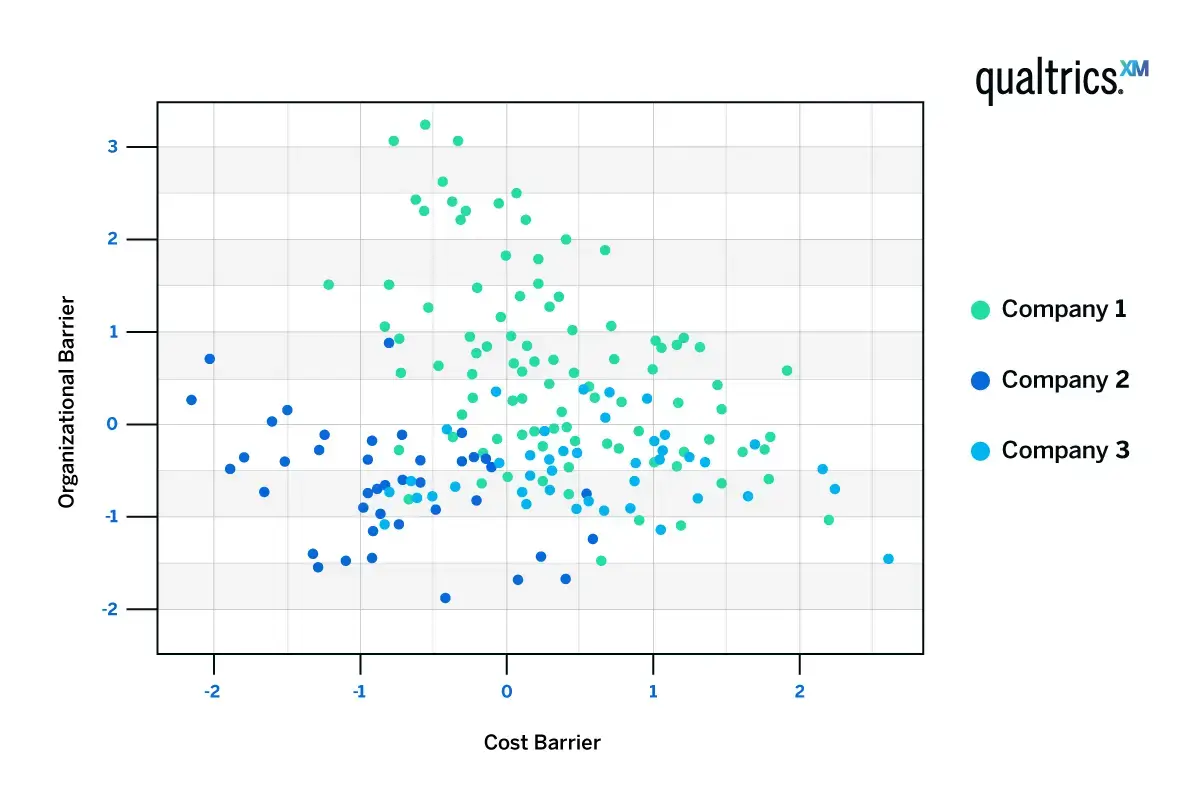
Considerations when using factor analysis
Factor analysis is a tool, and like any tool its effectiveness depends on how you use it. When employing factor analysis, it’s essential to keep a few key considerations in mind.

Oversimplification
While factor analysis is great for simplifying complex data sets, there’s a risk of oversimplification when grouping variables into factors. To avoid this you should ensure the reduced factors still accurately represent the complexities of your variables.
Subjectivity
Interpreting the factors can sometimes be subjective, and requires a good understanding of the variables and the context. Be mindful that multiple analysts may come up with different names for the same factor.
Supplementary techniques
Factor analysis is often just the first step. Consider how it fits into your broader research strategy and which other techniques you’ll use alongside it.
Examples of factor analysis studies
Factor analysis, including PCA, is often used in tandem with segmentation studies . It might be an intermediary step to reduce variables before using KMeans to make the segments.
Factor analysis provides simplicity after reducing variables. For long studies with large blocks of Matrix Likert scale questions, the number of variables can become unwieldy. Simplifying the data using factor analysis helps analysts focus and clarify the results, while also reducing the number of dimensions they’re clustering on.
Sample questions for factor analysis
Choosing exactly which questions to perform factor analysis on is both an art and a science. Choosing which variables to reduce takes some experimentation, patience and creativity. Factor analysis works well on Likert scale questions and Sum to 100 question types.
Factor analysis works well on matrix blocks of the following question genres:
Psychographics (Agree/Disagree):
- I value family
- I believe brand represents value
Behavioural (Agree/Disagree):
- I purchase the cheapest option
- I am a bargain shopper
Attitudinal (Agree/Disagree):
- The economy is not improving
- I am pleased with the product
Activity-Based (Agree/Disagree):
- I love sports
- I sometimes shop online during work hours
Behavioural and psychographic questions are especially suited for factor analysis.
Sample output reports
Factor analysis simply produces weights (called loadings) for each respondent. These loadings can be used like other responses in the survey.
Related resources
Analysis & Reporting
Margin of Error 11 min read
Text analysis 44 min read, sentiment analysis 21 min read, behavioural analytics 12 min read, descriptive statistics 15 min read, statistical significance calculator 18 min read, zero-party data 12 min read, request demo.
Ready to learn more about Qualtrics?
Factor Analysis in Marketing Research
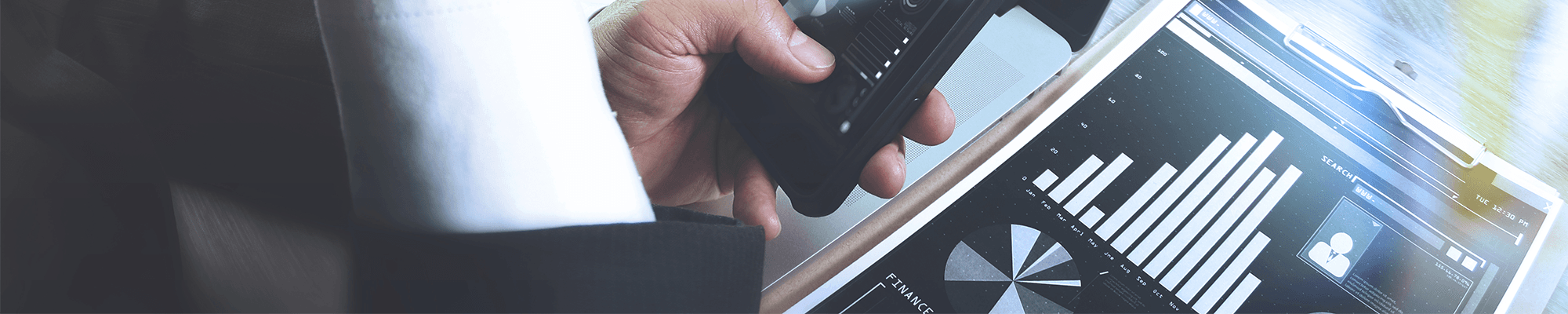
- Home > What We Do > Research Methods > Statistical Techniques > Factor Analysis in Marketing Research
Factor analysis in marketing research aims to describe a large number of variables or questions by using a reduced set of underlying variables, called factors.
Contact Us >
To find out more about factor analysis and how it can help your business
What is factor analysis in marketing research?
Factor analysis explains a pattern of similarity between observed variables. Questions which belong to one factor are highly correlated with each other; unlike cluster analysis, which classifies respondents, factor analysis groups variables.
There are two types of factor analysis in marketing research: exploratory and confirmatory. Exploratory factor analysis is driven by the data, i.e. the data determines the factors. Confirmatory factor analysis, used in structural equation modelling, tests and confirms hypotheses.
Factor analysis in market research is often used in customer satisfaction studies to identify underlying service dimensions, and in profiling studies to determine core attitudes. For example, as part of a national survey on political opinions, respondents may answer three separate questions regarding environmental policy, reflecting issues at the local, regional and national level. Factor analysis can be used to establish whether the three measures do, in fact, measure the same thing.
It can also prove to be useful when a lengthy questionnaire needs to be shortened, but still retain key questions. Factor analysis indicates which questions can be omitted without losing too much information.
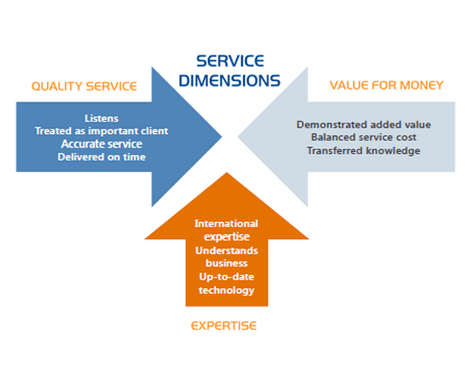
Contact Us >
Privacy Overview
- Privacy Policy
Buy Me a Coffee

Home » Factor Analysis – Steps, Methods and Examples
Factor Analysis – Steps, Methods and Examples
Table of Contents

Factor Analysis
Definition:
Factor analysis is a statistical technique that is used to identify the underlying structure of a relatively large set of variables and to explain these variables in terms of a smaller number of common underlying factors. It helps to investigate the latent relationships between observed variables.
Factor Analysis Steps
Here are the general steps involved in conducting a factor analysis:
1. Define the Research Objective:
Clearly specify the purpose of the factor analysis. Determine what you aim to achieve or understand through the analysis.
2. Data Collection:
Gather the data on the variables of interest. These variables should be measurable and related to the research objective. Ensure that you have a sufficient sample size for reliable results.
3. Assess Data Suitability:
Examine the suitability of the data for factor analysis. Check for the following aspects:
- Sample size: Ensure that you have an adequate sample size to perform factor analysis reliably.
- Missing values: Handle missing data appropriately, either by imputation or exclusion.
- Variable characteristics: Verify that the variables are continuous or at least ordinal in nature. Categorical variables may require different analysis techniques.
- Linearity: Assess whether the relationships among variables are linear.
4. Determine the Factor Analysis Technique:
There are different types of factor analysis techniques available, such as exploratory factor analysis (EFA) and confirmatory factor analysis (CFA). Choose the appropriate technique based on your research objective and the nature of the data.
5. Perform Factor Analysis:
a. Exploratory Factor Analysis (EFA):
- Extract factors: Use factor extraction methods (e.g., principal component analysis or common factor analysis) to identify the initial set of factors.
- Determine the number of factors: Decide on the number of factors to retain based on statistical criteria (e.g., eigenvalues, scree plot) and theoretical considerations.
- Rotate factors: Apply factor rotation techniques (e.g., varimax, oblique) to simplify the factor structure and make it more interpretable.
- Interpret factors: Analyze the factor loadings (correlations between variables and factors) to interpret the meaning of each factor.
- Determine factor reliability: Assess the internal consistency or reliability of the factors using measures like Cronbach’s alpha.
- Report results: Document the factor loadings, rotated component matrix, communalities, and any other relevant information.
b. Confirmatory Factor Analysis (CFA):
- Formulate a theoretical model: Specify the hypothesized relationships among variables and factors based on prior knowledge or theoretical considerations.
- Define measurement model: Establish how each variable is related to the underlying factors by assigning factor loadings in the model.
- Test the model: Use statistical techniques like maximum likelihood estimation or structural equation modeling to assess the goodness-of-fit between the observed data and the hypothesized model.
- Modify the model: If the initial model does not fit the data adequately, revise the model by adding or removing paths, allowing for correlated errors, or other modifications to improve model fit.
- Report results: Present the final measurement model, parameter estimates, fit indices (e.g., chi-square, RMSEA, CFI), and any modifications made.
6. Interpret and Validate the Factors:
Once you have identified the factors, interpret them based on the factor loadings, theoretical understanding, and research objectives. Validate the factors by examining their relationships with external criteria or by conducting further analyses if necessary.
Types of Factor Analysis
Types of Factor Analysis are as follows:
Exploratory Factor Analysis (EFA)
EFA is used to explore the underlying structure of a set of observed variables without any preconceived assumptions about the number or nature of the factors. It aims to discover the number of factors and how the observed variables are related to those factors. EFA does not impose any restrictions on the factor structure and allows for cross-loadings of variables on multiple factors.
Confirmatory Factor Analysis (CFA)
CFA is used to test a pre-specified factor structure based on theoretical or conceptual assumptions. It aims to confirm whether the observed variables measure the latent factors as intended. CFA tests the fit of a hypothesized model and assesses how well the observed variables are associated with the expected factors. It is often used for validating measurement instruments or evaluating theoretical models.
Principal Component Analysis (PCA)
PCA is a dimensionality reduction technique that can be considered a form of factor analysis, although it has some differences. PCA aims to explain the maximum amount of variance in the observed variables using a smaller number of uncorrelated components. Unlike traditional factor analysis, PCA does not assume that the observed variables are caused by underlying factors but focuses solely on accounting for variance.
Common Factor Analysis
It assumes that the observed variables are influenced by common factors and unique factors (specific to each variable). It attempts to estimate the common factor structure by extracting the shared variance among the variables while also considering the unique variance of each variable.
Hierarchical Factor Analysis
Hierarchical factor analysis involves multiple levels of factors. It explores both higher-order and lower-order factors, aiming to capture the complex relationships among variables. Higher-order factors are based on the relationships among lower-order factors, which are in turn based on the relationships among observed variables.
Factor Analysis Formulas
Factor Analysis is a statistical method used to describe variability among observed, correlated variables in terms of a potentially lower number of unobserved variables called factors.
Here are some of the essential formulas and calculations used in factor analysis:
Correlation Matrix :
The first step in factor analysis is to create a correlation matrix, which calculates the correlation coefficients between pairs of variables.
Correlation coefficient (Pearson’s r) between variables X and Y is calculated as:
r(X,Y) = Σ[(xi – x̄)(yi – ȳ)] / [n-1] σx σy
where: xi, yi are the data points, x̄, ȳ are the means of X and Y respectively, σx, σy are the standard deviations of X and Y respectively, n is the number of data points.
Extraction of Factors :
The extraction of factors from the correlation matrix is typically done by methods such as Principal Component Analysis (PCA) or other similar methods.
The formula used in PCA to calculate the principal components (factors) involves finding the eigenvalues and eigenvectors of the correlation matrix.
Let’s denote the correlation matrix as R. If λ is an eigenvalue of R, and v is the corresponding eigenvector, they satisfy the equation: Rv = λv
Factor Loadings :
Factor loadings are the correlations between the original variables and the factors. They can be calculated as the eigenvectors normalized by the square roots of their corresponding eigenvalues.
Communality and Specific Variance :
Communality of a variable is the proportion of variance in that variable explained by the factors. It can be calculated as the sum of squared factor loadings for that variable across all factors.
The specific variance of a variable is the proportion of variance in that variable not explained by the factors, and it’s calculated as 1 – Communality.
Factor Rotation : Factor rotation, such as Varimax or Promax, is used to make the output more interpretable. It doesn’t change the underlying relationships but affects the loadings of the variables on the factors.
For example, in the Varimax rotation, the objective is to minimize the variance of the squared loadings of a factor (column) on all the variables (rows) in a factor matrix, which leads to more high and low loadings, making the factor easier to interpret.
Examples of Factor Analysis
Here are some real-time examples of factor analysis:
- Psychological Research: In a study examining personality traits, researchers may use factor analysis to identify the underlying dimensions of personality by analyzing responses to various questionnaires or surveys. Factors such as extroversion, neuroticism, and conscientiousness can be derived from the analysis.
- Market Research: In marketing, factor analysis can be used to understand consumers’ preferences and behaviors. For instance, by analyzing survey data related to product features, pricing, and brand perception, researchers can identify factors such as price sensitivity, brand loyalty, and product quality that influence consumer decision-making.
- Finance and Economics: Factor analysis is widely used in portfolio management and asset pricing models. By analyzing historical market data, factors such as market returns, interest rates, inflation rates, and other economic indicators can be identified. These factors help in understanding and predicting investment returns and risk.
- Social Sciences: Factor analysis is employed in social sciences to explore underlying constructs in complex datasets. For example, in education research, factor analysis can be used to identify dimensions such as academic achievement, socio-economic status, and parental involvement that contribute to student success.
- Health Sciences: In medical research, factor analysis can be utilized to identify underlying factors related to health conditions, symptom clusters, or treatment outcomes. For instance, in a study on mental health, factor analysis can be used to identify underlying factors contributing to depression, anxiety, and stress.
- Customer Satisfaction Surveys: Factor analysis can help businesses understand the key drivers of customer satisfaction. By analyzing survey responses related to various aspects of product or service experience, factors such as product quality, customer service, and pricing can be identified, enabling businesses to focus on areas that impact customer satisfaction the most.
Factor analysis in Research Example
Here’s an example of how factor analysis might be used in research:
Let’s say a psychologist is interested in the factors that contribute to overall wellbeing. They conduct a survey with 1000 participants, asking them to respond to 50 different questions relating to various aspects of their lives, including social relationships, physical health, mental health, job satisfaction, financial security, personal growth, and leisure activities.
Given the broad scope of these questions, the psychologist decides to use factor analysis to identify underlying factors that could explain the correlations among responses.
After conducting the factor analysis, the psychologist finds that the responses can be grouped into five factors:
- Physical Wellbeing : Includes variables related to physical health, exercise, and diet.
- Mental Wellbeing : Includes variables related to mental health, stress levels, and emotional balance.
- Social Wellbeing : Includes variables related to social relationships, community involvement, and support from friends and family.
- Professional Wellbeing : Includes variables related to job satisfaction, work-life balance, and career development.
- Financial Wellbeing : Includes variables related to financial security, savings, and income.
By reducing the 50 individual questions to five underlying factors, the psychologist can more effectively analyze the data and draw conclusions about the major aspects of life that contribute to overall wellbeing.
In this way, factor analysis helps researchers understand complex relationships among many variables by grouping them into a smaller number of factors, simplifying the data analysis process, and facilitating the identification of patterns or structures within the data.
When to Use Factor Analysis
Here are some circumstances in which you might want to use factor analysis:
- Data Reduction : If you have a large set of variables, you can use factor analysis to reduce them to a smaller set of factors. This helps in simplifying the data and making it easier to analyze.
- Identification of Underlying Structures : Factor analysis can be used to identify underlying structures in a dataset that are not immediately apparent. This can help you understand complex relationships between variables.
- Validation of Constructs : Factor analysis can be used to confirm whether a scale or measure truly reflects the construct it’s meant to measure. If all the items in a scale load highly on a single factor, that supports the construct validity of the scale.
- Generating Hypotheses : By revealing the underlying structure of your variables, factor analysis can help to generate hypotheses for future research.
- Survey Analysis : If you have a survey with many questions, factor analysis can help determine if there are underlying factors that explain response patterns.
Applications of Factor Analysis
Factor Analysis has a wide range of applications across various fields. Here are some of them:
- Psychology : It’s often used in psychology to identify the underlying factors that explain different patterns of correlations among mental abilities. For instance, factor analysis has been used to identify personality traits (like the Big Five personality traits), intelligence structures (like Spearman’s g), or to validate the constructs of different psychological tests.
- Market Research : In this field, factor analysis is used to identify the factors that influence purchasing behavior. By understanding these factors, businesses can tailor their products and marketing strategies to meet the needs of different customer groups.
- Healthcare : In healthcare, factor analysis is used in a similar way to psychology, identifying underlying factors that might influence health outcomes. For instance, it could be used to identify lifestyle or behavioral factors that influence the risk of developing certain diseases.
- Sociology : Sociologists use factor analysis to understand the structure of attitudes, beliefs, and behaviors in populations. For example, factor analysis might be used to understand the factors that contribute to social inequality.
- Finance and Economics : In finance, factor analysis is used to identify the factors that drive financial markets or economic behavior. For instance, factor analysis can help understand the factors that influence stock prices or economic growth.
- Education : In education, factor analysis is used to identify the factors that influence academic performance or attitudes towards learning. This could help in developing more effective teaching strategies.
- Survey Analysis : Factor analysis is often used in survey research to reduce the number of items or to identify the underlying structure of the data.
- Environment : In environmental studies, factor analysis can be used to identify the major sources of environmental pollution by analyzing the data on pollutants.
Advantages of Factor Analysis
Advantages of Factor Analysis are as follows:
- Data Reduction : Factor analysis can simplify a large dataset by reducing the number of variables. This helps make the data easier to manage and analyze.
- Structure Identification : It can identify underlying structures or patterns in a dataset that are not immediately apparent. This can provide insights into complex relationships between variables.
- Construct Validation : Factor analysis can be used to validate whether a scale or measure accurately reflects the construct it’s intended to measure. This is important for ensuring the reliability and validity of measurement tools.
- Hypothesis Generation : By revealing the underlying structure of your variables, factor analysis can help generate hypotheses for future research.
- Versatility : Factor analysis can be used in various fields, including psychology, market research, healthcare, sociology, finance, education, and environmental studies.
Disadvantages of Factor Analysis
Disadvantages of Factor Analysis are as follows:
- Subjectivity : The interpretation of the factors can sometimes be subjective, depending on how the data is perceived. Different researchers might interpret the factors differently, which can lead to different conclusions.
- Assumptions : Factor analysis assumes that there’s some underlying structure in the dataset and that all variables are related. If these assumptions do not hold, factor analysis might not be the best tool for your analysis.
- Large Sample Size Required : Factor analysis generally requires a large sample size to produce reliable results. This can be a limitation in studies where data collection is challenging or expensive.
- Correlation, not Causation : Factor analysis identifies correlational relationships, not causal ones. It cannot prove that changes in one variable cause changes in another.
- Complexity : The statistical concepts behind factor analysis can be difficult to understand and require expertise to implement correctly. Misuse or misunderstanding of the method can lead to incorrect conclusions.
About the author
Muhammad Hassan
Researcher, Academic Writer, Web developer
You may also like

Cluster Analysis – Types, Methods and Examples

Discriminant Analysis – Methods, Types and...

MANOVA (Multivariate Analysis of Variance) –...

Documentary Analysis – Methods, Applications and...

ANOVA (Analysis of variance) – Formulas, Types...

Graphical Methods – Types, Examples and Guide

IMAGES
VIDEO
COMMENTS
4.1 Communalities matrix. 1 PCA is an approach that can be used to extract the number of underlying factors (Pallant, 2013). 2 Factor analysis (FA) an analysis which already assumed that the ...
the underlying theory or methodology of factor analy-sis. This rather blind use of factor analysis is a principal cause of the dissatisfaction with the technique. So widespread are current misconceptions about factor analysis in the marketing community that even its defenders and some prominent reviewers perpet-uate misinformation.
COMPAP.ISONOFIT:,-ROTATI:oandROTATf-DFACTORSCHITION'S Loadin^son factor unrotated LoadingGon factors rotated A B C h^ A B C h^ 68 -52 31 82 90 08 -05 82 69 -51 26 80 89 07 -01 80 63 -32 16 53 69 15 -17 53 58 53 27 69 14 81 -08 69 38 59 29 57 -02 • 76 03 57 63 44 16 62 20 73 -20 62 33-04 -77 70-02 -13 -83 70 65.-04 -52 69 28 13 -77 69 62 22 ...
Why Factor Analysis? 1. Testing of theory ! Explain covariation among multiple observed variables by ! Mapping variables to latent constructs (called "factors") 2. Understanding the structure underlying a set of measures ! Gain insight to dimensions ! Construct validation (e.g., convergent validity)
Factor analysis isn't a single technique, but a family of statistical methods that can be used to identif y the latent factors driving obser vable variables. Factor analysis is commonly used in market research , as well as other disciplines like technology, medicine, sociology, field biology, education, psychology and many more.
General Steps for EFA. Load and clean the data. Put it on a common scale (e.g., standardize) and address extreme skew. Examine correlation matrix to get a sense of possible factors. Determine the number of factors. Choose a factor rotation model (more in a moment) Fit the model and interpret the resulting factors.
Published 9 January 2016. Business. Journal of Educational and Social Research. The purpose of this paper is to allow researchers, instructors, and students to comprehend the nature of exploratory factor analysis (EFA) from marketing perspective. In particular, the paper offer realistic information from marketing literature on developing valid ...
Download reference work entry PDF. Introduction. Factor analysis (in the broad, informal sense of the term) refers to a collection of techniques designed to reduce the dimensionality of the data and account for the interdependencies among a set of measured (observed, manifest) variables. ... Most measurements in marketing research are ...
The application and misapplication of factor analysis in marketing research. Journal of Marketing Research , 18 (1), 51-62. David Stewart discusses procedures for determining when data are appropriate for factor analysis, as well as guidelines for determining the number of factors to extract, and for rotation.
Factor analysis is a multivariate method that can be used for analyzing large data sets with two main goals: 1. to reduce a large number of correlating variables to a fewer number of factors,. 2. to structure the data with the aim of identifying dependencies between correlating variables and examining them for common causes (factors) in order to generate a new construct (factor) on this basis.
The use of factor analysis as a method for examining the dimensional structure of data is contrasted with its frequent misapplication as a tool for identifying clusters and segments. Procedures for determining when a data set is appropriate for factoring, for determining the number of factors to extract, and for rotation are discussed.
Accordingly, it is recommended that: Survey data should be analyzed using a series of steps that involve simple (but important) tools (e.g. missing value analyses) before more complex techniques (like confirmatory factor analysis models) are employed to purify scales (Cadogan et al., 2009; Souchon et al., 2016).
Abstract. The purpose of the paper is to present a detailed application of the factor analysis technique within the domain of relationship marketing. A comprehensive literature review is undertaken regarding the concept of customer loyalty - an important variable of relationship marketing. Loyalty is defined by its two dimensions ...
ducted principal components analysis to reduce the number of these criteria and created seven factors. To confirm factors are accurate, we executed confirmatory factor analysis that proved that model consisting of the newly created factors fits the data well. Subjects: Marketing; Advertising; Marketing Research; Consumer Behavior
Factor analysis isn't a single technique, but a family of statistical methods that can be used to identify the latent factors driving observable variables. Factor analysis is commonly used in market research, as well as other disciplines like technology, medicine, sociology, field biology, education, psychology and many more.
There are two types of factor analysis in marketing research: exploratory and confirmatory. Exploratory factor analysis is driven by the data, i.e. the data determines the factors. Confirmatory factor analysis, used in structural equation modelling, tests and confirms hypotheses. Factor analysis in market research is often used in customer ...
Market Research: In marketing, factor analysis can be used to understand consumers' preferences and behaviors. For instance, by analyzing survey data related to product features, pricing, and brand perception, researchers can identify factors such as price sensitivity, brand loyalty, and product quality that influence consumer decision-making.
Multidimensional scaling (MDS) is a series of techniques that helps the analyst to identify key dimensions underlying respondents' evaluations of objects. It is often used in Marketing to identify key dimensions underlying customer evaluations of products, services or companies. Once the data is in hand, multidimensional scaling can help ...